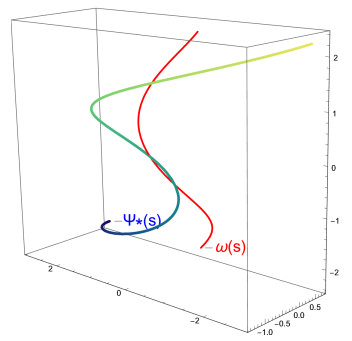
In this paper, we consider the statistical inferences for varying coefficient partially nonlinear model with missing responses. Firstly, we employ the profile nonlinear least squares estimation based on the weighted imputation method to estimate the unknown parameter and the nonparametric function, meanwhile the asymptotic normality of the resulting estimators is proved. Secondly, we consider empirical likelihood inferences based on the weighted imputation method for the unknown parameter and nonparametric function, and propose an empirical log-likelihood ratio function for the unknown parameter vector in the nonlinear function and a residual-adjusted empirical log-likelihood ratio function for the nonparametric component, meanwhile construct relevant confidence regions. Thirdly, the response mean estimation is also studied. In addition, simulation studies are conducted to examine the finite sample performance of our methods, and the empirical likelihood approach based on the weighted imputation method (IEL) is further applied to a real data example.
Citation: Liqi Xia, Xiuli Wang, Peixin Zhao, Yunquan Song. Empirical likelihood for varying coefficient partially nonlinear model with missing responses[J]. AIMS Mathematics, 2021, 6(7): 7125-7152. doi: 10.3934/math.2021418
[1] | Yasin Ünlütürk, Talat Körpınar, Muradiye Çimdiker . On k-type pseudo null slant helices due to the Bishop frame in Minkowski 3-space E13. AIMS Mathematics, 2020, 5(1): 286-299. doi: 10.3934/math.2020019 |
[2] | Ufuk Öztürk, Zeynep Büşra Alkan . Darboux helices in three dimensional Lie groups. AIMS Mathematics, 2020, 5(4): 3169-3181. doi: 10.3934/math.2020204 |
[3] | Zuhal Küçükarslan Yüzbașı, Dae Won Yoon . On geometry of isophote curves in Galilean space. AIMS Mathematics, 2021, 6(1): 66-76. doi: 10.3934/math.2021005 |
[4] | Esra Damar . Adjoint curves of special Smarandache curves with respect to Bishop frame. AIMS Mathematics, 2024, 9(12): 35355-35376. doi: 10.3934/math.20241680 |
[5] | Gülnur Şaffak Atalay . A new approach to special curved surface families according to modified orthogonal frame. AIMS Mathematics, 2024, 9(8): 20662-20676. doi: 10.3934/math.20241004 |
[6] | Kemal Eren, Hidayet Huda Kosal . Evolution of space curves and the special ruled surfaces with modified orthogonal frame. AIMS Mathematics, 2020, 5(3): 2027-2039. doi: 10.3934/math.2020134 |
[7] | Yanlin Li, Kemal Eren, Kebire Hilal Ayvacı, Soley Ersoy . Simultaneous characterizations of partner ruled surfaces using Flc frame. AIMS Mathematics, 2022, 7(11): 20213-20229. doi: 10.3934/math.20221106 |
[8] | Semra Kaya Nurkan, İlkay Arslan Güven . Construction of vectorial moments via direction curves. AIMS Mathematics, 2023, 8(6): 12857-12871. doi: 10.3934/math.2023648 |
[9] | Beyhan YILMAZ . Some curve pairs according to types of Bishop frame. AIMS Mathematics, 2021, 6(5): 4463-4473. doi: 10.3934/math.2021264 |
[10] | Bahar UYAR DÜLDÜL . On some new frames along a space curve and integral curves with Darboux q-vector fields in E3. AIMS Mathematics, 2024, 9(7): 17871-17885. doi: 10.3934/math.2024869 |
In this paper, we consider the statistical inferences for varying coefficient partially nonlinear model with missing responses. Firstly, we employ the profile nonlinear least squares estimation based on the weighted imputation method to estimate the unknown parameter and the nonparametric function, meanwhile the asymptotic normality of the resulting estimators is proved. Secondly, we consider empirical likelihood inferences based on the weighted imputation method for the unknown parameter and nonparametric function, and propose an empirical log-likelihood ratio function for the unknown parameter vector in the nonlinear function and a residual-adjusted empirical log-likelihood ratio function for the nonparametric component, meanwhile construct relevant confidence regions. Thirdly, the response mean estimation is also studied. In addition, simulation studies are conducted to examine the finite sample performance of our methods, and the empirical likelihood approach based on the weighted imputation method (IEL) is further applied to a real data example.
