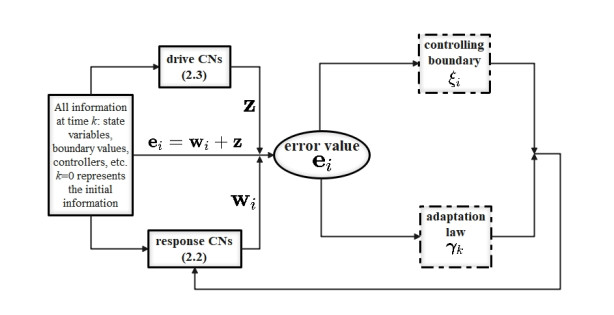
The modeling of discrete space-time stochastic heterogeneous complex networks with unknown factors was achieved through the utilization of differencing techniques with respect to the time and space variables of the nodes' states. Via the space-time discrete Lyapunov-Krasovskii functional and the approach of linear matrix inequality, this paper derived the mean-squared asymptotic anti-synchronization of the aforementioned discrete networks. This was achieved by defining an updated law for the hitherto unknown parameters and incorporating boundary values within the feedback controller. The theoretical and experimental findings indicated that the feedback controller at the boundary represented a more effective and cost-effective control technique for the networks. Furthermore, an adaptive rule has been designed to identify the uncertainties that occur in the networks with a high degree of accuracy. In particular, this rule enabled the response networks to identify unknown information in the drive networks with a high level of precision by incorporating an adaptive updating mechanism. Finally, a numerical example was provided to elucidate the viability of the ongoing investigation.
Citation: Huan Luo. Heterogeneous anti-synchronization of stochastic complex dynamical networks involving uncertain dynamics: an approach of the space-time discretizations[J]. Electronic Research Archive, 2025, 33(2): 613-641. doi: 10.3934/era.2025029
[1] | Moutian Liu, Lixia Duan . In-phase and anti-phase spikes synchronization within mixed Bursters of the pre-Bözinger complex. Electronic Research Archive, 2022, 30(3): 961-977. doi: 10.3934/era.2022050 |
[2] | Jiaqi Chang, Xiangxin Yin, Caoyuan Ma, Donghua Zhao, Yongzheng Sun . Estimation of the time cost with pinning control for stochastic complex networks. Electronic Research Archive, 2022, 30(9): 3509-3526. doi: 10.3934/era.2022179 |
[3] | Bin Wang . Random periodic sequence of globally mean-square exponentially stable discrete-time stochastic genetic regulatory networks with discrete spatial diffusions. Electronic Research Archive, 2023, 31(6): 3097-3122. doi: 10.3934/era.2023157 |
[4] | Yu-Jing Shi, Yan Ma . Finite/fixed-time synchronization for complex networks via quantized adaptive control. Electronic Research Archive, 2021, 29(2): 2047-2061. doi: 10.3934/era.2020104 |
[5] | Shuang Liu, Tianwei Xu, Qingyun Wang . Effect analysis of pinning and impulsive selection for finite-time synchronization of delayed complex-valued neural networks. Electronic Research Archive, 2025, 33(3): 1792-1811. doi: 10.3934/era.2025081 |
[6] | Ariel Leslie, Jianzhong Su . Modeling and simulation of a network of neurons regarding Glucose Transporter Deficiency induced epileptic seizures. Electronic Research Archive, 2022, 30(5): 1813-1835. doi: 10.3934/era.2022092 |
[7] | Minglei Fang, Jinzhi Liu, Wei Wang . Finite-/fixed-time synchronization of leakage and discrete delayed Hopfield neural networks with diffusion effects. Electronic Research Archive, 2023, 31(7): 4088-4101. doi: 10.3934/era.2023208 |
[8] | Xiangwen Yin . A review of dynamics analysis of neural networks and applications in creation psychology. Electronic Research Archive, 2023, 31(5): 2595-2625. doi: 10.3934/era.2023132 |
[9] | Xiaofeng Chen . Synchronization of heterogeneous harmonic oscillators for generalized uniformly jointly connected networks. Electronic Research Archive, 2023, 31(8): 5039-5055. doi: 10.3934/era.2023258 |
[10] | Janarthanan Ramadoss, Asma Alharbi, Karthikeyan Rajagopal, Salah Boulaaras . A fractional-order discrete memristor neuron model: Nodal and network dynamics. Electronic Research Archive, 2022, 30(11): 3977-3992. doi: 10.3934/era.2022202 |
The modeling of discrete space-time stochastic heterogeneous complex networks with unknown factors was achieved through the utilization of differencing techniques with respect to the time and space variables of the nodes' states. Via the space-time discrete Lyapunov-Krasovskii functional and the approach of linear matrix inequality, this paper derived the mean-squared asymptotic anti-synchronization of the aforementioned discrete networks. This was achieved by defining an updated law for the hitherto unknown parameters and incorporating boundary values within the feedback controller. The theoretical and experimental findings indicated that the feedback controller at the boundary represented a more effective and cost-effective control technique for the networks. Furthermore, an adaptive rule has been designed to identify the uncertainties that occur in the networks with a high degree of accuracy. In particular, this rule enabled the response networks to identify unknown information in the drive networks with a high level of precision by incorporating an adaptive updating mechanism. Finally, a numerical example was provided to elucidate the viability of the ongoing investigation.
Complex dynamical networks (CDNs) refer to systems composed of interconnected nodes or entities, where the relationships between nodes are not trivial and often exhibit nontrivial patterns or structures, e.g., transport networks and 5G communication networks, social networks and Covid-19 epidemic networks. The study of complex networks has become a significant interdisciplinary field, drawing insights and methodologies from mathematics, computer science, physics, biology, sociology, and other disciplines [1,2,3]. Complex networks have found applications in diverse fields, including biology (e.g., gene regulatory networks, protein-protein interaction networks), social sciences (e.g., social networks, collaboration networks), transportation (road networks, air traffic networks), and information systems (e.g., the World Wide Web, citation networks); see papers [4,5,6]. Researchers in this field often use tools from graph theory, statistical physics, and machine learning to analyze and model the intricate structures and behaviors of complex systems (see refs. [7,8,9]).
A system composed of nonidentical networks may refer to a heterogeneous network. It consists of different types of nodes or entities with diverse characteristics, and the connections between them can vary in nature. Examples include multiplex networks, where nodes can have different types of relationships in different layers, and interdependent networks, where the failure of nodes in one network can affect nodes in another. The synchronization of nonidentical networks is a complex and interdisciplinary topic that spans various fields, including physics, engineering, and network science. In the context of nonidentical networks, achieving synchronization implies that nodes with different dynamics or characteristics become correlated or coordinated in their behavior. A common approach is to consider a drive network influencing the behavior of a response network. The drive network might have more influence or a different role compared to the response network, and achieving synchronization involves aligning the dynamics of the two networks. For the synchronization issues of nonidentical CDNs, the readers could refer to the literatures [10,11,12,13,14].
Anti-synchronization of networks involves achieving a specific type of coordination or correlation between nodes in a network. In synchronization, nodes in a network tend to evolve in a coordinated manner. In contrast, anti-synchronization is a state where the nodes exhibit an antiphase relationship, meaning that their dynamics are such that when one node is in a certain state, the other is in the opposite state; see refs. [15,16,17,18,19,20]. Adaptive anti-synchronization involves incorporating mechanisms that allow the network to adapt to changes in its internal or external conditions. These adaptive mechanisms can include adjusting parameters, coupling strengths, or introducing control signals based on feedback from the system or external measurements. Adaptive anti-synchronization has potential applications in various fields, including secure communication systems, networked control systems, and complex systems where maintaining a specific antiphase relationship between components is important, see refs. [18,19,20].
Stochastic network plays a significant role in the evolution of the network. These uncertainties can arise from various sources, including random events, probabilistic interactions, or dynamic processes. Analyzing and modeling stochastic networks is essential for understanding their behavior and making informed decisions in scenarios where uncertainty is inherent; see refs. [21,22,23,24,25,26]. Uncertain networks refer to network structures where there is uncertainty or variability in the connections between nodes, the strengths of these connections, or other properties of the network. This uncertainty can arise from various sources, including incomplete information, dynamic changes in network topology, or random fluctuations. Understanding and modeling uncertain networks are crucial for various applications, and researchers often employ different approaches to address the challenges associated with uncertainty. Researchers have developed various approaches and methodologies to address these challenges; please see publications [27,28,29,30,31,32].
