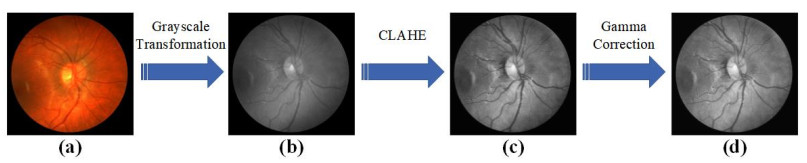
This paper analyses the impact of international spillovers on macroeconomic stability in developing countries. Specifically, the study investigates the impact of United States (US) monetary policy spillovers in the form of US inflation and Federal funds interest rate on Uganda and Kenya's inflation rates, interest rates and the exchange rates, key macroeconomic indicators of importance to macroeconomic stability. The focus on international spillovers from the USA is due to the dominant role it plays in determining global economic conditions. The study applies the Generalized Vector Autoregressive (GVAR) approach to quantify spillovers across these economies. The results shows that despite recent efforts towards East African regional integration, international spillovers from global economies like the US are more significant in determining macroeconomic stability in developing countries, underscoring the importance of global policy coordination. Specifically, we find an amplification of return and volatility spillovers after the onset of the Global financial crisis.
Citation: Lorna Katusiime. International monetary spillovers and macroeconomic stability in developing countries[J]. National Accounting Review, 2021, 3(3): 310-329. doi: 10.3934/NAR.2021016
[1] | G. Prethija, Jeevaa Katiravan . EAMR-Net: A multiscale effective spatial and cross-channel attention network for retinal vessel segmentation. Mathematical Biosciences and Engineering, 2024, 21(3): 4742-4761. doi: 10.3934/mbe.2024208 |
[2] | Caixia Zheng, Huican Li, Yingying Ge, Yanlin He, Yugen Yi, Meili Zhu, Hui Sun, Jun Kong . Retinal vessel segmentation based on multi-scale feature and style transfer. Mathematical Biosciences and Engineering, 2024, 21(1): 49-74. doi: 10.3934/mbe.2024003 |
[3] | Yanxia Sun, Xiang Li, Yuechang Liu, Zhongzheng Yuan, Jinke Wang, Changfa Shi . A lightweight dual-path cascaded network for vessel segmentation in fundus image. Mathematical Biosciences and Engineering, 2023, 20(6): 10790-10814. doi: 10.3934/mbe.2023479 |
[4] | Meenu Garg, Sheifali Gupta, Soumya Ranjan Nayak, Janmenjoy Nayak, Danilo Pelusi . Modified pixel level snake using bottom hat transformation for evolution of retinal vasculature map. Mathematical Biosciences and Engineering, 2021, 18(5): 5737-5757. doi: 10.3934/mbe.2021290 |
[5] | Jinzhu Yang, Meihan Fu, Ying Hu . Liver vessel segmentation based on inter-scale V-Net. Mathematical Biosciences and Engineering, 2021, 18(4): 4327-4340. doi: 10.3934/mbe.2021217 |
[6] | Chen Yue, Mingquan Ye, Peipei Wang, Daobin Huang, Xiaojie Lu . SRV-GAN: A generative adversarial network for segmenting retinal vessels. Mathematical Biosciences and Engineering, 2022, 19(10): 9948-9965. doi: 10.3934/mbe.2022464 |
[7] | Rafsanjany Kushol, Md. Hasanul Kabir, M. Abdullah-Al-Wadud, Md Saiful Islam . Retinal blood vessel segmentation from fundus image using an efficient multiscale directional representation technique Bendlets. Mathematical Biosciences and Engineering, 2020, 17(6): 7751-7771. doi: 10.3934/mbe.2020394 |
[8] | Yinlin Cheng, Mengnan Ma, Liangjun Zhang, ChenJin Jin, Li Ma, Yi Zhou . Retinal blood vessel segmentation based on Densely Connected U-Net. Mathematical Biosciences and Engineering, 2020, 17(4): 3088-3108. doi: 10.3934/mbe.2020175 |
[9] | Yun Jiang, Jie Chen, Wei Yan, Zequn Zhang, Hao Qiao, Meiqi Wang . MAG-Net : Multi-fusion network with grouped attention for retinal vessel segmentation. Mathematical Biosciences and Engineering, 2024, 21(2): 1938-1958. doi: 10.3934/mbe.2024086 |
[10] | Xiaoli Zhang, Kunmeng Liu, Kuixing Zhang, Xiang Li, Zhaocai Sun, Benzheng Wei . SAMS-Net: Fusion of attention mechanism and multi-scale features network for tumor infiltrating lymphocytes segmentation. Mathematical Biosciences and Engineering, 2023, 20(2): 2964-2979. doi: 10.3934/mbe.2023140 |
This paper analyses the impact of international spillovers on macroeconomic stability in developing countries. Specifically, the study investigates the impact of United States (US) monetary policy spillovers in the form of US inflation and Federal funds interest rate on Uganda and Kenya's inflation rates, interest rates and the exchange rates, key macroeconomic indicators of importance to macroeconomic stability. The focus on international spillovers from the USA is due to the dominant role it plays in determining global economic conditions. The study applies the Generalized Vector Autoregressive (GVAR) approach to quantify spillovers across these economies. The results shows that despite recent efforts towards East African regional integration, international spillovers from global economies like the US are more significant in determining macroeconomic stability in developing countries, underscoring the importance of global policy coordination. Specifically, we find an amplification of return and volatility spillovers after the onset of the Global financial crisis.
Fundus image diagnosis in the clinic can assist in screening various diseases, such as hypertension and diabetes. Ophthalmologists can make an initial diagnosis of the disease by observing the morphology of retinal blood vessels. However, the accuracy of automatic fundus vessel segmentation is still unsatisfactory, due to the complex morphology of the vessels and the observer dependence. Therefore, accurate retinal vessel segmentation technologies are extremely valuable in clinical environments.
Currently, the automatic segmentation methods of fundus blood vessels can be mainly divided into two categories: machine learning and deep learning. Furthermore, according to different strategies, machine learning-based approaches can be divided into unsupervised and supervised approaches.
For unsupervised machining methods, Chaudhuri et al. [1] introduced a Gaussian function in the segmentation task for the problem of low local contrast, and successfully designed a two-dimensional matched filter to detect blood vessel segments in images. Li et al. [2] constructed a simple and efficient multi-scale filtering method based on the response relationship of matched filters at three scales. After that, Sreejini et al. [3] introduced the particle swarm optimization algorithm in the multi-scale matched filter method, and discussed the process more comprehensively. The matched filter method is easy to implement, and the amount of calculation is relatively small. However, this method is highly restricted by factors, such as image contrast and noise, and its ability to distinguish vessel pixels from background ones is relatively poor.
