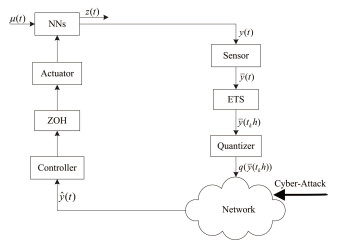
This paper is devoted to dealing with the dynamic event-triggered H∞ quantized control for neural networks with sensor saturations and stochastic deception attacks. To save the limited network resources, a dynamic event-triggered scheme is offered, which includes the general one. And a lower trigger frequency can be obtained by appropriately adjusting the triggering error. Then, a new closed-loop quantized control model is established under a dynamic event-triggered scheme, sensor saturations, and stochastic deception attacks, which is described by two independent Bernoulli-distributed variables. Moreover, by Lyapunov-Krasovskii functional theory, a new H∞ performance criterion is given, and based on the criterion, the controller design approach is derived. Finally, simulations are listed to verify the validity of derived methods.
Citation: Zongying Feng, Guoqiang Tan. Dynamic event-triggered H∞ control for neural networks with sensor saturations and stochastic deception attacks[J]. Electronic Research Archive, 2025, 33(3): 1267-1284. doi: 10.3934/era.2025056
[1] | Yawei Liu, Guangyin Cui, Chen Gao . Event-triggered synchronization control for neural networks against DoS attacks. Electronic Research Archive, 2025, 33(1): 121-141. doi: 10.3934/era.2025007 |
[2] | Xiaoming Wang, Yunlong Bai, Zhiyong Li, Wenguang Zhao, Shixing Ding . Observer-based event triggering security load frequency control for power systems involving air conditioning loads. Electronic Research Archive, 2024, 32(11): 6258-6275. doi: 10.3934/era.2024291 |
[3] | Xingyue Liu, Kaibo Shi, Yiqian Tang, Lin Tang, Youhua Wei, Yingjun Han . A novel adaptive event-triggered reliable $ H_\infty $ control approach for networked control systems with actuator faults. Electronic Research Archive, 2023, 31(4): 1840-1862. doi: 10.3934/era.2023095 |
[4] | Majed Alowaidi, Sunil Kumar Sharma, Abdullah AlEnizi, Shivam Bhardwaj . Integrating artificial intelligence in cyber security for cyber-physical systems. Electronic Research Archive, 2023, 31(4): 1876-1896. doi: 10.3934/era.2023097 |
[5] | Ramalingam Sakthivel, Palanisamy Selvaraj, Oh-Min Kwon, Seong-Gon Choi, Rathinasamy Sakthivel . Robust memory control design for semi-Markovian jump systems with cyber attacks. Electronic Research Archive, 2023, 31(12): 7496-7510. doi: 10.3934/era.2023378 |
[6] | Chengbo Yi, Jiayi Cai, Rui Guo . Synchronization of a class of nonlinear multiple neural networks with delays via a dynamic event-triggered impulsive control strategy. Electronic Research Archive, 2024, 32(7): 4581-4603. doi: 10.3934/era.2024208 |
[7] | Xinling Li, Xueli Qin, Zhiwei Wan, Weipeng Tai . Chaos synchronization of stochastic time-delay Lur'e systems: An asynchronous and adaptive event-triggered control approach. Electronic Research Archive, 2023, 31(9): 5589-5608. doi: 10.3934/era.2023284 |
[8] | Rizhao Cai, Liepiao Zhang, Changsheng Chen, Yongjian Hu, Alex Kot . Learning deep forest for face anti-spoofing: An alternative to the neural network against adversarial attacks. Electronic Research Archive, 2024, 32(10): 5592-5614. doi: 10.3934/era.2024259 |
[9] | Xinchang Guo, Jiahao Fan, Yan Liu . Maximum likelihood-based identification for FIR systems with binary observations and data tampering attacks. Electronic Research Archive, 2024, 32(6): 4181-4198. doi: 10.3934/era.2024188 |
[10] | Xiangwen Yin . A review of dynamics analysis of neural networks and applications in creation psychology. Electronic Research Archive, 2023, 31(5): 2595-2625. doi: 10.3934/era.2023132 |
This paper is devoted to dealing with the dynamic event-triggered H∞ quantized control for neural networks with sensor saturations and stochastic deception attacks. To save the limited network resources, a dynamic event-triggered scheme is offered, which includes the general one. And a lower trigger frequency can be obtained by appropriately adjusting the triggering error. Then, a new closed-loop quantized control model is established under a dynamic event-triggered scheme, sensor saturations, and stochastic deception attacks, which is described by two independent Bernoulli-distributed variables. Moreover, by Lyapunov-Krasovskii functional theory, a new H∞ performance criterion is given, and based on the criterion, the controller design approach is derived. Finally, simulations are listed to verify the validity of derived methods.
For several decades, neural networks (NNs) have aroused the research interest of scholars because of their applications in diverse fields, such as pattern recognition, rainfall forecasting, and optimization problems [1]. The stability is the prerequisite for NNs to work, and a large number of stabilization results for NNs have emerged [2]. For example, the dissipative synchronization issue was addressed for semi-Markovian jumping delayed NNs under random deception attacks by a novel event-triggered impulsive control strategy in [3], and the problem of event-triggered synchronization for master-slave NNs was done in [4].
Networked control systems (NCSs) are the closed-loop control systems, which have been successfully applied in smart grids, industrial automation, and mobile communications [5]. In NCSs, data are transmitted via a communication network among the sensor, controller, and actuator. Now, networked control problems have attracted a growing number of researchers. For example, event-triggered sliding-mode control for networked Markov jumping systems with channel fading was done in [6], and the paper [7] investigated event-triggered output feedback H∞ control for NCSs. Recently, Li et al. [8] researched H∞ synchronization of semi-Markovian switching complex-valued networks with time-varying delay; Zhao and Wu [9] studied fixed/prescribed stability criteria of stochastic systems with time-delay; Hou et al. [10] addressed observer-based prescribed-time synchronization and topology identification for complex networks of piecewise-smooth systems with hybrid impulses; Tan et al. [11] focused on the dual control for autonomous airborne source search with Nesterov accelerated gradient descent.
To realize the efficient utilization of network resources, an event-triggered scheme (ETS) was introduced. Now, researchers have focused extensively on event-triggered controller design. For example, the output feedback L∞ load frequency control of networked power systems was considered in [12] by an adaptive ETS. The leader-following consensus for linear multi-agent systems was studied in [13] by a dynamic ETS. The paper [14] investigated periodic event-triggered dynamic output feedback control for NCSs, and periodic event-triggered control for NCSs subject to input and output delays and external disturbance was studied in [15]. Recently, Wang et al. [16] addressed quantization-dependent dynamic event-triggered control for networked switched systems under Dos attacks; Zhang et al. [17] researched accumulated-state-error-based event-triggered sampling scheme and its application to H∞ control of sampled-data systems; Zhao et al. [18] investigated prescribed-time synchronization for complex dynamic networks of piecewise smooth systems by a hybrid event-triggering control approach; Hou et al. [19] focused on the practical finite-time synchronization for master-slave Lur'e nonlinear systems with performance constraint and time-varying actuator faults via the memory based quantized dynamic event-triggered control.