Discrete space or discrete time networks, as opposed to continuous space or continuous time networks, have distinct advantages in various applications. For example, discrete time systems are often easier to synchronize and coordinate. Events or updates can be synchronized at specific time intervals, simplifying the management of network-wide activities. Discrete time models are often simpler to develop and analyze. In signal processing applications, discrete time signals are often preferred for digital signal processing. Discrete time allows for the application of powerful digital filtering techniques and efficient algorithms [33]. On the other hand, discretizing space simplifies computations, making it more computationally efficient, especially for systems with a large number of nodes or complex interactions. Nodes in the network are located at specific points in space, simplifying the description of their positions and connections. In certain applications, discretizing space allows for more efficient utilization of resources [34]. To the authors' knowledge, despite numerous reports having focused on the research of discrete-time complex networks [9,15,35,36,37] or continuous-time complex networks with reaction diffusions [5,38,39,40,41,42], the studies of the discrete networks in both time and space are very few; please see ref. [43]. Specifically, almost no paper involves the adaptive anti-synchronization of time-space discrete drive-response nonidentical CDNs.
Based on the above discussion, the primary purpose of this paper is to study the adaptive anti-synchronization problems of space-time discrete heterogeneous stochastic multilayer CDNs with multiple delays. To achieve this, a space-time discrete Lyapunov-Krasovskii functional and the linear matrix inequality (LMI) method are used to design an adaptive updated law for unknown parameter and feedback controller that incorporates boundary information. In particular, the adaptive parameter in the response networks can achieve the accuracy identification to the unknown information in the drive networks. This document presents key contributions that are distinct from existing works. These contributions are briefly summarized.
1) This paper differs from the continuous time networks in [6,7,8,10,11,12,13,14,44,45,46] or reaction-diffusion networks in [5,38,39,40,41,42]; a CDNs in time and space is modeled by a discrete way.
2) In contrast to the feedback controller in the networks presented in previous reports [9,15,35,36,37], a feedback controller in the boundary is built to discrete space-time CDNs, which is a more effective and low-cost control technique for the networks.
3) In contrast to previous work on the incomplete synchronization to heterogeneous CDNs, e.g., quasi-synchronization [10,11,12,13,14], the present paper addresses the problem of anti-synchronization to heterogeneous discrete space-time CDNs, which belongs to the category of the complete synchronization.
4) In order to fill the gap that no report had been published for the adaptive control of discrete space-time CDNs until now, this paper considers the uncertainty in the model of CDNs and designs an adaptive updated rule. The adaptive parameter in the response networks can achieve the accuracy identification to the uncertain factor.
This article has the following structure. In Section 2, the drive-response discrete space-time heterogeneous CDNs with multiple delays have been established. The adaptive updated law of the unknown parameter and adaptive anti-synchronization of the drive-response heterogeneous CDNs are discussed in Section 3. In Section 4, the theoretical results are confirmed by a numerical example. A brief summary is given in Section 5.
Notations: Denote R0=[0,+∞), Z0={0,1,2,…}; Z+=Z0∖{0}. We use symbol ⊗ to denote the Kronecker product. Define ‖A‖∞=max1≤i≤m,1≤j≤n|aij| for A=(aij)m×n∈Rm×n. For any sets I,J, IJ stands for set I∩J. I represents some suitable dimensional identical matrix. Also, denote
Δz[⋅]k=z[⋅]k+1−z[⋅]k,Δℏz[δ]k=z[δ+1]k−z[δ]kℏ,Δ2ℏz[δ]k=Δℏ(Δℏz[δ]k), |
where z=z[δ]k:Z2→RM, (δ,k)∈Z2, ℏ>0.
In the following model, F,σ∈RM are the activation and noise functions in sequence; Γl∈RM×M and Gl=(Gij,l)m×m represent the inner and outer linking matrix in sequence l=1,2,…,p, i,j=1,2,…,m; w∈R denotes the Brownian motion on certain probability space. This paper discusses the general CDNs with reaction diffusions, unknown dynamics, and multiple delays, which are constructed by
dwi(x,t)=[A∂2wi(x,t)∂x2+Cwi(x,t)+γ(t)F(wi(x,t))+p∑l=1m∑j=1clGij,lΓlwj(x,t−τl)]dt+p∑l=1ˆσ(wi(x,t−τl))dw(t)+ζ, | (2.1) |
where (x,t)∈(0,L)×R0 for some L>0, or in a discrete form
w[δ]i,k+1=eChw[δ]i,k+(eCh−I)C−1[AΔ2ℏw[δ−1]i,k+γkF(w[δ]i,k)+p∑l=1m∑j=1clGij,lΓlw[δ]j,k−τl+p∑l=1σ(w[δ]i,k−τl)Δhwk+ζ], | (2.2) |
where (δ,k)∈(0,L)Z×Z0, L≥2 is some integer; wi=(wi1,wi2,…,wiM)T∈RM stands for the state of the ith transportation node; γk is adaptive; w[δ]i,k and wk are the simplified forms of wi(δℏ,kh) and w(kh) in sequence i=1,2,…,m; Δhwk=wk+1−wk√h; ℏ and h denote the space and time steps' length, respectively; A∈RM×M, C is nonsingular M-order square matrix; cl≥0, ˆσ(⋅)=√hσ(⋅), τl∈[1,+∞)Z is the time delay, l=1,2,…,p; ζ∈RM is a corrective vector. Furthermore, CDNs (2.2) possesses the following initial and controlling boundary values:
{w[δ]i,s=ϕ[δ]i,s,∀(δ,s)∈[0,L]Z×[−τ∞,0]Z,Δℏw[δ]i,k|δ=0=0,Δℏw[δ]i,k|δ=L−1=ξi,k,∀k∈Z0, |
where τ∞:=maxδ=1,2,…,pτl<∞, ξi denotes the boundary input, i=1,2,…,m.
Remark 2.1. CDNs (2.2) is the discrete form of CDNs (2.1) by using the exponential Euler difference (see [33]) for variable t and the central finite difference (see [47,48]) for variable x.
Remark 2.2. It can be seen from the boundary control described above that, in contrast to the feedback controls (FCs) that are designed within the networks, the boundary control described in this study is only active at the boundary point L−1. This control method is a more effective and low-cost technique than the FCs described in the paper [48], which occurs for all values of δ∈[1,L−1]Z.
Let the matrix G⋅, and the activation and noise functions F,σ meet the following assumptions.
(c1) Gl=(Gij,l)m×m is irreducible and Gij,l=Gji,l≥0 for i≠j, Gii,l=−∑mj=1,j≠iGij,l, i,j=1,2,…,m, l=1,2,…,p.
(c2) F(⋅) and σ(⋅) are odd, and there exist positive constant F0, and M-order matrices Lf,Lσ≥0 such that
‖F(z)‖∞≤F0,[F(z)−F(y)]T[F(z)−F(y)]≤(z−y)TLf(z−y),[σ(z)−σ(y)]T[σ(z)−σ(y)]≤(z−y)TLσ(z−y), |
where z,y∈RM.
Let us consider the drive CDNs below:
z[δ]k+1=eChz[δ]k+(eCh−I)C−1[AΔ2ℏz[δ−1]k+γF(z[δ]k)+p∑l=1m∑j=1clGij,lΓlz[δ]k−τl+p∑l=1σ(z[δ]k−τl)Δhwk−ζ], | (2.3) |
where (δ,k)∈(0,L)Z×Z0; γ is an unknown parameter. The initial and boundary values are described by
{z[δ]s=ψ[δ]s,∀(δ,s)∈[0,L]Z×[−τ∞,0]Z,Δℏz[δ]k|δ=0=0=Δℏz[δ]k|δ=L−1,∀k∈Z0. |
CDNs (2.2) can be regarded as the response networks of the drive networks (2.3). Let the anti-synchronous error between the drive-response networks (2.3) and (2.2) be represented by ei=wi+z for i=1,2,…,m. From CDNs (2.3) and (2.2), it follows
e[δ]i,k+1=eChe[δ]i,k+(eCh−I)C−1[AΔ2ℏe[δ−1]i,k+γ˜F(e[δ]i,k)+(γk−γ)F(w[δ]i,k)+p∑l=1m∑j=1clGij,lΓle[δ]j,k−τl+p∑l=1˜σ(e[δ]i,k−τl)Δhwk], |
where i=1,2,…,m, or in a compact form
ˉe[δ]k+1=(eCh)⊗mˉe[δ]k+(C∗)⊗m[(A)⊗mΔ2ℏˉe[δ−1]k+γ˜F⊗(ˉe[δ]k)+(γk−γ)F⊗(w[δ]k)+p∑l=1cl(Γl)⊗Glˉe[δ]k−τl+p∑l=1˜σw⊗(ˉe[δ]k−τl)], | (2.4) |
where (δ,k)∈(0,L)Z×Z0, ˉe=(eT1,…,eTm)T, C∗=(eCh−I)C−1,
˜F(ei)=F(wi)+F(z),˜σ(ei)=σ(wi)+σ(z),i=1,2,…,m,˜F⊗(ˉe)=(˜F(e1),…,˜F(em))T,F⊗(w)=(F(w1),…,F(wm))T,˜σ⊗(ˉe)=(˜σ(e1),…,˜σ(em))T,˜σw⊗(ˉe)=(˜σ(e1),…,˜σ(em))TΔhw. |
In the model above, (A)⊗m:=Im⊗A and (A)⊗B:=B⊗A for suitable dimensional matrices A,B and the m-order identical matrix Im.
