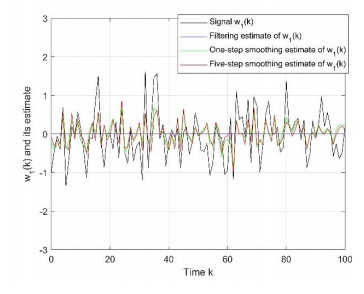
The process white noise (PWN) and observation white noise (OWN) estimation problem for linear discrete fractional order systems (LDFOS) is addressed in this study. By using the Grünwald-Letnikov (G-L) operator as a definition of the discrete fractional calculus (DFC), LDFOS is transformed into a class of linear discrete time-delay systems. However, it is different from the general time-delay system, in which the time-delay part is the cumulative sum from time 0 to the previous time. Based on the orthogonal projection theorem, a suboptimal one-step predictor of LDFOS is designed. Due to the existence of cumulative sum time-delay in system, the Riccati equation has one more cumulative sum state error variance term, which is different from the classical Kalman filter (KF). Moreover, using innovation analysis technology, the filtering and fixed-lag smoothing estimators of PWN and OWN in the form of noise orthogonal projection gain matrices are derived. Finally, two simulation examples are given to verify the effectiveness of PWN and OWN estimators.
Citation: Yantong Mu, Huihong Zhao, Zhifang Li. White noise estimation for linear discrete fractional order system[J]. AIMS Mathematics, 2022, 7(6): 10009-10023. doi: 10.3934/math.2022558
[1] | Zhifang Li, Huihong Zhao, Hailong Meng, Yong Chen . Variable step size predictor design for a class of linear discrete-time censored system. AIMS Mathematics, 2021, 6(10): 10581-10595. doi: 10.3934/math.2021614 |
[2] | Xintao Li . Wong-Zakai approximations and long term behavior of second order non-autonomous stochastic lattice dynamical systems with additive noise. AIMS Mathematics, 2022, 7(5): 7569-7594. doi: 10.3934/math.2022425 |
[3] | Zeliha Korpinar, Mustafa Inc, Ali S. Alshomrani, Dumitru Baleanu . The deterministic and stochastic solutions of the Schrodinger equation with time conformable derivative in birefrigent fibers. AIMS Mathematics, 2020, 5(3): 2326-2345. doi: 10.3934/math.2020154 |
[4] | Mohammad Sajid, Biplab Dhar, Ahmed S. Almohaimeed . Differential order analysis and sensitivity analysis of a CoVID-19 infection system with memory effect. AIMS Mathematics, 2022, 7(12): 20594-20614. doi: 10.3934/math.20221129 |
[5] | Hanan S. Gafel, Saima Rashid, Sayed K. Elagan . Novel codynamics of the HIV-1/HTLV-Ⅰ model involving humoral immune response and cellular outbreak: A new approach to probability density functions and fractional operators. AIMS Mathematics, 2023, 8(12): 28246-28279. doi: 10.3934/math.20231446 |
[6] | Jingen Yang, Zhong Zhao, Xinyu Song . Statistical property analysis for a stochastic chemostat model with degenerate diffusion. AIMS Mathematics, 2023, 8(1): 1757-1769. doi: 10.3934/math.2023090 |
[7] | Rou Lin, Min Zhao, Jinlu Zhang . Random uniform exponential attractors for non-autonomous stochastic Schrödinger lattice systems in weighted space. AIMS Mathematics, 2023, 8(2): 2871-2890. doi: 10.3934/math.2023150 |
[8] | Da Shi, Zhao Li, Dan Chen . New traveling wave solutions, phase portrait and chaotic patterns for the dispersive concatenation model with spatio-temporal dispersion having multiplicative white noise. AIMS Mathematics, 2024, 9(9): 25732-25751. doi: 10.3934/math.20241257 |
[9] | Dan Chen, Da Shi, Feng Chen . Qualitative analysis and new traveling wave solutions for the stochastic Biswas-Milovic equation. AIMS Mathematics, 2025, 10(2): 4092-4119. doi: 10.3934/math.2025190 |
[10] | Abdelkader Moumen, Khaled A. Aldwoah, Muntasir Suhail, Alwaleed Kamel, Hicham Saber, Manel Hleili, Sayed Saifullah . Investigation of more solitary waves solutions of the stochastics Benjamin-Bona-Mahony equation under beta operator. AIMS Mathematics, 2024, 9(10): 27403-27417. doi: 10.3934/math.20241331 |
The process white noise (PWN) and observation white noise (OWN) estimation problem for linear discrete fractional order systems (LDFOS) is addressed in this study. By using the Grünwald-Letnikov (G-L) operator as a definition of the discrete fractional calculus (DFC), LDFOS is transformed into a class of linear discrete time-delay systems. However, it is different from the general time-delay system, in which the time-delay part is the cumulative sum from time 0 to the previous time. Based on the orthogonal projection theorem, a suboptimal one-step predictor of LDFOS is designed. Due to the existence of cumulative sum time-delay in system, the Riccati equation has one more cumulative sum state error variance term, which is different from the classical Kalman filter (KF). Moreover, using innovation analysis technology, the filtering and fixed-lag smoothing estimators of PWN and OWN in the form of noise orthogonal projection gain matrices are derived. Finally, two simulation examples are given to verify the effectiveness of PWN and OWN estimators.
