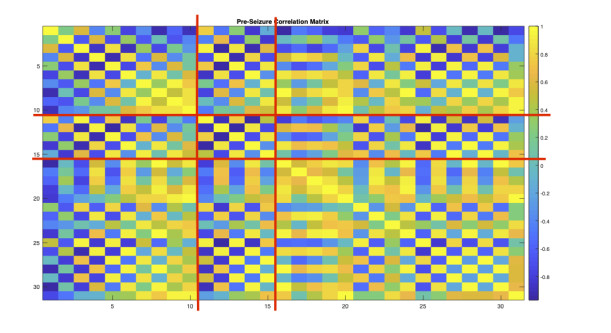
Epilepsy is a complex phenomena of a system of highly intensive and synchronized neurons simultaneously firing which can be traced to spatial and temporal patterns. Seizures are a well known physical feature for all types of epileptic disorders. The rhythms, patterns, and oscillatory dynamics explain the mechanistic nature of neurons especially in absence seizures. Previous models such as Wilson-Cowan (1973), introduced brain models showing the dynamics of a network of neurons consisting of excitatory and inhibitory neurons. Taylor et al. (2014) then adapted the Wilson-Cowan model to epileptic seizures using a thalamo-cortical based theory. Fan et al. (2018) projects that thalamic reticulus nuclei control spike wave discharges specifically in absence seizures. We identify brain activity patterns specific to Glucose (G1D) Transport Deficiency Epilepsy in a network model based on electroencephalogram device (EEG) data. Additionally, we study the EEG patterns to identify the plausible mechanism that causes G1D epileptic behavior. Our coupled thalamo-cortical model goes beyond a connection in a logical unidirectional pattern shown by Fan or in a bidirectional small world pattern. Our model is a network based on paired correlation of EEG signals more analogous to realistic seizure activity. Using our model, we are able to study stability analysis for equilibrium and periodic behavior. We also identify parameter values which cause synchronized activity or more stable activity. Lastly, we identify a synchronization index and sensitivity analysis regarding parameters that directly affect Spike Wave Discharges and other spiking behavior. We will show how our 32-unit data-driven network model reflects G1D seizure dynamics and discuss the limitations of the model.
Citation: Ariel Leslie, Jianzhong Su. Modeling and simulation of a network of neurons regarding Glucose Transporter Deficiency induced epileptic seizures[J]. Electronic Research Archive, 2022, 30(5): 1813-1835. doi: 10.3934/era.2022092
[1] | Zhihui Wang, Yanying Yang, Lixia Duan . Dynamic mechanism of epileptic seizures induced by excitatory pyramidal neuronal population. Electronic Research Archive, 2023, 31(8): 4427-4442. doi: 10.3934/era.2023226 |
[2] | Xiaolong Tan, Hudong Zhang, Yan Xie, Yuan Chai . Electromagnetic radiation and electrical stimulation controls of absence seizures in a coupled reduced corticothalamic model. Electronic Research Archive, 2023, 31(1): 58-74. doi: 10.3934/era.2023004 |
[3] | Xiaochun Gu, Fang Han, Zhijie Wang, Kaleem Kashif, Wenlian Lu . Enhancement of gamma oscillations in E/I neural networks by increase of difference between external inputs. Electronic Research Archive, 2021, 29(5): 3227-3241. doi: 10.3934/era.2021035 |
[4] | Hui Zhou, Bo Lu, Huaguang Gu, Xianjun Wang, Yifan Liu . Complex nonlinear dynamics of bursting of thalamic neurons related to Parkinson's disease. Electronic Research Archive, 2024, 32(1): 109-133. doi: 10.3934/era.2024006 |
[5] | Li Li, Zhiguo Zhao . Inhibitory autapse with time delay induces mixed-mode oscillations related to unstable dynamical behaviors near subcritical Hopf bifurcation. Electronic Research Archive, 2022, 30(5): 1898-1917. doi: 10.3934/era.2022096 |
[6] | Yan Xie, Rui Zhu, Xiaolong Tan, Yuan Chai . Inhibition of absence seizures in a reduced corticothalamic circuit via closed-loop control. Electronic Research Archive, 2023, 31(5): 2651-2666. doi: 10.3934/era.2023134 |
[7] | Denggui Fan, Yingxin Wang, Jiang Wu, Songan Hou, Qingyun Wang . The preview control of a corticothalamic model with disturbance. Electronic Research Archive, 2024, 32(2): 812-835. doi: 10.3934/era.2024039 |
[8] | Weijie Ding, Xiaochen Mao, Lei Qiao, Mingjie Guan, Minqiang Shao . Delay-induced instability and oscillations in a multiplex neural system with Fitzhugh-Nagumo networks. Electronic Research Archive, 2022, 30(3): 1075-1086. doi: 10.3934/era.2022057 |
[9] | Xianjun Wang, Huaguang Gu . Post inhibitory rebound spike related to nearly vertical nullcline for small homoclinic and saddle-node bifurcations. Electronic Research Archive, 2022, 30(2): 459-480. doi: 10.3934/era.2022024 |
[10] | Yunhai Wang, Guodong Huang, Rui Zhu, Shu Zhou, Yuan Chai . Response mechanism of heat-sensitive neurons under combined noise stimulation. Electronic Research Archive, 2024, 32(11): 6405-6423. doi: 10.3934/era.2024298 |
Epilepsy is a complex phenomena of a system of highly intensive and synchronized neurons simultaneously firing which can be traced to spatial and temporal patterns. Seizures are a well known physical feature for all types of epileptic disorders. The rhythms, patterns, and oscillatory dynamics explain the mechanistic nature of neurons especially in absence seizures. Previous models such as Wilson-Cowan (1973), introduced brain models showing the dynamics of a network of neurons consisting of excitatory and inhibitory neurons. Taylor et al. (2014) then adapted the Wilson-Cowan model to epileptic seizures using a thalamo-cortical based theory. Fan et al. (2018) projects that thalamic reticulus nuclei control spike wave discharges specifically in absence seizures. We identify brain activity patterns specific to Glucose (G1D) Transport Deficiency Epilepsy in a network model based on electroencephalogram device (EEG) data. Additionally, we study the EEG patterns to identify the plausible mechanism that causes G1D epileptic behavior. Our coupled thalamo-cortical model goes beyond a connection in a logical unidirectional pattern shown by Fan or in a bidirectional small world pattern. Our model is a network based on paired correlation of EEG signals more analogous to realistic seizure activity. Using our model, we are able to study stability analysis for equilibrium and periodic behavior. We also identify parameter values which cause synchronized activity or more stable activity. Lastly, we identify a synchronization index and sensitivity analysis regarding parameters that directly affect Spike Wave Discharges and other spiking behavior. We will show how our 32-unit data-driven network model reflects G1D seizure dynamics and discuss the limitations of the model.