In addition, Aibinu et al. [4] proposed a method for segmentation at the crossing and branching of vessels, which uses a hybrid crossing point method to identify the crossing and branching points of vessels, realize vessel tracking and extraction. Finally, Vlachos et al. [5] proposed a linear multi-scale tracking method, which tracks the gray-scale characteristics of blood vessel pixels from the initial seed node to form a gridded extraction of blood vessels. The blood vessel tracking method can obtain very accurate blood vessel width. However, the segmentation effect largely depends on the selection of the initial seed node. In addition, it is susceptible to noise interference, and the problem of segmenting blood vessel breakage occurs.
Moreover, Zana et al. [6] first determined the Gaussian-like contours of blood vessels, and then combined morphological processing with cross-curvature evaluation for segmentation. Fraz et al. [7] further obtained the blood vessel's skeleton based on detecting the centerline of the blood vessel. It received the direction map with the help of morphological plane slices, and generated the shape of the blood vessel at the same time. The vessel neutral line image is reconstructed through the orientation map and vessel shape, and, finally, the segmented vessel choroid map is obtained. Yang et al. [8] proposed a hybrid method to extract blood vessels based on mathematical morphology and fuzzy clustering. However, these traditional unsupervised-based image processing methods are less robust. Besides, they suffer from poor generalization ability due to customizing artificial features and expert annotations for specified datasets based on prior knowledge.
For supervised machine learning-based methods, its segmentation accuracy is higher than that of unsupervised ones, but with a possibility of overfitting. It has been widely used in retinal vessel segmentation. Staal et al. [9] used the K-nearest neighbor (KNN) algorithm to intercept the first k data to compare further and determine its pixel category, which is essentially a binary classification of each pixel. Soares et al. [10] used a two-dimensional Gabor filter to extract the overall features of retinal images, and then used a naive Bayesian classifier to classify retinal vessels and backgrounds. Osareh et al. [11] first computed feature vectors on a per-pixel basis, and then used a Gaussian mixture model combined with a support vector machine to classify the feature vectors. Khowaja et al. [12] proposed a framework based on a hybrid feature set and hierarchical classification approach. They first employed random forests for classification and evaluating the performance of each feature class for feature selection, and then combined the selected feature set with a hierarchical classification approach for vessel segmentation. Conventional machine learning methods is more suitable for scenarios with a small amount of data. However, deep learning performance will be more prominent if the amount of data increases rapidly.
Deep learning-based methods can automatically learn vessel features from the retinal image and avoid manual participation. Therefore, it has stronger robustness, higher frontal segmentation accuracy and more vital generalization ability. In recent years, deep learning has shown excellent performance in the field of medical image segmentation, and many researchers have conducted research in retinal blood vessel segmentation. Specifically, the proposal of U-Net [13] makes the U shape network a popular framework, and many improved models are proposed for retinal vessel segmentation. For example, Wu et al. [14] proposed a multi-scale network followed network (MS-NFN) to solve the small vessel segmentation problem. Zhuang et al. [15] introduced multiple encoding and decoding structures in their LadderNet, and increased the information flow path via skip connections. Alom et al. [16] proposed a recursive residual convolutional neural network (R2U-net) based on the U-shaped network model, which better retains feature information and achieves the effect of feature reuse. Finally, Li et al. [17] proposed a small U-Net segmentation method IterNet with multiple iterations, which expands the model's depth while considering the segmentation details. Gu et al. [18] proposed CE-Net, which introduces a cascaded upper and lower feature extraction module in the middle layer of the codec. It can ensure the acquisition of complete feature information, and extract deeper feature information. Lin et al. [19] proposed a multi-path high-resolution retinal vessel segmentation method combined with HR-Net. The feature map maintained high resolution in the feature extraction process, and enabled the information interaction between high and low-resolution branches, thus resulting in more accurate probability maps.
Although the models mentioned above achieved good results in retinal blood vessel segmentation, there are still some limitations:
➢ The codec structure transmits and receives information features in a single layer through skip connections, which aggravates the problem of information loss.
➢ The connecting layer of codec cannot thoroughly combine context information, and continuous pooling and convolution further cause a decrease in the recognition rate of the vessel ends.
To alleviate the above problems, this paper proposes a multi-scale integrated context network to improve blood vessel segmentation accuracy further. Aiming at the issues that existing algorithms have an insufficient feature extraction ability, serious feature information loss and low segmentation accuracy for color fundus retinal vascular images, an aggregated multi-scale integrated context model is proposed to further improve the accuracy of vascular segmentation. Its main new feature is that it has a multi-layer feature fusion mechanism, which can fully use the information in different scales. The main contributions of the network are as follows:
➢ A multi-layer feature fusion mechanism is proposed, combining the low-level details of feature maps at different scales with high-level semantic information through full-scale skip connections from the encoding path to the decoding path.
➢ In the encoder, a hybrid stride sampling (HSS) block is constructed to extract deeper semantic information while reducing the dimensionality of features.
➢ A Dense Hybrid Dilated Convolution (DHDC) block is designed between the encoder and the decoder to improve the accurate recovery of blood vessel details by obtaining richer contextual information.
➢ The Squeeze-and-Excitation module with residual connections is introduced into the decoder, and the weight of each scale feature is adaptively adjusted to strengthen the effective channel while suppressing redundant information.
The rest of this paper is organized as follows: Section Ⅱ introduces the proposed method in detail. Section Ⅲ describes the experimental implementation and illustrates the experimental results. Section Ⅳ gives the conclusions of this paper.
The retinal images in the fundus dataset have many samples with poor contrast and high noise. Therefore, proper preprocessing is critical for later training. This paper uses four preprocessing methods, including gray-scale transformation, data standardization, contrast limited adaptive histogram equalization (CLAHE) and gamma correction [18,20,21,22], to process each original retinal blood vessel image.
Figure 1 provides a schematic diagram of the staged processing results of the original color retinal image after gray-scale transformation, contrast-limited adaptive histogram equalization and gamma correction. It can be seen from the figure that the image texture after preprocessing is clear, the edge is prominent, and the detailed information is enhanced.
We use a patch extraction strategy to augment the experimental data and avoid the overfitting problem. There are three patch extraction ways: sequential crop, overlap crop and random crop.
This paper selects random cropping for data augmentation in the training phase. In addition, to maintain the consistency of the training data, we performed the same augmentation processing for the ground truth images manually segmented by experts.
