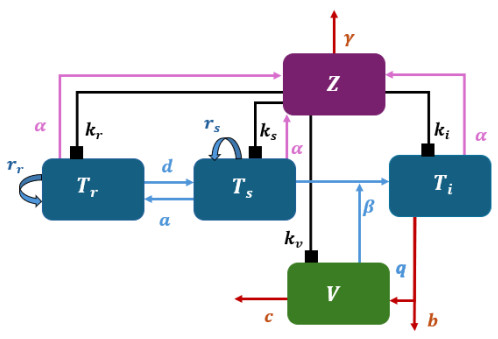
COVID-19 has generated an unprecedented shock to the global economy causing both the decrease in demand and supply. The purpose of this paper is to simulate the effect of COVID-19 on firms' financial statements in Brescia. The shocked information is then fed into two bankruptcy models with the aim of providing an up-to-date picture of firms' economic health in one of the most prosperous industrial areas in Italy and Europe.
Citation: Alberto Bernardi, Daniela Bragoli, Davide Fedreghini, Tommaso Ganugi, Giovanni Marseguerra. COVID-19 and firms' financial health in Brescia: a simulation with Logistic regression and neural networks[J]. National Accounting Review, 2021, 3(3): 293-309. doi: 10.3934/NAR.2021015
[1] | G. V. R. K. Vithanage, Hsiu-Chuan Wei, Sophia R-J Jang . Bistability in a model of tumor-immune system interactions with an oncolytic viral therapy. Mathematical Biosciences and Engineering, 2022, 19(2): 1559-1587. doi: 10.3934/mbe.2022072 |
[2] | Lu Gao, Yuanshun Tan, Jin Yang, Changcheng Xiang . Dynamic analysis of an age structure model for oncolytic virus therapy. Mathematical Biosciences and Engineering, 2023, 20(2): 3301-3323. doi: 10.3934/mbe.2023155 |
[3] | Cameron Browne . Immune response in virus model structured by cell infection-age. Mathematical Biosciences and Engineering, 2016, 13(5): 887-909. doi: 10.3934/mbe.2016022 |
[4] | Khaphetsi Joseph Mahasa, Rachid Ouifki, Amina Eladdadi, Lisette de Pillis . A combination therapy of oncolytic viruses and chimeric antigen receptor T cells: a mathematical model proof-of-concept. Mathematical Biosciences and Engineering, 2022, 19(5): 4429-4457. doi: 10.3934/mbe.2022205 |
[5] | Nada Almuallem, Dumitru Trucu, Raluca Eftimie . Oncolytic viral therapies and the delicate balance between virus-macrophage-tumour interactions: A mathematical approach. Mathematical Biosciences and Engineering, 2021, 18(1): 764-799. doi: 10.3934/mbe.2021041 |
[6] | Taeyong Lee, Adrianne L. Jenner, Peter S. Kim, Jeehyun Lee . Application of control theory in a delayed-infection and immune-evading oncolytic virotherapy. Mathematical Biosciences and Engineering, 2020, 17(3): 2361-2383. doi: 10.3934/mbe.2020126 |
[7] | Tinevimbo Shiri, Winston Garira, Senelani D. Musekwa . A two-strain HIV-1 mathematical model to assess the effects of chemotherapy on disease parameters. Mathematical Biosciences and Engineering, 2005, 2(4): 811-832. doi: 10.3934/mbe.2005.2.811 |
[8] | Jinhu Xu, Yicang Zhou . Bifurcation analysis of HIV-1 infection model with cell-to-cell transmission and immune response delay. Mathematical Biosciences and Engineering, 2016, 13(2): 343-367. doi: 10.3934/mbe.2015006 |
[9] | Zizi Wang, Zhiming Guo, Hal Smith . A mathematical model of oncolytic virotherapy with time delay. Mathematical Biosciences and Engineering, 2019, 16(4): 1836-1860. doi: 10.3934/mbe.2019089 |
[10] | Cassidy K. Buhler, Rebecca S. Terry, Kathryn G. Link, Frederick R. Adler . Do mechanisms matter? Comparing cancer treatment strategies across mathematical models and outcome objectives. Mathematical Biosciences and Engineering, 2021, 18(5): 6305-6327. doi: 10.3934/mbe.2021315 |
COVID-19 has generated an unprecedented shock to the global economy causing both the decrease in demand and supply. The purpose of this paper is to simulate the effect of COVID-19 on firms' financial statements in Brescia. The shocked information is then fed into two bankruptcy models with the aim of providing an up-to-date picture of firms' economic health in one of the most prosperous industrial areas in Italy and Europe.
Malignant tumors, recognized as one of the most lethal diseases globally, arise from uncontrolled cell growth [1,2]. Traditional treatment approaches encompass surgery, chemotherapy, and radiotherapy. An emerging strategy in the field is cancer immunotherapy, a therapeutic approach that entails stimulating and leveraging the immune system to combat the cancer [3].
Certain viruses possess the remarkable ability to precisely select and invade cancer cells while leaving normal cells unharmed. These specialized viruses are referred to as oncolytic viruses (OVs) and are pivotal in oncoviral therapy (OVT). OVT has demonstrated significant anti-tumor effects in diverse mouse models and clinical studies [3]. Apart from their oncolytic potential to direct towards cancer cells and destroy them, OVs can be engineered to incorporate specific genes or cytokines, enhancing their ability to activate T cell responses [3].
Cancer therapies encounter several formidable challenges, with drug resistance emerging as a major concern. Drug resistance is a frequent outcome in cancer treatment and is categorized as acquired or primary (inherent) resistance. Inherent resistance is intrinsic, whereas acquired resistance develops after exposure to drugs. In [4], Noll et al. presented significant confirmation of inherent resistance in the measles vaccine virus (MeV) against NCI-60 solid cancer cells. The authors concluded that inherent resistance in oncolytic viral therapy may be linked with epigenetic and genetic alterations following malignant transition.
While several computational and mathematical models have been devised to investigate chemotherapy resistance [5,6,7,8,9,10], there has been relatively less exploration of models focused on other therapeutic resistances. Notably, studies such as [10,11] delve into acquired/primary resistance in targeted therapy, while [12] utilizes spatial probabilistic models to investigate resistance in oncolytic virotherapy. In their investigation, Bhatt et al. [12] developed probabilistic, cell-based models to explore the dynamics of resistance in OVT. The model encompasses four distinct populations: normal healthy cells, infected cells, infection-sensitive cancer cells, and infection-resistant cancer cells. The authors systematically explored the model's outcomes, analyzing their dependence on various parameter values and assumptions. Key aspects investigated include the impact of the ratio of the death rate of infected cells to the viral spread rate on cancer eradication, the influence of the timing of virotherapy, and the effects of parameters such as the production rate of resistant cancer cells, virus diffusion distance, and the probability of virus infection.
The study [12] utilized simulations based on three different types of cell-based models: 2D lattice, 3D lattice, and Voronoi, demonstrating that the results exhibit qualitative similarity with some variations. Furthermore, the authors examined the scenario of allowing normal healthy cells to become infected. They found that while this approach may increase the likelihood of complete cancer elimination, representing a successful outcome, it comes with the risk of elevating the population of resistant cancer cells, potentially leading to therapy failure. The study suggests that improved therapeutic efficacy can be achieved by sensitizing healthy normal cells to infection [12].
Despite being relatively unexplored, resistance to OVT significantly limits therapeutic effectiveness. The article [13] extensively explores a spectrum of mechanisms contributing to resistance in OVT, shedding light on the multifaceted challenges faced in utilizing viruses as therapeutic agents against cancer. For example, interferon-mediated resistance stands out as a formidable obstacle, as host cells activate interferon pathways to establish an anti-viral state. In addition, epigenetic modifications, encompassing changes in DNA methylation and histone modifications, have been implicated in altering the gene expression landscape, potentially limiting the permissiveness of host cells to oncolytic viruses. The hypoxic microenvironment within tumors presents another layer of complexity, with low oxygen concentrations inhibiting viral replication. Furthermore, virus-entry barriers pose challenges by hindering efficient entry into target cells, while spatiotemporal restrictions to viral spread highlight the intricate dynamics within tumors that may limit the uniform distribution of therapeutic viruses. These identified mechanisms underscore the need for a nuanced understanding to develop strategies that can effectively overcome OVT resistance and enhance the therapeutic potential of oncolytic viral therapies in the realm of cancer treatment.
The viral cycle in oncolytic viral therapy typically consists of several stages, including attachment and entry, uncoating and gene expression, and replication and assembly [14]. Once the virus attaches to specific receptors on the surface of cancer cells, it enters the cancer cell, either through the cell membrane or via receptor-mediated endocytosis. Inside the cancer cell, the viral genome is released from its protein coat. The virus then utilizes the cellular machinery to express its genes. The viral genome is replicated, and new viral components are synthesized within the host cell. The components are assembled to form new viral particles. These processes take time to complete [14].
To our knowledge, the study [15] represents a pioneering use of deterministic models to explore OVT resistance, although it adopts a generic approach without considering specific mechanisms of resistance. In [15], the authors developed systems of differential equations to study the effects of OVT resistance, with the time delay associated with the infection process. The research presented conditions for the existence of equilibria and their stability, deriving critical delays for Hopf bifurcations. The Hopf bifurcation marks the point at which a stable equilibrium in the model becomes unstable, leading to the emergence of periodic oscillations. This can correspond to the transition from steady tumor growth to oscillatory tumor dynamics, where tumor size fluctuates over time. Understanding the occurrence of Hopf bifurcation in cancer models can provide insights into how tumors respond to different treatment strategies.
The study [15] concluded that if tumor cells resistant to treatment cannot revert naturally to sensitive cells or through other mechanisms, every cancer cell will inevitably develop resistance to the OVT, even when a time delay in the viral infection is present. Additionally, the authors proved that the delay in infection process cannot destabilize those equilibria with no viruses. The numerical investigations conducted by the authors showed that the existence of resistant cancer cells, along with time delay in the virus infection, substantially amplifies the number of tumor cells, particularly during periods of instability in the population interaction, i.e., when the interaction is not at equilibrium.
The immune system, a complex network of organs, cells, and proteins, serves as the body's defense against infections [1]. Malignant tumors often express antigens that can trigger an immune response, creating an anti-tumor effect [1, 2]. According to the Tumor Immuno-Surveillance Hypothesis, the immune system can potentially inhibit the growth of small tumors, eliminating them before they become clinically evident [1, 2]. Building upon the work in [15], this study introduces effector cells into the tumor-virus interaction. These immune cells, activated by tumor cells [16,17,18], have the ability to eliminate both tumor cells and viruses in oncolytic viral therapy. The primary objective is to investigate the impact of immune cells on the dynamics of the tumor-virus interaction. In addition, as the immune activation process in response to the presence of the tumor is not instantaneous, we introduce a time delay in the activation of immune cells. This results in a model formulated as delay differential equations (DDEs). In contrast to [15], where delay is incorporated into the viral cycle, this study considers a delay in immune activation, as it takes days or even weeks for the adaptive immune response to become established. We derive critical delays for which Hopf bifurcations occur at certain boundary equilibria, where one or more populations become extinct.
Contrary to the findings in [15], the introduction of immune cells in the tumor-virus interaction prevents all tumor cells from becoming resistant when there is no conversion from resistance to sensitivity, provided that the proliferation rate of immune cells exceeds their death rate. However, if the immune cells have a smaller recruitment rate, then eventually all tumor cells will become resistant. In [15], the model exhibits a unique positive equilibrium, whereas the model with the addition of immune cells into the interaction may have three positive equilibria. This new phenomenon can lead to a more complex interaction. Additionally, the numerical simulations conducted in this study demonstrate that the inclusion of immune cells significantly reduces the total tumor count in both stable and unstable scenarios compared to the results presented in [15]. Furthermore, this study includes global sensitivity analysis and numerical bifurcation analysis. In particular, combined therapies of OVT and CAR T-cell therapy are considered in Section 5.
The subsequent sections unfold as follows: model development is presented in Section 2, followed by the analysis of ordinary differential equations and DDE models in Sections 3 and 4, respectively. Section 5 delves into the numerical investigation, and the final section concludes with a brief discussion. Appendixes A and B discuss the models of special cases, while Appendix C presents the proofs of some analytical results.
Cancer cells are categorized as either infected or uninfected. The uninfected tumor cells are further separated into resistant or sensitive to OVT. Let Tr and Ts denote the numbers of uninfected resistant and sensitive cancer cells, respectively. The variable Ti denotes the class of infected tumor cells, and V represents the compartment of free viruses. The number of immune cells at the tumor site is denoted by Z. A non-sensitive cancer cell will produce resistant descendants except if it transforms into sensitivity before birth occurs. We assume that the resistant and sensitive tumor cells have logistic growth with intrinsic growth rates rr and rs, respectively, and a carrying capacity denoted by K. While resistant and sensitive tumor cells may differ in their response to oncolytic viral therapy, they still share the same physical environment within the tumor. This environment provides the necessary resources for cell growth and imposes limitations on cell proliferation. Therefore, both types of tumor cells are subject to the same constraints imposed by factors such as nutrient availability and spatial limitations, and consequently, they have the same carrying capacity.
Several researchers propose that non-sensitive cancer cells exhibit a smaller growth rate in comparison to cancer cells that are not resistant due to the associated costs of resistance [5,12]. To incorporate this finding, we will assume rr<rs in numerical simulations. The rate of mutation from sensitivity to resistance is represented by the parameter a. Because infected cancer cells have a short life duration and do not require substantial materials, their geographical occupancy, proliferation, and nutrient absorption are not considered. Refer to [19,20], in which the tumor's carrying capacity does not include infected cancer cells.
Immune cells are capable of killing sensitive, resistant, and infected tumor cells. Regarding the killing of viruses, it typically involves a separate immune response, such as the innate immune response mediated by phagocytes and the adaptive immune response mediated by antibodies and cytotoxic T cells [21]. The immune cells considered in the model include both innate and adaptive systems, where the immune cells are not activated by viruses directly but through infected tumor cells. This modeling aspect is consistent with the works [16,17,18]. Additionally, [22] distinguishes adaptive immune cells into those that are either tumor-specific or virus-specific, where virus-specific adaptive immune cells do kill viruses. Therefore, even the adaptive immune cells are capable of killing viruses according to Murphy et al. [21] and the studies by Vithanage et al., and Storey et al. [17,18,22]. The killings of sensitive, resistant, and infected tumor cells, as well as viruses, by immune cells are modeled using a simple mass action approach, with maximal rates denoted by ks, kr, ki, and kv, respectively.
