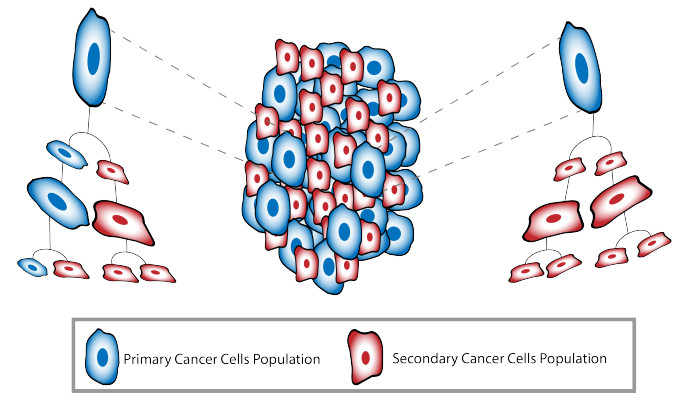
Pakistan's political instability has pushed its economic system to the brink of collapse. Considering this political turmoil, this study addresses the behavior of liquidity providers against microblogging-opinionated information. The behavioral perspective was quantified through multiple linear regressions, the Bayesian theorem, and the vector error correction technique. Before this political crisis, sentiment indicators were linked to the liquidity-conditional cost for the same trading session. In the political uncertainty environment, pessimistic opinions were the sole concern of the liquidity providers during the same trading session. The liquidity facilitator was observed to price the liquidity in light of pessimistic sentiments. The Bayesian theorem suggested a higher posterior probability for the occurrence of the liquidity-facilitating cost in response to the pessimistic sentiments. Nevertheless, the past time series changes for the sentiment indicators were irrelevant in determining changes in cost-based liquidity for the next trading session.
Citation: Jawad Saleemi. Political-obsessed environment and investor sentiments: pricing liquidity through the microblogging behavioral perspective[J]. Data Science in Finance and Economics, 2023, 3(2): 196-207. doi: 10.3934/DSFE.2023012
[1] | Xiangfei Meng, Guichen Zhang, Qiang Zhang . Robust adaptive neural network integrated fault-tolerant control for underactuated surface vessels with finite-time convergence and event-triggered inputs. Mathematical Biosciences and Engineering, 2023, 20(2): 2131-2156. doi: 10.3934/mbe.2023099 |
[2] | Qiushi Wang, Hongwei Ren, Zhiping Peng, Junlin Huang . Dynamic event-triggered consensus control for nonlinear multi-agent systems under DoS attacks. Mathematical Biosciences and Engineering, 2024, 21(2): 3304-3318. doi: 10.3934/mbe.2024146 |
[3] | Mingxia Gu, Zhiyong Yu, Haijun Jiang, Da Huang . Distributed consensus of discrete time-varying linear multi-agent systems with event-triggered intermittent control. Mathematical Biosciences and Engineering, 2024, 21(1): 415-443. doi: 10.3934/mbe.2024019 |
[4] | Duoduo Zhao, Fang Gao, Jinde Cao, Xiaoxin Li, Xiaoqin Ma . Mean-square consensus of a semi-Markov jump multi-agent system based on event-triggered stochastic sampling. Mathematical Biosciences and Engineering, 2023, 20(8): 14241-14259. doi: 10.3934/mbe.2023637 |
[5] | Wangming Lu, Zhiyong Yu, Zhanheng Chen, Haijun Jiang . Prescribed-time cluster practical consensus for nonlinear multi-agent systems based on event-triggered mechanism. Mathematical Biosciences and Engineering, 2024, 21(3): 4440-4462. doi: 10.3934/mbe.2024196 |
[6] | Siyu Li, Shu Li, Lei Liu . Fuzzy adaptive event-triggered distributed control for a class of nonlinear multi-agent systems. Mathematical Biosciences and Engineering, 2024, 21(1): 474-493. doi: 10.3934/mbe.2024021 |
[7] | Jiangtao Dai, Ge Guo . A leader-following consensus of multi-agent systems with actuator saturation and semi-Markov switching topologies. Mathematical Biosciences and Engineering, 2024, 21(4): 4908-4926. doi: 10.3934/mbe.2024217 |
[8] | Vladimir Djordjevic, Hongfeng Tao, Xiaona Song, Shuping He, Weinan Gao, Vladimir Stojanovic . Data-driven control of hydraulic servo actuator: An event-triggered adaptive dynamic programming approach. Mathematical Biosciences and Engineering, 2023, 20(5): 8561-8582. doi: 10.3934/mbe.2023376 |
[9] | Bin Hang, Beibei Su, Weiwei Deng . Adaptive sliding mode fault-tolerant attitude control for flexible satellites based on T-S fuzzy disturbance modeling. Mathematical Biosciences and Engineering, 2023, 20(7): 12700-12717. doi: 10.3934/mbe.2023566 |
[10] | Jiao Wu, Shi Qiu, Ming Liu, Huayi Li, Yuan Liu . Finite-time velocity-free relative position coordinated control of spacecraft formation with dynamic event triggered transmission. Mathematical Biosciences and Engineering, 2022, 19(7): 6883-6906. doi: 10.3934/mbe.2022324 |
Pakistan's political instability has pushed its economic system to the brink of collapse. Considering this political turmoil, this study addresses the behavior of liquidity providers against microblogging-opinionated information. The behavioral perspective was quantified through multiple linear regressions, the Bayesian theorem, and the vector error correction technique. Before this political crisis, sentiment indicators were linked to the liquidity-conditional cost for the same trading session. In the political uncertainty environment, pessimistic opinions were the sole concern of the liquidity providers during the same trading session. The liquidity facilitator was observed to price the liquidity in light of pessimistic sentiments. The Bayesian theorem suggested a higher posterior probability for the occurrence of the liquidity-facilitating cost in response to the pessimistic sentiments. Nevertheless, the past time series changes for the sentiment indicators were irrelevant in determining changes in cost-based liquidity for the next trading session.
The beginning of a primary solid tumour is the result of a single normal cell that is transformed as a result of mutations in certain key genes. Cells can mutate spontaneously, or mutations can be environmentally induced. Mutations occur during cell division, and most of the time the immune system can recognise mutated cells and eliminate them. When the immune system fails to eliminate cells with mutations in genes that control cell proliferation, cells become cancerous. It is known that all cancer cell lines have at least one mutation, with most cancer cell lines having more than one mutation; e.g., in [1] 137 oncogenic mutations were identified in 14 out of 24 known cancer genes in 60 human cancer cell lines. Moreover, cancer cells are genetically unstable and cells inside the solid tumours keep mutating leading to very heterogeneous tumour masses.
The mechanisms behind the mutation pressure are still not fully understood. Experimental studies have shown that some changes in the extracellular matrix (ECM) can correlate with sustained cell proliferative signalling and an increased risk of developing cancer [2]. Other studies have shown that culturing cells for long times in stiff hydrogels can lead to the subclonal selection of genomic aberrations in cells [3], thus suggesting that the ECM properties could impact the mutation status of cells in solid tumours. Other studies suggested that the maintenance of cells at high density in the absence of proliferation leads to an increase in mutagenesis following cell division [4]. Therefore, there seem to be different mechanisms that can trigger and influence the mutation rate of cells.
Mutated cells not only incur sustained proliferation (see Figure 1), but can also exhibit migrational and invasion properties [5], eventually leading to cancer metastasis. The invasion of surrounding tissue is the result of ECM degradation and remodelling by the cancer cells (which can secrete various proteolytic enzymes, such as matrix metalloproteinases (MMPs)) as well as other cells in the microenvironment. The last decades have seen the development of numerous theoretical studies based on mathematical models, which investigate computationally the biological mechanisms behind cancer cell invasion into the tissue. Most of these models are single-scale models; see [6,7,8,9,10,11] and references therein. More recently, multi-scale mathematical models have started to be developed to consider also the multi-scale aspects of various biological processes occurring during cancer invasion [12,13,14,15,16].
The main issue faced by all these single-scale and especially multi-scale mathematical models for cancer growth and invasion is parameter estimation. The last few years have seen the publication of various mathematical studies that try to estimate numerically different model parameters using inverse problem formulations [17,18,19,20,21]. These studies focus on identifying the magnitude of isotropic [21] and anisotropic tumour diffusion [18], the magnitude of tumour growth rate [19,21], the strength/location of tumour-induced tissue deformation [19], the position of the blood vessels that act as a source for the oxygen concentration that influences tumour growth [17], the location of the source of tumours [20].
To our knowledge, no studies have tried to estimate the mutation laws for cells inside heterogeneous tumours. To address this aspect, in this current study we consider a simplified problem with only two cancer cell populations (one mutating into the second one), that can exhibit both random and directed haptotactic movement. Moreover, we assume that the cells can degrade and remodel the surrounding ECM density. Furthermore, the type of interactions among cancer cells and between cells and ECM is not always very clear: some simpler mathematical models for cancer growth and invasion consider local cell-cell and cell-ECM interactions [8,9], while other more complex models consider non-local cell-cell and cell-ECM interactions [11,16,22,23]. In this study, we consider both type of models, local and non-local, and estimate the mutation laws for both cases. Finally, we assume here the knowledge of additional information in terms of both exact and noisy measurements of the tumour constituent density at some later time in the tumour evolution. We test our inversion approach on several cancer proliferation laws that are usually used in cancer modelling: logistic and Gompertz proliferation.
This paper is structured as follows. In Section 2 we describe the local mathematical model for the dynamics of the two cancer cell sub-populations and the extracellular matrix (ECM). In Sections 3 and 3.1 we formulate the inverse problem for this local model under the assumptions of logistic cell proliferation and mutation depending only on cancer cell density. In Section 3.4 we formulate the inverse problem for this local model under the assumption of logistic cell proliferation and mutation depending on both cell and ECM density. In Section 4 we describe a non-local model for two cancer cell populations and their interactions with the ECM, while in Section A we present the numerical approach for this forward nonlocal model. In Section 4.1 we formulate the inverse problem for this nonlocal model for cancer invasion. We summarise and discuss our results in Section 5.
In this study we consider two populations of tumour cells: a primary cell population c1(x,t) that can mutate into a more aggressive secondary cell population c2(x,t). These cell populations exercise a spatial redistribution via random movement (with diffusion coefficients D1 and D2) and directed movement towards extracellular matrix (ECM) gradients v(x,t) (with haptotactic coefficients η1 and η2). Moreover, the two populations undergo logistic growth (at the same rate μc), up to a carrying capacity (Kc). We also assume that the ECM undergoes degradation (at a rate ρ) and remodelling (at a rate μv). The above assumptions are described by the equations:
∂c1∂t=D1Δc1⏟diffusion−η1∇⋅(c1∇v)⏟haptotacticmovement+μcc1(1−c1+c2+vKc)⏟logisticproliferation−ω(t)⏟mutationswitchQ(⋅,⋅)⏟unknownmutation, | (2.1a) |
∂c2∂t=D2Δc2⏟diffusion−η2∇⋅(c2∇v)⏟haptotacticmovement+μcc2(1−c1+c2+vKc)⏟logisticproliferation+ω(t)⏟mutationswitchQ(⋅,⋅)⏟unknownmutation, | (2.1b) |
∂v∂t=−ρ(c1+c2)v⏟degradation+μv(Kc−v−c1−c2)+⏟remodellingterm. | (2.1c) |
where (Kc−v−c1−c2)+:=max{(Kc−v−c1−c2),0}. Finally, the unknown term Q(⋅,⋅) represents the mutation law of cell subpopulation c1 into cell subpopulation c2, which is assumed to be mediated by a time-dependant mutation enhancement ω(t) that is known a priori and is taken here of the form
ω(t):=(1+tanh(t−t1,2ts))2, |
where t1,2 is the time at which mutations from c1 to c2 start occurring, and ts>0 is a time-steepness coefficient for this mutation law.
