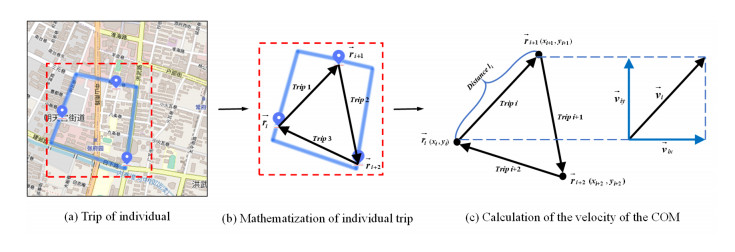
Citation: A. M. Elaiw, N. H. AlShamrani, A. D. Hobiny. Mathematical modeling of HIV/HTLV co-infection with CTL-mediated immunity[J]. AIMS Mathematics, 2021, 6(2): 1634-1676. doi: 10.3934/math.2021098
[1] | Haoqing Wang, Wen Yi, Yannick Liu . An innovative approach of determining the sample data size for machine learning models: a case study on health and safety management for infrastructure workers. Electronic Research Archive, 2022, 30(9): 3452-3462. doi: 10.3934/era.2022176 |
[2] | Tej Bahadur Shahi, Cheng-Yuan Xu, Arjun Neupane, William Guo . Machine learning methods for precision agriculture with UAV imagery: a review. Electronic Research Archive, 2022, 30(12): 4277-4317. doi: 10.3934/era.2022218 |
[3] | Xiaoyan Wu, Guowen Ye, Yongming Liu, Zhuanzhe Zhao, Zhibo Liu, Yu Chen . Application of Improved Jellyfish Search algorithm in Rotate Vector reducer fault diagnosis. Electronic Research Archive, 2023, 31(8): 4882-4906. doi: 10.3934/era.2023250 |
[4] | Xi Luo, Ran Yan, Shuaian Wang, Lu Zhen . A fair evaluation of the potential of machine learning in maritime transportation. Electronic Research Archive, 2023, 31(8): 4753-4772. doi: 10.3934/era.2023243 |
[5] | Jialiang Xiao, Yongtao Zheng, Wei Wang, Xuedong Hua . Robustness analysis of a multimodal comprehensive transportation network from the perspectives of infrastructure and operation: A case study. Electronic Research Archive, 2025, 33(4): 1902-1945. doi: 10.3934/era.2025086 |
[6] | Yufei Duan, Xian-Ming Gu, Tingyu Lei . Application of machine learning in quantitative timing model based on factor stock selection. Electronic Research Archive, 2024, 32(1): 174-192. doi: 10.3934/era.2024009 |
[7] | Bangna Li, Qingqing Zhang, Xingshi He . Multi-label feature selection via constraint mapping space regularization. Electronic Research Archive, 2024, 32(4): 2598-2620. doi: 10.3934/era.2024118 |
[8] | Nihar Patel, Nakul Vasani, Nilesh Kumar Jadav, Rajesh Gupta, Sudeep Tanwar, Zdzislaw Polkowski, Fayez Alqahtani, Amr Gafar . F-LSTM: Federated learning-based LSTM framework for cryptocurrency price prediction. Electronic Research Archive, 2023, 31(10): 6525-6551. doi: 10.3934/era.2023330 |
[9] | Qing Tian, Yanan Zhu, Yao Cheng, Chuang Ma, Meng Cao . Unsupervised domain adaptation through transferring both the source-knowledge and target-relatedness simultaneously. Electronic Research Archive, 2023, 31(2): 1170-1194. doi: 10.3934/era.2023060 |
[10] | Pinchao Meng, Xinyu Wang, Weishi Yin . ODE-RU: a dynamical system view on recurrent neural networks. Electronic Research Archive, 2022, 30(1): 257-271. doi: 10.3934/era.2022014 |
Rapid urbanization generates growing amounts of travel flows, urging the requirement for efficient transport planning policies. During 1980's, in Shanghai, China, the urbanization processes were in their nascent stages, with an approximate daily total of 20 million trips. By 2019, this daily total had surged to approximately 57.31 million trips. Similarly, in Beijing, since the inception of the first travel survey in 1986, the daily volume of trips in the central urban area has exhibited an average annual increase of 4%. By 2019, the daily volume of trips had reached a significant 39.57 million. The substantial growth has resulted in severe traffic congestion, with an average congestion duration of three hours in 2019 [1,2]. These trends underscore the urgent need for the development and implementation of efficient transport planning policies. Accurate comprehension of an individual's transport mode choice enables the development of customized guidance policies for different demographic groups. These policies can significantly contribute to the optimization of transportation system infrastructure, the promotion of increased public transportation utilization and the relief of traffic congestion. Consequently, the development of precise models for transport mode detection assumes paramount importance [3].
In fact, the choice of transport modes is influenced by many types of factors, including individual, household and built environment factors. Individual factors include age, gender, income, occupation, attitudes and trip purpose [4,5,6,7,8,9]. Household factors include household structure, household characteristics, number of household members and car ownership, etc. [10,11,12]. Furthermore, several attributes of the built environment have been recognized to influence the choice of transport modes, such as building density, land use mix and distance to the transport facilities [13,14,15,16]. The primary method of collecting the data is via travel survey. Collecting individual mobility patterns and rich sociodemographic information are critical advantages of travel surveys [17,18,19,20]. However, respondents may provide incorrect information out of a desire to protect their privacy, such as by providing lower-income information, etc. This reason results in insufficient data collection accuracy and impacts the validity of the subsequent study [21,22]. Additionally, ensuring the security of respondents' privacy can be challenging. Balancing the detection performance of transport mode with the imperative of ensuring respondents' privacy remains a significant challenge in this field of research.
Most detection models for transport mode are predicted by econometric discrete choice models (DCMs) based on the random utility framework, such as multinomial logit model, nested logit model and mixed logit model [23,24,25]. The main advantage of these models is that they have good interpretability because of explicit mathematical formulas. The utility functions in the model are defined manually before fitting the model. This step allows for the integration of established behavioral theory into the model [26,27]. However, manual definitions may be violated under real complex problems, leading to biased predictions [28,29]. In contrast to traditional DCMs, machine learning models applied to model the choice of transport modes are promising methods to address current limitations [30,31,32,33,34]. Instead of making the necessary prior definitions, machine learning models represent complex relationships between variables through data-driven learning. Although machine learning models have better predictive accuracy, they focus on prediction and lack model interpretability [35]. Novel techniques and experiments such as t-SNE, SHapley Additive exPlanations (SHAP) and ablation experiments are proposed to overcome the non-interpretability issue of the machine learning models [36,37,38]. At the same time, most of the current detection models detect transport modes, with their categories including car, walk, bike, and public transport. Some studies refine "bike" into bike and e-bike. For public transport, studies consider them as a broad category. For example, they do not distinguish between the bus and the subway [30,39,40]. The coarse-grained classification of public transport results in the inability to target groups with specific transport modes for interventions in transportation planning. Therefore, achieving accurate individual transport mode detection for improved travel demand management still presents many areas for research.
The detection of transport mode plays a crucial role in managing travel demand. The choice of transport mode is influenced by a variety of factors including age [4], gender [5], income [6], occupation [7], attitudes [8], trip purpose [9], household structure [10], household characteristics [11] number of household members [12], car ownership [13] and built environment [14]. For example, Kim et al. found that the built environment has a greater impact on young and elderly individuals compared to middle-aged individuals in the choice of public transportation [4]. Subjective determinants like travel satisfaction can also influence transport mode detection. A study found that pro-environmental attitudes have a positive impact on the utility of subway and walking as modes of travel, and this preference increases with the duration of walking trips [41]. Another study found that cars promote subjective well-being by facilitating leisure activities, but they also diminish a sense of belonging and achievement through shopping activities [42]. The acquisition of this information can be time-consuming and challenging [26,43]. Respondents may provide inaccurate information due to privacy concerns, which affect the efficacy of subsequent research. Therefore, there is potential to propose a method to both protect individual privacy and accurately detect the transport mode.
