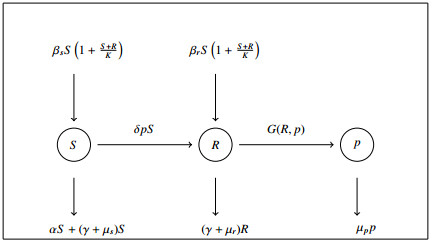
Antibiotic resistance is one of the top 10 public health problems that most affects humanity. In recent decades, plasmid-mediated antibiotic resistance (PMAR) has increased. However, due to the lack of knowledge about the biology of plasmids, there are gaps in the role played by them within antibiotic resistance. In this sense, properties that agree with the biological phenomenon and have contributed to the understanding of PMAR have been discovered from mathematical modeling. In this work, we focus on the role that the plasmid replication rate plays in the elimination or spread of bacteria both sensitive and resistant to antibiotics. Qualitative analysis reveals the existence of a free-bacteria equilibrium point, a resistant equilibrium point, and two coexistence equilibrium points (high and low bacterial load). If each bacterium (sensitive or resistant) produces at most one new bacterium, the infection will be controlled or eliminated. If each bacterium (sensitive or resistant) produces more than one bacteria, several scenarios of bacterial progression are presented that depend on the plasmid replication rate. The results suggest that plasmid replication is essential for the outcome of bacterial infection at the local level.
Citation: Eduardo Ibargüen-Mondragón, Miller Cerón Gómez, Edith M. Burbano-Rosero. Assessing the role of bacterial plasmid replication in a competition model of sensitive and resistant bacteria to antibiotics[J]. AIMS Mathematics, 2021, 6(9): 9446-9467. doi: 10.3934/math.2021549
[1] | Ziran Cheng, Sze-Bi Hsu . Dynamics of drug on-drug off models with mutations in morbidostat — Dedicated to the seventieth birthday of Professor Gail Wolkowicz. AIMS Mathematics, 2023, 8(9): 20815-20840. doi: 10.3934/math.20231061 |
[2] | Weiyu Li, Hongyan Wang . The instability of periodic solutions for a population model with cross-diffusion. AIMS Mathematics, 2023, 8(12): 29910-29924. doi: 10.3934/math.20231529 |
[3] | Joonwoo Park, Raechoong Kang . Analytic solution for the lightning current induced mutually coupled resistive filament wire model. AIMS Mathematics, 2023, 8(8): 19637-19655. doi: 10.3934/math.20231001 |
[4] | Yingyan Zhao, Changjin Xu, Yiya Xu, Jinting Lin, Yicheng Pang, Zixin Liu, Jianwei Shen . Mathematical exploration on control of bifurcation for a 3D predator-prey model with delay. AIMS Mathematics, 2024, 9(11): 29883-29915. doi: 10.3934/math.20241445 |
[5] | Sait Kıkpınar, Mahmut Modanli, Ali Akgül, Fahd Jarad . Finite difference method for transmission dynamics of Contagious Bovine Pleuropneumonia. AIMS Mathematics, 2022, 7(6): 10303-10314. doi: 10.3934/math.2022574 |
[6] | Liye Wang, Wenlong Wang, Ruizhi Yang . Stability switch and Hopf bifurcations for a diffusive plankton system with nonlocal competition and toxic effect. AIMS Mathematics, 2023, 8(4): 9716-9739. doi: 10.3934/math.2023490 |
[7] | Ruizhi Yang, Dan Jin, Wenlong Wang . A diffusive predator-prey model with generalist predator and time delay. AIMS Mathematics, 2022, 7(3): 4574-4591. doi: 10.3934/math.2022255 |
[8] | Chenxuan Nie, Dan Jin, Ruizhi Yang . Hopf bifurcation analysis in a delayed diffusive predator-prey system with nonlocal competition and generalist predator. AIMS Mathematics, 2022, 7(7): 13344-13360. doi: 10.3934/math.2022737 |
[9] | Jorge Luis Ramos-Castellano, Miguel Angel Dela-Rosa, Iván Loreto-Hernández . Bifurcation analysis for the coexistence in a Gause-type four-species food web model with general functional responses. AIMS Mathematics, 2024, 9(11): 30263-30297. doi: 10.3934/math.20241461 |
[10] | Anna Sun, Ranchao Wu, Mengxin Chen . Turing-Hopf bifurcation analysis in a diffusive Gierer-Meinhardt model. AIMS Mathematics, 2021, 6(2): 1920-1942. doi: 10.3934/math.2021117 |
Antibiotic resistance is one of the top 10 public health problems that most affects humanity. In recent decades, plasmid-mediated antibiotic resistance (PMAR) has increased. However, due to the lack of knowledge about the biology of plasmids, there are gaps in the role played by them within antibiotic resistance. In this sense, properties that agree with the biological phenomenon and have contributed to the understanding of PMAR have been discovered from mathematical modeling. In this work, we focus on the role that the plasmid replication rate plays in the elimination or spread of bacteria both sensitive and resistant to antibiotics. Qualitative analysis reveals the existence of a free-bacteria equilibrium point, a resistant equilibrium point, and two coexistence equilibrium points (high and low bacterial load). If each bacterium (sensitive or resistant) produces at most one new bacterium, the infection will be controlled or eliminated. If each bacterium (sensitive or resistant) produces more than one bacteria, several scenarios of bacterial progression are presented that depend on the plasmid replication rate. The results suggest that plasmid replication is essential for the outcome of bacterial infection at the local level.
Just as vaccines have saved the lives of millions of children and contributed to the eradication of diseases around the world, antibiotics have been effective in curing different types of infection caused by bacteria. Currently, antibiotics are the medications most prescribed by doctors to treat diseases caused by bacteria, and although it has not been a century since Sir Alexander Fleming discovered penicillin in 1928, there is already a global threat caused by the resistance of certain bacteria to first-line antibiotics.
Bacteria can acquire antibiotic resistance by transferring genetic material. They can be classified as vertical gene transfer (including spontaneous or acquired mutations) and horizontal gene transfer, which can occur through the transformation, conjunction, or transduction of mobile genetic elements that function as vehicles. Such elements include plasmids, transposons, integrons, and integrative conjugative elements.
Plasmid-mediated resistance (due to the transfer of antibiotic resistance genes carried on plasmids) is increasing worldwide. Bacterial plasmids are circular or linear extrachromosomal DNA molecules. They carry antimicrobial resistance genes coding for proteins such as colistin, ESBL (extended-spectrum β-lactamases), carbapenemases, fluoroquinolones, and aminoglycosides. This resistance has resulted in a global epidemic [1,2]. A plasmid is between 1 and 250 kb long (one kilobase is 1000 base pairs of DNA or RNA). A cell can have from a single copy of a plasmid to a few hundred copies [3]. Multi-drug resistance caused by plasmids has been reported for several bacterial strains [4,5].
Much are still unknown about the biology of plasmids. However, since they are associated with antibiotic resistance, a considerable amount of research has been conducted to establish their role in this process. Identification of the characteristics and properties of plasmids has contributed to our understanding of the dynamics of the acquisition of antibiotic resistance [6]. In particular, plasmid-mediated resistance has been studied by isolating E. coli strains in vitro, and interesting results have been obtained from experiments on the replication, transfer, and propagation of plasmids, which have implications in bacterial growth [7,8,9,10]. The results of research at the biological level can be complemented or used as a basis for new studies. In this sense, we find mathematical modeling mainly focuses on PMAR [11,12,13,14].