The interaction between sensitive cancer cells and viruses obeys the simple law of mass action where β denotes the maximum rate. The parameter b denotes an extra mortality rate of infected cancer cells due to infection, which quantifies pathogenicity of the virus. When infected cancer cells die, new viral progeny are discharged. The number of viruses released per infected tumor cell is represented by q. The viral clearance rate is represented by the parameter c. Every parameter is positive except potentially for d and a. Here, d≥0 denotes the rate of transition from resistance to sensitivity. Parameters a and d are identified as the Darwinian effect in [5] when studying acquired resistance in chemotherapy. With respect to resistance in OVT, the transition from resistance to sensitivity could be influenced by the solid tumor's geometric characteristics. After the demise of certain infected cancer cells, specific resistant cancer cells may become susceptible to infection and consequently transition to a sensitive state. Moreover, the drug ruxolitinib is employed to deal with pancreatic ductal adenocarcinoma (PDAC) cells that are not sensitive to oncolytic vesicular stomatitis virus (VSV), improving the susceptibility of resistant pancreatic cancer cells to VSV [23]. This treatment may result in an increase in d because of resistance therapy.
The immune cells are recruited by tumor cells using a Michaelis-Menten function α(Ts+Tr+Ti)Zm+Ts+Tr+Ti, where α represents the maximal proliferation rate, and m is the half-saturation constant. Immune cells can be eliminated or depleted through various processes, such as cell death (apoptosis), clearance by other immune cells, or inhibition of immune cell production [3]. We assume that this eliminate rate is constant and is denoted by the parameter γ. To incorporate the time delay between the encounter with tumor cells and the proliferation of immune cells, we introduce a discrete delay parameter, denoted as τ, into the activation term.
From the above discussion, the interaction between various types of tumor cells, viruses, and immune cells can be captured by the following system:
T′s(t)=rsTs(t)(1−Ts(t)+Tr(t)K)−aTs(t)−βTs(t)V(t)+dTr(t)−ksTs(t)Z(t)T′r(t)=rrTr(t)(1−Ts(t)+Tr(t)K)+aTs(t)−dTr(t)−krTr(t)Z(t)T′i(t)=βTs(t)V(t)−bTi(t)−kiTi(t)Z(t)V′(t)=qbTi(t)−cV(t)−kvV(t)Z(t)Z′(t)=α(Ts(t−τ)+Tr(t−τ)+Ti(t−τ))Z(t−τ)m+Ts(t−τ)+Tr(t−τ)+Ti(t−τ)−γZ(t). | (2.1) |
The initial data on [−τ,0] are given by
Ts(t)=ϕ1(t), Tr(t)=ϕ2(t), Ti(t)=ϕ3(t), V(t)=ϕ4(t), Z(t)=ϕ5(t), t∈[−τ,0],ϕj(t)∈C([−τ,0],R+), 1≤j≤5, ϕ1(0)>0, ϕ1(0)+ϕ2(0)≤K, | (2.2) |
where C([−τ,0],R+) represents the Banach space of all continuous functions mapping the interval [−τ,0] into R+={x∈R:x≥0}. A conceptual diagram of model (2.1) is given in Figure 1.
Let
X=(x1, x2, x3, x4)=(Ts(t), Tr(t), Ti(t), V(t))Y=(y1, y2, y3, y4)=(Ts(t−τ), Tr(t−τ), Ti(t−τ), V(t−τ)), | (2.3) |
and denote the right hand side of (2.1) as
f(X,Y)=(f1(X,Y), f2(X,Y), f3(X,Y), f4(X,Y)). | (2.4) |
In the following, we verify that model (2.1) is well-posed and ensure that the model is biologically feasible. Its proof is relegated to Appendix C.
Theorem 2.1. The solution to the systems (2.1) and (2.2) exists for t>0, and is nonnegative and bounded.
We next proceed to study model (2.1) without considering the delay in the activation of immune cells.
In this section, we shall investigate model (2.1) without delay, τ=0. Specifically, we study the ODE model without OVT in Section 3.1. The full system (2.1) with τ=0 is investigated in Section 3.2. The models of the special cases d=0 and no resistant tumor cells are briefly discussed at the end of this section, and a more detailed discussion is presented in Appendix A.
Suppose d>0. When τ=0 and there is no viral therapy, Ti(0)=0=V(0), Ti(t)=0=V(t) for t>0, and (2.1) is reduced to
T′s(t)=rsTs(t)(1−Ts(t)+Tr(t)K)−aTs(t)+dTr(t)−ksTs(t)Z(t)T′r(t)=rrTr(t)(1−Ts(t)+Tr(t)K)+aTs(t)−dTr(t)−krTr(t)Z(t)Z′(t)=α(Ts(t)+Tr(t))Z(t)m+Ts(t)+Tr(t)−γZ(t)Ts(0)>0, Tr(0)≥0, Ts(0)+Tr(0)≤K, Z(0)≥0. | (3.1) |
In addition, Z(0)=0 implies Z(t)=0 for t>0, and (3.1) reduces to the two-dimensional TsTr system. The TsTr subsystem of (3.1) has been studied in [15]. The subsystem has two equilibria: (0,0) and (ˆTs,ˆTr), with
ˆTs=dKa+d,ˆTr=aKa+d, | (3.2) |
where (ˆTs,ˆTr) is globally asymptotically stable as shown in [15]. Consequently, (3.1) always has two equilibria (0,0,0) and (ˆTs,ˆTr,0). The Jacobian matrix of (3.1) evaluated at (0,0,0) and (ˆTs,ˆTr,0) is given respectively by
[rs−ad0arr−d000−γ],and[−rsˆTs/K−a−rsˆTs/K+d−ksˉTs−rrˆTr/K+a−rrˆTr/K−d−krˆTr00αKm+K−γ]. | (3.3) |
It is evident that the stability of the equilibrium point (0,0,0) hinges on the eigenvalues of the matrix
[rs−adarr−d]. |
In the proof of Proposition 3.1 in [15], it is established that the eigenvalues of this matrix have positive real parts. Therefore, it follows that the equilibrium point (0,0,0) is unstable. From (3.3), the stability of (ˆTs,ˆTr,0) is determined by the eigenvalues of the left upper 2×2 submatrix, which is Eq (3.3) of [15], and the sign of
αKm+K−γ. |
It was shown in [15, Proposition 3,1] that the eigenvalues of the submatrix have negative real parts. As a result, (ˆTs,ˆTr,0) is asymptotically stable if αKm+K<γ and unstable if αKm+K>γ. Let
Γ={(x,y,z)∈R3+:0<x+y≤K}. | (3.4) |
Based on the proof provided in Appendix C, we can conclude that (ˆTs,ˆTr,0) is globally asymptotically stable in Γ if αKm+K<γ.
Proposition 3.1. Let τ=0, d>0, and αKm+K<γ. The equilibrium (ˆTs,ˆTr,0) is globally asymptotically stable in Γ for (3.1).
The fraction αKm+K is the maximal proliferation rate of immune cells induced by cancer cells. If this maximal rate is lower than the rate of immune cell death γ, the effector cells cannot sustain themselves, and the immune-free equilibrium (ˆTs,ˆTr,0) becomes globally asymptotically stable.
A positive equilibrium is an equilibrium in which each of its components is positive. To study the existence of positive equilibria, we assume αKm+K>γ by Proposition 3.1, since if the inequality is reversed, solutions all converge to (ˆTs,ˆTr,0), and a positive equilibrium cannot exist. It follows that α>γ. Define
ξ=mγα−γ. | (3.5) |
Setting Z′=0 and Z>0, we obtain Ts+Tr=ξ. Next, T′s=0 and T′r=0, imply respectively,
Z=rsTs(1−ξ/K)−aTs+d(ξ−Ts)ksTsandZ=rr(ξ−Ts)(1−ξ/K)+aTs−d(ξ−Ts)kr(ξ−Ts). |
Setting the above two expressions of Z equal, leads to the following second degree polynomial equation in Ts:
g(Ts):=a1T2s+a2Ts+a3=0, | (3.6) |
where
a1=[(a+d−rs)kr−ks(a+d−rr)]K+ξ(krrs−ksrr)K,a2=−ξ[(a+2d−rs)kr−ks(d−rr)]K+ξ(krrs−ksrr)K,a3=ξ2dkr. | (3.7) |
In addition, g(ξ)=−ksξ2a<0, and g(0)=a3>0. Since Tr=ξ−Ts holds for the two components of any positive equilibrium, we therefore require the root Ts<ξ as well. Also, setting 0=T′s+T′r, we have
Z=(rsTs+rrTr)(1−ξ/K)ksTs+krTr>0 |
provided ξ<K. i.e., ξ<K is a necessary condition for the existence of a positive equilibrium. Notice ξ<K is equivalent to αKm+K>γ, under which (ˆTs,ˆTr,0) is unstable.
We separate the discussion of g(Ts)=0 into the cases of a1<0, a1>0, and a1=0. If a1<0, then it is evident that (3.1) exhibits an interior equilibrium uniquely since g(ξ)<0. If a1>0, then since g(ξ)<0, g(x)=0 has 2 positive roots x1 and x2 with x1<ξ<x2. It follows that (3.1) also has a unique positive equilibrium. If a1=0, then a2=−ξ(aks+dkr)<0, and thus there exists a unique positive equilibrium. We conclude that (3.1) exhibits a unique interior steady state (ˉTs,ˉTr,ˉZ) if αKm+K>γ.
Let αKm+K>γ and let J=(ˉjij) denote the Jacobian matrix of (3.1) at (ˉTs,ˉTr,ˉZ), where
ˉj11=−rsˉTsK−dˉTrˉTs,ˉj12=d−rsˉTsK,ˉj13=−ksˉTs,ˉj21=a−rrˉTrK,ˉj22=−rrˉTrK−aˉTsˉTr,ˉj23=−krˉTr,ˉj31=αmˉZ(m+ˉTs+ˉTr)2,ˉj32=ˉj31,ˉj33=0. | (3.8) |
Observe that
ˉj11ˉj22−ˉj21ˉj12=(ˉTs+ˉTr)(rrd(ˉTr)2+rsa(ˉTs)2)KˉTsˉTr>0 |
and the characteristic polynomial is given by
ˉP2(λ)=λ3+b1λ2+b2λ+b3, | (3.9) |
where
b1=−(ˉj11+ˉj22)>0,b2=(ˉj11ˉj22−ˉj12ˉj21)−(ˉj13+ˉj23)ˉj31>0,b3=ˉj31(ˉj11ˉj23+(ˉj22−ˉj21)ˉj13−ˉj12ˉj23). | (3.10) |
Notice
ˉj11ˉj23+(ˉj22−ˉj21)ˉj13−ˉj12ˉj23=(ˉTr+ˉTs)((ˉTr)2dkr+(ˉTs)2aks)ˉTs+ˉTr>0, |
and thus b3>0.
By the Routh-Hurwitz condition [24], Re(λ)<0 for all roots of ˉP2(λ)=0 if and only if b1>0, b3>0, and b1b2>b3. Since b1>0 and b3>0, (ˉTs,ˉTr,ˉZ) is locally asymptotically stable for system (3.1) if the condition
b1b2>b3 | (3.11) |
is satisfied. We cannot obtain a simplified expression of (3.11) involving components of the equilibrium, so we leave (3.11) as it is. The above discussion is summarized as follows.
Proposition 3.2. Let d>0 and αKm+K>γ. Then, (3.1) exhibits a unique interior steady state (ˉTs,ˉTr,ˉZ), which is asymptotically stable if (3.11) is valid, and unstable if (3.11) is reversed.
The existence condition of a unique interior equilibrium implies that
ˉTs+ˉTr=ξ<K=ˆTs+ˆTr, |
and therefore the total tumor load in (ˉTs,ˉTr,ˉZ) is smaller than the corresponding tumor burden in (ˆTs,ˆTr,0). The immune cells are able to reduce the total tumor load if their maximal proliferation rate is greater than their death rate.
We assume d>0 and investigate the full system (2.1) without delay by examining the existence and stability of equilibria. The Jacobian matrix has the following entries:
j11=rs(1−2Ts+TrK)−a−βV−ksZ,j12=−rsTsK+d,j13=0,j14=−βTs,j15=−ksTs,j21=−rrTrK+a,j22=rr(1−Ts+2TrK)−d−krZ,j23=0,j24=0,j25=−krTr,j31=βV,j32=0,j33=−b−kiZ,j34=βTs, j35=−kiTi,j41=0, j42=0,j43=qb,j44=−c−kvZ,j45=−kvV,j51=αmZ(m+Ts+Tr)2,j52=j51,j53=j51,j54=0,j55=α(Ts+Tr+Ti)m+Ts+Tr+Ti−γ. | (3.12) |
Denote the two equilibria by E0=(0,0,0,0,0) and E1=(ˆTs,ˆTr,0,0,0), where ^Ts+^Tr=K. At E0, the Jacobian matrix becomes
J(E0)=[rs−ad000arr−d00000−b0000qb−c00000−γ]. |
Eigenvalues of J(E0) are the eigenvalues of
[rs−adarr−d],−b,−c,and−γ. |
Therefore, E0 is always unstable. At E1,
J(E1)=[−rs^TsK−a−rs^TsK+d0−β^Ts−ks^Ts−rr^TrK+a−rr^TrK−d00−kr^Tr00−bβ^Ts000qb−c00000αKm+K−γ]. |
The eigenvalues consist of the eigenvalues of the upper left 2×2 and the lower right 3×3 submatrices. It follows that E1 is locally asymptotically stable if
c>βq^TsandαKm+K<γ. |
The stability of E0 and E1 is summarized below.
Proposition 3.3. Let τ=0 and d>0. System (2.1) always has two equilibria E0=(0,0,0,0,0) and E1=(ˆTs,ˆTr,0,0,0), where E0 is unstable. The equilibrium E1 is asymptotically stable if c>βqˆTs and αKm+K<γ, and unstable if either c<βqˆTs or αKm+K>γ.
In the following, we show that there will be no viruses and infected tumor cells if the viral death rate c is sufficiently large. Since the proof is similar to that given in [17], it is omitted.
Proposition 3.4. If c>βqK, then solutions of (2.1) with τ=0 satisfy limt→∞Ti(t)=0=limt→∞V(t).
Based on Proposition 3.4, we provide a global asymptotic stability result of E1 under some constraints. Its proof is presented in Appendix C. Let
D={(X,Y,Z,V,W)∈R5+:0<X+Y≤K}. | (3.13) |
Theorem 3.1. Let τ=0 and d>0. If c>βqK and αKm+K<γ, then E1=(ˆTs,ˆTr,0,0,0) is globally asymptotically stable in D.
The lump parameter βqK can be viewed as the maximal virus production rate. If this rate is smaller than the viral clearance rate c, and the maximal proliferation rate of immune cells is not large enough, αKm+K<γ, then neither the effector cells nor the viruses can persist in the system.