According to the initial and boundary values in CDNs (2.2) and (2.3), the error system (2.4) possesses the initial and controlling boundary conditions
{ˉe[δ]s=φ[δ]s,∀(δ,s)∈[0,L]Z×[−τ∞,0]Z,Δℏˉe[δ]k|δ=0=0,Δℏˉe[δ]k|δ=L−1=ˉξk,∀k∈Z0, | (2.5) |
where φ=(ϕ1+ψ,…,ϕm+ψ)T, ˉξ=(ξ1,…,ξm)T.
The framework of the drive-response-error CDNs (2.2)–(2.4) is figured as that in Figure 1.
Remark 2.3. In [43], Zhang and Li discussed a class of discrete space-time CDNs and the issue of switching clusters' synchronization had been addressed perfectly in this article. Compared with the work above, this paper possesses some merits below. First, CDNs (2.2) of this paper is involved with the stochastic disturbances. Second, the drive-response networks (2.2) and (2.3) are heterogeneous, but the CDNs in [43] were homogeneous. Finally, it exists the uncertainty in CDNs (2.2), which extensively increases the researching difficulty and the applicable categories of the networks.
Remark 2.4. Literatures [44,45,46] used adaptive methods to identify and estimate unknown parameters of continuous time transportation networks without spatial variables. However, identifying uncertain parameters in spatiotemporal discrete heterogeneous networks presents two challenges that must be overcome. In the first, the introduction of spatial diffusion will complicate the research of this topic. Additionally, based on the applicant's recent research experience in [43], the calculation of the time discrete Lyapunov function is more complex than that of the continuous time Lyapunov function. You can see this point in Eq (3.6), where the term £k exists, but remember that there is no £k in the continuous case.
Definition 2.1 ([49]). The drive-response networks (2.3) and (2.2) are said to be mean squared asymptotic anti-synchronization (MSAAS) via boundary control ˉξk in (2.5) if
limk→∞m∑i=1L∑δ=0E‖e[δ]i,k‖2=limk→∞m∑i=1L∑δ=0E‖w[δ]i,k+z[δ]k‖2=0,∀k∈Z0. |
Remark 2.5. In the existing publications, papers [9,15,35,36,37] investigated single discrete-time CDNs and reports [5,38,39,40,41,42] considered the continuous-time CDNs with reaction diffusions. The drive-response CDNs (2.3) and (2.2) are distinct with them by discussing both time and space discretizations in the model of CDNs. Although the time-space discrete CDNs has been considered in our work in literature [43], it has some clear distinctions. The biggest difference is that the drive-response CDNs in literature [43] are identical, but drive-response CDNs (2.3) and (2.2) are nonidentical. Besides, this paper considers MSAAS with adaptive updated rule, which is different from the synchronization in literature [43].
Throughout this article, suppose that
m∑i=1L−1∑δ=1E‖ϕ[δ]i,s‖2<∞,L−1∑δ=1E‖ψ[δ]s‖2<∞, |
where s∈[−τ∞,0]Z. By right of initial values (2.5), it has
L−1∑δ=1E‖ˉe[δ]s‖2<∞,∀s∈[−τ∞,0]Z. | (2.6) |
In view of Corollary 3.1 of [50], Subsection 2.3, and Inequalities 3.2.1 and 3.2.3 of [51], one has the following lemma.
Lemma 2.1. ([50,51]). The inequalities below are valid for m-dimensional vector z and m-order positive definitive matrix G.
(i) L−1∑δ=0Δℏz[δ]TGΔℏz[δ]≤μLℏ2L∑δ=0z[δ]TGz[δ] when z0=0, where μL=4cos2π2L+1.
(ii) L−1∑δ=1Δℏz[δ]TGΔℏz[δ]≥χLℏ2L∑δ=1z[δ]TGz[δ]−1ℏ2(z1+zL)TG(z1+zL), where χL=4sin2π2L.
(iii) L−1∑δ=1Δℏz[δ]TGΔℏz[δ]+1ℏ2[z1+(−1)LzL]TG[z1+(−1)LzL]≤4−χLℏ2L∑δ=1z[δ]TGz[δ].
This section constructs the space-time discrete stochastic Lyapunov-Krasovskii functional to the error CDNs (2.4). Subsequently, an adaptive update law of uncertain parameter γ is designed. Further, boundary input and controlling gain are designed to perform mean squared asymptotic anti-synchronization of the drive-response networks (2.3) and (2.2), i.e., some asymptotical stability criterions in mean squared sense are established to the error CDNs (2.4). Meanwhile, it achieves the identification of the uncertain parameter.
In accordance with the error CDNs (2.4), a space-time discrete Lyapunov-Krasovskii functional candidate is constructed by
Vk=V0,k+V1,k+V2,k, |
where
V0,k=1α(γk−γ)2,V1,k=L−1∑δ=1ˉe[δ]Tk(P)⊗mˉe[δ]k,V2,k=L−1∑δ=1p∑l=1k−1∑q=k−τl[d2pˉe[δ]Tq(Lσ)⊗mˉe[δ]q+d3pc2lˉe[δ]Tq(ΓTl)⊗GTl(Γl)⊗Glˉe[δ]q], |
where P>0 is an M-order matrix, and d2,d3,α are some positive constants.
The chief research scheme of this paper can be summarized in the flowchart described by Figure 2.
Set η[δ]k=ˉe[δ]k+1−ˉe[δ]k, ∀(δ,k)∈(0,L)Z×Z0. We design the adaptive updated law for unknown parameter γk as below:
γk+1=γk−Υk,Υk=αL−1∑δ=1[ˉe[δ]Tk(PC∗)⊗m+η[δ]Tk(QC∗)⊗m]F⊗(w[δ]k), | (3.1) |
where k∈Z0. Hereby, Q is a positive definitive M-order matrix, which will be calculated by some LMIs later. Remarkably, it follows from (c2) that
(uTHv)2≤M3‖H‖2∞‖v‖2∞‖u‖2,‖F⊗(w)‖∞≤F0, | (3.2) |
where u,v∈RM,w∈RmM,H∈RM×M. So, it implies
EΥ2k≤2α2LEL−1∑δ=1{[ˉe[δ]Tk(PC∗)⊗mF⊗(w[δ]k)]2+[η[δ]Tk(QC∗)⊗mF⊗(w[δ]k)]2}≤2α2(mM)3LEL−1∑δ=1[‖PC∗‖2∞‖F⊗(w[δ]k)‖2∞ˉe[δ]Tkˉe[δ]k+‖QC∗‖2∞‖F⊗(w[δ]k)‖2∞η[δ]Tkη[δ]k]≤2α2(mM)3F20Lmax{‖PC∗‖2∞,‖QC∗‖2∞}EL−1∑δ=1[ˉe[δ]Tkˉe[δ]k+η[δ]Tkη[δ]k], | (3.3) |
for all k∈Z0. Suppose that
(c3) Constants α,α∗ satisfy 0<2α(mM)3F20Lmax{‖PC∗‖2∞,‖QC∗‖2∞}<α∗.
According to (3.3) and assumption (c3), it yields
E[ΔV0,k]=1αE(γk+1−γ)2−1αE(γk−γ)2=1αE(γk+1−γk)(γk+1+γk−2γ)=1αEΥ2k−2αE[(γk−γ)Υk]≤α∗EL−1∑δ=1[ˉe[δ]Tkˉe[δ]k+η[δ]Tkη[δ]k]−2EL−1∑δ=1(γk−γ)[ˉe[δ]Tk(PC∗)⊗m+η[δ]Tk(QC∗)⊗m]F⊗(w[δ]k),∀k∈Z0. | (3.4) |
Remark 3.1. In the designation of adaptive updated law (3.1), it is dependent of the value of η[δ]k=ˉe[δ]k+1−ˉe[δ]k, namely, the value of ˉe at time k+1. If the current time is k, by the iterative relationship (3.1), the states' values of the response network (2.2) at time k+1 can be determined by the whole relative values at time k or before the time k, and so are the states' values of the response network (2.3).