The mutation law Q(⋅,⋅) is considerd here unknown due to either unknown dependance on the primary cell population c1, or unknown dependance on the ECM v, or unknown dependance on both primary tumour cell population and ECM. In this study we investigate three assumptions related to this mutation term, namely: (i) mutation depends linearly on the density of primary tumour cells as experimental studies have shown that tumour hypoxia, generated by high tumour cell density, is linearly correlated with an increase in genomic changes toward more aggressive tumours [24]; (ii) mutation depends linearly on the density of primary tumour cells, and nonlinearly on the ECM density [3]; (iii) mutation law is very general and depends autonomously on both the primary tumour and ECM, as suggested by various experimental studies regarding the role of the tumour microenvironment in cancer cells mutation process [3,24,25]. Thus mathematically, these cases correspond to three inverse problems that seek to identify the unknown mutation law Q(⋅,⋅) in the following three situations:
(i) Mutation depends linearly on the density of primary tumour cell sub-population c1 but does not depend at all on ECM, and so this is given by the unknown term
Q(c1,v):=˜Q1(c1)=δ0c1, | (2.2) |
with δ0 representing the unknown mutation rate.
(ii) Mutation depends in a known linear manner on c1 and in an unknown nonlinear way on the density of ECM. The unknown dependence on v is denoted mathematically by the unknown function ˜Q2(v), and so the entire mutation law is therefore of the form
Q(c1,v):=δ0c1˜Q2(v), | (2.3) |
with δ0>0 here being considered known. A usual choice for ˜Q2(v) is of the form [26,27]:
˜Q2(v):={exp(−1κ2−(1−v(x,t))2)exp(−1κ2)if1−κ<v(x,t)<1,0,otherwise, | (2.4) |
where κ>0 is a certain level of ECM beyond which mutations can occur.
(iii) Mutation is given by an unknown general nonlinear law Q(c1,v) that is autonomous in c1 and v, which will be reconstructed from the data available at a specific later time in the tumour evolution.
To complete the description of the model, we mention that the initial conditions for the cancer-ECM dynamics described by model (2.1) are:
c1(x,0):=c1,0(x),c2(x,0):=c2,0(x)andv(x,0):=v0(x),forx∈Ω. | (2.5) |
Here, c1,0(⋅), c2,0(⋅) and v0(⋅) give the initial distributions of the primary cell subpopulation, mutated cell subpopulation and ECM, respectively. Furthermore, we assume that the cells and the ECM components do not leave the tissue region Ω, and therefore we consider zero Neumann boundary conditions:
∂c1∂n|∂Ω=0,∂c2∂n|∂Ω=0and∂v∂n|∂Ω=0, | (2.6) |
where n(ξ) is the usual normal direction at any given tissue boundary point ξ∈∂Ω.
Throughout the following sections, we refer to the tumour dynamics model (2.1) together with the initial and boundary conditions (2.5) and (2.6) as the "forward model".
We start with the forward model defined by the tumour dynamics model (2.1) in the presence of the initial and boundary conditions (2.5) and (2.6). Our goal is to reconstruct the unknown cancer cells mutation law Q(⋅) from additional information given by measurements of the cancer cells and ECM densities taken at some later time tf:=T>0 in the tumour evolution. These measurements are therefore given in the form of the following functions on Ω, which are considered to be known:
c∗1(⋅):Ω→Rforthecancersubpopulationc1, | (3.1a) |
c∗2(⋅):Ω→Rforthecancersubpopulationc2, | (3.1b) |
v∗(⋅):Ω→RfortheECMdensity. | (3.1c) |
In the following, we will explore the reconstruction of the unknown cancer mutation law Q(⋅) when the known measurements c∗1(x), c∗2(x) and v∗(x) will be given both as exact (accurate) data and as noisy data, ∀x∈Ω.
In this section we outline in a unitary manner the forward solver involved in the retrieval of the mutation laws corresponding to Cases (i) and (ii) that require the retrieval of ˜Q1(c1) and ˜Q2(v), respectively. To that end, for r=1,2, denoting by er either the primary tumour or the ECM, i.e., er∈{c1,v}, enables us to proceed with addressing simultaneously both cases by simply referring to the retrieval of the term compactly denoted as ˜Qr(er) that is specified by
er:={c1,ifr=1,v,ifr=2,andsubsequently˜Qr(er):={˜Q1(c1),ifr=1,˜Q2(v),ifr=2. | (3.2) |
We start by considering a uniform discretisation GΩ:={(xi,yj)}i,j=1…N of step size Δx=Δy>0 for a square maximal tissue region Ω⊂R2 where the tumour exercises its dynamics. At any given time t∈[0,tf] the discretisations of cancer cells densities c1(⋅,t) and c2(⋅,t) as well as the density of ECM v(⋅,t) are therefore given by the N×N matrices ˜c1(t):={˜c1,i,j(t)}i,j=1…N, ˜c2(t):={˜c2,i,j(t)}i,j=1…N and ˜v(t):={˜vi,j(t)}i,j=1…N, with ˜c1,i,j(t):=c1((xi,yj),t), ˜c2,i,j(t):=c2((xi,yj),t) and ˜vi,j(t):=v((xi,yj),t), ∀i,j=1…N. Correspondingly, in the following ˜er(t) will denote either ˜c1(t) or ˜v(t), i.e., ˜er(t)∈{˜c1(t),˜v(t)}, as required by model (3.2).
Throughout this study we assume that we have a priori knowledge that the cumulated ECM and cancer densities do not exceed the tissue carrying capacity Kc. Under this assumption, the unknown mutation law can be written in terms of an unknown (for the moment) function mc∗1,c∗2,v∗:[0,Kc]→[0,∞). Moreover, this unknown function mc∗1,c∗2,v∗ will be appropriately identified within a suitable family of functions M1 such that the corresponding solution for the tumour model (2.1) will match the measurements given in model (3.1). Thus, denoting by ˜Qc∗1,c∗2,v∗r(⋅) the unknown mutation term for which the corresponding solution of model (2.1) matches measurement model (3.1), at each (xi,yj) we can write this as
˜Qc∗1,c∗2,v∗r(˜emc∗1,c∗2,v∗r,i,j(t)):=M1i,j(˜emc∗1,c∗2,v∗r(t),mc∗1,c∗2,v∗), |
where M1(⋅,⋅):={M1i,j(⋅,⋅)}i,j=1…N, with M1(⋅,⋅):RN×N×M1→RN×N representing a "trial mutation operator" that will be specified below alongside the family of functions M1. Furthermore, ˜emc∗1,c∗2,v∗r(t):={˜emc∗1,c∗2,v∗r,i,j(t)}i,j=1..N represents the solution for the density of either the primary cell population (if r=1) or the ECM (if r=2) that is obtained for model (2.1) when, instead of the unknown term ˜Qr(er), in the mutation law we use the trial mutation term M1(⋅,mc∗1,c∗2,v∗).
Next, we consider an uniform discretisation for the domain [0,Kc] that is given by an equally spaced grid G1M:={ηl}l=1…M of step size Δη>0. On this discretised domain, the unknown function mc∗1,c∗2,v∗ is identified through a suitable approximation within the following M−dimensional space of functions associated with G1M, namely
M1:={m:[0,Kc]→R|m|El=∑p=0,1m(ηl+p)ϕl+p,∀El∈G1,tilesM}withthefamilyofintervalsG1,tilesM:={El:=[ηl,ηl+1]|l=1…M−1},and∀El∈G1,tilesM,{ϕl+p}p=0,1describetheusuallinearshapefunctionsonEl. | (3.3) |
Thus, for any candidate function m∈M1, the corresponding trial mutation operator M1 has each of its components Mi,j, ∀i,j=1…N, given by
M1i,j(˜emr(t),m):=m|El(˜emr,i,j(t)),withindexlbeingindependentofitschoicewithintheassociatedsetofindicesΛi,j,namely:Λi,j:={l∈{1,…,M−1}|∃El∈G1,tilesMsuchthat˜emr,i,j(t)∈El}. | (3.4) |
Here, as per model (3.2), ˜emr(t):={˜emri,j(t)}i,j=1…N represents either the solutions for the density of primary cancer cell population, {˜cm1,i,j(t)}i,j=1…N, or for the density of ECM, {˜vmi,j(t)}i,j=1…N, which alongside the density of mutated cell population, {˜cm1,i,j(t)}i,j=1…N, are obtained with model (2.1) when this uses within the mutation law the trial mutation term M1(⋅,m):={M1i,j(⋅,m)}i,j=1…N given in model (3.4) instead of the unknown term ˜Qr(⋅). Finally, the trial mutation form for the full mutation law given in models (2.2) and (2.3) for Cases (i) and (ii), respectively, is denoted by ¯Mr(˜cm1(t),˜vm(t),m):={¯Mr,i,j(˜cm1(t),˜vm(t),m)}i.j=1…N and is given by:
¯Mr,i,j(˜cm1(t),˜vm(t),m):={M1i,j(˜cm1(t),m),ifr=1,δ0˜cm1,i,j(t)M1i,j(˜vm(t),m),ifr=2. | (3.5) |
Therefore, in space-discretised form, model (3.4) that uses M1(⋅,m) can therefore be written as
∂∂t[˜cm1˜cm2˜vm]=[H1(˜cm1,cm2,˜vm,m)H2(˜cm1,cm2,˜vm,m)H3(˜cm1,cm2,˜vm)], | (3.6) |
Here, H1(⋅,⋅,⋅,⋅)={H1i,j(⋅,⋅,⋅,⋅)}i,j=1…N represents the spatial discretisation corresponding to Eq (2.1a). Each of its components H1i,j(⋅,⋅,⋅,⋅), ∀i,j=1…N, are given by
H1i,j(˜cm1(t),˜cm2(t),˜vm(t),m):=D1(Δx)2(˜cm1,i−1,j(t)+˜cm1,i+1,j(t)+˜cm1,i,j−1(t)+˜cm1,i,j+1(t)−4˜cm1,i,j(t))−η12(Δx)2((˜cm1,i,j(t)+˜cm1,i+1,j(t))(˜vmi+1,j(t)−˜vmi,j(t))−(˜cm1,i,j(t)+˜cm1,i−1,j(t))(˜vmi,j(t)−˜vmi−1,j(t))+(˜cm1,i,j(t)+˜cm1,i,j+1(t))(˜vmi,j+1(t)−˜vmi,j(t))−(˜cm1,i,j(t)+˜cm1,i,j−1(t))(˜vmi,j(t)−˜vmi,j−1(t)))+μc˜cm1,i,j(t)(Kc−˜cm1,i,j(t)−˜cm2,i,j(t)−˜vmi,j(t))−ω(t)¯Mr,i,j(˜cm1(t),˜vm(t),m). | (3.7) |
Similarly, H2(⋅,⋅,⋅,⋅)={H2i,j(⋅,⋅,⋅,⋅)}i,j=1…N represents the spatial discretisation corresponding to Eq (2.1d), and each of its components H2i,j(⋅,⋅,⋅,⋅), ∀i,j=1…N, are given by