The estimation of PWN and OWN for linear or nonlinear system, also known as deconvolution problem [1], have important applications in petroleum exploration, image restoration, voice processing, state estimation and fault diagnosis [1,2,3,4,5]. Mendel proposed an input white noise estimation (WNE) method based on KF under the application background of petroleum exploration [2]. In [3], Wang and Zheng considered the influence of white noise in the modeling of networked control system, constructed the KF of the system, and calculated the filter parameters. By comparing the filter output with the actual system, the sensor and actuator faults were diagnosed by generating residual quantities. In [4], Singh et al. used the method of linear partial differential equation to model the dynamic image field driven by Gaussian white noise (GWN), that is, through the noise measurement of the above image field, the magnetic resonance image was restored to the original image. By predicting the shielded speech spectrum envelope in real time, the spectrum energy of white noise could be adjusted to generate a shielded signal similar to white noise, so as to shield the signal more accurately to prevent speech information from being eavesdropped [5]. At present, the WNE of linear discrete system has achieved rich results, but the WNE of LDFOS is rarely studied.
It is generally believed that fractional order was introduced in 1695, and its important research began in the early 21st century. From a large number of reported literature, we can see that the fractional order and its related problems have been studied in mathematics and other fields. In [6], Atici and Eloe gave two solutions for the discrete constant coefficient half-order fractional equation, and studied the existence of its initial value problem, which was a good promotion for the development of DFC. In [7], Huang and Wu et al. presented the definition of DFC of interval valued functions, which laid a solid foundation for the applications of fuzzy fractional difference equations. Wu et al. presented the fractional q-deformation mapping, and reported chaos in fractional q-deformed maps [8]. In [9], Sierociuk and Dzielinski developed the KF for linear and nonlinear fractional discrete state space system, and explained the application under what circumstances. In [10], Zarei et al. designed a unknown input filter for LDFOS, which could decouple its interference in a noisy environment. In [11], the basic estimation problems of fractional order system (FOS) were solved in the form of KF. Based on the infinite dimensional form of LDFOS, Sierociuk et al. studied the application of fractional KF application over lossy network [12]. In [13], Ram and Mohanty applied the concept of fractional calculus to the enhanced signal, and used G-L, Caputo and Riemann-Liouville methods to decompose the signal to achieve the enhanced effect. In [14], Wu et al. approximately transformed Lévy noises into GWN, and proposed an improved KF for LDFOS with non-GWN. Based on the proposed fractional order dynamic equivalent circuit model of the ultracapacitor, Wang et al. studied the application of particle filter and fractional KF hybrid algorithm in state of charge estimation [15]. FOS and fractional KF were also often used to model and estimate the state of charge of lithium-ion batteries in electric vehicles, and many results have been achieved [16,17,18]. In [19], Raïssi and Aoun established a fractional order observer in the exceptional case where the estimation error can be positive, and then changed the coordinates to extend it to the general case, so as to design a robust observer for dynamical continuous linear FOS. Wu and Luo et al. proposed a fractional order short memory method, which resulted in equations that could improve the performance of neural networks, and suggested some potential application directions [20]. In [21], Dehestani et al. studied the optimal control problem of dynamic FOS with varying orders by using the method of fractional Bessel wavelet, and proposed fractional derivative operator of variable order in the sense of Caputo type. On the basis of the stability of FOS and the properties of Kronecker product, Li obtained sufficient conditions for the robust asymptotically stable augmented system, and a state estimator was designed to estimate the state of the same complex fractional networks [22]. In [23], Abdeljawad et al. proposed a new DFC based on time scale, through which the application of image encryption technology in information security can be further studied. In [24], based on recursive least square algorithm, Idiou et al. proposed an identification algorithm for linear fractional order differential equations. As far as the authors know, although some achievements have been made in the research of observer or filter for LDFOS, the research on WNE has not been carried out.
In this paper, the state estimation methods of LDFOS are summarized, and the one-step predictor is designed by using the projection theorem. Then, based on the proposed one-step predictor of LDFOS, using innovation analysis technology, we propose the filter and fixed-lag smoother for PWN and OWN. The main innovations and characteristics of this paper are as follows: (1) The WNE for LDFOS is studied for the first time. (2) At the same time, a unified approach to recursive PWN and OWN filtering and smoothing for LDFOS is proposed.
The structure of this paper is as follows. In the second section, we introduce the system model and describe the main problems. In Section 3, we introduce the one-step predictor for LDFOS. In Section 4, we obtain filter and fixed-lag smoother for PWN and OWN of linear discrete random FOS. Finally, in Section 5, the proposed PWN, OWN filter and fixed-lag smoother are simulated.