Remark 3.2. In the construction of adaptation rules for continuous CDNs ([44,45,46]), it was observed that all of them were not involved with the term η[δ]k. In other words, it was ascertained that the second term on the righthand side of Υk in (3.1) does not exist. Why? The rationale will be expounded in the ensuing discourse on heterogeneous anti-synchronization. The calculations of inequalities (3.3) and (3.4) demonstrate that the presence of term η[δ]k greatly increases the complexity and difficulty of the current research.
Remark 3.3. In the studies of nonidentical CDNs [10,11,12,13,14], nobody designs the adaptive updated rule such as Eq (3.1), so the concept of quasi-synchronization was proposed and researched. In this article, an adaptive parameter with updated rule Eq (3.1) has been designed to perform the complete MSAAS for the drive-response CDNs (2.3) and (2.2).
The boundary controlling input is designed by
ˉξk=−L−1∑δ=1(Π)⊗mˉe[δ]k,∀k∈Z0, | (3.5) |
where Π∈RM×M represents the controlling gain.
Theorem 3.1. Let assumptions (c1)–(c3) hold, det(A)≠0, det(C)≠0, ε∈(0,1), and the updated law for adaptive parameter γ and the boundary controlling input be designed as those in (3.1) and (3.5), respectively. Suppose further that
(c4) Eγ20<∞ and |γ|≤γ∗, where γ∗ is a known constant.
(c5) There exist matrices P>0, Q>0, R>0, Φ>0, ℧>0 and positive numbers α∗,d1,d2,d3 so that
−2PC∗A+Lℏ2χL℧+μL−1ℏ2R<0,Ω:=[Ω11Ω120(QC∗A)⊗m00(QC∗)⊗m∗Ω22−1ℏ(Φ)⊗m000(PC∗)⊗m∗∗−(℧)⊗m0000∗∗∗−(R)⊗m000∗∗∗∗Ω5500∗∗∗∗∗Ω660∗∗∗∗∗∗−(d3I)⊗m]<0, |
where
Ω11=(α∗I)⊗m−(2−ε−εγ2∗)(Q)⊗m+(P)⊗m,Ω12=[Q(eCh−I)]⊗m,Ω22=(α∗I)⊗m−2ℏ(Φ)⊗m+εγ2∗(P)⊗m+d1(Lf)⊗m+2[P(eCh−I)]⊗m+d2p2(Lσ)⊗m+d3pp∑l=1c2l(ΓTl)⊗GTl(Γl)⊗Gl,Ω55=−(d1I)⊗m+1ε(CT∗PC∗)⊗m+1ε(CT∗QC∗)⊗m,Ω66=−(d2I)⊗m+1ε(CT∗QC∗)⊗m. |
Then, the response CDNs (2.2) asymptotically inversely synchronizes the drive CDNs (2.3) in the mean squared sense, and E|γk−γ|2→0 as k→∞. Moreover, the controlling gain Π=[PC∗A]−1Φ.
Proof. Taking α fulfills assumption (c3). Based on the Lyapunov-Krasovskii functional as before and the error networks (2.4), it attains
E[ΔV1,k]=EL−1∑δ=1ˉe[δ]Tk+1(P)⊗mˉe[δ]k+1−E[V1,k]=EL−1∑δ=1(η[δ]Tk+ˉe[δ]Tk)(P)⊗m(η[δ]k+ˉe[δ]k)−E[V1,k]=EL−1∑δ=1η[δ]Tk(P)⊗mη[δ]k⏟£k+2EL−1∑δ=1ˉe[δ]Tk(P)⊗mη[δ]k⏟Λ1,k, | (3.6) |
where
Λ1,k=2EL−1∑δ=1ˉe[δ]Tk(P)⊗mη[δ]k=2EL−1∑δ=1ˉe[δ]Tk[P(eCh−I)]⊗mˉe[δ]k+2EL−1∑δ=1ˉe[δ]Tk(PC∗A)⊗mΔ2ℏˉe[δ−1]k⏟Λ2,k+2EL−1∑δ=1γˉe[δ]Tk(PC∗)⊗m˜F⊗(ˉe[δ]k)⏟Θ1,k+2EL−1∑δ=1ˉe[δ]Tk(PC∗)⊗mp∑l=1cl(Γl)⊗Glˉe[δ]k−τl+2EL−1∑δ=1ˉe[δ]Tk(γk−γ)(PC∗)⊗mF⊗(w[δ]k),∀k∈Z0. | (3.7) |
Via the discrete formula of integration by parts and boundary values in (2.5), it computes
Λ2,k=2EL−1∑δ=1ˉe[δ]Tk(PC∗A)⊗mΔ2ℏˉe[δ−1]k=2ℏEˉe[δ]Tk(PC∗A)⊗mΔℏˉe[δ−1]k|L1−2EL−1∑δ=1Δℏˉe[δ]Tk(PC∗A)⊗mΔℏˉe[δ]k=2ℏEˉe[L]Tk(PC∗A)⊗mˉξk⏟ℵk−2EL−1∑δ=1Δℏˉe[δ]Tk(PC∗A)⊗mΔℏˉe[δ]k,∀k∈Z0. | (3.8) |
Define ˆˉe[δ]k=ˉe[L]k−ˉe[δ]k, ∀(δ,k)∈[0,L]Z×Z0. It should be noted that
ˆˉe[L]k=0,ˆˉe[1]k=ˉe[L]k−ˉe[1]k,∀k∈Z0. |
Together with the discrete Wirtinger's inequalities in item (ii) of Lemma 2.1, it results in
EL−1∑δ=1Δℏˉe[δ]Tk(℧)⊗mΔℏˉe[δ]k=EL−1∑δ=1Δℏˆˉe[δ]Tk(℧)⊗mΔℏˆˉe[δ]k≥χLℏ2EL∑δ=1ˆˉe[δ]Tk(℧)⊗mˆˉe[δ]k−1ℏ2E(ˆˉe[L]Tk+ˆˉe[1]Tk)(℧)⊗m(ˆˉe[L]k+ˆˉe[1]k)=χLℏ2EL−1∑δ=1ˆˉe[δ]Tk(℧)⊗mˆˉe[δ]k−1ℏ2E(ˉe[L]Tk−ˉe[1]Tk)(℧)⊗m(ˉe[L]k−ˉe[1]k)=χLℏ2EL−1∑δ=1ˆˉe[δ]Tk(℧)⊗mˆˉe[δ]k−E(L−1∑δ=1Δℏˉe[δ]Tk)(℧)⊗m(L−1∑δ=1Δℏˉe[δ]k)≥χLℏ2EL−1∑δ=1ˆˉe[δ]Tk(℧)⊗mˆˉe[δ]k−(L−1)EL−1∑δ=1Δℏˉe[δ]Tk(℧)⊗mΔℏˉe[δ]k, |
which indicates
EL−1∑δ=1ˆˉe[δ]Tk(℧)⊗mˆˉe[δ]k≤Lℏ2χLEL−1∑δ=1Δℏˉe[δ]Tk(℧)⊗mΔℏˉe[δ]k,∀k∈Z0. | (3.9) |
According to the error CDNs (2.4), it gets
η[δ]k=(eCh−I)⊗mˉe[δ]k+(C∗)⊗m[(A)⊗mΔ2ℏˉe[δ−1]k+γ˜F⊗(ˉe[δ]k)+(γk−γ)F⊗(w[δ]k)+p∑l=1cl(Γl)⊗Glˉe[δ]k−τl+p∑l=1˜σw⊗(ˉe[δ]k−τl)], | (3.10) |
where (δ,k)∈(0,L)×Z0. It induces from (3.10) that
2EL−1∑δ=1η[δ]Tk(Q)⊗mη[δ]k=2EL−1∑δ=1η[δ]Tk[Q(eCh−I)]⊗mˉe[δ]k+2EL−1∑δ=1η[δ]Tk(QC∗A)⊗mΔ2ℏˉe[δ−1]k+2EL−1∑δ=1γη[δ]Tk(QC∗)⊗m˜F⊗(ˉe[δ]k)⏟Θ2,k+2EL−1∑δ=1η[δ]Tk(QC∗)⊗mp∑l=1cl(Γl)⊗Glˉe[δ]k−τl+2EL−1∑δ=1(γk−γ)η[δ]Tk(QC∗)⊗mF⊗(w[δ]k)⏟¶k+2EL−1∑δ=1η[δ]Tk(QC∗)⊗mp∑l=1˜σw⊗(ˉe[δ]k−τl)⏟Λ4,k,∀k∈Z0. | (3.11) |
According to the elementary inequality in Lemma 1.13 of [52], i.e., 2XTY≤εXTX+1εYTY for any X,Y∈RmM and ε>0, it generates
Θ1,k=2EL−1∑δ=1γˉe[δ]Tk(PC∗)⊗m˜F⊗(ˉe[δ]k)≤εγ2∗EL−1∑δ=1ˉe[δ]Tk(P)⊗mˉe[δ]k+1εEL−1∑δ=1˜FT⊗(ˉe[δ]k)(CT∗PC∗)⊗m˜F⊗(ˉe[δ]k), | (3.12) |
and, similarly,
Θ2,k=2EL−1∑δ=1γη[δ]Tk(QC∗)⊗m˜F⊗(ˉe[δ]k)≤εγ2∗EL−1∑δ=1η[δ]Tk(Q)⊗mη[δ]k+1εEL−1∑δ=1˜FT⊗(ˉe[δ]k)(CT∗QC∗)⊗m˜F⊗(ˉe[δ]k),∀k∈Z0. | (3.13) |
From (3.11), we have
Λ4,k=2EL−1∑δ=1η[δ]Tk(QC∗)⊗mp∑l=1˜σw⊗(ˉe[δ]k−τl)≤εEL−1∑δ=1η[δ]Tk(Q)⊗mη[δ]k+1εEL−1∑δ=1p∑l=1[˜σw⊗(ˉe[δ]k−τl)]T(CT∗QC∗)⊗mp∑l=1˜σw⊗(ˉe[δ]k−τl)=εEL−1∑δ=1η[δ]Tk(Q)⊗mη[δ]k+1εEL−1∑δ=1p∑l=1˜σT⊗(ˉe[δ]k−τl)(CT∗QC∗)⊗mp∑l=1˜σ⊗(ˉe[δ]k−τl), | (3.14) |
where k∈Z0. In (3.14), note that Δhwk is independent of ˉe[⋅]s for all s≤k, and we use the fact of E(Δhw⋅)2=1.
