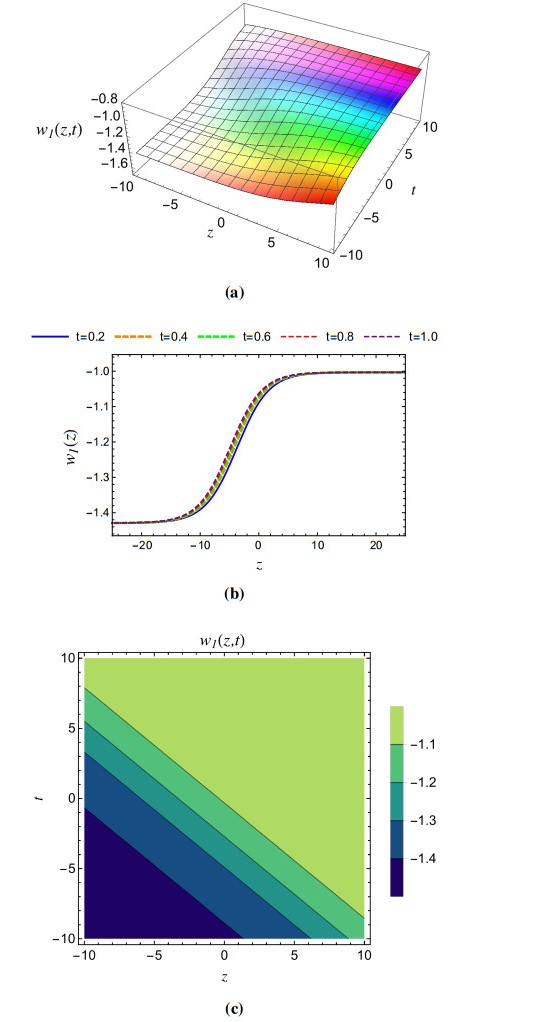
Citation: F. Z. Geng. Piecewise reproducing kernel-based symmetric collocation approach for linear stationary singularly perturbed problems[J]. AIMS Mathematics, 2020, 5(6): 6020-6029. doi: 10.3934/math.2020385
[1] | Feng Wang, Taotao Li . Dynamics of a stochastic epidemic model with information intervention and vertical transmission. Electronic Research Archive, 2024, 32(6): 3700-3727. doi: 10.3934/era.2024168 |
[2] | Wenxuan Li, Suli Liu . Dynamic analysis of a stochastic epidemic model incorporating the double epidemic hypothesis and Crowley-Martin incidence term. Electronic Research Archive, 2023, 31(10): 6134-6159. doi: 10.3934/era.2023312 |
[3] | Zhimin Li, Tailei Zhang, Xiuqing Li . Threshold dynamics of stochastic models with time delays: A case study for Yunnan, China. Electronic Research Archive, 2021, 29(1): 1661-1679. doi: 10.3934/era.2020085 |
[4] | E. A. Abdel-Rehim . The time evolution of the large exponential and power population growth and their relation to the discrete linear birth-death process. Electronic Research Archive, 2022, 30(7): 2487-2509. doi: 10.3934/era.2022127 |
[5] | Wenjie Zuo, Mingguang Shao . Stationary distribution, extinction and density function for a stochastic HIV model with a Hill-type infection rate and distributed delay. Electronic Research Archive, 2022, 30(11): 4066-4085. doi: 10.3934/era.2022206 |
[6] | Meng Gao, Xiaohui Ai . A stochastic Gilpin-Ayala nonautonomous competition model driven by mean-reverting OU process with finite Markov chain and Lévy jumps. Electronic Research Archive, 2024, 32(3): 1873-1900. doi: 10.3934/era.2024086 |
[7] | Yang Song, Beiyan Yang, Jimin Wang . Stability analysis and security control of nonlinear singular semi-Markov jump systems. Electronic Research Archive, 2025, 33(1): 1-25. doi: 10.3934/era.2025001 |
[8] | Wenhui Niu, Xinhong Zhang, Daqing Jiang . Dynamics and numerical simulations of a generalized mosquito-borne epidemic model using the Ornstein-Uhlenbeck process: Stability, stationary distribution, and probability density function. Electronic Research Archive, 2024, 32(6): 3777-3818. doi: 10.3934/era.2024172 |
[9] | Gaohui Fan, Ning Li . Application and analysis of a model with environmental transmission in a periodic environment. Electronic Research Archive, 2023, 31(9): 5815-5844. doi: 10.3934/era.2023296 |
[10] | Xiangyun Shi, Dan Zhou, Xueyong Zhou, Fan Yu . Predicting the trend of leptospirosis in China via a stochastic model with vector and environmental transmission. Electronic Research Archive, 2024, 32(6): 3937-3951. doi: 10.3934/era.2024176 |
In the fields of physics, chemistry, biology, and even social science, nonlinear partial differential equations (NPDEs) are frequently employed to represent a wide range of events. When exact solutions are available, they can be used to help understand nonlinear events. One of the most crucial and vital tasks in nonlinear science is finding and creating exact solutions, especially solitons, to NPDEs. Exact solutions in mathematics and physics are highly valued as they provide insights into the behavior of complex systems. Soliton solutions are a type of exact solution that represents solitary waves that maintain their shape and speed even after collisions with other solitons. Wave solutions, on the other hand, represent periodic or quasi-periodic waves that propagate through space with a certain frequency and amplitude. They are often used to model wave phenomena in various fields, such as fluid dynamics, electromagnetics, and acoustics. Lump solutions are another type of exact solution that represents localized, non-dispersive structures that do not spread out over time. They are often used to model coherent structures in various fields, such as turbulence, plasma physics, and nonlinear optics. The study of these exact solutions is important as they can provide valuable insights into the behavior of physical systems and aid in the development of mathematical models that can accurately predict the behavior of such systems [1,2,3,4].
To find exact solutions to NPDEs, numerous strong and effective techniques have recently been developed and enhanced. Among these strategies, we can highlight the homogeneous balance method [5,6], the tanh-function method [7], the extended tanh-function method [8], the Jacobi elliptic function method [9,10,11], the auxiliary equation method [12], invariant subspace method [13,14], and Lie symmetry method [15,16]. The following techniques have also been used to explore the analytical solutions of NPDEs: G′G− expansion method [17], the expansion function method and the modified exp(−Ω(φ))-expansion function method [18,19], the double sub-equation method [20,21], the F-expansion procedure [22] and many others.
In this study, we aim to use two powerful techniques to obtain new exact solutions to three nonlinear differential equations. The first technique we employ is Nucci's reduction method, which yields both generic implicit and explicit solutions for all the equations considered, as well as their reduced equations' first integrals. In addition to Nucci's method, we use the powerful GPREs method to create novel solutions to the equations.
The structure of this paper is organized as follows. Section 2 discusses the descriptions of the implied analytical methods. Section 3 summarizes the main findings of this paper, as well as the suggested strategies and analytical solutions. The final section includes a summary and conclusions.
In this section, we will briefly introduce the techniques used to find exact solutions to upcoming nonlinear differential equations.
To obtain exact solutions and first integrals, we use Nucci's reduction method. This technique was proposed for the first time in [23] to find nonlocal symmetries of differential equations. This method is used in the [24] for group analysis of the mathematical model for thin liquid films. The Nucci's reduction method is used to investigate evolution type differential equations with recently defined Atangana-Baleanu fractional derivatives in [25].