The discrete linear stochastic FOS is represented by the following state space representation:
Δγx(ζ+1)=Ax(ζ)+Bu(ζ)+ω(ζ), | (1) |
y(ζ)=Hx(ζ)+v(ζ), | (2) |
with
Δγx(ζ+1)=[Δn1x1,ζ+1⋮ΔnZxZ,ζ+1], |
where x(ζ)∈RZ is the state vector, y(ζ)∈Rm is observation vector, u(ζ)∈Rp is the system input, ω(ζ)∈RZ is the PWN, v(ζ)∈Rm is the OWN, n1,⋯,nZ are the orders of equations and are arbitrary constants, Z refers to the number of equations above, ΔnZxZ,ζ+1 is the nZ order difference of the Z component of x(ζ+1), ζϵZ+, and it can be obtained from the following G-L operator
Δnx(ζ)=1hn∑ζj=0(−1)j(nj)x(ζ−j), | (3) |
where is the sampling interval, it's assumed to be 1 throughout the paper, and the factor (nj) can be obtained by the following formula
(nj)={1forj=0,n(n−1)⋯(n−j+1)j!forj>0. |
Assumption 1. ω(ζ) and v(ζ) are uncorrelated white noise with zero mean and variance of Qζ and Rζ, respectively
Eω(ζ)=0,Ev(ζ)=0,E[ω(ζ)vT(ζ)]=0,∀ζ,j, |
E[ω(ζ)ωT(j)]=Qζδζj,E[v(ζ)vT(j)]=Rζδζj, | (4) |
where
δζζ=1,δζj=0(ζ≠j),Qζ>0,Rζ>0. |
Assumption 2. The initial state x(0) is disrelated to ω(ζ) and v(ζ), and
Ex(0)=μ0, | (5) |
E[(x(0)−μ0)(x(0)−μ0)T]=P0. | (6) |
Assumption 3. u(ζ) is a known deterministic control quantity.
The WNE problem for LDFOS (1) to (2) is given as follows:
Problem 1. Given an integer Z and based on the LDFOS (1) to (2) with observation {{y(j)}ζ+Zj=0}, find a suboptimal linear estimator ˆω(ζ|ζ+Z) of PWN ω(ζ), and a suboptimal linear estimator ˆv(ζ|ζ+Z) of OWN v(ζ) for Z=0, Z>0 or Z<0.
Remark 1. When Z=0, it corresponds to filtering WNE of LDFOS; when Z>0, it corresponds to fixed-lag smoothing WNE of LDFOS; when Z<0, it corresponds to multi-step predicting WNE of LDFOS, but according to the projection theorem, we can't get the estimation when Z<0. Therefore, we only discuss the cases when Z=0 and Z>0 in this paper.
According to system (1)–(2) and the definition of G-L operator, it is readily obtained the following state space model with delay of system (1) to (2), which is similar to Definition 3 in [9].
x(ζ+1)=Ax(ζ)+Bu(ζ)+ω(ζ)−∑ζ+1j=1(−1)jγjx(ζ+1−j), | (7) |
y(ζ)=Hx(ζ)+v(ζ), | (8) |
with
γζ=diag[(n1ζ)⋯(nZζ)]. |
The above formulas (7) and (8) can be proved by referring to (1)‒(3).
The state vector one-step predictor at time ζ is denoted as ˆx(ζ|ζ−1), the state vector filter at time ζ is denoted as ˆx(ζ|ζ).
Define the variance matrix of one-step predicting error, the variance matrix of filtering error, and innovation as
P(ζ|ζ−1)≜E[(x(ζ)−ˆx(ζ|ζ−1))(x(ζ)−ˆx(ζ|ζ−1))T], |
P(ζ|ζ)≜E[(x(ζ)−ˆx(ζ|ζ))(x(ζ)−ˆx(ζ|ζ))T], |
Z(ζ)≜y(ζ)−ˆy(ζ|ζ−1), |
where
ˆy(ζ|ζ−1)=Hˆx(ζ|ζ−1). |
In the following lemma, we give the one-step predictor for the LDFOS.