Epilepsy is a neurological disorder where neuron activity is highly intensive and synchronized which causes seizures. The abnormal behavior is classically considered to originate from an "imbalance between excitation and inhibition in a localized region, multiple brain areas or the whole brain" [1]. Furthermore as described in animal models of epilepsy, epilepsy can be caused by persistent long-term alterations in death of presynaptic connections in addition to synaptic fluid-filled vacuole recycling. For children, epileptic activity lies in the process of how the brain matures. A brain that is not fully formed or matured has the tendency to skew towards increased excitation, specifically mechanisms at the molecular level like the depolarization (increasingly non-negative voltage) of GABA and overly expressive NDMA (N-methyl-D-aspartate) receptors which are responsible for memory and learning impairments both cause excitation within the neuronal network. G1D Transporter Deficiency Epilepsy, also known as the GLUT1 Deficiency Syndrome, is a disorder which affects the nervous system. G1D is caused by mutations in the SLC2A1 gene. This gene gives instructions to produce Glucose Transporter Protein type 1 (GLUT1). Most importantly, the GLUT1 protein moves glucose between glia (cells within the brain), which protect and maintain neurons. G1D can be identified by the high number of seizures during the infant stage which is accompanied by rapid and irregular eye movement. Most are visually recognized when the size of the brain and skull grow at a very slow rate. As a result, the patient usually has a smaller head size, otherwise known as microcephaly. Due to microcephaly, G1D patients do experience developmental delays and mental/intellectual incapabilities.
Common Neurological issues are muscle tenseness which causes stiffness, ataxia or complications with correlating body movements, dysarthria which are speech difficulties, lethargy, headaches, muscle twitches mainly during times of fasting, and confusion.
GLUT1 then transports sugar into the cells from the blood or other cells to use as fuel. G1D is diagnosed through a blood test. GLUT1 is responsible for moving glucose around as the brain's main energy source, specifically within the blood-brain barrier, which gravely affects developmental growth. According to the National Institute of Health, G1D is a very rare disorder. Only about 500 cases have been reported across the world since its discovery in 1991. However, many researchers believe that this means the disorder is under diagnosed due to its similar symptoms to other neurological disorders.
Our starting point is considering full brain EEG from a patient with Glucose Transport Deficiency Type I Epilepsy (G1D). G1D is a genetic disorder where epilepsy is the main symptom along with microcephaly. G1D has a specific type of epilepsy where the patients suffer from absence seizures. We developed an ODE model adapted from the Taylor model where we added correlation coefficients and coupling strength terms. Our goal to understand how we can analyze G1D from an EEG data set aiming to learn specific identifying characteristics due to its rarity and high risk of misdiagnosis. Finding a standard set of data patterns is quite complex. We strive to give the fundamental characteristics of data seen from EEG electrodes of a patient with G1D.
Clinically, researchers have found a way to produce imaging bio-markers of spike-and-wave discharges from patients with G1D by using fMRI informed EEG data. In this case, with the use of EEG data, we extract a correlation matrix and base our equations within the thalamocortical model to give dynamic characters of the neuronal behavior. Our network relation is bidirectional due to the correlation coefficients.
We want to study the neuron patterns [2] to identify synchronization mechanisms of this brain disorder. Historically, all four neuronal populations (Pyramidal, Inhibitory, Thalamic Reticular, and Specific Relay) communicate at different time scales. To verify our model, we will use the EEG data in a case study where sample data was acquired in UT Southwestern Medical Center in Dallas) to compare Spike Wave Discharges (SWD) and swindle neuronal behavior within real patient data given that an underdeveloped brain is reflected on EEG data.
In thalamic sleep rhythms and other neuronal systems in the brain [3,4,5,6,7,8,9,10], neurons have been observed experimentally to engage in a rhythmic pattern of behavior referred to as bursting. In bursting, neuronal activity alternates between active phases, characterized by large amplitude oscillations, and quiescent phases, associated with oscillations of much smaller amplitudes (see Figures 5, 6 below). In this case, an absence seizure which also stems from the thalamus augments the burst a bit by adding noise to the onset of a burst causing small and large oscillatory behavior.
Mathematically, the complications involved in busting are related to a dynamical phenomenon known as delayed bifurcation or delay of stability loss, defined by Suczynski [11]. Very similar activity is seen regarding the G1D model. Solutions behave differently with slowly changing parameters at each time step. In Figure 10, we see Thalamo-Cortical oscillations whereas no spike in the coupled unit model. Whereas, as k6 grows larger, the coupled unit model exhibits very large oscilatory behavior and the single-unit model continues with periodic fast and low-threshold spiking. The averages between the cortical or thalamical units tend to stay close to a steady state as time increases, then spiking begins at t=59s. Subsequently, after a substantial time delay, solutions jump away from steady state.
De Vries and Sherman [12] both studied the electrical behavior of coupled pancreatic β-cells with a focus on the beneficial influence of noise. However, small random perturbations may have dramatic effects on dynamical systems and lead to the emergence of new dynamical behaviors [13]. Stochastic resonance is a well-known example [14,15]. The term stochastic resonance is given to a phenomenon that is manifest in nonlinear systems whereby generally feeble input information (such as a weak signal) can be amplified and optimized by the assistance of noise [14].