In terms of assumption (c2), it deduces
d1EL−1∑δ=1˜FT⊗(ˉe[δ]k)˜F⊗(ˉe[δ]k)≤EL−1∑δ=1ˉe[δ]Tkd1(Lf)⊗mˉe[δ]k, | (3.15) |
d2EL−1∑δ=1p∑l=1˜σT⊗(ˉe[δ]k−τl)p∑l=1˜σ⊗(ˉe[δ]k−τl)≤d2pEL−1∑δ=1p∑l=1ˉe[δ]Tk−τl(Lσ)⊗mˉe[δ]k−τl, | (3.16) |
d3EL−1∑δ=1p∑l=1[cl(Γl)⊗Glˉe[δ]k−τl]Tp∑l=1cl(Γl)⊗Glˉe[δ]k−τl≤d3pEL−1∑δ=1p∑l=1ˉe[δ]Tk−τlc2l(ΓTl)⊗GTl(Γl)⊗Glˉe[δ]k−τl, | (3.17) |
where k∈Z0. Based on the definition of V2,k, we have
E[ΔV2,k]=EV2,k+1−EV2,k=d2p2EL−1∑δ=1ˉe[δ]Tk(Lσ)⊗mˉe[δ]k+d3pEL−1∑δ=1ˉe[δ]Tkp∑l=1c2l(ΓTl)⊗GTl(Γl)⊗Glˉe[δ]k−d2pEL−1∑δ=1p∑l=1ˉe[δ]Tk−τl(Lσ)⊗mˉe[δ]k−τl−d3pEL−1∑δ=1p∑l=1ˉe[δ]Tk−τlc2l(ΓTl)⊗GTl(Γl)⊗Glˉe[δ]k−τl, | (3.18) |
where k∈Z0.
From Lemma 2.1, we have
EL−1∑δ=1Δ2ℏˉe[l−1]Tk(R)⊗mΔ2ℏˉe[δ−1]k≤EL−2∑δ=0Δ2ℏˉe[δ]Tk(R)⊗mΔ2ℏˉe[δ]k≤μL−1ℏ2EL−1∑δ=1Δℏˉe[δ]Tk(R)⊗mΔℏˉe[δ]k,∀k∈Z0. | (3.19) |
In view of (3.8) and by boundary input (3.5), it has
ℵk=2ℏEˉe[L]Tk(PC∗A)⊗mˉξk=−2ℏEL−1∑δ=1ˉe[L]Tk(PC∗AΠ)⊗mˉe[δ]k=−2ℏEL−1∑δ=1ˆˉe[δ]Tk(Φ)⊗mˉe[δ]k−2ℏEL−1∑δ=1ˉe[δ]Tk(Φ)⊗mˉe[δ]k,∀k∈Z0. | (3.20) |
Set
˜Ω=[Ω00−2PC∗A+Lℏ2χL℧+μL−1ℏ2R]. |
On the grounds of (3.4), (3.6)–(3.20), and assumption (c5), it induces ˜Ω<0 and
E[ΔVk]≤EL−1∑δ=1H[δ]Tk˜ΩH[δ]k,∀k∈Z0, |
where
H[δ]k=[η[δ]k,ˉe[δ]k,ˆˉe[δ]k,Δ2ℏˉe[δ−1]k,˜F⊗(ˉe[δ]k),p∑l=1˜σ⊗(ˉe[δ]k−τl),p∑l=1cl(Γl)⊗Glˉe[δ]k−τl,Δℏˉe[δ]k]T |
for any (δ,k)∈(0,L)Z×Z0. Obviously,
k−1∑j=0E[ΔVj]≤Ek−1∑j=0L−1∑δ=1H[δ]TjΩH[δ]j≤Ek−1∑j=0L−1∑δ=1ˉe[δ]TjΩˉe[δ]j≤λ+ΩEk−1∑j=0L−1∑δ=1ˉe[δ]Tjˉe[δ]j⇒Ek−1∑j=0L−1∑δ=1ˉe[δ]Tjˉe[δ]j≤1λ+Ωk−1∑j=0E[ΔVj]≤−1λ+ΩEV0<∞, |
which implies
EL−1∑δ=1ˉe[δ]Tkˉe[δ]k→0, |
as k→∞. Hereby, λ+Ω denotes the maximum eigenvalue of Ω. According to boundary information in (3.5), it exists constant M1>0 so that
‖ˉe[L]k‖2≤M1L−1∑δ=1‖ˉe[δ]k‖2,∀k∈Z0. | (3.21) |
Additionally,
‖ˉe[0]k‖2=‖ˉe[1]k‖2≤L−1∑δ=1‖ˉe[δ]k‖2,∀k∈Z0. | (3.22) |
Together with (3.21) and (3.22), it causes
L∑δ=0‖ˉe[δ]k‖2→0, ask→∞. |
Videlicet, the error CDNs (2.4) achieves global asymptotic stability in the mean squared sense. To wit, the response CDNs (2.2) asymptotically inversely synchronizes the drive CDNs (2.3). Based on the error CDNs (2.4) and the same discussions in [52, Theorem 4.20], it directly gains γk→γ, as k→∞. This achieves the proof.
If γk≡γ for all k∈Z0, then the error CDNs (2.4) is transformed into
ˉe[δ]k+1=(eCh)⊗mˉe[δ]k+[(eCh−I)C−1]⊗m[(A)⊗mΔ2ℏˉe[δ−1]k+γ˜F⊗(ˉe[δ]k)+p∑l=1cl(Γl)⊗Glˉe[δ]k−τl+p∑l=1˜σw⊗(ˉe[δ]k−τl)], | (3.23) |
where (δ,k)∈(0,L)Z×Z0.
By a similar proof to Theorem 3.1, we can gain the corollary below.
Corollary 3.1. Let assumptions (c1)–(c3) hold, det(A)≠0, det(C)≠0, ε∈(0,1), and the boundary input be designed as that in (3.5). Assume that
(c6) γk≡γ for all k∈Z0.
(c7) Assumption (c5) is valid with α∗=0.
Furthermore, the controlling gain is designed as before. Then, the response CDNs (2.2) asymptotically anti-synchronizes the drive CDNs (2.3) in the mean squared sense.
According to Theorem 3.1, a realizable algorithm for adaptive anti-synchronization of the drive-response CDNs (2.2) and (2.3) is designed as follows.
Remark 3.4. Discrete-time CDNs have been the focus of the authors' previous works [9,15,35,36,37]. This article deals with discrete-time CDNs with discrete spatial diffusion. The associated calculations, such as the Lyapunov-Krasovskii functional, are more complex than the case of a single time-variable. In this paper, we present a boundary feedback controller for discrete-time and spatial CDNs, whereas previous reports [9,15,35,36,37] have used a traditional feedback controller for CDNs. Previous studies [9,15,35,36,37] have focused on homogeneous CDNs, whereas this article examines heterogeneous CDNs.