Different from the cropping approach in the training phase, in the testing phase, each image block needs to be re-spliced into a complete image and then binarized to obtain the segmentation result map. All patches must be spliced to restore their separation ratios to the level of the original fundus image. However, if random cropping is used, the time and space complexity of splicing according to the index is extremely high. To avoid this problem, the overlap crop is chosen in the testing phase. We set the stride to 12 based on the trade-off of workstation performance.
As shown in Figure 2, each test image is divided into several patches by the overlapping cropping strategy, and Eq (1) calculates the number of patches for each image:
N_patches_per_img=(⌊img_h−patch_hstride_h⌋+1)×(⌊img_w−patch_wstride_w⌋+1), | (1) |
where img_h and img_w represent the height and width of the test image, patch_h and patch_w represent the height and width of the image block, and stride_h and stride_w represent the step size of horizontal and vertical sliding, respectively. After obtaining the prediction results of overlapping patches, a reconstruction algorithm continuously reconstructs the final average segmentation results (final_avg) by Eq (2).
final_avg=full_profull_sum, | (2) |
where full_pro and full_sum represent the sum of each pixel's prediction probability and extraction frequency in each patch, respectively.
Figure 3 presents the overall framework of the MIC-Net proposed in this paper (the source code is publicly available at https://github.com/Mamdanni/MIC-Net). The network preserves the two-layer end-to-end basic structure of U-Net. First,a hybrid stride sampling (HSS) block is designed in the encoder,which uses full-scale skip connections to replace the single one of U-Net. Second,the interconnection between encoding and decoding paths is redesigned as the dense hybrid dilated convolution (DHDC) block. Finally,the decoder utilized the Squeeze-and-Excitation module with residual connections. Each part is described in detail below.
The max pooling downsampling is mainly used to reduce the image's resolution. However, valuable information is lost while extracting features. To minimize the data loss caused by the downsampling, we designed an HSS block in the encoding process. It performs the downsampling process before two successive convolutions, which can reduce the feature dimension. Meanwhile, it alleviates the loss of helpful information as much as possible and thus extracts deeper semantic information.
As shown in Figure 4, the HSS module is implemented by paralleling a convolution operation with a convolution kernel size of 2 × 2 or 4 × 4, a stride of 2 or 4, and a pooling kernel size of 2 × 2 or 4 × 4. It is executed by max pooling downsampling with stride 2 or 4.
Compared with downsampling by a single max-pooling or a fixed stride convolution, the proposed sampling module could reduce the information loss caused by dimension reduction. Besides, it is worth noting that we also conducted the HSS block before the full-scale skip connection. On the other hand, upsampling uses transposed convolution to achieve the fusion of different scale features.
Skip connections can fuse the high-resolution information from the encoder with the decoder upsampled feature maps, thereby helping to refine the tiny features of the segmentation map. However, though the single skip connection is intuitive and straightforward, the single-layer transmission and reception information features lead to the inability to utilize the full-scale information fully.
However, the feature maps of different scales often contain extra information. Therefore, this paper introduces the full-scale skip connections mechanism, as shown in Figure 5. First, the feature map is re-sampled to a uniform size before downsampling at each layer. Then, we implement full-scale skip connections from the encoding path to the decoding path, thereby achieving a fusion of feature maps at different scales, that is, a complete fusion of low-level details and high-level semantic information.
To improve the receptive field without losing information and cascade convolutional convolutions with different dilation ratios to obtain multi-scale information gain, we developed the DHDC block (shown in Figure 6) between the encoder and the decoder inspired by DenseASPP [23]. A set of atrous convolutions are connected in the form of dense connections, and atrous convolutional layers share information through residual connections. Among them, d represents the expansion rate of the hole convolution. The convolution layers with different expansion rates are interdependent. The feedforward process will not only form a denser feature pyramid, but also increase the receptive field of the convolution kernel to perceive richer contextual information.
During the decoding process, we use twice transposed convolution with a kernel size of 3 × 3 to upsample the feature map. Besides, we introduce squeeze-and-excitation with residual connections (SERC) in the decoder (as shown in Figure 7).
We first fused the feature maps obtained by sampling with that of different scales, and then input the residual SERC block. As a result, the weight of each scale feature is adaptively adjusted. It, thus, strengthens the effective channel and suppresses redundant information. Finally, the channels are adjusted using a 1 × 1 convolution kernel.
Eight standard evaluation metrics for retinal vessel segmentation tasks include Accuracy (Acc), Specificity (Spe), Sensitivity (Sen), Precision (Pre), and F1_Score, Intersection over Union (IoU), Floating point operations (FLOPs) and Parameters (Params) [17,20,22,39].
In addition, we depicted the receiver operating characteristic curve (ROC), which was generated with TP as the ordinate and FP as the abscissa. We also provided the Area under the ROC curve (AUC), which considers the Sen and Spe under different thresholds, and is suitable for measuring retinal vessel segmentation.
We evaluated our proposed method on three public datasets of fundus images, including DRIVE, STARE and CHASE. Figure 8 shows some typical cases from the three datasets.
The DRIVE dataset (https://drive.grand-challenge.org/) contains 40 fundus retinal color images, seven of which are from patients with early diabetic retinopathy, with a resolution of 565 × 584 and stored in JPEG format. The original dataset uses 20 images for training and 20 for testing with masks, and two experts manually annotated the dataset. In this paper, we divide the dataset into a training set, a validation set, and a test set according to the ratio of 18:2:20, and choose the first expert's result as the ground truth. Specifically, 110,000 image patches were obtained based on the original dataset for the later training.
The STARE dataset (http://cecas.clemson.edu/~ahoover/stare/) provides 20 fundus color images with a resolution of 700 × 605. We use 15 of these images for training and five for testing. The original dataset is not divided into a validation set like the DRIVE dataset. Thus, we choose 10% of the training data for validation. The STARE dataset also provides annotated images of two experts, and we chose the first ones as the ground truth. Finally, a total of 130,000 image patches were obtained.
The CHASE dataset (https://blogs.kingston.ac.uk/retinal/chasedb1/) contains 28 color retinal images with a resolution of 996 × 960. It was taken from the left and right eyes of 14 children. We used 20 of these images for training and eight for testing, and a total of 230,000 patches were extracted for training.
All experiments were run on a GPU server with Intel Xeon Silver 4110 CPU, NVIDIA GeForce RTX 2080Ti GPU and 64GB RAM. The development environment is based on CUDA11.2 + cuDNN8.1 + TensorFlow2.6.0 + keras2.6.0, Python 3.7.13 and the Ubuntu 18.04 operating system.