In the current paper, all reduced ODEs are second order nonlinear ODEs. Therefore, we can assume the governing reduced ODE as:
U″(ζ)=F(U(ζ),U′(ζ)). | (2.1) |
Assume we change variables according to the proposed reduction method [16,26,27]:
ϖ1(ζ)=U(ζ), ϖ2(ζ)=U′(ζ). | (2.2) |
The following system of ODEs can be obtained from the Eq (2.1) using these assumptions:
{ϖ′1=ϖ2, ϖ′2=F(ϖ1,ϖ2). | (2.3) |
If we choose ϖ1 as a new independent variable, then the system (2.3) is converted into:
dϖ2(ϖ1)dϖ1=F(ϖ1,ϖ2(ϖ1))ϖ2(ϖ1). | (2.4) |
To solve Eq (2.4), we obtain ϖ2(ϖ1). Then, we can return the variable ϖ1 as the dependent variable and substitute it into the first equation of (2.3) to obtain the final first-order ODE. Solving the resultant ODE yields the final solution of the considered model.
In the study of nonlinear phenomena, it is necessary to obtain solutions of NPDEs of the following form:
N(Θ,Θt,Θz,Θtt,Θzz,Θzt,…)=0. | (2.5) |
The expression N involves a polynomial in Θ(z,t) as well as its partial derivatives, and includes nonlinear terms and higher order derivatives.
Here we present the basic steps of the GPREs method.
Step 1. By applying the wave transformation:
Θ(z,t)=Θ(φ),φ=ϱz+νt, | (2.6) |
where ϱ and ν are constants, the NPDE (2.5) is transformed into the following ODE:
H(Θ,Θ′,Θ″,…)=0, | (2.7) |
where H is a polynomial in Θ(φ) and its total derivatives, for example Θ′(φ)=dΘ(φ)dφ.
Step 2. We assume that the formal solution of the ODE (2.7) exists as [8,10,30,31]
Θ(φ)=b0+m∑i=1ηi−1(φ)(bi,κκ(φ)+bi,ηη(φ)), | (2.8) |
with b0, bi,κ and bi,η, are constants to be determined later. The functions η(φ) and κ(φ) satisfy the ODEs
η′(φ)=λη(φ)κ(φ), κ′(φ)=Υ−ζη(φ)+λκ(φ)2, | (2.9) |
which are related between them by the following first integral
κ(φ)2=−λ((ζ2−1)η(φ)2Υ+Υ−2ζη(φ)), | (2.10) |
for the general case when Υ and ζ are nonzero constants. If Υ=ζ=0, (2.7) has the formal solution
Θ(φ)=m∑i=1bi,κκi(φ), | (2.11) |
and κ(φ) satisfy the ODE
κ′(φ)=κ(φ)2. | (2.12) |
Step 3. In (2.8), the value of the positive integer number m must be determined by applying the homogeneous balance between the highest order derivatives and the nonlinear terms in (2.7).
It is possible that, for a given nonlinear equation, the balance procedure leads to a non-positive integer number m. If m=p/q, the following transformation will be applied:
Θ(φ)=Φq/p(φ), | (2.13) |
and when the substitution into the Eq (2.13) is performed the Eq (2.7) will be transformed to an ODE for the function Φ(φ) for which the balance procedure results into a positive integer number M, see for example [8].
Step 4. The substitution of the Eq (2.8) or (2.11) along with (2.9), (2.10) or (2.12) into (2.7), allow us to collect all terms of the same power order of κi(φ)ηj(φ)(j=0,1,...,i=0,1) (or ηj(φ)({j}=0,1,...). Then each of this coefficients in front of the functions κi(φ)ηj(φ) will be set equal to zero.
This procedure generates a set of algebraic equations that can be solved to determine the values of b0, bi,κ and bi,η, ϱ, ν, ζ and Υ.
Step 5. The Eq (2.9) has the following solutions (see for example [8]).
(i) If λ=−1, Υ≠0,
η1(φ)=Υsech(√Υφ)ζsech(√Υφ)+1,κ1(φ)=√Υtanh(√Υφ)ζsech(√Υφ)+1,η2(φ)=Υcsch(√Υφ)ζcsch(√Υφ)+1,κ2(φ)=√Υcoth(√Υφ)ζcsch(√Υφ)+1. | (2.14) |
(ii) If λ=1, Υ≠0,
η3(φ)=Υsec(√Υφ)ζsec(√Υφ)+1,κ3(φ)=√Υtan(√Υφ)ζsec(√Υφ)+1,η4(φ)=Υcsc(√Υφ)ζcsc(√Υφ)+1,κ4(φ)=√Υcot(√Υφ)ζcsc(√Υφ)+1. | (2.15) |
(iii) If ζ=0, Υ=0
η5(φ)=Ξφ, κ5(φ)=1λφ, | (2.16) |
where Ξ is constant different from zero.
Step 6. The substitution of the coefficients b0, bi,κ and bi,η, ϱ, ν, ζ and Υ and the functions (2.14)–(2.16) into (2.8) or (2.11), results into the exact solutions of (2.7).
We apply the Nucci's reduction method and the GPREs method to three nonlinear dynamical models to investigate their effectiveness: The (1+1)-dimensional classical Boussinesq equations, the generalized reaction Duffing model, and the nonlinear Pochhammer-Chree equation.
Wu and Zhang in [32] developed the (1+1)-dimensional classical Boussinesq equations to simulate nonlinear and dispersive long gravity waves traveling in two horizontal directions on shallow water of uniform depth. Different types of exact solutions with various analytical techniques such as bilinear method, modified (G′/G2)-expansion method and extended (G′/G)-expansion method are investigated for this derived equation in [33,34,35].
We consider the (1+1)-dimensional classical Boussinesq equations in its following formulation:
w(z,t)∂v(z,t)∂z+∂v(z,t)∂t+(v(z,t)+1)∂w(z,t)∂z=−13∂3w(z,t)∂z3,∂v(z,t)∂z+∂w(z,t)∂t+w(z,t)∂w(z,t)∂z=0. | (3.1) |
In Eq (3.1), the water wave's elevation is represented by v, and its surface velocity along the z-axis is {defined} by w. The Euler equation served as the direct inspiration for this system. The run-up of ocean waves, such as tsunami waves, on dykes and dams can be studied using this approach. Designing harbors and coastlines benefits from having a solid understanding of precise solutions. Finding more diverse solutions is therefore crucial for fluid dynamics.