In particular, Ibarguen et al.[15] developed a model of the interactions between bacteria sensitive and resistant to antibiotics. The model considers the acquisition of resistance due to plasmids. From a qualitative analysis of this model, we obtained three steady states: (1) a bacteria-free state, E0, (2) a state where only resistant bacteria are present, E1, and (3) a state where sensitive and resistant bacteria coexist, E2. Additionally, a stability region was determined for each equilibrium that depends on the values of parameters similar to basic reproductive numbers for sensitive and resistant bacteria. Ibarguen et al.[16] modified the model to use a dynamic variable for the acquisition of resistance due to plasmids. The results obtained from the qualitative analysis of this model were interesting because in addition to the previously mentioned scenarios, there was a new scenario in which a limit cycle emerges around a coexistence equilibrium (Hopf bifurcation). The limit cycle corresponds to the self-regulation of bacterial growth, which could minimize the transmission of plasmids between bacteria. In the previous model, the functional response for plasmid replication was key in the appearance of the limit cycle. These results confirm self-regulation processes in the dynamics of bacterial growth [17]. Furthermore, different studies reveal that plasmids play a fundamental role in PMAR [18,19].
In this work, we formulate and analyze a generalization of the model developed in [16], in which the plasmid replication rate is defined using a C1-function.
The World Health Organization has declared antimicrobial resistance among the top 10 global threats to public health. In this sense, the fact that aggravates the situation is the use of antibiotics for treating humans and animals. The above has allowed the horizontal transmission of resistance genes, driving the growing trend of PMAR. However, due to the lack of knowledge about the biology of the plasmids, many gaps are related to their role in antimicrobial resistance. This has led to the development of scientific production that reveals the importance of the transfer and replication of plasmids in biological phenomena. Nevertheless, discovering the properties and factors of the interaction dynamics of bacteria and plasmids is still a challenge. In particular, the form of plasmids replication is not yet clear. The above motivated us to study the role played by plasmids replication in the plamid-meadiated resistance. In [16,20] we formulated mathematical models considering specific functional responses for the plasmids growth rate. In the first one we supposed that plasmids growth rate, G, is proportional to the resistant bacteria; that is, G(R,P)=σpR where σp is a constant plasmid reproduction rate, and in the second one, we supposed that the plasmids replication rate follows a generalized law of mass action, G(R,P)=σpPaRb where a,b∈R are constants. We obtained consistent results with the biological phenomenon.
In this work, we develop a mathematical model that describes the interaction dynamics between bacteria and plasmids in which the plasmid replication rate is an undefined function that satisfies the common properties of the plasmid replication rate defined in [16,20].
In this section, we formulate a model on bacterial resistance that describes the interaction of susceptible bacteria, resistant bacteria and plasmids populations. Let us denote by S(t), and R(t) the population sizes of susceptible, and resistant bacteria to antibiotics at time t, respectively; and p(t) the number of plasmids at time t. As in [21], we assume that bacteria follow a logistic growth with carrying capacity K. Let βs and βr the birth rate of susceptible and resistant bacteria, respectively. Specific mutations that confer resistance to chemical control often have an inherent fitness cost which may be manifested through reduced reproductive capacity or competitive ability [22]. In this work, we quantify fitness cost as a reduction in the reproduction rate of the resistant strain, therefore βr≤βs. Susceptible and resistant bacteria have per capita natural death rates μs, and μr, respectively. Susceptible bacteria also die due to the action of the antibiotics, and we assume that the rate at which they are killed by the antibiotic is equal to αS. Further, it is assumed that during treatment with antibiotics, the process of bacterial conjugation for the transfer of resistant plasmids is conducted. In this process, susceptible bacteria are reservoir and resistant bacteria are donors of genetic material which is represented by the term δPR, with δ the rate of transfer of resistant plasmids among bacteria. Susceptible and resistant bacteria are eliminated by the host immune system at per capita rate γ. The plasmids degrade at a constant rate μp. There is a symbiosis between plasmids and host cells. Plasmids replicate autonomously in the bacterial cell and use them for their propagation. Plasmid replication is a function of the plasmid and the host cell [23]. Since we are only interested in the replication of resistant plasmids, we assume that plasmid replication rate is given by the functional response G(R,p) where G:R2→R+0 is a C1 function. Under the abovementioned assumptions, we obtain the following system of ordinary differential equations:
dSdt=βsS(1−S+RK)−αS−δpS−γS−μsSdRdt=βrR(1−S+RK)+δpS−γR−μrRdpdt=G(R,p)−μpp. | (2.1) |
The Figure 1 shows the flow diagram of system (2.1). With the following change of variables s=S/K and r=R/K the system (2.1) is reduced to
dsdt=βss[1−(s+r)]−αs−δps−(γ+μs)sdrdt=βrr[1−(s+r)]+δps−(γ+μr)rdpdt=g(r,p)−μpp, | (2.2) |
where g(r,p)=G(Kr,p).
The matrix form of (2.2) is given by
dxdt=f(x)=Ax+h(x), | (3.1) |
where
x=(srp),h(x)=(−βss(s+r)−δps−βrr(s+r)+δpsg(r,p)), | (3.2) |
and
A=(βs(1−1Rs)000βr(1−1Rr)000−μp), | (3.3) |
where
Rr=βrγ+μrandRs=βsα+γ+μs. | (3.4) |
The parameter Rr defined in (3.4) is interpreted as the number of bacteria produced by the fraction of resistant bacteria that evade the immune response, and Rs is interpreted as the number of bacteria generated by the fraction of sensitive bacteria that survive the effects due to antibiotics and the immune system response.
Since g∈C1(R2), then f∈C1(R3). Therefore, there exists an ε>0 such that the initial value problem (ivp) defined by (3.1) and x(0)=x0∈R3, has a solution x(t) on the interval [0,ε] (Fundamental theorem of existence and uniqueness, [24]). In addition, for a compact set Ω⊂E such that
{y∈Rn:y=x(t)for somet∈[0,β)}⊂Ω, |
it follows that ε=∞. In consequence, the ivp has a solution x(t) for all t≥0 (Corollary 2, page 91, [24]). Now, we will prove the existence of bounded solutions. To this end, we will use the results developed by W. Karpińska in [25], about bounded solutions of dynamical systems, which establish that under the following assumptions,
1. A∈(R3) defined in (6) is a self-adjoint operator, and 0 is its eigenvalue, and
2. h:R×R3→R3 defined in (3.2) is a Carathéodory function; that is,
(a) h is a map measurable with respect to the first variable.
(b) h is continuous with respect to the second one, and
(c) for any l∈R there exists a locally integrable function Ml:R→R that satisfies ‖h(t,x)‖≤Ml(t) for ‖x‖≤l.
