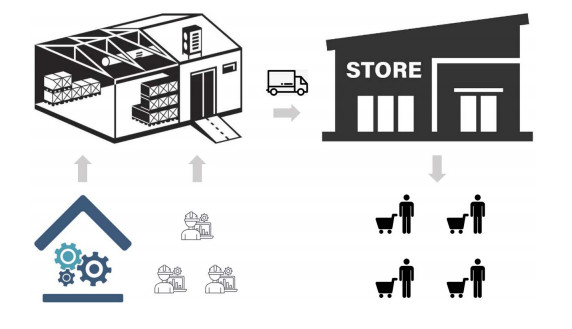
This paper focused on the decision and regulation for the production-warehousing-selling (P-W-S) model. A novel event-triggered mechanism (ETM) was meticulously developed to determine when to impose control, alongside the development of the corresponding impulsive strategy. By applying the input-to-state stability (ISS) theory, some quantitative relationships between system parameters and ETM were integrated into the estimation of the state of the P-W-S model. It was shown that under the designed ETM and impulsive strategy, the warehouse was able to autonomously adjust inventory levels based on factory production efficiency and market selling trends, and hence the quantity of goods could be maintained within a reasonable range, avoiding excessive inventory while adequately meeting market requirements. At last, an example with numerical simulations was presented to validate our results.
Citation: Ziqi Liu. An event-based decision and regulation strategy for the production-warehousing-selling model[J]. Electronic Research Archive, 2024, 32(7): 4614-4631. doi: 10.3934/era.2024210
[1] | Chengbo Yi, Jiayi Cai, Rui Guo . Synchronization of a class of nonlinear multiple neural networks with delays via a dynamic event-triggered impulsive control strategy. Electronic Research Archive, 2024, 32(7): 4581-4603. doi: 10.3934/era.2024208 |
[2] | Xiaoming Wang, Yunlong Bai, Zhiyong Li, Wenguang Zhao, Shixing Ding . Observer-based event triggering security load frequency control for power systems involving air conditioning loads. Electronic Research Archive, 2024, 32(11): 6258-6275. doi: 10.3934/era.2024291 |
[3] | Xingyue Liu, Kaibo Shi, Yiqian Tang, Lin Tang, Youhua Wei, Yingjun Han . A novel adaptive event-triggered reliable $ H_\infty $ control approach for networked control systems with actuator faults. Electronic Research Archive, 2023, 31(4): 1840-1862. doi: 10.3934/era.2023095 |
[4] | Yawei Liu, Guangyin Cui, Chen Gao . Event-triggered synchronization control for neural networks against DoS attacks. Electronic Research Archive, 2025, 33(1): 121-141. doi: 10.3934/era.2025007 |
[5] | Zongying Feng, Guoqiang Tan . Dynamic event-triggered $ H_{\infty} $ control for neural networks with sensor saturations and stochastic deception attacks. Electronic Research Archive, 2025, 33(3): 1267-1284. doi: 10.3934/era.2025056 |
[6] | Denggui Fan, Yingxin Wang, Jiang Wu, Songan Hou, Qingyun Wang . The preview control of a corticothalamic model with disturbance. Electronic Research Archive, 2024, 32(2): 812-835. doi: 10.3934/era.2024039 |
[7] | Yan Xie, Rui Zhu, Xiaolong Tan, Yuan Chai . Inhibition of absence seizures in a reduced corticothalamic circuit via closed-loop control. Electronic Research Archive, 2023, 31(5): 2651-2666. doi: 10.3934/era.2023134 |
[8] | Xinling Li, Xueli Qin, Zhiwei Wan, Weipeng Tai . Chaos synchronization of stochastic time-delay Lur'e systems: An asynchronous and adaptive event-triggered control approach. Electronic Research Archive, 2023, 31(9): 5589-5608. doi: 10.3934/era.2023284 |
[9] | Ran Yan, Shuaian Wang . Ship detention prediction using anomaly detection in port state control: model and explanation. Electronic Research Archive, 2022, 30(10): 3679-3691. doi: 10.3934/era.2022188 |
[10] | Shuang Liu, Tianwei Xu, Qingyun Wang . Effect analysis of pinning and impulsive selection for finite-time synchronization of delayed complex-valued neural networks. Electronic Research Archive, 2025, 33(3): 1792-1811. doi: 10.3934/era.2025081 |
This paper focused on the decision and regulation for the production-warehousing-selling (P-W-S) model. A novel event-triggered mechanism (ETM) was meticulously developed to determine when to impose control, alongside the development of the corresponding impulsive strategy. By applying the input-to-state stability (ISS) theory, some quantitative relationships between system parameters and ETM were integrated into the estimation of the state of the P-W-S model. It was shown that under the designed ETM and impulsive strategy, the warehouse was able to autonomously adjust inventory levels based on factory production efficiency and market selling trends, and hence the quantity of goods could be maintained within a reasonable range, avoiding excessive inventory while adequately meeting market requirements. At last, an example with numerical simulations was presented to validate our results.
With the vigorous development of modern market economy, a growing number of enterprises are inclined to build a complete industrial chain structure to ensure their dominant position in the market. The production-warehousing-selling (P-W-S) model refers to a comprehensive management model and stands as a pivotal framework. It describes the seamless integration of production, warehousing, and selling activities, and provides a comprehensive perspective on the dynamic interactions within supply chains [1,2], thereby addressing the fundamental challenges encountered in managing the flow of goods from production facilities to end consumers. First, the production phase involves the planning and execution of manufacturing processes. Efficient production scheduling and resource allocation are critical to ensuring optimal utilization of manufacturing capacities and minimizing production costs. Subsequently, the warehousing phase entails the storage, handling, and management of inventory within designated facilities. Effective warehouse management is essential for maintaining inventory accuracy, optimizing storage space utilization, and facilitating timely order fulfillment. Moreover, advanced inventory control techniques, such as just-in-time inventory management [3], can be employed to streamline warehouse operations and reduce holding costs. Finally, the selling phase encompasses the activities involved in marketing, selling, and distributing goods to customers. A robust selling strategy is imperative for driving revenue growth, expanding market reach, and enhancing customer satisfaction. By applying selling forecasting methods and customer relationship management systems [4], enterprises can adjust their selling strategy to meet evolving customer requirements and market trends. Overall, the P-W-S model serves as a foundational framework for supply chain optimization and strategic decision-making, which enables enterprises to enhance operational efficiency, minimize costs, and capitalize on market opportunities in the current business environment [5,6,7,8].