One of the most researched areas of differential geometry is curve theory, with studies presented in different dimensions and spaces. Generally, the geometric location of the different positions taken by a moving point in space during motion is a curve. In this motion, the parameter range of the curve represents the time elapsed through the motion. In addition, the parameter of the curve has an important place to characterize the curve. For example, the parameter of any given curve has many differences in terms of whether the curve is parameterized with respect to arc length. On the other hand, the characterization of a regular curve is one of the most important problems in curve studies in differential geometry, where curvature and torsion play a major role in solving the problem. The definitions of some special curves can be made by using the curvature and torsion [3,4,5,6,7,8,9,10,11]. One of them is the helices definition given by Lancret in 1806 [18]. Lancret defined the classical definition of helices as "curves whose tangent vector makes a constant angle with a constant direction" [18]. Starting from this definition, the helices drawn on a right circular cylinder were considered, and they were called a circular helix. It is among the first characterizations indicating that curvature and torsion are constants separately in these helices. Later, although these curvatures are not constant, curves with constant ratios were found, which were called the general helices, and the proof of this theory was proved by Venant in 1845 [11]. Helices have an impressive place both in nature and science. Fractal geometry, the arrangement of molecules in the structure of DNA, and its applications in physics and civil and mechanical engineering are some examples. In addition to that, Izumiya and Takeuchi named the curves slant helices, where the angle between the principal normal vector field and a constant direction is constant at every point of the curve, and they obtained that the necessary and sufficient condition for curves to be slant helices is that the geodesic curvature of the principal normals indicator of the given curves is a constant function [14]. Then, some studies about slant helices were conducted [2,24]. Ali and Lopez [1] studied slant helices in Lorenz space and investigated the tangent indicatrix on the binormal indicatrix of a slant helix in Minkowski space. On the other hand, Kula and Yaylı investigated the tangential and binormal indicatrices of slant helices and showed that these spherical indicatrices are spherical helices [17]. Later, Önder et al. introduced B2-slant helices in E4 and determined the properties of these curves [21]. In addition, Yaylı and Zıplar examined the relationship between slant helices and spherical helices in En space [26]. Then, Gök et al. defined Vn-slant helices in the space En [12], K\i z\i ltu\u{g} et al. [16] obtained the characterization of slant helices using a type-2 bishop frame and Lucas and Ortega-Yagües [20] investigated the relationship between helix surfaces and slant helices in a sphere S3. Recently, Uzuno\u{g}lu et al. [25] explained a recent alternative orthogonal frame as {N,C=N′‖N′‖,W} and gave a characterization of C-slant helices. Moreover, they investigated curves of C-constant precession according to an alternative frame [25]. After that, Kaya and Önder et al. [15] defined C-partner curve with respect to the frame {N,C,W}. On the other hand, the modified orthogonal frame has been defined by Sasai [23], and the helices and Bertrand curves for modified orthogonal frames in E3 have been given in [19].
In this section, we give some basic definitions of curves in Euclidean 3-space. Let α:I⊂R⟶E3 be a regular-unit speed curve with the arc-length parameter s (‖α′(s)‖=1). Therefore, the curve (α) is called a Frenet curve if it has non-zero curvature (α″(s)≠0). Moreover, the derivative formulas of the Frenet frame of a regular-unit speed curve can be given as
T′=κN,N′=−κT+τB,B′=−τN, |
where {T,N,B} constitutes the Frenet frame and κ=‖α″‖=⟨T′,N⟩; and, τ=⟨N′,B⟩ are the curvature and torsion of the unit speed curve (α), respectively. In addition to the Frenet frame, Uzunoǧlu et al. [25] explained a new alternative orthogonal frame such that {N,C,W}, where N is the principal normal vector field, C=N′‖N′‖, W=τT+κB√κ2+τ2 and W is the Darboux vector. Therefore, the derivative formulae of this alternative frame {N,C,W} are provided by
[N′C′W′]=[0f0−f0g0−g0][NCW], | (2.1) |
where
f=√κ2+τ2=κ√1+H2,g=κ2κ2+τ2(τκ)′, |
κ and τ are the curvature and torsion of the curve (α), respectively, and H is the harmonic curvature. Moreover, the relationship between the new alternative frame {N,C,W} and Frenet frame {T,N,B} are given as follows:
T=−κC+τW√κ2+τ2,B=τC+κW√κ2+τ2, |
where the vector field N is common [15].