The existence and uniqueness of bounded solutions on R of system (3.1) were obtained. Since A is a diagonal matrix, the first item is satisfied. On the other hand, h(⋅,x) is constant function which implies that h is measurable with respect to t. Since the function g is continuous with respect to x, then h is continuous with respect to x. By adding the first two equations of (2.2) we obtain
dsdt+drdt=(βss+βrr)[1−(s+r)]−αs−(γ+μs)s−(γ+μr)r≤(βss+βrr)[1−(s+r)]≤β(s+r)[1−(s+r)], | (3.5) |
where β=max(βs,βr). The solution of inequality (3.5) satisfies 0≤s(t)+r(t)≤1 for t≥0. Now, for t∈R we have
‖h(t,x)‖22=(βss(t)(s(t)+r(t))+δp(t)s(t))2+(−βrr(t)(s(t)+r(t))+δp(t)s(t))2+(g(r(t),p(t)))2=[(βss(t))2+(βrr(t))2](s(t)+r(t))2+2(βss(t)−βrr(t))(s(t)+r(t))δp(t)s(t)+2(δp(t)s(t))2+(g(r(t),p(t)))2≤β2s+β2r+2(βS+βr)δp(t)s(t)+2(δp(t)s(t))2+(g(r(t),p(t)))2=M2l(t), | (3.6) |
where
Ml(t)=√(βs+δp(t)s(t))2+(βr+δp(t)s(t))2+(g(r(t),p(t)))2. | (3.7) |
Since g∈C1(R), then Ml is a locally integrable function. Thefere, the item 2 is satisfied. The above implies the existence of an unique bounded solution of ivp defined by (3.1) and x(0)=x0. Let
K={˜p∈C1:˜p(t)is a bounded solution of the third equation of (2.2)}. |
The invariant set of system (2.2) is given by the following compact set
Ω={(s,r,p)∈R3+:0≤s,r≤1,0≤s+r≤1,0≤p≤M}, | (3.8) |
where M=sup{|˜p(t)|:t≥0}. The results of this section are summarized in the following lemma, which assures that system (2.2) is well posed in the sense that solutions with initial conditions in Ω remain there for all t≥0.
Lemma 3.1. The set Ω defined in (3.8) is positively invariant with respect to system (2.2).
At biological level, there are inhibition mechanisms of the host cell that control the plasmid replication rate, which allows reaching steady state conditions for the plasmid copy number [23]. The plasmid replication rate and inhibition period directly affect one another [18]. Since the reciprocal of the inhibition period is the degradation rate of plasmids, then a necessary condition for the existence of equilibria is g(r,p)=μpp. Another necessary condition is that the previous equation has at least one solution. For convenience, we suppose that the solution is a function of plasmids p∗ in terms of resistant bacteria r. Plasmid is generally replicated on constant average per cell and cell cycle, which implies that p∗ follows an exponential growth. This behavior has been experimentally verified in several research works [18,23]. Finally, since plasmids cannot replicate in the absence of resistant bacteria, then we assume p∗(0)=0. From the above, we obtain the following assumption.
C1: the equation g(r,p)=μpp has unique non-negative solution p∗(r) which is a increasing convex function that satisfies p∗(0)=0.
In this section, we will determine the equilibrium points of system (2.2) under the condition C1. The equilibria of system (2.2) are given by the solutions of the algebraic equations
Ax+h(x)=0. | (4.1) |
Now, we will find the equilibrium points for which s=0. In this case, the system (4.1) is reduced to
[βr(1−r)−(γ+μr)]r=0g(r,p)=μpp. | (4.2) |
The condition C1, implies the existence of an unique solution p(r) of the second equation of (4.2) that satisfies p(0)=0. Solutions of the first equation of (4.2) are r=0 and r=r1, where
r1=Rr−1Rr. | (4.3) |
By substituting r=r1 in p∗(r), we obtain p1=p∗(r1), which implies the exitence of the equilibrium point x1=(0,r1,p1)T. By substituting r=0 we obtain the equilibrium x0≡(0,0,0)T. The above results are summarized in the following proposition.
Proposition 4.1. If condition C1 is satisfied, then the systen (2.2) always has the equilibrium x0≡0 in Ω, and if R1>1, in addition there exists an equilibrium point x1=(0,r1,p1)T∈Ω.
Now, for s≠0 the system (4.1) is reduced to
βs(1−1Rs)−βs(s+r)−δp=0βrr[1−1Rr−(s+r)]+δps=0g(r,p)−μpp=0. | (4.4) |
From the first equation of (4.4) we obtain
Rs−1Rs=s+r+δβsp. | (4.5) |
From (4.5) is concluded that a necessary condition for the existence of plasmids, susceptible and resistant bacteria is Rs>1. Solving for s in (4.5) we obtain
s=Rs−1Rs−(r+δβsp). | (4.6) |
Note that a necessary and sufficient condition such that s defined in (4.6) be positive is
r+δβsp<Rs−1Rs. | (4.7) |
By Substituting (4.6) in the second equation of (4.4) we obtain
βrr(1−1Rr−r)+(δp−βrr)[Rs−1Rs−(r+δβsp)]=0. | (4.8) |
From (4.8) we obtain the following equation
p2+b(r)p+c(r)=0, | (4.9) |
where
b(r)=βs−βrδ(r−rnp)c(r)=(1Rr−1Rs)βsβrδ2r, | (4.10) |
being
rnp=1−1Rs1−βrβs. | (4.11) |
The solutions of (4.9) are given by
p±(r)=−b(r)2±√[b(r)2]2−c(r). | (4.12) |
Now, we will determine the conditions for the existence of coexistence equilibria. To this end, we will analyze the requirements for which p± defined in (4.12) are positive, negative or complex functions, considering the following cases Rs>Rr, Rs=Rr and Rs<Rr, and we will find the number of positive solutions of the following equations
p+(r)=p∗(r)andp−(r)=p∗(r), | (4.13) |
in the interval (0,1), where p∗(r) is the function of hypothesis C1 that satisfies p∗(0)=0.
Condition Rs<Rr : In this case c(r)<0, which implies that p± are real value functions. On the other hand, the sign of b(r) depends on the value of rnp. In consequence:
1. If r<rnp, then b(r)<0 which implies that p+ is a positive and increasing concave function (p+(r)>0), and p− is a negative and decreasing convex function (p−(r)<0). Since p−(0)=0 and p+(0)=−b(0)>0, then the functions p− and p∗ intersect at r=0 and the functions p+ and p∗ intersect at r+∈(0,1) if and only if p+(1)<p∗(1) (See Figure 2a). In consequence, the equation p−(r)=p∗(r) does not have a positive solution, and the equation p+(r)=p∗(r) only has one positive solution r+ if and only if p+(1)<p∗(1). Therefore, if Rs<Rr and p+(1)<p∗(1) there exists an equilibrium point x+=(s+,r+,p+) in the subset Ω1⊂Ω given by
Ω1={(s,r,p)∈R3+:0≤s+r≤1,r<rnp,p≤M}. |
2. If r=rnp, then b(r)=0 which implies that p+(r)=√−c(r) is a positive and increasing concave function, and p−(r)=−√−c(r) is a negative and decreasing convex function. Following a procedure similar to the previous case, we conclude that if Rs<Rr and p+(1)<p∗(1) there exists an equilibrium point x+=(s+,r+,p+) in the subset Ω2⊂Ω given by
Ω2={(s,r,p)∈R3+:0≤s+r≤1,r=rnp,p≤M}. |
3. If r>rnp, then b(r)>0. We verify that if Rs<Rr and p+(1)<p∗(1), there exits an equilibrium point x+=(s+,r+,p+) in the subset Ω3⊂Ω given by
Ω3={(s,r,p)∈R3+:0≤s+r≤1,r>rnp,p≤M}. |
Condition Rs=Rr : In this case c(r)=0, which implies that p± are reduced to real value functions
p±(r)=−b(r)2±|b(r)2|. | (4.14) |
Newly,
1. If r<rnp, then b(r)<0 which implies that p+(r)=−b(r) is a positive and increasing linear function, and p−(r)=0. Since p−(0)=0 and p+(0)=−b(0)>0, then the functions p− and p∗ intersect at r=0 and the functions p+ and p∗ intersect at r+∈(0,1) if and only if p+(1)<p∗(1). In consequence, the equation p−(r)=p∗(r) does not have positive solutions, and the equation p+(r)=p∗(r) only has a positive solution r+ if and only if p+(1)<p∗(1). Therefore, if Rs=Rr and p+(1)<p∗(1) there exists an equilibrium point x+=(s+,r+,p+) in the subset Ω1⊂Ω given by
Ω1={(s,r,p)∈R3+:0≤s+r≤1,r<rnp,p≤M}. |
2. If r=rnp, then b(r)=0 which implies that p+(r)=p−(r)=0. In consequence, there are not positive solutions of the equations p+(r)=p∗(r) and p−(r)=p∗(r). Therefore, there are not coexistence equilibria.