Nowadays, the security issue of NCSs has received broad interest. Cyber-attacks are mainly classified into deception attacks and DoS attacks [20]. Now, researchers have focused extensively on cyber-attacks. For example, event-triggered H∞ load frequency control for multi-area power systems was addressed in [21] with DoS attacks, and co-design of dynamic ETS and resilient observer-based control under aperiodic DoS attacks was done in [22]. The event-triggered control for networked Markovian jump systems was considered in [23] subject to a deception attack. Recently, the paper [24] investigated asynchronous sliding-mode control for discrete-time networked hidden stochastic jump systems with cyber attacks, and Yao et al. [25] solved prescribed-time output feedback control for cyber-physical systems under malicious attacks.
Sensor saturation brings nonlinear characteristics to the system by nonlinear sampled measurements, which can degrade the systems performance or even make it unstable. Therefore, it makes practical sense to take the sensor saturation into account. Now, some efforts have been devoted to researching the sensor saturation. For example, the H∞ control for time-delay systems was considered in [26] with sensor saturations, and the paper [27] was concerned with the robust non-fragile observer-based dynamic event-triggered sliding mode control for NCSs subject to sensor saturation. The paper [28] has addressed security output feedback control for T-S fuzzy systems with decentralized ETS and multi-sensor saturations. Recently, dual flexible prescribed performance control [29], flexible prescribed performance output feedback control [30], and sliding flexible prescribed performance control [31] are investigated with input saturation.
Based on the above discussion, we found that in the research of deception attacks, the accumulated dynamic cyber-attack is often ignored, and only conventional deception attack, such as those that satisfy the Lipschitz condition are considered. At the same time, with the continuous improvement of ETS, there is still room for further research on how to balance the control performance of the system and the utilization rate of network resources. As for sensor saturation, to our knowledge, few scholars have investigated event-triggered quantized control for neural networks (NNs) with sensor saturations and cyber-attacks. Based on these, the dynamic event-triggered quantized control for NNs with sensor saturations and stochastic deception attacks is studied. The main contributions of this article can be summarized as follows. (1) The dynamic ETS is offered to save limited system resources, which can be reduce to the static one. (2) An improved quantized control model is established for NNs with dynamic ETS, sensor saturations and stochastic deception attacks. (3) A reformative event-triggered H∞ quantized controller design is derived.
Notations: Sym{A}=A+AT; Pb{A} is the probability of event A that happens; ∗ denotes the symmetric term; E{⋅} is the expectation; B>0 shows B is a positive-definite matrix; diag{⋅} means block-diagonal matrix.
A framework of dynamic event-triggered quantized control for NNs with sensor saturations and cyber-attacks is presented in Figure 1.
The plant is described by:
{˙χ(t)=Aχ(t)+Du(t)+Eˆh(t−η(t))+Bμ(t)y(t)=Cχ(t)˜z(t)=Fχ(t) | (2.1) |
where A ∈ Rn×n, D ∈ Rn×p, C ∈ Rm×n, B ∈ Rn×r, E ∈ Rn×n, and F ∈ Rq×n; χ(t)=[χ1(t)χ2(t)⋯χn(t)]T ∈ Rn is the system state; u(t) ∈ Rp is the control input; μ(t) ∈ Rr is the disturbance input; y(t)=[y1(t)y2(t)⋯ym(t)]T ∈ Rm is the measured output; ˜z(t) ∈ Rq is the controlled output; ˆh(χ(t))=[ˆh1(χ1(t))ˆh2(χ2(t))⋯ˆhn(χn(t))]T ∈ Rn denotes the neuron activation function, and satisfies
ℓ−i≤ˆhi(l1)−ˆhi(l2)l1−l2≤ℓ+i,l1≠l2,i=1,⋯,n | (2.2) |
with ˆh(0)=0, ℓ−i and ℓ+i are known constants.
In Figure 1, sensor saturation is taken into account, which can be described by a saturation function sat(ν)=[sat(ν1),sat(ν2),⋯,sat(νm)] ∈ Rm, and it can be decomposed into a linear part and a nonlinear part, that is, sat(ν)=ν−ϕ(ν). Then, there exists a real number δ∈(0,1) such that
δνTν≥ϕT(ν)ϕ(ν) |
Therefore, the real output of the sensor is
ˉy(t)=sat(y(t))=y(t)−ϕ(y(t)) |
holds for the constraint that for δ∈(0,1),
δyT(t)y(t)≥ϕT(y(t))ϕ(y(t)) | (2.3) |
The ETS is given as follows:
eTˉy(lh)Ω1eˉy(lh)≤σˉyTρ(tklh)Ω2ˉyρ(tklh)+1αη(tklh) | (2.4) |
where the triggering error
eˉy(lh)=ˉy(tkh)−ˉyρ(tklh), tklh=tkh+lh |
and ˉyρ(tklh)=ρˉy(tklh)+(1−ρ)ˉy(tkh) with 0≤ρ≤1 and l ∈ N; Ωk>0 (k=1,2) are the weighting matrices; ˉy(tklh) and ˉy(tkh) are the currently sampled signal and the last transmitted signal, respectively; the variable η(t) satisfies
˙η(t)=−βη(t)+σˉyTρ(tklh)Ω2ˉyρ(tklh)−eTˉy(lh)Ω1eˉy(lh), t∈[0,∞) | (2.5) |
where β>0 and η(0)>0.
Remark 1. Compared with the reported ETSs in [32–34], a new triggering error ey(lh)=ˉy(tkh)−ˉyρ(tklh) is introduced, and when ρ=1, it can reduce to the general one e(t)=y(tkh)−y(tklh). By this triggering error, when the sampled data have a rapid change arising from the external disturbance, spurious triggering events may decrease. Second, more parameters are used to adjust the ETS (2.4) with (2.5), such as σ, ρ, α, β, Ω1, and Ω2. Third, the variable η(t) can be adjusted as the system changes instead of a preset constant.
Remark 2. When ρ=1, Ω1=Ω2 and α→∞ or η(t)→0, the ETS (2.4) degrades into the static ones in [28], [35], and [36]. So, the static ETSs in [28], [35], and [36] are the special case of ETS (2.4). When Ω1=Ω2, ρ=1, and y=x, the ETS (2.4) can reduce to state-based dynamic ETS in [37]. So, the dynamic ETS in [37] can also be the special case of ETS (2.4). Moreover, when Ω1=Ω2, the ETS (2.4) reduces to the dynamic ETS in [34].
Remark 3. Refer to the paper [38]; since the event-triggered condition is only tested at the periodic moment, the minimum value of the time interval of the adjacent event-triggered moment is the sampling period h, which can directly exclude Zeno behavior.