Impulsive control has obtained significant attention in recent years as a powerful technique for regulating and stabilizing dynamic systems in numerous fields, including engineering [9], physics [10], biology [11], economics [12], and so on. To be more precise, "impulsive control" refers to the use of impulses for control purposes at the predetermined impulsive instants [13]. This characteristic is advantageous for installation, implementation, and maintenance since it lowers the resource requirements for control updates and information transmission [14,15,16]. For the decision of an impulsive time sequence, the most advanced one is the so-called event-triggered strategy, whose principle lies in the utilization of an event-triggered mechanism (ETM) to determine when to update the control [17,18]. These events can be defined based on a variety of criteria, such as changes in system states, deviations from desired trajectories, or the occurrence of specific events in the environment. By monitoring these events and updating impulsive control only when necessary, event-triggered impulsive control (ETIC) minimizes the computational burden and communication cost, while ensuring that control objectives are met with high precision and efficiency. In summary, ETIC represents an effective approach for dynamic systems, and its discrete nature, combined with its adaptability and efficiency, makes it well-suited for addressing the challenges posed by complex and uncertain environments. Through intensive research, ETIC continues to offer innovative schemes to a wide range of control problems and remains a promising avenue in engineering practice [19,20,21,22].
Input-to-state stability (ISS) is a fundamental concept in the control field that provides a comprehensive framework for assessing the stability of nonlinear systems subject to external input [23]. It means that for any initial condition, system states will eventually approach a neighborhood of origin, and the state evolution is determined by the size of external input. As a result, ISS is crucial for designing robust control systems, and is a powerful tool for analyzing the dynamic performance of various control systems, especially for impulsive systems [24,25,26,27,28,29,30]. The challenge of designing robust model predictive control for linear parameter-varying systems, for example, was tackled by [26]. The triggered condition was derived using the ISS concept, which reduced the burden of information interaction. In the study of nonlinear impulsive systems with external disturbance, [27] searched for dwell-time conditions that ensured the ISS property. In particular, the obtained conditions included the possibility of simultaneous instability of continuous and discontinuous dynamics.
This paper aims to provide an effective decision and regulation strategy for the P-W-S model so as to enhance operational efficiency, minimize costs, and capitalize on market opportunities. For this purpose, we first model the P-W-S as a nonlinear system that presents original hybrid impulses and external input. A novel ETM coupled with impulsive strategy is then designed and the potential Zeno behavior is excluded. Specially, owing to the original hybrid impulses, the triggered cases are more complicated and some novel methods of analysis and induction are developed. Additionally, compared with the existing results [18,31,32], our designed ETM permits the system to autonomously decide the frequency and intensity of the control input according to its current state without any other artificial intervention. By applying the ISS theory, some sufficient estimation conditions for the P-W-S model are derived, under which the quantity of goods can be maintained within a reasonable range, thereby avoiding excessive inventory while adequately meeting market requirements.
In what follows, the fundamental P-W-S model and some preliminaries are introduced in Section 2. Subsequently, Section 3 designs an effective ETM and addresses the estimation problem for the quantity of goods. A numerical example is discussed in Section 4 to validate the results and, finally, Section 5 closes this paper along with some concluding remarks.
Notations: The supremum in the interval J is labeled as |⋅|J. Given a symmetric matrix A, its minimum and maximum eigenvalues are respectively expressed as λmin(A) and λmax(A). Given any set of constants {hi}i∈Z+, Σ0i=1hi is specified as 0.
In the P-W-S model, our primary goal lies in monitoring the quantity of each type of good in the warehouse and adjusting inventory levels based on factory production efficiency and market selling trends. Specifically, the main factory manufactures goods and stores them in the warehouse. Concurrently, several subsidiary factories also produce goods and periodically deliver them to the warehouse. Due to the simultaneous presence of multiple types of good in the warehouse, the quantity of each type of good is constrained, and this constraint fluctuates as the quantities of other goods vary. Once the quantity of a particular type of good in the warehouse reaches its constraint, it must be dispatched to various stores for selling. According to the market requirement, regular shipments are necessary to ensure adequate supply, even if the inventory levels have not reached their upper constraints. In this process, the rework and wastage of goods are possible, and the unqualified goods will be transformed to be qualified by factories. Besides, due to the changes in factory productivity or transportation capability, a certain nonlinearity should be taken into account in the P-W-S model. See Figure 1 for more details.
Based on the above statement, we consider the following P-W-S model with hybrid impulses:
{˙x(t)=Sx(t)+RF(x(t))+Bω(t), t≠τk,x(t)=Gkx(t−), t=τk, | (2.1) |
where x∈Rp represents the quantities of p types of goods in the warehouse, and ˙x denotes its right-hand derivative. F∈Rp is a smooth nonlinear vector-valued function and F(0)=0, which may be caused by the changes in factory productivity or transportation capability. ω(t)∈Rq is a measurable external input satisfying 0<|ω(t)|≤Δ, which represents the possible rework and wastage of goods. S∈Rp×p,R∈Rp×p, and B∈Rp×q are known parameter matrices, which stand for the coupling relationships between different types of goods, such as the binding relationships between camera and film, coffee and milk, and so on. Gk is the hybrid impulsive gain representing the sudden change in the quantities of goods at τk. {τk}k∈Z+ is a class of impulsive time sequences satisfying τk−τk−1≥τ with a positive constant τ. It is assumed that all of the signals are right continuous and have left limits at all instants. The solution to system (2.1) with initial value x(t0)=x0 uniquely exists in [t0,∞).