3. If r>rnp, then b(r)>0 which implies that p−(r)=−b(r)<0, and p+(r)=0. Therefore, there are not coexistence equilibria.
Condition Rs>Rr : In this case c(r)>0, which implies that p± could be real or complex value functions. Therefore, the values of p± depend on of the sign of b(r) and the sign of
[b(r)2]2−c(r)=[βs−βr2δ(r−rnp)]2−(1Rr−1Rs)βsβrδ2r=(βs−βr2δ)2(r2−2k1r+r2np), | (4.15) |
where
k1=rnp+2(1Rr−1Rs)βsβr(βs−βr)2. |
Eq (4.15) is equivalent to
[b(r)2]2−c(r)=(βs−βr2δ)2(r−rr)(r−rl), | (4.16) |
where
rr=k1+√k21−r2nprl=k1−√k21−r2np. |
Observe that 0<rl<rnp<rr. In consequence
[b(r)2]2−c(r){>0,r∈(0,rl)∪(rr,∞);=0,r=rl or r=rr;<0,r∈(rl,rr). |
Now, since the sign of b(r) depends on rnp we have the following options.
● For r∈(0,rl)∪(rr,∞) we have:
1. If r<rnp, then b(r)<0 which implies that p+ is a positive and increasing concave function (p+(r)>0), and p− is a positive and decreasing convex function (p−(r)<0). Since p−(0)=(βs−βr)rnp/(2δ)>0 and p+(0)=3(βs−βr)rnp/(2δ)>0, then the functions p− and p∗ intersect at positive root r−>0 and the functions p+ and p∗ intersect at positive root r+>0, which satisfy r−<r+ (See Figure 2b). In consequence, if p+(1)<p∗(1) there are two coexistence equilibria x+ and x−, if p−(r−)<p∗(1)<p+(r+) there exists an unique equilibrium x−, and if p∗(1)>p−(r−) there are nor equilibria. Therefore, if Rs>Rr and p+(1)<p∗(1) there are two equilibrium points x+ and x− in the subset Ω′1⊂Ω1 given by
Ω′1={(s,r,p)∈R3+:0≤s+r≤1,r<rl,p≤M}. |
If Rs>Rr and p−(r−)<p∗(1)<p+(r+) there exists an unique equilibrium x−∈Ω′1.
2. If r=rnp, then b(r)=0 which implies that p+(r)=√−c(r) is a positive and increasing concave function, and p−(r)=−√−c(r) is a negative and decreasing convex function. Following a procedure similar to the previous cases, we conclude that if Rs>Rr and p+(1)<p∗(1) there exists an unique equilibrium point x+ in the subset Ω′1⋂Ω2⊂Ω1.
3. If r>rnp, then b(r)>0 which implies that p−(r)<0, and p+(r)<0. Therefore, there are not coexistence equilibria.
● For r=rl or r=rr we have p±(r)=−b(r)/2. In consequence, if r<rnp, then b(r)<0 which implies that p± is a positive and increasing linear function. Therefore, if Rs>Rr and p±(1)<p∗(1) there is an unique equilibrium x+ in the subset Ω″1⋂Ω1⊂Ω1 given by
Ω″1={(s,r,p)∈R3+:0≤s+r≤1,r=rl,p≤M}. |
We can verify, that if r≥rnp there are not coexistence equilibria.
● For r∈(rl,rr), is follows that p± are complex value functions. Therefore, there are not coexistence equilibria.
The existence results of coexistence equilibrium points are summarized in the following proposition.
Proposition 4.2. By assuming that the functions p∗ defined in the hypothesis C1 and p+ defined in (4.12) satisfy p+(1)<p∗(1), Rs>1 and Rr>1 we have the following results:
1. If Rs<Rr, there exists an equilibrium x+∈Ω.
2. If Rs=Rr, there exists an equilibrium x+∈Ω1.
3. If Rs>Rr then
● there are two equilibrium points x+ and x− in Ω′1⊂Ω1.
● there exists an equilibrium point x+ in the subset Ω′1∩Ω2⊂Ω1.
● there is an equilibrium x+ in the subset Ω″1∩Ω1⊂Ω1.
On the other hand, If Rs>Rr and p−(r−)<p∗(1)<p+(r+) there exists an unique equilibrium x−∈Ω′1.
Figure 3 shows the existence region of the equilibria. The equilibrium x0 represents the state without bacterial load, x1 represents the state of resistant bacteria, x+ represents the coexistence state with high bacterial load, and x− represents the coexistence state with low bacterial load.
In this section, we determine the stability of the equilibrium points of the system (2.2). The linearization of the system (2.2) around of an equilibrium point ˉx is given by x′=J(ˉx)x, where the Jacobian matrix J evaluated at x is given by
J(x)=A+Dh(x)=A+(−βs(2s+r)−δp−βss−δs−βrr´++δp−βr(s+2r)δs0∂g∂r∂g∂p). | (5.1) |
The eigenvalues of J(0) are given by ∂g(0,0)/∂p−μp, (γ+μr)(Rr−1) and (α+γ+μs)(Rs−1) which are negative if and only if Rs<1, Rr<1 and ∂g(0,0)/∂p<μp. In consequence, x0 is locally asymptotically stable (l.a.s). Similarly, the eigenvalue of J(x1) are −βrr1, βs(1/Rr−1/Rs−δp1/βs) and ∂g(r1,p1)/∂p−μp. In consequence, it is verified that x1 is l.a.s when Rs<(1+δp1/(α+γ+μs))Rr and ∂g(r1,p1)/∂p<μp.
The Jacobian J evaluated x± is given by
J(x±)=[−βss±−βss±−δs±−βrr±+δp±−(βrr±+δp±s±r±)δs±0∂g∂r∂g∂p−μp]. | (5.2) |
The characteristic polynomial of J(x±) is given by
p(λ)=λ3+a1λ2+a2λ+a3, | (5.3) |
where
a1=−(∂g∂p−μp)+βrr±+δp±s±r±+βss±a2=−(∂g∂p−μp)(βrr±+δp±s±r±+βss±)+(1+s±r±)βss±δp±+δs±∂g∂ra3=−(∂g∂p−μp)(1+s±r±)βss±δp±−δs±∂g∂r(βss±+βrr±−δp±). | (5.4) |
The Routh-Hurwitz criterium establish that the roots of a polynomial p(λ) have negative real part if and only if their coefficients satisfy D1=a1>0, D2=a1a2−a3 and a3>0. Now, D3 is rewritten as
D2=a1a2−a3=(∂g∂p−μp)2(βrr±+δp±s±r±+βss±)−(∂g∂p−μp)(βrr±+δp±s±r±+βss±)2+(1+s±r±)βss±δp±(βrr±+δp±s±r±+βss±)−[−(∂g∂p−μp)+(1+s±r±)δp±]δs±∂g∂r. | (5.5) |
If
∂g∂r(r±,p±)<0and∂g∂p(r±,p±)<μp, | (5.6) |
then a1 defined in (5.4) and D3 defined in (5.5) are positive, and since p±≤M then a3 defined in the second equation of (4.1) satisfies
a3>−(∂g∂p−μp)(1+s±r±)βss±δp±−δs±∂g∂r(βss±+βrr±−δM). | (5.7) |
From (5.7), for δ small enough we have δM≪1 which implies a3>0. Therefore, if (5.6) holds then x+ and x− are l.a.s. The results about local stability are summarized in the following proposition.