Research on individual spatial mobility features has provided the potential for detecting modes. Gonzalez et al. first introduced physical concepts such as the COM and radius of gyration to study individuals' mobility features [44]. They found that individuals' trajectories exhibit high spatiotemporal regularities, with each individual displaying time-invariant features such as travel distance and a significant probability of returning to specific frequent locations. Another study revealed that if a person's future mobility behaviour is influenced by their historical movements, it can be considered a memory-dependent human mobility model [45]. Later, Hong et al. observed that individuals from both datasets maintain a consistent number of combinations of travel modes and activity locations over time [46]. However, the currently proposed mobility features alone may not be sufficient for detecting travel modes. Velocity and acceleration play crucial roles in transport mode detection. To improve the accuracy of transport mode detection using trip information, we have introduced velocity and acceleration of the COM in the mobility features.
Traditionally, DCMs have been employed to detect transport modes of individuals. DCMs based on the random utility maximization theory commonly include forms such as the multinomial logit model, nested logit model and mixed logit model [34]. These models have a mathematical structure, making them highly interpretable. Nevertheless, DCMs require many specific features for assessing their impact on transport mode detection, demanding the collection of extensive and detailed data [26]. The acquisition of this data is time-consuming and involves significant implementation costs. Additionally, DCMs are based on model assumptions, such as the independence of irrelevant alternatives (IIA) for the multinomial logit model. If these assumptions are violated, it will lead to biases in parameter estimation and model detection.
Machine learning models which are driven by data are emerging as feasible alternatives for detecting individual transport modes. Various machine learning methods like support vector machines (SVM) [47], k-nearest neighbors (KNN) [27], decision trees (DT) [48] and artificial neural networks (ANN) [49] have been employed in transport mode detection without stringent assumptions. As machine learning models develop, individual models have exhibited limitations in predicting various aspects. To enhance detection accuracy and robustness, ensemble models such as random forests (RF) [30], XGBoost [50] and CatBoost [51] have been developed. For example, a study compared the performance of seven different machine learning models in detecting transport modes and successfully detected four modes: walk, bike, public transport and car [27]. Omrani used four machine learning models to detect individual transport modes in Luxembourg, aggregating walking and biking into one category and recognizing three transport modes: car, public transport and soft mode [52]. In another study, Zhao et al. compared four different types of machine learning models, namely Naive Bayes, tree-based models, SVM and neural networks (NN) for predicting individual choices among four transport modes: car, walk, bike and public transport [26].
Recently, a novel machine learning model called Deep Forest (DF) has been developed and has achieved success in various domains, including image recognition, natural language processing and anomaly detection [53]. The DF model demonstrates the ability to handle high-dimensional data, capture intricate patterns, and make accurate predictions with limited data. The advantages of the DF model make it a potential method to enhance the accuracy and reliability of transport mode detection models. However, most of the methods proposed in literature lack interpretability, which limits their applicability in policy-making. Additionally, these models can detect only a limited number of transport modes, typically three or four. They can detect public transport modes but are unable to distinguish between buses and subways within the category of public transport.
The review of these studies reveals that some aspects need to be improved in the current research: (ⅰ) The traditional DCMs employ individual and household attribute data to detect transport modes. These attribute data involve individual privacy and affect the applicability of the model. (ⅱ) The DCMs produce biased detections, though they reveal the contribution of the variables. The machine learning models are more effective for mode detection, but most models need more interpretability. (ⅲ) Current studies mainly treat public transport as a broad category, making it ill-suited for implementing fine transportation planning.
Therefore, an interpretable machine learning model is proposed for the detection of transport modes. We ignore individual and household attribute data and consider individual mobility features to train the detection models. A wide range of multi-source data that can be utilized to detect transport modes is easily accessible with the rapid development of big data, such as land use mix, GDP, population and online map service data. We combine individual mobility features and multi-source data as detection features for the models. The different machine learning models using detection features were applied to detect fine-grained transport modes, which were divided into seven categories. Specifically, the categories of public transport are subdivided into bus, metro and public transport (including transfers). We calculate four performance metrics to verify the performance of the proposed models for the transport modes. Furthermore, t-SNE and ablation experiments are applied to improve the interpretability of the machine learning models.
The main contributions of this study are as follows. First, the individual and household factors are ignored in the transport mode detection models in order to protect individual privacy and improve the applicability of the model. Thus, the study innovatively considers individual mobility features and combines them with multi-source data, including land use mix, GDP, population and online map service data as detection features. Third, the performance of the deep forest machine learning models has been investigated in a comparative study with traditional classifiers for transport mode detection. We detect the transport modes at the fine-grained level, which are divided into seven categories: car, walk, bike, e-bike, bus, metro and public transport (including transfers). Finally, t-SNE and ablation experiments are employed to enhance the interpretability of the machine learning models.
The remainder of the paper is organized as follows: Section 2 describes the proposed method for transport mode detection, including the extraction of individual mobility features, processing of multi-source data and interpretable machine learning detection models. Sections 3 and 4 discuss the experimental results of different machine learning models using Nanjing as an example. Finally, in Section 5, we summarize the study and propose future research directions.
The proposed model for detecting transport modes compromises three steps. First, individual mobility features are extracted, making use of the spatial movement. Next, land use mix and online map service data are extracted as multi-source data to enhance the performance of transport mode detection. Finally, these extracted features and multi-source data serve as inputs for the proposed detection model. The performance of models is assessed using performance metrics such as accuracy, recall, precision and F1-measure to verify its effectiveness. The following sections discuss in detail the steps and substeps of the proposed method.
Historical movements influence mobility of individuals. Information about the choice of transport modes is implied in individual mobility features [45,54]. The COM of trips is the center of individual spatial mobility, which is a vital feature in describing individual mobility. Radius of gyration is used to measure the extent of individual travel activity. These are the essential features of individual mobility [44,55]. Velocity and acceleration are critical features in transport mode detection. These two features are introduced to detect the transport mode more accurately from the trip information. We innovatively introduce the velocity and acceleration of the COM in the mobility features.
Many multi-source datasets used to detect transport modes are readily available with the rapid development of big data [56,57]. First, the share of different transport modes varies in areas with different land uses. Land use mix is an important indicator of the choice of transport mode [58,59]. Second, individual income levels and regional development levels also influence the choice of transport modes [60]. Owing to the anonymity of the data, it is impossible to identify the socioeconomic and demographic backgrounds of individuals. The proposed model considers the analysis in different administrative regions, using regional GDP and population as detection features. Finally, online map service data can be used to obtain the travel path, distance and time of different transport modes, which can further improve the performance of the detection models [61]. Hence, this study uses the land use mix, GDP, population, and online map service data as the features to detect the transport modes of individuals.
We combine individual mobility features and multi-source data, including land use mix, GDP, population and online map service data as detection features. The machine learning models are applied to detect transport modes, which are divided into seven categories: car, walk, bike, e-bike, bus, metro and public transport (including transfers). To enhance the interpretability of the machine learning models, t-SNE and ablation experiments are conducted in the analysis.
Center of mass. COM →cm for individual trips is determined by considering an individual's stay locations and the frequency of their stays at various spatial positions. This metric can be viewed as the central location within an individual's spatial mobility range. To calculate the COM for individual trips, we map the stay location of an individual onto a two-dimensional (2D) coordinate system and use the following formula:
→cm=∑ini→ri∑i∈Lni | (1) |
where i is the stay location of the individual, ni is the number of stays at location I, L is the set of stay locations and →ri is the 2D vector coordinate of stay location i.