The logarithmic quantizer is presented as
q(⋅)=[q1(⋅),q2(⋅),⋯,qm(⋅)]T |
where qi(⋅) is the i-th subquantizer, and qi(⋅) is described by
qi(ˉyi(tkh))={μ(l)i 11+wiμ(l)i<ˉyi(tkh)≤11−wiμ(l)i0ˉyi(tkh)=0−gi(−ˉyi(tkh)) ˉyi(tkh)<0 |
with quantized levels set
{±μ(l)i|μ(l)i=(di)lμ(0)i,l=0,±1,±2,⋯}∪{0} |
where wi=1−di1+di, di∈(0,1), u(0)i>0 denote quantizer parameter, quantizer density, and initial quantization, respectively. From [39], the quantizer is the characteristic of
q(ˉy(tkh))=ˉy(tkh)+h(ˉy(tkh)) | (2.6) |
where
h(ˉy(tkh))=[h1(ˉy1(tkh))h2(ˉy2(tkh))⋯hm(ˉym(tkh))]T |
with
−wi[ˉyi(tkh)]2≤ˉyi(tkh)hi(ˉyi(tkh))≤wi[ˉyi(tkh)]2 (i=1,2,⋯,m) | (2.7) |
Assumed that τk satisfies τ_≤τk≤¯τ (k=1,2,⋯), where τ_ and ¯τ are two constants. For t∈[tkh+τk,tk+1h+τk+1), the controller input
ˆy(t)=δ(tk)q(ˉy(tkh))+κ(tk)Cf(x(t−ν(t)))+λ(tk)C∫tt−θ(t)g(x(q))dq |
where f(⋅) and g(⋅) are the cyber-attacks; Pb{δ(tk)=1}=ˉδ, Pb{δ(tk)=0}=1−ˉδ, Pb{φ(tk)=1}=ˉφ, Pb{φ(tk)=0}=1−ˉφ; κ(tk)=[1−δ(tk)]φ(tk), λ(tk)=[1−δ(tk)][1−φ(tk)] with δ(tk)∈{0,1}, φ(tk)∈{0,1}; ν(t)∈(0,νM], θ(t)∈(0,θM].
Assumption 1 [40]: Deception attacks f(⋅) and g(⋅) are bounded and satisfy
p−j≤fj(ℓ1)−fj(ℓ2)ℓ1−ℓ2≤p+j, q−j≤gj(ℓ1)−gj(ℓ2)ℓ1−ℓ2≤q+j (ℓ1≠ℓ2) | (2.8) |
where p−j, p+j, q−j, q+j (j=1,⋯,n) are constants, and f(0)=g(0)=0.
Similar to [41], the interval [tkh+τk,tk+1h+τk+1) can be divided as
[tkh+τk,tk+1h+τk+1)=∪dkd=0χdtk, dk=tk+1−tk−1 |
where χdtk=[tkh+dh+τdk,tkh+dh+h+τd+1k) with τ0k=τk and τdk+1k=τk+1. For t∈χdtk, we define τ(t)=t−tkh−dh and e(t)=ρˉy(tkh)−ρˉy(tkh+dh). It is clear that
0≤τm≤τ(t)≤τM, ˉy(tkh)=ρ−1e(t)+ˉy(t−τ(t)) |
where τm:=τ_ and τM:=¯τ+h. So, the dynamic ETS (2.4) can be rewritten as
eT(t)Ω1e(t)≤σ[ϱe(t)+ˉy(t−τ(t))]TΩ2[ϱe(t)+ˉy(t−τ(t))]+1αη(t−τ(t)) | (2.9) |
where ϱ=ρ−1−1, and
˙η(t)=−βη(t)−eT(t)Ω1e(t)+σ[ϱe(t)+ˉy(t−τ(t))]TΩ2[ϱe(t)+ˉy(t−τ(t))] | (2.10) |
The controller is formulated as
u(t)=Kˆy(t) | (2.11) |
where K is the controller gain.
Substituting (2.11) into (2.1), we can obtain the following system subject to (2.3), (2.7)–(2.9):
{˙χ(t)=Aχ(t)+Eˆh(t−η(t))+ˉκDKCf(χ(t−ν(t)))+ˉλDKC∫tt−θ(t)g(x(r))dr+Bμ(t)+ˉδDK[Cχ(t−τ(t))+ρ−1e(t)−ϕ(Cχ(t−τ(t)))+h(ˉy(tkh)]+[δ(tk)−ˉδ]DK[Cχ(t−τ(t))+ρ−1e(t)−ϕ(Cχ(t−τ(t)))+h(ˉy(tkh)]+[κ(tk)−ˉκ]DKCf(χ(t−ν(t)))+[λ(tk)−ˉλ]DKC∫tt−θ(t)g(χ(r))dr˜z(t)=Fχ(t) | (2.12) |
where t∈[tkh+τk,tk+1h+τk+1), ˉκ=(1−ˉδ)ˉφ and ˉλ=(1−ˉδ)(1−ˉφ).
Remark 4. The problem of dynamic event-triggered H∞ networked control for NNs subject to stochastic deception attacks is studied. Compared to the reported paper [42], a new event-triggered quantized control model for NNs is presented under the dynamic ETS, sensor saturations, and stochastic deception attacks.
Lemma 1 ([42]). For a scalar 0≤μ(t)≤μM, any matrices W∈S+n, U∈Rn×n satisfying [W∗UW]≥0 and integral function {˙x(r)|r∈[−μM,0]}, we have
−μM∫tt−μM˙xT(r)W˙x(r)dr≤ψT(t)Λψ(t) |
where
ψ(t)=[x(t)x(t−μ(t))x(t−μM)], Λ=[−W∗∗W−U−2W+U+UT∗UW−U−W] |
Lemma 2 ([43]). D=MΣNT ∈Rn×m is a matrix with full column rank, where Σ∈Rm×n is a rectangular diagonal matrix, and M and N are orthogonal matrices. For P∈Sn, X∈Rm×m, the sufficient and necessary condition of PD=DX is P=Mdiag{P1,P2}MT with P1∈Rm×m and P2∈R(n−m)×(n−m).
We will design a controller such that 1) the system (2.12) is mean-square asymptotically stable; 2) For μ(t)≠0 ∈ L2[0,∞), ˜z(t) satisfies
‖˜z(t)‖E2<γ‖μ(t)‖2 |
under zero initial condition.