Let's now solve (3.1) using the suggested techniques for the original Boussinesq equations. To do this, we will take into consideration the following traveling wave variable transformation (2.6)
v(z,t)=v(φ), w(z,t)=w(φ),φ=ϱz+νt. | (3.2) |
The above transformation permit us to convert the Eq (3.1) into the following ODEs
ϱw(φ)v′(φ)+ϱ(1+v(φ))w′(φ)+13ϱ3w(3)(φ)+νv′(φ)=0,ϱv′(φ)+ϱw(φ)w′(φ)+νw′(φ)=0. |
When the aforementioned system is integrated, we get
ϱ(1+v(φ))w(φ)+13ϱ3w″(φ)+νv(φ)+K2=0,ϱv(φ)+12ϱw(φ)2+νw(φ)+K1=0, | (3.3) |
where K1 and K2 are arbitrary integration constants [34] and
v(φ)=−ϱw(φ)2+2K1+2νw(φ)2ϱ. | (3.4) |
Thus, the following ODE is obtained by combining Eqs (3.3) and (3.4):
K2−K1νϱw(φ)(−ν2ϱ+ϱ−K1)−12ϱw(φ)2−32νw(φ)2+13ϱ3w″(φ)=0. | (3.5) |
a) Nucci's reduction method
By using the change of variables (2.2), the system of (2.3) can be written as
{ϖ′1=ϖ2, ϖ′2=−3ϱ3(K2−K1νϱϖ1(−ν2ϱ+ϱ−K1)−12ϱϖ21−32νϖ21). | (3.6) |
If ϖ1 is chosen as a new independent variable, the system (3.6) transforms into
dϖ2(ϖ1)dϖ1=−3ϱ3ϖ2(ϖ1)(K2+λϖ1+μϖ21), |
where λ=−K1νϱ(−ν2ϱ+ϱ−K1), and μ=−12(ϱ+3ν). Solving this equation concludes
ϖ2(ϖ1)=√ϱ(R1ϱ3−2μϖ31−3λϖ21−6K2ϖ1)ϱ2, | (3.7) |
where R1 is an arbitrary constant, and the first integral that corresponds to it is denoted by
(w′(φ))2+(2μ+6K2)w3(φ)+3λw2(φ)ϱ=R1. |
Returning the ϖ1 as dependent variable and substituting the solution (3.7), into the first equation of (3.6), concludes
dϖ1dφ=√ϱ(R1ϱ3−2μϖ31−3λϖ21−6K2ϖ1)ϱ2. |
One time integration of this ODE, gives the following implicit solution
φ−∫ϱ2dϖ1√−ϱ(−R1ϱ3+2ϖ31μ+3λϖ21+6K2ϖ1)+R2=0. |
Assuming K2=0, and R1=0, concludes the following explicit solution
w(φ)=ϖ1(φ)=−3λ2μ(tan2(√3ϱλ(R3+φ)2ϱ2)+1),=−3K1ν(−ν2ϱ+ϱ−K1)ϱ(ϱ+3ν)(tan2(√−3K1ν(−ν2ϱ+ϱ−K1)(R3+φ)2ϱ2)+1). |
Lastly, from (3.2), we obtain
v(z,t)=−9ν2K212ϱ2(ϱ+3ν)2(−ν2ϱ+ϱ−K1)2(tan2(νt+ϱz+R32ϱ2√−3K1ν(−ν2ϱ+ϱ−K1))+1)2+36K1ν2ϱ2(ϱ+3ν)(−ν2ϱ+ϱ−K1)(tan2(νt+ϱz+R32ϱ2√−3K1ν(−ν2ϱ+ϱ−K1))+1)+2K1,w(z,t)=−3K1ν(−ν2ϱ+ϱ−K1)ϱ(ϱ+3ν)(tan2(√−3K1ν(−ν2ϱ+ϱ−K1)(R3+ϱz+νt)2ϱ2)+1). |
b) Generalized projective Riccati equations method
Using the algorithm for the GPREs method described in Section 3, by performing the balance between the terms w″(φ) and, w3(φ) for the formal solution
w(φ)=b0+m∑i=1ηi−1(φ)(bi,0κ(φ)+b0,iη(φ)), |
yields m=1. Therefore, we have
w(φ)=b0+b0,1η(φ)+b1,0κ(φ). | (3.8) |
Substituting (3.8) into (3.5) and considering the projective Riccati equations (2.9) the left-hand side of (3.5) becomes a polynomial in terms of η(φ) and κ(φ).
−16ϱ(ϱ(3(b0,1η(φ)+b1,0κ(φ))(3ν(b0,1η(φ)+b1,0κ(φ))+2K1)−6K2)+3b0(ϱ(ϱ(3(b0,1η(φ)+b1,0κ(φ))2−2)+6ν(b0,1η(φ)+b1,0κ(φ))+2K1)+2ν2)+3ϱ2(b0,1η(φ)+b1,0κ(φ))((b0,1η(φ)+b1,0κ(φ))2−2)−2ϱ4λ(b1,0κ(φ)(−3ζη(φ)+2Υ+2λκ(φ)2)+b0,1η(φ)(−ζη(φ)+Υ+2λκ(φ)2))+9b20ϱ(ϱ(b0,1η(φ)+b1,0κ(φ))+ν)+6ν(ν(b0,1η(φ)+b1,0κ(φ))+K1)+3b30ϱ2)=0. | (3.9) |
By taking into account the first integral of the projective Riccati method
κ(φ)2=−λ((ζ2−1)η(φ)2Υ+Υ−2ζη(φ)), |
the algebraic equation (3.9) of the different powers of in η(φ) and κ(φ) can be converted into a simplified algebraic equation in terms only of the independent functions η(φ)j and κ(φ)η(φ)ϱ
32b0ϱΥλb21,0+32Υνλb21,0−b0ν2ϱ−12b30ϱ+b0ϱ−b0K1−32b20ν−K1νϱ+K2+η(φ)(−23ϱ3Υλ3b0,1+13ϱ3Υλb0,1+32ϱΥλb0,1b21,0−ν2b0,1ϱ−3b0ϱζλb21,0−32b20ϱb0,1+ϱb0,1−K1b0,1−3ζνλb21,0−3b0νb0,1)+η(φ)2(3b0ϱζ2λb21,02Υ−3b0ϱλb21,02Υ+43ϱ3ζλ3b0,1−13ϱ3ζλb0,1−3ϱζλb0,1b21,0−32b0ϱb20,1+3ζ2νλb21,02Υ−3νλb21,02Υ−32νb20,1)+η(φ)3(−2ϱ3ζ2λ3b0,13Υ+2ϱ3λ3b0,13Υ+3ϱζ2λb0,1b21,02Υ−3ϱλb0,1b21,02Υ−12ϱb30,1)+κ(φ)(−23ϱ3Υλ3b1,0+23ϱ3Υλb1,0+12ϱΥλb31,0−ν2b1,0ϱ−32b20ϱb1,0+ϱb1,0−K1b1,0−3b0νb1,0+η(φ)(43ϱ3ζλ3b1,0−ϱ3ζλb1,0−ϱζλb31,0−3b0ϱb0,1b1,0−3νb0,1b1,0)+η(φ)2(−2ϱ3ζ2λ3b1,03Υ+2ϱ3λ3b1,03Υ+ϱζ2λb31,02Υ−ϱλb31,02Υ−32ϱb20,1b1,0))=0. |
Setting each coefficient of this polynomial's independent functions η(φ)j and κ(φ)η(φ)ϱ to zero, results in a system of algebraic equations.