Now, for a regular-unit speed curve α=α(s), we can say that, if the vector field T of the curve (α) makes a constant angle with a fixed-unit vector field, α=α(s) is called a general helix [11,18]. Moreover, a helix curve is characterized by κτ=constant, where κ and τ are the curvature and torsion of the curve (α), respectively [11]. Then, Izumiya and Takeuchi described a slant helix as "a curve α whose principal normal vector make a constant angle with such a constant direction, that is
⟨N,U⟩=cosθ |
for every s∈I and θ≠π2" [7]. At the same time, they also stated that (α) is a slant helix where the necessary and sufficient condition is that its curvatures satisfy the equation
κ2(κ2+τ2)32(τκ)′=constant. |
After that, Uzunoǧlu et al. [25] gave the following definition of a slant helix according to the alternative orthogonal frame {N,C,W}.
Definition 1. Let (α) be a unit speed curve in E3 and {N,C,W} be the alternative frame of the curve (α). In that case, (α) is called a C-slant helix according to the alternative frame if the vector field C makes a constant angle with a constant direction V, that is, if
⟨C,V⟩=cosδ,(δ≠π2) |
for all s∈I. Therefore, the ratio
fg=constant | (2.2) |
for the adapted curvatures fandg [25].
In this section, we first define the modified adapted frame; later, we give the definitions of the N∗-slant helix and C∗-slant helix and some of their characterizations using the modified adapted frame in E3.
Let α:I⊂R⟶E3 be a unit speed curve that can be parameterized in terms of the arc length s. Now, we define a new and different frame i.e., a modified adapted frame. The motivation for us to define this new frame in this study is that the frame defined by Uzunoǧlu et al. [25] cannot be created at every position of the curve. If the equation f(s)=‖N′(s)‖=0 holds in the frame {N,C,W} defined by them, then the vector field C=N′f=N′‖N′‖ cannot be defined. Therefore, the frame {N,C,W} cannot be constructed for ∀s∈I. In this case, the alternative frame given by them [25] needs to be modified. In this study, we modified this frame and named this new frame the "modified adapted frame". As a result, we defined this new frame {N∗,C∗,W∗} such that
N∗=κN,C∗=fC,W∗=fW, | (3.1) |
where C∗(s0)=W∗(s0)=0 if f(s0)=0. Now, we give the derivative formulas of the modified adapted frame {N∗,C∗,W∗}. For this, if we use Eqs (2.1) and (3.1), then we get
[(N∗)′(C∗)′(W∗)′]=[κ′κκ0−f2κf′fg0−gf′f][N∗C∗W∗], | (3.2) |
where ⟨N∗,N∗⟩=κ2,⟨C∗,C∗⟩=f2,⟨W∗,W∗⟩=f2, f=√κ2+τ2, κ and τ are the Frenet curvature and torsion of the curve (α), respectively, and f and g are the modified adapted curvatures. Moreover, the relationship between the modified adapted frame {N∗,C∗,W∗} and Frenet frame {T,N,B} can be written as
T=−κC∗+τW∗κ2+τ2,N=N∗κ,B=τC∗+κW∗κ2+τ2. |
Now, we give some characterizations of the N∗-slant helices and C∗-slant helices with the modified adapted frame {N∗,C∗,W∗}.
Theorem 1. Let (α) be a unit speed curve with the modified adapted apparatus {N∗,C∗,W∗,f,g} in E3. In that case, if the unit speed curve (α) is an N∗-slant helix according to the modified adapted frame, then the axis of the N∗-slant helix (α) is the constant unit vector X, which can be written as
X=κcosζN∗+f2gcosζW∗, |
where κ is the Frenet curvature of (α), f=√κ2+τ2 and ζ is the angle between the vector fields N∗ and X.
Proof. Suppose that (α) is an N∗-slant helix with respect to the modified adapted frame {N∗,C∗,W∗} in E3. Then, for the vector fields N∗ and X, we get
⟨N∗,X⟩=κcosζ, | (3.3) |
where ζ is the constant angle between the vector fields N∗ and X. In that case, differentiating (3.3) according to s with the aid of Eq (3.2), we obtain
⟨C∗,X⟩=0. | (3.4) |
Thus, we can write the constant unit vector X as
X=κcosζN∗+λW∗, | (3.5) |
where λ is a differentiable function. If we apply the differentiation process again to Eq (3.4), we have
−f2κ⟨N∗,X⟩+f′f⟨C∗,X⟩+g⟨W∗,X⟩=0 |
and
⟨W∗,X⟩=f2gcosζ. | (3.6) |
Finally, considering (3.5) and (3.6), we obtain
X=κcosζN∗+f2gcosζW∗. |
Theorem 2. Consider that (α) is a unit speed curve with the modified adapted apparatus {N∗,C∗,W∗,f,g} in E3; then, (α) is a C∗-slant helix such that the constant unit vector Y characterizing this C∗-slant helix is given by
Y=μcosηN∗+fcosηC∗+δcosηW∗, |
where f=√κ2+τ2, η is the angle between the vector fields C∗ and Y, μ=gκ(f2+g2)g′f−f′g and δ=f2(f2+g2)g′f−f′g.