Velocity and Acceleration of the COM. The velocity and acceleration of the COM are applied to calculate the velocity and acceleration of the COM for the trips of an individual. The velocity of the COM indicates the speed at which individuals move through space, while the acceleration of the COM reflects changes in an individual's mobility, such as instances of acceleration or deceleration during a journey. Stay location i to stay location i + 1 constitutes one trip i, where each trip includes a transport mode. The distance from stay location i to i + 1 is li, and the trip duration is ti. The velocity →vi and acceleration →ai of trip i are calculated for each individual trip.
li=√(→ri+1−→ri)2 | (2) |
→vi=liti,→ai=lit2i | (3) |
Except for the velocity of the COM mentioned below, the calculation procedure for the acceleration of the COM is the same. The calculation procedure is illustrated in Figure 1. Specifically, the velocity of trip i is decomposed into velocity in the horizontal →vix and velocity in the vertical direction →viy.
→viy=|yri+1−yri|li→vi=|yri+1−yri|ti,→vix=|xri+1−xri|li→vi=|xri+1−xri|ti | (4) |
where xri is the latitude of stay location I and yri is the longitude of stay location i.
Then the velocity of COM vc is calculated using the frequency of each stay location.
→vcx=N−1∑i=1ni→vix∑i∈Lni,→vcy=N−1∑i=1ni→viy∑i∈Lni | (5) |
vc=√→vcx2+→vcy2 | (6) |
where →vcx is the horizontal velocity of the COM and →vcy is the vertical velocity of the COM.
Radius of gyration. Radius of gyration rg represents the weighted average of distances from an individual's stay locations to the COM. This metric is employed to measure the spatial extent covered by individual mobility. COM is used as the axis of rotation, and the frequency of the individual at the stay location is used as the mass. rg for the individual trip is calculated as follows:
rg=√1N∑i∈Lni(→ri−→cm)2 | (7) |
Land Use Mix. Land use mix is an important indicator that reflects the choice of the transport mode. In this study, the land use mix were used as detection features. Land use data were obtained from the Chinese essential urban land use categories (EULUC) dataset [62]. We draw on the code for planning standards of development land, and classify land use into six categories: residential, administration and public services, commercial and business facilities, industrial, street and transportation and green space. The processing method involves the following steps, as illustrated in Figure 2.
First, the size of the land use map was chosen as the basis for creating the fishnet. The width and height of the cell size were set to 1 km in the fishnet. We clip the fishnet and overlay it with land use data. The entropy index (ENT) is usually applied to measure land use heterogeneity. The calculation procedure is as follows:
ENT=−∑(AijlnAij)lnNj | (8) |
where Aij is the proportion of land use type i in cell j and Nj is the number of land use types in grid j. ENT ranges from zero to one.
Finally, the coordinate data of the trip origin and destination were imported to calculate ENT at each destination and origin.
Online Map Service Data. We introduce online map service data as multi-source data to improve detection performance [61]. Specifically, the Baidu application programming interface (API) is utilized to obtain the shortest travel path of individuals in seven transport modes: car, walk, bike, e-bike, bus, metro and public transport-including transfers.
The Baidu API is an open map solution interface for developers that provides route planning, real-time navigation, global positioning and other services. We mainly used the route planning function, which can query travel routes. The parameters include the latitude and longitude coordinates of the origin and destination of the trip, geographic coordinate system type and developer key. We consider that the four transport modes of route change less in a short period (including driving, riding a bike or e-bike and walking), while public transport needs to consider departure time. Therefore, the parameters of public transport include departure time, date and tactics. It is worth mentioning that the parameters named "Departure_date" and "Departure_time" respectively represent the date and time of the trip's departure. Therefore, it is possible to return the route parameters based on the specified date and time. Table 1 lists the public transport API web addresses and parameters.
Parameters | Value | Description |
Origin | 32.048245,118.796519 | Trip origin (Latitude, longitude) |
Destination | 32.052371,118.890583 | Trip destination (Latitude, longitude) |
Coord_type | WGS84 | Type of geographic coordinate system |
Departure_date | 20150618 | Date of trip origin |
Departure_time | 08:00 | Time of trip origin |
Tactics_incity | 0 | 0: Recommended mode; 1: Minimal interchanges mode; 2: Minimal walking mode; 3: No metro mode; 4: Short time mode; 5: Metro priority mode. |
URL | https://api.map.baidu.com/direction/v2/transit? |
These parameter values are input to a uniform resource locator (URL) and requested using Python to obtain the shortest travel path for each transport mode. The returned results include the travel time, distance and path for the entire trip. This method determines the transport mode of individual trips by comparing the travel time from the survey data with the time taken by the Baidu API.
Many machine learning models are used for classification. The task of the models is to detect the transport modes using key feature sets, with the entire process being supervised. Python and open-source libraries were used for all these models, and the parameters were transparent during the detection process.
Nonetheless, it is unclear which model is the best for our detection. Therefore, we tested several machine learning models. Specifically, we used logistic regression (LogisticReg), SVM, KNN, DT and RF. All the models were applied using the scikit-learn open-source library. In addition, we used artificial neural networks (ANN) [63], XGBoost [50], CatBoost [51] and DF [53]. Moreover, one or more hyperparameters must be optimized to achieve the best performance for each model. We tuned each model to the optimal parameters using a grid search to avoid the problem of unfairness due to improperly set hyperparameters. Unless otherwise stated, the default hyperparameter configuration of the respective models was maintained.
To verify the performance of the fine-grained detection models for the transport modes, we used the confusion matrix to calculate the number of true positives (TP), true negatives (TN), false positives (FP) and false negatives (FN). Then, we calculated four performance metrics through the classification results, including accuracy, recall, precision and F1-measure [64]. The performance metrics are calculated using the following equation:
Accuracy=TP+TNTP+FP+TN+FN | (9) |
Precision=TPTP+FP | (10) |
Recall=TPTP+FN | (11) |
F1−measure=2TP2TP+FP+FN | (12) |
The dataset used in this study is collected in the Nanjing Transport Development White Paper project. The collection date was June 18, 2015. The survey randomly selected 22,191 participants from 8547 households in 11 administrative districts of Nanjing.
In this study, the dataset was cleaned because the scope of our research included intra-city transport modes. The cleaned dataset comprises a total of 43,039 trips, including 13,301 walking trips, 4692 bike trips, 10,300 e-bike trips, 4996 bus trips, 8135 car trips, 335 public transport trips (including transfers) and 1280 metro trips. Public transport encompasses a broad category, including both buses and metro transfers. By adopting this classification approach, we can better capture the diversity and nuances of travel behaviours, particularly concerning the usage of public transport involving bus and metro transfers. We only use trip information in the survey data, including coordinates of the trip origin, coordinates of the trip destination, time of the trip origin, time of the trip destination and the label of the transport mode. The extracted features of the proposed method are presented in Table 2. These features are used as inputs to the model. It is worth mentioning that the indicators of the calculation process are used as features, including →vix, →viy, →vcx, →vcy, →aix, →aiy, →acx and →acy.We used 80% of the dataset for training and 20% for testing.