Theorem 1. For parameters γ, ˉδ, ˉφ, α, β, ρ, σ, ηM, τM, vM, θM, and matrix K, if matrices P, U, Qℓ, Rℓ (ℓ=1,⋯,4) ∈ S+n, diagonal matrices Faj≥0, Gaj≥0, Fbj≥0, Gbj≥0, Fdj≥0, Gdj≥0 (j=1,2,3), Ω1≥0, Ω2≥0, Nk (k=1,⋯,4) and X such that:
[Ξ∗∗∗BTPe1−γ2I∗∗ˆΥ1∇Λ∗ˆΥ200Λ]<0 | (3.1) |
[Qi∗NiQi]≥0(i=1,⋯,4),[UX∗U]≥0 | (3.2) |
where
Ξ=Ξ1+Ξ2+Ξ3+eT1FTFe1Ξ1=Sym{eT1(PAe1+ˉηPDK(Ce4+ρ−1e21−e22+e23)+ˉφPDKCe14+ˉγPDKCe19+PEe11)}Ξ2=eT1(R1+R2+R3+R4)e1−eT3R1e3−eT5R2e5−eT7R3e7−eT9R4e9+[e1e2e3]TΨ1[e1e2e3]+[e1e4e5]TΨ2[e1e4e5]+[e1e6e7]TΨ3[e1e6e7]+[e1e8e9]TΨ4[e1e8e9]+v2MeT16Ue16−[e19e20]T[UX∗U][e19e20]+(1+αβ)[(Ce4+ϱe21−e22)TσΩ2(Ce4+ϱe21−e22)]−(1+αβ)eT21Ω1e21+δeT4CTCe4−eT22e22−eT23ˆDe23+(ρ−1e21+Ce4−e22)TWTˆDW(ρ−1e21+Ce4−e22)Ξ3=3∑i=1Sym{(Wpei−ei+9)TFai(ei+9−Wmei)}+3∑i=1{eTiWGaiWei−eTi+9Gaiei+9}+Sym{(Kpe1−e13)TFb1(e13−Kme1)}+3∑i=2Sym{(Kpei+4−ei+12)TFbi(ei+12−Kmei+4)}+eT1ˆKGb1ˆKe1−eT13Gb1e13+3∑i=2{eTi+4ˆKGbiˆKei+4−eTi+12Gbiei+12}+Sym{(Lpe1−e16)TFd1(e16−Lme1)}+3∑i=2Sym{(Lpei+6−ei+15)TFdi(ei+15−Lmei+6)}+eT1LGd1Le1−eT16Gd1e16+3∑i=2{eTi+6LGdiLei+6−eTi+15Gdiei+15} |
Ψi=[−Qi∗∗Qi−Ni−2Qi+Ni+NTi∗NiQi−Ni−Qi] (i=1,⋯,4)Υi=[ηMΓTiτMΓTidMΓTivMΓTi]T(i=1,2)Γ1=PAe1+ˉηPDK(Ce4+ρ−1e21−e22+e23)+ˉφPDKCe14+ˉγPDKCe19+PEe11Γ2=λ1PDK(Ce4+ρ−1e21−e22+e23)+λ2PDKCe14+λ3PDKCe19∇=[ηM(PD)TτM(PD)TvM(PD)TθM(PD)T]TΛ=diag{−PQ−11P,−PQ−12P,−PQ−13P,−PQ−14P}ei=[0n×(i−1)nIn×n0n×(23−i)n] (i=1,⋯,23)μ1=√ˉδ(1−ˉδ), μ2=√ˉκ(1−ˉκ), μ3=√ˉλ(1−ˉλ) |
Then the system (2.12) is asymptotically stable.
Proof. The LKF candidate is chosen as
V(t)=V1(t)+V2(t)+V3(t)+V4(t) |
where
V1(t)=χT(t)Pχ(t)V2(t)=∫tt−ηMχT(s)R1χ(s)ds+∫tt−τMχT(s)R2χ(s)ds+∫tt−dMχT(s)R3χ(s)ds+∫tt−vMχT(s)R4χ(s)dsV3(t)=ηM∫tt−ηM∫ts˙χT(u)Q1˙χ(u)duds+τM∫tt−τM∫ts˙χT(u)Q2˙χ(u)duds+vM∫tt−vM∫ts˙χT(u)Q3˙χ(u)duds+θM∫tt−θM∫ts˙χT(u)Q4˙χ(u)dudsV4(t)=θM∫tt−θM∫tshT(χ(v))Uh(χ(v))dvds |
For convenience, we define:
ξ1(t)=col{χ(t) χ(t−η(t)) χ(t−ηM) χ(t−τ(t))}ξ2(t)=col{χ(t−τM) χ(t−v(t)) χ(t−vM) χ(t−θ(t))}ξ3(t)=col{χ(t−θM) ˆh(χ(t)) ˆh(χ(t−η(t))) ˆh(χ(t−ηM))}ξ4(t)=col{g(χ(t)) g(χ(t−v(t))) g(χ(t−vM))}ξ5(t)=col{h(χ(t)) h(χ(t−θ(t))) h(χ(t−θM))}ξ6(t)=col{∫tt−θ(t)h(χ(u))du ∫t−θ(t)t−θMh(χ(u))du}ξ7(t)=col{e(t) ϕ(ˉy(t−τ(t))) h(ˉy(tkh))}ξ(t)=col{ξ1(t) ξ2(t) ξ3(t) ξ4(t) ξ5(t) ξ6(t) ξ7(t)} |
From P>0, U>0, Qℓ>0, Rℓ>0 (ℓ=1,⋯,4), we have V(t)>0. Computing dV(t)dt, we have
˙V1(t)=2χT(t)P˙χ(t) | (3.3) |
˙V2(t)=χT(t)(R1+R2+R3+R4)χ(t)−χT(t−ηM)R1χ(t−ηM)−χT(t−τM)R2χ(t−τM)−χT(t−vM)R3χ(t−vM)−χT(t−θM)R4χ(t−θM) | (3.4) |
˙V3(t)=˙χT(t)(η2MQ1+τ2MQ2+d2MQ3+v2MQ4)˙χ(t)−ηM∫tt−ηM˙χT(s)Q1˙χ(s)ds−τM∫tt−τM˙χT(s)Q2˙χ(s)ds−vM∫tt−vM˙χT(s)Q3˙χ(s)ds−θM∫tt−θM˙χT(s)Q4˙χ(s)ds˙V4(t)=θ2MhT(χ(t))Uh(χ(t))−θM∫tt−θMhT(χ(v))Uh(χ(v))dv |
From Lemma 1, there exist Ni (i=1,⋯,4) satisfying (3.2) such that
−ηM∫tt−ηM˙χT(s)Q1˙χ(s)ds≤[χ(t)χ(t−η(t))χ(t−ηM)]TΨ1[χ(t)χ(t−η(t))χ(t−ηM)]−τM∫tt−τM˙χT(s)Q2˙χ(s)ds≤[χ(t)χ(t−τ(t))χ(t−τM)]TΨ2[χ(t)χ(t−τ(t))χ(t−τM)]−vM∫tt−vM˙χT(s)Q3˙χ(s)ds≤[χ(t)χ(t−v(t))χ(t−vM)]TΨ3[χ(t)χ(t−v(t))χ(t−vM)]−θM∫tt−θM˙χT(s)Q4˙χ(s)ds≤[χ(t)χ(t−θ(t))χ(t−θM)]TΨ4[χ(t)χ(t−θ(t))χ(t−θM)] |
By the reciprocally convex inequality, we have
−θM∫tt−θMhT(χ(v))Uh(χ(v))dv≤−θMθ(t)∫tt−θ(t)hT(χ(v))dvU∫tt−θ(t)h(χ(v))dv−θMθM−θ(t)∫t−θ(t)t−θMhT(χ(v))dvU∫t−θ(t)t−θMh(χ(v))dv≤−[∫tt−θ(t)h(χ(v))dv∫t−v(t)t−θMh(χ(v))dv]T[UX∗U][∫tt−θ(t)h(χ(v))dv∫t−θ(t)t−θMh(χ(v))dv] |