Proof. Suppose that (α) is a C∗-slant helix with respect to the modified adapted frame {N∗,C∗,W∗}. In that case, with aid of Definition 1, we have
⟨C∗,Y⟩=fcosη | (3.7) |
such that η is the constant angle between the vector fields C∗ and Y. Now, we take successive derivatives of Eq (3.7) with respect to the arc-length parameter s of (α); thus, we obtain
−f2κ⟨N∗,Y⟩+f′f⟨C∗,Y⟩+g⟨W∗,Y⟩=f′cosη, |
−2ff′κ2⟨N∗,Y⟩−(f2+g2)⟨C∗,Y⟩+(g′+gf′f)⟨W∗,Y⟩=0, |
and, as a result,
⟨N∗,Y⟩=gκ(f2+g2)g′f−f′gcosη, |
⟨W∗,Y⟩=f2(f2+g2)g′f−f′gcosη, |
respectively. Consequently, considering μ=gκ(f2+g2)g′f−f′g and δ=f2(f2+g2)g′f−f′g, we can easily get
Y=μcosηN∗+fcosηC∗+δcosηW∗. |
In this section, we define the C∗-partner curves considering the modified adapted frame {N∗,C∗,W∗} and we give some characterizations of these curves.
Let α:I⊂R⟶E3 and β:J⊂R⟶E3 be considered as two different unit speed curves with arc-length parameters s∈I and s1∈J, respectively. Moreover, we consider that the Frenet frames and curvatures of the curves (α) and (β) are {T,N,B,κ,τ} and {T1,N1,B1,κ1,τ1}, respectively. In that case, the following definitions and theorems can be given.
Definition 2. Consider that α:I⟶E3 and β:J⟶E3 are two regular curves and the modified adapted frames and curvature of the curves (α) and (β) are {N∗,C∗,W∗,f,g} and {N∗1,C∗1,W∗1,f1,g1}, respectively. In that case, if the vector fields C∗ and C∗1 are linear-dependent at the corresponding noncoincident points of these curves, then (α) and (β) are called C∗-partner curves.
Theorem 3. Suppose that (α) and (β) are C∗-partner curves in E3. In that case, the distance between the corresponding noncoincident points of (α) and (β) is not constant.
Proof. Suppose that (α) and (β) are C∗-partner curves in E3. {N∗,C∗,W∗} and {N∗1,C∗1,W∗1} are the modified adapted frames of the curves (α) and (β), respectively. In that case, the relationship between the C∗-partner curves at the corresponding noncoincident points can be written as
β(s)=α(s)+D(s)C∗(s), | (3.8) |
where D=D(s) is the distance function and the arc-length parameter of the curve (α) is s∈I. If we take the derivative of Eq (3.8) with respect to the parameter s∈I, we get
T1ds1ds=T+D′C∗+D(C∗)′, |
where the tangent vectors of the C∗-partner curves (α) and (β) are, respectively, T and T1, and the arc-length parameter of the curve (β) is s1. Now, we use the relationships between the Frenet and modified adapted frames of the C∗-partner curves. Therefore, we have
T1ds1ds=T+(D′+Df′f)C∗−Df2κN∗+DgW∗ |
and
−κ1f21ds1dsC∗1+τ1f21ds1dsW∗1=(−κf2+D′+Df′f)C∗−Df2κN∗+(τf2+Dg)W∗, |
where {κ,τ} and {κ1,τ1} are the Frenet curvatures of C∗-partner curves (α) and (β), respectively. By considering the linear-dependent pair {C∗,C∗1}, the equation
κ−κ1ds1dsf=(Df)′ |
holds. Consequently, we see that the distance function D=D(s) is not constant.
Now, we assume that the vector fields C∗ and C∗1 satisfy the equation C∗=C∗1. In this case, for the adapted curvatures f and f1 of the C∗-partner curves, the equation f2=f21 holds. As a result, the following theorem can be given:
Theorem 4. Suppose that (α) and (β) are C∗-partner curves. In that case, if the angle between N∗ and N∗1 is ϱ, then the relationships between the modified adapted frames {N∗,C∗,W∗} and {N∗1,C∗1,W∗1} of the curves (α) and (β), respectively, are
[N∗C∗W∗]=[κκ1cosϱ0κf1sinϱ010−fκ1sinϱ0cosϱ][N∗1C∗1W∗1], | (3.9) |
where κ,κ1 and f,f1 are the Frenet curvatures and adapted curvatures of the C∗-partner curves (α,β), respectively.