Lemma 1. According to system (1)‒(2), Assumptions 1‒3 and Problem 1, the one-step predictor is given by the following equations
ˆx(ζ+1|ζ)=Aˆx(ζ|ζ)+Bu(ζ)−∑ζ+1j=1(−1)jγjˆx(ζ+1−j|ζ+1−j), | (9) |
P(ζ|ζ−1)=(A+γ1)P(ζ−1|ζ−1)(A+γ1)T+Qζ−1+∑ζj=2γjP(ζ−j|ζ−j)γTj, | (10) |
ˆx(ζ|ζ)=ˆx(ζ|ζ−1)+K(ζ)Z(ζ), | (11) |
P(ζ|ζ)=(In−K(ζ)H)P(ζ|ζ−1), | (12) |
where
K(ζ)=P(ζ|ζ−1)HT(HP(ζ|ζ−1)HT+Rζ)−1, | (13) |
with the initial conditions
ˆx(0|−1)=μ0,P(0|0)=E[(x(0)−ˆx(0|0))(x(0)−ˆx(0|0))T]. |
Proof. Using projection formula and (7), we have
ˆx(ζ+1|ζ)=∑ζj=0E[x(ζ+1)ZT(j)][E[Z(j)ZT(j)]]−1Z(j)=∑ζj=0E[(Ax(ζ)+Bu(ζ)+ω(ζ)−∑ζ+1j=1γjx(ζ+1−j))ZT(j)][E[Z(j)ZT(j)]]−1Z(j)≈Aˆx(ζ|ζ)+Bu(ζ)−∑ζ+1j=1(−1)jγjˆx(ζ+1−j|ζ+1−j). | (14) |
Employing the same method, the filter can be computed by
ˆx(ζ|ζ)=∑ζj=0E[x(ζ)ZT(j)][E[Z(j)ZT(j)]]−1Z(j)=∑ζj=0E[(Ax(ζ−1)+Bu(ζ−1)+ω(ζ−1)−∑ζj=1(−1)jγjx(ζ−j))ZT(j)][E[Z(j)ZT(j)]]−1Z(j)=ˆx(ζ|ζ−1)+K(ζ)Z(ζ). |
According to (9) and innovation analysis technology, we have
P(ζ|ζ−1)=E[(x(ζ)−ˆx(ζ|ζ−1))(x(ζ)−ˆx(ζ|ζ−1))T]≈(A+γ1)P(ζ−1|ζ−1)(A+γ1)T+Qζ−1+∑ζj=2γjP(ζ−j|ζ−j)γTj. | (15) |
Applying the same analysis, we have P(ζ|ζ)=(In−K(ζ)H)P(ζ|ζ−1). The one-step gain matrix K(ζ) can be proved by the following formula
K(ζ)=E[x(ζ)ZT(ζ)][E[Z(ζ)ZT(ζ)]]−1=E[x(ζ)(v(ζ)+Hx(ζ)−Hˆx(ζ|ζ−1))T]×E[(v(ζ)+Hx(ζ)−Hˆx(ζ|ζ−1))(v(ζ)+Hx(ζ)−Hˆx(ζ|ζ−1))T]−1=P(ζ|ζ−1)HT(HP(ζ|ζ−1)HT+Rζ)−1. |
The proof is now completed.
Remark 2. In order to make it easier to calculate (14) and (15), we make a simplified assumption E[x(ζ+1−j|ζ)]≊E[x(ζ+1−j|ζ+1−j)],i=1,⋯,ζ+1.
This assumption implies that the past state vector is not updated by the newer data at time ζ. In this way, the calculation amount can be reduced without affecting the accuracy of the algorithm. Therefore, under this simplified assumption, the estimator obtained in this paper is suboptimal.
Based on the innovation analysis method, we have the following theorem.
Theorem 1. According to system (1)‒(2), Assumptions 1‒3 and Problem 1, the PWN estimator is proposed by the following equations
ˆω(ζ|ζ)=0,(Z=0), | (16) |
ˆω(ζ|ζ+1)=HTQζ[Rζ+H(P(ζ+1|ζ))HT]−1Z(ζ+1),(Z=1), | (17) |
ˆω(ζ|ζ+Z)=∑Zi=1Mω(ζ|ζ+i)Z(ζ+i),(Z>0), | (18) |
where
Mω(ζ|ζ+Z)=HT((A+γ1)T)Z−1∏Z−1i=1(In−K(ζ+i)H)T((A+γ1)TQζ−∑ζ+Zj=2(−1)jγTjQζ)[Rζ+Z+H(P(ζ+Z|ζ+Z−1))HT]−1. |
Proof. Note that L({{y(j)}j=ζj=1})⊂L({{v(j)}j=ζj=1},{{ω(j)}j=ζ−1j=0},x(0)) and Assumption 1, thus we have ω(ζ)⊥L({{y(j)}j=ζj=1}), that is ˆω(ζ|ζ)=proj(ω(ζ)|{{y(j)}j=ζj=1})=0.
We can prove (17) by projection theorem, namely one-step fixed-lag smoother
ˆω(ζ|ζ+1)=∑ζ+1j=0E[ω(ζ)ZT(j)][E[Z(j)ZT(j)]]−1Z(j)=ˆω(ζ|ζ)+E[ω(ζ)ZT(ζ+1)][E[Z(ζ+1)ZT(ζ+1)]]−1Z(ζ+1)=E[ω(ζ)ZT(ζ+1)][E[Z(ζ+1)ZT(ζ+1)]]−1Z(ζ+1), |
with
E[ω(ζ)ZT(ζ+1)]=E[ω(ζ)(v(ζ+1)+Hx(ζ+1)−Hˆx(ζ+1|ζ))T]≈E[ω(ζ)vT(ζ+1)]+HTE[ω(ζ)(Ax(ζ)+Bu(ζ)+ω(ζ)−∑ζ+1j=1(−1)jγjx(ζ+1−j)−Aˆx(ζ|ζ)−Bu(ζ)+∑ζ+1j=1(−1)jγjˆx(ζ+1−j|ζ+1−j))T]=HTQζ. |
For the calculation of E[Z(ζ+1)ZT(ζ+1)], please refer to the proof of K(ζ) in Lemma 1. Thus, we have
ˆω(ζ|ζ+1)=HTQζ[Rζ+H((A+γ1)P(ζ|ζ)(A+γ1)T+Qζ+∑ζ+1j=2γjP(ζ+1−j|ζ+1−j)γTj)HT]−1Z(ζ+1)=HTQζ[Rζ+H(P(ζ+1|ζ))HT]−1Z(ζ+1). |
Let the estimator gain be Mω(ζ|ζ+Z)=E[ω(ζ)ZT(ζ+Z)][E[Z(ζ+Z)ZT(ζ+Z)]]−1, so we have ˆω(ζ|ζ+Z)=ˆω(ζ|ζ+Z−1)+Mω(ζ|ζ+Z)Z(ζ+Z).