Suppose now the maximal proliferation rate of effector cells exceeds their death rate, αKm+K>γ. Then (2.1) has an equilibrium of the form E2=(ˉTs,ˉTr,0,0,ˉZ), where (ˉTs,ˉTr,ˉZ) is the positive equilibrium of the virus-free subsystem (3.1). Its stability depends on the eigenvalues of
J(E2)=[ˉj11ˉj120j14ˉj13ˉj21ˉj2200ˉj2300j33j34000j43j440ˉj31ˉj31ˉj3100], | (3.14) |
where ˉJik, 1≤i,k≤2, and ˉj13,ˉj23,ˉj31 are defined in (3.8). By interchanging columns 3 and 5, and the corresponding rows, J(E2) is similar to
[ˉj11ˉj12ˉj13j140ˉj21ˉj22ˉj2300ˉj31ˉj3100ˉj31000j44j43000j34j33]. | (3.15) |
It follows that the characteristic polynomial of J(E2) is the product of ˉP2(λ) defined in (3.9) and
λ2−(j33+j44)λ+j33j44−j34j43. |
Since j33<0, j44<0, j34>0 and j43>0, in addition to the condition of (3.11), one would also require j33j44−j34j43>0, i.e.,
(b+kiˉZ)(c+kvˉZ)−βqbˉTs>0 | (3.16) |
for E2 to be locally asymptotically stable.
Proposition 3.5. Let τ=0 and αKm+K>γ. Then, (2.1) has the equilibrium E2=(ˉTs,ˉTr,0,0,ˉZ), where E2 is asymptotically stable if (3.11) and (3.16) are satisfied, and E2 is unstable if the inequality in (3.11) or (3.16) is reversed.
Observe that system (2.1) has an equilibrium of the form E3=(T∗s,T∗r,T∗i,V∗,0), provided c<βqˆTs, where (T∗s,T∗r,T∗i,V∗) is the unique positive equilibrium in the tumor-virus subsystem without immune cells by a previous analysis [15]. In particular,
T∗s=cβq,T∗r=K(rr−d−rrT∗s/K)+K√(rr−d−rrT∗s/K)2+4aT∗srr/K2rr, |
V∗=(rsT∗s+rrT∗r)(1−T∗s+T∗rK)βT∗s,T∗i=βT∗sV∗b. |
The equilibrium E3 has the Jacobian matrix given by
J(E3)=(a11a120a14a15a21a2200a25a310a33a34a3500a43a44a450000a55), | (3.17) |
where
a11=−(dT∗rT∗s+rsT∗sK),a12=d−rsT∗sK,a14=−βT∗s,a15=−ksT∗s,a21=a−rrT∗rK,a22=−(aT∗sT∗r+rrT∗rK),a25=−krT∗r,a31=βV∗,a33=−b,a34=βT∗s,a35=−kiT∗i,a43=qb,a44=−c,a45=−kvV∗,a55=α(T∗s+T∗r+T∗i)m+T∗s+T∗r+T∗i−γ. | (3.18) |
The characteristic polynomial of J(E3) can be expressed by
P3(λ)=(λ−a55)(λ4+a1λ3+a2λ2+a3λ+a4), |
where ai, 1≤i≤4, are given as
a4=((a33a44−a34a43)a11+a31a14a43)a22−a12a21(a33a44−a34a43)>0,a3=(−(a33+a44)a11−a33a44+a34a43)a22+(−a33a44+a34a43)a11+a12a21a44+a12a21a33−a14a43a31>0,a2=(a11+a33+a44)a22+a33a44−a12a21−a34a43+(a33+a44)a11>0,a1=−(a11+a22+a33+a44)>0. | (3.19) |
By the Routh-Hurwitz criterion [24], E3 is asymptotically stable if
a55<0anda1a2a3>a23+a4a21. | (3.20) |
We now summarize the stability properties of equilibrium point E3.
Proposition 3.6. Let τ=0 and βqˆTs>c. Then, (2.1) has an equilibrium of the form E3=(T∗s,T∗r,T∗i,V∗,0), where E3 is asymptotically stable if the inequalities in (3.20) are satisfied, and E3 is unstable if a55>0 or a1a2a3<a23+a4a21.
The conditions for the existence and local stability of the boundary equilibria of (2.1) with τ=0 are summarized in Table 1.
Boundary equilibrium (Ts,Tr,Ti,V,Z) | Existence | Stability |
E0=(0,0,0,0,0) | Always | Unstable |
E1=(ˆTs,ˆTr,0,0,0) | Always | c>βqˆTs and αKm+K<γ |
E2=(ˉTs,ˉTr,0,0,ˉZ) | αKm+K>γ | (3.11) and (3.16) |
E3=(T∗s,T∗r,T∗i,V∗,0) | c<βqˆTs | (3.20) |
In the cases where resistant tumor cells cannot be converted to sensitivity, for example, if there are no drugs available to reverse the resistance with OVT for a certain tumor type, then the parameter d is set to 0. We can provide a similar analysis as in the previous discussion and obtain some biological conclusions. In particular, according to Theorem B.1, every sensitive tumor cell becomes resistant, and there are no immune cells involved in the interaction if the following two conditions are met: First, the death rate c of viruses must exceed its maximum production rate βqK. Second, the immune cell activation rate αKm+K must be smaller than its clearance rate γ. This result differs from the findings presented in [15], where it was shown that every tumor cell becomes resistant if c>βqK, as there were no immune cells to control the tumor.
For the situation of no resistant tumor cells, it represents the best-case scenario where the tumor cells are not resistant to OVT. From the analysis given in Appendix A.2, we conclude that even in the absence of resistance to OVT, therapy can fail if the virus death rate is high and the immune activation rate is low, as demonstrated in Theorem A.2.
The main goal of this section is to study the effects of delay τ on the stability of equilibria of model (2.1). Our discussion parallels the ODE model discussed in Section 3. Specifically, the model of no OVT is studied in Section 4.1, and Section 4.2 investigates the full system (2.1). The models with d=0 and with no resistant tumor cells are briefly summarized at the end of Section 4.2, with a more detailed presentation given in Appendix B. For an analysis of the local stability of equilibria in DDE models, we refer the reader to the works of Hale [25], Kuang [26], and Smith [27].
Let d>0. The three-dimensional model of delay differential equations without infected cancer cells is given as
T′s(t)=rsTs(t)(1−Ts(t)+Tr(t)K)−aTs(t)+dTr(t)−ksTs(t)Z(t),T′r(t)=rrTr(t)(1−Ts(t)+Tr(t)K)+aTs(t)−dTr(t)−krTr(t)Z(t),Z′(t)=α(Ts(t−τ)+Tr(t−τ))m+Ts(t−τ)+Tr(t−τ)Z(t−τ)−γZ(t),Ts(t)=ψ1(t), Tr(t)=ψ2(t), Z(t)=ψ3(t), t∈[−τ,0],ψj(t)∈C([−τ,0],R+), 1≤j≤3, ψ1(0)>0, ψ1(0)+ψ2(0)≤K. | (4.1) |
The stability of an equilibrium (ˆX0,ˆX0) of (4.1) depends on the stability of the linearized system
X′(t)=ˆAX(t)+ˆBX(t−τ) |
at the zero equilibrium. Here,
ˆA=ˆfˆX(ˆX0,ˆX0),andˆB=ˆfˆY(ˆX0,ˆX0), |
where ˆf refers to the right-hand side of (4.1) with ˆX and ˆY similarly defined as in (2.3). The characteristic equation for the linearized system is given as
det(λI−ˆA−ˆBe−λτ)=0. |
At ˆX0=(0,0,0), since
ˆA+ˆBe−λτ=[rs−ad0arr−d000−γ] |
does not depend on τ, (0,0,0) remains unstable for τ>0. At ˆX0=(ˆTs,ˆTr,0),
ˆA+ˆBe−λτ=[−rsˆTs/K−a−rsˆTs/K+d−ksˆTs−rrˆTr/K+a−rrˆTr/K−d−krˆTr00αKm+Ke−λτ−γ], |
where the upper left 2×2 submatrix is the Jacobian matrix of the two-dimensional TsTr ODE system evaluated at (ˆTs,ˆTr), which was analyzed in [15, Proposition 3.1] with eigenvalues consisting of negative real parts. Therefore, the stability of (ˆTs,ˆTr,0) depends on the zeros of
αKm+Ke−λτ−γ. |
Assume αKm+K<γ, i.e., (ˆTs,ˆTr,0) is stable asymptotically for the corresponding non-delay system. Consider
αKm+Ke−λτ−γ−λ=0. | (4.2) |
It is known that λ=αKm+K−γ<0 when τ=0. If (ˆTs,ˆTr,0) alters its stability for some τ>0, then (4.2) will possess a pair of purely imaginary roots ±iω, ω>0, at some τ>0. Replacing λ by iw in (4.2), we obtain the following two equations:
αKm+Kcos(ωτ)−γ=0andαKm+Ksin(ωτ)+ω=0. |
Then, from the trigonometric identity cos2(θ)+sin2(θ)=1 for θ∈R, it follows that ω>0 must satisfy
(m+K)2ω2+γ2(m+K)2−α2k2=0. |
The quadratic equation has a unique root
ω2=(αK−γ(m+K))(αK+γ(m+K))(m+K)2<0 |
by the assumption. We obtain a contradiction and conclude that all the roots λ of (4.2) satisfy Re(λ)<0. Therefore, (ˆTs,ˆTr,0) is asymptotically stable for all τ≥0 if αKm+K<γ. The proof of the last part of Proposition 4.1(b) is presented in Appendix C.
Proposition 4.1. The following statements hold for system (4.1).
(a) (0,0,0) is always unstable for τ≥0.
(b) (ˆTs,ˆTr,0) is asymptotically stable for all τ≥0 if αKm+K<γ, and it is is unstable for all τ≥0 if αKm+K>γ.
Let αKm+K>γ and the condition in (3.11) be satisfied. That is, (3.1) has the unique positive equilibrium (ˉTs,ˉTr,ˉZ) by Proposition 3.2 and is moreover asymptotically stable for the ODE model (3.1). Since
α(ˉTs+ˉTr)m+ˉTs+ˉTr=γ, |
the stability of (ˉTs,ˉTr,ˉZ) depends on the eigenvalues of
[ˉj11ˉj12ˉj13ˉj21ˉj22ˉj23ˉj31e−λτˉj31e−λτ−γ+γe−λτ], | (4.3) |
where ˉjik, 1≤i,k≤2, ˉj13,ˉj23, and ˉj31, defined in (3.8), are the entries of the Jacobian matrix of the ODE model (3.1) evaluated at (ˉTs,ˉTr,ˉZ). The determinantal equation for (4.3) can be written as
λ3+p1λ2+p2λ+p3+(−γλ2+q1λ+q2)e−λτ=0, | (4.4) |
where
p1=γ−ˉj11−ˉj22>0,p2=(ˉj11ˉj22−ˉj12ˉj21)−γ(ˉj11+ˉj22)>0,p3=(ˉj11ˉj22−ˉj12ˉj21)γ>0,q1=(ˉj11+ˉj22)γ−ˉj31(ˉj13+ˉj23),q2=(−ˉj11ˉj22+ˉj12ˉj21)γ+ˉj31(ˉj11ˉj23−ˉj12ˉj23−ˉj13ˉj21+ˉj13ˉj22). | (4.5) |
If (ˉTs,ˉTr,ˉZ) undergoes a stability change for some τ>0, then (4.4) will have a pair of purely imaginary eigenvalues λ=±iω, ω>0, at some τ>0. Replacing λ by iω in (4.4), we arrive at
p1ω2−p3=(γω2+q2)cos(ωτ)+q1ωsin(ωτ), | (4.6) |
ω3−p2ω=q1ωcos(ωτ)−(γω2+q2)sin(ωτ). | (4.7) |
By squaring both sides of (4.6) and (4.7), and adding the resulting two equations, we have
ω6+(p21−2p2−γ2)ω4+(p22−2p1p3−2γq2−q21)ω2+p23−q22=0. | (4.8) |
In terms of x=ω2, Eq (4.8) becomes a polynomial equation of degree three:
F(x):=x3+(p21−2p2−γ2)x2+(p22−2p1p3−2γq2−q21)x+p23−q22=0. | (4.9) |
Further, Eqs (4.6) and (4.7) imply
sin(ωτ)=p1q1ω3−p3q1ω+(γω2+q2)(p2ω−ω3)(γω2+q2)2+q21ω2:=ρs, | (4.10) |
cos(ωτ)=q1ω(ω3−p2ω)+(p1ω2−p3)(γω2+q2)(γω2+q2)2+q21ω2:=ρc. | (4.11) |
If (4.9) has no positive roots, then (4.4) has no pure imaginary roots, and (ˉTs,ˉTr,ˉZ) remains stable for all τ>0. Applying the Routh-Hurwitz criterion [24], all roots of (4.9) have negative real parts if and only if the following conditions are satisfied:
p21−2p2−γ2>0,p23−q22>0,(p21−2p2−γ2)(p22−2p1p3−2γq2−q21)>p23−q22. | (4.12) |
We conclude the following with respect to the stability of (ˉTs,ˉTr,ˉZ).
Proposition 4.2. Let αKm+K>γ and suppose (3.11) holds true. Then, (ˉTs,ˉTr,ˉZ) is asymptotically stable for τ>0 if the inequalities in (4.12) are satisfied.
On the other hand, if F(x)=0 has a simple positive root, the following result is valid. The proof is presented in Appendix C.
Theorem 4.1. Let αKm+K>γ and assume (ˉTs,ˉTr,ˉZ) is asymptotically stable for (3.1). If (4.9) has at least one positive simple root, then one can find τ0>0 for which (ˉTs,ˉTr,ˉZ) is asymptotically stable for τ∈[0,τ0) and Sign(dRe(λ)dτ|τ=τ0)≠0.
It is expected that a Hopf bifurcation occurs at τ=τ0, causing the equilibrium (ˉTs,ˉTr,ˉZ) to become unstable.
We assume d>0. To study the effect of delay τ on the equilibria for the full model (2.1), we examine the eigenvalues of the Jacobian matrix A+Be−λτ, where A=fX(X0,X0), B=fY(X0,X0), and (X0,X0) is an arbitrary equilibrium. At E0=(0,0,0,0,0),
A+Be−λτ=[rs−ad000arr−d00000−b0000qb−c00000−γ] |
does not depend on τ, and therefore E0 is always unstable for τ≥0. At E1=(ˆTs,ˆTr,0,0,0),
A+Be−λτ=[−rsˆTs/K−a−rsˆTs/K+d0−βˆTs−ksˆTs−rrˆTr/K+a−rrˆTr/K−d00−krˆTr00−bβˆTs000qb−c00000αKm+Ke−λτ−γ] |
has eigenvalues consisting of the eigenvalues of the upper left 2×2 and the lower right 3×3 submatrices. We assume βqˆTs<c and αKm+K<γ to ensure that E1 is asymptotically stable for the ODE system (2.1) with τ=0. It follows that the stability of E1 for the DDE model (2.1) then depends on Eq (4.2). By Proposition 4.1, we conclude that if βqˆTs<c and αKm+K<γ, all of the eigenvalues of A+Be−λτ have negative real parts, and E1=(ˆTs,ˆTr,0,0,0) is asymptotically stable for all τ≥0. If βqˆTs>c, then E1 is clearly unstable. If αKm+K>γ, one can also show that E1 is unstable by the proof of Proposition 3.5(b). The above discussion is summarized as follows.
Proposition 4.3. The following statements are valid for system (2.1).
(a) E0=(0,0,0,0,0) is always unstable for τ≥0.