Proof. Consider that (α,β) are C∗-partner curves and C∗=C∗1. In that case, if we consider that the angle between the vector fields N∗ and N∗1 is ϱ, we can write
⟨N∗,N∗1⟩=κκ1cosϱ,⟨W∗,W∗1⟩=ff1cosϱ,⟨N∗,W∗1⟩=κf1sinϱ,⟨W∗,N∗1⟩=−κ1fsinϱ. |
Consequently, considering the basic arrangements in differential geometry, we obtain
N∗=κκ1cosϱN∗1+κf1sinϱW∗1,W∗=−fκ1sinϱN∗1+cosϱW∗1. |
Alternatively, we can write
N∗1=κ1κcosϱN∗−κ1fsinϱW∗,W∗1=fκsinϱN∗+cosϱW∗, | (3.10) |
where f=f1.
Theorem 5. Consider that (α) and (β) are C∗-partner curves in E3. Therefore, the angle between N∗ and N∗1 of these curves is constant.
Proof. We know that (α) and (β) are C∗-partner curves, and that there is the relationship
N∗1=κ1κcosϱN∗−κ1fsinϱW∗ | (3.11) |
from (3.10), where {N∗,C∗,W∗,f,g} and {N∗1,C∗1,W∗1,f1,g1} are the modified adapted frames and κ and κ1 are the Frenet curvatures of the C∗-partner curves (α,β), respectively. In that case, by differentiating (3.11) with respect to s, we obtain
dN∗1ds1ds1ds=(κ1cosϱκ)′N∗+κ1cosϱκ(N∗)′−(κ1sinϱf)′W∗−κ1sinϱf(W∗)′, |
and by considering Eqs (3.2) and (3.11), we can write
κ′1κ1cosϱds1dsN∗+κ1ds1dsC∗1−κ′1fsinϱds1dsW∗=(κ′1cosϱ−κ1ϱ′sinϱκ)N∗+(κ1cosϱ+κ1gfsinϱ)C∗−(κ′1sinϱ+κ1ϱ′cosϱf)W∗. |
As a result, we get
(κ1cosϱ)′=κ′1cosϱds1ds | (3.12) |
and
(κ1sinϱ)′=κ′1sinϱds1ds. | (3.13) |
Moreover, we obtain from Eqs (3.12) and (3.13) the equations
(κ1cosϱ)′cosϱ=(κ1sinϱ)′sinϱ |
and
κ1ϱ′=0. |
Consequently, for κ1≠0, we have ϱ′=0, and it is easy to see that the angle ϱ is constant.
Theorem 6. Let the curves (α) and (β) be considered C∗-partner curves and D=D(s) be the distance function between corresponding noncoincident points of these curves. Therefore, the distance D is a constant necessary and sufficient condition, i.e.,
(τf3)′f2(τf2g)′g2=−tanϱ=constant, |
where τ and f and g are the Frenet torsion and adapted curvatures of the curve (α), respectively, and ϱ is the angle between the vector fields N∗ and N∗1.
Proof. If (α) and (β) are C∗-partner curves, then we know that the relationship between these curves is
β(s)=α(s)+D(s)C∗(s). |
In that case, considering the derivative of the last equation with respect to s, we get
−κ1f21ds1dsC∗1+τ1f21ds1dsW∗1=−Df2κN∗+(−κf2+D′+Df′f)C∗+(τf2+Dg)W∗. |
If we make necessary arrangements using (3.9), then we have
−κ1f21ds1dsC∗1+τ1f21ds1dsW∗1=(−Df2κ1cosϱ−τfκ1sinϱ−Dgfκ1sinϱ)N∗1+(−κf2+D′+Df′f)C∗+(−Df2f1sinϱ+τf2cosϱ+Dgcosϱ)W∗1. |
The vector field C∗ and C∗1 are linear-dependent; therefore, the equation
Df3cosϱ+Dgf2sinϱ+τsinϱ=0 |
is satisfied. In that case, we obtain
D=−τsinϱf2(fcosϱ+gsinϱ) |
and
D′=−τ′sinϱ(f2gsinϱ+f3cosϱ)+τsinϱ((2ff′g+f2g′)sinϱ+3f2f′cosϱ)f4(fcosϱ+gsinϱ)2. |
Now, we assume that the distance function D(s) is a constant necessary and sufficient condition, where D′(s)=0. As a result, we get
τ′sinϱfg+τ′cosϱf2−2τsinϱf′g−τsinϱfg′−3τff′cosϱ=0 |
from the last equation. Consequently, we obtain
(τf3)′f2(τf2g)′g2=−tanϱ=constant, |
where ϱ is constant.