In the training process, we set the maximum number of training epochs to 30, batch_size to 4, and initial learning rate to 0.0001. A binary cross-entropy loss (BCE) was used as the objective function to supervise the model's training process. The DRIVE, STARE and CHASE datasets followed the same data augmentation strategy: randomly extract image patches with a resolution of 48 × 48 from the preprocessed images.
Unlike the training phase, we performed overlap cropping in the original image with a fixed step size. Since these patches had overlapping areas (i.e., each pixel appears multiple times in different patches), we averaged the probability value of each pixel belonging to retinal blood vessels and set the threshold to obtain a binarized prediction map. In addition, we used an early stopping mechanism to prevent the occurrence of overfitting,
The proposed MIC-Net needed to convert the vessel segmentation task into pixel-level classification. Therefore, we chose the BCE loss function to complete the classification task in this paper. Its equation is defined as follows:
Loss=−1NN∑i=1[gilogpi+(1−gi)log(1−pi)] | (3) |
In the above formula, g represents the label value. There are only two possible values of 0 and 1, and p represents the predicted value of the pixel. When g is 0, the first half of the formula equals 0. If you want the loss value to be smaller, p should be as close to 0 as possible; conversely, when g is 1, the second half of the formula is 0. For a minor loss, p should be as close to 1 as possible. Furthermore, the sigmoid activation function is necessary to ensure that the model output is in the range of (0, 1).
We conducted ablation experiments on the DRIVE dataset to verify each module's contribution to the entire model's performance. As can be seen from Table 1, the AUC, Acc, Spe and Sen of MIC-Net reached 98.62, 97.02, 98.80 and 80.02%, respectively. Compared with the baseline model in the DRIVE dataset, the performance of the final model is improved by 0.2, 0.22, 0.23 and 0.31%, respectively.
Methods | AUC (%) | Acc (%) | Spe (%) | Sen (%) |
Baseline | 98.42 | 96.80 | 98.57 | 78.50 |
No FSC | 98.48 | 96.89 | 98.85 | 76.40 |
No DHDC | 98.54 | 96.96 | 98.58 | 78.81 |
No SERC | 98.58 | 96.99 | 98.69 | 79.23 |
MIC-Net | 98.62 | 97.02 | 98.80 | 80.02 |
*Note: For each metric, the bold value indicates that column's best result. |
Figure 9 shows the segmentation results of the MIC-Net model for different combinations of ablation models. It can be observed from the figure that the segmentation results of the complete MIC-Net model proposed by us have more minor errors than other combinations, and are closer to the standard segmentation images, thus verifying the rationality of the model combination.
Generally, the number of parameters of a model is directly proportional to its computational complexity, while fewer model parameters often degrade the performance of the network. Table 2 lists the number of parameters, FLOPs and AUC (on DRIVE dataset) of different methods. Compared with other existing models, the parameters and FLOPs of our proposed MIC-Net are comparable to those of Att-Unet, MultiResUNet, and FCN = 8s (all less than 10M). Besides, the segmentation performance of our proposed method is slightly higher than other models. Therefore, the proposed MIC-Net achieves high segmentation performance with lower computational complexity.
Methods | Parameters (M) | FLOPs (M) | AUC |
SegNet | 29.46 | 58.91 | 0.9294 |
FCN_8s | 9.01 | 18.01 | 0.9410 |
MultiResUNet | 7.26 | 14.55 | 0.9451 |
LinkNet | 11.55 | 23.62 | 0.9492 |
DeepLabV3+ | 41.06 | 82.23 | 0.9575 |
Att-UNet | 8.91 | 17.82 | 0.9793 |
R2U-Net | 17.65 | 51.03 | 0.9804 |
MIC-Net | 9.13 | 18.23 | 0.9862 |
*Note: For each metric, the bold value indicates that column's best result. |
To verify the model's generalization performance, we trained on the DRIVE dataset, and applied the saved weights to testing on the STARE dataset. Figure 10 shows our segmentation results. The generalization visualization experiment shows that the detection of vessels is relatively complete, and the ends and bifurcations can also be completely segmented, which verifies the consistency of the proposed method in different data distributions, and its generalization ability is strong.
In addition, Table 3 lists the generalization performance comparison between the proposed method and other methods. Among them, the method proposed in this paper has obtained the optimal value in the two indicators of AUC and Sen, thus verifying that the proposed method has a good consistency and generalization ability.
Methods | AUC (%) | Acc (%) | Spe (%) | Sen (%) |
Yan et al. [41] | 97.08 | 95.69 | 98.40 | 72.11 |
Jin et al. [42] | 94.45 | 96.90 | 97.59 | 70.00 |
Wu et al. [22] | 96.35 | 95.44 | 97.85 | 73.78 |
Proposed method | 97.70 | 96.44 | 97.51 | 76.02 |
Figure 11 and Table 4 present our proposed method's partial segmentation results on three datasets. As can be seen from the table and figure, the segmentation result of our proposed MIC-Net is very close to the ground truth, which cannot only extract the main vessel from the background, but also correctly segment the vessel edge.
Dataset | AUC (%) | Acc (%) | Spe (%) | Sen (%) | Pre (%) | F1-score (%) | IoU (%) |
DRIVE | 98.62 | 97.02 | 98.80 | 80.02 | 86.32 | 82.20 | 68.32 |
STARE | 98.60 | 97.76 | 98.61 | 87.72 | 93.70 | 85.99 | 69.51 |
CHASE | 98.73 | 97.38 | 98.44 | 81.60 | 77.95 | 79.73 | 64.07 |
In addition, we also give the ROC curves of the three datasets in Figure 12. It can be seen from the figure that the value of AUC is relatively close to 1, which proves the superior performance of our proposed method on retinal vessel segmentation.
Although the proposed MIC-Net can successfully segment blood vessels, some intractable abnormalities still occur. For example, as shown in Figure 13, in the segmentation results of the DRIVE dataset and the CHASE dataset, we found two cases where the optic disc boundary was identified as a blood vessel, which indicates that the specificity of the proposed method needs to be improved.
*Note: For each metric, bold values are the best result in that column.
We further compare the proposed method with existing state-of-the-art (SOTA) methods to verify the effectiveness of the proposed method. Table 5 presents our quantitative experimental results on the DRIVE, STARE and CHASE datasets. All compared methods refer to open-source codes on GitHub. The experiment's environment configuration and the specific parameter settings, including batch size, epoch, learning rate, etc., are consistent with the settings of our proposed method in this paper during training and testing.