Proposition 5.1. If Rs<1, Rr<1 and ∂g(0,0)/∂p<μp, then x0 is l.a.s in Ω. If Rr>1 and Rs<(1+δp1/(α+γ+μs))Rr and ∂g(r1,p1)/∂p<μp, then x1 is l.a.s in Ω. If g satisfies the conditions (5.6), δ≪1 and under hypothesis of Proposition 4.2 it follows that x+ is l.a.s in Ω and x− is l.a.s in Ω′1.
Empirically, the existence of a control system for plasmid replication that depends on the genetics, molecular biology and physiology of both plasmids and host cells has been demonstrated. Much is still unknown about the mechanisms underlying this theory. However, the first evidence that plasmids control, at least partially, their own replication rate was the isolation of copy mutants, which have a higher steady-state copy number than the wild-type parent. Under steady state conditions, the plasmid concentration is constant and therefore the size of the plasmid population must double every time the cell population doubles. This means that each plasmid molecule must, on average, replicate once per cell and cell cycle. In our model, this property was analyzed by means of the gradient vector of the function that defines the plasmids replication rate. Specifically, for the stability of the equilibria x0 and x1 it is required that ∂g(0,0)/∂p<μp and ∂g(r1,p1)/∂p<μp, respectively. For the equilibria x± is required that g satisfies the conditions (5.6). As we can see, to control the replication of plasmids in the cases of equilibrium points x0 and x1, it is necessary to limit the variation of the plasmid replication rate with respect to plasmids. In the case of x±, in addition to the above, it is necessary that the plasmid replication rate be decreasing with respect to resistant bacteria.
The global stability of x0 is presented in the following proposition
Proposition 5.2. If Rs≤1 and Rr≤1, then x0 is globally asymptotically stable in Ω defined in (3.8).
Proof. Since g∈C1(Ω), then there exists L≥0 such that δμpβr∫t0g(x(τ))dτ=L. The function V defined by
V(x)=1βss+1βrr+δμpβrp+L−δμpβr∫t0g(x(τ))dτ, |
satisfies V(x0)=0 and V(x)≥0 for all x∈Ω. The orbital derivative of V is given by
˙V=1βsdsdt+1βrdrdt+δμpβrdpdt−δμpβrddt(∫t0g(x(τ))dτ)=(s+r)(1−(s+r))−δpsβs+δpsβr−δpβr−(1Rss+1Rrr). |
For Rs≤1 and Rr≤1 we obtain
˙V≤(s+r)(1−(s+r))−δpsβs+δpsβr−δpβr−(s+r)=−(s+r)2−δpsβs−δpβr(1−s)<0. |
In consequence ˙V(x)<0 for all x∈Ω. Therefore, x0 is globally asymptotically stable in Ω.
In the last section, we verified that the equilibria x± are l.a.s when the conditions defined in (5.6) are satisfied. In this section, we will verify that if
∂g∂r(r±,p±)>0and∂g∂p(r±,p±)<μp, |
then there exists a Hopf bifurcation for a suitable parameter of system (2.2). In this case, a change of stability of x+ or x− occur, and a limit cycle appears with amplitude and frequency depending on the value of the bifurcation parameter. To this end, we will use the following version of the Hopf theorem
Theorem 6.1 (Hopf Theorem). Let n-dimensional autonomous system of differential equation given by
˙x=F(x,μ), | (6.1) |
which depends on the real parameter μ, and where F(x,u) is twice differentiable in both variables. We suppose that
1. The system (6.1) possess an analytic family x(μ) of equilibrium points; that is F(x(μ),μ)=0.
2. For certain value of μ, say μ0, the jacobian matrix J(x(μ0),μ0)=Fx(x(μ0),μ0) has two purely imaginary eigenvalues λ±(μ0)=±iβ and no other eigenvalue of J(x(μ0),μ0) is an integral multiple of iβ.
3. If λ(μ)=α(μ)+iβ(μ) is the continuation of eigenvalue iβ, then α′(μ0)=d(Re(λ)(μ0))/dμ≠0.
Under the above conditions there exists differentiable functions μ(ϵ) and T(ϵ) depending on a parameter ϵ with μ0 and T(0)=2πβ−1 such that there are nonconstant periodic solutions x(t,ϵ) of (6.1) with period T(ϵ) which collapse into x(μ) as ϵ→0.
See Schmidt [26] for a prove of Theorem 6.1. From (3.1) we observe that μ is one of the following parameters βs, βr, α, δ, γ, σp, μs,μr, or μp, and F(x,μ)=A(μ)x+h(x,μ). In the Proposition 5.1 we proved the existence of equilibriium x+ which depends of μ, and satisfies F(x+,μ)=0. Consequently the first item of the Theorem 6.1 is satisfied. For convenience we choose the bifurcation parameter μ=βs, which implies x(μ)=x+(βs).
Now, we will prove that the Jacobian matrix at x+(βs) has a negative real eigenvalue and a pair of eigenvalues on the imaginary axis. To this end, we define
ξ=βss+βrr+δpsr. |
In term of the parameter ξ, the constants a1, a2 and a3 defined in (5.4) are rewritten as
a1=−(∂g∂p−μp)+Aa2=−(∂g∂p−μp)A+(1+sr)βssδp−δs∂g∂ra3=−(∂g∂p−μp)(1+sr)βssδp−δs∂g∂r(ξ−δp(1+sr)). |
Now, D2 in terms of ξ is given by
D2(ξ)=c1ξ2+c2ξ+c3, | (6.2) |
where
c1=−(∂g∂p−μp)c2=(∂g∂p−μp)2+(1+sr)βssδpc3=[∂g∂p−μp−(1+sr)δp]δs∂g∂r. |
Observe that D2(ξ) defined in (6.2) can be rewritten as D2(ξ)=(ξ−ξ+(βs))(ξ−ξ−(βs)) where
ξ±(βs)=−c2(βs)±√(c2(βs))2−4c1(βs)c3(βs)2c1(βs). | (6.3) |
Observe that c2>0, if ∂g/∂p<μp then c1>0, in addition, if ∂g/∂r>0 then c3<0, which implies that ξ+ is the only positive solution of D2(ξ)=0. If ∂g/∂p=μp, then c1=0 and c3<0, then the solution of D2(ξ)=0 is ξ=−c3/c2>0. In consequence, for ξ=ξ+(βs) or ξ=−c3(βs)/c2(βs) we obtain D2(ξ)=0 which implies a1(βs)a(βs)=a3(βs) for some βs=β0s. By substituting the previous equation in p1(λ) we obtain
λ3+a1(β0s)λ2+a2(β0s)λ+a1(β0s)a2(β0s)=0(λ+a1(β0s))(λ2+a2(β0s))=0. | (6.4) |
From (6.4) we establish that the eigenvalues of J(x+) are −a1(β0s) and λ±(β0s)=±√−a2(β0s). Since a1(β0s)>0 and a2(β0s)>0 then −a1(β0s) is a negative real number and λ±(β0s)=±i√a2(β0s) are imaginary numbers which implies that the second item of the Theorem 6.1 is satisfied.