Based on the inequality (2.3), we have
0≤δˉyT(t−τ(t))ˉy(t−τ(t))−ϕT(ˉy(t−τ(t)))ϕ(ˉy(t−τ(t)))=δχT(t−τ(t))CTCχ(t−τ(t))−ϕT(ˉy(t−τ(t)))ϕ(ˉy(t−τ(t))) | (3.5) |
From (2.7), we obtain
0≤−[h(ˉy(tkh))+Wˉy(tkh)]TˆD[h(ˉy(tkh))−Wˉy(tkh)]=[ρ−1e(t)+Cχ(t−τ(t))−ϕ(ˉy(t−τ(t)))]TWTˆDW ×[ρ−1e(t)+Cχ(t−τ(t))−ϕ(ˉy(t−τ(t)))]−hT(y(tkh))ˆDh(y(tkh)) | (3.6) |
From (2.9), we have
0=−βη(t−τ(t))+[ϱe(t)+ˉy(t−τ(t))]TσΩ2[ϱe(t)+ˉy(t−τ(t))]−eT(t)Ω1e(t)≤(1+αβ)[(ϱe(t)+Cχ(t−τ(t))−ϕ(ˉy(t−τ(t))))TσΩ2×(ϱe(t)+Cχ(t−τ(t))−ϕ(ˉy(t−τ(t))))−eT(t)Ω1e(t)] | (3.7) |
Note that
ˆf1i(ℓ):=2(Wpχ(ℓ)−ˆh(χ(ℓ)))TFai(ˆh(χ(ℓ))−Wmχ(ℓ))≥0ˆg1i(ℓ):=χT(ℓ)WGaiWχ(ℓ)−ˆhT(χ(ℓ))Gaiˆh(χ(ℓ))≥0ˆf2i(ℓ):=2(Kpχ(ℓ)−g(χ(ℓ)))TFbi(g(χ(ℓ))−Kmχ(ℓ))≥0ˆg2i(ℓ):=χT(ℓ)ˆKGbiˆKχ(ℓ)−gT(χ(ℓ))Gbig(χ(ℓ))≥0ˆf3i(ℓ):=2(Lpχ(ℓ)−h(χ(ℓ)))TFdi(h(χ(ℓ))−Lmχ(ℓ))≥0ˆg3i(ℓ):=χT(ℓ)LGdiLχ(ℓ)−hT(χ(ℓ))Gdih(χ(ℓ))≥0 |
where Fai, Fbi, Fdi, Gai, Gbi, Gdi are n-dimensional diagonal matrices, and
Wm=diag{ℓ−1,⋯,ℓ−n}, Wp=diag{ℓ+1,⋯,ℓ+n}W=diag{ℓ1,⋯,ℓn}, ℓk=max{|ℓ+k|,|ℓ−k|}(k=1,⋯,n)Km=diag{p−1,⋯,p−n}, Kp=diag{p+1,⋯,p+n}ˆK=diag{p1,⋯,pn}, pj=max{|p+j|,|p−j|}(j=1,⋯,n)Lm=diag{q−1,⋯,q−n}, Lp=diag{q+1,⋯,q+n}L=diag{q1,⋯,qn}, qm=max{|q+m|,|q−m|}(m=1,⋯,n) |
Thus, we have
ˆf11(t)+ˆf12(t−η(t))+ˆf13(t−ηM)+ˆg11(t)+ˆg12(t−η(t))+ˆg13(t−ηM)+ˆf21(t)+ˆf22(t−d(t))+ˆf23(t−dM)+ˆg21(t)+ˆg22(t−v(t))+ˆg23(t−vM)+ˆf31(t)+ˆf32(t−θ(t))+ˆf33(t−θM)+ˆg31(t)+ˆg32(t−θ(t))+ˆg33(t−θM)=ξT(t)Ξ3ξ(t)≥0 | (3.8) |
Now, using (3.3)–(3.8) yields
˙V(t)≤2χT(t)P˙χ(t)+˙χT(t)˜Q˙χ(t)+ξT(t)(Ξ2+Ξ3)ξ(t) |
where ˜Q=η2MQ1+τ2MQ2+d2MQ3+φ2MQ4.
When μ(t)≠0, we have
E{2χT(t)P˙χ(t)}=2ξT(t)eT1P(Π0ξ(t)+Eω(t))=ξT(t)Ξ1ξ(t)+2ξT(t)eT1PEω(t) | (3.9) |
and
E{˙χT(t)˜Q˙χ(t)}=E{(Π0ξ(t)+Eω(t))T˜Q(Π0ξ(t)+Eω(t))+ξT(t)ΠT1˜QΠ1ξ(t)} | (3.10) |
where
Π0=Ae1+ˉηDK(Ce4+ρ−1e21−e22+e23)+ˉφDKCe14+ˉγDKCe19+Ee11Π1=λ1DK(Ce4+ρ−1e21−e22+e23)+λ2DKCe14+λ3BKCe19 |
Furthermore, from (2.1), we have
˜zT(t)˜z(t)−γ2μT(t)μ(t)=ξT(t)eT1FTFe1ξ(t)−γ2μT(t)μ(t) | (3.11) |
We can obtain
E{˙V(t)+˜zT(t)˜z(t)−γ2μT(t)μ(t)}≤E{ˆξT(t)[Ξ∗BTPe1−γ2I]ˆξ(t)−ˆξT(t)([Υ1ϝ]TΛ−1[Υ1ϝ]+[Υ20]TΛ−1[Υ20])ˆξ(t)} | (3.12) |
where ˆξ(t)=col{ξ(t) μ(t)}. Since the system (2.12) is asymptotically stable, under zero initial conditions, we have
E{∫∞0˙V(s)ds}=E{V(∞)−V(0)}=0 |
Then by (3.12) and (3.1), we can obtain
E{∫∞0[˜zT(s)˜z(s)−γ2μT(s)μ(s)]ds}=E{∫∞0[˙V(s)+˜zT(s)˜z(s)−γ2μT(s)μ(s)]ds}<0 |
which means ‖˜z(t)‖E2<γ‖μ(t)‖2.
Remark 5. Compared with recently reported works [32], [37], and [42], more system information was used. First, the information of sensor saturation and quantization error h(y(tkh)) was used, as seen from (3.5) and (3.6). Second, the cyber-attack functions f(x(t)), g(x(t)), and their ramifications
f(x(t−d(t))),f(x(t−dM)),g(x(t−v(t))),g(x(t−vM))∫tt−v(t)g(x(s))ds,∫t−v(t)t−vMg(x(s))ds |
are all employed. Finally, based on the ETS (2.4), the dynamic variable η(t) is utilized, as seen from (3.7).
Remark 6. Theorem 1 is derived based on a Jensen integral inequality. If we employ the Bessel-Legendre inequality [44], it is expected to derive some less conservative results.
Based on Theorem 1, we will give an event-triggered quantized controller design for NNs with sensor saturation and stochastic deception attacks.