In the light of Assumptions 1‒3, system (1) and (2), we have the following suboptimal recursive PWN estimator
ˆω(ζ|ζ+Z)=∑ζ+Zj=0E[ω(ζ)ZT(j)][E[Z(j)ZT(j)]]−1Z(j)=∑ζ+Z−1j=0E[ω(ζ)ZT(j)][E[Z(j)ZT(j)]]−1Z(j)+Mω(ζ|ζ+Z)Z(ζ+Z)=ˆω(ζ|ζ+Z−1)+Mω(ζ|ζ+Z)Z(ζ+Z). |
Through the recurrence relation, we can get the formula of
ˆω(ζ|ζ+Z)=∑Zi=1Mω(ζ|ζ+i)Z(ζ+i), |
then
[ω(ζ)ZT(ζ+Z)]=E{ω(ζ)[v(ζ+Z)+Hx(ζ+Z)−Hˆx(ζ+Z|ζ+Z−1)]}≈E[ω(ζ)vT(ζ+Z)]+(A+γ1)THTE[ω(ζ)(x(ζ+Z−1)−ˆx(ζ+Z−1|ζ+Z−1))T]+HTE[ω(ζ)ωT(ζ+Z−1)]−HT∑ζ+Zj=2(−1)jγTjE[ω(ζ)(x(ζ+Z−j)−ˆx(ζ+Z−j|ζ+Z−j))T]=(A+γ1)THTE[ω(ζ)(x(ζ+Z−1)−ˆx(ζ+Z−1|ζ+Z−1))T]−HT∑ζ+Zj=2(−1)jγTjE[ω(ζ)(x(ζ+Z−j)−ˆx(ζ+Z−j|ζ+Z−j))T]. |
Using (14) in Lemma 1, we get
E[ω(ζ)(x(ζ+Z−1)−ˆx(ζ+Z−1|ζ+Z−1))T]=(In−K(ζ+Z−1)H)T{(A+γ1)THT×E[ω(ζ)(x(ζ+Z−2)−ˆx(ζ+Z−2|ζ+Z−2))T]+HTE[ω(ζ)ωT(ζ+Z−2)]−HT∑ζ+Z−1j=2(−1)jγTjE[ω(ζ)(x(ζ+Z−1−j)−ˆx(ζ+Z−1−j|ζ+Z−1−j))T]}, |
then
(A+γ1)THTE[ω(ζ)(x(ζ+Z−1)−ˆx(ζ+Z−1|ζ+Z−1))T]=((A+γ1)T)ZHT∏Z−1i=1(In−K(ζ+i)H)TQζ. |
Likewise, we have
∑ζ+Zj=2(−1)jγTjE[ω(ζ)(x(ζ+Z−j)−ˆx(ζ+Z−j|ζ+Z−j))T]=HT((A+γ1)T)Z−1∏Z−1i=1(In−K(ζ+i)H)T∑ζ+Zj=2(−1)jγTjQζ. |
Based on the above discussion, we have
Mω(ζ|ζ+Z)=HT((A+γ1)T)Z−1∏Z−1i=1(In−K(ζ+i)H)T((A+γ1)TQζ−∑ζ+Zj=2(−1)jγTjQζ)[Rζ+Z+H(P(ζ+Z|ζ+Z−1))HT]−1). |
Thus, the proof is completed.
Employing similar technical schemes, we can get the following theorem.