Variables | Source of variables | Description | |
Continuous Variables | Mean | Std. | |
Longitude of trip origin | Collected | 118.801758 | 0.077547 |
Latitude of trip origin | Collected | 32.025352 | 0.156764 |
Longitude of trip destination | Collected | 118.801786 | 0.077544 |
Latitude of trip destination | Collected | 32.025306 | 0.156759 |
Time of trip origin (hours, minutes) | Collected | 11.63, 16.04 | 4.68, 16.70 |
Time of trip destination (hours, minutes) | Calculated | 11.92, 24.71 | 4.69, 16.82 |
Trip duration (h) | Calculated | 0.44 | 0.30 |
Distance li (km) | Calculated | 4.30 | 6.60 |
GDP of administrative districts | Collected | 781.53 | 336.50 |
Population of the administrative district | Collected | 90.16 | 30.12 |
Center of mass →cm | Calculated | 13224.95, 3766.65 | 8281.63, 20296.36 |
Velocity of COM vc (km/h) | Calculated | 8.30 | 8.56 |
Acceleration of COM ac (km/h2) | Calculated | 24.15 | 28.40 |
Radius of gyration rg (km) | Calculated | 2.28 | 3.33 |
Entropy index ENT | Calculated | 0.46 | 0.35 |
Categorical variables | |||
Transport modes determined by Baidu API | From Baidu API | Car; walk; bike; e-bike; bus; metro; public transport (including transfers) |
Many studies have determined the radius of gyration distribution P(rg) using a truncated power-law statistic for all individuals to explore the statistical properties of individual trip features [65].We also used statistics to distribute the mobility of individuals in Nanjing:
P(rg)=(rg+r0g)−βrexp(−rg/−rgkrkr) | (13) |
where r0g = 0.91, βr = 0.81, kr = 8. The r-squared statistic is used to show how well the data fit the regression model. The r-squared statistic is calculated as follows:
r2=1−∑i(yi−ˆyi)2∑i(yi−ˉyi)2 | (14) |
where yi is the observed values of the data. ˆyi represents the predicted values by the model. ˉyi represents the mean of the observed values.
The r-squared statistic for the truncated power-law function mentioned above is 0.999. It is evident that the radius of gyration decreases rapidly from 1 to 10 km, and the long tail part shows a discrete state, as shown in Figure 3. This phenomenon indicates that the daily activities of most individuals were limited to from 1 to 10 km. The share of long-distance travelers was relatively low.
Meanwhile, we counted the velocity and acceleration of the COM introduced in this study to discover the patterns. Surprisingly, the distribution is also very close to the truncated power-law:
P(vc)=(vc+v0c)−βvexp(−vc/−vckvkv) | (15) |
P(ac)=(ac+a0c)−βaexp(−ac/−ackaka) | (16) |
where v0c = 1954.9, βv = -0.015, kv = 7.03, r-squared = 0.998, a0c = 0.9, βa = -0.11, ka = 16.4 and r-squared = 0.997. As previously depicted in Figures 4 and 5, slow-speed transport modes account for a relatively large proportion of all transport modes, whereas the acceleration of all travel modes is low. The main reason is that the study only considers intra-city transport modes excluding high-speed rail and airplanes, resulting in a lower velocity of the COM.
In order to validate the detection performance of various machine learning models, experiments were conducted using travel survey data from Nanjing, China in 2015. The primary focus of these experiments was to assess the accuracy and effectiveness of these models in transport mode detection. For the most promising model in terms of performance, further analysis was performed to provide detailed detection results for each transport mode, aiming to explore the reasons for any shortcomings in the detection performance of model. Furthermore, we explored the interpretability of the model, examining its role in the process of transport mode detection.
The four standard metrics used to assess the performance of the detection process are accuracy, recall, precision and the F1-measure. The F1-measure is the harmonic average of precision and recall. Hence, the accuracy and F1-measure were selected as performance metrics in this study. Meanwhile, we selected several machine learning models for comparison. These models include logisticReg, SVM, KNN, DT, RF, ANN, CatBoost, XGBoost and DF. We also compared the results obtained directly from the Baidu API for detecting transport modes. The SVM used a radial basis function kernel to obtain the best results, and the cascade layer of the deep forest was automatically fitted to four layers. We used five layers (including three hidden layers) in the ANN to detect transport modes. The ANN includes 28 input neurons and 7 output neurons. Figure 6 shows the performance of all models.
Table 3 demonstrates that the deep forest model achieves the best detection results among all the models, with an accuracy and F1-measure of approximately 0.82 and 0.74, respectively. While the RF model also performs well, it does not match the performance of the deep forest model. Our results suggest that the deep forest may have better applicability to our proposed detection model and further supports our decision to use the deep forest model for the remainder of the study.
Models | Accuracy | Precision | Recall | F1-measure |
Baidu API | 0.45 | 0.37 | 0.39 | 0.35 |
logisticReg | 0.58 | 0.35 | 0.34 | 0.31 |
SVM | 0.64 | 0.43 | 0.73 | 0.41 |
KNN | 0.68 | 0.6 | 0.56 | 0.58 |
DT | 0.71 | 0.71 | 0.71 | 0.71 |
RF | 0.8 | 0.67 | 0.83 | 0.73 |
ANN | 0.63 | 0.52 | 0.64 | 0.56 |
CatBoost | 0.74 | 0.62 | 0.72 | 0.65 |
XGBoost | 0.75 | 0.64 | 0.76 | 0.68 |
DF | 0.82 | 0.82 | 0.7 | 0.74 |
In the previous experiments, we evaluated the performance of several machine learning models. These results suggest that the deep forest model is an effective and robust machine learning model for detecting fine-grained transport modes. The specific detection results for each transport mode were output further to explore the reasons for the imperfect detection performance of the DF model. The results are presented in Figure 6.
As seen in Figure 6, the performance of detecting walking is the best for either performance metric. The walking speed is lower than that of other transport modes, resulting in better detection through the velocity of the COM. Meanwhile, public transport has the worst detection performance, with an accuracy of only 0.31. Public transport may involve a transfer process that includes waiting and transfer times. Moreover, interchange transport modes are unknown, and detecting public transport (including transfers) is the most challenging.
In fact, it is unclear which individual transport mode is actually misidentified in the data or which features are more important. The reason is due to the need for more interpretability of machine learning models. We can find the reason for the detection error and improve detection accuracy if a model can capture the misidentification among different modes or obtain the importance ranking of features. Therefore, we performed t-SNE and ablation experiments to overcome the non-interpretability issue of the machine learning models. We also analysed the confusion matrix of the model.
First, we extracted augmented features of the deep forest detection process; there were four layers of features in the detection process of this study in total. They are challenging to understand and visualize because their features are highly dimensional. t-SNE, which is used for high-dimensional feature visualization, is used to reduce the dimensionality of the features [36,66] achieved by minimizing the Kullback-Leibler divergence between a joint probability distribution in high and low dimensional spaces. High-dimensional data are eventually mapped into 2D, which is more suitable for human observation. A visualization of t-SNE is shown in Figure 7. Subsequently, we extracted the confusion matrix of the model, as shown in Table 4.
Actual result | ||||||||
walk | bike | e-bike | bus | car | public transport | metro | ||
Predicted Result | walk | 2578 | 26 | 47 | 18 | 10 | 0 | 0 |
bike | 133 | 637 | 90 | 33 | 30 | 0 | 1 | |
e-bike | 154 | 43 | 1642 | 75 | 129 | 0 | 9 | |
bus | 38 | 11 | 98 | 750 | 127 | 1 | 8 | |
car | 35 | 12 | 158 | 77 | 1303 | 5 | 7 | |
public transport | 0 | 0 | 2 | 13 | 21 | 24 | 2 | |
metro | 1 | 1 | 26 | 28 | 60 | 2 | 143 |
Figure 7 and Table 4 illustrate the reasons for the imperfect detection of transport modes by the deep forest model. As depicted in the figure, some of the green, orange and blue-green dots are heavily intermingled. This is consistent with the results of the confusion matrix. This phenomenon means that the detection of cars, e-bikes and buses is biased. One potential explanation is that their travel speeds are not significantly different when urban traffic congestion occurs, resulting in inaccurate detection. Additionally, some of the red and yellow dots are also mixed, indicating a biased detection of walking and bikes due to similar travel speeds. The biased detection of transport modes is a result of the slight speed differences during actual travel process. Therefore, the results indicate that, although the velocity of the COM introduced by the paper can substantially enhance the detection performance, further improvements are necessary in the detection of details.