Assumption 1. There is a positive constant lf such that |F(x)−F(y)|≤lf|x−y|, where x,y∈Rp.
Definition 1. Given a locally Lipschitz function V:Rp→R+, define the upper right-hand Dini derivative of V with respect to system (2.1) as
D+V(x)=lim suph→0+1h(V(x+hg(x,ω))−V(x)). |
Definition 2. [33] For the prescribed sequence {τk}k∈Z+, system (2.1) is said to be input-to-state stable (ISS) if there exist functions ϑ∈KL and χ∈K∞ such that for every initial condition x0 and each bounded external input ω(t), it holds that
|x(t)|≤ϑ(|x0|,t−t0)+χ(|ω|[t0,t]), t≥t0. |
Assumption 2. [34] For any real vectors x,y and symmetric positive matrix Q with compatible dimension, the following inequality holds:
2xTy≤xTQ−1x+yTQy. |
By constructing a Lyapunov candidate V(x(t))=xT(t)Px(t), where P∈Rp×p, the following lemma can be derived to estimate the dynamic behavior of system (2.1). For convenience, we denote V(t)=V(x(t)).
Lemma 1. Under Assumptions 1 and 2, if there exist positive matrices P,U,Q, constant μk, and positive constants γ,lf such that
−γP+PS+STP+PRU−1RTU+l2fU+PBQ−1BTP≤0, | (2.2) |
−exp(μk)P+GTkPGk≤0, | (2.3) |
then we have
D+V(t)≤cV(t), whenever V(t)≥φ(|ω|[t0,t]),V(τk)≤exp(μk)V(τ−k), k∈Z+, |
where c=γ+υ,φ(s)=λmax(Q)(s2)υ, and υ is an arbitrary positive constant.
Proof. Assume that x(t)=x(t,t0,x0) is the solution to system (2.1) with initial value (t0,x0). Taking the time derivative of V(t) along the trajectory of (2.1) in [τk−1,τk) yields
D+V(t)=2xT(t)P˙x(t)=xT(t)(PS+STP)x(t)+2xT(t)PRF(x(t))+2xT(t)PBω(t). |
According to Assumptions 1 and 2, one gets 2xT(t)PRF(x(t))≤xT(t)PRU−1RTPx(t)+l2fxT(t)Ux(t) and 2xT(t)PBω(t)≤xT(t)PBQ−1BTPx(t)+ωT(t)Qω(t). Then condition (2.2) derives that
D+V(t)≤γV(t)+λmax(Q)|ω(t)|2≤γV(t)−υV(t)+υV(t)+λmax(Q)|ω(t)|2, |
which further implies that
D+V(t)≤cV(t), whenever V(t)≥φ(|ω|[t0,t]), | (2.4) |
where c=γ+υ>0,φ(s)=λmax(Q)(s2)υ, and υ is an arbitrary positive constant.
When t=τk, condition (2.3) means that
V(τk)=xT(τk)Px(τk)=xT(τ−k)GTkPGkx(τ−k)≤exp(μk)V(τ−k). |
Assumption 3. [35] There is a constant ˉμ such that ˉμ=maxk∈Z+μk. Also, impulsive gain sequence {μk}k∈Z+ satisfies the average impulsive gain condition. That is, there is a constant μ such that
μm+μm+1+...+μm+hh+1≤μ. |
This section will apply the ISS theory into the estimation of the dynamic behavior of the P-W-S model. First of all, we tend to develop an effective decision strategy for determining when to dispatch goods to various stores for selling purposes, thereby enhancing operational efficiency, minimizing costs, and capitalizing on market opportunities.
In light of the Lyapunov candidate V(t) given previously, we construct the following event:
Ξn−1(t)=exp(ζn−λ(t−tn−1))V(tn−1)+δφ(|ω|[t0,t]), | (3.1) |
where λ>0,δ>1, and ζn>0 satisfying Σ+∞n=1ζn=+∞. Then we present an event-triggered mechanism (ETM) as
tn=inf{t≥tn−1|V(t)≥Ξn−1(t)}, | (3.2) |
where {tn}n∈Z+ is the triggered time sequence. Subsequently, impulsive control strategy is designed for regulation purposes and is in the form of x(tn)=Knx(t−n), where Kn represents the control gain.
Remark 1. ETM (3.2) allows the system to autonomously decide the frequency of impulsive control input according to its current state, and provides a dynamic threshold for the state of the system (2.1). Please refer to Figure 2 for details (the red line represents the original hybrid impulses and the gray line represents the designed stabilized impulse).
Remark 2. It is possible that the original hybrid impulses and the event-based impulse occur simultaneously at some instants. However, in this scenario, it does not mean that the original impulse is ignored. Without loss of generality, it is assumed that the original impulse is the destabilizing impulse. Within the framework of our studied P-W-S model, this process means that the quantity of goods in the warehouse reaches the constraint and, at the same time, the goods produced by subsidiary factories are delivered to the warehouse. In this scenario, the goods in the warehouse should be dispatched to stores first, so as to make room for the newly produced goods, which are then put into the warehouse. That is, the system state will be reset first according to the event-based impulse and then the original destabilizing impulse updates the system state. Clearly, the occurring order of the event-based impulse and the original destabilizing impulse should be more realistic in the P-W-S model. Besides, it is possible that the original destabilizing impulse triggers the event (3.2), and then the event-based impulse will be input at the next instant. It is shown that the goods produced by subsidiary factories are delivered to the warehouse, which makes the quantity of goods in the warehouse reach the constraint. Then, these goods must be dispatched to various stores for selling.