Since s, r and p implicitly depend on all parameters of mathematical model (2.2), then the constants a1, a2 and a3 defined in (5.4) implicitly depend on the same parameters. In consequence, the calculation of the crossing speed is cumbersome, no matter which bifurcation parameter is chosen. From Lemma 8.1 (appendix), it is verified the existence of an unique function v:R7→R such that βs=v(βr,α,δ,γ,μs,μr,μp). On the other hand, since the eigenvalues of Jacobian matrix defined in (5.2) are given by −a1(β0s) and λ±(β0s)=±iβ(β0s) where β(β0s)=√a2(β0s), then using the canonical form theory of Jordan (See Hirsch and Smale[27]) we obtain
J(x(β0s),β0s)=DF(x(β0s),β0s)∼(0−β00β00000−a01), |
with β0=β(β0s) and a01=a1(β0s). Suppose that for βs≈β0s we have
λ+=ˉα(βs)+iβ(βs)λ−=ˉα(βs)−iβ(βs), |
being ˉα(β0s)=0 and β(β0s)=β0. Since we wish to calculate
ˉα′(βs)=ddβs[Re(λ(βs))]|βs=β0s. |
which is the crossing speed of the eigenvalues λ±(βs) in the imaginary axis. For βs≈β0s the characteristic polynomial associated with the Jacobian matrix
Jβs=DF(x(βs),βs)=(ˉα(βs)−β(βs)0β(βs)ˉα(βs)000−a1(βs)), | (6.5) |
is
Pβs(λ)=det(λI−Jβs)=λ3+L1(βs)λ2+L2(βs)λ+L3(βs), |
where
L1(βs)=a1(βs)−2ˉα(βs)L2(βs)=−2ˉα(βs)a1(βs)+ˉα(βs)2+β(βs)2L3(βs)=a1(βs)[ˉα(βs)2+β(βs)2]. | (6.6) |
Since the coefficients L1, L2 and L3 must satisfy the same conditions that the coefficients a1, a2 and a3 of the characteristic equation of J(x(σp)); that is, L3(βs)−L1(βs)L2(βs)=0. Substituting (6.6) in above equation we obtain the followin equation
2ˉα(μ)[(a1(μ)+ˉα(μ))2+β(μ)2]=0. | (6.7) |
For ˉα(μ)≠0, then (6.7) is rewritten as
(a1(μ)+ˉα(μ))2+β(μ)2=0. | (6.8) |
Applying implicit differentiation in (6.8) with respect to βs we obtain
ˉα′(β0s)==−[β(βs)a1(βs)+ˉα(βs)dβdβs+da1dβs]=−12(a1(βs)+ˉα(βs))[(β(βs)2+a1(βs)2)′+ˉα(βs)a1(βs)(a1(βs)2)′]. | (6.9) |
Evaluating (6.9) in βs=β0s we obtain
ˉα′(β0s)=−12a01(β(βs)2+a1(βs)2)′(β0s)=−[β02a01√a2(β0s)a′2(β0s)+a′1(β0s)]=−[12a01a′2(β0s)+a′1(β0s)]=−12a01(1+sr)β0ssδp≠0. | (6.10) |
In consequence, there exits a a Hopf bifurcation to the system (2.2) in the endemic equilibrium x(βs)=x+. Similar way, we verify the existence of a Hopf bifurcation around x−.
Currently, plasmid-mediated resistance is a threat to humanity. One of the key factors in tackling this challenge is to understand the replication dynamics of resistance plasmids. However, the lack of knowledge about the biology of plasmids makes this task difficult. In this regard, efforts by researchers in different fields have clarified some aspects of plasmids. The replication rate has been modeled as a Holling type II functional response [28]. However, it is still unclear which functional responses best fit the relevant rates. In this work, we studied the functional response of plasmid replication on antibiotic resistance. Thus, we modeled the competitive interaction between plasmids, sensitive and resistant bacteria with a system of three nonlinear ordinary differential equations. We used a function g∈C1(R2) for the plasmid replication rate.
We proved the existence of bounded solutions of system (2.2) in the set Ω defined in (3.8). Qualitative analysis was made under hypothesis C1; the equation g(r,p)=μpp has unique non-negative solution p∗(r) which is a increasing convex function that satisfies p∗(0)=0. The system (2.2) always has the bacteria-free equilibrium, x0≡0∈Ω, if Rr>1 there exists a resistant bacteria equilibrium x1∈Ω where Rr represents the number of bacteria produced by the fraction of resistant bacteria that evade the immune response, the existence results of coexistence equilibrium points, x±, are summarized in the Proposition 4.2. This proposition explicitly determines the regions of existence of x±. For example, if Rs>Rr then the equilibria x+ and x− coexist in the subset Ω′1⊂Ω, where Rs represents the number of bacteria generated by the fraction of sensitive bacteria that survive the effects due to antibiotics and the immune system response, x+ and x− are associated with high and low bacterial load, respectively. If Rs<1, Rr<1 and ∂g(0,0)/∂p<μp, then x0 is l.a.s. In addition, we proved that x0 is globally asymptotically stable when Rs≤1 and Rr≤1. In consequence, if each bacterium (sensitive or resistant) produces at most one new bacteria, then the infection will be controlled or eliminated. If Rr>1, Rs<(1+δp1/(α+γ+μs))Rr and ∂g(r1,p1)/∂p<μp, then x1 is l.a.s in Ω. In this scenario, the infection is caused only by resistant bacteria. For δ≪1 and under hypothesis of Proposition 4.2 we proved that x+ is l.a.s in Ω and x− is l.a.s in Ω′1. Furthermore, we verified the existence of a Hopf bifurcation when βs≪1, ∂g(r+,p+)/∂p<μp and ∂g(r+,p+)/∂r>0. In this cases, when x+ loses its stability, a stable limit cycle appears. The growth of plasmids, sensitive and resistant bacteria have an oscillatory behavior with initial period T=2πβ−1S.
The analysis of the model shows three possible scenarios in the outcome of the infection; bacterial progression is cleared, persists only with resistant bacteria, or persists with both of them. The only equilibrium point in the region
U1=Ω×{(Rs,Rr)∈R2:0<Rs≤1,0<Rr≤1}, |
is x0. Since x0 is globally asymptotically stable in U1, then regardless of the initial conditions or the plasmid replication rate, the bacterial progression is always eliminated in U1. The region
U2=Ω×{(Rs,Rr)∈R2:0<Rs≤1,Rr>1}, |
contains the equilibria x0 and x1. The point x0 is unstable and x1 is l.a.s when tha plasmid replication rate satisfies ∂g(rr,p1)/∂p<μp. Therefore, in the subregion W1⊂U2 defined by
W1=Ω×{(Rs,Rr)∈R2:0<Rs≤1,Rr>1,∂g∂p(r1,p1)<μp}, |
x0 is unstable and x1 is l.a.s, which implies that the infection tends to spread only with resistant bacteria. For Rs>1 and Rr>1 there are several regions. In the region
U3=Ω×{(Rs,Rr)∈R2:Rs>1,Rr>1,Rs<Rr,p+(1)<p+(1),Rs<(1+δp1(α+γ+μs))Rr,∂g∂p(rr,p1)<μp}, |
the equilibrium x0 is unstable, x+ and x1 are l.a.s. In consequence, U3 is a region of bi-stability in which infection progresses with resistant bacteria or with high levels of both bacterial populations. In the region
U4=Ω×{(Rs,Rr)∈R2:Rs>1,Rr>1,Rs<Rr,p+(1)>p+(1),Rs<(1+δp1(α+γ+μs))Rr,∂g∂p(rr,p1)<μp}, |
the equilibrium x0 is unstable x1 is l.a.s and x+ does not exist. Then, in U4 the bacterial progression is only with resistant bacteria. In the regions
U5=Ω×{(Rs,Rr)∈R2:Rs>1,Rr>1,Rs<Rr,p+(1)<p+(1),Rs>(1+δp1(α+γ+μs))Rror∂g∂p(rr,p1)>μp}U6=Ω×{(Rs,Rr)∈R2:Rs>1,Rr>1,Rs=Rr}U7=(Ω′1∩Ω2)×{(Rs,Rr)∈R2:Rs>1,Rr>1,Rs>Rr}U8=(Ω′1∩Ω1)×{(Rs,Rr)∈R2:Rs>1,Rr>1,Rs>Rr}, |
the equilibria x0 and x1 are unstable x+ is l.a.s. Then, in the regions U5−U8 the infection progresses with high levels of both bacterial populations. In the region
U9=Ω′1×{(Rs,Rr)∈R2:Rs>1,Rr>1,Rs>Rr}, |
the equilibria x0 and x1 are unstable, x+ and x− are l.a.s, which means that the infection progresses with high and low levels of both bacterial populations. In the region
U10=Ω′1×{(Rs,Rr)∈R2:Rs>1,Rr>1,∂g∂p(r+,p+)<μp,∂g∂r(r+,p+)>0}, |
the equilibrium x0 and x+ are unstable, and there are limit cycles.