Theorem 2. For parameters γ, ˉδ, ˉφ, α, β, σ, ρ, ηM, τM, θM, vM, ϵi (i=1,⋯,4), if matrices P, U, Qℓ, Rℓ (ℓ=1,⋯,4) ∈ S+n, diagonal matrices Faj≥0, Gaj≥0, Fbj≥0, Gbj≥0, Fdj≥0, Gdj≥0 (j=1,2,3), Ω1≥0, Ω2≥0, Nk (k=1,⋯,4), X and Y such that (3.2) and the following LMI hold:
[˜Π∗∗∗BTPe1−γ2I∗∗˜Υ1∇˜Λ∗˜Υ200˜Λ]<0 | (3.13) |
where
˜Π=˜Ξ1+Ξ2+Ξ3+eT1FTFe1˜Ξ1=Sym{eT1(PAe1+ˉηDY(Ce4+ρ−1e21−e22+e23)+ˉφDYCe14+ˉγDYCe19+PEe11)}˜Υi=[ηM˜ΓTiτM˜ΓTivM˜ΓTiθM˜ΓTi]T(i=1,2)˜Γ1=PAe1+ˉηDY(Ce4+ρ−1e21−e22+e23)+ˉφDYCe14+ˉγDYCe19+PEe11˜Γ2=λ1DY(Ce4+ρ−1e21−e22+e23)+λ2DYCe14+λ3DYCe19˜Λ=diag{−2ϵ1P+ϵ21Q1,−2ϵ2P+ϵ22Q2,−2ϵ3P+ϵ23Q3,−2ϵ4P+ϵ24Q4} |
Then the system (2.12) with K=(DTPD)−1DTDY is asymptotically stable.
Proof. Because of D is a matrix with full column rank, we have D=M[D00]NT, where D0∈Rm×m is a diagonal matrix. Let P=Mdiag{P1,P2}MT; by Lemma 2, we can find a matrix S∈Rm×m such that PDK=DSK. Defining Y=SK, we have PDK=DY and K=(DTPD)−1DTDY, which implies
[˜Π∗∗∗BTPe1−γ2I∗∗˜Υ1∇Λ∗˜Υ200Λ]<0 | (3.14) |
There exist ϵℓ>0 (ℓ=1,⋯,4) satisfy
−PQ−1iP≤−2ϵiP+ϵ2iQi |
Replace −PQ−1iP with −2ϵiP+ϵ2iQi in (3.14), and we can obtain (3.13).
Remark 7. By introducing dynamic ETS, quantization, sensor saturations, and stochastic deception attacks, we derived a new stability criterion. Based on the criterion, a reformative event-triggered quantized controller design was derived.
Here, we list a three-order system example to verify the validity of derived results.
Example 1. Considering an NN (2.1) with
A=[−2.10.101−2000−1],D=[0.10.20.1],E=[0.10.20−0.10.20.1−0.20−0.3]B=[0.10.10.1],C=F=[0.10000.10000.1],ˆh(x)=[tanh(0.03x1)tanh(0.06x2)tanh(0.03x3)] |
and μ(t)=e−0.08t, the cyber-attacks are
f(x)=[tanh(0.04x1)tanh(0.04x2)tanh(0.04x3)],g(x)=[tanh(0.03x1)tanh(0.06x2)tanh(0.03x3)] |
Thus, Wp = diag{0.03,0.06,0.03}, Kp = diag{0.04,0.04,0.04}, Lp = diag{0.03,0.06,0.03}, Km = diag{0,0,0}, Wm = diag{0,0,0}, Lm = diag{0,0,0}.
For γ=1, h=0.1, ˉδ=0.5, ˉφ=0.5, ηM=0.5, τM=0.5, θM=0.5, vM=0.5, ϵℓ=1(ℓ=1,⋯,4), α=5, β=0.1, σ=1 and ρ=0.3, by Theorem 2, we have
P=[3.7741−0.1598−0.0006−0.15982.9349−0.0041−0.0006−0.00413.0832], Y=[−0.15120.1667−0.0239]TΩ1=[5.3130−0.00240.0008−0.00245.8848−0.00080.0008−0.00085.3116], Ω2=[0.2312−0.00020.0001−0.00020.2213−0.00010.0001−0.00010.2312] |
Then we can obtain
K=[−0.05060.0558−0.0080] |
For x(0)=[−0.5−0.30.4]T, Figure 2 displays the state trajectory of system (2.12). It is not difficult to see that the state trajectory of system (2.12) is convergent to zero, which shows the system (2.12) is stochastically asymptotically stable.
Choose η(0)=0.0001. Figure 3 shows event-based signal transmission instants and release intervals. It is not difficult to see that only 38 times are triggered during the period [0, 50s], and we can work out the average trigger rate is 7.6%. Thus, our ETS can save more system resources. Moreover, by adjusting the parameters η(0) and ρ, we can obtain the desired result.
Set γ=1, ˉδ=0.5, ˉφ=0.5, τM=0.3, νM=0.4, θM=0.4, α=5, β=0.1, δ=0.5, ρ=0.5, σ=0.01, and η0=0.0001. For different values of h, the number of triggered data during [0, 50s] are listed in Table 1.
It is clear that the number of triggered data for various h are far less than those in [36,37], which means our ETS is more advanced.
Dynamic event-triggered quantized control for neural networks with sensor saturations and stochastic deception attacks has been studied. With the dynamic ETS and quantization being introduced, an improved networked control model was formulated with stochastic deception attacks and sensor saturations. By the LKF approach, the event-triggered quantized controller design under sensor saturations and deception attacks was solved. Finally, a numerical example was listed to display the availability of derived methods. In the future, we will study distributed event-triggered H∞ filtering for NCSs.
The authors declare they have not used Artificial Intelligence (AI) tools in the creation of this article.
The paper was supported by the National Natural Science Foundation of China (62073188) and Natural Science Foundation of Rizhao City, China (RZ2022ZR65).
The authors declare there are no conflicts of interest.