(b) E1=(ˆTs,ˆTr,0,0,0) is asymptotically stable for τ≥0 if βqˆTs<c and αKm+K<γ, whereas E1 is unstable for all τ≥0 if βqˆTs>c or αKm+K>γ.
Observe that Proposition 4.3 implies the delay in the immune activation has no effect on the tumor-free equilibrium E0 as well as the immune-free equilibrium E1.
Let αKm+K>γ. Then, E2=(ˉTs,ˉTr,0,0,ˉZ) exists, and its stability depends on the eigenvalues of
(ˉj11ˉj120j14ˉj13ˉj21ˉj2200ˉj2300j33j34000j43j440ˉj31e−λτˉj31e−λτˉj31e−λτ0−γ+γe−λτ) | (4.13) |
with ˉjij given in (3.8). The characteristic equation can be written as
(λ2−(j33+j44)λ+j33j44−j34j43)(λ3+p1λ2+p2λ+p3+(−γλ2+q1λ+q2)e−λτ)=0, |
where pi, 1≤i≤3, and qi,1≤i≤2, are defined in (4.5).
Assume E2 is asymptotically stable for the ODE model, i.e., both conditions (3.11) and (3.16) hold. Then the two roots of
λ2−(j33+j44)λ+j33j44−j34j43=0 |
have negative real parts. The stability of E2 therefore depends on the roots of (4.4), and we have the following conclusions.
Theorem 4.2. Let d>0 and let αKm+K>γ. Then, E2=(ˉTs,ˉTr,0,0,ˉZ) exists. Suppose (3.11) and (3.16) are satisfied. In addition, if (4.12) is true, then E2 is asymptotically stable for τ≥0.
The delay may not affect the stability of the virus-free equilibrium E2 in the presence of immune cells, provided that the parameters satisfy the conditions outlined in Theorem 4.2. On the other hand, if F(x)=0 has positive root, then the following is true.
Theorem 4.3. Let d>0 and let αKm+K>γ, and (3.11) and (3.16) be satisfied. Assume F(x)=0 exhibits at least one simple positive root. Then, one can find τ0>0 such that E2=(ˉTs,ˉTr,0,0,ˉZ) is asymptotically stable for τ∈[0,τ0) and dRe(λ)dτ|τ=τ0≠0.
It follows that the delay τ in the immune cell proliferation can destabilize the equilibrium E2. As τ increases beyond the critical value τ0, the virus-free equilibrium E2 becomes unstable, leading to oscillations in the tumor-virus-immune interaction as a result of the delay in immune recruitment.
Let c<βqˆTs. Then, E3=(T∗s,T∗r,T∗i,V∗,0) exists, and the stability of E3 depends on the corresponding Jacobian matrix, written as
(a11a120a14a15a21a2200a25a310a33a34a3500a43a44a450000−γ+(a55+γ)e−λτ), | (4.14) |
where aij, 1≤i,j≤5, are defined in (3.18). The characteristic equation is given by
(λ+γ−(a55+γ)e−λτ)(λ4+a1λ3+a2λ2+a3λ+a4)=0, |
with ai, 1≤i≤4, defined in (3.18). Assume a55<0 and a1a2a3>a23+a21a4 such that E3 is asymptotically stable for the ODE model. That is,
λ4+a1λ3+a2λ2+a3λ+a4=0 |
has only roots with negative real parts. Let
g(λ)=λ+γ−(a55+γ)e−λτ, |
where τ>0 is fixed. If g(λ) has a pair of pure imaginary roots λ=±iw, w>0, then w2=a55(a55+2γ), where a55<0 and a55+2γ>0. We obtain a contradiction and conclude that all roots of g(λ) have negative real parts. On the other hand, if a55>0, then g(0)=−a55<0, g(∞)=∞, and g′(λ)>0 for λ≥0. Thus, g(λ)=0 has one positive root. Therefore, E3 is unstable. Further, E3 is clearly unstable if a1a2a3<a23+a21a4. Below is a summary of the discussion.
Theorem 4.4. Let βqˆTs>c. Then, the equilibrium E3=(T∗s,T∗r,T∗i,V∗,0) exists, and the following statements hold true.
(a) E3 is asymptotically stable for τ≥0 if a55<0 and a1a2a3>a23+a21a4.
(b) E3 is unstable for τ≥0 if either a55>0 or a1a2a3<a23+a21a4.
Comparing Theorem 4.4 with Proposition 3.6, we observe that the delay τ in immune cell proliferation does not affect the stability of the immune-free tumorous equilibrium E3.
For the scenario of no conversion from resistance to sensitivity, it is concluded from Appendix B that the delay in the immune activation has no effects on the stability of the two equilibria E0=(0,0,0,0,0) and E01=(0,K,0,0,0). In addition, if αKm+K>γ, then E02=(0,ξ,0,0,~Z0) exists, where ξ=mγα−γ and ~Z0=rrkr(1−ξK). It follows from Appendix B.1 that the delay can destabilize E02.
In the best scenario where there is no resistance to OVT, the four-dimensional TsTiVZ system is further reduced to the two-dimensional TsZ system, as shown in (14.5), if no viruses and infected tumor cells are present. System (14.5) possesses an equilibrium of the form (ξ,˜Z), provided that αKm+K>γ, where ξ is defined in (3.5) and ˜Z is given in (14.6). Therefore, the four-dimensional system has an equilibriun of the form (ξ,0,0,˜Z). This equilibrium is no longer asymptotically stable for τ>0. The time delay τ can destabilize this equilibrium. However, it can be verified that the delay has no destabilization effects on the equilibria E0=(0,0,0,0) and ˜E1=(K,0,0,0). In addition, when the parameters satisfy the conditions given in Theorem C.2(b), the tumorous equilibrium ˜E2=(ξ,0,0,˜Z) in the presence of immune cells becomes unstable, as the delay τ passes beyond the critical value τ0. Consequently, the tumor-virus-immune interaction may exhibit oscillations due to the time delay in the immune activation. For the equilibrium ~E3=(˜Ts,˜Ti,˜V,0), since there are no immune cells present in the equilibrium, it is trivial to verify that the delay cannot have any effect on its stability.
In this section, we employ numerical tools to study system (2.1), addressing the effects of immune cells compared to the previous work in [15] in Section 5.1, incorporating global sensitivity analysis as discussed in Section 5.2, and covering numerical techniques for bifurcation analysis in Section 5.3. Section 5.4 explores the impact of parameters such as the virus transmission rate and half-saturation constant of immune activation on treatment success. Additionally, Section 5.5 considers a combination therapy of OVT and CAR T-cell therapy.
In our prior study [15], we explored the interactions between tumor cells and oncolytic viruses. This section now focuses on demonstrating the impact of immune cells, in comparison with our previous work. Initially, we compare the outcomes of models that do not incorporate delays in either viral infection or immune cell activation. For this comparison, we set the common parameter values in both the four-dimensional and five-dimensional systems to be identical to those in the parameter set given in [15, Eq (4.1)], as follows:
K=1/(1.02×10−9),rs=0.45,rr=0.01×rs,b=1.333,q=250,c=0.1,d=10−3,a=1×10−5,β=7×10−12. |
The initial conditions chosen are (7×106,102,0,5×106) for the model with no immune cells and (7×106,102,0,5×106,2×103) for the five-dimensional system. In numerous mouse experiments, such as those documented in [28,29], researchers typically inoculate mice subcutaneously with either 106, 2×106, or 5×106 cancer cells. At the time of injection, it is generally assumed that no immune cells are present in the mice. To allow the immune cells to proliferate to a quantity of 2×103, we increase the tumor burden to 7×106 cells, while maintaining a small number of resistant tumor cells, around 100 in this case. The doses of oncolytic viruses (OVs) administered vary, ranging from 2×106 in [29] to 108 pfu in [28]. For this part of the numerical simulation, we select 5×106, but slight variations in the initial conditions should not impede our biological conclusions.
Figure 2(a) depicts the outcome of the four-dimensional TsTrTiV model before incorporating immune cells, while Figure 2(b)–(d) depicts the outcome of the ODE five-dimensional model (2.1) with τ=0 for various parameter values. Specifically, the additional parameters for the five-dimensional TsTrTiVZ model are set as follows: (b) γ=0.03, ks=1.9×10−4, kr=1.9×10−4, ki=4, kv=0.025, m=500 with varied α=0.03, 0.035, 0.04; (c) α=0.035, ks=1.9×10−4, kr=1.9×10−4, ki=4, kv=0.025, m=500 with varied γ=0.03, 0.035, 0.04; and (d) α=0.035, γ=0.03, ks=1.9×10−4, kr=1.9×10−4, ki=4, kv=0.025 with varied m=500, 5000, 105.
In Figure 2(b), the ratio Km+K=0.999, indicating that when α=0.03=γ, the immune system is weak, and the total tumor load is large. As α is increased, the total tumor burden is significantly reduced. This finding is further confirmed by the scenarios shown in plots (c) and (d). Obviously, the solutions of those in Figure 2(b) with α=0.03, and (c) with γ=0.05 and 0.1 converge to an equilibrium level. By utilizing a three-dimensional TsTrZ plot, we can determine that the remaining solutions in Figure 2(b) with α=0.035 and 0.4 converge to a periodic solution, and so does the solution in (c) with γ=0.03. Moreover, each solution presented in (d) also converges to a periodic solution.
Through further adjustments to these parameters, we compared the outcomes and consistently observed that the immune-incorporated system maintained a lower total tumor count compared to the four-dimensional model without immune cells, especially when the recruitment rate of immune cells exceeds the death rate.
Next, we compare the results of the corresponding five-dimensional delay model TsTrTiVZ with the four-dimensional TsTrTiV ODE model, which does not include immune cells. Here, we consider the delay in immune cell activation with τ=5. In previous results without delay, as shown in Figure 2(b), an increase in the maximal immune cell proliferation rate α visibly lowered the total tumor burden. However, as depicted in Figure 3(a), with a small α value of 0.03 and delay, the stability of the equilibrium remains unchanged. When α is increased to 0.035, the amplitude of oscillation is larger in the delay model, and the total tumor load may exceed that for α=0.03. A similar phenomenon is observed as α is further increased to 0.04. However, the tumor burden is clearly smaller than that of the model without immune cells.
Regarding the immune cell death rate γ, it is apparent from Figure 3(b) that immune cells are not effective in controlling the tumor if the death rate γ=0.1 is large. As γ is reduced to 0.03, it is evident that the immune cells can lower the total tumor load even with a recruitment delay as shown in Figure 3(b). Further, the tumor load attains a larger maximum value with a delay compared to that without delay presented in Figure 2(c). A similar conclusion is also obtained as m is varied. The time delay in immune recruitment can increase the amplitude of the tumor burden.
The feasible parameter ranges of the model are compiled from the literature and presented in Table 2. The killing rate kr of resistant cancer cells by immune cells was estimated over a broader range of 10−4–10−2 cell−1 day−1, while the killing rate ks of susceptible tumor cells by immune cells is set at 10−5–10−3 cell−1 day−1. From the previous subsection, the initial conditions for Eq (2.1) with τ=0 are set as (7×106,102,0,5×106,2×103). For Eq (2.1) with τ>0, the history function is set as
ϕ1(t)=7×106,ϕ2(t)=102,ϕ3(t)=0,ϕ5(t)=2×103,t∈[−τ,0], | (5.1) |
Parameter | Description | Range | Reference |
K | Carrying capacity of tumor | 108–9.7×109 cells | [19,22] |
rs | Rate of growth of sensitive tumor cells | 0.18–0.97 day−1 | [18,19,36,37] |
β | Virus transmission rate | 6×10−12–0.862 pfu−1day−1 | [22,38] |
rr | Growth rate of resistant tumor cells | rs×[0.01 0.97] day−1 | [8,39] |
b | Death rate of infected tumor cells | 1.333–2.667 day−1 | [16] |
q | Virus burst size per infected tumor cell | 10–1350 pfu cell−1 | [16] |
c | Viral clearance rate | 0.024–24 day−1 | [40] |
a | Rate of mutation of sensitive cancer cells | 10−9–10−3 day−1 | [12,41] |
d | Rate of transition from resistance to sensitivity | 0−1 day−1 | guess |
[1ex] m | Half-saturation constant of immune cell proliferation rate | 40−105 cells | [16,22] |
γ | Death rate of immune cells | 0.024–0.178 day−1 | [22] |
α | Maximal immune cell proliferation rate | 0.024–2.4 day−1 | [22] |
ks | Killing rate of susceptible tumor cells | 10−5–10−3 cell−1 day−1 | [17] |
kr | Killing rate of resistant tumor cells | 10−4–10−2 cell−1 day−1 | guess |
ki | Killing rate of infected tumor cells | 9.6×10−3–4.8 cell−1 day−1 | [16,22] |
kv | Killing rate of viruses | 0.024−48 cell−1 day−1 | [22] |
τ | Delay in the activation of immune cells | 1–8 days | [42,43] |
and
ϕ4(t)=0,t∈[−τ,0),ϕ4(0)=5×106. | (5.2) |
In OVT, OVs can be administered following single dose or multiple dose regimens [35]. In this paper, single dose regimens are used, and OVs are administered at time t=0. Population dynamics are solved numerically using the built-in function ode23tb in Matlab for τ=0, and a history for population levels is created for solving Eq (2.1) with τ>0.
Sensitivity analysis is a common method used to identify critical inputs in a model. Global sensitivity analysis (GSA) is a method to analyze the impact of each input or parameter on the output uncertainty when all model inputs or parameters change randomly [44]. In this subsection, we conduct GSA utilizing the Partial Rank Correlation Coefficient (PRCC) to assess the relationship between each parameter and tumor size, Ts+Tr. Furthermore, GSA aids in selecting parameters for the bifurcation analysis to be performed in the next section. The parameter ranges are shown in Table 2. For the introduction and implementation of this method, we refer to the works by Marino et al. [44] and Vithanage et al. [17,18].
According to Liu et al. [45] and De Matos et al. [46], rapid immune-mediated clearance of oncolytic viruses can affect the efficacy of OVT. Following virus administration, oncolytic virus infection and replication within the tumor play a crucial role in the initial days [47,48]. Selecting T=7 for a short time interval enables the identification of parameters potentially related to the early-stage anti-tumor or anti-viral effects. In contrast, choosing T=21 for a longer time interval helps pinpoint parameters associated with the asymptotic tumor size and the treatment outcome. GSA will be conducted with two endpoints, T=7 and T=21.
In Figure 4, PRCC is computed for each parameter. The criteria for the strength of a correlation in [49] are used in this paper. A correlation is considered non-important if |PRCC|<0.2, weak if 0.2<|PRCC|<0.5, strong if 0.5<|PRCC|<0.7, and very strong if 0.7<|PRCC|. Figure 4(a), (b) depicts the PRCC for each parameter in Eq (2.1) with τ=0. The maximum immune cell proliferation rate, α, shows a strong negative correlation with the tumor size at T=7. The virus transmission rate, β, exhibits a weak positive correlation with the tumor size when τ=0. All other parameters show unimportant correlations with the final tumor size.