To further investigate the effectiveness of each feature and obtain the importance ranking of a feature, we designed an ablation experiment (including four features and two multi-source data) [67,68]. The results of the experiments are listed in Table 5. ENT and OMS respectively represent the entropy index of land use and the travel modes recognized by the Baidu API. The checked ones in the table are the reduced features. With each feature reduction, we kept the rest of the features and compared the performance metrics between the current model and our model. This step allows for an explicit analysis of the features that more significantly impact mode detection.
Features | Performance metrics | ||||||||
→cm | rg | vc | ac | ENT | OMS | Accuracy | Precision | Recall | F1-Measure |
Ours | 0.82 | 0.82 | 0.70 | 0.74 | |||||
√ | -0.02 | -0.02 | - | -0.01 | |||||
√ | -0.01 | -0.02 | -0.01 | -0.01 | |||||
√ | -0.04 | -0.03 | -0.05 | -0.04 | |||||
√ | -0.07 | -0.04 | -0.06 | -0.06 | |||||
√ | -0.01 | -0.01 | -0.02 | -0.02 | |||||
√ | -0.02 | -0.02 | -0.02 | -0.02 |
The accuracy decreases by 0.07 when the velocity of the COM is ignored. The second important feature is the acceleration of the COM, which decreases the accuracy by 0.04. The results directly indicate that the two mobility features introduced in this study play crucial roles in mode detection. Additionally, there is a relationship between transport mode and gyration radius, indicating that travel distance influences the choice of transport mode. Thus, individual mobility features are the most critical features that improve the detection performance, enhancing accuracy by 0.14. Furthermore, the land use mix improves the accuracy of detection by 0.01, implying little correlation between the urban land use mix and the choice of transport modes. The potential reason may be that the dataset of our study includes weekdays and the effect of the land use mix is restricted [69].
In summary, our proposed model exhibits several advantages. Firstly, the DF model has demonstrated exceptional performance in accurately detecting between seven different transport modes, exhibiting a superior performance compared to alternative models. The high detection performance of the DF model emphasizes the model's robustness in effectively detecting various transport modes. Additionally, the DF model performs well in terms of interpretability, providing valuable insights into the factors that influence individuals' mode choices. However, the model also has some disadvantages. Specifically, the model faces challenges when it comes to detecting public transport modes, particularly those involving transfers. Furthermore, the influence of land use mix on the detection of the model is relatively modest. In conclusion, there is the potential for further research in the future.
Precisely detecting various transport modes of individuals provides valuable insights for transportation management authorities. Tailored policies can be formulated to encourage the adoption of sustainable and low-carbon transportation modes for groups with specific transport modes, such as public transit, cycling and walking. This proactive approach helps address growing urban transportation challenges. For example, managers can adjust transportation policies, including fare structures, service frequencies and accessibility to offer more appealing public transportation options. This approach can help reduce urban traffic emissions, relieve congestion issues and promote the overall sustainability of cities.
Meanwhile, recent research has witnessed a surge in the development of innovative approaches for data collection, especially Global Positioning System (GPS) loggers or smartphone travel surveys. Once we detect individual transport modes through our proposed method, these approaches eliminate the necessity for extensive follow-up surveys or direct participant interactions, making data collection more efficient. Moreover, these data collection approaches emphasize the potential of smartphone and GPS travel surveys in providing highly precise transport mode data while preserving participant privacy. Managers can actively embrace these innovative data collection methods to better understand individuals' travel behavior, guide policy formulation and inform transportation planning.
Analysis of transport mode choice is a crucial element in developing and evaluating planning policies. Though many kinds of factors influence the transport mode choice of the individual, many of these factors involve the privacy of individuals. Meanwhile, detection models of transport modes widely use discrete choice models. In order to improve the limitations of existing discrete choice models, we propose an interpretable machine learning model for detecting transport modes by multi-source data. The mobility features of individuals, which innovatively introduce the velocity and acceleration of the center of mass (COM), are considered in the detection model. We combine these features with multi-source data as detection features and apply machine learning models to detect transport modes at the fine-grained level, which were divided into seven categories.
Four performance metrics are calculated through the classification results, including accuracy, recall, precision and F1-measure. The results show that the deep forest algorithm exhibits the best detection performance, achieving a detection accuracy of 0.82 in the test dataset. Meanwhile, recall, precision and F1-measure also achieved optimal performance. The evaluation shows that the detection performance for other transport modes is excellent apart from public transport detection. Compared to previous work, this study is a significant step forward in terms of granularity and detection performance. To address the issue of non-interpretability of machine learning models, we also perform t-distributed stochastic neighbor embedding (t-SNE) and ablation experiments and rank the importance of features. These experiments demonstrate a weak correlation between the land use mix and the choice of transportation modes. Moreover, the most critical features are the individual mobility features in the study, and the accuracy improves by 0.13.
Meanwhile, recent research has witnessed a surge in the development of innovative approaches for data collection, especially Global Positioning System (GPS) loggers or smartphone travel surveys. They leverage these travel surveys to collect accurate travel behavior details without involving the collection of individual and household attribute data. Our proposed approach enhances the potential of these data collection methods in maintaining privacy protection for survey participants without the need for extensive follow-up surveys. On the other hand, if individual travel modes can be accurately detected, transportation government agencies can intervene by targeting specific groups with specific transport modes and effectively promote sustainable and low-carbon transportation options. The results of the study can guide government transportation agencies in formulating effective policies to achieve long-term sustainable and efficient urban transportation goals.
In future research, the utilization of multi-source data will be further expanded to enhance the performance and applicability of the proposed model. The expansion may involve incorporating additional data, such as real-time traffic information, weather conditions or other factors that can influence transport mode choice. The objective is to continuously improve the accuracy and reliability of the model in detecting transport modes, and its effectiveness in guiding transportation policies and addressing urban transportation challenges.
The authors declare that they have not used Artificial Intelligence (AI) tools in the creation of this article.
This work was supported by the Postgraduate Research & Practice Innovation Program of Jiangsu Province (Grant No. SJCX22_0061); the General Project of National Natural Science Foundation of China (Grant No. 52172317); and the Key Program of National Natural Science Foundation of China (Grant No. 52131203).
The authors declare there is no conflict of interest.