32b0ϱΥλb21,0+32Υνλb21,0−b0ν2ϱ−12b30ϱ+b0ϱ−b0K1−32b20ν−K1νϱ+K2=0,−23ϱ3Υλ3b0,1+13ϱ3Υλb0,1+32ϱΥλb0,1b21,0−ν2b0,1ϱ−3b0ϱζλb21,0−32b20ϱb0,1+ϱb0,1−K1b0,1−3ζνλb21,0−3b0νb0,1=0,3b0ϱζ2λb21,02Υ−3b0ϱλb21,02Υ+43ϱ3ζλ3b0,1−13ϱ3ζλb0,1−3ϱζλb0,1b21,0−32b0ϱb20,13ζ2νλb21,02Υ−3νλb21,02Υ−32νb20,1=0,−2ϱ3ζ2λ3b0,13Υ+2ϱ3λ3b0,13Υ+3ϱζ2λb0,1b21,02Υ−3ϱλb0,1b21,02Υ−12ϱb30,1=0,−23ϱ3Υλ3b1,0+23ϱ3Υλb1,0+12ϱΥλb31,0−ν2b1,0ϱ−32b20ϱb1,0+ϱb1,0−K1b1,0−3b0νb1,0=0,(43ϱ3ζλ3b1,0−ϱ3ζλb1,0−ϱζλb31,0−3b0ϱb0,1b1,0−3νb0,1b1,0)=0,−2ϱ3ζ2λ3b1,03Υ+2ϱ3λ3b1,03Υ+ϱζ2λb31,02Υ−ϱλb31,02Υ−32ϱb20,1b1,0=0. |
The solution of the above system of equations is given by
b1,0=√ϱ2(ζ2−1)(−λ3)√3√λ−ζ2λ, b1,0=√ϱ2(ζ2−1)(−λ3)√3√λ−ζ2λ, b0=ϱζ√Υ(λ2−1)√3√1−ζ2√λ−νϱ,ν=√ϱ√(ζ2−1)(ϱ3Υ+6ϱλ−6K1λ)√3√1−ζ2√λ, K1=K222ϱ+ϱ3Υ(3ζ2−2(ζ2+2)λ2+3λ4)6(ζ2−1)λ+ϱ, |
for the set of parameters |ζ|>1,ϱ<0,K2>0,λ=−1,Υ>0 and |ζ|<1,ϱ<0,K2>0,λ=1,Υ>0.
For these set of coefficients solutions for the functions w(φ) and v(φ) are given as
w(φ)=−K2ϱ−ϱ(Υλ2κ(φ)+η(φ)√−Υλ(ζ2−1))√3Υλ, |
v(φ)=−1−ϱ2η(φ)(ζΥ+κ(φ)√−Υλ(ζ2−1)+−η(φ)(1−ζ2)3Υλ, | (3.10) |
when |ζ|>1,ϱ<0,K2>0,λ=−1,Υ>0.
Furthermore, the functions w(φ) and v(φ) are given as
w(φ)=K2ϱ+ϱ(√Υκ(φ)+η(φ)√λ(1−ζ2)√3√Υλ, |
v(φ)=−1+ϱ2η(φ)(−ζΥ+√Υλκ(φ)√λ(1−ζ2+(ζ2−1)η(φ))3Υλ, | (3.11) |
when |ζ|<1,ϱ<0,K2>0,λ=1,Υ>0.
If we consider the solutions (2.14), when λ=−1,
η1(φ)=Υsech(√Υφ)ζsech(√Υφ)+1, κ1(φ)=√Υtanh(√Υφ)ζsech(√Υφ)+1, |
solutions (3.10) take the following expressions
w1(φ)=ϱ(Υ3/2tanh(φ√Υ)ζsech(φ√Υ)+1+Υ√(ζ2−1)Υsech(φ√Υ)ζsech(φ√Υ)+1)√3Υ−K2ϱ, |
v1(φ)=ϱ2sech(φ√Υ)(−ζ2Υsech(φ√Υ)ζsech(φ√Υ)+1+√ζ2−1Υtanh(φ√Υ)ζsech(φ√Υ)+1+Υsech(φ√Υ)ζsech(φ√Υ)+1+ζΥ)3(ζsech(φ√Υ)+1)−1. | (3.12) |
By considering the second pair of solutions of the Eq (2.14), when λ=1,
η2(φ)=Υcsch(√Υφ)ζcsch(√Υφ)+1, κ2(φ)=√Υcoth(√Υφ)ζcsch(√Υφ)+1, |
solutions (3.10) take the following expressions
w2(φ)=ϱ(Υ3/2coth(φ√Υ)ζcsch(φ√Υ)+1+Υ√(ζ2−1)Υcsch(φ√Υ)ζcsch(φ√Υ)+1)√3Υ−K2ϱ, |
v2(φ)=ϱ2csch(φ√Υ)(−ζ2Υcsch(φ√Υ)ζcsch(φ√Υ)+1+√ζ2−1Υcoth(φ√Υ)ζcsch(φ√Υ)+1+Υcsch(φ√Υ)ζcsch(φ√Υ)+1+ζΥ)3(ζcsch(φ√Υ)+1)−1. | (3.13) |
Solutions w1(z,t) and v1(z,t) are solutions of the classical Boussinesq equations with a well defined behavior of a dark soliton type for w1(z,t) and one bright soliton solution for v1(z,t). Figure 1 depicts the shape of the solitary solution w1(z,t) of the classical Boussinesq equation, with Υ=0.995,ζ=2.098,K2=0.45,ϱ=0.37,λ=−1, in a 3-D plot. The 2-D plot for different time values is shown in Figure 1a, and the corresponding contour plot is shown in Figure 1c. Figure 2 depicts the shape of the bright soliton solution v1(z,t) for the classical Boussinesq equation, with Υ=0.995,ζ=2.098,K2=0.45,ϱ=0.37,λ=−1, in a 3-D plot, Figure 2a, the contour plot is shown in Figure 2c and the 2-D plot for different time values is shown in Figure 2b.
Solutions w2(z,t) and v2(z,t) are solutions of the classical Boussinesq equations with a well defined behavior of a singular soliton type for w2(z,t), where this soliton solution descent to −∞ from one asymptotical state at φ→−∞ and then rises to ∞ and finally descending to another asymptotical state at φ→∞. For the soliton solution v2(z,t), we observe a different behavior associated with one singular soliton solution showing a symmetrical behavior where this soliton descent to −∞ from one asymptotical state at φ→−∞ and then rises to the same original asymptotical state at φ→∞. Figure 3 illustrates the shape of the solitary solution w2(z,t) of the classical Boussinesq equation, with Υ=0.995,ζ=2.098,K2=0.45,ϱ=0.37,λ=−1, in a 3-D plot in Figure 3a, Figure 3b illustrates a 2-D plot for different time values and the contour plot is exhibited in Figure 3c. Figure 4 illustrates the shape of the singular soliton v2(z,t) of the classical Boussinesq equation, with Υ=0.995,ζ=2.098,K2=0.45,ϱ=0.37,λ=−1, Figure 4a, shows a 3-D plot, Figure 4b shows a 2-D plot for different time values, and Figure 4c shows a contour plot.
Next if we consider the solutions (2.15), when λ=1:
η3(φ)=Υsec(√Υφ)ζsec(√Υφ)+1, κ3(φ)=√Υtan(√Υφ)ζsec(√Υφ)+1, |
solutions (3.11) take the following expressions
w3(φ)=K2ϱ+ϱ(√1−ζ2Υsec(√Υφ)ζsec(√Υφ)+1+Υtan(√Υφ)ζsec(√Υφ)+1)√3√Υv3(φ)=−ϱ2sec(√Υz)(ζΥ−ζ2Υsec(√Υφ)ζsec(√Υφ)+1+√1−ζ2Υtan(√Υφ)ζsec(√Υφ)+1+Υsec(√Υφ)ζsec(√Υφ)+1)3(ζsec(√Υφ)+1)−1. |
By considering the second pair of solutions of the Eq (2.15), when λ=1:
η4(φ)=Υcsc(√Υφ)ζcsc(√Υφ)+1, κ4(φ)=√Υcot(√Υφ)ζcsc(√Υφ)+1, |
solutions (3.11) take the following expressions
w4(φ)=K2ϱ+ϱ(√1−ζ2Υcsc(√Υφ)ζcsc(√Υφ)+1+Υcot(√Υφ)ζcsc(√Υφ)+1)√3√Υ,v4(φ)=ϱ2csc(√Υφ)(ζ(−Υ)+(ζ2−1)Υcsc(√Υφ)ζcsc(√Υφ)+1+√1−ζ2Υcot(√Υφ)ζcsc(√Υφ)+1)3(ζcsc(√Υφ)+1)−1. |
Figures 5 and 6 display the singular periodic solutions w3(z,t) and v3(z,t), respectively. The singular periodic solution w3(z,t) exhibits a well-defined tan(φ) pattern and is a solution of the classical Boussinesq equations. On the other hand, the singular periodic solution v3(z,t) shows a symmetrical behavior of the sec(φ), where the solution descends to −∞ and then rises to its original value. This behavior repeats an infinite number of times.