It was suggested previously that stochastic fluctuations of ion channels in the plasma membrane are responsible for disrupting bursting behavior and transforming isolated cells to spikers, but that the effective sharing of channels by electrically coupled cells averages the noise and allows the bursting phenomena to appear [15]. This was later analyzed in [15,16] using mathematical modeling. In [18], the work of Pedersen and Sorensen supports previous investigations of the channel sharing hypothesis by the application of two recent methods, which allow an analytic treatment of stochastic effects on the location of the saddle-node and homoclinic bifurcations that are relevant to bursting activity. The work of Su et al. [19] also analytically characterizes the influence of noise on phase switching, in the case of elliptic bursting dynamics.
The order of this research paper is as follows, we begin to find the correlations between different electrode-based EEG data and incorporate Fang model to build a full network model. The full network with minimal and large coupling parameter. We will then use this information to analyze the interference terms within the behavior of the full neuronal network.
We first import EEG recording data through an MNE-toolbox and Python interface, explained in further detail in the next section. From the Python interface we can produce a correlation matrix between different nodes. Each node of network is built from previous knowledge of how its four neuronal populations are intertwined. The full brain data then leads to a branch point and node graph produced from common connections seen from correlation matrix.
Neuronal regions or electrodes will be used interchangeably as the electrodes represent a spatially localized neuronal region and not individual neurons.
Neurons form a large yet complex network. Before developing the mathematical model, we must first observe how the brain naturally communicates. Under the assumption of a seizure free state. The goal is to visualize how each neuron signals target specific areas elsewhere in the brain. A detection of activity is a pattern seen, specific to a patients brain during the resting or non-seizure state.
In Figure 1, a correlation matrix is shown for the non-seizure data. We set the central voltage as ground zero. The rest of the network dynamics is 31 nodes. The recording data is for 2 minutes. The correlation matrix, A∈R31×31 matrix where each element of the matrix is calculated by the measure of their linear dependence between the two values based from the Pearson Correlation equation.
There are two correlation coefficient formulas used to measure the strength of the relationship between two variables. In the case of seizure and non-seizure data, Pearson was used to gauge the linear relationship between electrodes where r changes as the strength and direction of the relationship adjusts. Whereas the Spearman correlation can evaluate a monotonic relationship between two variables — Continuous or Ordinal and it is based on the ranked values for each variable rather than the raw data. Given our model is based from raw data, the Pearson correlation formula was deemed more applicable. The following Matlab algorithm shows how the figure was produced:
s_ccp = corrcoef(nonseizure_correlation);imagesc(s_ccp)title('Pre-Seizure Correlation Matrix') |
ρ(X,Y)=1N−1N∑i=1(Xi−μXσX)(Yi−μYσY) | (2.1) |
where X=(Xi) and Y=(Yi).
The range of the correlation coefficient ρ(X,Y)∈[−1,1] where 1 is a direct positive correlation and −1 is a direct negative correlation.
Symbol | |
the correlation coefficient of the relationship between the variables |
|
the values of the X-variable in column i | |
mean of the values of the X-variable | |
standard deviation of the values of the X-variable | |
the values of the Y-variable in column i | |
mean of the values of the Y-variable | |
standard deviation of the values of the Y-variable | |
number of measurements taken for |
The value indicates the strength of the relationship, specifically a(i,j) is the correlation between the voltage in electrode i and the voltage in electrode j. In our case, each vector represents average membrane voltage of a neuronal region recorded by the electrode over a period of time.
From this correlation matrix, we are able to see how each electrode is positively correlated by a value greater than 0 or negatively correlated, a value less than zero. We then can produce a network, starting with a data structure graph. In Figure 2, branch points and nodes diagram depicting how each electrode builds its network.
Each node represents an electrode or neuronal region. The branches form between each node if the correlation value a(i,j) is greater than 0.6 or less than −0.6, our assumption is that electrode i is highly positively or negatively correlated to electrode j, converse is true as well.
On average, each electrode connects with 9.5 other electrodes in an excitatory fashion. On the other hand, an average of 5.8 inhibitory connections take place within this patients brain pre-seizure EEG data. The electrode mapping for positive and negative correlation is to show initial arguments of how well the brain synchronizes or connects when seizures are not present. All connections are a reflection of the EEG data which tracks electrical activity on the scalp. Deeper connections between the thalamus and cortex cannot be accurately depicted through the EEG. The diagram above shows symbolic excitatory and inhibitory relationships between the electrodes on a microscopic level.
Looking further, regarding the positive correlation graph, there are several neuronal regions that are central or commonly interactive to excitatory behavior:
Electrode: 11, 25, 13, 15, 7, 31, 10, 30, 6, 24, 26 |
Overall, electrodes 3,4,6,8,14,20,22,24,26,27,28,29,30,31 are the 14 electrodes whom have above average connections which are outer nodes to the central electrodes.
Whereas the graph for negative correlations is not as linear or uniform in pattern. In this case, electrodes 1,2,3,4,5,6,11,12,13,14,21,22,25,26,27,28,29 are the 17 electrodes whom have above average connections. Furthermore, only 9 electrodes are well above average, meaning their number of connection is greater than the variance of the set of number of total connections. We use this information as a control, a way to compare brain activity at resting state to disease state.
In order to see how the neurons build up their seizure network, we produced a correlation color plot. All data is based from the work of [2]. A spectral, visual representation of the correlation matrix. As described in section 2.1, correlation is a mutual relationship or connection between two or more neuronal regions. It shows the level of interdependence of variable quantities. In the figure below, we see the correlation color plot for the patient's seizure data, recorded in 2 minutes.
Two neuronal regions may work together to either inhibit or excite one another, producing an inhibitory relationship or an excitatory relationship. Voltage quantities for one region can increase otherwise known as depolarize and cause the other to decrease in voltage or hyper-polarize.
Brain activity during a seizure produces a different pattern compared to the pre-seizure pattern. A general circular pattern is seen for the positive correlation graph, Figure 4 (top) for the seizure period.