It has been reported that the activation of the adaptive immune response takes at least several days [43], and Pulendran et al. and Sun et al. have noted that after infection, T lymphocytes go through an expansion phase, reaching their peak on day 8 in response to antigen stimulation [42,50]. To examine the effect of delay in immune cell proliferation, we choose a larger delay in its range (Table 2). In Figure 4(c), (d), we consider Eq (2.1) with τ=7. The maximum immune cell proliferation rate, α, and the killing rate, ks, have strong negative correlations with the tumor size at T=7. All other parameters show weak or unimportant correlations with the final tumor size.
Figure 4 shows consistency of important parameters in all cases. Parameters α and ks related to immune cell proliferation and immune response have strong correlations with the tumor size. We explore the correlations by studying the tumor dynamics, Ts(t)+Tr(t) for 0≤t≤21, with selected parameter values for α, ks, and β. All other parameter values are fixed as follows:
K=109,rs=0.45, rr=0.3, b=1.33, a=0.01, c=1.83, γ=0.17, m=105, | (5.3) |
d=0.1, β=10−6, q=102, α=0.2, kr=10−4, ks=10−5, ki=1.8, kv=0.15. | (5.4) |
Figure 5(a), (d) shows the tumor dynamics Ts+Tr for selected α values. Larger proliferation rates result in smaller tumor sizes. Equation (2.1) models a delay in immune cell proliferation. Figure 5(d) shows a delay in tumor cell elimination compared with Figure 5(a), which reflects a delay in immune cell proliferation. Figure 5(b), (e) shows larger tumor killing rates, ks, resulting in smaller tumor sizes. Interestingly, Figure 5(b), (e) suggests that the killing rate, ks, must exceed 5×10−4 to effectively destroy the tumor cells. Hale et al. have reported that the immune response is important for the success of treatment and preventing tumor recurrence [51].
Figure 5(c), (f) shows the same tumor dynamics when β<10−6, implying that the virus transmission rate is insufficient to induce an anti-tumoral effect if β<10−6. In contrast, the tumor population drops dramatically initially when β=10−4 and β=10−3, indicating that tumor cells are infected after viruses are administered. As the virus transmission rate increases, more tumor cells are infected, leading to a smaller population level of Ts+Tr. The tumor size for τ=7 is slightly smaller than that for τ=0, implying that a delay in immune cell proliferation may reduce anti-viral response. Based on the above numerical result, we assume β≥10−6 from this point onward.
Bifurcation analysis is the study of changes in the asymptotic behavior of a model under parameter variation and can provide a complete picture of asymptotic dynamics on a parameter domain. Bifurcation analysis usually begins with the identification of equilibria. Given a set of parameter values, the numerical method constructed in [18] can be directly applied to locate the equilibrium E2 and all positive equilibria. The mathematical analysis given in Section 3 can be used to find all other boundary equilibria. The numerical method in [52,53] is then applied to compute the bifurcations of equilibria. To study the effect of OVT, the virus transmission rate β is used as a bifurcation parameter. Note that Figure 5(c), (f) shows that the virus transmission rate β must be greater than 10−6. We use the range [10−6,1] for β. From GSA, the immune response is an important factor associated with the tumor size. The half-saturation constant, m, of the immune cell proliferation rate measures the steepness of the curve for the activation of immune cells in response to the presence of tumor cells. A small half saturation constant results in a sharp increase in immune cells with increasing tumor cells. We use m as the other bifurcation parameter.
Mahasa et al. [16] have suggested a half saturation constant of 40 cells in their model. The term describing immune cell proliferation in their model is independent of immune cells. With such a small half-saturation constant in Eq (2.1), immune cell proliferation is almost constant in the presence of tumor cells. The process of activation of immune cells involves the interaction between tumor cells and APCs (antigen presenting cells) [54]. Once activated, CD8+ T cells undergo clonal expansion with interleukin-2 (IL-2) stimulation [55]. Based on the biological mechanism of the activation and expansion process, the immune cell proliferation term in the fifth equation of the ODE system is modeled as a function of both tumor cells and immune cells. To match a similar proliferation rate in the model by Mahasa et al. [16], the half-saturation constant m may be a large number. Storey et al. [22] has suggested a range of 40−105 cells for m based on the work by Banerjee et al. [56]. The model considered in [56] does not consider a half saturation constant in the recruitment term for immune cells. Thus, the range of the half-saturation constant, m, has not been validated or justified in these works [16,22,56].
It has been shown that a small half-saturation constant may cause destabilization and produce sustained oscillations in population sizes [57,58,59]. Incorporating a delay in the ODE system can also lead to population oscillations in the solution. Using a range of small half-saturation constants may hinder the study of the destabilization effect caused by the time delay. Furthermore, as pointed out above, the half-saturation constant m may be a large number, and it affects the tumor size in the equilibria. Therefore, we use a wide range for m. Let [10−6,1]×[105,109] be the parameter domain for β and m. Other parameters are fixed as in Eqs (5.3) and (5.4). An adaptive grid method in Section 2.1 in [52] is applied with a 10 by 10 grid in the parameter domain. The regions containing bifurcation curves are refined with 6 levels. The code is implemented in Matlab, where the Newton method is used to find the equilibrium that has no analytic solution, and the built-in function eig is used to solve the eigensystem of the Jacobian matrix. The bifurcation diagram is shown in Figure 6(a).
All six equilibria coexist in a region of the parameter domain. Proposition 3.3 has shown that the tumor-free equilibrium E0 is unstable. From Eqs (3.2), (5.3) and (5.4), along with Proposition 3.3, the equilibrium E1 is unstable in the parameter domain (Figure 6(a)). The numerical simulation agrees with Proposition 3.3. There are one saddle node bifurcation curve SN, one Hopf bifurcation curve Hopf0, and two transcritical bifurcation curves Tr1 and Tr2. Both positive equilibria appear through saddle-node bifurcations on the curve SN. We use the notation E41 for the positive equilibrium that might be stable. The equilibrium E42 is never stable. The positive equilibrium E41 changes its stability through Hopf bifurcations on the curve Hopf0 when τ=0. Other bifurcations such as bifurcations of limit cycles may occur in the parameter domain. These bifurcations do not affect the stability property of the equilibria shown in Figure 6(a) and are out of the scope of this paper. Therefore, they will not be studied in this paper. The positive equilibria E41 loses its stability through transcritical bifurcations, Tr2, and the equilibrium E3 becomes stable when m increases. Note that the region above Tr2 satisfies the condition in Eq (3.20), and the curve Tr2 satisfies the condition a55=0 in Eq (3.20). The equilibrium E2 is stable when (β,m) lies below the transcritical bifurcation curve Tr1 for τ=0, and it is unstable when (β,m) lies above Tr1. Our numerical computation agrees with Proposition 3.5. The conditions Eqs (3.11) and (3.16) are satisfied in the region where E2 is stable, and the curve Tr1 satisfies the left hand side of Eq (3.16) equal to zero. Our numerical computation agrees with Proposition 3.6. Bistability exists in a region below Tr1.
Consider Eq (2.1) with τ>0. Recall that ODE and DDE systems have the same equilibria. The local stability of an equilibrium depends on the signs of the real parts of the solutions to det(A−λI+Be−λτ)=0, where A and B are defined in Section 4.2. If λ=0 is a solution to det(A−λI+Be−λτ)=0, it is also a solution to det(A+B−λI)=0. System (2.1) goes through the same bifurcations as the ODE system does on the bifurcation curves SN, Tr1, and Tr2. It is known that incorporating a time delay τ into the ODE system may produce destabilization effects and cause fluctuations in population dynamics through Hopf bifurcations [60]. A numerical method is proposed to identify such Hopf bifurcations for system (2.1) in a parameter domain when τ is fixed.
Based on the bifurcation curves for τ=0 shown in Figure 6, we focus on the Hopf bifurcations for system (2.1) in the region where E41 is stable. At a Hopf bifurcation of E41 for τ>0, det(A−λI+Be−λτ)=0 has a pair of pure imaginary roots. All other solutions have negative real parts. Starting with any Hopf bifurcation point on Hopf0, say (β0,m0), let ±iω0 be the pure imaginary eigenvalues for A+B. We fix β0 and use ω0 and m0 as an initial guess to find ω and m for a Hopf bifurcation point of system (2.1) with a fixed τ>0. It may be assumed that all other solutions of det(A−λI+Be−λτ)=0 at the parameter point (β0,m) have negative real parts. Otherwise, another bifurcation curve other than Hopf0, SN, Tr1, and Tr2 would appear in Figure 6(a). It is worth noting that ω and m appear in several entries of the matrix A−iωI+Be−iωτ. The Newton method is not practical since it involves taking derivatives with respect to ω and m. In this paper, the Nelder-Mead simplex method [61], which is a direct method, is employed for finding ω and m. The detailed algorithm can be found in [61], or the built-in function "fminsearch" in Matlab can be directly applied for this purpose.
Mahasa et al. [16] studied a mathematical model of OVT with a time delay in the stimulation of virus-specific immune cells. The time delay τ in their work was fixed at 7 hours, and their work showed that the time delay τ=7 hours does not affect the stability of the virus-free equilibrium. In this work, we use τ=1 day and τ=7 days, which are at both ends of the parameter range shown in Table 2. When (β0,m) is not close enough to (β0,m0), the continuation technique with some smaller increments in τ helps with the success of identifying m for τ=1. With each point on Hopf0 as an initial guess for the corresponding point on Hopf1, parallel computation helps accelerate the process. Hopf bifurcation curves Hopf1 with τ=1, Hopf2 with τ=1, and Hopf3 with τ=7 are computed and shown in Figure 6(b). The equilibrium E41 changes its stability on the curves Hopf1 and Hopf3 when τ=1 and τ=7, respectively. The curve Hopf3 is above Hopf1 which is above Hopf0, indicating that the presence of time delay destabilizes the system. For Eq (2.1) with τ=1, the curve Hopf2 shows Hopf bifurcations where E2 changes its stability. The equilibrium E2 is stable in the region bounded by the curves Tr1 and Hopf2. This shows again time delay exhibits a destabilization effect in Eq (2.1). Theorem 4.2 gives a condition for the stability of E2 when τ>0. The stability condition is given in Eq (4.12). Equation (4.12) is independent of β. Therefore, we examine the condition with the parameter values used in Figure 6. According to Theorem 4.2, the equilibrium E2 is stable for τ>0 when m>1.208×107. We then use the numerical method proposed in this section to compute the Hopf bifurcation for E2 for τ<1000 and find that E2 is stable when m>1.205×107 for τ<1000. Our numerical computation agrees with Eq (4.12). Since such large τ values are not within the plausible range of τ, the figure is not shown in this paper.
In this subsection, we study the effect of virus transmission rate β and the steepness of immune cell proliferation m on the outcome of treatment. Let β=0.1, which corresponds to a relatively large virus transmission rate for the range of β in Table 2. Let m=2×106. Note that this set of parameter values lies in the region where both E2 and E41 are stable, as shown in Figure 6(a). The tumor persists with a population size of Ts+Tr+Ti=γm/(α−γ). Furthermore, the tumor-free equilibrium E0 is unstable, meaning that either the treatment may fail or tumor relapse may occur. This simulation can help identify factors that may cause unsuccessful treatment or tumor recurrence and further help design strategies to improve OVT treatment.
Consider the initial condition (7×106,100,0,5×106,2×103) for treating a tumor of 7×106 cells with a therapeutic dose of 5×106 pfu. Figure 7(a) shows that the population dynamics approach the stable equilibrium E41. The stability of E41 implies that the virus is persistent. Virus persistence has been observed in experimental studies and proven as a means to slow down tumor growth [62]. The sensitive tumor cells (red curve) are infected quickly after the treatment, and thus the infected tumor cells (black curve) increase rapidly. The tumor cells respond to the treatment initially but develop resistance to the therapy causing an increase in resistant tumor cells (blue curve). Note that the elimination of resistant tumor cells relies on immune cells (green curve), and the immune system is not able to control the resistant tumor cells. Strategies to reduce resistant tumor cells or enhance the immune response to resistant tumor cells are required to overcome this challenge.
Let τ=1, and all parameter values remain the same as used for Figure 7(a). Figure 7(b) shows population oscillations. The equilibrium E41 becomes unstable, demonstrating the destabilization effect caused by time delay. A slightly larger m value is used with τ=1 and τ=7. Let m=3×106. The equilibrium E41 is stable when τ=1 and unstable when τ=7, where the population dynamics are similar to the population dynamics in Figure 7(a), (b), respectively. Equation (2.1) with τ>0 exhibits a destabilization effect. Treatment is not successful in either case, τ=0 or τ>0.
Figure 7(a), (b) shows that the OVT is effective initially when the transmission rate β=0.1, where the susceptible tumor cells are infected and killed. The immune cells go through the contraction phase after eliminating the infected cells. However, part of the susceptible tumor cells develop resistance to treatment, as shown in Figure 7(c), which presents a closer look at Figure 7(a). The resistant tumor population grows progressively, and the treatment ultimately fails. Consider m=104, which corresponds to a quicker immune cell proliferation in response to tumor growth. Figure 7(d) shows that a quicker immune response eliminates infected cells and viruses but not the other two populations of tumor cells. The anti-viral response, where viruses are cleared by the immune system, is a challenge in OVT [45,46]. Furthermore, a low half-saturation constant tends to cause an oscillation of populations [57,58]. Such persistent large scale oscillations are unlikely to be seen in tumor immune interactions [63]. Nevertheless, oscillations in tumor and immune cell populations may account for the transitional state between elimination and equilibrium phases in immunoediting [63].
Let β=10−6, which corresponds to a small virus transmission rate compared with the previous simulation. Let m=2×106. Figure 8 shows that the OVT is ineffective when the virus transmission rate β is small. The infected tumor cell population is small and eliminated soon, but the immune system is not able to eliminate the other two populations of tumor cells. This result agrees with the result shown in Figure 5(c), (f). Again, a quicker immune response by using a smaller half saturation constant m or a time delay τ>0 does not improve the outcome of OVT but causes an oscillation of populations. The figures are not shown here as the population dynamics are similar to those in Figure 7(d).
It is known that the interactions between the immune system and oncolytic viruses, resulting in both anti-viral and anti-tumoral responses, can have both beneficial and detrimental effects on the outcome of OVT [3,45,64]. Several studies have suggested the use of combination therapy with other immunotherapeutic approaches to improve the efficacy of treatment [64,65,66]. Guedan and Alemany [67] have published a review article addressing the potential role of combination therapy using OVT and CAR T-cell therapy in fighting cancer. It is expected that combination therapy using OVT and CAR T-cell therapy can improve the outcome of the OVT studied in this subsection.
Chimeric antigen receptor T-cell (CAR T-cell) immunotherapy is a novel revolutionary cancer treatment. The process of CAR T-cell therapy involves deriving T cells from the patient and engineering these T cells in vitro to promote the recognition of cancer cells and improve T-cell function. CAR T cells are then expanded and infused back into the patient to enhance the elimination of tumor cells [68,69,70]. Preclinical studies in combination therapies of OVT and CAR T-cell therapy have been conducted to investigate the synergistic effects [71], and the results have shown sustained anti-tumor immune response yielding promising treatment outcomes [72].