Figures 7 and 8 show the periodical singular solution of w4(z,t) and v4(z,t), respectively. The solution w4(z,t) is a singular periodical solution of the classical Boussinesq equations with a well defined cot(φ) pattern. For the singular periodic solution v4(z,t), we observe a typical behavior of the csc(φ) with a symmetrical behavior where this solution descent to −∞ and then rises to its original state with and infinity number of repetitions of this type of singularities.
We have applied the GPREs method to obtain the analytical solutions of the generalized reaction Duffing model [36,37] in the form:
∂2v(z,t)∂t2+α∂2v(z,t)∂z2+βv(z,t)+γv3(z,t)=0, | (3.14) |
where α, β, and γ are constants.
The importance of generalized reaction Duffing model is highlighted by the fact that if certain values are chosen for its coefficients, it produces well known models [5,7,39,40].
● For α=−1,β>0,γ>0, it produces the Landau-Ginzburg-Higgs equation, which is used to study both low-temperature and high-temperature superconductors.
● For α=−1,β<0,γ<0, it is the Klein-Gordon equation, which arises in the applications of relativistic condensed matter physics.
● For α=−1,β=1,γ=−1, we get the ϕ4 equation and appears in the applications of quantum mechanics.
● For α=1,β=1,γ=−1/6, it results in the sine-Gordon equation, which appears in relativistic field theory and mechanical transmission lines.
● For α=0, it turns into the classical Duffing equation, which is used to detect weak signal of early machinery fault.
If we proceed in a similar way as in the previous example, by considering the wave transformation
v(z,t)=v(φ),φ=ϱz+νt, |
then} Eq (3.14) is reduced to the following ordinary differential equation:
αϱ2v″(φ)+ν2v″(φ)+βv(φ)+γv(φ)3=0. | (3.15) |
a) Nucci's reduction method
By using the change of variables (2.2), the system of (2.3) for the Eq (3.15) can be written as
{ϖ′1=ϖ2, ϖ′2=−βϖ1+γϖ31αϱ2+ν2. | (3.16) |
If we choice ϖ1 as a new independent variable, then the system (3.16) converts into
dϖ2(ϖ1)dϖ1=−βϖ1+γϖ31(αϱ2+ν2)ϖ2(ϖ1). |
Solving this equation concludes
ϖ2(ϖ1)=√−γϖ41−2βϖ21+2(αϱ2+ν2)R12(αϱ2+ν2), | (3.17) |
where R1 is an arbitrary constant and corresponding first integral can be written by
(v′(φ))2+γv4(φ)+2βv2(φ)2(αϱ2+ν2)=R1. |
Returning the ϖ1 as dependent variable and substituting the solution (3.17), into the first equation of (3.16), concludes
dϖ1dφ=√−γϖ41−2βϖ21+2(αϱ2+ν2)R12(αϱ2+ν2). |
One time integration of this ODE, gives the following implicit solution
φ+∫√2(αϱ2+ν2)−γϖ41−2βϖ21+2(αϱ2+ν2)R1+R2=0. | (3.18) |
Solving this equation with respect to the ϖ1(φ), concludes the following explicit solution
ϖ1=√2Ξ3γ√2Ξ+β2+γβ[JCN(Θ1φ,Θ2)JDN(Θ1φ,Θ2)JSN(Θ1R2,Θ2)(−β√2Ξ+β2+Ξ+β2)(JSN(Θ1φ,Θ2))2(JSN(Θ1R2,Θ2))2+γΞ+JSN(Θ1φ,Θ2)JCN(Θ1R2,Θ2)JDN(Θ1R2,Θ2)(−β√2Ξ+β2+Ξ+β2)(JSN(Θ1φ,Θ2))2(JSN(Θ1R2,Θ2))2+γΞ], |
where JCN, JDN, JSN, are JacobiCN, JacobiDN, and JacobiSN functions respectively, and
Ξ=R1γ(αϱ2+ν2),Θ1=√2(αϱ2+ν2)(√2γR1(αϱ2+ν2)+β2+β)2αϱ2+2ν2,Θ2=√β√2γR1(αϱ2+ν2)+β2−γR1(αϱ2+ν2)−β2γR1(αϱ2+ν2). |
Lastly, from the transformation (3.2), we obtain the final solution. Moreover, in order to derive a simple solution for the considered equation, we can assume R1=0. Then, from (3.18), we get
ϖ1=4Ξ(φ)(−2αβϱ2−2βν2−2Φ(32γΦα2βϱ4+64γΦαβν2ϱ2+32γΦβν4−√−(Ξ(φ))4αβϱ2−(Ξ(φ))4βν2)32γβα2ϱ4+64γβαϱ2ν2+32γβν4−(Ξ(φ))2), |
where
Φ=√−(αϱ2+ν2)β, Ξ(φ)=e−√−(αϱ2+ν2)β(φ+R2)αϱ2+ν2. |
Similarly, we obtain the final solution from the transformation (3.2).
b) Generalized projective Riccati equations method
Based on the issues discussed, we balance v″(φ) and v3(φ) in Eq (3.15) for the formal solution
v(φ)=a0+m∑i=1ηi−1(φ)(ai,0κ(φ)+a0,iη(φ)), |
yields m=1. Therefore, we have
v(φ)=a0+a0,1η(φ)+a1,0κ(φ). | (3.19) |
Substituting (3.19) into (3.15) and considering the projective Riccati equations (2.9) and taking the value λ=−1, the left-hand side of (3.15) becomes a polynomial in terms of η(φ)j and κ(φ)η(φ)ϱ. To obtain the values of the coefficients, we set the polynomial's coefficients to zero, resulting in a system of algebraic equations similar to that used for the Boussinesq equation's system of equations.