Remark 3.5. Previous publications [10,11,12,13,14] have examined continuous-time heterogeneous networks. In contrast, this article addresses the following aspects: 1) The paper considers the issue of discrete space transportation networks, taking into account their heterogeneity. 2) It achieves mean squared asymptotic anti-synchronization for the networks, which differs significantly from the quasi-synchronization discussed in previous publications [10,11,12,13,14]. 3) The response networks in these publications [10,11,12,13,14] have a predetermined heterogeneous coefficient. We have designed an adaptive parameter in the response networks that tends to drive-response CDNs homogeneously by continuously adjusting it according to the updated law. At this moment, the adaptive parameter in the response CDNs can perfectly identify the unknown parameter in the drive CDNs according to the updated law.
Supposing the drive CDNs are expressed by
z[δ]k+1=eChz[δ]k+(eCh−I)C−1{0.1Δ2ℏz[δ−1]k+γ[0.3000.1][sin(z[δ]1,k)sin(z[δ]2,k)]+[0.10.10.50.5][z[δ]1,k−11+|z[δ]2,k−1|z[δ]2,k−21+|z[δ]1,k−2|]Δhwk−[5.20275.6240]},∀(δ,k)∈(0,6)Z×Z0, | (4.1) |
where z=[z1z2], C=−[80824],h=0.01, ℏ=0.25, γ=0.1. The boundary values of the drive CDNs (4.1) are given by Δℏz[0]k=Δℏz[5]k=0, ∀k∈Z0. The response CDNs of the drive CDNs (4.1) are described as
{w[δ]1,k+1=eChw[δ]1,k+(eCh−I)C−1{0.1Δ2ℏw[δ−1]1,k+˜γk[0.3000.1][sin(w[δ]11,k)sin(w[δ]12,k)]−0.5Γ1w[δ]1,k−1+0.5Γ1w[δ]2,k−1−0.8Γ2w[δ]1,k−2+0.8Γ2w[δ]2,k−2+[0.10.10.50.5][w[δ]11,k−11+|w[δ]12,k−1|w[δ]12,k−21+|w[δ]11,k−2|]Δhwk+[5.20275.6240]},w[δ]2,k+1=eChw[δ]2,k+(eCh−I)C−1{0.1Δ2ℏw[δ−1]2,k+˜γk[0.3000.1][sin(w[δ]21,k)sin(w[δ]22,k)]−0.5Γ1w[δ]1,k−1+0.5Γ1w[δ]2,k−1−0.8Γ2w[δ]1,k−2+0.8Γ2w[δ]2,k−2+[0.10.10.50.5][w[δ]21,k−11+|w[δ]22,k−1|w[δ]22,k−21+|w[δ]21,k−2|]Δhwk+[5.20275.6240]}, | (4.2) |
where (δ,k)∈(0,6)Z×Z0, ˜γ0=0,
w1=[w11w12],w2=[w21w22],Γ1=[0−0.20.70],Γ2=[00.5−0.30]. |
The boundary values of the response CDNs (4.2) are given by Δδw[0]1,k=Δδw[0]2,k=0, Δδw[5]1,k=ξ1,k, Δδw[5]2,k=ξ2,k, ∀k∈Z0.
According to the information above and Algorithm 1, a flow diagram of achieving the adaptive anti-synchronization of the drive-response CDNs (4.1) and (4.2) is drawn in Figure 3.
Algorithm 1 Adaptive anti-synchronization of the drive-response CDNs (2.2) and (2.3) |
(a1) Initialing the values of the coefficients to the drive-response CDNs (2.2) and (2.3) and the value of parameter ε∈(0,1). |
(a2) Computing LMIs in Theorem 3.1. If it is unviable, modify the values of in step (a1); otherwise, switch to next step. |
(a3) Receiving the values of α∗ and m-order matrices in assumption (c5). Calculating the controlling gain defined in (c5) and determining the boundary input in (3.5). |
(a4) Take α to satisfy 0<2α(mM)3F20Lmax{‖PC∗‖2∞,‖QC∗‖2∞}<α∗ and calculate the updated law (3.1) of the unknown parameter γ. |
(a5) Write the iterative program based on the drive-response CDNs (2.2) and (2.3) and plot the response trajectories. |
Obviously, (c1)–(c2) in Theorem 3.1 hold. Taking ε=0.5, γ∗=0.2 and computing the LMIs in Theorem 3.1, the LMIs in (c5) are valid for α∗=1.5763, d1=29.0039, d2=9.1156, d3=10.6818,
P=[1229.112133.173333.1733603.1577],Q=[1149.408538.084938.0849655.5776],R=[0.02040.00060.00060.0103],Φ=[0.33850.04920.04920.2912],℧=[0.6374−0.0027−0.00270.2390]. |
Further, taking α in (c3) small enough, it is easy to ensure that (c3) is satisfied.
So, the gain and controller are determined as
Π=[0.28470.02810.08630.5425],[ξ1,kξ2,k]=−5∑δ=1[0.28470.0281000.08630.542500000.28470.0281000.08630.5425][e[δ]11,ke[δ]12,ke[δ]21,ke[δ]22,k], |
where e[δ]11,k=w[δ]11,k+z[δ]1,k, e[δ]12,k=w[δ]12,k+z[δ]2,k, e[δ]21,k=w[δ]21,k+z[δ]1,k, e[δ]22,k=w[δ]22,k+z[δ]2,k, (δ,k)∈(0,6)Z×Z0. The update law for the adaptive parameter ˜γk is outlined as
˜γk+1=˜γk−0.015∑δ=1[e[δ]11,ke[δ]12,ke[δ]21,ke[δ]22,k]T[11.80040.2949000.10175.3624000011.80040.2949000.10175.3624][sin(w[δ]11,k)sin(w[δ]12,k)sin(w[δ]21,k)sin(w[δ]22,k)]−0.015∑δ=1[e[δ]11,k+1−e[δ]11,ke[δ]12,k+1−e[δ]12,ke[δ]21,k+1−e[δ]21,ke[δ]22,k+1−e[δ]22,k]T[11.03260.3386000.13005.8284000011.03260.3386000.13005.8284][sin(w[δ]11,k)sin(w[δ]12,k)sin(w[δ]21,k)sin(w[δ]22,k)], | (4.3) |
where the initial value is ˜γ0=0, k∈Z+. Apparently, (c4) holds. In sum, all assumptions in Theorem 3.1 are valid. By Theorem 3.1, the response CDNs (4.2) asymptotically inversely synchronizes the drive CDNs (4.1); please see Figures 4–19.
In Figures 4–11, we picture the 3-dimensional asymptotical anti-synchronization of the drive-response CDNs (4.1) and (4.2). Specifically, by taking the space variable δ=3, we also show the trajectories of the errors between the drive-response CDNs (4.1) and (4.2) in the 2-dimensional space; see Figures 12–19. Finally, based upon the update law (4.3) for adaptive parameter ˜γk, we give a variational curve in Figure 20, which tends to γ=0.1 over time.
In the first place, by using mixed approaches of Euler time-difference and finite central space-difference, we consider a delayed multivariable discrete stochastic transportation networks with unknown parameter. In the second place, based on the theory of matrix, the compact drive-response nonidentical stochastic transportation networks with the adaptive parameter are built. Via the method of the Lyapunov-Krasovskii functional and the designation of the update law for the unknown parameter in the response networks, the article performs the mean squared asymptotic anti-synchronization for the drive-response nonidentical stochastic transportation networks. Besides, by designing an adaptive parameter updated rule, the adaptive parameter in the response networks can achieve the accuracy identification to the unknown information in the drive networks. The current work establishes a theory of the problem of asymptotic anti-synchronization for the drive-response nonidentical stochastic transportation networks under the framework of space-time discrete schemes.
The authors declare they have not used Artificial Intelligence (AI) tools in the creation of this article.
This work was supported by the Scientific Research Funds of Educational Department of Yunnan Province under Grant No. 2024J1169.
The authors declare there is no conflicts of interest.