Under the designed ETM (3.2), the following result is presented to rule out the potential Zeno behavior [36].
Theorem 1. Under Assumptions 1–3 and Lemma 1, there is no Zeno behavior for system (2.1) under ETM (3.2). Moreover, the triggered time sequence {tn}n∈Z+ satisfies
tn−tn−1≥ζnμτ+c+λ∧lnδμτ+c. | (3.3) |
Proof. Assume that x(t)=x(t,t0,x0) is the solution to system (2.1) with initial value (t0,x0). Specially, the Zeno behavior can be ruled out naturally if the event is triggered finitely many times. Thereby, we here focus on when the event is triggered infinitely many times. For any interval [tn−1,tn),n∈Z+, if V(t)≥φ(|ω|[t0,t]) holds for all t∈[tn−1,tn), then Lemma 1 gives D+V(t)≤cV(t). Let N(t,s) denote the number of the original hybrid impulses in [s,t), and one then gets
V(t)≤exp(ΣN(t,t0)k=N(tn−1,t0)+1μk+c(t−tn−1))V(tn−1), t∈[tn−1,tn), |
which gives
V(t−n)≤exp(ΣN(tn,t0)k=N(tn−1,t0)+1μk+c(tn−tn−1))V(tn−1). |
Due to the existence of the original hybrid impulses, it can be obtained from (3.2) that
V(t−n)≥exp(ζn−λ(tn−tn−1))V(tn−1)+δφ(|ω|[t0,tn]), |
which leads to
exp(ζn−λ(tn−tn−1))V(tn−1)+δφ(|ω|[t0,tn])≤exp(ΣN(tn,t0)k=N(tn−1,t0)+1μk+c(tn−tn−1))V(tn−1). |
Thus, it holds that
ζn−λ(tn−tn−1)≤ΣN(tn,t0)k=N(tn−1,t0)+1μk+c(tn−tn−1). |
Note that Assumption 3 means that ΣN(tn,t0)k=N(tn−1,t0)+1μk≤tn−tn−1τμ, from which we can get that
tn−tn−1≥ζnμτ+c+λ. |
If there exist t∈[tn−1,tn) such that V(t)≤φ(|ω|[t0,t]), due to that δ>1, then it holds that V(t−n)≥exp(ζn−λ(tn−tn−1))V(tn−1)+δφ(|ω|[t0,tn])≥φ(|ω|[t0,tn]). Thus, given t∗n:=sup{t≥tn−1|V(t)≤φ(|ω|[t0,t])}, one has that V(t)≥φ(|ω|[t0,t]),t∈[t∗n,tn). Similarly, we have
V(t−n)≤exp(ΣN(tn,t0)k=N(t∗n,t0)+1μk+c(tn−t∗n))V(t∗n). |
According to the definition of t∗n, one gets V(t∗n)≤φ(|ω|[t0,t∗n]), under which one has
exp(ζn−λ(tn−tn−1))V(tn−1)+δφ(|ω|[t0,tn])≤exp(ΣN(tn,t0)k=N(t∗n,t0)+1μk+c(tn−t∗n))φ(|ω|[t0,tn]). |
Moreover, it is derived that
δ≤exp((μτ+c)(tn−t∗n)), |
which gives that
tn−tn−1≥tn−t∗n≥lnδμτ+c. |
Thus in all cases, one finally obtains
tn−tn−1≥ζnμτ+c+λ∧lnδμτ+c. |
Summarily, the Zeno behavior can be excluded with
tn≥n∑i=1(ζiμτ+c+λ∧lnδμτ+c)+t0. |
Remark 3. Theorem 1 is presented to rule out the possible Zeno behavior in ETM (3.2), thereby preventing the event from being triggered infinitely fast. Otherwise, it will greatly increase the transportation cost. Besides, by analyzing condition (3.3), it is clear that both the larger μ and the lower τ can decrease the triggered intervals, meaning that the high strength and high density of the original impulses will drive the event to be triggered more frequently to achieve the ISS property.
Remark 4. Owing to the fact that τk−τk−1≥τ holds for all k∈Z+, the extreme case that Remark 2 discussed will not cause the Zeno behavior.
Theorem 2. Under Assumptions 1–3 and Lemma 1, if conditions (2.2), (2.3), and the following hold
−exp(−ϱn)P+KTnPKn≤0, n∈Z+, | (3.4) |
infn∈Z+{ϱn−ζn+1−ˉμ}>0, | (3.5) |
where ϱn>0, then system (2.1) is ISS under ETM (3.2).