a1,0=√2a0,1√β−(1−ζ2)(αϱ2+ν2), Υ=2βαϱ2+ν2, γ=−(ζ2−1)(αϱ2+ν2)24βa20,1, |
giving the following solution for the generalized reaction Duffing model
v(φ)=a0,1(√2βκ(φ)√−β(1−ζ2)(αϱ2+ν2)+η(φ)), | (3.20) |
with
λ=−1, a0,1>0, β>0, αϱ2+ν2>0, 1−ζ2<0, |
and
a0=0, Υ=−2βαϱ2+ν2<0, γ=−(ζ2−1)(αϱ2+ν2)24βa20,1,a1,0=√2a0,1√β(ζ2−1)(αϱ2+ν2), | (3.21) |
giving the following solution for the generalized reaction Duffing model
v(φ)=a0,1(√2βκ(φ)√β(ζ2−1)(αϱ2+ν2)+η(φ)), | (3.22) |
with
λ=1, a0,1<0, β<0, αϱ2+ν2<0,1−ζ2>0. | (3.23) |
If we consider the solutions (2.14), when λ=−1,
η1(φ)=Υsech(√Υφ)ζsech(√Υφ)+1, κ1(φ)=√Υtanh(√Υφ)ζsech(√Υφ)+1, |
solution (3.20) takes the following expression
v1(φ)=a0,1(√2β√Υtanh(φ√Υ)(ζsech(φ√Υ)+1)√β(ζ2−1)(αϱ2+ν2)+Υsech(φ√Υ)ζsech(φ√Υ)+1), | (3.24) |
where Υ is given by the Eq (3.21). By considering the second pair of solutions of the Eq (2.14), when λ=1,
η2(φ)=Υcsch(√Υφ)ζcsch(√Υφ)+1, κ2(φ)=√Υcoth(√Υφ)ζcsch(√Υφ)+1, |
solution (3.20) takes the following expression
v2(φ)=a0,1(√2β√Υcoth(φ√Υ)(ζcsch(φ√Υ)+1)√β(ζ2−1)(αϱ2+ν2)+Υcsch(φ√Υ)ζcsch(φ√Υ)+1), | (3.25) |
where Υ is given by the Eq (3.21).
The solutions v1(z,t) and v2(z,t) obtained from Eqs (3.24) and (3.25) are soliton solutions of the generalized reaction Duffing model equations, exhibiting well-defined behavior. In Figure 9, we observe a dark soliton behavior for v1(z,t), while in Figure 10, a singular soliton solution for v2(z,t) is shown. This soliton solution descends to −∞ from one asymptotic state at φ→−∞, then rises to ∞, and finally descends to another asymptotic state at φ→∞. Both types of solutions were obtained using the following parameter values: ζ=2.098, ϱ=0.37, a0,1=1, β=2, α=1, ν=0.87, λ=−1 (shown in blue). In Figure 9, we present the properties of v1(z,t) in a 3-D plot (Figure 9a), a contour plot (Figure 9c), and a 2-D plot for different time values (Figure 9b). Similarly, in Figure 10, we show the properties of v2(z,t) in a 3-D plot (Figure 2a), a contour plot (Figure 10c), and a 2-D plot for different time values (Figure 10b).
The GPREs method is not able to produce periodic singular solutions with λ=1 for the analytical solutions of the generalized reaction Duffing model equations. This is due to the negative value of Υ observed in Eq (3.21), which can be expressed as Υ=−2βαϱ2+ν2. This result stems from the conditions set forth in Eq (3.23). As a consequence, any analytical solutions obtained using the GPREs method and following Eq (3.22) will lack any physical meaning, as the original condition for Υ requires it to be positive in all possible solutions.
Another important nonlinear system that can be analyzed by the projective Riccati equations method is the Pochhammer-Chree equation [9,43,44]:
∂2(−αv(z,t)−βv(z,t)n+1−γv(z,t)2n+1)∂z2−∂4v(z,t)∂z2∂t2+∂2v(z,t)∂t2=0, | (3.26) |
where α, β and γ are constants. In the Eq (3.26) v(z,t) describes the nonlinear longitudinal wave propagation into elastic rods and is valid for a cylinder with finite length if the radius is small compared with the wavelength [45,46]. Bifurcations of solitary waves and kink waves for Generalized Pochhammer-Chree Equation are considered in [47]. Travelling wave solutions, which include kink-shaped solitons, bell-shaped solitons, periodic solutions, rational solutions, singular solitons, are obtained in [48] for this equation.
We will apply the GPREs method to obtain the analytical solutions of the nonlinear Pochhammer-Chree equation. We can use the following transformation to get the analytical solutions:
v(z,t)=v(φ),φ=ϱz+νt. | (3.27) |
This travelling wave change of variable permits us to convert the nonlinear Pochhammer-Chree equation into the following ODE:
ϱ2(β(−n−1)v(φ)n(nv′(φ)2+v(φ)v″(φ)))v(φ)+ϱ2(−γ(2n+1)v(φ)2n(2nv′(φ)2+v(φ)v″(φ)))v(φ)+ϱ2(−v(φ)(ν2v(4)(φ)+αv″(φ)))v(φ)+ν2v″(φ)=0. | (3.28) |
By twice integrating the (3.28) with respect to φ and vanishing the integration constants, the following result is obtained:
−ϱ2(v(φ)(v(φ)n(a0(n+2)ν22n2a21,0−(n+1)ν2v(φ)n4n2a21,0)))−ϱ2(v(φ)(+a20(n+1)ν2n2a21,0−a20(n+2)ν2n2a21,0+ν2ϱ2))−ϱ2ν2v″(φ)+ν2v(φ)=0. | (3.29) |
By balancing with in (3.29), the terms v″(φ) and, v2n+1(φ), we can consider the next change for the dependent variable (see for example [8])
v(φ)=u(φ)1n, | (3.30) |
where u(φ) is a new function of φ. Substituting (3.30) into (3.29) we get the new ODE
(n2ν2ϱ2−αn2)u(φ)2−βn2u(φ)3−γn2u(φ)4+(n−1)ν2u′(φ)2−nν2u(z)u″(φ)=0. | (3.31) |
a) Nucci's reduction method
By using the change of variables (2.2), the system of (2.3) for the Eq (3.31) can be written as
{ϖ′1=ϖ2, ϖ′2=1nν2ϖ1((n2ν2ϱ2−αn2)ϖ12−βn2ϖ13−γn2ϖ14+(n−1)ν2ϖ22). | (3.32) |
If we introduce a new independent variable, ϖ1, the system (3.32), converts into
dϖ2(ϖ1)dϖ1=1nν2ϖ1ϖ2(ϖ1)((n2ν2ϱ2−αn2)ϖ12−βn2ϖ13−γn2ϖ14+(n−1)ν2(ϖ2(ϖ1))2). |
Solving this equation concludes
ϖ2(ϖ1)=√R1n2Ξϖ12+Φϖ12n+2n−γ(n(n+2))2ϱ2ϖ12+4nn−2βϱ2(n(n+1))2ϖ12+3nnϖ112nΞ, | (3.33) |
where
Ξ=ν2ϱ2(n2+3n+2)2, Φ=n2(n2+3n+2)(−αϱ2+ν2), |
and R1 is an arbitrary constant and corresponding first integral can be written by
u2n(φ)Ψ1(u′(φ))2−Ψ2u2n+2n(φ)+γ(n(n+2))2ϱ2u2+4nn(φ)+2βϱ2(n(n+1))2(u(φ))2+3nnn2ν2(ϱ(n2+3n+2))2(u(φ))2=R1, |
where
Ψ1=(n2+3n+2)ν2ϱ2, Ψ2=n2(n+2)(n+1)(−αϱ2+ν2). |
Returning the ϖ1 as dependent variable and substituting the solution (3.33), into the first equation of (3.32), concludes
dϖ1dφ=√R1n2Ξϖ21+Φϖ2n+2n1−γ(n(n+2))2ϱ2ϖ2+4nn1−2βϱ2(n(n+1))2ϖ2+3nn1ϖ12n1Ξ. |
One time integration of this ODE, by assuming R1=0, gives the following implicit solution
φ+ϱν(n+1)(n+2)∫ϖ12n1Ωdϖ1+R2=0, |
where
Ω=√n2(n+2)(n+1)(−2βϱ2(n+1)ϖ4+3nn1+(n+2)(−γϱ2ϖ4n+4n1+ϖ2n+4n1(−αϱ2+ν2)(n+1))). |
Solving this equation with respect to the ϖ1(φ), and different values of n, concludes the following explicit solutions:
∙ n=1,
u(φ)=ϖ1(φ)=12eΛ1(−αϱ2+ν2)e2Λ1+4βϱ2eΛ1+(−18αγ+4β2)ϱ4+18γν2ϱ2, |
where Λ1=−2ln(2)νϱ−ln(3)νϱ+√(−αϱ2+ν2)(φ+R2)2ϱν.