According to Figure 5 (c), (d), the killing rate must be at least 5×10−4 to effectively fight tumor cells. From Section 5.4, the development of resistance to OV is a challenge to the success of OVT. Assume that the engineered T cells have higher killing rates of susceptible and resistant cells than used in Section 5.4. Let ks=5×10−4, kr=5×10−3. Consider β=0.1, and m=2×106. Gruber et al. studied the relationship between tumor cells and T lymphocytes in breast cancer patients, and the patients had CTL counts of 365 ± 194 cells/μL (Mean ± SD) [73]. Within two standard deviations of the mean, it is safe to say that CTL counts for most patients range from several tens to 750 cells/μL. The dose levels for CAR T-cell therapy are reported to range from 60 million to 600 million cells [74,75]. For a person with body weight of 70 kg, about 7.2 percent of body weight is blood [76]. That is about 5 liters of blood. The concentration of CAR T cells in blood is about 12–120 cells/μL. The goal of CAR T cell therapy is to generate a sufficient number of CAR T cells to go into the tumor site to effectively target and attack the cancer cells [77]. From the simulation in the previous section, the numbers of effector cells in the stable equilibria E2 (Figure 8) and E41 (Figure 7(a)) are 43,000 and 2000, respectively. In this example, we simulate the concurrent administration of both agents. Using the initial condition (7×106,100,0,5×106,8000), where the number of the initial immune cells is 8000, Figure 9(a) shows that combination therapy is able to eradicate tumor cells. After the elimination of tumor cells, the immune cells go through the contraction phase. Further simulation shows that monotherapy using either CAR T-cell immunotherapy ((Figure 9(b)) or OVT (Figure 7(a)) is not able to eliminate the tumor. It has been reported that CAR T cell therapy is ineffective for solid tumors [77]. Combination therapy produces a synergistic effect [72].
Consider a larger tumor with 109 cells, which corresponds to a tumor of approximately 1 cm in diameter [34]. It is known that virus clearance mediated by innate and adaptive immune cells is a significant challenge in OVT [78]. The innate immune response acts rapidly [79,80]. We use time delay to simulate the strategy to circumvent this virus clearance in the initial days. Fu et al. [62] conducted experiments to show that genetically coating oncolytic virus with CD47 allows OVs to evade the immune response and promotes virus persistence at the tumor site. We use a lower kv value to simulate evasion of the immune response and to enhance virus persistence. Let kv=0.03 in this simulation. Figure 10(a) shows that the combination treatment is successful with a low dose of 5000 CAR T cells, compared with the simulation in Figure 9(a). The results of further simulation of other cases are summarized in Table 3. Combination therapy allows the use of lower doses of each agent and achieves better efficacy. According to Eldar-Boock et al. [81], combination therapy can reduce the toxicity and side effects due to lower doses.
Therapy | Delay | Number of | Efficacy |
Strategy | 0≤τ≤7 | CAR T cells | Number of Tumor cells |
OVT | τ≥0 | — | Ineffective (Figures 7 and 8) |
CAR T cell | τ≥0 | 8000 | Ineffective (Figure 9(b)) |
CAR T cell+OVT | τ=0 | 8000 | 108 |
CAR T cell+OVT | τ≥4 | 5000 | 109 |
CAR T cell+OVT+CD7 | τ=0 | 8000 | 109 |
CAR T cell+OVT+CD7 | τ≥4 | 5000 | 109 |
Recall that E0 is unstable. This implies a small perturbation may cause population dynamics to move away from E0. In Figure 10(b) we simulate that one susceptible cell is formed at t=1000. Note that 5–10% of T cells become memory T cells during the contraction phase[82,83]. These memory T cells can quickly respond to previously encountered pathogens [82]. The number of immune cells increases in response to the presence of tumor cells. However, the immune cells cannot control the growth of tumor cells leading to tumor recurrence. It has been reported that tumor recurrence is a formidable challenge for cancer treatment [84].
Drug resistance is a common phenomenon encountered in cancer therapy, posing a critical barrier that requires urgent overcoming. The issue of viral resistance is also encountered in oncolytic viral therapy. While various mathematical and computational models that have appeared in the literature focus on investigating resistance to chemotherapy, only one article [15] employs differential equations to address resistance in OVT.
In this study, we extended the work of [15] by incorporating immune cells into the tumor-virus interaction. These immune cells have the capability to eliminate both tumor cells and viruses. We established the existence and stability of boundary equilibria and presented global stability results. We proved that the delay in immune cell recruitment cannot destabilize boundary equilibria in which immune cells are not present. For those boundary equilibria with the presence of immune cells, we provided sufficient conditions based on model parameters for which the delay has no destabilization effects. Additionally, we derived a critical delay value, under which a boundary equilibrium with the presence of immune cells is destabilized when the delay passes through this critical value, leading to tumor oscillations. The introduction of immune cells results in an additional virus-free equilibrium when the recruitment rate of immune cells is sufficiently high. At this virus-free equilibrium, the total tumor burden is smaller compared to the virus-free equilibrium without immune cells. Consequently, we conclude that immune cells can effectively reduce the tumor load under conditions of sufficient immune strength. We also demonstrated numerically, using reasonable parameter values in Section 5.1, that the inclusion of immune cells can lower the tumor burden compared to the model where immune cells are not included.
GSA showed that the virus transmission rate β and the parameters associated with immune response, such as immune cell proliferation rate α and tumor killing rate ks, were important factors that affected the final tumor population (Figure 4). Strengthening the immune system is crucial for treatment success and preventing tumor recurrence [51]. The numerical simulation demonstrated that the virus transmission rate must exceed a certain threshold to induce an anti-tumoral effect (Figure 5(c), (f)). A numerical method of Hopf bifurcations was developed for system (2.1) with a time delay. Bifurcation analysis (Figure 6), using the virus transmission rate and the half-saturation constant of immune cell proliferation rate as bifurcation parameters, showed that the system (2.1) exhibited rich dynamics. The results of bifurcation analysis agreed with the mathematical analysis in Sections 3 and 4. The time delay τ in the activation of immune cells had a destabilization effect (Figures 6 and 7(a)–(d)).
Numerical simulation showed that the OVT was effective if the virus transmission rate was sufficiently high; however, the treatment may ultimately fail due to the development of resistance (Figure 7(c)). Resistance to oncolytic viruses has been reported to jeopardize the success of treatment [4]. Another simulation for a lower virus transmission rate showed that the viruses were cleared out before susceptible cells were infected (Figure 8), and thus the OVT was ineffective. Virus clearance is known to be a challenge in OVT [45,46]. Literature has suggested that OVT as monotherapy frequently fails to control tumor progression and sustain a reduction in tumor masses due to anti-viral effect or development of resistance [3,45,64,85]; combination therapy is a preferable solution to overcome such challenges [65,67,86,87].
To enhance the success of treatment, combination therapy using OVT and CAR T-cell therapy was considered in this paper. Numerical simulation showed that the combination therapy produced synergistic effects and enhanced the success of treatment (Figure 9(a)). Monotherapy using either OVT or CAR T-cell therapy is ineffective (Table 3). Further simulation studied treatment strategies to improve the outcome of treatment (Table 3). Circumventing immune clearance of OVs in the initial days allowed the use of a lower dose of CAR T cells and concurrently promoted the efficacy of the combination therapy. This agrees with the viewpoint of experimental and clinical reports. Virus infection and replication during the initial days of OVT significantly impact its efficacy. [47,48]; one goal of combination therapy is to improve efficacy while reducing toxicity [81]. Additional simulation showed that Prolonged virus persistence enhanced the outcome of treatment, which agreed with the experimental report that prolonging virus persistence improved the efficacy of OVT [62].
Finally, our simulation showed that cancer recurrence occurred after a tumor cell was formed (Figures 10(b)). Evidence has suggested that cancer recurrence has been a major challenge after the initial treatment response [88]. Our simulation results agreed with clinical and experimental research studies. It is expected that these results can be applied to clinical practice. Further studies including clinical tests are needed to further verify these results.
The immune cell assumptions made in this work were simplified. In future research, we intend to introduce more complexity into the interaction. We propose segregating them into two groups: tumor-specific immune cells and virus-specific immune cells. Tumor-specific immune cells will be activated by both resistant and sensitive tumor cells, capable of targeting and eliminating both types of cancer cells. On the other hand, virus-specific immune cells will be recruited by infected tumor cells and viruses, focusing solely on eliminating infected tumor cells and viruses. This revised model will encompass six nonlinear ordinary differential equations. Furthermore, we may introduce time delays, either in the viral cycle or in immune cell recruitment, to further enhance the model's accuracy.
The authors declare they have not used AI tools in the creation of this article.
We appreciate all four reviewers for their valuable suggestions, which have significantly improved the paper. The research of Hsiu-Chuan Wei was partially supported by the National Science and Technology Council of Taiwan under the grant NSTC 112-2115-M-035-006. The work of S. Jang was partially supported through Travel Support for Mathematicians from the Simons Foundation under MPS-TSM-00002532.
The authors declare there is no conflict of interest.
The ODE models of the special cases d=0 and no resistant cancer cells are briefly discussed in this appendix.
Since d=0, it follows that (ˆTs,ˆTr,0,0,0)=(0,K,0,0,0). If the maximal immune cell proliferation rate αKm+K is greater than its death rate γ, then the interaction is able to support another equilibrium, E02=(0,ξ,0,0,˜Z0), where
ξ=mγα−γ and ˜Z0=rr(1−ξ/K)kr. | (A.1) |
It can be verified that E02 is asymptotically stable if
(rs−rrkskr)(1−ξK)−a<0, | (A.2) |
and unstable if the above inequality is reversed. Moreover, if
α>γ,(1−ξK)(rs−rrkskr)>a, | (A.3) |
then the model (2.1) with τ=0 and d=0 has an equilibrium of the form E03=(ˉT0s,ˉT0r,0,0,ˉZ0), where
ˉT0s=ζ−aζa+(1−ζK)(krrsks−rr)−akrks,ˉT0r=aζa+(1−ζK)(krrsks−rr)−akrks,ˉZ0=1ks(rs(1−ζK)−a). | (A.4) |
Observe that the last inequality in (A.3) implies
(rs−rrkskr)(1−ξK)−a>0. |
Therefore, the existence of E03 implies that E02 is unstable. The Jacobian matrix at E03 has the same entries as J(E2) defined in (3.14). We can conclude that E03 is asymptotically stable if (3.11) and (3.16) are satisfied with d being replaced by 0. The existence and stability of boundary equilibria of (2.1) with τ=0 and d=0 are summarized in Table A1.
Boundary equilibrium (Ts,Tr,Ti,V,Z) | Existence | Stability |
E0=(0,0,0,0,0) | Always | Unstable |
E01=(0,K,0,0,0) | Always | αKm+K<γ |
[1ex] E02=(0,ξ,0,0,˜Z0) | αKm+K>γ | (A.2) |
[1.8ex] E03=(ˉT0s,ˉT0r,0,0,ˉZ0) | (A.3) | (3.11)* & (3.16)* |
Comparing Table A1 with Table 1, it is observed that E01=E1 when d=0, as in this instance ˆTs=0. Therefore, the condition c>βqˆTs given in Table 1 is trivially true and the stability of E01 in Table A1 reduces to αKm+K<γ only. Further, the second inequality in (A.3) implies ξ<K, i.e., αKm+K>γ. Thus, the equilibrium E03 given in Table A1 is the equilibrium E2 given in Table 1, and therefore the stability of E03 is based on E2. The existence of E3 in Table 1 requires that c<βqˆTs, which is impossible in the case d=0, and there is no such corresponding equilibrium in Table A1.
Parallel to Theorem 3.1, we can also derive sufficient conditions for the global stability of E01 as follows, without providing a proof.
Theorem A.1. Let τ=0 and d=0. The equilibrium E01=(0,K,0,0,0) is globally asymptotically stable in D for (2.1) if αKm+K<γ and c>βqK.
Assume that there are no resistant tumor cells initially, Tr(0)=0, and no mutation, a=0. Then, Tr(t)=0 for all t>0, and model (2.1) with τ=0 becomes a four-dimensional system involving only Ts,Ti,V, and Z. If, in addition, there are no infected tumor cells and viruses initially, Ti(0)=0=V(0), then Ti(t)=0=V(t) for all t>0, and we obtain the following two-dimensional system:
T′s(t)=rsTs(t)(1−Ts(t)K)−ksTs(t)Z(t)Z′(t)=αTs(t)Z(t)m+Ts(t)−γZ(t). | (A.5) |
The system (A.5) has three equilibria, where (0,0) and (K,0) always exist, and (ξ,˜Z) is biologically feasible only if αKm+K>γ, with ξ=γmα−γ defined in (3.5) and
˜Z=rsks(1−ξK). | (A.6) |
It is straightforward to see that (0,0) is always unstable, while (K,0) is asymptotically stable if αKm+K<γ and unstable if αKm+K>γ. We assume αKm+K>γ so that (ξ,˜Z) exists. It follows from the Dulac criterion [24] that (A.5) has no positive periodic solutions, which implies that (ξ,˜Z) is globally asymptotically stable in ˚R2+ for the two-dimensional model (A.5) by the Poincareˊ-Bendixson Theorem [24]. As a result, we see that \tilde E_2 = (\xi, 0, 0, \tilde Z) is asymptotically stable if
\begin{equation} (b+k_i\tilde Z)(c+k_v \tilde Z) > qb\beta \xi. \end{equation} | (A.7) |
Let c < \beta qK so that \tilde E_3 = (\tilde T_s, \tilde T_i, \tilde V, 0) exists, where
\begin{equation} \tilde T_{s} = \cfrac{c}{\beta q}, \quad \tilde V_0 = \cfrac{r_s}{\beta}(1-\cfrac{\tilde T_{s}}{K}), \quad \tilde T_{i} = \cfrac{c}{qb}\tilde V. \end{equation} | (A.8) |
See Section 3.3 of [15]. Applying the Routh-Hurwitz criterion [24], without delving into all the details, \tilde E_3 is locally asymptotically stable if it satisfies the following conditions
\begin{equation} \cfrac{\alpha(\tilde T_s+\tilde T_i)}{m+\tilde T_s+\tilde T_i} < \gamma \ \mbox{and} \ \cfrac{r_s(b+c)}{K}(b+c+\cfrac{r_s\tilde T_s}{K})-\beta^2qb\tilde V > 0. \end{equation} | (A.9) |
The above results are summarized in the following table.
Parallel to Theorem 3.1, we can also derive sufficient conditions for the global stability of \tilde E_1 as follows, without providing a proof.
Theorem A.2. Let \tau = 0 , a = 0 , and T_r(0) = 0 . The equilibrium \tilde E_1 = (K, 0, 0, 0) is globally asymptotically stable in \{(T_s, T_i, V, Z)\in\mathbb{R}_+^4: T_s > 0\} if \frac{\alpha K}{m+K} < \gamma and c > \beta q K .