[1] |
Z. Feng, H. Shao, L. Shao, Further improved stability results for generalized neural networks with time-varying delays, Neurocomputing, 367 (2019), 308–318. https://doi.org/10.1016/j.neucom.2019.07.019 doi: 10.1016/j.neucom.2019.07.019
![]() |
[2] |
Y. Sheng, H. Zhang, Z. Zeng, Stabilization of fuzzy memristive neural networks with mixed time delays, IEEE Trans. Fuzzy Syst., 26 (2018), 2591–2606. https://doi.org/10.1109/TFUZZ.2017.2783899 doi: 10.1109/TFUZZ.2017.2783899
![]() |
[3] |
H. Wei, K. Zhang, M. Zhang, Q, Li, J. Wang, Dissipative synchronization of Semi-Markovian jumping delayed neural networks under random deception attacks: An event-triggered impulsive control strategy, J. Franklin Inst., 361 (2024), 106835. https://doi.org/10.1016/j.jfranklin.2024.106835 doi: 10.1016/j.jfranklin.2024.106835
![]() |
[4] |
A. Kazemy, J. Lam, X. Zhang, Event-triggered output feedback synchronization of master-slave neural networks under deception attacks, IEEE Trans. Neural Networks Learn. Syst., 33 (2022), 952–961. https://doi.org/10.1109/TNNLS.2020.3030638 doi: 10.1109/TNNLS.2020.3030638
![]() |
[5] |
X. Liang, J. Xu, Control for networked control systems with remote and local controllers over unreliable communication channel, Automatica, 98 (2018), 86–94. https://doi.org/10.1016/j.automatica.2018.09.015 doi: 10.1016/j.automatica.2018.09.015
![]() |
[6] |
W. Qi, N. Zhang, G. Zong, S. Su, H. Yan, R. Yeh, Event-triggered SMC for networked markov jumping systems with channel fading and applications: Genetic algorithm, IEEE Trans. Cybern., 53 (2023), 6503–6515. https://doi.org/10.1109/TCYB.2023.3253701 doi: 10.1109/TCYB.2023.3253701
![]() |
[7] |
Y. Shen, F. Li, D. Zhang, Y. Wang, Y. Liu, Event-triggered output feedback H∞ control for networked control systems, Int. J. Robust Nonlinear Control, 29 (2019), 166–179. https://doi.org/10.1002/rnc.4380 doi: 10.1002/rnc.4380
![]() |
[8] |
Q. Li, H. Wei, W. Gong, J. Wang, H∞ synchronization of semi-Markovian switching complex-valued networks with time-varying delay: A delay-dependent event-triggered mechanism, Int. J. Robust Nonlinear Control, 35 (2025), 1539–1556. https://doi.org/10.1002/rnc.7739 doi: 10.1002/rnc.7739
![]() |
[9] |
Y. Zhao, H. Wu, Fixed/prescribed stability criterions of stochastic system with time-delay, AIMS Math., 9 (2024), 14425–14453. https://doi.org/10.3934/math.2024701 doi: 10.3934/math.2024701
![]() |
[10] |
X. Hou, H. Wu, J. Cao, Observer-based prescribed-time synchronization and topology identification for complex networks of piecewise-smooth systems with hybrid impulses, Comput. Appl. Math., 43 (2024), 180. https://doi.org/10.1007/s40314-024-02701-x doi: 10.1007/s40314-024-02701-x
![]() |
[11] |
G. Tan, W. Chen, J. Yang, X. Tran, Z. Li, Dual control for autonomous airborne source search with Nesterov accelerated gradient descent: Algorithm and performance analysis, Neurocomputing, 630 (2025), 129729. https://doi.org/10.1016/j.neucom.2025.129729 doi: 10.1016/j.neucom.2025.129729
![]() |
[12] |
Z. Wu, H. Mo, J. Xiong, M. Xie, Adaptive event-triggered observer-based output feedback L∞ load frequency control for networked power systems, IEEE Trans. Ind. Inf., 16 (2020), 3952–3962. 10.1109/TII.2019.2942637 doi: 10.1109/TII.2019.2942637
![]() |
[13] |
D. Liu, G. Yang, A dynamic event-triggered control approach to leader-following consensus for linear multiagent systems, IEEE Trans. Syst. Man Cybern.: Syst., 51 (2021), 6271–6279. https://doi.org/10.1109/TSMC.2019.2960062 doi: 10.1109/TSMC.2019.2960062
![]() |
[14] |
C. Li, X. Zhao, C. Wu, L. Liu, N. Zhao, Periodic event-triggered dynamic output feedback control for networked control systems subject to packet dropouts, ISA Trans., 140 (2023), 97–108. https://doi.org/10.1016/j.isatra.2023.06.001 doi: 10.1016/j.isatra.2023.06.001
![]() |
[15] |
J. Sun, Z. Zeng, Periodic event-triggered control for networked control systems with external disturbance and input and output delays, IEEE Trans. Cybern., 53 (2023), 6386–6394. 10.1109/TCYB.2022.3164214 doi: 10.1109/TCYB.2022.3164214
![]() |
[16] |
W. Wang, Y. Wang, X. Liu, Z. Liu, Quantization-dependent dynamic event-triggered control for networked switched systems under denial-of-service attacks, IEEE Trans. Syst. Man Cybern.: Syst., 54 (2024), 3822–3833. https://doi.org/10.1109/TSMC.2024.3373006 doi: 10.1109/TSMC.2024.3373006
![]() |
[17] |
X. Zhang, Q. Han, B. Zhang, X. Ge, D. Zhang, Accumulated-state-error-based event-triggered sampling scheme and its application to H∞ control of sampled-data systems, Sci. China Inf. Sci., 67 (2024), 162206. https://doi.org/10.1007/s11432-023-4038-3 doi: 10.1007/s11432-023-4038-3
![]() |
[18] |
X. Zhao, H. Wu, J. Cao, L. Wang, Prescribed-time synchronization for complex dynamic networks of piecewise smooth systems: A hybrid event-triggering control approach, Qual. Theor. Dyn. Syst., 24 (2025), 11. https://doi.org/10.1007/s12346-024-01166-x doi: 10.1007/s12346-024-01166-x
![]() |
[19] |
X. Hou, H. Wu, J. Cao, Practical finite-time synchronization for Lur'e systems with performance constraint and actuator faults: A memory-based quantized dynamic event-triggered control strategy, Appl. Math. Comput., 487 (2025), 129108. https://doi.org/10.1016/j.amc.2024.129108 doi: 10.1016/j.amc.2024.129108
![]() |
[20] |
J. Cao, D. Ding, J. Liu, E. Tian, S. Hu, X. Xie, Hybrid-triggered-based security controller design for networked control system under multiple cyber attacks, Inf. Sci, 548 (2021), 69–84. https://doi.org/10.1016/j.ins.2020.09.046 doi: 10.1016/j.ins.2020.09.046
![]() |
[21] |
C. Peng, J. Li, M. Fei, Resilient event-triggering H∞ load frequency control for multi-area power systems with energy-limited dos attacks, IEEE Trans. Power Syst., 32 (2017), 4110–4118. https://doi.org/10.1109/TPWRS.2016.2634122 doi: 10.1109/TPWRS.2016.2634122
![]() |
[22] |
S. Hu, D. Yue, Z. Cheng, E. Tian, X. Xie, X. Chen, Co-design of dynamic event-triggered communication scheme and resilient observer-based control under aperiodic dos attacks, IEEE Trans. Cybern., 51 (2021), 4591–4601. https://doi.org/10.1109/TCYB.2020.3001187 doi: 10.1109/TCYB.2020.3001187
![]() |