Let (α) and (β) be considered C∗-partner curves. Now, we give some applications to show the character of the C∗-partner curve, where any given curve (α) or (β) is helix or slant helix. For this, the following theorems can be given.
Theorem 7. Let (α,β) be C∗-partner curves in E3. In that case, the modified adapted curvatures {f1,g1} of the curve (β) in terms of {f,g} of the curve (α) is given by
g1f1=gfcosϱ−sinϱgfsinϱ+cosϱ, | (3.14) |
where ϱ is the constant angle between N∗ and N∗1.
Proof. Suppose that (α) and (β) are C∗-partner curves. Therefore, if we differentiate W∗1 in (3.10) with respect to s∈I of (α), we obtain
−g1ds1dsC∗1+f′1f1ds1dsW∗1=f′sinϱκN∗+(fsinϱ−gcosϱ)C∗+f′cosϱfW∗, | (3.15) |
where, by using the derivative formulas given by Eqs (3.2) and (3.10) in (3.15), the equation
−g1ds1dsC∗+f′1κsinϱds1dsN∗+f′1f1cosϱds1dsW∗=f′κsinϱN∗+(fsinϱ−gcosϱ)C∗+f′fcosϱW∗ |
holds. We know that C∗=C∗1; therefore, we obtain
−g1ds1ds=fsinϱ−gcosϱ; |
consequently, we get
g1f1=gcosϱ−fsinϱgsinϱ+fcosϱ. |
The following corollaries can be given as consequences of Theorem 7.
Corollary 1. Consider that (α,β) are C∗-partner curves in E3. In addition, let (τ,τ1) be the Frenet torsions of the C∗-partner curves (α,β) and {f,g} and {f1,g1} be the modified adapted curvatures of the curves (α,β), respectively. Therefore, the torsions (τ,τ1) can be written by modified adapted curvatures such that
τ1τ=cosϱ−g1f1sinϱcosϱ+gfsinϱ. | (3.16) |
Corollary 2. Let the curves (α,β) be C∗-partner curves. If the curve (α) is a slant helix, then the necessary and sufficient condition is that (β) is a slant helix.
Proof. Consider that (α,β) are C∗-partner curves and the curve (α) is a slant helix. In that case, we know that gf=constant from (2.2). If we consider Eq (3.14), we have g1f1=constant. As a result, (β) is a slant helix. Similarly, if the curve (β) is a slant helix, then g1f1=constant. Therefore, from Eq (3.14), we obtain
gf=−g1f1cosϱ−sinϱg1f1sinϱ−cosϱ. | (3.17) |
Consequently, we have gf=constant and the curve (α) is a slant helix.
Corollary 3. Suppose that the curves (α,β) are C∗-partner curves. If (α) is a helix, then (β) is a slant helix. If (β) is a helix, then (α) is a slant helix.
Proof. We assume that the curve (α) is a helix. The Frenet curvature ratio τκ=constant and g=0; therefore, considering Eq (3.14) we obtain
g1f1=−tanϱ(ϱ=constant), |
and the curve (β) is a slant helix. Similarly, if we take that the curve (β) is a helix, then g1=0. Consequently, from Eq (3.17), we see that gf=tanϱ=constant and the curve (α) is a slant helix.
Corollary 4. Consider that the curves (α,β) are the C∗-partner curves. In that case, if the curve (α) (or(β)) is a slant helix, then τ1τ=constant where (τ,τ1) are the Frenet torsions of the C∗-partner curves (α) and (β), respectively.
Proof. Let the curve (α) (or(β)) be a slant helix from the C∗-partner curves (α,β). Therefore, we know that (α) is a slant helix necessary and sufficient condition whereby the curve (β) is a slant helix from Corollary 2. As a result, the ratio gf=constant with the necessary and sufficient condition of g1f1=constant. Consequently, consider Eq (3.16); we obtain τ1τ=constant.
Example 1. Consider a circular helix ω given by the parameterization
ω(ρ)=1√2(−cosρ,sinρ,ρ). | (3.18) |
Then, let the C∗-partner curves of the curve ω be ψ∗, ψ∗∗ for the distance function D(s);
i) for D(ρ)=√3ρ, the C∗-partner curve ψ∗ of ω is obtained as
ψ∗(s)=(−cosρ√2−√3ρsinρ2,sinρ√2+√3ρcosρ2,ρ2). | (3.19) |
Figure 1 shows the graph of the curve ω and the C∗-partner curve ψ∗.
ii) for D(ρ)=√3ρ, the C∗-partner curve ψ∗∗ of ω is obtained as
ψ∗∗(s)=(−cosρ√2−(√2+ρ2)sinρ6,sinρ√2+(√2+ρ2)cosρ6,ρ2). | (3.20) |
Figure 2 shows the graph of the curve ω and the C∗-partner curve ψ∗∗.