Notice the stability of \tilde E_2 given in Table A2, (A.7), is the stability condition (3.16) for E_2 in Table 1, while the other condition (3.11) is trivially true for the case of no resistant tumor cells a = 0 and T_r\equiv 0 . In addition, the equilibrium \tilde E_1 in Table A2 corresponds to the equilibrium point E_1 = (\hat T_s, \hat T_r, 0, 0, 0) in Table 1 with T_r\equiv 0 , and they have the same existence condition. In addition, the inequalities (3.20) are equivalent to those given in (A.9), and therefore both equilibria have the same stability conditions.
Boundary equilibrium (T_s, T_i, V, Z) | Existence | Stability |
E_0=(0, 0, 0, 0) | Always | Unstable |
\tilde E_1=(K, 0, 0, 0) | Always | \beta qK < c and \cfrac{\alpha K}{m+K} < \gamma |
[1ex] \tilde E_2=(\xi, 0, 0, \tilde Z) | \cfrac{\alpha K}{m+K} > \gamma | (A.7) |
[1.8ex] \tilde E_3=(\tilde T_s, \tilde T_i, \tilde V, 0) | \beta qK > c | (A.9) |
The resulting model always has equilibria E_0 = (0, 0, 0, 0, 0) , and E_1^0 = (0, K, 0, 0, 0) . Similar to Section 4.2, it can be seen that E_0 is always unstable for \tau\geq 0 and E_1 is asymptotically stable for \tau\geq 0 if \cfrac{\alpha K}{m+K} < \gamma , and unstable for \tau\geq 0 if \cfrac{\alpha K}{m+K} > \gamma .
Let \cfrac{\alpha K}{m+K} > \gamma . Then, E^0_2 = (0, \xi, 0, 0, \tilde{Z^0}) exits, where \xi = \cfrac{m\gamma}{\alpha-\gamma} , and \tilde{Z^0} = \dfrac{r_r}{k_r}(1-\dfrac{\xi}{K}) . It can be argued that E_2^0 is asymptotically stable for \tau\geq 0 if certain conditions on the parameters are met. On the other hand, under certain constraints on the parameters, there exists a critical delay \tau_c beyond which E_2^0 becomes unstable as \tau increases. Indeed, let
\begin{equation*} \begin{array}{l} p_1 = (\dfrac{r_r\zeta}{K}+\gamma), \quad p_2 = \dfrac{\gamma r_r\xi}{K}, \quad q_1 = -\gamma , \quad q_2 = \dfrac{\gamma m k_r \tilde{Z^0}}{m+\xi}-\dfrac{\gamma r_r\xi}{K}. \end{array} \end{equation*} |
We summarize the following without proof as the proof is similar to that given previously.
Theorem B.1. Let d = 0 , \cfrac{\alpha K}{m+K} > \gamma , a_{11} < 0 , and p_2-q_2 < 0 . Then, there exists \tau_0 > 0 such that E^0_2 is asymptotically stable for \tau \in [0, \tau_0) and \dfrac{d(Re\lambda)}{d\tau}\Big|_{\tau = \tau_0} > 0 .
Assume (A.3) so that E_3^0 = (\bar T_s^0, \bar T_r^0, 0, 0, \bar Z^0) exists. Suppose that (3.11) and (3.16) are satisfied with d = 0 , i.e., E_3^0 is asymptotically stable for the ODE model with d = 0 . Then, one can also find a critical delay \tau_c such that E_3^0 is asymptotically stable for \tau\in[0, \tau_c) , and the transversality condition for \lambda can also be verified. We do not present the results here since the proof and conclusion are similar.
The proof of the following proposition is routine and is omitted.
Proposition 8.1. The following is true for system (2.1) with a = 0 and T_r(0) = 0 .
(a) E_0 = (0, 0, 0, 0) is unstable for \tau\geq 0 .
(b) \tilde{E_1} = (K, 0, 0, 0) is asymptotically stable for \tau\geq0 if c > \beta qK and \dfrac{\alpha K}{m+K} < \gamma , and unstable if either c < \beta q K or \dfrac{\alpha K}{m+K} > \gamma .
Let
\begin{equation*} \begin{array}{l} \tilde p_1^2-\tilde q_1^2-2\tilde p_2 = \Big(\dfrac{r_s\zeta}{K}\Big)^2,\quad \tilde p_2+\tilde q_2 = \dfrac{k_sm\tilde Z\gamma}{m+\zeta},\\[2ex] \tilde p_2-\tilde q_2 = \dfrac{2r_s\zeta\gamma}{K}-\dfrac{k_sm\tilde Z\gamma}{m+\zeta}. \end{array} \end{equation*} |
The delay \tau can affect the stability of \tilde E_2 as illustrated below.
Theorem B.2. Let \cfrac{\alpha K}{m+K} > \gamma . Then, \tilde E_2 = (\zeta, 0, 0, \tilde Z) exists, and
(a) \tilde{E_2} is asymptotically stable for \tau\geq 0 if (k_i\tilde Z+b)(k_v\tilde Z+c) > qb \beta\zeta and \tilde p_2-\tilde q_2 > 0 .
(b) If (k_i\tilde Z+b)(k_v\tilde Z+c) < qb \beta\zeta or \tilde p_2-\tilde q_2 < 0 , then there exists \tau_0 > 0 such that \tilde E_2 is asymptotically stable for \tau\in[0, \tau_0) and \dfrac{d(Re\lambda)}{d\tau}\Big|_{\tau = \tau_0}\neq 0 .
Proof of Theorem 2.1. Since f and \cfrac{\partial f}{\partial X} exist and are continuous on \mathbb{R}_+^5\times\mathbb{R}_+^5 , (2.1) has a unique solution on [-\tau, t_0) for some t_0 > 0 . Moreover, if X, Y \geq 0 with x_j = 0 , then f_j(X, Y)\geq 0 , and thus solutions remain nonnegative on [-\tau, t_0) by [27].
As
T_s^\prime(t)+T_r^\prime(t)\leq (r_sT_s(t)+r_rT_r(t))(1- \cfrac{T_s(t)+T_r(t)}{K}) |
and
T_s(0)+T_r(0)\leq K, |
we have T_s(t)+T_r(t)\leq K on [0, t_0) . Next,
T_s^{\prime}(t)+T_r^{\prime}(t)+T_i^{\prime}(t)\leq (r_sT_s(t)+r_rT_r(t))(2- \cfrac{T_s(t)+T_r(t)}{K})-r_sT_s(t)-r_rT_r(t)-bT_i(t)\leq C_1-b_1(T_s(t)+T_r(t)+T_i(t)) |
on [0, t_0) for some C_1 > 0 and b_1 = \min\{r_s, r_r, b\} > 0 . It follows that T_i(t)\leq \hat{C_1} for 0\leq t < t_0 for some \hat{C_1} > 0 . Then, V^{\prime}(t)\leq qb\hat{C_1}-cV , and we have V(t)\leq C_2 on [0, t_0) for some C_2 > 0 .
Notice
Z^{\prime}(t)\leq \cfrac{\alpha}{m}\Big(T_s(t-\tau)+T_r(t-\tau)+T_i(t-\tau)\Big)Z(t-\tau)-\gamma Z(t). |
Let
M(t) = T_s(t-\tau)+T_r(t-\tau)+T_i(t-\tau)+V(t-\tau)+Z(t). |
Then, by the given assumption,
\begin{equation*} \begin{array}{l} M^\prime(t)\leq[r_sT_s(t-\tau)+r_rT_r(t-\tau)]\Big(2-\cfrac{T_s(t-\tau)+T_r(t-\tau)}{K}\Big)+qbT_i(t-\tau)-r_sT_s(t-\tau)\\[1ex]-r_rT_r(t-\tau)-bT_i(t-\tau)-cV(t-\tau)-\gamma Z(t)\\[1ex] \leq \hat{M}-\hat {\gamma}M(t)\end{array} \end{equation*} |
on [0, t_0) for some \hat{M} > 0 , and
\hat{\gamma} = \min\{r_s, r_r, b, c, \gamma\} > 0. |
Hence, M(t) is bounded on [0, t_0) , and solutions cannot blow up as t \uparrow t_0 . Therefore, solutions exist on [0, \infty) and remain nonnegative. Similar arguments can be applied to show that solutions are bounded on [0, \infty) .
Proof of Proposition 3.1. Clearly, (\hat T_s, \hat T_r, 0) is asymptotically stable, and
Z^{\prime}(t) \leq ( \frac{\alpha K}{m+K}-\gamma ) Z(t) |
for all t \geq 0 implies \lim_{t\to\infty} Z(t) = 0 by the assumption. Thus, (3.1) is asymptotically autonomous [30] to the two-dimensional T_sT_r subsystem, under which (\hat T_s, \hat T_r) is globally asymptotically stable in the region for which T_s(0)+T_r(0) > 0 by [15]. Therefore, (\hat T_s, \hat T_r, 0) is globally asymptotically stable in \Gamma .
Proof of Theorem 3.1. By Proposition 3.4, \lim\limits_{t \to \infty}{T_i(t)} = 0 = \lim\limits_{t \to \infty}{V(t)} , and thus system (2.1) with \tau = 0 is asymptotically autonomous [30] to the three-dimensional model (3.1), where (\hat T_s, \hat T_r, 0) is globally asymptotically stable in \Gamma by Proposition 3.1. Since c > \beta qK > \beta q \hat T_s and \frac{\alpha K}{m+K} < \gamma , E_1 is asymptotically stable. It follows that E_1 is globally asymptotically stable in D .
Proof of Proposition 4.1. We only need to prove the second part of (b) for \tau > 0 . Let
h(\lambda) = \frac{\alpha K}{m+K}e^{-\lambda \tau}-\gamma-\lambda |
with \tau > 0 . Then,
h(0) = \frac{\alpha K}{m+K}-\gamma > 0, \quad h^\prime(\lambda) < 0 |
for \lambda\geq 0 , and
h(\infty) = -\infty. |
Therefore, h(\lambda) = 0 has one positive root, and hence (\hat T_s, \hat T_r, 0) is unstable for all \tau > 0 .
Proof of Theorem 4.1 Suppose (4.9) has j positive roots x_n, \ 1\leq j\leq 3 , each of which is simple. Then, (4.4) has j simple pure imaginary roots
\pm i\sqrt{x_n}, \quad 1\leq n \leq j. |
Clearly, for each n , 1\leq n \leq j , (4.10) and (4.11) have a unique solution \omega_n \tau in (0, 2\pi] , \omega_n = \sqrt{x_n} . Let
\begin{equation} \tau^l_n = \frac{1}{\omega_n}\left[ \arccos{\left( \frac{q_1\omega_n(\omega_n^3-p_2\omega_n)+(p_1\omega_n^2-p_3)(\gamma\omega_n^2+q_2)}{(\gamma \omega_n^2+q_2)^2+q_1^2\omega_n^2} \right)} +2\pi l \right],\ 1\leq n\leq j, \ l = 0,1,2,... \end{equation} | (C.1) |
if \rho_s > 0 , and
\begin{equation} \tau^l_n = \frac{1}{\omega_n}\left[ 2\pi-\arccos{\left( \frac{q_1\omega_n(\omega_n^3-p_2\omega_n)+(p_1\omega_n^2-p_3)(\gamma\omega_n^2+q_2)}{(\gamma \omega_n^2+q_2)^2+q_1^2\omega_n^2} \right)} +2\pi l \right],\ 1\leq n\leq j, \ l = 0,1,2,... \end{equation} | (C.2) |
if \rho_s\leq 0 . Define
\begin{equation} \tau_0 = \min\{\tau^l_n:1\leq n \leq j, \ l = 0,1,2,...\} = \tau^{l_0}_{n_0}, \end{equation} | (C.3) |
and set \omega_0 = \omega_{n_0} . We verify the transversality condition at \tau = \tau_0 , i.e., \frac{d Re(\lambda)}{ d\tau}|_{\tau = \tau_0}\neq 0 , by using
{\rm Sign}\Big{(}\cfrac{dRe(\lambda)}{d\tau}\big{|}_{\tau = \tau_0}\Big{)} = {\rm Sign}\Big{(}Re(\cfrac{d\lambda}{d\tau})^{-1}\big{|}_{\tau = \tau_0}\Big{)}. |
The transversality condition is a necessary condition for a Hopf bifurcation to occur [27].
Implicitly differentiate (4.4) with respect to \tau , and we obtain
\begin{equation*} \left(\frac{d\lambda}{d \tau}\right)^{-1} = -\frac{\tau}{\lambda}+\frac{-2\gamma\lambda+q_1}{\lambda(-\gamma\lambda^2+q_1\lambda+q_2)}-\frac{3\lambda^2+2p_1\lambda+p_2}{\lambda(\lambda^3+p_1\lambda^2+p_2\lambda+p_3)}. \end{equation*} |
It follows that
\begin{equation*} \Big( Re \left(\frac{d\lambda}{ d\tau}\right)^{-1}|_{\tau = \tau_0}\Big) = \cfrac{-2\gamma^2\omega_0^2-2\gamma q_2-q_1^2}{\gamma^2\omega_0^4+2\gamma q_2\omega_0+q_1\omega_0^2 +q_2^2}+ \frac{3\omega_0^4+(2p_1^2-4p_2)\omega_0^2-2p_1p_3+p_2^2}{\omega_0^6+(p_1^2-2p_2)\omega_0^4+(p_2^2-2p_1p_3)\omega_0^2+p_3^2}, \end{equation*} |
and by (4.8),
\omega_0^6+(p_1^2-2p_2)\omega_0^4+(p_2^2-2p_1p_3)\omega_0^2+p_3^2 = \gamma^2\omega_0^4+2\gamma q_2\omega_0^2+q_1^2\omega_0^2+q_2^2. |
As a result,
\begin{equation*} \Big(Re (\frac{d\lambda}{ d\tau})^{-1}|_{\tau = \tau_0}\Big) = \frac{3\omega_0^4+(2p_1^2-4p_2-2\gamma^2)\omega_0^2-2\gamma q_2-q_1^2-2p_1p_3+p_2^2}{\gamma^2\omega_0^4+(2\gamma q_2+q_1^2)\omega_0^2+q_2^2}. \end{equation*} |
The denominator of the above expression is clearly positive, and the numerator is F^{\prime}(x_0) , where x_0 = \omega_0^2 and F is defined in (4.9). Since x_0 is a simple root, F^{\prime}(x_0)\neq0 , and the transversality condition is satisfied.