In summary, we find that our graphs do give a useful visual as to how well the brain is functionally connected in a healthy or non seizure activity. Yet it may not give an effective or accurate analysis of how synchronized various brain regions are connected before and during a seizure. Each electrode is numbered a quantitative view of how the electrodes connect at one particular instant, in a healthy and unhealthy state. We look for most electrodes to have above average connections yet our goal would be to pinpoint more electrode connections made during a seizure. The reason for this occurrence is we need to calculate only at the very brief time intervals of large spike waves during the epilepsy period, rather than a fixed time period.
Our overarching goal of characterizing the seizure will be achieved through several quantitative studies. The answer is within the following two questions:
1. What is phase synchrony?
2. Once a phase has been defined, how does one know that all channels are in synch?
Seizures are characterized by highly synchronized neuronal activity across the brain, in localized regions, or multiple spatially localized regions simultaneously connecting with other regions.
The full network model is based on the early work of Fang [28], yet the coupling between each thalami-cortical unit is based on data calculated in Section 2 for functional connectivity for seizure recording. We consider the following 31-network model:
dPYjdt=(hp−PYj+κ1sig(PYj)−κ2sig(INj)+κ3sig(TCj))τ1 | (3.1) |
+λ1Σi,jA(i,j)(SRNj+α2TRNj),dINjdt=(hi−INj+κ4sig(PYj))τ2, | (3.2) |
dSRNjdt=(ht−SRNj+κ5sig(PYj)−κ62(sTRNj))τ3+λ2Σi,jA(i,j)(PYi+INi), | (3.3) |
dTRNjdt=(hr−TRNj+κ7sig(PYj)+κ82(sSRNj)−κ92(sTRNj))τ4 | (3.4) |
where PY, IN, SRN, TRN represent population average voltage, and j is the index for the number of thalami-cortical units. The couplings between each units are based on calculated correlation coefficient from brain EEG data during seizure periods. We avoid any additional assumptions.
For reference,
Symbol | |
Firing rates (voltage) | |
additive input constants | |
-0.35, -3.4, -2.0, -5 | |
external control input into response module | |
-0.3/-0.01, -0.3/-0.01, 0, 0 | |
connectivity strengths within different neuronal populations | |
listed below | |
activation functions for the cortical and thalamic modules | |
time scale parameters for each neuronal population | |
26, 1.25*26, 26*0.1, 26*0.1 | |
correlation matrix | |
approximation function |
The large network consists of 31 units with 4 neuronal populations each with coupled terms to connect the total 124 equations. In order to analyze a system of this size, we used MATLAB function ODE45 to solve the system of ordinary differential equations and Mathematica NDSolve function to detect changes in neuronal activity and based our values from those listed in Table 3. With MATLAB, the behavior is simulated within two minutes for 100 seconds of a neuronal process. Through many simulations and parameter sensitivity analysis, we have substantiated the fact that k6 is the altering parameter as seen in the Taylor model.
Parameter | Origin | Target Area | Value |
κ1 | PY | PY | 1.8 |
κ2 | IN | PY | 1.5 |
κ3 | SRN | PY | 1 |
κ4 | PY | IN | 4 |
κ5 | PY | SRN | 3 |
κ6 | TRN | SRN | varying |
κ7 | PY | TRN | 3 |
κ8 | SRN | TRN | 10.5 |
κ9 | TRN | TRN | 0.2 |
The network is based on actual connectivity data for seizure brain, as described in section 2. Specifically, before and after the Hopf-Bifurcation point in order to highlight the major differences in behavior. This Hopf-Bifurcation point is first realized through Fang et al. [28,29,30] which has a focus on one unit. In this paper, a build up of the Fan study focuses on the comparison from non-seizure brain network to seizure brain network is shown below then onto the Hopf-Bifurcation point delays or lack thereof with coupling and other mathematical techniques.
In this section, we study how the neuronal spiking behavior changes as a result of parameter k6. This particular parameter represents the relationship between Thalamic Reticular Nuclei and Specific Relay Nuclei, both populations of neurons located in the thalamus. Our goal is to not only find exact parameter values which drive these changes seen when adjusting the coupling strength but also to identify the induced transitions. Using values found in Table 3, the value of the k6 parameter is augmented at each time step, k6=k6,i+ϵt in order to highlight the varying spiking behavior that could occur. In this section, see Figure 6, we investigate the specific range of parameter values that lead to transitions of one phase to other firing rates that may be induced from inhibitory synaptic coupling strength from TRN onto SRN.
For reference,
Symbol | Coupling Coefficient Starting Value | Coupling Coefficient Final Value | Increment Size |
0.2 | 1.2 | 0.01 | |
0.005 | 0.105 | 0.001 |
In Figure 6, we depict the average value of (PYj+INj)/2, where j=1,...,31 when λ1,λ2 are increases slowly according to the time t and k6=4 is below the Hopf-Bifurcation point. We observe as the coupling strength λ1, λ2 increase, the behavior of PYj, INj reduces their oscillation frequency and converges to steady states. These figures shows actual behavior seen from our mathematical model. Disease state spiking behavior which can be seen in three phases. The first phase is fast spiking or inhibitory spiking, followed by a slower paced thalamocortical spiking with a bit of spike wave discharges. And lastly, a periodic spindle episode with slower frequency of 0.1- 0.2 Hz that lay on top of a low threshold spike then to end with a slow wave.
Through extensive simulations and data analysis, Fan [28,29], projects that k6 is the key parameter that alters stability for a linear or small world type model. We completed stability analysis with the use of MATLAB and also found that within the full network system, k6 is still a key parameter even with the addition of network structure from real data and a coupling strength term. We solve our system of ODEs with MATLAB ODE45 which then simulates the brain rhythms during an epileptic seizure within four minutes.
We studied the changes seen when adding periodic perturbations while keeping the coupling coefficient at a fixed value, then varying the coupling coefficient along with the periodic perturbation as seen below in section 4.3.1, based from adaptive resonance theory for brain processing information [10]. The model becomes Eqs 3.1–3.4 with an addition of ϵsin(πtfreq) to the PYj, SRNj equations in Eqs 3.1–3.4 as seen in Section 3.1.
Where all equations and parameters remain the same as in section 3, Eqs (3.1–3.4), and the small periodic forcing is a sine function with β=π,freq∈[0.0507,0,0508].