Proof. Assume that x(t)=x(t,t0,x0) is the solution to system (2.1) with initial value (t0,x0). When t=tn, it follows from condition (3.4) that
V(tn)=xT(tn)Px(tn)=xT(t−n)KTnPKnx(t−n)≤exp(−ϱn)V(t−n). | (3.6) |
In view of the existence of the original hybrid impulses, here we define an auxiliary function as
σ(t−n)={1, if tn∈{τk}∩{tn},0, if tn∉{τk}∩{tn}. |
Given the characteristics of ETM (3.2), we consider three cases:
Case 1: Event (3.2) is never triggered. In this case, one has
V(t)≤exp(ζ1−λ(t−t0))V(t0)+δφ(|ω|[t0,t]), ∀t∈[t0,t). | (3.7) |
Case 2: Event (3.2) is triggered finitely many times. For t∈[t0,t1), one gets
V(t)≤exp(σ(t−1)ˉμ)[exp(ζ1−λ(t−t0))V(t0)+δφ(|ω|[t0,t])]=exp(σ(t−1)ˉμ+ζ1−λ(t−t0))V(t0)+exp(σ(t−1)ˉμ)δφ(|ω|[t0,t]), ∀t∈[t0,t1). | (3.8) |
For all [t1,t2), it is clear that
V(t)≤exp(σ(t−2)ˉμ)[exp(ζ2−λ(t−t1))V(t1)+δφ(|ω|[t0,t])]=exp(σ(t−2)ˉμ+ζ2−λ(t−t1))V(t1)+exp(σ(t−2)ˉμ)δφ(|ω|[t0,t]). |
According to (3.8), it holds that
V(t−1)≤exp(σ(t−1)ˉμ+ζ1−λ(t1−t0))V(t0)+exp(σ(t−1)ˉμ)δφ(|ω|[t0,t1]), |
which combining with (3.6) gives
V(t1)≤exp(−ϱ1)V(t−1)=exp(σ(t−1)ˉμ+ζ1−ϱ1−λ(t1−t0))V(t0)+exp(σ(t−1)ˉμ−ϱ1)δφ(|ω|[t0,t1]). |
Thereby, it is deduced that
V(t)≤exp(σ(t−2)ˉμ+σ(t−1)ˉμ+ζ2+ζ1−ϱ1−λ(t−t0))V(t0)+exp(σ(t−2)ˉμ+σ(t−1)ˉμ+ζ2−ϱ1)δφ(|ω|[t0,t])+exp(σ(t−2)ˉμ)δφ(|ω|[t0,t]), t∈[t1,t2). | (3.9) |
For all t∈[t2,t3), it is clear that
V(t)≤exp(σ(t−3)ˉμ)[exp(ζ3−λ(t−t2))V(t2)+δφ(|ω|[t0,t])]=exp(σ(t−3)ˉμ+ζ3−λ(t−t2))V(t2)+exp(σ(t−3)ˉμ)δφ(|ω|[t0,t]). |
According to (3.6) and (3.9), one can obtain
V(t2)≤exp(σ(t−2)ˉμ+σ(t−1)ˉμ+ζ2+ζ1−ϱ2−ϱ1−λ(t2−t0))V(t0)+exp(σ(t−2)ˉμ+σ(t−1)ˉμ+ζ2−ϱ2−ϱ1)δφ(|ω|[t0,t2])+exp(σ(t−2)ˉμ−ϱ2)δφ(|ω|[t0,t2]), |
which further gives
V(t)≤exp(σ(t−3)ˉμ+σ(t−2)ˉμ+σ(t−1)ˉμ+ζ3+ζ2+ζ1−ϱ2−ϱ1−λ(t−t0))V(t0)+exp(σ(t−3)ˉμ+σ(t−2)ˉμ+σ(t−1)ˉμ+ζ3+ζ2−ϱ2−ϱ1)δφ(|ω|[t0,t])+exp(σ(t−3)ˉμ+σ(t−2)ˉμ+ζ3−ϱ2)δφ(|ω|[t0,t])+exp(σ(t−3)ˉμ)δφ(|ω|[t0,t]), ∀t∈[t2,t3). |
Eventually, after repeating the above steps, we come to a conclusion as follows:
V(t)≤exp[ˉμ+ζ1−λ(t−t0)+n−1∑i=1(σ(t−i+1)ˉμ+ζi+1−ϱi)]V(t0)+exp(ˉμ)[1+n−1∑j=1exp(n−1∑i=j(σ(t−i+1)ˉμ+ζi+1−ϱi))]δφ(|ω|[t0,t]), ∀t∈[tn−1,tn). | (3.10) |
Case 3: Event (3.2) is triggered infinitely many times. According to Theorem 1, there is no Zeno behavior. Repeating the similar discussions as in Case 2, (3.10) still holds for all t∈[tn−1,tn).
Summarily, with all three cases, it can be obtained that (3.10) is always true. Let ϖ=infn∈Z+{ϱn−ζn+1−ˉμ}, then according to condition (3.5), one eventually concludes that
V(t)≤exp(ˉμ+ζ1−λ(t−t0))V(t0)+exp(ˉμ)δ1−e−ϖφ(|ω|[t0,t]), ∀t∈[tn−1,tn). | (3.11) |
Thereby, the ISS property for system (2.1) under ETM (3.2) is achieved.
Remark 5. Recently, using event-triggered impulsive control, extensive research on the ISS issue has been reported, see [18,31,32] and the references therein. It should be pointed out that the ETMs designed in the previous results generally involve an additional time-triggered mechanism. In this scene, the event will be triggered to impose unnecessary control input if the event is not triggered for a given period, even if the function V(t) does not reach the event threshold, possibly causing waste of resources. By contrast, ETM (3.2) allows the system to autonomously decide the frequency and intensity of control input according to its current state, without any other artificial intervention.
In view of the fact that there are some unknown matrices involved in the LMI-based conditions when we guarantee the ISS property of the P-W-S model (2.1), a feasible algorithm deserves to be developed. Through some reasonable transformations, the crucial conditions can be respectively rewritten as
γ,lf,μk,ϱn,ζn, s.t.{[−γP+PS+STP+l2fUPRPB⋆−U0⋆⋆−Q]≤0,[−exp(μk)PGTkP⋆−P]≤0, k∈Z+,[−exp(−ϱn)PZn⋆−P]≤0, n∈Z+,infn∈Z+{ϱn−ζn+1−ˉμ}>0. | (3.12) |
Based on the above translation processes, the feasible solutions P,U,Q,Zn can be obtained. Moreover, the control gain can be designed by Kn=P−1ZTn. Next, some crucial discussions will be given for decision and regulation of the P-W-S model (2.1) under ETM (3.2):
● It is shown that the triggered frequency may be adjusted by varying the event parameters ζn and λ : i) The event threshold for the system state will be small if we select a tiny value for ζn, thereby resulting in more frequent triggering. Conversely, it can potentially reduce the frequency of impulsive control with a larger ζn; ii) Between any two adjacent triggered instants, event Ξn−1(t) presents the tendency of convergence. Specially, the system state will converge to the origin with decay rate λ for any vanishing external input ω(t). Therefore, in real-world scenarios, ζn and λ can be varied to attain the required performance of the system.