H2i,j(˜cm2(t),˜cm2(t),˜vm(t),m):=D2(Δx)2(˜cm2,i−1,j(t)+˜cm2,i+1,j(t)+˜cm2,i,j−1(t)+˜cm2,i,j+1(t)−4˜cm2,i,j(t))−η22(Δx)2((˜cm2,i,j(t)+˜cm2,i+1,j(t))(˜vmi+1,j(t)−˜vmi,j(t))−(˜cm2,i,j(t)+˜cm2,i−1,j(t))(˜vmi,j(t)−˜vmi−1,j(t))+(˜cm2,i,j(t)+˜cm2,i,j+1(t))(˜vmi,j+1(t)−˜vmi,j(t))−(˜cm2,i,j(t)+˜cm2,i,j−1(t))(˜vmi,j(t)−˜vmi,j−1(t)))+μc˜cm2,i,j(t)(Kc−˜cm1,i,j(t)−˜cm2,i,j(t)−˜vmi,j(t))+ω(t)¯Mr,i,j(˜cm1(t),˜vm(t),m). | (3.8) |
Finally, H3(⋅,⋅,⋅)={H3i,j(⋅,⋅,⋅)}i,j=1…N represents the discretisation of the ECM Eq (2.1g), and each of its components H3i,j(⋅,⋅), ∀i,j=1…N, are given by
H3i,j(˜cm1(t),˜cm2(t),˜vm(t)):=−ρ(˜cm1,i,j(t)+˜cm2,i,j(t))˜vmi,j(t)+μv(Kc−˜cm1,i,j(t)−˜cm2,i,j(t)−˜vmi,j(t))+. | (3.9) |
Consider now a time discretisation {tn}n=0…L with time step Δt:=T/L. For each n∈{0,…,L}, a simple Euler time-marching scheme can be written for model (3.6) via the associated operator
Km:RN×N×RN×N×RN×N→RN×N×RN×N×RN×NgivenbyKm([˜cm,n1˜cm,n2˜vm,n]):=[˜cm,n1˜cm,n2˜vm,n]+Δt[H1(˜cm,n1,˜cm,n2,˜vm,n,m)H2(˜cm,n1,˜cm,n2,˜vm,n,m)H3(˜cm,n1,˜cm,n2,˜vm,n)], | (3.10) |
where ˜cm,n1:=˜cm1(tn), ˜cm,n2:=˜cm2(tn) and ˜vm,n:=˜vm(tn). The right-hand-side operators are given by
H1(˜cm,n1,˜cm,n2,˜vm,n,m):=H1(˜cm1(tn),˜cm2(tn),˜vm(tn),m),H2(˜cm,n1,˜cm,n2,˜vm,n,m):=H2(˜cm1(tn),˜cm2(tn),˜vm(tn),m),H3(˜cm,n1,˜cm,n2,˜vm,n):=H3(˜cm1(tn),˜cm2(tn),˜vm(tn)). |
This allows us to formulate the "forward operator" K between the family of functions M1 (where we search for the appropriate cancer cells mutation function mc∗1,c∗2,v∗) and the space where the discretised measurements model (3.1) are recorded. Hence, the "forward operator" K is defined as
K:S→RN×N×RN×N×RN×NgivenbyK(m):=Km∘Km∘⋯⋯∘Km⏟Ltimes([˜c1,0˜c2,0˜v0]) | (3.11) |
where ˜c1,0:={c1,0(xi,yj)}i,j=1,…,N, ˜c2,0:={c2,0(xi,yj)}i,j=1,…,N and ˜v0:={v0(xi,yj)}i,j=1,…,N are the discretised initial conditions (2.5) for the governing tumour forward model (2.1). Hence, for each m∈M1, the forward operator K gives the spatio-temporal progression of the initial condition [˜c1,0,˜c2,0,˜v0]T under the invasion model (2.1), which is obtained when the cell mutation law at each instance of time t>0 involves the corresponding trial mutation operator M(⋅,m) instead of the unknown mutation terms ˜Qr(⋅).
From models (3.10) and (3.11) we have that our forward operator K is given as a finite composition of affine functions of the form
M1∋m⟼Km∈ℓ2(ℓ2(E×E×E);ℓ2(E×E×E)). | (3.12) |
Here, ℓ2(ℓ2(E×E×E);ℓ2(E×E×E)) is the usual finite-dimensional Bochner space of square integrable vector-value functions [28] with respect to the counting measure (see [29]) that are defined on ℓ2(E×E×E) and take values in ℓ2(E×E×E), and E:={Ei,j}i,j=1…N represents the standard basis of elementary matrices associated with the grid GΩ. As a direct consequence, we immediately obtain that this operator is both continuous and compact, from where we obtain that K is also closed and sequentially bounded [28]. Therefore, K satisfies the hypotheses assumed in [30] that ensure convergence for the nonlinear Tikhonov regularisation strategy given by the functionals {Jα}α>0,
Jα:M1→R,∀α>0,definedbyJα(m):=‖K(m)−[˜c∗1˜c∗2˜v∗]‖22+α‖m‖22,∀m∈M1. | (3.13) |
The minimisation of these functionals enable us to identify mc∗1,c∗2,v∗ as the limit α→0 of the points of minimum mα of Jα (these points correspond to the smallest discrepancy between the data measurements and the solution of our system that uses mα as a mutation law). The two norms involved in model (3.13) represent the usual Euclidean norms on the corresponding finite dimensional spaces. Indeed, while the first is the standard Euclidean norm on RN×N×RN×N×RN×N, the second is also the Euclidean norm induced on the M−dimensional space of functions M1 via the standard isomorphism that we establish between M1 and RM by which each m∈M1 is uniquely represented through its nodal values {m(ηl)}l=1…M with respect to the linear basis functions {ˉϕl}l=1…M associated to GM [31]:
sincem=∑l=1…Mm(ηl)ˉϕl,wethereforemaketheidentification:m≡{m(ηl)}l=1…M. | (3.14) |
Finally, in model (3.13), ˜c∗1, ˜c∗2 and ˜v∗ represent the discretised measurements of the densities of cancer cells and ECM given in Eqs (3.1a)–(3.1c), i.e., ˜c∗1:={c∗1(xi,yj)}i,j=1,…,N, ˜c∗2:={c∗2(xi,yj)}i,j=1,…,N and ˜v∗:={v∗(xi,yj)}i,j=1,…,N. We assume that these data measurements are either exact or are corrupted by a certain noise level δ≥0. Thus, maintaining for simplicity the measurements notation unchanged, these measurements are given by
˜c∗1(x)=˜c∗1exact(x)+δγc1(x), | (3.15a) |
˜c∗2(x)=˜c∗2exact(x)+δγc2(x), | (3.15b) |
˜v∗(x)=˜v∗exact(x)+δγv(x), | (3.15c) |
where, ∀x∈Ω, we have that ˜c∗1exact(x), ˜c∗2exact(x) and ˜v∗exact(x) describe the exact data, and γc1(x), γc2(x) and γv(x) are signal-independent noise generated from a Gaussian normal distribution with mean zero and standard deviations σc1, σc2 and σv, respectively, given by
{σc1:=1λ(Ω)∫Ω˜c∗1exact(x)dx,σc2:=1λ(Ω)∫Ω˜c∗2exact(x)dx,σv:=1λ(Ω)∫Ω˜v∗exact(x)dx, | (3.16) |
with λ(⋅) being the usual Lebesgue measure. In the numerical results below, we generate the random variables γc1(x), γc2(x) and γv(x) via MATLAB function normrnd by taking {γc1(xi,yj)}i,j=1…N:=normrnd(0,σc1,N×N), {γc2(xi,yj)}i,j=1…N:=normrnd(0,σc2,N×N) and {γv(xi,yj)}i,j=1…N:=normrnd(0,σv,N×N).
We explore now the inversion approach that we formulated so far in the context of the forward model (2.1) by proceeding with the reconstruction of the unknown terms involved in the mutation laws in Cases (i) and (ii), namely ˜Q1(c1) and ˜Q2(v), respectively.
Initial Conditions The initial conditions (2.5) that we consider in the computations for the forward model (2.1) are as follows:
c1,0(x):=0.5(exp(−‖x−(2,2)‖220.03)−exp(−9.407)), | (3.17a) |
c2,0(x):=0, | (3.17b) |
v0(x):=0.5+0.3⋅sin(4π⋅‖x‖2),∀x∈Ω. | (3.17c) |
Here, we assume that c2,0(x)=0 because this second cell population will arise after a period of time following mutations of the first cell population c1.
To identify the cancer cells mutation law, we consider both exact and noisy measurement data model (3.15) as additional information for the forward model (2.1) in the presence of initial conditions (3.17) and boundary conditions (2.6). Specifically, in each of the two cases, we assume that the exact data (namely ˜c∗1,exact(x), ˜c∗2,exact(x) and ˜v∗exact(x)) that appear in model (3.15) are given by the solutions densities for primary tumour cells population, ˉc1(x,t), mutated cells population, ˉc2(x,t), and ECM, and ˉv(x,t), evaluated at the final time tf>0, i.e.,
˜c∗1,exact(x):=ˉc1(x,tf),˜c∗2,exact(x):=ˉc2(x,tf)and˜v∗exact(x):=ˉv(x,tf),∀x∈Ω, | (3.18) |
which are obtained from the forward model (2.1) as follows:
● for Case (i): we assume that the mutation law is of the form given in model (2.2) but when parameter δ0>0 is considered known and has the value given in parameter Table 1;
Parameter | Value | Description | Reference |
D1 | 0.00675 | diffusion of primary tumour | [11] |
D2 | 0.00675 | diffusion of secondary tumour | [11] |
η1 | 2.85×10−2 | haptotaxis to ECM from c1 | [37] |
η2 | 2.85×10−2 | haptotaxis to ECM from c2 | [37] |
μc | 0.25 | proliferation of tumour cells c | [38] |
Kc | 1 | tissue carrying capacity | [16] |
ρ | 2 | ECM degradation coefficient | [39] |
μv | 0.40 | ECM remodelling coefficient | [16] |
Scc | (0.5000.3) | cell-cell adhesion function | [11] |
Scv | (0.1000.5) | cell matrix adhesion function | [11] |
t1,2 | 10 | time initiation for mutations | [38,40] |
ts | 3 | time-steepness coefficient | [38] |
δ0 | 0.3 | mutation from primary tumour | [38,40] |
Δx | 0.03125 | discretisation step size for GΩ | [16] |
Δt | 10−3 | time step size | [16] |
Δη | 0.0625 | mesh size used for G1M and G2M | Estimated |
● for Case (ii): we assume that the mutation law is of the form given by model (2.3) with the known term ˜Q2(v) specified in model (2.4).
For each regularisation parameter α>0 considered here, the minimisation process for Jα is initiated with m0=I×10−3, (where I represents the M vector of ones), and for the actual minimisation we employed here the nonlinear minimisation MATLAB function lsqnonlin. Finally, since there are no data to test the trial mutation operators beyond the maximal accessible region Ac and Av defined by the minimum and maximum values of the solution, i.e.,
Ac:=[ˉcmin1,ˉcmax1],with:ˉcmin1:=min | (3.19) |
\begin{equation} {\mathcal{A}}_{v}: = [\bar{v}^{min}, \bar{v}^{max}] , {\rm{with}}: \quad \bar{v}^{min} : = \min\limits_{\begin{array}{c} (x, t)\in \Omega \times [0, T]\\ \end{array}} v(x, t), \quad \quad \quad \bar{v}^{max} : = \max\limits_{\begin{array}{c} (x, t) \in \Omega \times [0, T]\\ \end{array}} v(x, t), \\ \end{equation} | (3.20) |
the reconstructions in this section will be attempted only for the restriction of the sought mutation laws to {\mathcal{A}}_{c} and {\mathcal{A}}_{v} . We need to emphasise that {\mathcal{A}}_{c} is used to reconstruct \widetilde{{\mathcal{Q}}}_{1}(c_{1}) , while {\mathcal{A}}_{v} is used to reconstruct \widetilde{{\mathcal{Q}}}_{2}(v) .