Periodic perturbation leads to dynamics in accordance to the resonance theory. Within neuronal networks, the affect of increasing external periodic forcing is the spiking behavior altering from steady state to small oscillations, large oscillations, bursting, and tonic behavior. In Figure 7 we see little to no activity for the first 7 seconds then a sudden change occurs just before time t=8. Simulation shows the solution exhibiting continuous tonic behavior.
Secondly, we observed the changes to the system as white Gaussian noise was added. The coupling coefficients were held constant, fixed at λ1=0.2 and λ2=0.005, as seen in Table 5, in order to view the spiking changes or lack thereof without varying the coupling strengths although noise will be added via W(t) as time progresses. The model becomes Eqs 3.1–3.4 with an addition of W(t) to the PYj, SRNj equations in Eqs 3.1–3.4 as seen in Section 3.1. Where the W(t) term is the awgn(t) MATLAB function which will add white Gaussian noise to the signal as the time variable t increases.
When noise is added, numerical computations [7,20] and asymptotic methods [8] suggest that the amount of delay due to Hopf-Bifurcation is significantly reduced as seen below in Figure 8. When noise is introduced into a bursting system [8], depending on the amplitude of the noise, it was found that there are regular patterns of alternations between a long active phase and a long silent phase, regular patterns of alternations between short active and silent phases, as well as irregular patterns of alternations of phases with various time durations. When the noise amplitude is set to be extremely close to zero, the irregular patterns give way to a pattern that strongly resembles deterministic bursting. But even with a noise of quite small magnitude, the irregularity is significant. Kuske and Baer [31] determine that this irregularity follows from random variation in the delay of stability loss, based on an asymptotic approximation of the probability density function for the state of the system in the silent phase and an asymptotic analysis of the effect of noise on transitions out of the active phase.
A drive response mechanism is a result of synchronized brain activity commonly seen during an epileptic seizure [7,9]. The first section show explicitly how we evaluate frequency analysis and define phase synchrony and degree of synchrony.
The interest of frequency analysis of EEG data has become increasingly popular, as a result various methods have been developed to evaluate amplitude and phase assessments in comparison to the various Fourier Transforms. Frequency domains have assumptions regarding tasks and physical state of the matter involved. Various oscillations can be visualized in different parts of the brain. A particular task in association with a change in frequency character in brain network.
Figures 9–11 imply a drive response mechanism which is a result of synchronized brain activity commonly seen during an epileptic seizure [7,9]. Given that we are continually processing signals from EEG data, we must communicate vital information such as frequency, phase synchrony, general degree of synchrony and instantaneous amplitude, frequency relation to gain insight of seizure mechanism.
For the full network without any changes to the model, we evaluated the frequency by counting the number of spikes over a fixed duration of time as a control. The resulting frequency was used in simulations regarding periodic perturbation.
This process was repeated for the 31-unit model with noise, increasing coupling strength, and a combination of both noise and coupling strength. Our main focus is before and during the suspected point of bifurcation.
A particular task can be viewed in change in frequency. The figures above show a comparison before and after the Hopf-Bifurcation point specifically focused on frequency levels between seizure and non-seizure data. There is a visible in the increased frequency level.
Phase Synchrony is a process commonly used for full brain analysis due to its adaptability to time resolution. It is a three-step technique used to give further neurophysiological information aside from frequency analysis about a patients brain rhythms. The overarching goal is to interrelate the results to cognitive processes, neurological connections, attentiveness, and much more.
The calculations of phase synchrony for the time series xi(t),i=1,...,n consists of:
(1) Hilbert Transform:
H[x1(t)]=1πP.V. ∫∞−∞x1(τ)t−τdτ. |
Where P.V. is the Cauchy Principal Value, a method used to assign a value to singular integrals with odd or even integrands (or neither) and non-symmetric intervals, under the assumption that the limit converges at the same rate.
(2) Instantaneous Phase:
ϕ1(t)=arctanH[x1(t)]x1(t) |
(3) Mean Phase Coherence:
Computed in the complex planeλ=1N|ΣNt=1ej[ϕ1(t)−ϕ2(t)]| |
An averaging over the membrane potential, Xi between two electrode (signals) at time t, which measures the oscillatory populations disposition to develop total synchrony [10].
A common practice in analyzing neurologically based models is to find the value of the level of synchrony of a network of neurons or a comprehensive grouping of neurons within a network, a neuronal synchrony measure. The value of neuronal synchrony lies between 0 and 1. To truly understand the level of synchrony, one must define synchrony in terms of a group of neurons. A group of neurons are in synch when all neurons fire action potential simultaneously, gaining a level of synchrony of 1. Conversely, a degree of synchrony of 0 corresponds to asynchronization of action potentials.
Degree of synchrony is a very similar term in that pertains to a large grouping of neurons or network but incorporates the signaling process. Locally found by evaluating two signals based on the direction of their trajectory at time t. Some researchers use a geometrical approach to evaluate the angular trajectory using cosine and sine waves while others define a measure χ(x,N) to calculate spatially decaying relationships between electrodes. In our case, due to the narrow yet long data matrix, using the method of comparing singular values was most suitable.
We complete the following steps with the use of MATLAB:
● Average four variable which represent the four neuronal populations within the 31-unit network, then the separate excitatory and inhibitory populations, for a full 10 seconds.
● Find the singular value matrix, left singular matrix, and right singular matrix
● For each ms, the top 2 singular values we placed into a general singular value matrix, resulting in 200 singular values along the diagonal of the S matrix
● Evaluate a ratio of the singular values from the 31 electrodes for every ms
Explicit ratio formula for degree of synchrony (DOS) is:
DOS=(M/(M−1))(L(31))/(L(1)−1/M) | (4.1) |
where M is the number of electrodes and L is the list of singular values found along the diagonal of matrix S. The resulting value lies between 0 and 1, with 1 representing fully synchronized versus 0 meaning no synchrony between the channels in question. Figure 12 shows the varying values between 0 and 1 as DOS is computed throughout the full length of seizure data along with various coupling strengths.* We have found that multiple pathways of connection lead to various phases of synchronizations between the network of neurons, including the degree of synchronization with specific parameters.