● Condition (3.5) reveals the relationships among ETM, original impulses, and impulsive control. Specially, the larger value of ϱn suggests that impulsive control has a stronger effect and performs better when stabilizing the system. The value of ζn+1 is permitted to be larger in this situation. In other words, the event's boundedness increases, permitting the longer triggered interval. Conversely, a lower value of ϱn suggests that the role of impulsive control might be weakened, meaning that ζn+1 should have a lower value and the event should occur more frequently so as to stabilize the system. Thereby, our developed ETM (3.2) offers a relatively flexible approach to impulsive control.
● It also deserves to be mentioned that original hybrid impulses may prompt the system state to exceed the threshold of event (3.1), i.e., V(t)>Ξn−1(t), and hence there are some k and n satisfying that τk=tn. In this case, condition (3.5) means that the control gain must be larger than the disturbance gain, thereby counteracting the negative effect of hybrid impulses on the ISS property.
At the end of this section, the event-based impulsive strategy for decision and regulation of the P-W-S model is illustrated by Algorithm 1.
Algorithm 1 An event-based impulsive strategy for decision and regulation of the P-W-S model |
Input: Step size h; initial instant t0; total time Δ; dimension p; system parameters S,R,B,Gk, event parameters ζn,λ,δ; control gains Kn. |
Output: The quantities of p types of goods. |
1: Initialization with given x0; |
2: for t do t0 to t0+Δ |
3: Update the state x(t) using (2.1); |
4: Compute the threshold by formal (3.1); |
5: if V(t)≥Ξn−1(t) then |
6: The event has been triggered, and record triggered instant tn←t,n←n−1; |
7: Update the state x(t) at triggered instant tn with x(tn)=Knx(t−n); |
8: else |
9: Update the state x(t) at instant t which belongs to interval [tn−1,tn); |
10: if t=τk then |
11: Update the state x(t) at instant τk with x(τk)=Gnx(τ−k); |
12: end if |
13: end if |
14: end for |
This section provides a numerical example to validate our results. Specially, consider the P-W-S model with two types of goods that are proportionally bundled, such as cameras and film, coffee and milk, and so on. The corresponding model is given as follows:
[˙ξ˙θ]=[0.50.20.20.4][ξθ]+[0.20.20.30.2][sat(ξ)sat(θ)]+[0.20.30.10.1][tanh(t)sin(t)], | (4.1) |
where ξ and θ are respectively the quantities of good I and good II. Figure 3 shows that the dynamic behavior of (4.1) is non-ISS. Our purpose here is to design ETM (3.2) for model (4.1) such that it is ISS. For the original impulses in (4.1), it is assumed that τ3k−2=2k−1.5,τ3k−1=2k−1, and τ3k=2k−0.5 with
G3k−2=[1.10.10.11.1], G3k−1=[10.20.11], G3k=[0.50.200.5], k∈Z+. |
Without loss of generality, we let Kn≡K,ϱn≡ϱ, and ζn≡ζ for all n∈Z+. Given γ=3.01,lf=1,μ3k−2=0.38,μ3k−1=0.27,μ3k=−1,ϱ=0.89, and ζ=0.3 satisfying ϱ−ζ−ˉμ>0, by solving LMI (3.13), it is deduced that
P=[3.1821−0.7084−0.70845.6998], U=[2.52101.27201.27203.2495],Q=[4.0662−2.6556−2.65564.0327], Zn≡[1.57830.7637−1.09423.1925], |
from which we deduce that
K=[0.5408−0.02540.00120.5321]. |
Consequently, the ISS performance of (4.1) is guaranteed theoretically. Let λ=2 and δ=1.1, the corresponding ETM is designed as
tn=inf{t≥tn−1|V(t)≥exp(0.3−2(t−tn−1))V(tn−1)+1.1φ(|ω|[t0,t])}. | (4.2) |
For simulation purposes, given ξ0=2 and θ0=1.5, then under ETM (4.2), the state trajectories of (4.1) and the corresponding triggered time sequence are displayed by Figure 4. Besides, based on the discussion in Subsection 3.3, Figure 5 illustrates that the triggered time sequence varies as ζ changes. Specially, the event will be triggered in a lower frequency as the value of ζ increases.
Note that it has been pointed out in Remark 5 that the ETMs containing the time-triggered mechanism in [18,31,32] may impose unnecessary control input and hence cause waste of resources. To support this statement, here we insert the time-triggered period T=1 into ETM (4.2), and then one has
{tn=t∘n∧(tn−1+T),t∘n=inf{t≥tn−1|V(t)≥exp(0.3−2(t−tn−1))V(tn−1)+1.1φ(|ω|[t0,t])}, | (4.3) |
under which Figure 6 displays the state trajectories of (4.1) and the corresponding triggered time sequence. Comparing Figure 4 with 6, it is clear that the total triggered number under (4.3) is 6 times more than that under (4.2). Thus, the transmission burden and control cost can be reduced significantly under our designed ETM, which corresponds with the discussion in Remark 5. Moreover, as is shown in Figure 7, the total triggered number will increase as the time-triggered period is shortened, indicating that the frequent artificial intervention should be avoided.