Example 2. Consider a circular helix ω given by the parameterization
ω(ρ)=(112cos4ρ+112cos2ρ,112sin4ρ+112sin2ρ,2√23). | (3.21) |
Then, let the C∗-partner curves of the curve ω be ψ∗, ψ∗∗ for the distance function D(s);
i) for D(ρ)=√32ρ, the C∗-partner curve ψ∗ of ω is obtained as ψ∗(s)=ω(s)+D(s)C∗(s)
ψ∗(s)=(112cos4ρ+112cos2ρ+2ρsin3ρ,112sin4ρ+112sin2ρ−2ρcos3ρ,2√23). | (3.22) |
Figure 3 shows the graph of the curve ω and the C∗-partner curve ψ∗.
ii) for D(ρ)=8√2+ρ26√2ρ, the C∗-partner curve ψ∗∗ of ω is obtained as
ψ∗∗(s)=(112cos4ρ+112cos2ρ+8√2+ρ23sin3ρ,112sin4ρ+112sin2ρ−8√2+ρ23cos3ρ,2√23). | (3.23) |
Figure 4 shows the graph of the curve ω and the C∗-partner curve ψ∗∗.
The fundamental theorem of regular curves is that there is a unit speed curve with curvature and torsion κ and τ, respectively, where κ>0 and τ are differentiable functions. In addition, the principal normal and binormal vector fields of the curve are not continuous at the zero points of the curvature. In this case, at a point where the curvature of a generally considered space curve disappears, the Frenet frame for this curve causes uncertainty. To solve this problem, Hord [13] and Sasai [22,23] found a simple but useful way and introduced a new orthogonal frame i.e., a the modified frame. On the other hand, another frame, as an alternative to the Frenet frame i.e., an adapted frame, was defined by Uzunoǧlu et. al [25] with the help of the Darbuox vector and Frenet normal vector of a regular curve. However, if the equation f=0 holds in the adapted frame {N,C,W} defined by them, then the vector field C cannot be defined. Therefore, the frame {N,C,W} cannot be constructed for all points on the curve. In that case, the alternative frame given by them [25] needs to be modified. So, the purpose of our study was to define a new modified adapted frame that removes all singular points in a curve. Moreover, with this new frame we have defined in this study, we believe that the curve theory, which will be studied with this frame in future studies, will be open to many innovations by bringing a different perspective to the curve theory in differential geometry. In addition, with this new frame, curve theory will gain a different perspective in many fields such as engineering and physics. Our theoretical and practical studies on this new frame continue.
The authors declare that they have no conflict of interests.
[1] |
T. Z. Li, C. L. Mei, Estimation and inference for varyig coefficient partially nonlinear models, J. Stat. Plann Inference, 143 (2013), 2023–2037. doi: 10.1016/j.jspi.2013.05.011
![]() |
[2] |
J. Yang, H. Yang, Smooth-threshold estimating equations for varying coefficient partially nonlinear models based on orthogonality-projection method, J. Comput. Appl. Math., 302 (2016), 24–37. doi: 10.1016/j.cam.2016.01.038
![]() |
[3] |
Y. Y. Qian, Z. S. Huang, Statistical inference for a varying-coefficient partially nonlinear model with measurement errors, Stat. Methodol., 32 (2016), 122–130. doi: 10.1016/j.stamet.2016.05.004
![]() |
[4] |
X. S. Zhou, P. X. Zhao, X. L. Wang, Empirical likelihood inferences for varying coefficient partially nonlinear models, J. Appl. Stat., 44 (2017), 474–492. doi: 10.1080/02664763.2016.1177496
![]() |
[5] |
Y. L. Jiang, Q. H. Ji, B. J. Xie, Robust estimation for the varying coefficient partially nonlinear models, J. Comput. Appl. Math., 326 (2017), 31–43. doi: 10.1016/j.cam.2017.04.028
![]() |
[6] |
Y. T. Xiao, Z. S. Chen, Bias-corrected estimations in varying-coefficient partially nonlinear models with measurement error in the nonparametric part, J. Appl. Stat., 45 (2018), 586–603. doi: 10.1080/02664763.2017.1288201
![]() |