* Full MATLAB code is available upon request.
Considering the full 31-unit model, we alter the periodic perturbation frequency and visualize the resulting degree of synchrony. To do so, we calculated frequency with increasing time. A varying κ6 value, which increases over time given the Hopf-Birfurcation point lies 4<κ6<5. As seen below, the increased forcing in addition to augmented k6 value, the degree of synchrony goes through a phase of toggling between very gradual incline and relatively steep decline then an abrupt increase that continues until the end of the time duration. In some cases, a sudden decrease occurs as t>0.85, indicates the end of seizure.
The subgrapghs in Figure 13 are an indication of heightened synchrony within the full network based upon seizure data. Range of the y-axis, Degree of Synchrony is the same for all graphs which shows that the synchrony remains between (0.48,0.52) with increasing noise levels due to the synchronizing nature of a brain during a seizure. Coupling strength also enforces the synchronized behavior within zeizure data.
Daci et al. [27] research concludes that a ketogenic diet - a diet high in fat and low in protein - allows for a significantly lower consumption of glucose within the brain. Which then allows for an alternaitve sources known as ketone bodies. Ketone bodies have an action through "GABA synthesis and reception". This action mechanism results in "neuroprotective effect and antiepileptogenesis". Daci et al. also make note of drug therapies which certain genes have been receptive to which means pharmokinetics influence brain excitability. There were no identified biomarkers but extensive data through various treatment options.
Several computational neurological models have been developed over the last 40 years for small and large neuronal networks, cellular models for ion channel dynamics, and mathematical models to visualize the effect of neuronal connections between the cortex and thalamus. In order to retrieve a full picture of patterns seen in EEG data, it has been proven in literature that a thalamocortical coupled model is best.
In Section 2.1 and 2.2, we found that our graphs do give a useful visual as to how well the brain is connected in a healthy or non seizure activity. Yet it may not give an effective or accurate analysis of how synchronized various brain regions are connected before and during a seizure. The correlation plot will only give an average at just one time point considered rather than a fixed time period so incorporating this information within the model was imperative. It was found that an absence seizure, a sign of G1D, augments the elliptic burst a bit by adding noise to the onset of an elliptic burst, as shown in section 4.3. Consequently, after a substantial time delay, solutions jump away from steady state and Hopf-Bifurcations are formed. Our major results that coupling strength and self-interference terms impact stability of the system in addition to delaying the Hopf-Bifurcation. Additionally, a comparison of preseizure and seizure network as seen in section 4.5, we found that the seizure data causes the system to be highly synchronized as coupling strength increases and the addition of noise.
In this study, we produced an ODE model comprised of four neuronal populations that are most active in Glucose Transport Deficiency Type 1 Epilepsy. We characterized spiking behavior changes seen as a result of varying coupling strength and noise. Overall, our research has given a better picture of the dynamical properties seen from this form of epilepsy. This model described the spiking neuronal activity seen an epileptic induced seizure network through. By dissecting the correlation association of neuronal units or electrodes, the subpopulations of the cortex and thalamus in seizure data, certain patterns were classified. Our model is versatile in the manner in which simulations can be produced with a change in coupling and the addition of coupling strengths. The model is built to highlight the connections in the network derived from data.
Neurons show significant variation in the presence and timing of action potentials across stimulus trials, a phenomenon whose function and significance has been the subject of great interest. Cortical activity is characterized by highly irregular interspike intervals.
From our simulations, we are able to find specific parameter ranges that which cause a class or classes of diseased state spiking neuron. t=0−77 all signify a change in spiking neuron characterized as tonic bursting, thalmaocortical oscillations, and spindles. One positive effect on noise and bifurcation is they have modulated the synchronization of the network, both through delayed bifurcation and noise, as a result of coupling.
More so, the role of the κ6 parameter originating from the TRN targeting SRN as the inhibition of thalamic reticular to excitatory neurons in the Thalams is crucial in thalamus-cortex interaction. A larger κ6 value causes more inhibition. The latency of signal transmission may provide a different mechanism to induce synchronization in neuronal network [26]. Additionally, the setting of the mathematical results are very general, the mechanism is working for a large class of equations. However, we were able to see a comparison of non-seizure and seizure network in this study, concluding that the seizure is highly synchronized as coupling strength increases and the addition of noise.
We plan to continue our work by closely studying sensitivity analysis regarding parameters that directly affect SWD and spindles using analysis such as Partial Rank Correlation Coefficient. We would also consider neurological sensitivities with respects to the self- interference terms represented by ci values in our model. Our large model could be more accurate with an increased data based model comparison to more G1D patients. Lastly, we plan to perform analyzing a visual representation of sparsity within the larger spatial inverse problem yielded from the source patterns.
Funding for this research was provided by University of Texas at Arlington (UTA) Department of Mathematics Graduate Assistance for Areas of National Need via the US Department of Education. In addition to, the National Science Foundation (NSF) Louis Stokes Alliance for Minority Participation Bridge to the Doctorate Fellowship (Grant No. HRD-1026806).
The authors declare there is no conflict of interest.