The ETM and impulsive control are respectively designed for the decision and regulation purposes of the P-W-S model. Unlike traditional time-triggered control, ETM can autonomously adjust the frequency of control according to the current system state, thereby avoiding the excessive consumption of resources. By using the ISS theory, some sufficient criteria are derived, which can effectively exclude infinitely fast triggering behavior and guarantee that the quantity of goods can be maintained in a reasonable range. It is shown that our designed strategy of decision and regulation can reasonably allocate resources and dynamically adjust the relationship between production and selling according to market requirements.
The authors declare they have not used Artificial Intelligence (AI) tools in the creation of this article.
The authors thank the College of Economics and Management, Northwest A & F University.
The authors declare there is no conflict of interest.
[1] |
L. M. A. Chan, D. Simchi-Levi, J. Swann, Pricing, production, and inventory policies for manufacturing with stochastic demand and discretionary sales, Manuf. Serv. Oper. Manage., 8 (2006), 149–168. https://doi.org/10.1287/msom.1060.0100 doi: 10.1287/msom.1060.0100
![]() |
[2] |
H. Andersson, A. Hoff, M. Christiansen, G. Hasle, A. Løkketangen, Industrial aspects and literature survey: combined inventory management and routing, Comput. Oper. Res., 37 (2010), 1515–1536. https://doi.org/10.1016/j.cor.2009.11.009 doi: 10.1016/j.cor.2009.11.009
![]() |
[3] | T. Cheng, S. Podolsky, Just-in-time Manufacturing: An Introduction, Springer Science & Business Media, 1996. |
[4] | F. Buttle, S. Maklan, Customer Relationship Management: Concepts and Technologies, Routledge, 2019. https://doi.org/10.4324/9781351016551 |
[5] |
H. AL-Khazraji, C. Cole, W. Guo, Optimization and simulation of dynamic performance of production–inventory systems with multivariable controls, Mathematics, 9 (2021), 568. https://doi.org/10.3390/math9050568 doi: 10.3390/math9050568
![]() |
[6] |
M. Çimen, C. Kirkbride, Approximate dynamic programming algorithms for multidimensional flexible production-inventory problems, Int. J. Prod. Res., 55 (2017), 2034–2050. https://doi.org/10.1080/00207543.2016.1264643 doi: 10.1080/00207543.2016.1264643
![]() |
[7] |
R. Li, L. Xiao, D. Yao, Dynamic pricing and production control in a two-item make-to-stock system with flexible dual sourcing and lost sales, Prod. Oper. Manage., 32 (2023), 3119–3137. https://doi.org/10.1111/poms.14026 doi: 10.1111/poms.14026
![]() |
[8] |
Y. S. Huang, C. C. Fang, Y. A. Lin, Inventory management in supply chains with consideration of logistics, green investment and different carbon emissions policies, Comput. Ind. Eng., 139 (2020), 106207. https://doi.org/10.1016/j.cie.2019.106207 doi: 10.1016/j.cie.2019.106207
![]() |
[9] |
S. Baldi, A. Papachristodoulou, E. B. Kosmatopoulos, Adaptive pulse width modulation design for power converters based on affine switched systems, Nonlinear Anal. Hybrid Syst., 30 (2018), 306–322. https://doi.org/10.1016/j.nahs.2018.07.002 doi: 10.1016/j.nahs.2018.07.002
![]() |
[10] |
G. Wolfowicz, J. J. Morton, Pulse techniques for quantum information processing, eMagRes, 5 (2016), 1515–1528. https://doi.org/10.1002/9780470034590.emrstm1521 doi: 10.1002/9780470034590.emrstm1521
![]() |
[11] |
B. Liu, L. Chen, Y. Zhang, The effects of impulsive toxicant input on a population in a polluted environment, J. Biol. Syst., 11 (2003), 265–274. https://doi.org/10.1142/S0218339003000907 doi: 10.1142/S0218339003000907
![]() |
[12] |
R. Korn, Portfolio optimisation with strictly positive transaction costs and impulse control, Finance Stochastics, 2 (1998), 85–114. https://doi.org/10.1007/s007800050034 doi: 10.1007/s007800050034
![]() |
[13] |
J. Lu, B. Jiang, W. X. Zheng, Potential impacts of delay on stability of impulsive control systems, IEEE Trans. Autom. Control, 67 (2022), 5179–5190. https://doi.org/10.1109/TAC.2021.3120672 doi: 10.1109/TAC.2021.3120672
![]() |
[14] |
W. H. Chen, W. Xu, W. X. Zheng, Sliding-mode-based impulsive control for a class of time-delay systems with input disturbance, Automatica, 164 (2024), 111633. https://doi.org/10.1016/j.automatica.2024.111633 doi: 10.1016/j.automatica.2024.111633
![]() |
[15] |
S. Yu, Z. Yu, H. Jiang, A rumor propagation model in multilingual environment with time and state dependent impulsive control, Chaos, Solitons Fractals, 182 (2024), 114779. https://doi.org/10.1016/j.chaos.2024.114779 doi: 10.1016/j.chaos.2024.114779
![]() |
[16] |
Z. Liu, M. Luo, J. Cheng, I. Katib, K. Shi, Security synchronization problem for stochastic complex networks via event-triggered impulsive control with actuation delays, Commun. Nonlinear Sci. Numer. Simul., 133 (2024), 107958. https://doi.org/10.1016/j.cnsns.2024.107958 doi: 10.1016/j.cnsns.2024.107958
![]() |
[17] |