[1] | WHO, Global Health Observatory (GHO) data. HIV/AIDS, 2018. Available from: http://www.who.int/gho/hiv/en/. |
[2] | M. A. Nowak, R. M. May, Virus dynamics: Mathematical principles of immunology and virology, Oxford University Press, 2001. |
[3] |
M. A. Nowak, C. R. M. Bangham, Population dynamics of immune responses to persistent viruses, Science, 272 (1996), 74-79. doi: 10.1126/science.272.5258.74
![]() |
[4] |
K. D. Pedro, A. J. Henderson, L. M. Agosto, Mechanisms of HIV-1 cell-to-cell transmission and the establishment of the latent reservoir, Virus Res., 265 (2019), 115-121. doi: 10.1016/j.virusres.2019.03.014
![]() |
[5] | L. Rong, A. S. Perelson, Modeling latently infected cell activation: viral and latent reservoir persistence, and viral blips in HIV-infected patients on potent therapy, PLoS Comput. Biol., 5 (2009), 1-18. |
[6] |
D. S. Callaway, A. S. Perelson, HIV-1 infection and low steady state viral loads, Bull. Math. Biol., 64 (2002), 29-64. doi: 10.1006/bulm.2001.0266
![]() |
[7] |
A. M. Elaiw, S. A. Azoz, Global properties of a class of HIV infection models with Beddington-DeAngelis functional response, Math. Method. Appl. Sci., 36 (2013), 383-394. doi: 10.1002/mma.2596
![]() |
[8] |
A. M. Elaiw, M. A. Alshaikh, Stability of discrete-time HIV dynamics models with three categories of infected CD4+ T-cells, Adv. Differ. Equ., 2019 (2019), 1-24. doi: 10.1186/s13662-018-1939-6
![]() |
[9] |
A. M. Elaiw, N. H. AlShamrani, Stability of a general CTL-mediated immunity HIV infection model with silent infected cell-to-cell spread, Adv. Differ. Equ., 2020 (2020), 1-25. doi: 10.1186/s13662-019-2438-0
![]() |
[10] |
A. M. Elaiw, E. K. Elnahary, A. A. Raezah, Effect of cellular reservoirs and delays on the global dynamics of HIV, Adv. Differ. Equ., 2018 (2018), 1-36. doi: 10.1186/s13662-017-1452-3
![]() |
[11] |
B. Buonomo, C. Vargas-De-Leon, Global stability for an HIV-1 infection model including an eclipse stage of infected cells, J. Math. Anal. Appl., 385 (2012), 709-720. doi: 10.1016/j.jmaa.2011.07.006
![]() |
[12] |
H. Liu, J. F. Zhang, Dynamics of two time delays differential equation model to HIV latent infection, Physica A, 514 (2019), 384-395. doi: 10.1016/j.physa.2018.09.087
![]() |
[13] | S. Iwami, J. S. Takeuchi, S. Nakaoka, F. Mammano, F. Clavel, H. Inaba, et al. Cell-to-cell infection by HIV contributes over half of virus infection, eLife, 4 (2015), 1-16. |
[14] |
M. Sourisseau, N. Sol-Foulon, F. Porrot, F. Blanchet, O. Schwartz, Inefficient human immunodeficiency virus replication in mobile lymphocytes, J. Virol., 81 (2007), 1000-1012. doi: 10.1128/JVI.01629-06
![]() |
[15] |
A. Sigal, J. T. Kim, A. B. Balazs, E. Dekel, A. Mayo, R. Milo, et al. Cell-to-cell spread of HIV permits ongoing replication despite antiretroviral therapy, Nature, 477 (2011), 95-98. doi: 10.1038/nature10347
![]() |
[16] |
Y. Gao, J. Wang, Threshold dynamics of a delayed nonlocal reaction-diffusion HIV infection model with both cell-free and cell-to-cell transmissions, J. Math. Anal. Appl., 488 (2020), 124047. doi: 10.1016/j.jmaa.2020.124047
![]() |
[17] | A. Mojaver, H. Kheiri, Mathematical analysis of a class of HIV infection models of CD4+T-cells with combined antiretroviral therapy, Appl. Math. Comput., 259 (2015), 258-270. |
[18] |
X. Wang, S. Tang, X. Song, L. Rong, Mathematical analysis of an HIV latent infection model including both virus-to-cell infection and cell-to-cell transmission, J. Biol. Dynam., 11 (2017), 455-483. doi: 10.1080/17513758.2016.1242784
![]() |
[19] |
H. R. Norrgren, S. Bamba, O. Larsen, Z. Da Silva, P. Aaby, T. Koivula, et al. Increased prevalence of HTLV-1 in patients with pulmonary tuberculosis coinfected with HIV, but not in HIV-negative patients with tuberculosis, J. Acq. Imm. Def., 48 (2008), 607-610. doi: 10.1097/QAI.0b013e31817efb83
![]() |
[20] |
A. G. Lim, P. K. Maini, HTLV-Iinfection: A dynamic struggle between viral persistence and host immunity, J. Theor. Biol., 352 (2014), 92-108. doi: 10.1016/j.jtbi.2014.02.022
![]() |
[21] |
X. Pan, Y. Chen, H. Shu, Rich dynamics in a delayed HTLV-I infection model: Stability switch, multiple stable cycles, and torus, J. Math. Anal. Appl., 479 (2019), 2214-2235. doi: 10.1016/j.jmaa.2019.07.051
![]() |
[22] |
M. Y. Li, A. G. Lim, Modelling the role of Tax expression in HTLV-1 persisence in vivo, Bull. Math. Biol., 73 (2011), 3008-3029. doi: 10.1007/s11538-011-9657-1
![]() |
[23] |
B. Asquith, C. R. M. Bangham, The dynamics of T-cell fratricide: application of a robust approach to mathematical modeling in immunology, J. Theor. Biol., 222 (2003), 53-69. doi: 10.1016/S0022-5193(03)00013-4
![]() |
[24] | S. Tokudome et al., Incidence of adult T cell leukemia/lymphoma among human T lymphotropic virus type 1 carriers in Saga, Japan, Cancer Res., 49 (1989), 226-228. |
[25] |
N. I. Stilianakis, J. Seydel, Modeling the T-cell dynamics and pathogenesis of HTLV-I infection, Bull. Math. Biol., 61 (1999), 935-947. doi: 10.1006/bulm.1999.0117
![]() |
[26] |
H. Gomez-Acevedo, M. Y. Li, Backward bifurcation in a model for HTLV-I infection of CD4+ T cells, Bull. Math. Biol., 67 (2005), 101-114. doi: 10.1016/j.bulm.2004.06.004
![]() |
[27] | C. Vargas-De-Leon, The complete classification for global dynamics of amodel for the persistence of HTLV-1 infection, Appl. Math. Comput., 237 (2014), 489-493. |
[28] | X. Song, Y. Li, Global stability and periodic solution of a model for HTLV-1 infection and ATL progression, Appl. Math. Comput., 180 (2006), 401-410. |
[29] |
E. S. Rosenberg, M. Altfeld, S. H. Poon, M. N. Phillips, B. M. Wilkes, R. L. Eldridge, et al., Immune control of HIV-1 following early treatment of acute infection, Nature, 407 (2000), 523-526. doi: 10.1038/35035103
![]() |
[30] |
A. M. Elaiw, N. H. AlShamrani, Global stability of a delayed adaptive immunity viral infection with two routes of infection and multi-stages of infected cells, Commun. Nonlinear Sci., 86 (2020), 105259. doi: 10.1016/j.cnsns.2020.105259
![]() |
[31] |
B. Asquith, C. R. M. Bangham, Quantifying HTLV-I dynamics, Immunol. Cell Biol., 85 (2007), 280-286. doi: 10.1038/sj.icb.7100050
![]() |
[32] |
C. Bartholdy, J. P. Christensen, D. Wodarz, A. R. Thomsen, Persistent virus infection despite chronic cytotoxic T-lymphocyte activation in gamma interferon-deficient mice infected with lymphocytic choriomeningitis virus, J. Virol., 74 (2000), 10304-10311. doi: 10.1128/JVI.74.22.10304-10311.2000