[1] |
G. Deco, A. Ponce-Alvarez, P. Hagmann, G. L. Romani, D. Mantini, M. Corbetta, How Local Excitation–Inhibition Ratio Impacts the Whole Brain Dynamics, J. Neurosci., 34 (2014), 7886–7898. https://doi.org/10.1523/JNEUROSCI.5068-13.2014 doi: 10.1523/JNEUROSCI.5068-13.2014
![]() |
[2] |
H. Zhang, J. Su, Q. Wang, Y. Liu, L. Good, J. Pascual, Predicting seizure by modeling synaptic plasticity based on EEG signals—a case study of inherited epilepsy, Commun. Nonlinear Sci. Numer. Simul., 56 (2018), 330–343. https://doi.org/10.1016/j.cnsns.2017.08.020 doi: 10.1016/j.cnsns.2017.08.020
![]() |
[3] | J. M. Bekkers, Pyramidal Neurons, Curr. Biol., 21 (2011), R975. https://doi.org/10.1016/j.cub.2011.10.037 |
[4] |
R. B. Gonzales, C. J. DeLeon Galvan, Y. M. Rangel, B. J. Claiborne, Distribution of thorny excrescences on CA3 pyramidal neurons in the rat hippocampus, J. Comp. Neurol., 430 (2001), 357–368. https://doi.org/10.1002/1096-9861(20010212)430:3<357::AID-CNE1036>3.0.CO;2-K doi: 10.1002/1096-9861(20010212)430:3<357::AID-CNE1036>3.0.CO;2-K
![]() |
[5] |
S. B. Nelson, C. Hempel, K. Sugino, Probing the transcriptome of neuronal cellmtypes, Curr. Opin. Neurobiol., 16 (2006), 571–576. https://doi.org/10.1016/j.conb.2006.08.006 doi: 10.1016/j.conb.2006.08.006
![]() |
[6] | P. N. Taylor, G. Baier, S. S. Cash, J. Dauwels, J. Slotine, Y. Wang, A model of stimulus induced epileptic spike-wave discharges, 2013 IEEE Symposium on Computational Intelligence, Cognitive Algorithms, Mind, and Brain (CCMB), (2013), 53–59. https://doi.org/10.1109/CCMB.2013.6609165 |
[7] | R. Swenson, The Thalamus - Thalamic Organization, Dartmouth Medical School, 2006, available from: www.dartmouth.edu/rswenson/NeuroSci/chapter_10.html. |
[8] |
H. R. Wilson, J. D. Cowan, A mathematical theory of the functional dynamics of cortical and thalamic nervous tissue, Kybernetik, 13 (1973), 55–80. https://doi.org/10.1007/BF00288786 doi: 10.1007/BF00288786
![]() |
[9] | D. Golomb, Neuronal Synchrony Measures, Scholarpedia, 2 (2007), 1347. https://doi.org/10.4249/scholarpedia.1347 |
[10] |
N. Kopell, G. B. Ermentrout, M. A. Whittington, R. D. Traub, Gamma Rhythms and Beta Rhythms Have Different Synchronization Properties, Proc. Natl. Acad. Sci. U.S.A., 97 (2000), 1867–1872. https://doi.org/10.1073/pnas.97.4.1867 doi: 10.1073/pnas.97.4.1867
![]() |
[11] |
P Suczynski, S Kalitzin, F. L. Da Silva, Dynamics of non-convulsive epileptic phenomena modeled by a bistable neuronal network, Neurosci., 12 (2004), 467–484. https://doi.org/10.1016/j.neuroscience.2004.03.014 doi: 10.1016/j.neuroscience.2004.03.014
![]() |
[12] | G. De Vries, A. Sherman, Channel sharing in pancreatic β-cell revisited: enhancement of emergent bursting by noise, J. Theor. Biol., 207 (2000), 513–530. https://doi.org/10.1006/jtbi.2000.2193 |
[13] |
S. Intep, D. J. Higham, Zero, one and two-switch models of gene regulation, Discrete Contin. Dyn. Syst. B, 14 (2010), 495–513. https://doi.org/10.3934/dcdsb.2010.14.495 doi: 10.3934/dcdsb.2010.14.495
![]() |
[14] |
M. I. Freidlin, Quasi-deterministic approximation, metastability and stochastic resonance, Phys. D, 137 (2000), 333–352. https://doi.org/10.1016/S0167-2789(99)00191-8 doi: 10.1016/S0167-2789(99)00191-8
![]() |
[15] | L. Gammaitoni, P. Hanggi, P. Jung, F. Marchesoni, Stochastic Resonance, Rev. Mod. Phys., 70 (1998), 223–287. https://doi.org/10.1103/RevModPhys.70.223 |
[16] |
T. R. Chay, H. S. Kang, Role of single-channel stochastic noise on bursting clusters of pancreatic_-cells, Biophys. J., 54 (1988), 427–435. https://doi.org/10.1016/S0006-3495(88)82976-X doi: 10.1016/S0006-3495(88)82976-X
![]() |
[17] |
A. Sherman, J. Rinzel, J. Keizer, Emergence of organized bursting in clusters of pancreatic beta-cells by channel sharing, Biophys. J., 54 (1988), 411–425. https://doi.org/10.1016/S0006-3495(88)82975-8 doi: 10.1016/S0006-3495(88)82975-8
![]() |
[18] |
M. Pedersen, M. Serensen, The effect of noise on β-cell burst period, SIAM J. Appl. Math., 67 (2007), 530–542. https://doi.org/10.1137/060655663 doi: 10.1137/060655663
![]() |
[19] |
J. Su, J. Rubin, D. Terman, Effects of noise on elliptic bursters, Nonlinearity, 17 (2004), 133–157. https://doi.org/10.1088/0951-7715/17/1/009 doi: 10.1088/0951-7715/17/1/009
![]() |
[20] |
P. N. Taylor, Y. Wang, M. Goodfellow, J. Dauwels, F. Moeller, U. Stephani, A computational study of stimulus driven epileptic seizure abatement, PloS One, 9 (2014), e114316. https://doi.org/10.1371/journal.pone.0114316 doi: 10.1371/journal.pone.0114316
![]() |
[21] |
R. D. Traub, D. Contreras, M. O. Cunningham, H. Murray, F. E. N. Lebeau, A. Roopun, et al., Single-column thalamocortical network model exhibiting gamma oscillations, sleep spindles, and epileptogenic bursts, J. Neurophysiol., 93 (2005), 2194–2232. https://doi.org/10.1152/jn.00983.2004 doi: 10.1152/jn.00983.2004
![]() |
[22] |
Z. Feng, A. Lubbe, Q. Lu, J. Su, On bursting solutions near chaotic regimes in a neuron model, Discrete Contin. Dyn. Syst. S, 7 (2014), 1363–1383. https://doi.org/10.3934/dcdss.2014.7.1363 doi: 10.3934/dcdss.2014.7.1363