An acceptable numerical reconstruction of the mutation law {m}^{c_{1}^{*}, c_{2}^{*}, v^{*}} , i.e.,
\begin{equation} {m}^{c_{1}^{*}, c_{2}^{*}, v^{*}} : = m^{\alpha^{*}}, \end{equation} | (3.21) |
is obtained for the choice of the regularisation parameter \alpha^{*} , which throughout this work is selected based on a standard discrepancy principle argument [32].
Figure 2 shows the reconstruction of the cancer cell mutation law \widetilde{{\mathcal{Q}}}_{1}(c_{1}) for model (2.1) in the presence of the measurements given by models (3.15) and (3.18) that are considered here both exact and affected by a level of noise \delta\in\{1\%, 3\%\} . The first row of this figure shows from left to right the true mutation law restricted at the maximal accessible region {\mathcal{A}}_{c} (where the reconstruction is being attempted) and the reconstruction of the mutation law equation \widetilde{{\mathcal{Q}}}_{1}(c_{1}) given by model (2.2) on {\mathcal{A}}_{c} with no noise, respectively. The second row of the figure show from left to right the reconstruction of the mutation law on {\mathcal{A}}_{c} with 1\% , and 3\% of noise in the measured data, respectively.
Figure 3 shows the reconstruction of unknown nonlinear cancer cell mutation term \widetilde{Q}_{2}(v) (that is part of cell mutation law introduced in model (2.3)) for model (2.1) in the presence of the measurements given by models (3.15) and (3.18) that are considered here both exact and affected by a level of noise \delta\in\{1\%, 3\%\} . Again, the first row shows from left to right the true mutation law restricted to {\mathcal{A}}_{v} where the reconstruction is attempted, and the reconstruction of the mutation law model (2.3) on {\mathcal{A}}_{v} with no noise, respectively. The second row of the figure show from left to right the mutation reconstruction on {\mathcal{A}}_{v} with 1\% , and 3\% of noise in the measured data, respectively.
From Figures 2 and 3 we observe that when the measurement data is not affected by noise, we obtain good mutation laws reconstructions in both cases, i.e., (i) mutation depends on primary tumour only, and (ii) mutation depend on the ECM only. However, as expected, as soon as the level of noise in the measurements increases, the reconstruction gradually looses accuracy in both cases (i.e., Case (i) shown in Figure 2 lower panels, and Case (ii) shown in Figure 3 lower panels). This loss in accuracy increases with the increase in the c_{1} density (horizontal axis in Figure 2) and in the v density (horizontal axis in Figure 3).
In this section, we study the inverse problem of identifying the general mutation law in case (iii), namely {\mathcal{Q}}(c_{1}, v) , which is unknown and depends nonlinearly in an autonomous manner on both primary tumour cell density c_{1} and on ECM density v .
Maintaining here the same spatio-temporal discretisation notations introduced in Section 3.1 for the cancer cell populations and ECM, we seek to identify the unknown nonlinear mutation law {\mathcal{Q}}(c_{1}, v) such that we match the measurements model (3.1). To achieve this, similar to the previous two cases addressed in Sections 3.1-3.3, within an suitable space of functions {\mathfrak{M}}^{2} that will be detailed below, we proceed to identify an appropriate a mutation approximating function defined on the 2-dimensional region where the pair (c_{1}, v) ranges during its spatio-temporal evolution, namely \overline{m}^{\, c_{1}^{*}, c_{2}^{*}, v^{*}}:[0, K_{c}]\times[0, K_{c}]\to [0, \infty) . The function \overline{m}^{\, c_{1}^{*}, c_{2}^{*}, v^{*}} will be identified such that this will directly determine an acceptable "mutation law candidate", denoted here by {\mathcal{Q}}^{c_{1}^{*}, c_{2}^{*}, v^{*}}(\cdot, \cdot) , which will enable a solution in forward model (2.1) that matches the measurements model (3.1). To select this mutation law candidate {\mathcal{Q}}^{c_{1}^{*}, c_{2}^{*}, v^{*}}(\cdot, \cdot) , adopting a similar approach to the one in Cases (i) and (ii) , we involve again an appropriately constructed mutation trial operator {\mathcal{M}}^{2}(\cdot, \cdot, \cdot): = \{{\mathcal{M}}^{2}_{i, j}(\cdot, \cdot, \cdot)\}_{i, j = 1\dots N} , {\mathcal{M}}^{2}(\cdot, \cdot, \cdot):{\mathbb{R}}^{N\times N}\times {\mathbb{R}}^{N\times N}\times {\mathfrak{M}}^{2}\to {\mathbb{R}}^{N\times N} that will be specified in a moment and will enable us the express the acceptable mutation law candidate as
\begin{equation} {\mathcal{Q}}^{c_{1}^{*}, c_{2}^{*}, v^{*}}(\tilde c^{\overline{m}^{\, c_{1}^{*}, c_{2}^{*}, v^{*}}}_{1, i, j}(t), \tilde v^{\overline{m}^{\, c_{1}^{*}, c_{2}^{*}, v^{*}}}_{i, j}(t)): = {\mathcal{M}}^{2}_{i, j}(\tilde c^{\overline{m}^{\, c_{1}^{*}, c_{2}^{*}, v^{*}}}_{1}(t), \tilde v^{\overline{m}^{\, c_{1}^{*}, c_{2}^{*}, v^{*}}}(t), \overline{m}^{\, c_{1}^{*}, c_{2}^{*}, v^{*}}) \end{equation} | (3.22) |
where \tilde c^{\overline{m}^{\, c_{1}^{*}, c_{2}^{*}, v^{*}}}_{1}(t): = \{\tilde c^{\overline{m}^{\, c_{1}^{*}, c_{2}^{*}, v^{*}}}_{1}(t)\}_{i, j = 1\dots N} and \tilde v^{\overline{m}^{\, c_{1}^{*}, c_{2}^{*}, v^{*}}}(t): = \{\tilde v^{\overline{m}^{\, c_{1}^{*}, c_{2}^{*}, v^{*}}}(t)\}_{i, j = 1\dots N} represents the solution for the density of the primary cell population and of the ECM, respectively, which are obtained for model (2.1) when, instead of the unknown term {\mathcal{Q}}(\cdot, \cdot) , in the mutation law we use the trial mutation term {\mathcal{M}}^{2}(\cdot, \cdot, m^{c_{1}^{*}, c_{2}^{*}, v^{*}})
Denoting by {\mathcal{G}}^{2}_{_{M}}: = \{(\eta_{l}, \zeta_{k})\}_{l, k = 1\dots M} the equally spaced grid given by the uniform discretisation of the [0, K_{c}]\times[0, K_{c}] with step size \Delta \eta = \Delta \zeta > 0 , the space of functions where we seek to identify the function \overline{m}^{\, c_{1}^{*}, c_{2}^{*}, v^{*}} is an M\times M - dimensional space of potential mutation law shape candidates, which is given by
\begin{equation} \begin{split} {\mathfrak{M}}^{2}: = &\bigg\{\overline{m}:[0, K_{c}]\times[0, K_{c}]\to {\mathbb{R}}\, \bigg|\, \, \overline{m} \raise-.5ex\hbox{|}_{{E_{l, k}}} = \sum\limits_{p, q = 0, 1} \overline{m} (\eta_{l+p}, \zeta_{k+q}) \phi_{l+p, k+q}, \quad \forall E_{l, k}\in {\mathcal{G}}_{_{M}}^{2, \, tiles}\bigg\} \\ & {\rm{with}} \quad {\mathcal{G}}_{_{M}}^{2, \, tiles}: = \{ E_{l, k}: = [\eta_{l}, \eta_{l+1}]\times [\zeta_{k}, \zeta_{k+1}]\, |\, l, k = 1\dots M-1\}, \, \, and\\ & \quad \qquad \forall \, \, E_{l, k}\in {\mathcal{G}}_{_{M}}^{2, \, tiles}, \, \, \{\phi_{l+p, k+q}\}_{p, q = 0, 1} {\rm{\;are\;the\;usual\;bilinear\;shape\;functions\;on}}\; E_{l, k}. \end{split} \end{equation} | (3.23) |
Therefore, for any \overline{m}\in {\mathfrak{M}}^{2} , the trial proliferation operator {\mathcal{M}}^{2} is given by
\begin{equation} \begin{split} &\qquad\qquad {\mathcal{M}}^{2}_{i, j}(\tilde c^{\overline{m}}_{1}(t), \tilde v^{\overline{m}}(t), \overline{m}): = \overline{m}\raise-.5ex\hbox{|}_{{E_{l, k}}}(\tilde c^{\overline{m}}_{1}(t), \tilde v^{\overline{m}}(t))\\ & {\rm{with}}\;(l, k)\in \Lambda_{i, j}: = \left\{l, \, k \!\in\! \{1, \dots, M\!-\!1\}\, \big| \, \exists E_{l'\!, k'}\!\in{\mathcal{G}}_{_{M}}^{tiles}\;{\rm{ such\;that }}\;(\tilde c^{\overline{m}}_{1, i, j}(t), \tilde v^{\overline{m}}_{i, j}(t))\!\in\! E_{l'\!, k'} \!\right\}, \\ & {\rm{and\;noting\;also\;here\;that}}\;(l, k)\;{\rm{is\;independent\;of\;its\;choice\;within}}\;\Lambda_{i, j}. \end{split} \end{equation} | (3.24) |
Here, \tilde c^{\overline{m}}_{1}(t): = \{\tilde c^{\overline{m}}_{1, i, j}(t)\}_{i, j = 1\dots N} , \tilde c^{\overline{m}}_{2}(t): = \{\tilde c^{\overline{m}}_{2, i, j}(t)\}_{i, j = 1\dots N} and \tilde v^{\overline{m}}(t) : = \{\tilde v^{\overline{m}}_{i, j}(t)\}_{i, j = 1\dots N} represent the solutions at the grid points and time t > 0 for the cancer cells and ECM densities obtained with model (2.1) when this uses {\mathcal{M}}^{2}_{i, j}(\cdot, \cdot, \overline{m}) as mutation law given in model (3.24). Therefore, in space-discretised form, model (2.1) can be recasted also in this case as
\begin{equation} \frac{\partial}{\partial{t}} \begin{bmatrix} \tilde c^{\overline{m}}_{1} \\ \tilde c^{\overline{m}}_{2} \\ \tilde v^{\overline{m}} \end{bmatrix} = \left [ \begin{array}{l} {\mathcal{H}}^{1}(\tilde c^{\overline{m}}_{1}, \tilde c^{\overline{m}}_{2}, \tilde{v}^{\overline{m}}, \overline{m})\\ {\mathcal{H}}^{2}(\tilde c^{\overline{m}}_{1}, \tilde c^{\overline{m}}_{2}, \tilde{v}^{\overline{m}}, \overline{m})\\ {\mathcal{H}}^{3}(\tilde c^{\overline{m}}_{1}, \tilde c^{\overline{m}}_{2}, \tilde{v}^{\overline{m}})\\ \end{array} \right ], \end{equation} | (3.25) |
Also in in this case (i.e., case (iii) ) we have that {\mathcal{H}}^{1}(\cdot, \cdot, \cdot, \cdot) and {\mathcal{H}}^{2}(\cdot, \cdot, \cdot, \cdot) are correspondingly defined through Eqs (3.7) and (3.8) when the trial mutation form for the full mutation law (which in Cases (i) and (ii) was given by operator \overline{{\mathcal{M}}}_{r} ) is given here by {\mathcal{M}}^{2}_{i, j}(\tilde c^{\overline{m}}_{1}(t), \tilde v^{\overline{m}}(t), \overline{m}) .