∙ n=2,
u(φ)=ϖ1(φ)=24eΛ1(−αϱ2+ν2)6βϱ2eΛ1+e2Λ1+(−48αγ+9β2)ϱ4+48γν2ϱ2, |
where Λ1=−ln(2)νϱ−ln(3)νϱ+2√(−αϱ2+ν2)(φ+R2)2ϱν.
∙ n=3,
u(φ)=ϖ1(φ)=20eΛ1(−αϱ2+ν2)4βϱ2eΛ1+eΛ2+(−25αγ+4β2)ϱ4+25γν2ϱ2, |
where Λ1=−2ln(2)νϱ−ln(5)νϱ+3√(−αϱ2+ν2)(φ+R2)2ϱν.
Similarly, we obtain the final solution from the transformation (3.30) and (3.27).
b) Generalized projective Riccati equations method
Performing the balance in the (3.31), between the terms u(φ)u″(φ) and u4(φ), we introduce the following guess solution
u(φ)=a0+a0,1η(φ)+a1,0κ(φ), | (3.34) |
with a0, a0,1 and a0,1 are constants that have to be determinated in a similar way as they were obtained in the previous two examples.
Substituting (3.34) into (3.31) and considering the projective Riccati equations (2.9), with the value of λ=−1 and a procedure similar to the one used for the Boussinesq equation system and the generalized reaction Duffing model, the obtained coefficient values are as follows:
Υ=a20a21,0, a0,1=√ζ2−1√n−2a21,0√a20(n−2),β=a0(n+2)ν22n2a21,0, γ=−(n+1)ν24n2a21,0, α=ν2(1ϱ2−a20n2a21,0), | (3.35) |
which concludes the following solution for the nonlinear Pochhammer-Chree equation
u(φ)=−√ζ2−1a21,0η(φ)a0+a1,0κ(φ)+a0, | (3.36) |
with
λ=−1,|ζ|>1,n>2. |
Moreover
Υ=−a20a21,0, a0,1=−√ζ2−1√n−2a21,0√a20(n−2),β=a0(n+2)ν22n2a21,0, γ=−(n+1)ν24n2a21,0, α=ν2(1ϱ2−a20n2a21,0), | (3.37) |
concludes the following solution for the nonlinear Pochhammer-Chree equation
u(φ)=−√ζ2−1a21,0η(φ)a0+a1,0κ(φ)+a0, | (3.38) |
with
λ=1,|ζ|<1,n>2. | (3.39) |
If we consider the solution (2.14), when λ=−1,
η1(φ)=Υsech(√Υφ)ζsech(√Υφ)+1, κ1(φ)=√Υtanh(√Υφ)ζsech(√Υφ)+1, |
solution (3.36) takes the following expression
u1(φ)=a0−a21,0√ζ2−1Υsech(φ√Υ)a0(ζsech(φ√Υ)+1)+a1,0√Υtanh(φ√Υ)ζsech(φ√Υ)+1, | (3.40) |
where Υ is given by the Eq (3.35). By considering the second pair of solutions of the Eq (2.14), when λ=1,
η2(φ)=Υcsch(√Υφ)ζcsch(√Υφ)+1, κ2(φ)=√Υcoth(√Υφ)ζcsch(√Υφ)+1, | (3.41) |
solution (3.20) takes the following expression
u2(φ)=a0−a21,0√ζ2−1Υcsch(φ√Υ)a0(ζcsch(φ√Υ)+1)+a1,0√Υcoth(φ√Υ)ζcsch(φ√Υ)+1, | (3.42) |
where Υ is given by the Eq (3.35).
The solutions u1(z,t) and u2(z,t) obtained from Eqs (3.40) and (3.42), respectively, are valid solutions of the nonlinear Pochhammer-Chree equation and exhibit distinct behavior. Figure 11 shows a dark soliton-like behavior for u1(z,t), while Figure 12 demonstrates a singular soliton solution for u2(z,t). In the latter case, the soliton rises to infinity from one asymptotic state as φ→−∞, then descends to −∞, and finally rises again to another asymptotic state as φ→∞. We used the following parameter values for both types of solutions: ζ=2.098, ϱ=0.37, a0,1=1, β=2, α=1, ν=0.87, and λ=−1. Figure 9 illustrates the properties of the solution v1(z,t) with a 3-D plot (Figure 9a), a 2-D plot for various time values (Figure 9b), and a contour plot (Figure 9c). Similarly, Figure 10 displays the properties of the solution v2(z,t) with a 3-D plot (Figure 2a), a 2-D plot for various time values (Figure 10b), and a contour plot (Figure 10c).
The GPREs method is unable to provide us with periodic singular solutions with λ=1 for the analytical solutions of the nonlinear Pochhammer-Chree equation. This is explained by analyzing the Eq (3.37), where we observe a negative value of Υ, i.e., Υ=−a20a21,0<0. This result is due to the set of conditions in Eq (3.39). As a result, the analytical solutions (3.38) lack any kind of physical meaning since the original condition for Υ was imposed to be positive in any possible solutions obtained by the GPREs method.
The GPREs method has been applied previously to the nonlinear Pochhammer-Chree equation in [8], but the case λ=1 was not analyzed and the condition that all allowed solutions require a positive value for Υ has not been imposed in their analysis.
This study utilized the Nucci's reduction method and the generalized projective Ricatti equations method to obtain novel traveling wave solutions and first integrals for the (1+1)-dimensional classical Boussinesq equations, the generalized reaction Duffing model, and the nonlinear Pochhammer-Chree equation. By directly applying the generalized projective Ricatti equations method to the (1+1)-dimensional classical Boussinesq equations, a new set of analytical solutions was derived. These solutions can be expressed as a combination of hyperbolic or trigonometric functions, which were determined using the solutions of the projective Ricatti equations method described in Eqs (3.10) to (3.11). To ensure the validity of these solutions, certain restrictions must be satisfied for the parameters, such as |ζ|>1, ϱ<0, K2>0, λ=−1, and Υ>0 for solutions (3.10); and |ζ|<1, ϱ<0, K2>0, λ=1, and Υ>0 for solutions (3.11). These newly discovered hyperbolic function solutions are expected to be relevant to physical phenomena and may provide insight into challenging physical aspects of nonlinear systems.
This research work was funded by Institutional Fund Projects under grant no. (IFPIP: 1264-130-1443). The authors gratefully acknowledge technical and financial support provided by the Ministry of Education and King Abdulaziz University, DSR, Jeddah, Saudi Arabia.
The authors declare no conflicts of interest.