Methods | Year | DRIVE(%) | STARE(%) | CHASE(%) | |||||||||||
AUC | Acc | Spe | Sen | AUC | Acc | Spe | Sen | AUC | Acc | Spe | Sen | ||||
Azzopardi [24] | 2015 | 96.14 | 94.42 | 97.04 | 76.55 | 95.63 | 94.97 | 97.01 | 77.16 | 94.87 | 93.87 | 95.87 | 75.85 | ||
Li et al. [25] | 2015 | 97.38 | 95.27 | 98.16 | 75.69 | 98.79 | 96.28 | 98.44 | 77.26 | 97.16 | 95.81 | 97.93 | 75.07 | ||
Liskowski [26] | 2016 | 97.20 | 94.95 | 97.68 | 77.63 | 97.85 | 95.66 | 97.54 | 78.67 | - | - | - | - | ||
Fu et al. [27] | 2016 | - | 95.23 | - | 76.03 | - | 95.85 | - | 74.12 | - | 94.89 | - | 71.30 | ||
Dasgupta [28] | 2016 | 97.44 | 95.33 | 98.01 | 76.91 | - | - | - | - | - | - | - | - | ||
Chen et al. [29] | 2017 | 95.16 | 94.53 | 97.35 | 74.26 | 95.57 | 94.49 | 96.96 | 72.95 | - | - | - | - | ||
Yan et al. [30] | 2018 | 97.52 | 95.42 | 98.18 | 76.53 | 98.01 | 96.12 | 98.46 | 75.81 | 97.81 | 96.10 | 98.09 | 76.33 | ||
Wu et al. [14] | 2018 | 98.07 | 95.67 | 98.19 | 78.44 | - | - | - | - | 98.25 | 96.37 | 98.47 | 75.38 | ||
Yan et al. [31] | 2019 | 97.50 | 95.38 | 98.20 | 76.31 | 98.33 | 96.38 | 98.57 | 77.35 | 97.76 | 96.07 | 98.06 | 76.41 | ||
Jin et al. [20] | 2019 | 98.02 | 95.66 | 98.00 | 79.63 | 98.32 | 96.41 | 98.78 | 75.95 | 98.04 | 96.10 | 97.52 | 81.55 | ||
Wang et al. [32] | 2020 | 98.23 | 95.81 | 98.13 | 79.91 | 98.81 | 96.73 | 98.44 | 81.86 | - | - | - | - | ||
Li et al. [17] | 2020 | 98.16 | 95.73 | 98.38 | 77.35 | 98.81 | 97.01 | 98.86 | 77.15 | 98.51 | 96.55 | 98.23 | 79.70 | ||
Shi et al. [33] | 2021 | - | 96.76 | 98.26 | 80.65 | - | 97.32 | 98.66 | 82.90 | - | 97.31 | 98.89 | 75.04 | ||
Guo et al. [34] | 2021 | 98.53 | 96.67 | 98.17 | 82.21 | 98.97 | 97.24 | 98.59 | 82.10 | 98.69 | 96.97 | 98.45 | 81.89 | ||
Xu et al. [35] | 2015 | 96.70 | 96.30 | 98.23 | 87.45 | - | - | - | - | 96.77 | 96.94 | 97.94 | 89.16 | ||
Zhang et al. [36] | 2022 | 88.95 | 97.01 | 97.99 | 77.19 | 83.91 | 96.91 | 99.11 | 69.12 | 91.42 | 98.11 | 99.81 | 85.06 | ||
Deng et al. [37] | 2022 | 97.93 | 95.39 | 97.12 | 83.68 | 98.55 | 96.43 | 97.79 | 84.35 | 98.06 | 95.87 | 96.93 | 85.43 | ||
Our MIC-Net | 2022 | 98.62 | 97.02 | 98.80 | 80.02 | 98.60 | 97.76 | 98.61 | 87.72 | 98.73 | 97.38 | 98.44 | 81.60 |
In the comparative experiment, the statistical method of t-test is also used to verify whether the proposed method is significantly different from other methods on the accuracy. All statistical hypothesis tests are based on the representative metrics of Acc.
It can be seen that the overall performance of our proposed method achieves significant improvements on all performance metrics, outperforming existing SOTA methods on the three datasets. Besides, regarding AUC metrics, our AUC is 0.46% higher than the second on the DRIVE dataset, 0.52% higher than the second on the STARE dataset and 0.41% higher than the second on the CHASE dataset.
However, compared with the experiments of existing methods, the proposed module has improved the performance of retinal vessel detection. Still, Spe and Sen performances on the three datasets are not outstanding, which indicates that, although the performance improvement of our proposed method is achieved to a certain extent, there is still a significant error on FP and FN.
Figure 13 compares the visualization results of several SOTA methods on three datasets. Our method achieves satisfactory segmentation results from the locally zoom-in images. Compared with other improved approaches, such as scSEU-Net [38] and R2U-Net [16], our proposed MIC-Net can detect retinal vessels more correctly and reduce misclassified retinal vessel pixels. In addition, better recognition is achieved for tiny vessels and edge regions.
This paper proposes an end-to-end fundus retinal vessel segmentation network called MIC-Net. This multi-layer feature fusion mechanism can fully utilize the information on different scales to improve information flow. First, the HSS block we designed on the encoder side can minimize the loss of helpful information caused by the downsampling operation. Second, the DHDC block between the encoder and decoder can perceive richer contextual information without sacrificing feature resolution. Third, the SERC module at the decoder can strengthen the effective channel, while suppressing redundant information.
The experimental results show that the performance of our proposed method on the DRIVE, STARE and CHASE datasets could achieve comparable segmentation results to existing SOTA methods on retinal vessel segmentation. Thus, it has significant application prospects in the early screening of diabetic retinopathy. Nevertheless, the proposed MIC-Net still has some limitations for segmenting tiny blood vessels that cannot be effectively distinguished by direct observation of the human eye. Therefore, our future work will focus on the cascaded semantic segmentation framework for segmenting small blood vessels.
This work was supported by the National Nature Science Foundation (No. 61741106, 61701178).
We declare that there are no conflicts of interest.