Finally, adopting again the same time discretisation as in Section 3.1 and using the Euler method, a time marching step for model (3.25) is given by following operator
\begin{array}{l} & {{\bar K}_{\bar m}}:{\mathbb{R}^{N \times N}} \times {\mathbb{R}^{N \times N}} \times {\mathbb{R}^{N \times N}} \to {\mathbb{R}^{N \times N}} \times {\mathbb{R}^{N \times N}} \times {\mathbb{R}^{N \times N}}\\ {\rm{given}}\;{\rm{by}}\\& {{\bar K}_{\bar m}}\left( {\left[ {\begin{array}{*{20}{c}} {\tilde c_1^{{m_1},n}}\\ {\tilde c_2^{{m_1},n}}\\ {{{\tilde v}^{{m_1},n}}} \end{array}} \right]} \right): = \left[ {\begin{array}{*{20}{c}} {\tilde c_1^{{m_1},n}}\\ {\tilde c_2^{{m_1},n}}\\ {{{\tilde v}^{{m_1},n}}} \end{array}} \right] + \Delta t\left[ {\begin{array}{*{20}{c}} {{{\cal H}^1}(\tilde c_1^{\bar m,n},\tilde c_2^{\bar m,n},{{\tilde v}^{\bar m,n}},\bar m)}\\ {{{\cal H}^2}(\tilde c_1^{\bar m,n},\tilde c_2^{\bar m,n},{{\tilde v}^{\bar m,n}},\bar m)}\\ {{{\cal H}^3}(\tilde c_1^{\bar m,n},\tilde c_2^{\bar m,n},{{\tilde v}^{\bar m,n}})} \end{array}} \right], \end{array} | (3.26) |
where, for any n\in \{0, \dots, L\} , we have \tilde c^{\overline{m}, n}_{1} : = \tilde c^{\overline{m}}_{1}(t_{n}) , \tilde c^{\overline{m}, n}_{2} : = \tilde c^{\overline{m}}_{2}(t_{n}) , and \tilde{v}^{\overline{m}, n}: = \tilde{v}^{\overline{m}}(t_{n}) , while
\begin{eqnarray*} \begin{array}{l} {\mathcal{H}}^{1}(\tilde c^{\overline{m}, n}_{1}, \tilde c^{\overline{m}, n}_{2}, \tilde{v}^{\overline{m}, n}, \overline{m}) : = {\mathcal{H}}^{1}(\tilde c^{\overline{m}}_{1}(t_{n}), c^{\overline{m}}_{2}(t_{n}), \tilde{v}^{\overline{m}}(t_{n}), \overline{m}), \\ {\mathcal{H}}^{2}(\tilde c^{\overline{m}, n}_{1}, \tilde c^{\overline{m}, n}_{2}, \tilde{v}^{\overline{m}, n}, \overline{m}) : = {\mathcal{H}}^{2}(\tilde c^{\overline{m}}_{1}(t_{n}), c^{\overline{m}}_{2}(t_{n}), \tilde{v}^{\overline{m}}(t_{n}), \overline{m}), \\ {\mathcal{H}}^{3}(\tilde c^{\overline{m}, n}_{1}, \tilde c^{\overline{m}, n}_{2}, \tilde{v}^{\overline{m}, n}): = {\mathcal{H}}^{3}(\tilde c^{\overline{m}}_{1}(t_{n}), \tilde c^{\overline{m}}_{2}(t_{n}), \tilde{v}^{\overline{m}}(t_{n})). \end{array} \end{eqnarray*} |
Therefore, this allows us formulate "forward operator" denoted here by \overline{K} defined by
\begin{equation} \begin{split} &\overline{K}:{\mathfrak{M}}^{2}\to {\mathbb{R}}^{N\times N}\times {\mathbb{R}}^{N\times N}\times {\mathbb{R}}^{N\times N}\\ {\rm{given\;by}}&\\ &\overline{K}(\overline{m}): = \underbrace{\overline{K}_{\overline{m}} \circ \overline{K}_{\overline{m}} \circ \cdots \cdots \circ \overline{K}_{\overline{m}} }_{L\, \text{times}}\left( \begin{bmatrix} \tilde{c}_{1, 0}\\ \tilde{c}_{2, 0}\\ \tilde{v}_{0} \end{bmatrix} \right) \end{split} \end{equation} | (3.27) |
where \tilde{c}_{1, 0} , \tilde{c}_{2, 0} and \tilde{v}_{0} are the discretised initial conditions for model (2.1) (as introduced in model (3.11)).
Similar to the previous two inverse problem cases, the forward operator \overline{K} will enable us to identify the \overline{m}^{c_{1}^{*}, c_{2}^{*}, v^{*}}\in{\mathfrak{M}}^{2} such that the solution of the resulting model matches measurements model (3.1). Thus, similar to Section 3.2, we observe again that {\overline{K}} can be written down as
\begin{equation} {\mathfrak{M}}^{2}\ni \overline{m}\longmapsto \overline{K}_{\overline{m}}\in \boldsymbol{\ell}^{2}(\boldsymbol{\ell}^{2}({\mathcal{E}}\times {\mathcal{E}}\times {\mathcal{E}}); \boldsymbol{\ell}^{2}({\mathcal{E}}\times {\mathcal{E}}\times {\mathcal{E}})) \end{equation} | (3.28) |
Here, \boldsymbol{\ell}^{2}(\boldsymbol{\ell}^{2}({\mathcal{E}}\times {\mathcal{E}}\times {\mathcal{E}}); \boldsymbol{\ell}^{2}({\mathcal{E}}\times {\mathcal{E}}\times {\mathcal{E}})) is again the finite-dimensional Bochner space of square integrable vector-value functions defined on \boldsymbol{\ell}^{2}({\mathcal{E}}\times {\mathcal{E}}\times {\mathcal{E}}) with values in the same space. As in Section 3.2, also here we have that the mappings defined in model (3.28) are continuous and compact. Therefore, we obtain that \overline{K} is also closed sequentially bounded, and as a consequence we have that also in this case we satisfy the inverse problems hypotheses adopted [30], and thus, the convergence of the subsequent nonlinear Tikhonov regularisation is ensured. The Tikhonov functionals \{\overline{J}_{\alpha}\}_{ \alpha > 0} will have essentially the same form as in the first two cases, except that the space where these are defined is different (i.e., in this case we have {\mathfrak{M}}^{2} of dimension M\times M rather that {\mathfrak{M}}^{1} of dimension M that we had in Cases (i) and (ii) ), namely:
\begin{equation} \begin{split} &\overline{J}_{\alpha}: {\mathfrak{M}}^{2} \to{\mathbb{R}}, \quad \forall \, \alpha > 0, \\ {\rm{defined\;by}}&\\ &\overline{J}_{\alpha}(\overline{m}) : = \left\|\overline{K}(\overline{m})- \begin{bmatrix} \tilde{c}_{1}^{*}\\ \tilde{c}_{2}^{*}\\ \tilde{v}^{*} \end{bmatrix}\right\|^{2}_{2} + \alpha \|\, \overline{m}\, \|^{2}_{2} , \quad \forall \, \overline{m}\in {\mathfrak{M}}^{2}. \end{split} \end{equation} | (3.29) |
Computationally, we address here the reconstruction of the general mutation law {\mathcal{Q}}(c_{1}, v) that appears in Case (iii) for model (2.1) in the presence of zero flux boundary conditions and the initial conditions prescribed in Eqs (3.17a)–(3.17c). Furthermore, the inverse problem is addressed in the presence of both exact and noisy measurements of the form detailed in Eqs (3.15) and (3.16), with the exact measurements given by
\begin{equation} \tilde{c}^{*}_{1, exact}(x): = \bar{c}_{1}(x, t_{f}), \quad \tilde{c}^{*}_{2, exact}(x): = \bar{c}_{2}(x, t_{f}), \quad and \quad \tilde{v}^{*}_{exact}(x): = \bar{v}(x, t_{f}), \quad \forall x\in \Omega, \end{equation} | (3.30) |
where \bar{c}_{1}(x, t), \, \, \bar{c}_{2}(x, t) and \bar{v}(x, t) represent the solution densities for primary cell population, mutated cell population and ECM, respectively, which are obtained when model (2.1) uses as mutation law the expression given by model (2.3) with the known term \widetilde{{\mathcal{Q}}}_{2}(v) specified in model (2.4).
Although the dimensionality is different with respect to Cases (i) and (ii) , the inversion method in this case follows the same steps and numerical minimisation procedure and steps that were described in Section 3.3 (reason for which we skip here details already outlined there). Indeed, for any \alpha \in \{10^{-i}\, |\, i = 1, \dots 12 \} , the minimisation of the Tikhonov functional J_\alpha starts with an initial guess \overline{m}_{0} = \mathbb{I} \times10^{-3} , (where \mathbb{I} represents the M\times M matrix of ones), and leads to the numerical identification of the associated point of minimum m^{\alpha} that correspond to the smalles mismatch between the associated solution (that is obtained when model (2.1) uses m^{\alpha} as mutation law) and the measurements. Furthermore, since no data is available beyond the maximal region maximal accessible regions {\mathcal{A}}_{cv} given by
\begin{eqnarray*} \begin{array}{l} {\mathcal{A}}_{cv}: = {\mathcal{A}}_{c}\times {\mathcal{A}}_{v} = [\bar{c}_{1}^{min}, \bar{c}_{1}^{max}]\times [\bar{v}^{min}, \bar{v}^{max}], {\rm{with}} \;{\mathcal{A}}_{c}\;{\rm{and}}\; A_{v}\; {\rm{described\;in\;Eqs\;(3.19)\;and\;(3.20)}}. \end{array} \end{eqnarray*} |
the reconstruction of the mutation law is explored only on {\mathcal{A}}_{cv} . Finally, using again an discrepancy principle-based argument to choose the regularisation parameter \alpha*\in \{10^{-i}\, |\, i = 1, \dots 12 \} , the reconstructed mutation law will be given by the corresponding M\times M matrix \overline{m}^{\, c_{1}^{*}, c_{2}^{*}, v^{*}}: = \overline{m}^{\alpha*} , which in turn will determine the precise shape of the reconstructed mutation law {\mathcal{Q}}^{c_{1}^{*}, c_{2}^{*}, v^{*}}(\tilde c^{\overline{m}^{\, c_{1}^{*}, c_{2}^{*}, v^{*}}}_{1, i, j}(t), \tilde v^{\overline{m}^{\, c_{1}^{*}, c_{2}^{*}, v^{*}}}_{i, j}(t)) that is defined as per model (3.22). Figure 4 shows the reconstruction of the most general cancer cell mutation law {\mathcal{Q}}(c_{1}, v) , starting from the measurements given by models (3.15) and (3.18) for exact data as well as data impacted by noise \delta\in\{1\%, 3\%\} . For comparison, the first row of this figure shows the true mutation law restricted at the maximal accessible region {\mathcal{A}}_{cv} where the reconstruction is being attempted. The second row of the figure show from left to right the reconstruction of the mutation law on {\mathcal{A}}_{cv} with no noise (left), with 1\% noise (center), and 3\% noise (right) in the measured data. From this figure, we observe that we obtain a good reconstruction of the mutation law when the measurement data are not affected by noise. However, as expected, as soon as the level of noise increases in the measurements, the reconstruction gradually looses accuracy.