![]() |
[23] |
P. N. Taylor, Y. Wang, M. Goodfellow, J. Dauwels, F. Moeller, U. Sephani, et al., A computational study of stimulus induced driven epileptic seizure abatement, PLoS One, 9 (2014), e114316. https://doi.org/10.1371/journal.pone.0114316 doi: 10.1371/journal.pone.0114316
![]() |
[24] |
R. D. Traub, R. K. Wong, R. Miles, H. Michelson, A Model of a CA3 Hippocampal Pyramidal Neuron Incorporating Voltage-Clamp Data on Intrinsic Conductances, J. Neurophysiol., 66 (1991), 635–650. https://doi.org/10.1152/jn.1991.66.2.635 doi: 10.1152/jn.1991.66.2.635
![]() |
[25] |
G. Baier, M. Goodfellow, P. N. Talor, Y. Wang, D. J. Garry, The Importance of Modeling Epileptic Seizure Dynamics as Spatio-Temporal Patterns, Front. Physiol., 3 (2012), 281. https://doi.org/10.3389/fphys.2012.00281 doi: 10.3389/fphys.2012.00281
![]() |
[26] |
Q. Wang, X. Shi, G. Chen, Delay-induced synchronization transition in small-world Hodgkin-Huxley neuronal networks with channel blocking, Discrete Contin. Dyn. Syst. B, 16 (2011), 607–621. https://doi.org/10.3934/dcdsb.2011.16.607 doi: 10.3934/dcdsb.2011.16.607
![]() |
[27] |
A. Daci, A. Bozalija, F. Jashari, S. Krasniqi, Individualizing Treatment Approaches for Epileptic Patients with Glucose Transporter TYPE1 (GLUT-1) Deficiency, Int. J. Mol. Sci., 19 (2018), 122. https://doi.org/10.3390/ijms19010122 doi: 10.3390/ijms19010122
![]() |
[28] |
D. Fan, S. Liu, Q. Wang, Stimulus-Induced Epileptic Spike-Wave Discharges in Thalamocortical Model with Disinhibition, Sci. Rep., 6 (2016), 1–21. https://doi.org/10.1038/srep37703 doi: 10.1038/srep37703
![]() |
[29] | D. Fan, J. Su, A. Bowman, Rich Dynamics Induced by Synchronization Varieties in the Coupled Thalamocortical Circuitry Model, In Brain Informatics: International Conference, BI 2018, Springer, 2018, 78–84. https://doi.org/10.1007/978-3-030-05587-5_8 |
[30] |
D. Fan, Q. Wang, J. Su, H. Xi, Stimulus-Induced Transitions between Spike-Wave Discharges and Spindles with the Modulation of Thalamic Reticular Nucleus, J. Comput. Neurosci., 43 (2017), 203–225. https://doi.org/10.1007/s10827-017-0658-4 doi: 10.1007/s10827-017-0658-4
![]() |
[31] |
R. Kuske, S. M. Baer, Asymptotic analysis of noise sensitivity in a neuronal burster, Bull. Math. Biol., 64 (2002), 447–481. https://doi.org/10.1006/bulm.2002.0279 doi: 10.1006/bulm.2002.0279
![]() |
[32] |
H. Zhang, J. Jacobs, Traveling Theta Waves in the Human Hippocampus, J. Neurosci., 35 (2015), 12477–12487. https://doi.org/10.1523/jneurosci.5102-14.2015 doi: 10.1523/jneurosci.5102-14.2015
![]() |
Symbol | |
the correlation coefficient of the relationship between the variables |
|
the values of the X-variable in column i | |
mean of the values of the X-variable | |
standard deviation of the values of the X-variable | |
the values of the Y-variable in column i | |
mean of the values of the Y-variable | |
standard deviation of the values of the Y-variable | |
number of measurements taken for |
Symbol | |
Firing rates (voltage) | |
additive input constants | |
-0.35, -3.4, -2.0, -5 | |
external control input into response module | |
-0.3/-0.01, -0.3/-0.01, 0, 0 | |
connectivity strengths within different neuronal populations | |
listed below | |
activation functions for the cortical and thalamic modules | |
time scale parameters for each neuronal population | |
26, 1.25*26, 26*0.1, 26*0.1 | |
correlation matrix | |
approximation function |
Parameter | Origin | Target Area | Value |
κ1 | PY | PY | 1.8 |
κ2 | IN | PY | 1.5 |
κ3 | SRN | PY | 1 |
κ4 | PY | IN | 4 |
κ5 | PY | SRN | 3 |
κ6 | TRN | SRN | varying |
κ7 | PY | TRN | 3 |
κ8 | SRN | TRN | 10.5 |
κ9 | TRN | TRN | 0.2 |
Symbol | Coupling Coefficient Starting Value | Coupling Coefficient Final Value | Increment Size |
0.2 | 1.2 | 0.01 | |
0.005 | 0.105 | 0.001 |
Symbol | |
the correlation coefficient of the relationship between the variables |
|
the values of the X-variable in column i | |
mean of the values of the X-variable | |
standard deviation of the values of the X-variable | |
the values of the Y-variable in column i | |
mean of the values of the Y-variable | |
standard deviation of the values of the Y-variable | |
number of measurements taken for |
Symbol | |
Firing rates (voltage) | |
additive input constants | |
-0.35, -3.4, -2.0, -5 | |
external control input into response module | |
-0.3/-0.01, -0.3/-0.01, 0, 0 | |
connectivity strengths within different neuronal populations | |
listed below | |
activation functions for the cortical and thalamic modules | |
time scale parameters for each neuronal population | |
26, 1.25*26, 26*0.1, 26*0.1 | |
correlation matrix | |
approximation function |
Parameter | Origin | Target Area | Value |
κ1 | PY | PY | 1.8 |
κ2 | IN | PY | 1.5 |
κ3 | SRN | PY | 1 |
κ4 | PY | IN | 4 |
κ5 | PY | SRN | 3 |
κ6 | TRN | SRN | varying |
κ7 | PY | TRN | 3 |
κ8 | SRN | TRN | 10.5 |
κ9 | TRN | TRN | 0.2 |
Symbol | Coupling Coefficient Starting Value | Coupling Coefficient Final Value | Increment Size |
0.2 | 1.2 | 0.01 | |
0.005 | 0.105 | 0.001 |