![]() |
[33] |
H. Gomez-Acevedo, M. Y. Li, S. Jacobson, Multi-stability in a model for CTL response to HTLV-I infection and its consequences Bull. Math. Biol., 72 (2010), 681-696. doi: 10.1007/s11538-009-9465-z
![]() |
[34] |
M. Y. Li, H. Shu, Multiple stable periodic oscillations in a mathematical model of CTL response to HTLV-I infection, Bull. Math. Biol., 73 (2011), 1774-1793. doi: 10.1007/s11538-010-9591-7
![]() |
[35] | L. Wang, Z. Liu, Y. Li, D. Xu, Complete dynamical analysis for a nonlinear HTLV-I infection model with distributed delay, CTL response and immune impairment, Discrete Cont. Dyn. B, 25 (2020), 917-933. |
[36] |
Y. Wang, J. Liu, J. M. Heffernan, Viral dynamics of an HTLV-I infection model with intracellular delay and CTL immune response delay, J. Math. Anal. Appl., 459 (2018), 506-527. doi: 10.1016/j.jmaa.2017.10.027
![]() |
[37] |
F. Li, W. Ma, Dynamics analysis of an HTLV-1 infection model with mitotic division of actively infected cells and delayed CTL immune response, Math. Method. Appl. Sci., 41 (2018), 3000-3017. doi: 10.1002/mma.4797
![]() |
[38] |
S. Li, Y. Zhou, Backward bifurcation of an HTLV-I model with immune response, Discrete Cont. Dyn. B, 21 (2016), 863-881. doi: 10.3934/dcdsb.2016.21.863
![]() |
[39] | C. Casoli, E. Pilotti, U. Bertazzoni, Molecular and cellular interactions of HIV-1/HTLV coinfection and impact on AIDS progression, AIDS Rev., 9 (2007), 140-149. |
[40] | E. Pilotti, M. V. Bianchi, A. De Maria, F. Bozzano, M. G. Romanelli, U. Bertazzoni, et al. HTLV-1/-2 and HIV-1 co-infections: retroviral interference on host immune status, Front. Microbiol., 4 (2013), 1-13. |
[41] |
M. A. Beilke, K. P. Theall, M. O'Brien, J. L. Clayton, S. M. Benjamin, E. L. Winsor, et al. Clinical outcomes and disease progression among patients coinfected with HIV and human T lymphotropic virus types 1 and 2, Clin. Infect. Dis., 39 (2004), 256-263. doi: 10.1086/422146
![]() |
[42] |
C. Isache, M. Sands, N. Guzman, D. Figueroa, HTLV-1 and HIV-1 co-infection: A case report and review of the literature, IDCases, 4 (2016), 53-55. doi: 10.1016/j.idcr.2016.03.002
![]() |
[43] | M. Tulius Silva, O. de Melo Espíndola, A. C. Bezerra Leite, A. Araújo, Neurological aspects of HIV/human T lymphotropic virus coinfection, AIDS Rev., 11 (2009), 71-78. |
[44] | N. Rockwood, L. Cook, H. Kagdi, S. Basnayake, C. R. M. Bangham, A. L. Pozniak, et al. Immune compromise in HIV-1/HTLV-1 coinfection with paradoxical resolution of CD4 lymphocytosis during antiretroviral therapy: A case report, Medicine, 94 (2015), 1-4. |
[45] | C. Brites, J. Sampalo, A. Oliveira, HIV/human T-cell lymphotropic virus coinfection revisited: impact on AIDS progression, AIDS Rev., 11 (2009), 8-16. |
[46] |
V. E. V. Geddes, D. P. José, F. E. Leal, D. F. Nixond, A. Tanuri, R. S. Aguiar, HTLV-1 Tax activates HIV-1 transcription in latency models, Virology, 504 (2017), 45-51. doi: 10.1016/j.virol.2017.01.014
![]() |
[47] |
R. Bingham, E. Dykeman, R. Twarock, RNA virus evolution via a quasispecies-based model reveals a drug target with a high barrier to resistance, Viruses, 9 (2017), 347. doi: 10.3390/v9110347
![]() |
[48] |
E. Ticona, M. A. Huaman, O. Yanque, J. R. Zunt, HIV and HTLV-1 coinfection: the need to initiate antiretroviral therapy, J. Int. Assoc. Provid. AIDS Care, 12 (2013), 373-374. doi: 10.1177/2325957413500988
![]() |
[49] | N. Bellomo, R. Bingham, M. A. J. Chaplain, G. Dosi, G. Forni, D. A. Knopoff, et al. A multi-scale model of virus pandemic: Heterogeneous interactive entities in a globally connected world, Math. Mod. Meth. Appl. S., 30 (2000), 1591-1651. |
[50] |
A. Korobeinikov, Global properties of basic virus dynamics models, Bull. Math. Biol., 66 (2004), 879-883. doi: 10.1016/j.bulm.2004.02.001
![]() |
[51] | E. A. Barbashin, Introduction to the theory of stability, Wolters-Noordhoff, 1970. |
[52] | J. P. LaSalle, The stability of dynamical systems, Philadelphia, SIAM, 1976. |
[53] |
A. M. Lyapunov, The general problem of the stability of motion, Int. J. Control, 55 (1992), 531-534. doi: 10.1080/00207179208934253
![]() |
[54] | A. Vandormael, F. Rego, S. Danaviah, L. Carlos Junior Alcantara, D. R. Boulware, T. de Oliveira, CD4+ T-cell count may not be a useful strategy to monitor antiretroviral therapy response in HTLV-1/HIV co-infected patients, Curr. HIV Res., 15 (2017), 225-231. |
[55] |
T. Inoue, T. Kajiwara, T. Saski, Global stability of models of humoral immunity against multiple viral strains, J. Biol. Dynam., 4 (2010), 282-295. doi: 10.1080/17513750903180275
![]() |
[56] |
G. Huang, Y. Takeuchi, W. Ma, Lyapunov functionals for delay differential equations model of viral infections, SIAM J. Appl. Math., 70 (2010), 2693-2708. doi: 10.1137/090780821
![]() |
[57] |
A. M. Elaiw, I. A. Hassanien, S. A. Azoz, Global stability of HIV infection models with intracellular delays, J. Korean Math. Soc., 49 (2012), 779-794. doi: 10.4134/JKMS.2012.49.4.779
![]() |
[58] |
A. M. Elaiw, S. F. Alshehaiween, Global stability of delay-distributed viral infection model with two modes of viral transmission and B-cell impairment, Math. Method. Appl. Sci., 43 (2020), 6677-6701. doi: 10.1002/mma.6408
![]() |
[59] |
P. W. Nelson, J. D. Murray, A. S. Perelson, A model of HIV-1 pathogenesis that includes an intracellular delay, Math. Biosci., 163 (2000), 201-215. doi: 10.1016/S0025-5564(99)00055-3
![]() |
[60] |
A. M. Elaiw, A. A. Raezah, Stability of general virus dynamics models with both cellular and viral infections and delays, Math. Method. Appl. Sci., 40 (2017), 5863-5880. doi: 10.1002/mma.4436
![]() |
[61] |
A. M. Elaiw, N. A. Almuallem, Global dynamics of delay-distributed HIV infection models with differential drug efficacy in cocirculating target cells, Math. Method. Appl. Sci., 39 (2016), 4-31. doi: 10.1002/mma.3453
![]() |
[62] | A. M. Elaiw, S. F. Alshehaiween, A. D. Hobiny, Global properties of a delay-distributed HIV dynamics model including impairment of B-cell functions, Mathematics, 7 (2019), 1-27. |
[63] | A. M. Elaiw, E. K. Elnahary, Analysis of general humoral immunity HIV dynamics model with HAART and distributed delays, Mathematics, 7 (2019), 1-35. |
[64] |
R. V. Culshaw, S. Ruan, G. Webb, A mathematical model of cell-to-cell spread of HIV-1 that includes a time delay, J. Math. Biol., 46 (2003), 425-444. doi: 10.1007/s00285-002-0191-5
![]() |
[65] |