Throughout the previous sections we assumed that both cancer cell populations proliferate logistically: \mu_{c} c_{1, 2}\left(1\frac{c_{1}+c_{2}+v}{K_{c}}\right) . However, the sigmoid shape of tumour growth that is given by the logistic term can be obtained also with other proliferation rules, such as the Gompertz rule [33,34,35]: \mu_{c}c_{1, 2}\log \left(\frac{K_{c}}{c_{1}+c_{2}+v} \right) . This raises the question as to what happens when different cancer cell populations use different proliferation laws.
In this subsection we reconstruct the general mutation law {\mathcal{Q}}(c_{1}, v, t) in case (iii) when we assume that the primary c_{1} population proliferation proliferates logistically, while the second (mutated) cell population c_{2} proliferates according to Gompertz law. In Figure 5 we present the numerical reconstruction results. We observe that the results are similar to those in Figure 4; this could be explained by the fact that the mutation starts in the primary tumour which proliferate logistically.
Since various mathematical studies have assumed nonlocal cell-cell and cell-ECM interactions to explain the invasion of cancer cells [11,16,22,23], in the following we generalise the model (2.1) by replacing the local haptotaxis towards local ECM gradients with a nonlocal haptotaxis flux generated by these cell-cell and cell-ECM interactions.
As before, we consider a primary cancer cell subpopulation c_{1}(x, t) and a secondary mutated cancer cell subpopulation c_{2}(x, t) . These cancer cell populations interact with each other as well as with the extracellular matrix (ECM), v(x, t) , which they degrade and remodel. For compact notation, we consider the combined vector of primary cancer cells c_{1} , mutated cancer cells c_{2} and extracellular matrix v defined as
{\textbf{u}}(x, t) : = \left[c_{1}(x, t), c_{2}(x, t), v(x, t)\right]^{T}. |
We use this vector to describe in a compact manner the flux term generated by the nonlocal cell-cell and cell-ECM interactions ( {\mathcal{A}}_{1, 2}(x, t, {\textbf{u}}{\cdot, t)} ). Therefore the coupled tumour dynamics in this case is given by:
\begin{align} \frac{\partial{c_{1}}} {\partial {t}} & = \underbrace{D_{1} \Delta c_{1} }_{\rm{random\;motility}} - \underbrace{\nabla \cdot [c_{1}\mathcal{A}_{1}\left(x, t, {\textbf{u}}\left(\cdot, t\right)\right)]}_{\rm{adhesion}} + \underbrace{\mu_{c} {c_{1}} \left( 1- \frac{c_{1}+c_{2} + v}{K_{c}} \right)}_{\rm{logistic\;proliferation}} - {\underbrace{\omega(t)}_{\rm{mutation\;switch}}\;remod\underbrace{{\mathcal{Q}}(\cdot, \cdot)}_{\rm{unknown\;mutation}}}, \end{align} | (4.1a) |
\begin{align} \frac{\partial{c_{2}}} {\partial {t}} & = \underbrace{D_{2} \Delta c_{2} }_{\rm{random\;motility}} - \underbrace{\nabla \cdot [c_{2}\mathcal{A}_{2}\left(x, t, {\textbf{u}}\left(\cdot, t\right)\right)]}_{\rm{adhesion}} + \underbrace{\mu_{c} {c_{2}} \left( 1 - \frac{c_{1}+c_{2} +v}{K_{c}} \right)}_{\rm{logistic\;proliferation}} + {\underbrace{\omega(t)}_{\rm{mutation\;switch}}\;remod\underbrace{{\mathcal{Q}}(\cdot, \cdot)}_{\rm{unknown\;mutation}}}, \end{align} | (4.1b) |
\begin{align} \frac{\partial {v}} {\partial {t} } & = \underbrace{- \rho (c_{1}+c_{2}) v}_{\rm{degradation}} + \underbrace{\mu_{v} \left(K_{c} - c_{1}-c_{2} - v \right)^{+}}_{\rm{ECM\;remodelling}}. \end{align} | (4.1c) |
As before, D_{1, 2} are the diffusion coefficients, \mu_{c} is the cancer cell proliferation rate, \rho is the ECM degradation rate, and \mu_{v} is the ECM remodelling rate. The flux term \mathcal{A}_{p}\left(x, t, {\textbf{u}}\left(\cdot, t\right)\right) , p = 1, 2 , has been proposed in [11,23] to describe the directed movement of cells due to cell-cell and cell-matrix adhesion:
\begin{equation} \mathcal{A}_{p}\left(x, t, {\textbf{u}}\left(\cdot, t\right)\right) : = \frac{1}{R}\int\limits_{\textbf{B}\left(\left(0, 0\right), R\right)} n\left(y\right) \cdot \mathcal{K}\left(\|y\|_{2}\right) \cdot g_{{p}}\left({\textbf{u}}\left(x+y, t\right), t\right)\chi_{_{\Omega}}\left(x+y\right) \ dy. \end{equation} | (4.2) |
By using these notations the approximation for D_{p} \Delta c_{p}- \nabla \cdot [c_{p} \mathcal{A}_{p}\left(x, t, {\textbf{u}}\left(\cdot, t\right)\right)] in (4.1) is as follows:
It is assumed that the interactions between a cell and its neighbouring cells as well as the components of the ECM occur inside a sensing region \textbf{B}\left(\left(0, 0\right), R\right) \subset \mathbb{R} , where R > 0 is the sensing radius, as illustrated in Figure 6. In the above equation, \chi_{_{\Omega}}\left(\cdot\right) represents the characteristic function of \Omega . Further, n\left(y\right) is the unit radial vector given by
\begin{equation} n\left(y\right): = \left\{ \begin{array}{lr} y / \|y\|_{2} \quad \quad \quad \text{if } \quad y \in \textbf{B}\left(0, R\right) \setminus \{\left(0, 0\right)\}, \\ \left(0, 0\right) \quad \quad \text{otherwise}. \end{array} \right. \end{equation} | (4.3) |
Furthermore, g_{{p}}\left({\textbf{u}}(x+y, t\right), t) represents the p- th component of the adhesion function g\left({\textbf{u}}\left(x+y, t\right), t\right) that is given by
\begin{equation} \begin{array}{c} g\left({\textbf{u}}, t\right) = [\textbf{S}_{cc} {\textbf{c}} + \textbf{S}_{cv} v] \cdot \left(K_{c} - c_{1}-c_{2} - v\right)^{+}, \\[0.5cm] {\rm{with}}\quad \textbf{S}_{cc} = \begin{pmatrix} S_{c_{1}c_{1}} & S_{c_{1}c_{2}} \\ S_{c_{2}c_{2}} & S_{c_{2}c_{2}} \end{pmatrix} \quad\quad {\rm{and}} \quad\quad \textbf{S}_{cv} = \begin{pmatrix} S_{c_{1}v} & 0 \\ 0 & S_{c_{2}v} \end{pmatrix}. \end{array} \end{equation} | (4.4) |
Here \{S_{c_{i}c_{j}}\}_{i = 1, 2} are the non-negative cell-cell adhesion strengths of the adhesion bonds established between the primary and mutated cancer cell populations, while \{S_{c_{i}v}\}_{i = 1, 2} are the non-negative cell-matrix adhesion stands for the strengths for the adhesive interactions between each of the two cancer subpopulation and the ECM. Finally, no overcrowding of the cell population and ECM over the tumour domain is ensured here through the term (K_{c} - c_{1}-c_{2} - v)^{+}: = \max(K_{c} - c_{1}-c_{2} - v, 0) .
The nonlocal dynamics model (4.1) is assumed to take place in the presence of the same initial and boundary condition as those assumed in for the local model and given by models (2.5) and (2.6) in Section 2. The numerical approximation of the forward cancer invasion model (4.1) is presented in Appendix Section A. This includes the discretisation of the main spatial operators as well as the off grid numerical approach for the nonlocal adhesion flux terms \mathcal{A}_{p}\left(x, t, {\textbf{u}}\left(\cdot, t\right)\right) whose schematic is given by the right side of Figure 6. Finally, the general mutation law {\mathcal{Q}}(c_{1}, v) is assumed to be unknown and its identification will be our main focus in this section.
Building on the inverse problems approach developed for the local cancer invasion model in Section 3.4 and applying this to the nonlocal model (4.1), we proceed now to address the reconstruction of the unknown mutation law {\mathcal{Q}}(c_{1}, v) within the nonlocal cancer invasion model (4.1) from exact and noisy measured data that are considered here again to be of the form prescribed in models (3.15) and (3.16). Here, the exact measurement data that are given by
\begin{equation} \tilde{c}^{*}_{1, exact}(x): = \bar{c}_{1}(x, t_{f}), \quad \tilde{c}^{*}_{2, exact}(x): = \bar{c}_{2}(x, t_{f}), \quad and \quad \tilde{v}^{*}_{exact}(x): = \bar{v}(x, t_{f}), \quad \forall x\in \Omega, \end{equation} | (4.5) |
where \bar{c}_{p}(x, t) p = 1, 2 and \bar{v}(x, t) are the corresponding solution densities of the primary and mutated cancer cell populations as well as that of ECM that are obtained for model (4.1) obtained when this uses as mutation law given by model (2.3) with the known term \widetilde{{\mathcal{Q}}}_{2}(v) detailed in model (2.4). For the numerical reconstruction we consider here the same initial and boundary conditions as in Section 3.3. Figure 7 shows the reconstruction of the cancer cell mutation law Q(c_{1}, v) for model (4.1) in the presence of the measurements given by model (4.5) that are considered here both exact and affected by a level of noise \delta\in\{1\%, 3\%\} . As for the previous figures, the first row shows the true mutation law restricted at the maximal accessible region {\mathcal{A}}_{cv} where the reconstruction is being attempted. The second row of the figure shows, from left to right, the reconstruction of the mutation law on {\mathcal{A}}_{cv} with no noise, with 1\% , and with 3\% of noise in the measured data, respectively. From this figure, we observe that we obtain a good mutation law reconstruction when the measurement data are not affected by noise. However, as expected, as soon as the level of noise increases in the measurements, the reconstruction gradually looses accuracy.
As in Section 3.4.3, here we investigate the reconstruction of the general mutation law Q(c_{1}, v) when cancer cell growth is described by different rules: logistic proliferation for the c_{1} cells, and Gompertz proliferation for the second (mutated) cancer cell population c_{2} . Again, we do not see any significant differences between the reconstruction of mutation law in this case (see Figure 8) and in the previous case where both cell populations grow logistically (see Figure 7). We believe that is because in both cases, the population that starts mutating (i.e., the c_{1} population) grows logistically.
In this work we explored a new inverse problem that addresses the reconstruction of the cancer cells mutation law in two heterotypic cancer invasion models: a model with a local cancer cell haptotaxis flux towards ECM (see model (2.1)), and a model with a nonlocal haptotaxis flux generated by cell-cell and cell-ECM adhesion forces (see model (4.1)). For the reconstruction of this mutation law through an inverse problem Tikhonov regularisation-based approach, we used a numerically-generated spatial tumour snapshot data assumed to be acquired at a later stage in the tumour evolution (in practice, the data can be provided through a medical imaging scan).