The results of the analysis of the model (2.2) confirm that the form of replication of bacterial plasmids plays a fundamental role in PMAR. Under hypothesis C1 and additional conditions of ∂g/∂p and ∂g/∂r we obtained results similar to those presented in [16]. However, since the number of equilibrium solutions of (2.2) depends on the number of solutions of the equations defined in (4.13) we can modify C1 to obtain new scenarios on the set of equilibrium points. For the following hypothesis
C2: the equation g(r,p)=μpp has unique non-negative solution p∗(r) such that the equations p+(r)=p∗(r) and p−(r)=p∗(r) have n and m solutions in (0,1), respectively.
we have the following existence results for the coexistence equilibria.
Proposition 7.1. By assuming that the hypothesis C2 is satisfied, Rs>1 and Rr>1 we have the following results:
1. If Rs<Rr, then there are n coexistence equilibria x+1,…,x+n∈Ω.
2. If Rs=Rr, there are n coexistence equilibria x+1,…,x+n∈Ω1.
3. If Rs>Rr, then
● there are n+m coexistence equilibria x+1,…,x+n and x−1,…,x−m in Ω′1⊂Ω1.
● there are n coexistence equilibria x+1,…,x+n∈Ω′1∩Ω2⊂Ω1.
● there are n coexistence equilibria x+1,…,x+n∈Ω″1∩Ω1⊂Ω1.
Proof. The same procedure carried out to prove the Proposition 4.2.
The stability results for the coexistence equilibria of Proposition 7.1 are summarized in the following proposition.
Proposition 7.2. If g satisfies the conditions (5.6), δ≪1 and under hypothesis of Proposition 7.1 it follows that x+1,…,x+n are l.a.s in Ω and x−1,…,x−m are l.a.s in Ω′1.
Proof. The same procedure carried out to prove the Proposition 5.1.
Now, under following hypothesis,
C3: the equation g(r,p)=μpp has unique non-negative solution p∗(r) such that the equations p±(r)=p∗(r) have an infinite countable number of equilibrium solutions {ri}∞i=1∈(0,1).
It is follows the next proposition.
Proposition 7.3. By assuming that the hypothesis C3 is satisfied, Rs>1 and Rr>1, then there are an infinite countable number of non isolated equilibrium points {xi}∞i=1⊂Ω and a isolated equilibrium point ˉx∈Ω of system (2.2) such that xi→ˉx when i→∞. In addition,
1. If Rs<Rr, then {xi}∞i=1∈Ω and ˉx∈Ω.
2. If Rs=Rr, then {xi}∞i=1∈Ω1 and ˉx∈Ω1.
3. If Rs>Rr, then
● {xi}∞i=1∈Ω′1⊂Ω1 and ˉx∈Ω′1⊂Ω1.
● {xi}∞i=1∈Ω′1∩Ω2⊂Ω1 and ˉx∈Ω′1∩Ω2⊂Ω1.
● {xi}∞i=1∈Ω″1∩Ω1⊂Ω1 and ˉx∈Ω″1∩Ω1⊂Ω1.
Proof. If we assume that the Eq (4.13) has an infinite countable set of solutions {ri}∞i=1∈(0,1), then there exits ˉr∈[0,1] such that ri→ˉr when i→∞. Therefore, the system (2.2) have an infinite countable set of equilibrium solutions {xi}∞i=1 such that xi→ˉx when i→∞, where ˉx is an equilibrium point of system (2.2). Following a similar idea, we could obtain continuous curves of non-isolated equilibria of (2.2).
The elimination of the bacterial population is the most relevant scenario to the host. In this regard, the qualitative analysis reveals that to reach this objective in a neighborhood of x0, N(x0), it is necessary that the surviving bacteria of both the effect of antibiotic and the immune response do not generate new bacteria, and the variation of the plasmid replication rate respect to plasmids would be less than its degradation rate. While eliminating the bacterial population in Ω, it is required only that each bacterium mentioned above generates at most one new bacterium. Here, the plasmid replication rate plays no role. These results suggest that within the host plasmid replication produces local effects on the outcome of infection. Now, when each surviving bacterium generates more than one bacterium, different types of bacterial spread occur, and although the effect of plasmid replication remains local, the plasmid replication rate is essential to determine the type of spread.
The authors acknowledges support from projects No 106-25/09/2018 (1678) and No.118-25/09/2018 (1669) financed by Vicerectoría de Investigaciones e Interacción Social (VIIS)-Universidad de Nariño.
The authors declare that they have no competing interests.
Lemma 8.1. There exists an unique function v:R7→R that satisfies βs=v(βr,α,δ,γ,μs,μr,μp).
Proof. Let D2(z,βS)=a2(z,βs)a1(z,βs)−a3(z,βs) where z=(βr,α,δ,γ,μs,μr,μp), then we had verified that D2(z0,β0s)=0 where z0 is the vector corresponding to the parameter β0s. In addition, from (5.5) we verify
∂D2∂βs(z0,β0s)=[∂g∂p−μp−(βrr+δpsr+β0ss)]2s+(βrr+δpsr+β0ss)(δp−βrr−β0ss)s+(1+sr)β0ssδps. |
Observe that ∂D2(z0,β0s)/∂βs≠0 for β0s≪1. In consequence, the Implicit function theorem implies the existence of an open ball U∈R7 containing z0 and an interval V⊂R containing β0s such that there is an unique function βs=v(z) defined for z∈U and βs∈V which satisfies F(x(β0s),β0s)=0, where F is the right side of the system (2.2) [29].