[7] | X. L. Wang, P. X. Zhao, H. Y. Du, Statistical inferences for varying coefficient partially nonlinear model with missing covariates, Commun. Stat. Theory Methods, (2019). |
[8] | R. J. A. Little, D. B. Rubin, Statistical analysis with missing data, 2 Eds., New York: Wiley, 2002. |
[9] |
P. E. Cheng, Nonparametric estimation of mean functional with data missing at random, J. Am. Stat. Assoc., 89 (1994), 81–87. doi: 10.1080/01621459.1994.10476448
![]() |
[10] | Q. H. Wang, J. N. K. Rao, Empirical likelihood for linear regression models under imputation for missing responses, Can. J. Stat., 29 (2001), 596–608. |
[11] | Q. H. Wang, J. N. K. Rao, Empirical likelihood-based inference under imputation for missing response data, Ann. Stat., 30 (2002), 896–924. |
[12] |
Q. H. Wang, O. Linton, W. Härdle, Semiparametric regression analysis with missing response at random, J. Am. Stat. Assoc., 99 (2004), 334–345. doi: 10.1198/016214504000000449
![]() |
[13] |
L. G. Xue, Empirical likelihood for linear models with missing responses, J. Multivar. Anal., 100 (2009), 1353–1366. doi: 10.1016/j.jmva.2008.12.009
![]() |
[14] |
L. G. Xue, Empirical likelihood confidence intervals for response mean with data missing at Random, Scand. J. Stat., 36 (2009), 671–685. doi: 10.1111/j.1467-9469.2009.00651.x
![]() |
[15] |
X. L. Wang, F. Chen, L. Lin, Empirical likelihood inference for estimating equation with missing data, Sci. China Math., 56 (2013), 1233–1245. doi: 10.1007/s11425-012-4504-x
![]() |
[16] |
A. Owen, Empirical likelihood ratio confidence intervals for a single functional, Biometrika, 75 (1988), 237–249. doi: 10.1093/biomet/75.2.237
![]() |
[17] | A. Owen, Empirical likelihood ratio confidence regions, Ann. Stat., 18 (1990), 90–120. |
[18] | P. P. Chen, S. Y. Feng, L. G. Xue, Statistical inference for semiparametric varying coefficient partially linear model with missing data (in Chinese), Acta Math. Sci., 35 (2015), 345–358. |
[19] |
Y. P. Mack, B. W. Silverman, Weak and strong uniform consistency of kernel regression estimates, Z. Wahrsch. Verw. Gebiete., 61 (1982), 405–415. doi: 10.1007/BF00539840
![]() |
[20] |
J. Shi, T. S. Lau, Empirical Likelihood for partially linear models, Multivar. Anal., 72 (2000), 132–148. doi: 10.1006/jmva.1999.1866
![]() |
[21] |
X. L. Wang, Y. Q. Song, L. Lin, Handling estimating equation with nonignorably missing data based on SIR algorithm, J. Comput. Appl. Math., 326 (2017), 62–70. doi: 10.1016/j.cam.2017.05.016
![]() |
[22] |
X. L. Wang, Y. Q. Song, S. X. Zhang, An efficient estimation for the parameter in additive partially linear models with missing covariates, J. Korean Stat. Soc., 49 (2020), 779–801. doi: 10.1007/s42952-019-00036-6
![]() |
[23] |
H. M. Sun, Y. H. Luan, J. M. Jiang, A new classified mixed model predictor, J. Stat. Plann. Inference, 207 (2020), 45–54. doi: 10.1016/j.jspi.2019.11.001
![]() |
[24] |
Y. Fang, G. Cheng, Z. F. Qu, Optimal reinsurance for both an insurer and a reinsurer under general premium principles, AIMS Math., 5 (2020), 3231–3255. doi: 10.3934/math.2020208
![]() |
[25] |
Y. Fang, X. Wang, H. L. Liu, T. Li, Pareto-optimal reinsurance for both the insurer and the reinsurer with general premium principles, Commun. Stat. Theory Methods, 48 (2019), 6134–6154. doi: 10.1080/03610926.2018.1528364
![]() |
1. | Ana Savić, Kemal Eren, Soley Ersoy, Vladimir Baltić, Alternative View of Inextensible Flows of Curves and Ruled Surfaces via Alternative Frame, 2024, 12, 2227-7390, 2015, 10.3390/math12132015 | |
2. | ESRA PARLAK, TEVFİK ŞAHİN, GEOMETRIC PERSPECTIVE OF BERRY'S PHASE ACCORDING TO ALTERNATIVE ORTHOGONAL MODIFIED FRAME, 2025, 25, 2068-3049, 11, 10.46939/J.Sci.Arts-25.1-a02 |