This inverse problem approach was implemented computationally via a mixed finite differences - finite element numerical scheme. Specifically, on one hand, we used a Crank-Nicholson-type finite difference scheme for the discretisation of the forward models that arises in each of the two tumour invasion dynamics considered here (i.e., local cancer cell invasion, and nonlocal cancer cell invasion). On the other hand, we developed a finite element approach involving a bilinear shape functions on a square mesh for the discretisation of mutation law candidates recruited from a proposed space of functions {\mathcal{S}} , as well as for their evaluation on a maximal accessible regions where the mutation law reconstruction was performed. Finally, these two parts were appropriately assembled in an optimisation solver that sought to reconstruct the cancer cell mutation law by minimising over the space {\mathcal{S}} the emerging Tikhonov functionals that were formulated in each of the two cases considered.
Finally, this inversion approach was explored and tested on the reconstruction of different cancer cell mutation laws used in local and nonlocal cancer modelling: (i) reconstructing the cancer cell mutation assuming a linear dependence only on the c_{1} cell population; (ii) reconstructing the cancer cell mutation assuming a linear dependence on the c_{1} population and a nonlinear dependence on the ECM density, and (iii) reconstructing a very general mutation law, while assuming no prior knowledge about the mutation. To explore the robustness of the proposed inverse problem approach we used "in silico" constructed data that mathematically could be expressed in the general form given by models (3.15) and (3.16), but that were specified individually for each of the cases considered for the local and non-local models (2.1) and (4.1), respectively. Indeed, while the noise was always added the same way (as per model (3.16)), the exact measurements considered here were maps given by solutions at the final time for the densities of the cancer cell populations (i.e, both primary and mutated populations) and the ECM, namely (\bar{c}_{1}(\cdot, t_{f}), \bar{c}_{2}(\cdot, t_{f}), \bar{v}(\cdot, t_{f})) , which were obtained by equipping the local and nonlocal forward models with a selection of known mutation laws (given either by model (2.2) or by models (2.3) and (2.4)) considered as appropriate for each of the cases explored in the context of both local and nonlocal models for tumour dynamics. We note that the results in Figures 4, 5, 7, and 8 look correspondingly similar because we reconstruct the same mutation law Q(c, v), while for the second (mutated) cancer cell population, c_{2} , we use logistic proliferation for Figures 4 and 7 and Gompertz proliferation law for Figures 5 and 8. While there are small numerical differences, these are hard to be distinguished among the figures without zooming in. Furthermore, this similarity of the results is not significantly affected when switching between the Logistic and Gompertz proliferation laws for the second cancer cells population. An explanation for this similarity is that in addition to that fact that the mutation starts in the primary tumour which proliferate logistically, the logistic and Gompertz laws considered for c_{2} are part of the same class of size growth laws [33,34,35], with similar profiles on the parts of their domain where these are evaluated by the cancer models (2.1) and (4.1). All numerical reconstruction results for the local and nonlocal models showed that for exact measurements we obtained a good reconstruction of the mutation law, while for increasingly noisy measurements the reconstruction gradually deteriorates. Finally, these in silico reconstruction tests recommend the inversion framework developed in this work also for the context of real measurements data that come in the form of imaging data (such as MRI) with levels of noise that may not be a priori known. Nevertheless, the accuracy of the reconstruction in the real data case is expected to deteriorate for significant levels of noise.
The first author would like to thank Saudi Arabian Cultural Bureau in the United Kingdom (UKSACB) on behalf of Taif University and the University College of Al-Khurmah in Saudi Arabia for supporting and sponsoring his PhD studies at the University of Dundee.
The authors declare no conflict of interest.
In this section, we briefly discuss the numerical methods used to solve the forward models (4.1a)–(4.1c). To discretise the system in space we use the method of lines approach. For the time-evolution of the system, we use a predictor-corrector scheme introduced in [16], using the Euler method as the predictor and a trapezoidal-type rule as the corrector. In the reaction-diffusion models (4.1a) and (4.1b), the terms D_{p} \Delta c_{p}- \nabla \cdot [c_{p} \mathcal{A}_{p}\left(x, t, \textbf{u}\left(\cdot, t\right)\right)] , p = 1, 2 , will be approximated through a second-order mid-point rule [36] as detailed below. In brief, \forall p\in\{1, 2\}, \; n = \overline{0, L}, \; i, j = \overline{1, N} , these midpoint approximations are given by:
\begin{equation} \begin{cases} c^n_{p, i, j+\frac{1}{2}}: = \frac{c^n_{p, i, j} + c^n_{p, i, j+1}}{2}, \\ c^n_{p, i, j-\frac{1}{2}}: = \frac{c^n_{p, i, j} + c^n_{p, i, j-1}}{2}, \\ c^n_{p, i+\frac{1}{2}, j}: = \frac{c^n_{p, i, j} + c^n_{p, i+1, j}}{2}, \\ c^n_{p, i-\frac{1}{2}, j}: = \frac{c^n_{p, i, j} + c^n_{p, i-1, j}}{2} . \end{cases} \; \rm{and }\ \begin{cases} \mathcal{A}^n_{p, i, j+\frac{1}{2}}: = \frac{\mathcal{A}^n_{p, i, j} + \mathcal{A}^n_{p, i, j+1}}{2}, \\ \mathcal{A}^n_{p, i, j-\frac{1}{2}}: = \frac{\mathcal{A}^n_{p, i, j} + \mathcal{A}^n_{p, i, j-1}}{2}, \\ \mathcal{A}^n_{p, i+\frac{1}{2}, j}: = \frac{\mathcal{A}^n_{p, i, j} + \mathcal{A}^n_{p, i+1, j}}{2}, \\ \mathcal{A}^n_{p, i-\frac{1}{2}, j}: = \frac{\mathcal{A}^n_{p, i, j} + \mathcal{A}^n_{p, i-1, j}}{2} . \end{cases} \end{equation} | (A.1) |
Further, the central differences are given by
\begin{equation} \begin{cases} {[c_{p, y}]}^n_{i, j+\frac{1}{2}} : = \frac{c^n_{p, i, j+1} - c^n_{p, i, j}}{\Delta y}, \\ {[c_{p, y}]}^n_{i, j-\frac{1}{2}} : = \frac{c^n_{p, i, j} - c^n_{p, i, j-1}}{\Delta y}, \\ {[c_{p, x}]}^n_{i+\frac{1}{2}, j} : = \frac{c^n_{p, i+1, j} - c^n_{p, i, j}}{\Delta x}, \\ {[c_{p, x}]}^n_{i-\frac{1}{2}, j} : = \frac{c^n_{p, i, j} - c^n_{p, i-1, j}}{\Delta x}. \end{cases} \end{equation} | (A.2) |
\begin{equation} \begin{split} D_{p}\Delta c_{p}-\nabla \cdot [c_{p} \mathcal{A}_{p}\left(x, t, \textbf{u}\left(\cdot, t\right)\right)] & = \\ & \simeq \frac{ D_{p} [c_{p, x}]^{n}_{i+\frac{1}{2}, j} - D_{p} [c_{p, x}]^{n}_{i-\frac{1}{2}, j} - c^{n}_{p, i+\frac{1}{2}, j} \cdot \mathcal{A}^{n}_{p, i+\frac{1}{2}, j} + c^{n}_{p, i-\frac{1}{2}, j} \cdot \mathcal{A}^{n}_{p, i-\frac{1}{2}, j} }{ \Delta{x} }\\ & + \frac{ D_{p}[c_{p, y}]^{n}_{i, j+\frac{1}{2}} - D_{p} [c_{p, y}]^{n}_{i, j-\frac{1}{2}} - c^{n}_{p, i, j+\frac{1}{2}} \cdot \mathcal{A}^{n}_{p, i, j+\frac{1}{2}} + c^{n}_{p, i, j-\frac{1}{2}} \cdot \mathcal{A}^{n}_{p, i, j-\frac{1}{2}} }{ \Delta{y} }. \end{split} \end{equation} | (A.3) |
Next, we shift our attention to the numerical approach of the adhesive flux \mathcal{A}_{p} (that explores the effects of cell-cell and cell-matrix adhesion of cancer cells subpopulations). We carry out these computations off-grid by decomposing the sensing region \textbf{B}\left(x, R\right) in s\in \mathbb{N}^{*} annuli centred at x (with the inner most central circle being of numerically negligible size), and for each annuli k\in\{1, \dots, s\} (counted from the inner most to the outer most), we consider a radial decomposition of this in 2^{h+(k-1)} uniformly distributed radial sectors (with h\in\mathbb{N} fixed), as illustrated in the right side of Figure 6 (for h = 2 ). This leads to a collection of sectors \{\mathcal{S}_{\nu}\}_{\nu = 1, N_{s}} , where
N_{s}: = \sum\limits_{k = 1}^{s}2^{h+(k-1)}. |
Then for each annulus sector \mathcal{S}_{\nu} , we evaluate the total primary cancer cell population c_{1} , the total mutated cancer cell population c_{2} and the total ECM mass distributed on \mathcal{S}_{\nu} that are given by
\begin{equation} \omega_{\mathcal{S}_{\nu}, c_{1}} : = \frac{1}{\lambda\left(\mathcal{S}_{\nu}\right)} \int\limits_{\mathcal{S}_{\nu}} c_{1}\left(x, t\right) \, dx, \quad \omega_{\mathcal{S}_{\nu}, c_{2}} : = \frac{1}{\lambda\left(\mathcal{S}_{\nu}\right)} \int\limits_{\mathcal{S}_{\nu}} c_{2}\left(x, t\right) \, dx, \quad {\rm{and}} \quad \omega_{\mathcal{S}_{\nu}, v} : = \frac{1}{\lambda\left(\mathcal{S}_{\nu}\right)} \int\limits_{\mathcal{S}_{\nu}} v\left(x, t\right) \, dx. \end{equation} | (A.4) |
Further, on each sector \mathcal{S}_{\nu} , we consider the off-grid barycentres of each annulus sector by \textbf{b}_{\mathcal{S}_{\nu}} and the values of each of the three densities at these barycentres, namely c_{1}(\textbf{b}_{\mathcal{S}_{\nu}}, t) c_{2}(\textbf{b}_{\mathcal{S}_{\nu}}, t) and v(\textbf{b}_{\mathcal{S}_{\nu}}, t) , are obtained via interpolation with bilinear shape functions on the grid rectangles \{ y^{i}_{\textbf{b}_{\mathcal{S}_{\nu}}} \}_{i = 1, 4} that contain \textbf{b}_{\mathcal{S}_{\nu}} . Therefore, the approximation of the adhesion integral at each instance of time t > 0 is given by
\begin{equation} \mathcal{A}_{p}\left(x, t, \textbf{u}\left(\cdot, t\right)\right) = \sum\limits_{\nu = 1}^{s2^{m}} \frac{\lambda \left(\mathcal{S}_{\nu}\right)}{R} \textbf{n}\left(\textbf{b}_{\mathcal{S}_{\nu}}\right) \cdot \mathcal{K}\left(\textbf{b}_{\mathcal{S}_{\nu}}\right) g_{p}(\tilde{\textbf{u}}(\textbf{b}_{\mathcal{S}_{\nu}}, t)), \end{equation} | (A.5) |
where
\tilde{\textbf{u}}\left(\textbf{b}_{\mathcal{S}_{\nu}}, t\right) : = [\omega_{\mathcal{S}_{\nu}, c_{1}}\left(t\right), \omega_{\mathcal{S}_{\nu}, c_{2}}\left(t\right), \omega_{\mathcal{S}_{\nu}, v}\left(t\right)]^{T}, |
and g_{p}(\tilde{\textbf{u}}(\textbf{b}_{\mathcal{S}_{\nu}}, t)) is the p- th component of
g\left(\tilde{\textbf{u}}\left(\textbf{b}_{\mathcal{S}_{\nu}}, t\right)\right) = \left[ \textbf{S}_{cc} \left[\omega_{\mathcal{S}_{\nu}, c_{1}}\left(t\right), \omega_{\mathcal{S}_{\nu}, c_{2}}\left(t\right)\right]^{T} + {\omega_{\mathcal{S}_{\nu}, v}(t)}\textbf{S}_{cv} \right]\cdot \left(K_{c} - \textbf{c}(\textbf{b}_{\mathcal{S}_{\nu}}, t) - v(\textbf{b}_{\mathcal{S}_{\nu}}, t)\right)^{+}. |
For all the cancer cells mutation laws reconstructions considered in this work, we use the non-dimensional parameter set specified in Table 1.