[1] | H. Lodish, A. Berk, P. Matsudaira, C. A. Kaiser, M. Krieger, M. P. Scott, et al., Biología Celular y Molecular, Editorial Médica Panamericana, Bogotá, 2016. |
[2] |
L. A. Actis, M. E. Tolmasky, J. H. Crosa, Bacterial plasmids: Replication of extrachromosomal genetic elements encoding resistance to antimicrobial compounds, Front. Biosci., 4 (1999), 43–62. doi: 10.2741/Actis
![]() |
[3] |
R. X. Wang, J. Y. Wang, Y. C. Sun, B. L. Yang, A. L. Wang, Antibiotic resistance monitoring in Vibrio spp. isolated from rearing environment and intestines of abalone Haliotis diversicolor, Mar. Pollut. Bull., 101 (2015), 701–706. doi: 10.1016/j.marpolbul.2015.10.027
![]() |
[4] |
M. Zhou, Y. Xu, P. Ouyang, J. Ling, Q. Cai, L. Huang, et al., Evolution and distribution of resistance genes and bacterial community in water and biofilm of a simulated fish-duck integrated pond with stress, Chemosphere, 245 (2020), 125549. doi: 10.1016/j.chemosphere.2019.125549
![]() |
[5] | C. Schultsz, S. Geerlings, Plasmid-mediated resistance in Enterobacteriaceae, Drugs, 72 (2012), 1–16. |
[6] | M. S. Ramirez, G. M. Traglia, D. L. Lin, T. Tran, M. E. Tolmasky, Plasmid‐mediated antibiotic resistance and virulence in gram‐negatives: The Klebsiella pneumoniae paradigm, Microbiol. Spectr., 2 (2014), 1–15. |
[7] |
B. Li, F. Yin, X. Zhao, Y. Guo, W. Wang, P. Wang, et al., Colistin resistance gene mcr-1 mediates cell permeability and resistance to hydrophobic antibiotics, Front. Microbiol., 10 (2020), 1–7. doi: 10.3389/fcimb.2020.00001
![]() |
[8] |
L. G. Burman, R-plasmid transfer and its response to nalidixic acid, J. Bacteriol., 131 (1977), 76–81. doi: 10.1128/jb.131.1.76-81.1977
![]() |
[9] | E. A. Yurtsev, H. X. Chao, M. S. Datta, T. Artemova, J. Gore, Bacterial cheating drives the population dynamics of cooperative antibiotic resistance plasmids, Mol. Syst. Biol., 9 (2013), 1–7. |
[10] |
D. Godwin, J. H. Slater, The influence of the growth environment on the stability of a drug resistance plasmid in Escherichia coli K12, J. Gen. Microbiol., 111 (1979), 201–210. doi: 10.1099/00221287-111-1-201
![]() |
[11] |
B. Daşbaşı, İ. Öztürk, Mathematical modelling of bacterial resistance to multiple antibiotics and immune system response, Springerplus, 5 (2016), 1–17. doi: 10.1186/s40064-015-1659-2
![]() |
[12] | M. Merdan, Z. Bekiryazici, T. Kesemen, T. Khaniyev, Comparison of stochastic and random models for bacterial resistance, Adv. Differ. Equations, 133 (2017), 1–19. |
[13] | Q. J. Leclerc, J. A. Lindsay, G. M. Knight, Mathematical modelling to study the horizontal transfer of antimicrobial resistance genes in bacteria: Current state of the field and recommendations, J. R. Soc. Interface., 16 (2018), 1–10. |
[14] |
J. N. Tetteh, F. Matthüs, E. A. Hernandez-Vargas, A survey of within-host and between-hosts modelling for antibiotic resistance, Biosystems, 196 (2020), 1–13. doi: 10.1016/j.biosystemseng.2020.05.010
![]() |
[15] |
E. Ibargüen-Mondragón, J. P. Romero-Leiton, L. Esteva, E. M. Burbano-Rosero, Mathematical modeling of bacterial resistance to antibiotics by mutations and plasmids, J. Biol. Syst., 24 (2016), 129–146. doi: 10.1142/S0218339016500078
![]() |
[16] |
E. Ibarguen-Mondragon, J. P. Romero-Leiton, L. Esteva, M. C. Gomez, S. P. Hidalgo-Bonilla, Stability and periodic solutions for a model of bacterial resistance to antibiotics caused by mutations and plasmids, Appl. Math. Model., 76 (2019), 238–251. doi: 10.1016/j.apm.2019.06.017
![]() |
[17] |
C. S. Hayes, D. A. Low, Signals of growth regulation in bacteria, Curr. Opin. Microbiol., 12 (2009), 667–673. doi: 10.1016/j.mib.2009.09.006
![]() |
[18] | K. N. Timmis, M. I. Gonzalez-Carrero, T. Sekizaki, F. Rojo, Biological activities specified by antibiotic resistance plasmids, J. Antimicrob. Chemother., 18 (1986), 1–12. |
[19] | T. Wein, F. T. Stücker, N. F. Hülter, T. Dagan, Quantification of plasmid-mediated antibiotic resistance in an experimental evolution approach, J. Vis. Exp., 154 (2019), 1–7. |
[20] | E. Ibarguen-Mondragon, K. Prieto, S. P. Hidalgo-Bonilla, A model on bacterial resistance considering a generalized law of mass action for plasmid replication, J. Biol. Syst., 28 (2021), 1–38. |
[21] |
E. Ibargüen-Mondragón, L. Esteva, J. P. Romero-Leiton, S. P. Hidalgo-Bonilla, E. M. Burbano-Rosero, M. Cerón, et al., Mathematical modeling on bacterial resistance to multiple antibiotics caused by spontaneus mutations, Biosystems, 117 (2014), 60–67. doi: 10.1016/j.biosystems.2014.01.005
![]() |
[22] | J. P. Romero-Leiton, E. Ibargüen-Mondragón, Sobre la resistencia bacteriana a antibióticos de acción bactericida y bacteriostática, Rev. Integración, 32 (2014), 101–116. |
[23] |
K. Nordström, S. Molin, J. Light, Control of replication of bacterial plasmids: Genetics, molecular biology, and physiology of the plasmid R1 system, Plasmid, 12 (1984), 71–90. doi: 10.1016/0147-619X(84)90054-4
![]() |
[24] | L. Perko, Differential Equations and Dynamical Systems, 2 Eds, New Yorks: Springer Science & Business Media, 2013. |
[25] |
W. Karpińska, On bounded solutions of nonlinear first and second order equations with a Carathéodory function, J. Math. Anal. Appl., 334 (2007), 1462–1480. doi: 10.1016/j.jmaa.2007.01.037
![]() |
[26] |
D. S. Schmidt, Hopf's bifurcation theorem and the center theorem of Liapunov with resonance cases, J. Math. Anal. Appl., 63 (1978), 354–370. doi: 10.1016/0022-247X(78)90081-1
![]() |
[27] | M. W. Hisrch, S. Smale, Differential Equations, Dynamical Systems and Linear Algebra, 2 Eds, New York: Academic Press, 1974. |
[28] |
V. N. Satyagal, P. Agrawal, A generalized model of plasmid replication, Biotechnol. Bioeng., 33 (1989), 1135–1144. doi: 10.1002/bit.260330909
![]() |
[29] | T. M. Apostol, Mathematical Analysis, Massachusetts: Addison-Wesley, 1960. |
1. | Bahatdin Daşbaşı, Fractional order bacterial infection model with effects of anti-virulence drug and antibiotic, 2023, 170, 09600779, 113331, 10.1016/j.chaos.2023.113331 | |
2. | Eduardo Ibargüen-Mondragón, M. Victoria Otero-Espinar, Miller Cerón Gómez, On the contribution of qualitative analysis in mathematical modeling of plasmid-mediated ceftiofur resistance, 2023, 31, 2688-1594, 6673, 10.3934/era.2023337 | |
3. | Edgar Alberto Vega Noguera, Simeón Casanova Trujillo, Eduardo Ibargüen-Mondragón, A within-host model on the interactions of sensitive and resistant Helicobacter pylori to antibiotic therapy considering immune response, 2025, 22, 1551-0018, 185, 10.3934/mbe.2025009 | |
4. | Zienab Ali, Karim Abdelkader, Maha M. Abdel-Fattah, Ahmed Farag Azmy, Ahmed O. El-Gendy, Tarek Dishisha, Therapeutic potential of a newly isolated bacteriophage against multi-drug resistant Enterococcus faecalis infections: in vitro and in vivo characterization, 2025, 25, 1471-2180, 10.1186/s12866-025-03785-z |