[1] | Agénor PR, Pereira da Silva LA (2018) Financial spillovers, spillbacks, and the scope for international macroprudential policy coordination. Bank Int. Settlements BIS Paper. |
[2] | Bank of Uganda (2018) State of the Economy June 2018. Bank of Uganda, Kampala. |
[3] | Bank of Uganda (2011) Bank of Uganda Annual Report 2010/11. Bank of Uganda, Kampala. |
[4] | Bank of Uganda (n.d.) Welcome To Bank of Uganda[WWW Document]. Bank Uganda. |
[5] | Baruník J, Kocenda E, Vácha L (2015) Volatility spillovers across petroleum markets. Energy J 36. |
[6] |
Baruník J, Kočenda E, Vácha L (2017) Asymmetric volatility connectedness on the forex market. J Int Money Financ 77: 39-56. doi: 10.1016/j.jimonfin.2017.06.003
![]() |
[7] | Becketti S, Sellon GHJ (1989) Has financial market volatility increased? Econ Rev Reserv Bank Kansas City 74: 17. |
[8] |
Bruno V, Shin HS (2015) Capital flows and the risk-taking channel of monetary policy. J Monet Econ 71: 119-132. doi: 10.1016/j.jmoneco.2014.11.011
![]() |
[9] |
Canova F (2005) The transmission of US shocks to Latin America. J Appl Econom 20: 229-251. doi: 10.1002/jae.837
![]() |
[10] | Central Bank of Kenya (n.d.) Central Bank of Kenya[WWW Document]. Cent. Bank Kenya. |
[11] | Central Bank of Kenya (n.d) Inflation Rates[WWW Document]. Inflat. Rates. |
[12] |
Dedola L, Rivolta G, Stracca L (2017) If the Fed sneezes, who catches a cold? J Int Econ 108: S23-S41. doi: 10.1016/j.jinteco.2017.01.002
![]() |
[13] | Dickey DA, Fuller WA (1979) Distribution of the estimators for autoregressive time series with a unit root. J Am Stat Assoc 74: 427-431. |
[14] |
Diebold FX, Yilmaz K (2012) Better to give than to receive: Predictive directional measurement of volatility spillovers. Int J Forecast 28: 57-66. doi: 10.1016/j.ijforecast.2011.02.006
![]() |
[15] |
Diebold FX, Yilmaz K (2009) Measuring Financial Asset Return and Volatility Spillovers, With Application To Global Equity Markets. Econ J 119: 158-171. doi: 10.1111/j.1468-0297.2008.02208.x
![]() |
[16] |
Diebold FX, Yılmaz K (2014) On the network topology of variance decompositions: Measuring the connectedness of financial firms. J Econom 182: 119-134. doi: 10.1016/j.jeconom.2014.04.012
![]() |
[17] | Drummond P, Aisen A, Alper CE, et al. (2015) Toward a monetary union in the East African Community: asymmetric shocks, exchange rates, and risk-sharing mechanisms. International Monetary Fund, Washington, D.C. |
[18] |
Georgiadis G (2016) Determinants of global spillovers from US monetary policy. J Int Money Financ 67: 41-61. doi: 10.1016/j.jimonfin.2015.06.010
![]() |
[19] | Gupta P, Masetti O, Rosenblatt D (2017) Should emerging markets worry about U.S. monetary policy announcements? (No. WPS 8100), Policy Research working paper no. WPS 8100. Washington, D.C. |
[20] | Hofmann B, Takats E (2015) International monetary spillovers. BIS Q Rev 105. |
[21] |
Iacoviello M, Navarro G (2018) Foreign effects of higher U.S. interest rates. J Int Money Financ 95: 232-250. doi: 10.1016/j.jimonfin.2018.06.012
![]() |
[22] | International Monetary Fund (2018) International Financial Statistics (IFS)[WWW Document]. IMF Data. |
[23] | International Monetary Fund (2003) Fund Assistance for Countries Facing Exogenous Shocks, International Monetary Fund, Policy Papers. Washington, DC. |
[24] | Kasekende L, Atingi-Ego M, Sebudde R (2004) The African Growth Experience: Uganda Country Case Study, AERC. |
[25] | Katusiime L (2019) Investigating Spillover Effects between Foreign Exchange Rate Volatility and Commodity Price Volatility in Uganda. Economies 7: 1. |
[26] |
Koop G, Pesaran MH, Potter SM (1996) Impulse response analysis in nonlinear multivariate models. J. Econom 74: 119-147. doi: 10.1016/0304-4076(95)01753-4
![]() |
[27] | Kose MA, Lakatos C, Ohnsorge FL, et al. (2017) The global role of the U.S. economy: linkages, policies and spillovers (No. WPS7962), Policy Research Working Paper. Washington, D.C. |
[28] |
Kwiatkowski D, Phillips PCB, Schmidt P, et al. (1992) Testing the null hypothesis of stationarity against the alternative of a unit root: How sure are we that economic time series have a unit root? J. Econom 54: 159-178. doi: 10.1016/0304-4076(92)90104-Y
![]() |
[29] |
Milunovich G, Thorp S (2006) Valuing volatility spillovers. Glob Financ J 17: 1-22. doi: 10.1016/j.gfj.2006.06.007
![]() |
[30] | Miranda-Agrippino S, Rey H (2015) World asset markets and the global financial cycle, Cambridge, MA: National Bureau of Economic Research. |
[31] | Palanska T (2020) Measurement of Volatility Spillovers and Asymmetric Connectedness on Commodity and Equity Markets. Czech J Econ Financ (Finance a uver) 70: 42-69. |