[1] |
M. Ding, Y. Guo, Z. Huang, B. Lin, H. Luo, GROM: A generalized routing optimization method with graph neural network and deep reinforcement learning, J. Network Comput. Appl., 229 (2024), 103927. https://doi.org/10.1016/j.jnca.2024.103927 doi: 10.1016/j.jnca.2024.103927
![]() |
[2] |
F. Dabaghi-Zarandi, P. KamaliPour, Community detection in complex network based on an improved random algorithm using local and global network information, J. Network Comput. Appl., 206 (2022), 103492. https://doi.org/10.1016/j.jnca.2022.103492 doi: 10.1016/j.jnca.2022.103492
![]() |
[3] |
Z. Zhang, L. Cui, J. Wu, Exploring an edge convolution and normalization based approach for link prediction in complex networks, J. Network Comput. Appl., 189 (2021), 103113. https://doi.org/10.1016/j.jnca.2021.103113 doi: 10.1016/j.jnca.2021.103113
![]() |
[4] |
B. D. Nallamuthu, S. Rathinasamy, H. Shanmugam, G. Ran, Disturbance rejection-based cluster synchronization of nonlinear transportation networks with hybrid-driven mechanism, Int. J. Robust Nonlinear Control, 33 (2023), 8715–8730. https://doi.org/10.1002/rnc.6845 doi: 10.1002/rnc.6845
![]() |
[5] |
B. Lu, H. Jiang, C. Hu, A. Abdurahman, M. Liu, Adaptive pinning cluster synchronization of a stochastic reaction-diffusion complex network, Neural Networks, 166 (2023), 524–540. https://doi.org/10.1016/j.neunet.2023.07.034 doi: 10.1016/j.neunet.2023.07.034
![]() |
[6] |
P. Khanra, S. Ghosh, K. Alfaro-Bittner, P. Kundu, S. Boccaletti, C. Hens, et al., Identifying symmetries and predicting cluster synchronization in transportation networks, Chaos, Solitons Fractals, 155 (2022), 111703. https://doi.org/10.1016/j.chaos.2021.111703 doi: 10.1016/j.chaos.2021.111703
![]() |
[7] |
E. S. Mwanandiye, B. Wu, Q. Jia, Synchronization of delayed dynamical networks with multi-links via intermittent pinning control, Neural Comput. Appl., 32 (2019), 11277–11284. https://doi.org/10.1007/s00521-019-04614-x doi: 10.1007/s00521-019-04614-x
![]() |
[8] |
L. H. Zhao, S. Wen, M. Xu, K. Shi, S. Zhu, T. Huang, PID control for output synchronization of multiple output coupled transportation networks, IEEE Trans. Network Sci. Eng., 9 (2022), 1553–1566. https://doi.org/10.1109/TNSE.2022.3147786 doi: 10.1109/TNSE.2022.3147786
![]() |
[9] |
R. Sakthivel, O. M. Kwon, M. J. Park, S. G. Choi, R. Sakthivel, Robust asynchronous filtering for discrete-time T–S fuzzy transportation networks against deception attacks, IEEE Trans. Fuzzy Syst., 30 (2022), 3257–3269. https://doi.org/10.1109/TFUZZ.2021.3111453 doi: 10.1109/TFUZZ.2021.3111453
![]() |
[10] |
D. Xu, N. Hong, H. Su, Quasi-synchronization of stochastic heterogeneous networks via intermittent pinning sampled-data control, Expert Syst. Appl., 238 (2024), 121867. https://doi.org/10.1016/j.eswa.2023.121867 doi: 10.1016/j.eswa.2023.121867
![]() |
[11] |
J. Sun, L. Xiang, Finite-time quasi-synchronization of multi-layer heterogeneous networks with distributed hybrid control, Neurocomputing, 566 (2024), 127046. https://doi.org/10.1016/j.neucom.2023.127046 doi: 10.1016/j.neucom.2023.127046
![]() |
[12] |
C. Jin, Z. Wang, L. Gong, M. Xiao, G. P. Jiang, Quasi-synchronization of heterogeneous Lur'e networks with uncertain parameters and impulsive effect, Neurocomputing, 482 (2022), 252–263. https://doi.org/10.1016/j.neucom.2021.11.057 doi: 10.1016/j.neucom.2021.11.057
![]() |
[13] |
T. Chen, New effective approach to quasi synchronization of coupled heterogeneous transportation networks, Neural Networks, 145 (2022), 139–143. https://doi.org/10.1016/j.neunet.2021.10.019 doi: 10.1016/j.neunet.2021.10.019
![]() |
[14] |
D. M. Semenov, A. L. Fradkov, Adaptive synchronization in the complex heterogeneous networks of Hindmarsh-Rose neurons, Chaos, Solitons Fractals, 150 (2021), 111170. https://doi.org/10.1016/j.chaos.2021.111170 doi: 10.1016/j.chaos.2021.111170
![]() |
[15] |
K. S. R. Priyanka, G. Soundararajan, A. Kashkynbayev, G. Nagamani, Exponential H∞ synchronization and anti-synchronization of delayed discrete-time complex-valued neural networks with uncertainties, Math. Comput. Simul., 207 (2023), 301–321. https://doi.org/10.1016/j.matcom.2023.01.002 doi: 10.1016/j.matcom.2023.01.002
![]() |
[16] |
Y. Huang, L. Su, General decay anti-synchronization and H∞ anti-synchronization of coupled reaction-diffusion complex-valued memristive neural networks, Commun. Nonlinear Sci. Numer. Simul., 126 (2023), 107451. https://doi.org/10.1016/j.cnsns.2023.107451 doi: 10.1016/j.cnsns.2023.107451
![]() |
[17] |
P. Wan, D. Sun, M. Zhao, Finite-time and fixed-time anti-synchronization of Markovian neural networks with stochastic disturbances via switching control, Neural Networks, 123 (2020), 1–11. https://doi.org/10.1016/j.neunet.2019.11.012 doi: 10.1016/j.neunet.2019.11.012
![]() |
[18] | J. Jiang, X. Jin, J. Chi, X. Wu, Distributed adaptive fixed-time synchronization for disturbed transportation networks, Chaos, Solitons Fractals, 173 (2023). https://doi.org/10.1016/j.chaos.2023.113612 |
[19] |
F. W. Alsaade, Q. Yao, S. Bekiros, M. S. Al-zahrani, A. S. Alzahrani, H. Jahanshahi, Chaotic attitude synchronization and anti-synchronization of master-slave satellites using a robust fixed-time adaptive controller, Chaos, Solitons Fractals, 165 (2022), 112883. https://doi.org/10.1016/j.chaos.2022.112883 doi: 10.1016/j.chaos.2022.112883
![]() |
[20] |
W. Xu, S. Zhu, X. Y. Fang, W. Wang, Adaptive anti-synchronization of memristor-based complex-valued neural networks with time delays, Physica A, 535 (2019), 122427. https://doi.org/10.1016/j.physa.2019.122427 doi: 10.1016/j.physa.2019.122427
![]() |
[21] |
F. Tatari, H. Modares, Deterministic and stochastic fixed-time stability of discrete-time autonomous systems, IEEE/CAA J. Autom. Sin., 10 (2023), 945–956. https://doi.org/10.1109/JAS.2023.123405 doi: 10.1109/JAS.2023.123405
![]() |
[22] |
Z. C. Su, A. H. F. Chow, C. L. Fang, E. M. Liang, R. X. Zhong, Hierarchical control for stochastic network traffic with reinforcement learning, Transp. Res. Part B Methodol., 167 (2023), 196–216. https://doi.org/10.1016/j.trb.2022.12.001 doi: 10.1016/j.trb.2022.12.001
![]() |
[23] |
Q. Q. Li, Z. P. Wang, T. Huang, H. N. Wu, H. X. Li, J. Qiao, Fault-tolerant stochastic sampled-data fuzzy control for nonlinear delayed parabolic PDE systems, IEEE Trans. Fuzzy Syst., 31 (2023), 2679–2693. https://doi.org/10.1109/TFUZZ.2023.3235400 doi: 10.1109/TFUZZ.2023.3235400
![]() |
[24] |
B. Li, X. Xie, Convergence of a spatial semidiscretization for a backward semilinear stochastic parabolic equation, SIAM J. Control Optim., 61 (2023), 47–71. https://doi.org/10.1137/21M1453700 doi: 10.1137/21M1453700
![]() |
[25] |
R. Zhang, H. Wang, J. H. Park, P. He, D. Zeng, X. Xie, Fuzzy secure control for nonlinear N-D parabolic PDE-ODE coupled systems under stochastic deception attacks, IEEE Trans. Fuzzy Syst., 30 (2022), 3347–3359. https://doi.org/10.1109/TFUZZ.2021.3113580 doi: 10.1109/TFUZZ.2021.3113580
![]() |
[26] |
H. Bessaih, M. J. Garrido-Atienza, V. Köpp, B. Schmalfuß, Synchronization of stochastic lattice equations and upper semicontinuity of attractors, Stochastic Anal. Appl., 40 (2021), 1067–1103. https://doi.org/10.1080/07362994.2021.1981383 doi: 10.1080/07362994.2021.1981383
![]() |
[27] |