N. Bellomo, K. J. Painter, Y. Tao, M. Winkler, Occurrence vs. Absence of taxis-driven instabilities in a May-Nowak model for virus infection, SIAM J. Appl. Math., 79 (2019), 1990-2010. doi: 10.1137/19M1250261
![]() |
[66] |
A. M. Elaiw, A. D. AlAgha, Global analysis of a reaction-diffusion within-host malaria infection model with adaptive immune response, Mathematics, 8 (2020), 1-32. doi: 10.3390/math8101793
![]() |
Parameters | Value | Description |
Origin | 32.048245,118.796519 | Trip origin (Latitude, longitude) |
Destination | 32.052371,118.890583 | Trip destination (Latitude, longitude) |
Coord_type | WGS84 | Type of geographic coordinate system |
Departure_date | 20150618 | Date of trip origin |
Departure_time | 08:00 | Time of trip origin |
Tactics_incity | 0 | 0: Recommended mode; 1: Minimal interchanges mode; 2: Minimal walking mode; 3: No metro mode; 4: Short time mode; 5: Metro priority mode. |
URL | https://api.map.baidu.com/direction/v2/transit? |
Variables | Source of variables | Description | |
Continuous Variables | Mean | Std. | |
Longitude of trip origin | Collected | 118.801758 | 0.077547 |
Latitude of trip origin | Collected | 32.025352 | 0.156764 |
Longitude of trip destination | Collected | 118.801786 | 0.077544 |
Latitude of trip destination | Collected | 32.025306 | 0.156759 |
Time of trip origin (hours, minutes) | Collected | 11.63, 16.04 | 4.68, 16.70 |
Time of trip destination (hours, minutes) | Calculated | 11.92, 24.71 | 4.69, 16.82 |
Trip duration (h) | Calculated | 0.44 | 0.30 |
Distance li (km) | Calculated | 4.30 | 6.60 |
GDP of administrative districts | Collected | 781.53 | 336.50 |
Population of the administrative district | Collected | 90.16 | 30.12 |
Center of mass →cm | Calculated | 13224.95, 3766.65 | 8281.63, 20296.36 |
Velocity of COM vc (km/h) | Calculated | 8.30 | 8.56 |
Acceleration of COM ac (km/h2) | Calculated | 24.15 | 28.40 |
Radius of gyration rg (km) | Calculated | 2.28 | 3.33 |
Entropy index ENT | Calculated | 0.46 | 0.35 |
Categorical variables | |||
Transport modes determined by Baidu API | From Baidu API | Car; walk; bike; e-bike; bus; metro; public transport (including transfers) |
Models | Accuracy | Precision | Recall | F1-measure |
Baidu API | 0.45 | 0.37 | 0.39 | 0.35 |
logisticReg | 0.58 | 0.35 | 0.34 | 0.31 |
SVM | 0.64 | 0.43 | 0.73 | 0.41 |
KNN | 0.68 | 0.6 | 0.56 | 0.58 |
DT | 0.71 | 0.71 | 0.71 | 0.71 |
RF | 0.8 | 0.67 | 0.83 | 0.73 |
ANN | 0.63 | 0.52 | 0.64 | 0.56 |
CatBoost | 0.74 | 0.62 | 0.72 | 0.65 |
XGBoost | 0.75 | 0.64 | 0.76 | 0.68 |
DF | 0.82 | 0.82 | 0.7 | 0.74 |
Actual result | ||||||||
walk | bike | e-bike | bus | car | public transport | metro | ||
Predicted Result | walk | 2578 | 26 | 47 | 18 | 10 | 0 | 0 |
bike | 133 | 637 | 90 | 33 | 30 | 0 | 1 | |
e-bike | 154 | 43 | 1642 | 75 | 129 | 0 | 9 | |
bus | 38 | 11 | 98 | 750 | 127 | 1 | 8 | |
car | 35 | 12 | 158 | 77 | 1303 | 5 | 7 | |
public transport | 0 | 0 | 2 | 13 | 21 | 24 | 2 | |
metro | 1 | 1 | 26 | 28 | 60 | 2 | 143 |
Features | Performance metrics | ||||||||
→cm | rg | vc | ac | ENT | OMS | Accuracy | Precision | Recall | F1-Measure |
Ours | 0.82 | 0.82 | 0.70 | 0.74 | |||||
√ | -0.02 | -0.02 | - | -0.01 | |||||
√ | -0.01 | -0.02 | -0.01 | -0.01 | |||||
√ | -0.04 | -0.03 | -0.05 | -0.04 | |||||
√ | -0.07 | -0.04 | -0.06 | -0.06 | |||||
√ | -0.01 | -0.01 | -0.02 | -0.02 | |||||
√ | -0.02 | -0.02 | -0.02 | -0.02 |
Parameters | Value | Description |
Origin | 32.048245,118.796519 | Trip origin (Latitude, longitude) |
Destination | 32.052371,118.890583 | Trip destination (Latitude, longitude) |
Coord_type | WGS84 | Type of geographic coordinate system |
Departure_date | 20150618 | Date of trip origin |
Departure_time | 08:00 | Time of trip origin |
Tactics_incity | 0 | 0: Recommended mode; 1: Minimal interchanges mode; 2: Minimal walking mode; 3: No metro mode; 4: Short time mode; 5: Metro priority mode. |
URL | https://api.map.baidu.com/direction/v2/transit? |
Variables | Source of variables | Description | |
Continuous Variables | Mean | Std. | |
Longitude of trip origin | Collected | 118.801758 | 0.077547 |
Latitude of trip origin | Collected | 32.025352 | 0.156764 |
Longitude of trip destination | Collected | 118.801786 | 0.077544 |
Latitude of trip destination | Collected | 32.025306 | 0.156759 |
Time of trip origin (hours, minutes) | Collected | 11.63, 16.04 | 4.68, 16.70 |
Time of trip destination (hours, minutes) | Calculated | 11.92, 24.71 | 4.69, 16.82 |
Trip duration (h) | Calculated | 0.44 | 0.30 |
Distance li (km) | Calculated | 4.30 | 6.60 |
GDP of administrative districts | Collected | 781.53 | 336.50 |
Population of the administrative district | Collected | 90.16 | 30.12 |
Center of mass →cm | Calculated | 13224.95, 3766.65 | 8281.63, 20296.36 |
Velocity of COM vc (km/h) | Calculated | 8.30 | 8.56 |
Acceleration of COM ac (km/h2) | Calculated | 24.15 | 28.40 |
Radius of gyration rg (km) | Calculated | 2.28 | 3.33 |
Entropy index ENT | Calculated | 0.46 | 0.35 |
Categorical variables | |||
Transport modes determined by Baidu API | From Baidu API | Car; walk; bike; e-bike; bus; metro; public transport (including transfers) |
Models | Accuracy | Precision | Recall | F1-measure |
Baidu API | 0.45 | 0.37 | 0.39 | 0.35 |
logisticReg | 0.58 | 0.35 | 0.34 | 0.31 |
SVM | 0.64 | 0.43 | 0.73 | 0.41 |
KNN | 0.68 | 0.6 | 0.56 | 0.58 |
DT | 0.71 | 0.71 | 0.71 | 0.71 |
RF | 0.8 | 0.67 | 0.83 | 0.73 |
ANN | 0.63 | 0.52 | 0.64 | 0.56 |
CatBoost | 0.74 | 0.62 | 0.72 | 0.65 |
XGBoost | 0.75 | 0.64 | 0.76 | 0.68 |
DF | 0.82 | 0.82 | 0.7 | 0.74 |
Actual result | ||||||||
walk | bike | e-bike | bus | car | public transport | metro | ||
Predicted Result | walk | 2578 | 26 | 47 | 18 | 10 | 0 | 0 |
bike | 133 | 637 | 90 | 33 | 30 | 0 | 1 | |
e-bike | 154 | 43 | 1642 | 75 | 129 | 0 | 9 | |
bus | 38 | 11 | 98 | 750 | 127 | 1 | 8 | |
car | 35 | 12 | 158 | 77 | 1303 | 5 | 7 | |
public transport | 0 | 0 | 2 | 13 | 21 | 24 | 2 | |
metro | 1 | 1 | 26 | 28 | 60 | 2 | 143 |
Features | Performance metrics | ||||||||
→cm | rg | vc | ac | ENT | OMS | Accuracy | Precision | Recall | F1-Measure |
Ours | 0.82 | 0.82 | 0.70 | 0.74 | |||||
√ | -0.02 | -0.02 | - | -0.01 | |||||
√ | -0.01 | -0.02 | -0.01 | -0.01 | |||||
√ | -0.04 | -0.03 | -0.05 | -0.04 | |||||
√ | -0.07 | -0.04 | -0.06 | -0.06 | |||||
√ | -0.01 | -0.01 | -0.02 | -0.02 | |||||
√ | -0.02 | -0.02 | -0.02 | -0.02 |