[32] | Parliamentary Budget Office (2018) Half Year Economic & Fiscal Update, August 2018[WWW Document]. |
[33] | Perry G (2009) Causes and Consequences of High Volatility in Developing Countries, in: Beyond Lending: How Multilateral Banks Can Help Developing Countries Manage Volatility. Center for Global Development, Washington, D.C., 14. |
[34] |
Pesaran HH, Shin Y (1998) Generalized impulse response analysis in linear multivariate models. Econ Lett 58: 17-29. doi: 10.1016/S0165-1765(97)00214-0
![]() |
[35] |
Phillips PCB, Perron P (1988) Testing for a unit root in time series regression. Biometrika 75: 335-346. doi: 10.1093/biomet/75.2.335
![]() |
[36] | Prasad ES, Rogoff K, Wei SJ, et al. (2003) Effects of Financial Globalization on Developing Countries: Some Empirical Evidence, Occasional Paper 220. International Monetary Fund, Washington D. C. |
[37] |
Raddatz C (2007) Are external shocks responsible for the instability of output in low-income countries? J Dev Econ 84: 155-187. doi: 10.1016/j.jdeveco.2006.11.001
![]() |
[38] | Ramirez G, Drummond P (2009) Spillover Effects and the East African Community: Explaining the Slowdown and the Recovery. International Monetary Fund, Washington, D.C. |
[39] | Rey H (2015) Dilemma not trilemma: the global financial cycle and monetary policy independence. National Bureau of Economic Research. |
[40] | Tumusiime-Mutebile E (2012) Macroeconomic management in turbulent times. |
[41] | United Nations (2017) Emerging Economies and the Monetary Tightening Path in the United States. Development Issues No. 12. |
[42] | Varangis P, Varma S, DePlaa A, et al. (2004) Exogenous shocks in low income countries: economic policy issues and the role of the international community, Background Paper prepared for the Report: Managing the Debt Risk of Exogenous Shocks in Low Income Countries. |
[43] | Zheng S, Silwal A, Newhouse D (2019) Here are the top 10 Sub-Saharan African countries that have reduced poverty the most[WWW Document]. World Bank Data Blog. Available from: https://blogs.worldbank.org/opendata/here-are-top-10-sub-saharan-african-countries-have-reduced-poverty-most (accessed 7.21.21). |
1. | Caixia Zheng, Huican Li, Yingying Ge, Yanlin He, Yugen Yi, Meili Zhu, Hui Sun, Jun Kong, Retinal vessel segmentation based on multi-scale feature and style transfer, 2023, 21, 1551-0018, 49, 10.3934/mbe.2024003 | |
2. | Mohd Zulfaezal Che Azemin, Mohd Izzuddin Mohd Tamrin, Firdaus Yusof, Adzura Salam, Nur Syazriena Ghazali, High-resolution retinal imaging system: diagnostic accuracy and usability, 2025, 6, 2735-0584, 69, 10.31436/ijohs.v6i1.357 |
Methods | AUC (%) | Acc (%) | Spe (%) | Sen (%) |
Baseline | 98.42 | 96.80 | 98.57 | 78.50 |
No FSC | 98.48 | 96.89 | 98.85 | 76.40 |
No DHDC | 98.54 | 96.96 | 98.58 | 78.81 |
No SERC | 98.58 | 96.99 | 98.69 | 79.23 |
MIC-Net | 98.62 | 97.02 | 98.80 | 80.02 |
*Note: For each metric, the bold value indicates that column's best result. |
Methods | Parameters (M) | FLOPs (M) | AUC |
SegNet | 29.46 | 58.91 | 0.9294 |
FCN_8s | 9.01 | 18.01 | 0.9410 |
MultiResUNet | 7.26 | 14.55 | 0.9451 |
LinkNet | 11.55 | 23.62 | 0.9492 |
DeepLabV3+ | 41.06 | 82.23 | 0.9575 |
Att-UNet | 8.91 | 17.82 | 0.9793 |
R2U-Net | 17.65 | 51.03 | 0.9804 |
MIC-Net | 9.13 | 18.23 | 0.9862 |
*Note: For each metric, the bold value indicates that column's best result. |
Dataset | AUC (%) | Acc (%) | Spe (%) | Sen (%) | Pre (%) | F1-score (%) | IoU (%) |
DRIVE | 98.62 | 97.02 | 98.80 | 80.02 | 86.32 | 82.20 | 68.32 |
STARE | 98.60 | 97.76 | 98.61 | 87.72 | 93.70 | 85.99 | 69.51 |
CHASE | 98.73 | 97.38 | 98.44 | 81.60 | 77.95 | 79.73 | 64.07 |
Methods | Year | DRIVE(%) | STARE(%) | CHASE(%) | |||||||||||
AUC | Acc | Spe | Sen | AUC | Acc | Spe | Sen | AUC | Acc | Spe | Sen | ||||
Azzopardi [24] | 2015 | 96.14 | 94.42 | 97.04 | 76.55 | 95.63 | 94.97 | 97.01 | 77.16 | 94.87 | 93.87 | 95.87 | 75.85 | ||
Li et al. [25] | 2015 | 97.38 | 95.27 | 98.16 | 75.69 | 98.79 | 96.28 | 98.44 | 77.26 | 97.16 | 95.81 | 97.93 | 75.07 | ||
Liskowski [26] | 2016 | 97.20 | 94.95 | 97.68 | 77.63 | 97.85 | 95.66 | 97.54 | 78.67 | - | - | - | - | ||
Fu et al. [27] | 2016 | - | 95.23 | - | 76.03 | - | 95.85 | - | 74.12 | - | 94.89 | - | 71.30 | ||
Dasgupta [28] | 2016 | 97.44 | 95.33 | 98.01 | 76.91 | - | - | - | - | - | - | - | - | ||
Chen et al. [29] | 2017 | 95.16 | 94.53 | 97.35 | 74.26 | 95.57 | 94.49 | 96.96 | 72.95 | - | - | - | - | ||
Yan et al. [30] | 2018 | 97.52 | 95.42 | 98.18 | 76.53 | 98.01 | 96.12 | 98.46 | 75.81 | 97.81 | 96.10 | 98.09 | 76.33 | ||
Wu et al. [14] | 2018 | 98.07 | 95.67 | 98.19 | 78.44 | - | - | - | - | 98.25 | 96.37 | 98.47 | 75.38 | ||