R. Tang, X. Yang, P. Shi, Z. Xiang, L. Qing, Finite-time L2 stabilization of uncertain delayed T-S fuzzy systems via intermittent control, IEEE Trans. Fuzzy Syst., 32 (2024), 116–125. https://doi.org/10.1109/TFUZZ.2023.3292233 doi: 10.1109/TFUZZ.2023.3292233
![]() |
[28] |
Y. Kao, Y. Cao, Y. Chen, Projective synchronization for uncertain fractional reaction-diffusion systems via adaptive sliding mode control based on finite-time scheme, IEEE Trans. Neural Networks Learn. Syst., 35 (2024), 15638–15646. https://doi.org/10.1109/TNNLS.2023.3288849 doi: 10.1109/TNNLS.2023.3288849
![]() |
[29] |
C. Hao, B. Wang, D. Tang, Global asymptotic stability and projective lag synchronization for uncertain inertial competitive neural networks, Math. Methods Appl. Sci., 46 (2023), 17137–17157. https://doi.org/10.1002/mma.9492 doi: 10.1002/mma.9492
![]() |
[30] |
Y. Wang, J. Zhao, Periodic event-triggered sliding mode control for switched uncertain T-S fuzzy systems with a logistic adaptive event-triggering scheme, IEEE Trans. Fuzzy Syst., 30 (2022), 4115–4126. https://doi.org/10.1109/TFUZZ.2022.3141749 doi: 10.1109/TFUZZ.2022.3141749
![]() |
[31] | W. Sun, Z. Wang, X. Lv, F. E. Alsaadi, H. Liu, H∞ fusion estimation for uncertain discrete time-delayed Hamiltonian systems with sensor saturations: An event-triggered approach, Inf. Fusion, 86–87 (2022), 93–103. |
[32] |
S. Kuppusamy, Y. H. Joo, Stabilization criteria for T–S fuzzy systems with multiplicative sampled-data control gain uncertainties, IEEE Trans. Fuzzy Syst., 30 (2022), 4082–4092. https://doi.org/10.1109/TFUZZ.2021.3138633 doi: 10.1109/TFUZZ.2021.3138633
![]() |
[33] |
T. W. Zhang, Y. K. Li, Global exponential stability of discrete-time almost automorphic Caputo-Fabrizio BAM fuzzy neural networks via exponential Euler technique, Knowl.-Based Syst., 246 (2022), 108675. https://doi.org/10.1016/j.knosys.2022.108675 doi: 10.1016/j.knosys.2022.108675
![]() |
[34] |
T. W. Zhang, Y. Y. Yang, S. F. Han, Node-to-node clustering asymptotic synchronized discrete stochastic neural networks in time and space with Bernoulli switching delay, Chin. J. Phys., 92 (2024), 743–754. https://doi.org/10.1016/j.cjph.2024.09.007 doi: 10.1016/j.cjph.2024.09.007
![]() |
[35] |
N. Sakthivel, S. Pallavi, Y. K. Ma, V. Vijayakumar, Finite-time dissipative synchronization of discrete-time semi-Markovian jump transportation networks with actuator faults, Soft Comput., 26 (2022), 8371–8386. https://doi.org/10.1007/s00500-022-07207-4 doi: 10.1007/s00500-022-07207-4
![]() |
[36] |
J. Y. Li, Z. Wang, R. Lu, Y. Xu, Cluster synchronization control for discrete-time transportation networks: when data transmission meets constrained bit rate, IEEE Trans. Neural Networks Learn. Syst., 34 (2023), 2554–2568. https://doi.org/10.1109/TNNLS.2021.3106947 doi: 10.1109/TNNLS.2021.3106947
![]() |
[37] |
S. Ding, Z. Wang, X. Xie, Periodic event-triggered synchronization for discrete-time transportation networks, IEEE Trans. Neural Networks Learn. Syst., 33 (2022), 3622–3633. https://doi.org/10.1109/TNNLS.2021.3053652 doi: 10.1109/TNNLS.2021.3053652
![]() |
[38] |
M. S. Ali, L. Palanisamy, N. Gunasekaran, A. Alsaedi, B. Ahmad, Finite-time exponential synchronization of reaction-diffusion delayed complex-dynamical networks, Discrete Contin. Dyn. Syst. - Ser. S, 14 (2021), 1465–1477. https://doi.org/10.3934/dcdss.2020395 doi: 10.3934/dcdss.2020395
![]() |
[39] |
Y. Luo, Y. Yao, Z. Cheng, X. Xiao, H. Liu, Event-triggered control for coupled reaction–diffusion complex network systems with finite-time synchronization, Physica A, 562 (2021), 125219. https://doi.org/10.1016/j.physa.2020.125219 doi: 10.1016/j.physa.2020.125219
![]() |
[40] |
X. Liu, Y. Kao, Aperiodically intermittent pinning outer synchronization control for delayed complex dynamical networks with reaction-diffusion terms, Appl. Math. Comput., 410 (2021), 126406. https://doi.org/10.1016/j.amc.2021.126406 doi: 10.1016/j.amc.2021.126406
![]() |
[41] |
D. Y. Wang, J. L. Wang, Boundary control for passivity of multiple state or spatial diffusion coupled parabolic transportation networks with and without control input constraint, Int. J. Control, 94 (2020), 2783–2794. https://doi.org/10.1080/00207179.2020.1736340 doi: 10.1080/00207179.2020.1736340
![]() |
[42] |
M. S. Ali, J. Yogambigai, O.M. Kwon, Finite-time robust passive control for a class of switched reaction-diffusion stochastic transportation networks with coupling delays and impulsive control, Int. J. Syst. Sci., 49 (2018), 718–735. https://doi.org/10.1080/00207721.2017.1421723 doi: 10.1080/00207721.2017.1421723
![]() |
[43] |
T. W. Zhang, Z. H. Li, Switching clusters' synchronization for discrete space-time transportation networks via boundary feedback controls, Pattern Recognit., 143 (2023), 109763. https://doi.org/10.1016/j.patcog.2023.109763 doi: 10.1016/j.patcog.2023.109763
![]() |
[44] |
X. Wang, H. B. Gu, Q. Y. Wang, J. H. Lü, Identifying topologies and system parameters of uncertain time-varying delayed transportation networks, Sci. China Technol. Sci., 62 (2019), 94–105. ttps://doi.org/10.1007/s11431-018-9287-0 doi: 10.1007/s11431-018-9287-0
![]() |
[45] |
X. Chen, J. Zhang, T. Ma, Parameter estimation and topology identification of uncertain general fractional-order transportation networks with time delay, IEEE/CAA J. Autom. Sin., 3 (2016), 295–303. https://doi.org/10.1109/JAS.2016.7508805 doi: 10.1109/JAS.2016.7508805
![]() |
[46] |
H. Liu, J. A. Lu, J. H. Lü, D. J. Hill, Structure identification of uncertain general complex dynamical networks with time delay, Automatica, 45 (2009), 1799–1807. https://doi.org/10.1016/j.automatica.2009.03.022 doi: 10.1016/j.automatica.2009.03.022
![]() |
[47] |
T. W. Zhang, Y. Y. Yang, S. F. Han, Exponential heterogeneous anti-synchronization of multi-variable discrete stochastic inertial neural networks with adaptive corrective parameter, Eng. Appl. Artif. Intell., 142 (2025), 109871. https://doi.org/10.1016/j.engappai.2024.109871 doi: 10.1016/j.engappai.2024.109871
![]() |
[48] |
T. W. Zhang, H. Z. Qu, Y. T. Liu, J. W. Zhou, Weighted pseudo θ-almost periodic sequence solution and guaranteed cost control for discrete-time and discrete-space stochastic inertial neural networks, Chaos, Solitons Fractals, 173 (2023), 113658. https://doi.org/10.1016/j.chaos.2023.113658 doi: 10.1016/j.chaos.2023.113658
![]() |
[49] |
D. Zhang, Q. G. Wang, D. Srinivasan, H. Li, L. Yu, Asynchronous state estimation for discrete-time switched complex networks with communication constraints, IEEE Trans. Neural Networks Learn. Syst., 29 (2018), 1732–1746. https://doi.org/10.1109/TNNLS.2017.2678681 doi: 10.1109/TNNLS.2017.2678681
![]() |
[50] |
A. Seuret, E. Fridman, Wirtinger-like Lyapunov-Krasovskii functionals for discrete-time delay systems, IMA J. Math. Control Inf., 35 (2018), 861–876. https://doi.org/10.1093/imamci/dnx002 doi: 10.1093/imamci/dnx002
![]() |
[51] | K. Mollaiyan, Generalization of Discrete-Time Wirtinger Inequalities and A Preliminary Study of Their Application to SNR Analysis of Sinusoids Buried in Noise, Masters thesis, Concordia University, 2008. |
[52] | W. N. Zhou, J. Yang, L. W. Zhou, D. B. Tong, Stability and Synchronization Control of Stochastic Neural Networks, Springer-Verlag, Berlin, Heidelberg, 2016. |