Theorem 2. According to system (1)‒(2), Assumptions 1‒3 and Problem 1, the OWN estimator is proposed by the following equations
ˆv(ζ|ζ)=Rζ[Rζ+H(P(ζ|ζ−1))HT]−1Z(ζ)(Z=0), | (19) |
ˆv(ζ|ζ+1)=ˆv(ζ|ζ)−RζKT(ζ)(A+γ1)THT[Rζ+H(P(ζ+1|ζ))HT]−1Z(ζ+1),(Z= |
1)ˆv(ζ|ζ+Z)=ˆv(ζ|ζ)+∑Zi=1Mv(ζ|ζ+i)Z(ζ+i),(Z>0), | (21) |
where
Mv(ζ|ζ+Z)=HTKT(ζ)((A+γ1)T)Z−1∏Z−1i=1(In−K(ζ+i)H)T((A+γ1)TRζ−∑ζ+Zj=2(−1)jγTjRζ)[Rζ+Z+H(P(ζ+Z|ζ+Z−1))HT]−1. |
Proof. We can prove the formula (19) by projection theorem, namely OWN filter
ˆv(ζ|ζ)=∑ζj=0E[v(ζ)ZT(j)][E[Z(j)ZT(j)]]−1Z(j)=ˆv(ζ|ζ−1)+E[v(ζ)ZT(ζ)][E[Z(ζ)ZT(ζ)]]−1Z(ζ)=0+E[v(ζ)ZT(ζ)][E[Z(ζ)ZT(ζ)]]−1Z(ζ)=E[v(ζ)ZT(ζ)][E[Z(ζ)ZT(ζ)]]−1Z(ζ), |
with
E[v(ζ)ZT(ζ)]=E{v(ζ)[v(ζ)+Hx(ζ)−Hˆx(ζ|ζ−1)]T}≈E[v(ζ)vT(ζ)]+HTE[v(ζ)(Ax(ζ−1)+Bu(ζ−1)+ω(ζ−1)−∑ζ+1j=1(−1)jγjx(ζ−j)−Aˆx(ζ−1|ζ−1)−Bu(ζ−1)+∑ζ+1j=1(−1)jγjˆx(ζ−j|ζ−j))T]=Rζ. |
And E[Z(ζ)ZT(ζ)] is computed by the proof of K(ζ) in Lemma 1. Thus, we have
ˆv(ζ|ζ)=Rζ[Rζ+H((A+γ1)P(ζ−1|ζ−1)(A+γ1)T+Qζ−1+∑ζj=2γjP(ζ−j|ζ−j)γTj)HT]−1Z(ζ)=Rζ[Rζ+H(P(ζ|ζ−1))HT]−1Z(ζ). |
By projection formula and filter Eq (19), we have
ˆv(ζ|ζ+1)=Rζ[Rζ+H(P(ζ|ζ−1))HT]−1Z(ζ)−KT(ζ)Rζ(A+γ1)THT[Rζ+H((A+γ1)P(ζ|ζ)(A+γ1)T+Qζ+∑ζj=2γjP(ζ+1−j|ζ+1−j)γTj)HT]−1Z(ζ+1)=ˆv(ζ|ζ)−RζKT(ζ)(A+γ1)THT[Rζ+H(P(ζ+1|ζ))HT]−1Z(ζ+1). |
In the light of Assumptions 1‒3, system (1) and (2), we have the following suboptimal recursive OWN estimator
ˆv(ζ|ζ+Z)=ˆv(ζ|ζ)+∑ζ+Zj=0[v(ζ)ZT(j)][E[Z(j)ZT(j)]]−1Z(j)=ˆv(ζ|ζ)+ˆv(ζ|ζ+Z−1)+E[v(ζ)ZT(ζ+Z)][E[Z(ζ+Z)ZT(ζ+Z)]]−1Z(ζ+Z). |
Let the estimator gain be Mv(ζ|ζ+Z)=E[v(ζ)ZT(ζ+Z)][E[Z(ζ+Z)ZT(ζ+Z)]]−1, so we get ˆv(ζ|ζ+Z)=ˆv(ζ|ζ)+ˆv(ζ|ζ+Z−1)+Mv(ζ|ζ+Z)Z(ζ+Z).
Through the recurrence relation, we can get the formula of
ˆv(ζ|ζ+Z)=ˆv(ζ|ζ)+∑Zi=1Mv(ζ|ζ+i)Z(ζ+i), |
with
E[v(ζ)ZT(ζ+Z)]=E{v(ζ)[v(ζ+Z)+Hx(ζ+Z)−Hˆx(ζ+Z|ζ+Z−1)]T}≈E[v(ζ)vT(ζ+Z)]+HT(A+γ1)TE[v(ζ)(x(ζ+Z−1)−ˆx(ζ+Z−1|ζ+Z−1))T]+HTE[v(ζ)ωT(ζ+Z−1)]−HT∑ζ+Zj=2(−1)jγTjE[v(ζ)(x(ζ+Z−j)−ˆx(ζ+Z−j|ζ+Z−j))T]=HT(A+γ1)TE[v(ζ)(x(ζ+Z−1)−ˆx(ζ+Z−1|ζ+Z−1))T]−HT∑ζ+Zj=2(−1)jγTjE[v(ζ)(x(ζ+Z−j)−ˆx(ζ+Z−j|ζ+Z−j))T]. |
Using (14) in Lemma 1, we can get
E[v(ζ)(x(ζ+Z−1)−ˆx(ζ+Z−1|ζ+Z−1))T]=(In−K(ζ+Z−1)H)T{(A+γ1)T×E[v(ζ)(x(ζ+Z−2)−ˆx(ζ+Z−2|ζ+Z−2))T+HTE[v(ζ)ωT(ζ+Z−2)]−HT∑ζ+Z−1j=2(−1)jγTjE[v(ζ)(x(ζ+Z−1−j)−ˆx(ζ+Z−1−j|ζ+Z−1−j))T]. |
With the same recursion method as Theorem 1, we get
Mv(ζ|ζ+Z)=HTKT(ζ)((A+γ1)T)Z−1∏Z−1i=1(In−K(ζ+i)H)T((A+γ1)TRζ−∑ζ+Zj=2(−1)jγTjRζ)[Rζ+Z+H(P(ζ+Z|ζ+Z−1))HT]−1. |
The proof is now completed.