Yan et al. [31] | 2019 | 97.50 | 95.38 | 98.20 | 76.31 | 98.33 | 96.38 | 98.57 | 77.35 | 97.76 | 96.07 | 98.06 | 76.41 | ||
Jin et al. [20] | 2019 | 98.02 | 95.66 | 98.00 | 79.63 | 98.32 | 96.41 | 98.78 | 75.95 | 98.04 | 96.10 | 97.52 | 81.55 | ||
Wang et al. [32] | 2020 | 98.23 | 95.81 | 98.13 | 79.91 | 98.81 | 96.73 | 98.44 | 81.86 | - | - | - | - | ||
Li et al. [17] | 2020 | 98.16 | 95.73 | 98.38 | 77.35 | 98.81 | 97.01 | 98.86 | 77.15 | 98.51 | 96.55 | 98.23 | 79.70 | ||
Shi et al. [33] | 2021 | - | 96.76 | 98.26 | 80.65 | - | 97.32 | 98.66 | 82.90 | - | 97.31 | 98.89 | 75.04 | ||
Guo et al. [34] | 2021 | 98.53 | 96.67 | 98.17 | 82.21 | 98.97 | 97.24 | 98.59 | 82.10 | 98.69 | 96.97 | 98.45 | 81.89 | ||
Xu et al. [35] | 2015 | 96.70 | 96.30 | 98.23 | 87.45 | - | - | - | - | 96.77 | 96.94 | 97.94 | 89.16 | ||
Zhang et al. [36] | 2022 | 88.95 | 97.01 | 97.99 | 77.19 | 83.91 | 96.91 | 99.11 | 69.12 | 91.42 | 98.11 | 99.81 | 85.06 | ||
Deng et al. [37] | 2022 | 97.93 | 95.39 | 97.12 | 83.68 | 98.55 | 96.43 | 97.79 | 84.35 | 98.06 | 95.87 | 96.93 | 85.43 | ||
Our MIC-Net | 2022 | 98.62 | 97.02 | 98.80 | 80.02 | 98.60 | 97.76 | 98.61 | 87.72 | 98.73 | 97.38 | 98.44 | 81.60 |
Methods | AUC (%) | Acc (%) | Spe (%) | Sen (%) |
Baseline | 98.42 | 96.80 | 98.57 | 78.50 |
No FSC | 98.48 | 96.89 | 98.85 | 76.40 |
No DHDC | 98.54 | 96.96 | 98.58 | 78.81 |
No SERC | 98.58 | 96.99 | 98.69 | 79.23 |
MIC-Net | 98.62 | 97.02 | 98.80 | 80.02 |
*Note: For each metric, the bold value indicates that column's best result. |
Methods | Parameters (M) | FLOPs (M) | AUC |
SegNet | 29.46 | 58.91 | 0.9294 |
FCN_8s | 9.01 | 18.01 | 0.9410 |
MultiResUNet | 7.26 | 14.55 | 0.9451 |
LinkNet | 11.55 | 23.62 | 0.9492 |
DeepLabV3+ | 41.06 | 82.23 | 0.9575 |
Att-UNet | 8.91 | 17.82 | 0.9793 |
R2U-Net | 17.65 | 51.03 | 0.9804 |
MIC-Net | 9.13 | 18.23 | 0.9862 |
*Note: For each metric, the bold value indicates that column's best result. |
Methods | AUC (%) | Acc (%) | Spe (%) | Sen (%) |
Yan et al. [41] | 97.08 | 95.69 | 98.40 | 72.11 |
Jin et al. [42] | 94.45 | 96.90 | 97.59 | 70.00 |
Wu et al. [22] | 96.35 | 95.44 | 97.85 | 73.78 |
Proposed method | 97.70 | 96.44 | 97.51 | 76.02 |
Dataset | AUC (%) | Acc (%) | Spe (%) | Sen (%) | Pre (%) | F1-score (%) | IoU (%) |
DRIVE | 98.62 | 97.02 | 98.80 | 80.02 | 86.32 | 82.20 | 68.32 |
STARE | 98.60 | 97.76 | 98.61 | 87.72 | 93.70 | 85.99 | 69.51 |
CHASE | 98.73 | 97.38 | 98.44 | 81.60 | 77.95 | 79.73 | 64.07 |
Methods | Year | DRIVE(%) | STARE(%) | CHASE(%) | |||||||||||
AUC | Acc | Spe | Sen | AUC | Acc | Spe | Sen | AUC | Acc | Spe | Sen | ||||
Azzopardi [24] | 2015 | 96.14 | 94.42 | 97.04 | 76.55 | 95.63 | 94.97 | 97.01 | 77.16 | 94.87 | 93.87 | 95.87 | 75.85 | ||
Li et al. [25] | 2015 | 97.38 | 95.27 | 98.16 | 75.69 | 98.79 | 96.28 | 98.44 | 77.26 | 97.16 | 95.81 | 97.93 | 75.07 | ||
Liskowski [26] | 2016 | 97.20 | 94.95 | 97.68 | 77.63 | 97.85 | 95.66 | 97.54 | 78.67 | - | - | - | - | ||
Fu et al. [27] | 2016 | - | 95.23 | - | 76.03 | - | 95.85 | - | 74.12 | - | 94.89 | - | 71.30 | ||
Dasgupta [28] | 2016 | 97.44 | 95.33 | 98.01 | 76.91 | - | - | - | - | - | - | - | - | ||
Chen et al. [29] | 2017 | 95.16 | 94.53 | 97.35 | 74.26 | 95.57 | 94.49 | 96.96 | 72.95 | - | - | - | - | ||
Yan et al. [30] | 2018 | 97.52 | 95.42 | 98.18 | 76.53 | 98.01 | 96.12 | 98.46 | 75.81 | 97.81 | 96.10 | 98.09 | 76.33 | ||
Wu et al. [14] | 2018 | 98.07 | 95.67 | 98.19 | 78.44 | - | - | - | - | 98.25 | 96.37 | 98.47 | 75.38 | ||
Yan et al. [31] | 2019 | 97.50 | 95.38 | 98.20 | 76.31 | 98.33 | 96.38 | 98.57 | 77.35 | 97.76 | 96.07 | 98.06 | 76.41 | ||
Jin et al. [20] | 2019 | 98.02 | 95.66 | 98.00 | 79.63 | 98.32 | 96.41 | 98.78 | 75.95 | 98.04 | 96.10 | 97.52 | 81.55 | ||
Wang et al. [32] | 2020 | 98.23 | 95.81 | 98.13 | 79.91 | 98.81 | 96.73 | 98.44 | 81.86 | - | - | - | - | ||
Li et al. [17] | 2020 | 98.16 | 95.73 | 98.38 | 77.35 | 98.81 | 97.01 | 98.86 | 77.15 | 98.51 | 96.55 | 98.23 | 79.70 | ||
Shi et al. [33] | 2021 | - | 96.76 | 98.26 | 80.65 | - | 97.32 | 98.66 | 82.90 | - | 97.31 | 98.89 | 75.04 | ||
Guo et al. [34] | 2021 | 98.53 | 96.67 | 98.17 | 82.21 | 98.97 | 97.24 | 98.59 | 82.10 | 98.69 | 96.97 | 98.45 | 81.89 | ||
Xu et al. [35] | 2015 | 96.70 | 96.30 | 98.23 | 87.45 | - | - | - | - | 96.77 | 96.94 | 97.94 | 89.16 | ||
Zhang et al. [36] | 2022 | 88.95 | 97.01 | 97.99 | 77.19 | 83.91 | 96.91 | 99.11 | 69.12 | 91.42 | 98.11 | 99.81 | 85.06 | ||
Deng et al. [37] | 2022 | 97.93 | 95.39 | 97.12 | 83.68 | 98.55 | 96.43 | 97.79 | 84.35 | 98.06 | 95.87 | 96.93 | 85.43 | ||
Our MIC-Net | 2022 | 98.62 | 97.02 | 98.80 | 80.02 | 98.60 | 97.76 | 98.61 | 87.72 | 98.73 | 97.38 | 98.44 | 81.60 |