[1] |
Altman EI (1968) Financial ratios, discriminant analysis and the prediction of corporate bankruptcy. J Financ 23: 589-609. doi: 10.1111/j.1540-6261.1968.tb00843.x
![]() |
[2] |
Altman EI, Haldeman RG, Narayanan P (1977) ZETA analysis. A new model to identify bankruptcy risk of corporations. J Bank Financ 1: 29-54. doi: 10.1016/0378-4266(77)90017-6
![]() |
[3] |
Altman EI, Marco G, Varetto F (1994) Corporate distress diagnosis: Comparisons using linear discriminant analysis and neural networks (the Italian experience). J Bank Financ 18: 505-529. doi: 10.1016/0378-4266(94)90007-8
![]() |
[4] | Amatori F, Bugamelli M, Colli A (2013) Technology, firm size, and entrepreneurship. In: The Oxford Handbook of the Italian Economy Since Unification. |
[5] |
Back B, Laitinen T, Sere K (1996) Neural networks and genetic algorithms for bankruptcy predictions. Expert Syst Appl 11: 407-413. doi: 10.1016/S0957-4174(96)00055-3
![]() |
[6] |
Barboza F, Kimura H, Altman EI (2017) Machine learning models and bankruptcy prediction. Expert Syst Appl 83: 405-417. doi: 10.1016/j.eswa.2017.04.006
![]() |
[7] | Bartik AW, Bertrand M, Cullen ZB, et al. (2020) How are small businesses adjusting to Covid-19? Early evidence from a survey. Technical report, National Bureau of Economic Research. |
[8] |
Beaver WH (1966) Financial ratios as predictors of failure. J Accounting Res 4: 71-111. doi: 10.2307/2490171
![]() |
[9] | Blum M (1974) Failing company discriminant analysis. J Accounting Res 12: 1-25. |
[10] | Bosio E, Djankov S, Jolevski F, et al. (2020) Survival of Firms during Economic Crisis. Technical report, Policy Research Working Paper 9239. The World Bank. |
[11] |
Carletti E, Oliviero T, Pagano M, et al. (2020) The covid-19 shock and equity shortfall: Firm-level evidence from italy. Rev Corporate Finannc Stud 9: 534-568. doi: 10.1093/rcfs/cfaa014
![]() |
[12] | Chetty R, Friedman JN, Hendren N, et al. (2020) How Did COVID-19 and Stabilization Policies Affect Spending and Employment? A New Real-Time Economic Tracker Based on Private Sector Data. Technical report, NBER Working Paper 27431. |
[13] | Coibion O, Gorodnichenko Y, Weber M (2020) Labor markets during the Covid-19 crisis: A preliminary view. Technical report, NBER Working Paper 27017. |
[14] | Core F, De Marco F (2020) Public Guarantees for Small Businesses in Italy during COVID-19. Technical report, CEPR DP15799. |
[15] | Cox N, Ganong P, Noel P, et al. (2020) Initial impacts of the pandemic on consumer behavior: Evidence from linked income, spending, and savings data. University of Chicago, Becker Friedman Institute for Economics Working Paper, (2020-82). |
[16] |
Danenas P, Garsva G (2015) Selection of support vector machines based classifiers for credit risk domain. Expert Syst Appl 42: 3194-3204. doi: 10.1016/j.eswa.2014.12.001
![]() |
[17] |
Deakin EB (1972) A discriminant analysis of predictors of business failure. J Accounting Res 10: 167-179. doi: 10.2307/2490225
![]() |
[18] | Demmou L, Franco G, Calligaris S, et al. (2021) Liquidity shortfalls during the COVID-19 outbreak: Assessment and policy responses. Technical report, OECD Economics Department Working Papers, No. 1647. |
[19] |
Dingel JI, Neiman B (2020) How many jobs can be done at home? J Public Econ 189: 104-235. doi: 10.1016/j.jpubeco.2020.104235
![]() |
[20] |
Gordini N (2014) A genetic algorithm approach for SMEs bankruptcy prediction: Empirical evidence from Italy. Expert Syst Appl 41: 6433-6445. doi: 10.1016/j.eswa.2014.04.026
![]() |
[21] | Gourinchas PO, Kalemli-Özcan E, Penciakova V, et al. (2020) Covid-19 and SME failures. Technical report, NBER Working Paper 27877. |
[22] | Guerini M, Nesta L, Ragot X, et al. (2020) Firm liquidity and solvency under the Covid-19 lockdown in France. OFCE Policy Brief, 76. |
[23] |
King G, Zeng L (2001) Logistic regression in rare events data. Political Anal 9: 137-163. doi: 10.1093/oxfordjournals.pan.a004868
![]() |
[24] |
Kruppa J, Schwarz A, Arminger G, et al. (2013) Consumer credit risk: Individual probability estimates using machine learning. Expert Syst Appl 40: 5125-5131. doi: 10.1016/j.eswa.2013.03.019
![]() |
[25] |
Kumar PR, Ravi V (2007) Bankruptcy prediction in banks and firms via statistical and intelligent techniques-A review. Eur J Oper Res 180: 1-28. doi: 10.1016/j.ejor.2006.08.043
![]() |
[26] | Ohlson JA (1980) Financial ratios and the probabilistic prediction of bankruptcy. J Accounting Res, 109-131. |
[27] | Schivardi F, Romano G (2020) A simple method to estimate firms' liquidity needs during the Covid-19 crisis with an application to Italy. Technical report, CEPR Issue 35. |
[28] |
Son H, Hyun C, Phan D, et al. (2019) Data analytic approach for bankruptcy prediction. Expert Syst Appl 138: 112816. doi: 10.1016/j.eswa.2019.07.033
![]() |
[29] |
Tsai CF, Wu JW (2008) Using neural network ensembles for bankruptcy prediction and credit scoring. Expert Syst Appl 34: 2639-2649. doi: 10.1016/j.eswa.2007.05.019
![]() |
[30] |
Wang G, Ma J, Yang S (2014) An improved boosting based on feature selection for corporate bankruptcy prediction. Expert Syst Appl 41: 2353-2361. doi: 10.1016/j.eswa.2013.09.033
![]() |
[31] |
Zelenkov Y, Fedorova E, Chekrizov D (2017) Two-step classification method based on genetic algorithm for bankruptcy forecasting. Expert Syst Appl 88: 393-401. doi: 10.1016/j.eswa.2017.07.025
![]() |
[32] |
Zhang G, Hu MY, Patuwo BE, et al. (1999) Artificial neural networks in bankruptcy prediction: General framework and cross-validation analysis. Eur J Oper Res 116: 16-32. doi: 10.1016/S0377-2217(98)00051-4
![]() |
[33] |
Zhao D, Huang C, Wei Y, et al. (2017) An effective computational model for bankruptcy prediction using kernel extreme learning machine approach. Comput Econ 49: 325-341. doi: 10.1007/s10614-016-9562-7
![]() |
![]() |
![]() |
Boundary equilibrium (T_s, T_r, T_i, V, Z) | Existence | Stability |
E_0=(0, 0, 0, 0, 0) | Always | Unstable |
E_1=(\hat T_s, \hat T_r, 0, 0, 0) | Always | c > \beta q\hat T_s and \cfrac{\alpha K}{m+K} < \gamma |
E_2=(\bar T_s, \bar T_r, 0, 0, \bar Z) | \cfrac{\alpha K}{m+K} > \gamma | (3.11) and (3.16) |
E_3=(T_s^*, T_r^*, T_i^*, V^*, 0) | c < \beta q \hat T_s | (3.20) |
Parameter | Description | Range | Reference |
K | Carrying capacity of tumor | 10^8 – 9.7\times 10^9 cells | [19,22] |
r_s | Rate of growth of sensitive tumor cells | 0.18 – 0.97 day ^{-1} | [18,19,36,37] |
\beta | Virus transmission rate | 6\times 10^{-12} – 0.862 pfu ^{-1} day ^{-1} | [22,38] |
r_r | Growth rate of resistant tumor cells | r_s\times [0.01 \ 0.97] day ^{-1} | [8,39] |
b | Death rate of infected tumor cells | 1.333 – 2.667 day ^{-1} | [16] |
q | Virus burst size per infected tumor cell | 10 – 1350 pfu cell ^{-1} | [16] |
c | Viral clearance rate | 0.024 – 24 day ^{-1} | [40] |
a | Rate of mutation of sensitive cancer cells | 10^{-9} – 10^{-3} day ^{-1} | [12,41] |
d | Rate of transition from resistance to sensitivity | 0-1 day ^{-1} | guess |
[1ex] m | Half-saturation constant of immune cell proliferation rate | 40-10^5 cells | [16,22] |
\gamma | Death rate of immune cells | 0.024 – 0.178 day ^{-1} | [22] |
\alpha | Maximal immune cell proliferation rate | 0.024 – 2.4 day ^{-1} | [22] |
k_s | Killing rate of susceptible tumor cells | 10^{-5} – 10^{-3} cell ^{-1} day ^{-1} | [17] |
k_r | Killing rate of resistant tumor cells | 10^{-4} – 10^{-2} cell ^{-1} day ^{-1} | guess |
k_i | Killing rate of infected tumor cells | 9.6\times 10^{-3} – 4.8 cell ^{-1} day ^{-1} | [16,22] |
k_v | Killing rate of viruses | 0.024-48 cell ^{-1} day ^{-1} | [22] |
\tau | Delay in the activation of immune cells | 1–8 days | [42,43] |
Therapy | Delay | Number of | Efficacy |
Strategy | 0 \leq \tau \leq 7 | CAR T cells | Number of Tumor cells |
OVT | \tau \geq 0 | — | Ineffective (Figures 7 and 8) |
CAR T cell | \tau \geq 0 | 8000 | Ineffective (Figure 9(b)) |
CAR T cell+OVT | \tau = 0 | 8000 | 10^8 |
CAR T cell+OVT | \tau \geq 4 | 5000 | 10^9 |
CAR T cell+OVT+CD7 | \tau=0 | 8000 | 10^9 |
CAR T cell+OVT+CD7 | \tau \geq 4 | 5000 | 10^9 |
Boundary equilibrium (T_s, T_r, T_i, V, Z) | Existence | Stability |
E_0=(0, 0, 0, 0, 0) | Always | Unstable |
E_1^0=(0, K, 0, 0, 0) | Always | \cfrac{\alpha K}{m+K} < \gamma |
[1ex] E_2^0=(0, \xi, 0, 0, \tilde Z^0) | \cfrac{\alpha K}{m+K} > \gamma | (A.2) |
[1.8ex] E_3^0=(\bar T_s^0, \bar T_r^0, 0, 0, \bar Z^0) | (A.3) | (3.11)* & (3.16)* |
Boundary equilibrium (T_s, T_i, V, Z) | Existence | Stability |
E_0=(0, 0, 0, 0) | Always | Unstable |
\tilde E_1=(K, 0, 0, 0) | Always | \beta qK < c and \cfrac{\alpha K}{m+K} < \gamma |
[1ex] \tilde E_2=(\xi, 0, 0, \tilde Z) | \cfrac{\alpha K}{m+K} > \gamma | (A.7) |
[1.8ex] \tilde E_3=(\tilde T_s, \tilde T_i, \tilde V, 0) | \beta qK > c | (A.9) |
Boundary equilibrium (T_s, T_r, T_i, V, Z) | Existence | Stability |
E_0=(0, 0, 0, 0, 0) | Always | Unstable |
E_1=(\hat T_s, \hat T_r, 0, 0, 0) | Always | c > \beta q\hat T_s and \cfrac{\alpha K}{m+K} < \gamma |
E_2=(\bar T_s, \bar T_r, 0, 0, \bar Z) | \cfrac{\alpha K}{m+K} > \gamma | (3.11) and (3.16) |
E_3=(T_s^*, T_r^*, T_i^*, V^*, 0) | c < \beta q \hat T_s | (3.20) |
Parameter | Description | Range | Reference |
K | Carrying capacity of tumor | 10^8 – 9.7\times 10^9 cells | [19,22] |
r_s | Rate of growth of sensitive tumor cells | 0.18 – 0.97 day ^{-1} | [18,19,36,37] |
\beta | Virus transmission rate | 6\times 10^{-12} – 0.862 pfu ^{-1} day ^{-1} | [22,38] |
r_r | Growth rate of resistant tumor cells | r_s\times [0.01 \ 0.97] day ^{-1} | [8,39] |
b | Death rate of infected tumor cells | 1.333 – 2.667 day ^{-1} | [16] |
q | Virus burst size per infected tumor cell | 10 – 1350 pfu cell ^{-1} | [16] |
c | Viral clearance rate | 0.024 – 24 day ^{-1} | [40] |
a | Rate of mutation of sensitive cancer cells | 10^{-9} – 10^{-3} day ^{-1} | [12,41] |
d | Rate of transition from resistance to sensitivity | 0-1 day ^{-1} | guess |
[1ex] m | Half-saturation constant of immune cell proliferation rate | 40-10^5 cells | [16,22] |
\gamma | Death rate of immune cells | 0.024 – 0.178 day ^{-1} | [22] |
\alpha | Maximal immune cell proliferation rate | 0.024 – 2.4 day ^{-1} | [22] |
k_s | Killing rate of susceptible tumor cells | 10^{-5} – 10^{-3} cell ^{-1} day ^{-1} | [17] |
k_r | Killing rate of resistant tumor cells | 10^{-4} – 10^{-2} cell ^{-1} day ^{-1} | guess |
k_i | Killing rate of infected tumor cells | 9.6\times 10^{-3} – 4.8 cell ^{-1} day ^{-1} | [16,22] |
k_v | Killing rate of viruses | 0.024-48 cell ^{-1} day ^{-1} | [22] |
\tau | Delay in the activation of immune cells | 1–8 days | [42,43] |
Therapy | Delay | Number of | Efficacy |
Strategy | 0 \leq \tau \leq 7 | CAR T cells | Number of Tumor cells |
OVT | \tau \geq 0 | — | Ineffective (Figures 7 and 8) |
CAR T cell | \tau \geq 0 | 8000 | Ineffective (Figure 9(b)) |
CAR T cell+OVT | \tau = 0 | 8000 | 10^8 |
CAR T cell+OVT | \tau \geq 4 | 5000 | 10^9 |
CAR T cell+OVT+CD7 | \tau=0 | 8000 | 10^9 |
CAR T cell+OVT+CD7 | \tau \geq 4 | 5000 | 10^9 |
Boundary equilibrium (T_s, T_r, T_i, V, Z) | Existence | Stability |
E_0=(0, 0, 0, 0, 0) | Always | Unstable |
E_1^0=(0, K, 0, 0, 0) | Always | \cfrac{\alpha K}{m+K} < \gamma |
[1ex] E_2^0=(0, \xi, 0, 0, \tilde Z^0) | \cfrac{\alpha K}{m+K} > \gamma | (A.2) |
[1.8ex] E_3^0=(\bar T_s^0, \bar T_r^0, 0, 0, \bar Z^0) | (A.3) | (3.11)* & (3.16)* |
Boundary equilibrium (T_s, T_i, V, Z) | Existence | Stability |
E_0=(0, 0, 0, 0) | Always | Unstable |
\tilde E_1=(K, 0, 0, 0) | Always | \beta qK < c and \cfrac{\alpha K}{m+K} < \gamma |
[1ex] \tilde E_2=(\xi, 0, 0, \tilde Z) | \cfrac{\alpha K}{m+K} > \gamma | (A.7) |
[1.8ex] \tilde E_3=(\tilde T_s, \tilde T_i, \tilde V, 0) | \beta qK > c | (A.9) |