Example 1. The following example considers LDFOS described by (1) and (2), where the system matrix is given by
A=[−0.110−0.2],B=[00],H=[0.60.3]. |
PWN and OWN are both uncorrelated white noises, satisfying Rζ=0.09 and Qζ=[0.360;01]. The fractional order n=0.6, and set u(ζ)=0. According to Theorem 1, when N=0, the filtering estimate of PWN is 0. The signal, filtering estimate, one-step smoothing estimate and five-step smoothing estimate of PWN are shown in Figures 1 and 2, in which Figure 1 is the simulation result of the first component ω1 in PWN and Figure 2 is the simulation result of the second component ω2 in PWN. The signal, filtering estimate, one-step smoothing estimate and five-step smoothing estimate of OWN are shown in Figure 3. It is shown that the smoothing estimate has higher accuracy, and the five-step smoothing estimate of ω2 has better estimation performance than the one-step smoothing estimate.
Example 2. In order to further illustrate the effectiveness of our algorithm, in this example, it is assumed that the parameter matrices of LDFOS is the same as the simulation system in [12], that is
A=[0−0.110.2],B=[0.20.3],H=[0.40.3], |
where the fractional order n = 0.5, the PWN, OWN and input u(ζ) are the same as Example 1.
The signal, filtering estimation, one-step smoothing estimation and five-step smoothing estimation of PWN and OWN are shown in Figures 4‒6. It can be seen from the figures that our algorithm has accurate estimation performance, especially the fixed-lag smoothing estimation performance is better. Although fixed-lag smoothing estimation will lead to estimation delay, we can make an appropriate compromise between accuracy and fixed-lag steps to meet the requirements of rapidity and accuracy in practical application.
Based on the orthogonal projection theory in time domain, a unified approach to PWN and OWN filtering and smoothing for LDFOS has been given. Through G-L operator, LDFOS has been converted into a class of linear discrete system with cumulative sum time-delay. Suboptimal approximation algorithm has been used to reduce the complexity caused by cumulative sum time-delay in the system. Nevertheless, the Riccati equation and white noise estimator gain matrices are still different from the normal white noise estimators, which naturally include cumulative sum gain terms respectively. The results of this paper can be applied to the normal systems (n = 0) as a special case. The algorithm has recursive characteristics and is suitable for real-time online calculation.
Thanks to the research was partially funded by Shandong Province Higher Educational Science and Technology Program (Grant No. J18KB157), and National Natural Science Foundation of China (Grant Nos. 62001261 and 61403061).
The authors declare no conflict and interest.
[1] |
A. Ahlén, M. Sternad, Optimal deconvolution based on polynomial methods, IEEE Transactions on Acoustics, Speech, and Signal Processing, 37 (1989), 217‒226. http://dx.doi.org/10.1109/29.21684 doi: 10.1109/29.21684
![]() |
[2] |
J. Mendel, White-noise estimators for seismic data processing in oil exploration, IEEE T. Automat. Contr., 22 (1977), 694‒706. http://dx.doi.org/10.1109/TAC.1977.1101597 doi: 10.1109/TAC.1977.1101597
![]() |
[3] |
Y. Wang, Y. Zheng, Kalman filter based fault diagnosis of networked control system with white noise, J. Control Theory Appl., 1 (2005), 55‒59. http://dx.doi.org/10.1007/s11768-005-0061-y doi: 10.1007/s11768-005-0061-y
![]() |
[4] |
R. Singh, H. Parthasarathy, J. Singh, Time-varying image restoration using extended Kalman filter, Proceedings of 15th IEEE India Council International Conference (INDICON), 2018, 1‒6. http://dx.doi.org/10.1109/INDICON45594.2018.8986978 doi: 10.1109/INDICON45594.2018.8986978
![]() |
[5] |
J. Jiang, Y. Li, P. Zhang, Q. Hao, X. Ma, Y. Fan, Adaptive acoustic masking based on spectral envelope, Proceedings of International Conference on Audio, Language and Image Processing (ICALIP), 2016,442‒446. http://dx.doi.org/10.1109/ICALIP.2016.7846619 doi: 10.1109/ICALIP.2016.7846619
![]() |
[6] |
F. Atici, P. Eloe, Initial value problems in discrete fractional calculus, P. Am. Math. Soc., 137 (2009), 981‒989. http://dx.doi.org/10.1090/s0002-9939-08-09626-3 doi: 10.1090/s0002-9939-08-09626-3
![]() |
[7] |
L. Huang, G. Wu, D. Baleanu, H. Wang, Discrete fractional calculus for interval-valued systems, Fuzzy Set. Syst., 404 (2021), 141‒158. http://dx.doi.org/10.1016/j.fss.2020.04.008 doi: 10.1016/j.fss.2020.04.008
![]() |
[8] |