X. Li, P. Li, Input-to-state stability of nonlinear systems: event-triggered impulsive control, IEEE Trans. Autom. Control, 67 (2022), 1460–1465. https://doi.org/10.1109/TAC.2021.3063227 doi: 10.1109/TAC.2021.3063227
![]() |
[18] |
B. Liu, D. J. Hill, Z. Sun, Stabilisation to input-to-state stability for continuous-time dynamical systems via event-triggered impulsive control with three levels of events, IET Control Theory Appl., 12 (2018), 1167–1179. https://doi.org/10.1049/iet-cta.2017.0820 doi: 10.1049/iet-cta.2017.0820
![]() |
[19] |
Z. Hu, X. Mu, Event-triggered impulsive control for stochastic networked control systems under cyber attacks, IEEE Trans. Syst. Man Cybern.: Syst., 52 (2021), 5636–5645. https://doi.org/10.1109/TSMC.2021.3130614 doi: 10.1109/TSMC.2021.3130614
![]() |
[20] |
K. Zhang, E. Braverman, Event-triggered impulsive control for nonlinear systems with actuation delays, IEEE Trans. Autom. Control, 68 (2022), 540–547. https://doi.org/10.1109/TAC.2022.3142127 doi: 10.1109/TAC.2022.3142127
![]() |
[21] |
S. Baek, H. Lee, S. Han, Communication-efficient event-triggered time-delay control and its application to robot manipulators, IEEE Trans. Ind. Electron., 69 (2021), 9288–9297. https://doi.org/10.1109/TIE.2021.3114696 doi: 10.1109/TIE.2021.3114696
![]() |
[22] |
Y. Zhou, Y. Guo, C. Liu, H. Peng, H. Rao, Synchronization for markovian master-slave neural networks: an event-triggered impulsive approach, Int. J. Syst. Sci., 54 (2023), 2551–2565. https://doi.org/10.1080/00207721.2022.2122904 doi: 10.1080/00207721.2022.2122904
![]() |
[23] |
E. Sontag, Smooth stabilization implies coprime factorization, IEEE Trans. Autom. Control, 34 (1989), 435–443. https://doi.org/10.1109/9.28018 doi: 10.1109/9.28018
![]() |
[24] |
H. Zhu, X. Li, S. Song, Input-to-state stability of nonlinear impulsive systems subjects to actuator saturation and external disturbance, IEEE Trans. Cybern., 53 (2023), 173–183. https://doi.org/10.1109/TCYB.2021.3090803 doi: 10.1109/TCYB.2021.3090803
![]() |
[25] |
G. Şahan, D. Özdemir, Uniform asymptotic and input to state stability by indefinite lyapunov functions, Eur. J. Control, 76 (2024), 100945. https://doi.org/10.1016/j.ejcon.2023.100945 doi: 10.1016/j.ejcon.2023.100945
![]() |
[26] |
R. Heydari, M. Farrokhi, Robust event-triggered model predictive control of polytopic lpv systems: an input-to-state stability approach, Syst. Control Lett., 163 (2022), 105202. https://doi.org/10.1016/j.sysconle.2022.105202 doi: 10.1016/j.sysconle.2022.105202
![]() |
[27] |
S. Dashkovskiy, V. Slynko, Dwell-time stability conditions for infinite dimensional impulsive systems, Automatica, 147 (2023), 110695. https://doi.org/10.1016/j.automatica.2022.110695 doi: 10.1016/j.automatica.2022.110695
![]() |
[28] |
P. Bachmann, N. Bajcinca, Average dwell-time conditions for input-to-state stability of impulsive systems, IFAC-PapersOnLine, 53 (2020), 1980–1985. https://doi.org/10.1016/j.ifacol.2020.12.2564 doi: 10.1016/j.ifacol.2020.12.2564
![]() |
[29] |
S. Dashkovskiy, P. Feketa, Input-to-state stability of impulsive systems and their networks, Nonlinear Anal. Hybrid Syst., 26 (2017), 190–200. https://doi.org/10.1016/j.nahs.2017.06.004 doi: 10.1016/j.nahs.2017.06.004
![]() |
[30] |
H. Zhu, J. Lu, J. Lou, Y. Liu, Saturated control for uncertain nonlinear impulsive systems with non-uniformly distributed packet loss, Nonlinear Anal. Hybrid Syst., 51 (2024), 101438. https://doi.org/10.1016/j.nahs.2023.101438 doi: 10.1016/j.nahs.2023.101438
![]() |
[31] |
X. Li, H. Zhu, S. Song, Input-to-state stability of nonlinear systems using observer-based event-triggered impulsive control, IEEE Trans. Syst. Man Cybern.: Syst., 51 (2020), 6892–6900. https://doi.org/10.1109/TSMC.2020.2964172 doi: 10.1109/TSMC.2020.2964172
![]() |
[32] |
H. Zhu, J. Lu, J. Lou, Event-triggered impulsive control for nonlinear systems: the control packet loss case, IEEE Trans. Circuits Syst. II Express Briefs, 69 (2022), 3204–3208. https://doi.org/10.1109/TCSII.2022.3140346 doi: 10.1109/TCSII.2022.3140346
![]() |
[33] |
J. P. Hespanha, D. Liberzon, A. R. Teel, Lyapunov conditions for input-to-state stability of impulsive systems, Automatica, 44 (2008), 2735–2744. https://doi.org/10.1016/j.automatica.2008.03.021 doi: 10.1016/j.automatica.2008.03.021
![]() |
[34] | S. Boyd, L. El Ghaoui, E. Feron, V. Balakrishnan, Linear Matrix Inequalities in System and Control Theory, SIAM, 1994. https://doi.org/10.1137/1.9781611970777 |
[35] | K. Gao, J. Lu, W. X. Zheng, X. Chen, Synchronization in coupled neural networks with hybrid delayed impulses: average impulsive delay-gain method, IEEE Trans. Neural Networks Learn. Syst., (2024), 1–10. https://doi.org/10.1109/TNNLS.2024.3357515 |
[36] |
S. Dashkovskiy, P. Feketa, Asymptotic properties of zeno solutions, Nonlinear Anal. Hybrid Syst., 30 (2018), 256–265. https://doi.org/10.1016/j.nahs.2018.06.005 doi: 10.1016/j.nahs.2018.06.005
![]() |