[23] |
J. Lian, Y. Han, Switching-like event-triggered control for networked Markovian jump systems under deception attack, IEEE Trans. Circuits Syst. II: Express Briefs, 68 (2021), 3271–3275. https://doi.org/10.1109/TCSII.2021.3065679 doi: 10.1109/TCSII.2021.3065679
![]() |
[24] |
W. Qi, N. Zhang, G. Zong, S. Su, J. Cao, J. Cheng, Asynchronous sliding-mode control for discrete-time networked hidden stochastic jump systems with cyber attacks, IEEE Trans. Cybern., 54 (2024), 1934–1946. https://doi.org/10.1109/TCYB.2023.3300120 doi: 10.1109/TCYB.2023.3300120
![]() |
[25] |
Y. Yao, Y. Kang, Y. Zhao, P. Li, J. Tan, Prescribed-time output feedback control for cyber–physical systems under output constraints and malicious attacks, IEEE Trans. Cybern., 54 (2024), 6518–6530. https://doi.org/10.1109/TCYB.2024.3418384 doi: 10.1109/TCYB.2024.3418384
![]() |
[26] |
H. Ji, H. Zhang, C. Li, S. Tian, J. Lu, Y. Wei, H∞ control for time-delay systems with randomly occurring nonlinearities subject to sensor saturations, missing measurements and channel fadings, ISA Trans., 75 (2018), 38–51. https://doi.org/10.1016/j.isatra.2018.02.015 doi: 10.1016/j.isatra.2018.02.015
![]() |
[27] |
X. Chu, M. Li, H∞ non-fragile observer-based dynamic event-triggered sliding mode control for nonlinear networked systems with sensor saturation and dead-zone input, ISA Trans., 94 (2019), 93–107. https://doi.org/10.1016/j.isatra.2019.04.018 doi: 10.1016/j.isatra.2019.04.018
![]() |
[28] |
L. Zha, J. Liu, J. Cao, Security control for T-S fuzzy systems with multi-sensor saturations and distributed event-triggered mechanism, J. Franklin Inst., 357 (2020), 2851–2867. https://doi.org/10.1016/j.jfranklin.2020.02.013 doi: 10.1016/j.jfranklin.2020.02.013
![]() |
[29] | Y. Yao, X. Liang, Y. Kang, Y. Zhao, J. Tan, L. Gu, Dual flexible prescribed performance control of input saturated high-order nonlinear systems, IEEE Trans. Cybern., 2025. https://doi.org/10.1109/TCYB.2024.3524242 |
[30] |
Y. Yao, Y. Kang, Y. Zhao, P. Li, J. Tan, Flexible prescribed performance output feedback control for nonlinear systems with input saturation, IEEE Trans. Fuzzy Syst., 32 (2024), 6012–6022. https://doi.org/10.1109/TFUZZ.2024.3418772 doi: 10.1109/TFUZZ.2024.3418772
![]() |
[31] | Y. Yao, Y. Kang, Y. Zhao, J. Tan, L. Gu, G. Shi, Sliding flexible prescribed performance control for input saturated nonlinear systems, IEEE Trans. Fuzzy Syst., (2024), 1–12. https://doi.org/10.1109/TFUZZ.2024.3516132 |
[32] |
L. Cheng, H. Yan, Y. Tian, X. Zhan, C. Chen, Dynamic event-triggered H∞ control for networked control systems with denial-of-service attacks, IEEE Trans. Circuits Syst. II: Express Briefs, 71 (2024), 642–646. https://doi.org/10.1109/TCSII.2022.3194998 doi: 10.1109/TCSII.2022.3194998
![]() |
[33] |
Y. Yao, J. Tan, J. Wu, X. Zhang, Decentralized adaptive neural safe tracking control for nonlinear systems with conflicted output constraints, ISA Trans., 137 (2023), 263–274. https://doi.org/10.1016/j.isatra.2023.01.002 doi: 10.1016/j.isatra.2023.01.002
![]() |
[34] |
Z. Feng, H. Shao, Quantized H∞ filtering for networked systems with stochastic cyber attacks: a dynamic event-triggered scheme, Trans. Inst. Meas. Control, 46 (2024), 611–623. https://doi.org/10.1177/01423312231181964 doi: 10.1177/01423312231181964
![]() |
[35] |
J. Liu, Y. Gu, J. Cao, S. Fei, Distributed event-triggered H∞ filtering over sensor networks with sensor saturations and cyber-attacks, ISA Trans., 81 (2018), 63–75. https://doi.org/10.1016/j.isatra.2018.07.018 doi: 10.1016/j.isatra.2018.07.018
![]() |
[36] |
J. Liu, E. Tian, X. Xie, H. Lin, Distributed event-triggered control for networked control systems with stochastic cyber-attacks, J. Franklin Inst., 356 (2019), 10260–10276. https://doi.org/10.1016/j.jfranklin.2018.01.048 doi: 10.1016/j.jfranklin.2018.01.048
![]() |
[37] |
Q. Zhang, H. Yan, H. Zhang, S. Chen, M. Wang, H∞ control of singular system based on stochastic cyber-attacks and dynamic event-triggered mechanism, IEEE Trans. Syst. Man Cybern.: Syst., 51 (2021), 7510–7516. https://doi.org/10.1109/TSMC.2020.2972395 doi: 10.1109/TSMC.2020.2972395
![]() |
[38] |
Y. Wang, Y. Lei, T. Bian, Z. Guan, Distributed control of nonlinear multiagent systems with unknown and nonidentical control directions via event-triggered communication, IEEE Trans. Cybern., 50 (2020), 1820–1832. https://doi.org/10.1109/TCYB.2019.2908874 doi: 10.1109/TCYB.2019.2908874
![]() |
[39] |
H. Shao, Q. Han, Z. Zhang, X. Zhu, Sampling-interval-dependent stability for sampled-data systems with state quantization, Int. J. Robust Nonlinear Control, 24 (2014), 2995–3008. https://doi.org/10.1002/rnc.3038 doi: 10.1002/rnc.3038
![]() |
[40] |
Y. Liu, Z. Wang, X. Liu, Global exponential stability of generalized recurrent neural networks with discrete and distributed delays, Neural Networks, 19 (2006), 667–675. https://doi.org/10.1016/j.neunet.2005.03.015 doi: 10.1016/j.neunet.2005.03.015
![]() |
[41] |
J. Wang, X. Zhang, Q. Han, Event-triggered generalized dissipativity filtering for neural networks with time-varying delays, IEEE Trans. Neural Networks Learn. Syst., 27 (2016), 77–88. https://doi.org/10.1109/TNNLS.2015.2411734 doi: 10.1109/TNNLS.2015.2411734
![]() |
[42] |
Z. Feng, H. Shao, L. Shao, Further results on event-triggered H∞ networked control for neural networks with stochastic cyber-attacks, Appl. Math. Comput., 386 (2020), 125431. https://doi.org/10.1016/j.amc.2020.125431 doi: 10.1016/j.amc.2020.125431
![]() |
[43] |
Q. Han, Y. Liu, F. Yang, Optimal communication network-based H∞ quantized control with packet dropouts for a class of discrete-time neural networks with distributed time delay, IEEE Trans. Neural Networks Learn. Syst., 27 (2016), 426–434. https://doi.org/10.1109/TNNLS.2015.2411290 doi: 10.1109/TNNLS.2015.2411290
![]() |
[44] |
X. Zhang, Q. Han, X. Ge, D. Ding, An overview of recent developments in Lyapunov-Krasovskii functionals and stability criteria for recurrent neural networks with time-varying delays, Neurocomputing, 313 (2018), 392–401. https://doi.org/10.1016/j.neucom.2018.06.038 doi: 10.1016/j.neucom.2018.06.038
![]() |