[1] |
Acharya VV, Pedersen LH (2005) Asset pricing with liquidity risk. J Financ Econ 77: 375–410. https://doi.org/10.1016/j.jfineco.2004.06.007 doi: 10.1016/j.jfineco.2004.06.007
![]() |
[2] |
Amihud Y, Mendelson H (2008) Liquidity, the value of the firm, and corporate finance. J Applied Corp Financ 20: 32–45. https://doi.org/10.1111/j.1745-6622.2008.00179.x doi: 10.1111/j.1745-6622.2008.00179.x
![]() |
[3] |
Amihud Y, Hameed A, Kang W, Zhang H (2015) The Illiquidity Premium: International Evidence. J Financ Econ 117: 350–368. https://doi.org/10.1016/j.jfineco.2015.04.005 doi: 10.1016/j.jfineco.2015.04.005
![]() |
[4] |
Bank S, Yazar EE, Sivri U (2019) Can social media marketing lead to abnormal portfolio returns? Europ Research Manag Bus Econ 25: 54–62. https://doi.org/10.1016/j.iedeen.2019.04.006 doi: 10.1016/j.iedeen.2019.04.006
![]() |
[5] |
Bartov E, Faurel L, Mohanram P (2018) Can Twitter help predict firm-level earnings and stock returns? Accounting Rev 93: 25–27. https://doi.org/10.2308/accr-51865 doi: 10.2308/accr-51865
![]() |
[6] |
Broadstock D, Zhang D (2019) Social-media and intraday stock returns: The pricing power of sentiment. Financ Res Lett 30: 116–123. https://doi.org/10.1016/j.frl.2019.03.030 doi: 10.1016/j.frl.2019.03.030
![]() |
[7] |
Cervelló-Royo R, Guijarro F (2020) Forecasting stock market trend: a comparison of machine learning algorithms. Financ Mark Valuation 6: 37–49. https://doi.org/10.46503/NLUF8557 doi: 10.46503/NLUF8557
![]() |
[8] |
Corwin SA, Schultz P (2012) A Simple Way to Estimate Bid-Ask Spreads from Daily High and Low Prices. J Finance 67: 719–760. https://doi.org/10.1111/j.1540-6261.2012.01729.x doi: 10.1111/j.1540-6261.2012.01729.x
![]() |
[9] | Guijarro F, Moya-Clemente I, Saleemi J (2019) Liquidity Risk and Investors' Mood: Linking the Financial Market Liquidity to Sentiment Analysis through Twitter in the S & P500 Index. Sustainability 11: 7048. |
[10] |
Guijarro F, Moya-Clemente I, Saleemi J (2021) Market Liquidity and Its Dimensions: Linking the Liquidity Dimensions to Sentiment Analysis through Microblogging Data. J Risk Financ Manag 14: 394. https://doi.org/10.3390/jrfm14090394 doi: 10.3390/jrfm14090394
![]() |
[11] |
Huang RD, Stoll HR (1997) The Components of the Bid-Ask Spread: A General Approach. Rev Financ Studies 10: 995–1034. https://doi.org/10.1093/rfs/10.4.995 doi: 10.1093/rfs/10.4.995
![]() |
[12] |
Mazboudi M, Khalil S (2017) The attenuation effect of social media: Evidence from acquisitions by large firms. J Financ Stability 28: 115–124. https://doi.org/10.1016/j.jfs.2016.11.010 doi: 10.1016/j.jfs.2016.11.010
![]() |
[13] |
Oliveira N, Cortez P, Areal N (2013) On the predictability of stock market behavior using stocktwits sentiment and posting volume. Prog artific intellig. Lect notes comput science 8154: 355–365. https://doi.org/10.1007/978-3-642-40669-0_31 doi: 10.1007/978-3-642-40669-0_31
![]() |
[14] |
Oliveira N, Cortez P, Areal N (2017) The impact of microblogging data for stock market prediction: using twitter to predict returns, volatility, trading volume and survey sentiment indices. Expert Syst Applications 73: 125–144. https://doi.org/10.1016/j.eswa.2016.12.036 doi: 10.1016/j.eswa.2016.12.036
![]() |
[15] |
Prokofieva M (2015) Twitter-based dissemination of corporate disclosure and the intervening effects of firms' visibility: Evidence from Australian-listed companies. J Inform Systems 29: 107–136. https://doi.org/10.2308/isys-50994 doi: 10.2308/isys-50994
![]() |
[16] |
Roll R (1984) A Simple Implicit Measure of the Effective Bid‐Ask Spread in an Efficient Market. J Finance 39: 1127–1139. https://doi.org/10.1111/j.1540-6261.1984.tb03897.x doi: 10.1111/j.1540-6261.1984.tb03897.x
![]() |
[17] |
Saleemi J (2020) An estimation of cost-based market liquidity from daily high, low and close prices. Financ Mark Valuation 6: 1–11. https://doi.org/10.46503/VUTL1758 doi: 10.46503/VUTL1758
![]() |
[18] |
Saleemi J (2022) Asymmetric information modelling in the realized spread: A new simple estimation of the informed realized spread. Financ Mark Valuation 8: 1–12. https://doi.org/10.46503/JQYH3943 doi: 10.46503/JQYH3943
![]() |
[19] | Sarr A, Lybek T (2002) Measuring liquidity in financial markets. Intern Monet Fund 2: 1–64. |
[20] |
Smailović J, Grčar M, Lavrač N, Žnidaršič M (2013) Predictive sentiment analysis of Tweets: a stock market application. Human-Comput Interac Know Dis Comp, Unstructured, Big Data, 77–88. https://doi.org/10.1007/978-3-642-39146-0_8 doi: 10.1007/978-3-642-39146-0_8
![]() |
[21] |
Sprenger TO, Tumasjan A, Sandner PG, Welpe IM (2014) Tweets and trades: the information content of stock microblogs. Europ Financ Manag 20: 926–957. https://doi.org/10.1111/j.1468-036X.2013.12007.x doi: 10.1111/j.1468-036X.2013.12007.x
![]() |
[22] |
Yu Y, Duan W, Cao Q (2013) The impact of social and conventional media on firm equity value: A sentiment analysis approach. Dec Support Systems 55: 919–926. https://doi.org/10.1016/j.dss.2012.12.028 doi: 10.1016/j.dss.2012.12.028
![]() |
1. | Xiaofeng Chen, Double-integrator consensus for a switching network without dwell time, 2023, 20, 1551-0018, 11627, 10.3934/mbe.2023516 | |
2. | Yuhang Yao, Jiaxin Yuan, Tao Chen, Xiaole Yang, Hui Yang, Distributed convex optimization of bipartite containment control for high-order nonlinear uncertain multi-agent systems with state constraints, 2023, 20, 1551-0018, 17296, 10.3934/mbe.2023770 | |
3. | Yihang Dou, Guansheng Xing, Aohua Ma, Guanwu Zhao, A review of event-triggered consensus control in multi-agent systems, 2024, 2330-7706, 1, 10.1080/23307706.2024.2388551 | |
4. | Yingxue Hou, Yan-Jun Liu, Shaocheng Tong, Event-triggered fault-compensation-based fuzzy finite-time FTC for MIMO switched nonlinear systems, 2025, 496, 00963003, 129316, 10.1016/j.amc.2025.129316 |
Parameter | Value | Description | Reference |
D_{1} | 0.00675 | diffusion of primary tumour | [11] |
D_{2} | 0.00675 | diffusion of secondary tumour | [11] |
\eta_{1} | 2.85\times 10^{-2} | haptotaxis to ECM from c_{1} | [37] |
\eta_{2} | 2.85\times 10^{-2} | haptotaxis to ECM from c_{2} | [37] |
\mu_{c} | 0.25 | proliferation of tumour cells c | [38] |
K_{c} | 1 | tissue carrying capacity | [16] |
\rho | 2 | ECM degradation coefficient | [39] |
\mu_{v} | 0.40 | ECM remodelling coefficient | [16] |
\textbf{S}_{cc} | \left({\begin{array}{*{20}{c}} {0.5} &0\\ 0&{0.3} \end{array}} \right) | cell-cell adhesion function | [11] |
\textbf{S}_{cv} | \left({\begin{array}{*{20}{c}} {0.1}&0\\ 0&{0.5} \end{array}} \right) | cell matrix adhesion function | [11] |
t_{1, 2} | 10 | time initiation for mutations | [38,40] |
t_{s} | 3 | time-steepness coefficient | [38] |
\delta_{0} | 0.3 | mutation from primary tumour | [38,40] |
\Delta{x} | 0.03125 | discretisation step size for {\mathcal{G}}_{\Omega} | [16] |
\Delta t | 10^{-3} | time step size | [16] |
\Delta{\eta} | 0.0625 | mesh size used for {\mathcal{G}}^{1}_{M} and {\mathcal{G}}^{2}_{M} | Estimated |
Parameter | Value | Description | Reference |
D_{1} | 0.00675 | diffusion of primary tumour | [11] |
D_{2} | 0.00675 | diffusion of secondary tumour | [11] |
\eta_{1} | 2.85\times 10^{-2} | haptotaxis to ECM from c_{1} | [37] |
\eta_{2} | 2.85\times 10^{-2} | haptotaxis to ECM from c_{2} | [37] |
\mu_{c} | 0.25 | proliferation of tumour cells c | [38] |
K_{c} | 1 | tissue carrying capacity | [16] |
\rho | 2 | ECM degradation coefficient | [39] |
\mu_{v} | 0.40 | ECM remodelling coefficient | [16] |
\textbf{S}_{cc} | \left({\begin{array}{*{20}{c}} {0.5} &0\\ 0&{0.3} \end{array}} \right) | cell-cell adhesion function | [11] |
\textbf{S}_{cv} | \left({\begin{array}{*{20}{c}} {0.1}&0\\ 0&{0.5} \end{array}} \right) | cell matrix adhesion function | [11] |
t_{1, 2} | 10 | time initiation for mutations | [38,40] |
t_{s} | 3 | time-steepness coefficient | [38] |
\delta_{0} | 0.3 | mutation from primary tumour | [38,40] |
\Delta{x} | 0.03125 | discretisation step size for {\mathcal{G}}_{\Omega} | [16] |
\Delta t | 10^{-3} | time step size | [16] |
\Delta{\eta} | 0.0625 | mesh size used for {\mathcal{G}}^{1}_{M} and {\mathcal{G}}^{2}_{M} | Estimated |