G. Wu, M. Cankaya, S. Banerjee, Fractional q-deformed chaotic maps: a weight function approach, Chaos, 12 (2020), 112106. http://dx.doi.org/10.1063/5.0030973 doi: 10.1063/5.0030973
![]() |
[9] | D. Sierociuk, A. Dzielinski, Fractional Kalman filter algorithm for the states, parameters and order of fractional system estimation, Int. J. Appl. Math. Comput. Sci., 16 (2006), 129‒140. |
[10] |
J. Zarei, M. Tabatabaei, R. Razavi-Far, M. Saif, Fractional order unknown input filter design for fault detection of discrete linear systems, Proceedings of IECON - 43rd Annual Conference of the IEEE Industrial Electronics Society, 2017, 4333‒4338. http://dx.doi.org/10.1109/IECON.2017.8216745 doi: 10.1109/IECON.2017.8216745
![]() |
[11] |
H. Sadeghian, H. Salarieh, A. Alasty, A. Meghdari, On the general Kalman filter for discrete time stochastic fractional systems, Mechatronics, 23 (2013), 764‒771. http://dx.doi.org/10.1016/J.MECHATRONICS.2013.02.006 doi: 10.1016/J.MECHATRONICS.2013.02.006
![]() |
[12] |
D. Sierociuk, I. Tejado, B. Vinagre, Improved fractional Kalman filter and its application to estimation over lossy networks, Signal Process., 91 (2011), 542‒552. http://dx.doi.org/10.1016/j.sigpro.2010.03.014 doi: 10.1016/j.sigpro.2010.03.014
![]() |
[13] |
R. Ram, M. Mohanty, Application of fractional calculus in speech enhancement: a novel approach, Proceedings of 2nd International Conference on Data Science and Business Analytics (ICDSBA), 2018,123‒126. http://dx.doi.org/10.1109/ICDSBA.2018.00029 doi: 10.1109/ICDSBA.2018.00029
![]() |
[14] |
X. Wu, Y. Sun, Z. Lu, Z. Wei, M. Ni, W. Yu, A modified Kalman filter algorithm for fractional system under Lévy noises, J. Franklin. I., 352 (2015), 1963‒1978. http://dx.doi.org/10.1016/j.jfranklin.2015.02.008 doi: 10.1016/j.jfranklin.2015.02.008
![]() |
[15] |
J. Wang, L. Zhang, J. Mao, J. Zhou, D. Xu, Fractional order equivalent circuit model and SOC estimation of supercapacitors for use in HESS, IEEE Access, 7 (2019), 52565‒52572. http://dx.doi.org/10.1109/ACCESS.2019.2912221 doi: 10.1109/ACCESS.2019.2912221
![]() |
[16] |
G. Jin, L. Li, Y. Xu, M. Hu, C. Fu, D. Qin, Comparison of SOC estimation between the integer-order model and fractional-order model under different operating conditions, Energies, 13 (2020), 1785. http://dx.doi.org/10.3390/en13071785 doi: 10.3390/en13071785
![]() |
[17] |
Q. Zhu, M. Xu, W. Liu, M. Zheng, A state of charge estimation method for lithium-ion batteries based on fractional order adaptive extended kalman filter, Energy, 187 (2019), 115880. http://dx.doi.org/10.1016/J.ENERGY.2019.115880 doi: 10.1016/J.ENERGY.2019.115880
![]() |
[18] |
M. Xu, Q. Zhu, M. Zheng, A state of charge estimation approach based on fractional order adaptive extended Kalman filter for lithium-ion batteries, Proceedings of IEEE 7th Data Driven Control and Learning Systems Conference (DDCLS), 2018,271‒276. http://dx.doi.org/10.1109/DDCLS.2018.8516091 doi: 10.1109/DDCLS.2018.8516091
![]() |
[19] | T. Raïssi, M. Aoun, On robust pseudo state estimation of fractional order systems, In: Lecture notes in control and information sciences, Switzerland: Springer, 2016, 97‒111. http://dx.doi.org/10.1007/978-3-319-54211-9_8 |
[20] |
G. Wu, M. Luo, L. Huang, S. Banerjee, Short memory fractional differential equations for new neural network and memristor design, Nonlinear Dyn., 100 (2020), 3611‒3623. http://dx.doi.org/10.1007/s11071-020-05572-z doi: 10.1007/s11071-020-05572-z
![]() |
[21] |
H. Dehestani, Y. Ordokhani, M. Razzaghi, Fractional-order Bessel wavelet functions for solving variable order fractional optimal control problems with estimation error, Int. J. Syst. Sci., 51 (2020), 1032‒1052. http://dx.doi.org/10.1080/00207721.2020.1746980 doi: 10.1080/00207721.2020.1746980
![]() |
[22] |
H. Li, State estimation for fractional-order complex dynamical networks with linear fractional parametric uncertainty, Abstr. Appl. Anal., 2013 (2013), 178718. http://dx.doi.org/10.1155/2013/178718 doi: 10.1155/2013/178718
![]() |
[23] |
T. Abdeljawad, S. Banerjee, G. Wu, Discrete tempered fractional calculus for new chaotic systems with short memory and image encryption, Optik, 218 (2020), 163698. http://dx.doi.org/10.1016/j.ijleo.2019.163698 doi: 10.1016/j.ijleo.2019.163698
![]() |
[24] |
D. Idiou, A. Charef, A. Djouambi, Linear fractional order system identification using adjustable fractional order differentiator, IET Signal Process., 8 (2014), 398‒409. http://dx.doi.org/10.1049/iet-spr.2013.0002 doi: 10.1049/iet-spr.2013.0002
![]() |