[1] | M. K. Kadalbajoo, P. Arora, V. Gupta, Collocation method using artificial viscosity for solving stiff singularly perturbed turning point problem having twin boundary layers, Comput. Math. Appl., 61 (2014), 1595-1607. |
[2] |
G. M. Amiraliyev, F. Erdogan, Uniform numerical method for singularly perturbed delay differential equations, Comput. Math. Appl., 53 (2007), 1251-1259. doi: 10.1016/j.camwa.2006.07.009
![]() |
[3] | G. M. Amiraliyev, E. Cimen, Numerical method for a singularly perturbed convection diffusion problem with delay, Appl. Math. Comput., 216 (2010), 2351-2359. |
[4] |
P. Rai, K. K. Sharma, Numerical study of singularly perturbed differential-difference equation arising in the modeling of neuronal variability, Comput. Math. Appl., 63 (2012), 118-132. doi: 10.1016/j.camwa.2011.10.078
![]() |
[5] | P. Rai, K. K. Sharma, Numerical analysis of singularly perturbed delay differential turning point problem, Appl. Math. Comput., 218 (2011), 3483-3498. |
[6] |
S. Natesan, J. Jayakumar, J. Vigo-Aguiar, Parameter uniform numerical method for singularly perturbed turning point problems exhibiting boundary layers, J. Comput. Appl. Math., 158 (2003), 121-134. doi: 10.1016/S0377-0427(03)00476-X
![]() |
[7] |
A. T. Chekole, G. F. Duresssa, G. G. Kiltu, PNon-polynomial septic spline method for singularly perturbed two point boundary value problems of order three, J. Taibah Univ. Sci., 13 (2019), 651-660. doi: 10.1080/16583655.2019.1617986
![]() |
[8] |
F. Z. Geng, S. P. Qian, Modified reproducing kernel method for singularly perturbed boundary value problems with a delay, Appl. Math. Model., 39 (2015), 5592-5597. doi: 10.1016/j.apm.2015.01.021
![]() |
[9] |
F. Z. Geng, S. P. Qian, Reproducing kernel method for singularly perturbed turning point problems having twin boundary layers, Appl. Math. Lett., 26 (2013), 998-1004. doi: 10.1016/j.aml.2013.05.006
![]() |
[10] |
D. V. Lukyanenko, M. A. Shishlenin, V. T. Volkov, Solving of the coefficient inverse problems for a nonlinear singularly perturbed reaction-diffusion-advection equation with the final time data, Commun. Nonlinear Sci., 54 (2018), 233-247. doi: 10.1016/j.cnsns.2017.06.002
![]() |
[11] |
L. Beilina, M. V. Klibanov, A globally convergent numerical method for a coefficient inverse problem, SIAM J. Sci. Comput., 31 (2008), 478-509. doi: 10.1137/070711414
![]() |
[12] |
A. B. Bakushinskii, M. V. Klibanov, N. A. Koshev, Carleman weight functions for a globally convergent numerical method for ill-posed Cauchy problems for some quasilinear PDEs, Nonlinear Anal. Real., 34 (2017), 201-224. doi: 10.1016/j.nonrwa.2016.08.008
![]() |
[13] |
F. Z. Geng, S. P. Qian, An optimal reproducing kernel method for linear nonlocal boundary value problems, Appl. Math. Lett., 77 (2018), 49-56. doi: 10.1016/j.aml.2017.10.002
![]() |
[14] | F. Z. Geng, A new higher order accurate reproducing kernel-based approach for boundary value problems, Appl. Math. Lett., 107 (2020), 106494. |
[15] | F. Z. Geng, Numerical methods for solving Schröinger equations in complex reproducing kernel Hilbert spaces, Math. Sci., (2020), https://doi.org/10.1007/s40096-020-00337-6. |
[16] |
X. Y. Li, B. Y. Wu, A new reproducing kernel method for variable order fractional boundary value problems for functional differential equations, J. Comput. Appl. Math., 311 (2017), 387-393. doi: 10.1016/j.cam.2016.08.010
![]() |
[17] |
X. Y. Li, B. Y. Wu, A numerical technique for variable fractional functional boundary value problems, Appl. Math. Lett., 43 (2015), 108-113. doi: 10.1016/j.aml.2014.12.012
![]() |
[18] | X. Y. Li, B. Y. Wu, A new kernel functions based approach for solving 1-D interface problems, Appl. Math. Comput., 380 (2020), 125276. |
[19] |
X. Y. Li, Y. Gao, B. Y. Wu, Approximate solutions of Atangana-Baleanu variable order fractional problems, AIMS Math., 5 (2020), 2285-2294. doi: 10.3934/math.2020151
![]() |
[20] |
M. Q. Xu, Z. H. Zhao, J. Niu, et al. A simplified reproducing kernel method for 1-D elliptic type interface problems, J. Comput. Appl. Math., 351 (2019), 29-40. doi: 10.1016/j.cam.2018.10.027
![]() |
[21] | H. Sahihi, S. Abbasbandy, T. Allahviranloo, Computational method based on reproducing kernel for solving singularly perturbed differential-difference equations with a delay, Appl. Math. Comput., 361 (2019), 583-598. |
[22] |
O. Abu Arqub, B. Maayah, Modulation of reproducing kernel Hilbert space method for numerical solutions of Riccati and Bernoulli equations in the Atangana-Baleanu fractional sense, Chaos Soliton. Fract., 125 (2019), 163-170. doi: 10.1016/j.chaos.2019.05.025
![]() |
[23] |
O. Abu Arqub, Computational algorithm for solving singular Fredholm time-fractional partial integrodifferential equations with error estimates, J. Appl. Math. Comput., 59 (2019), 227-243. doi: 10.1007/s12190-018-1176-x
![]() |
[24] | O. Abu Arqub, M. Al-Smadi, Fuzzy conformable fractional differential equations: Novel extended approach and new numerical solutions, Soft Comput., 2020, https://doi.org/10.1007/s00500-020-04687-0. |
[25] |
O. Abu Arqub, Numerical algorithm for the solutions of fractional order systems of Dirichlet function types with comparative analysis, Fund. Inform., 166 (2019), 111-137. doi: 10.3233/FI-2019-1796
![]() |
[26] |
A. Akgül, Reproducing kernel Hilbert space method based on reproducing kernel functions for investigating boundary layer flow of a Powell-Eyring non-Newtonian fluid, J. Taibah Univ. Sci., 13 (2019), 858-863. doi: 10.1080/16583655.2019.1651988
![]() |
[27] |
A. Akgül, E. K. Akgül, S. Korhan, New reproducing kernel functions in the reproducing kernel Sobolev spaces, AIMS Math., 5 (2020), 482-496. doi: 10.3934/math.2020032
![]() |
[28] |
Z. H. Zhao, Y. Z. Lin. J. Niu, Convergence order of the reproducing kernel method for solving boundary value problems, Math. Model. Anal., 21 (2016), 466-477. doi: 10.3846/13926292.2016.1183240
![]() |
[29] |
H. Sahihi, T. Allahviranloo, S. Abbasbandy, Solving system of second-order BVPs using a new algorithm based on reproducing kernel Hilbert space, Appl. Numer. Math., 151 (2020), 27-39. doi: 10.1016/j.apnum.2019.12.008
![]() |
[30] | N. Aronszajn, Theory of reproducing kernel, Trans. A.M.S., 168 (1950), 1-50. |
[31] | H. Wendland, Scattered data approximation, New York: Cambridge University Press, 2004. |
1. | Pichaya Voottipruex, Nichaphat Patanarapeelert, Klot Patanarapeelert, Treatment failure and the threshold of disease extinction, 2024, 24680427, 10.1016/j.idm.2024.12.007 |