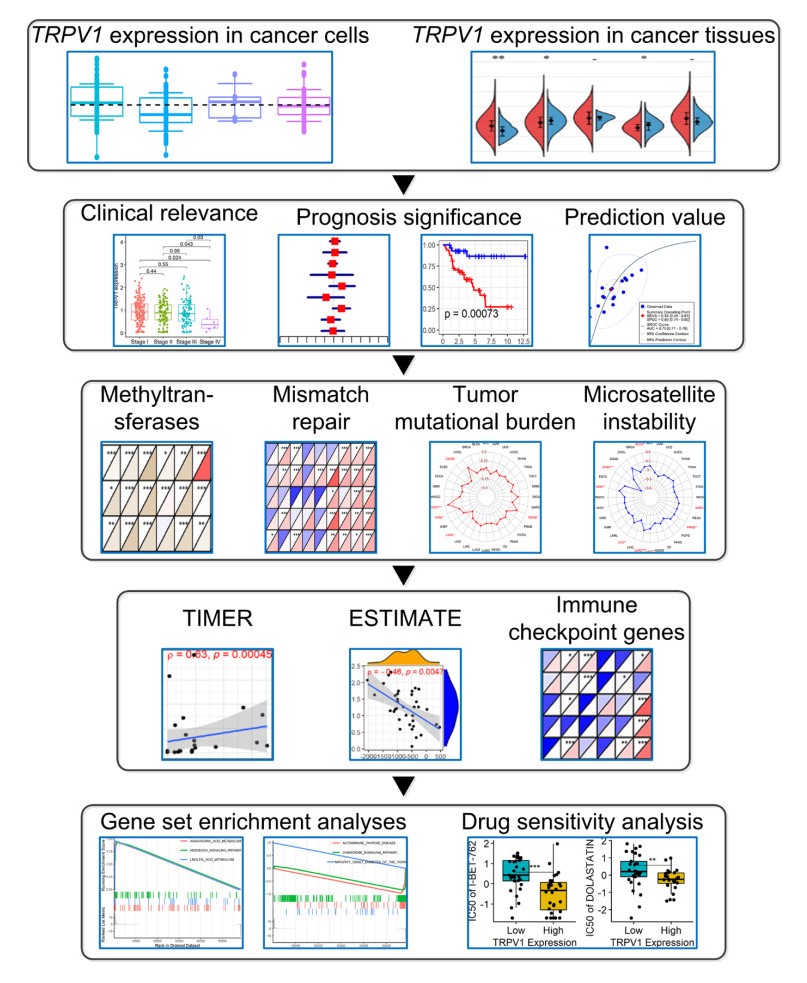
Transient receptor potential cation channel subfamily V member 1 (TRPV1) was considered to play pivotal roles in multiple cancers; however, the expression and clinical significance of the TRPV1 remain unclear, which were explored in this study.
The pan-cancer analysis was performed based on 10,236 samples in 32 cancers. Differential TRPV1 expression levels were detected in 12 cancers (p < 0.05). TRPV1 demonstrated its conspicuous prognosis significance and prediction effects for some cancers (e.g., lung adenocarcinoma), indicating its potential as a valuable and novel biomarker in treating and predicting cancers. TRPV1 expression was relevant to DNA methyltransferases, mismatch repair genes, tumor mutational burden, and microsatellite instability. TRPV1 expression was associated with the immune microenvironment of some cancers, and its roles in different cancers may be mediated by affecting various immune cells. Gene set enrichment analysis discloses the significant relevance of TRPV1 expression with a series of metabolic and immunoregulatory-related pathways.
This study provided a comprehensive workflow of the expression, clinical significance, and underlying mechanisms of TRPV1 in pan-cancer. TRPV1 may be an underlying biomarker for predicting and treating multiple cancer.
Citation: Tao Huang. TRPV1 is a potential biomarker for the prediction and treatment of multiple cancers based on a pan-cancer analysis[J]. Mathematical Biosciences and Engineering, 2022, 19(8): 8361-8379. doi: 10.3934/mbe.2022389
[1] | Ye Hu, Meiling Wang, Kainan Wang, Jiyue Gao, Jiaci Tong, Zuowei Zhao, Man Li . A potential role for metastasis-associated in colon cancer 1 (MACC1) as a pan-cancer prognostic and immunological biomarker. Mathematical Biosciences and Engineering, 2021, 18(6): 8331-8353. doi: 10.3934/mbe.2021413 |
[2] | Zhiyue Su, Chengquan Li, Haitian Fu, Liyang Wang, Meilong Wu, Xiaobin Feng . Improved prognostic prediction model for liver cancer based on biomarker data screened by combined methods. Mathematical Biosciences and Engineering, 2023, 20(3): 5316-5332. doi: 10.3934/mbe.2023246 |
[3] | Chunpei Ou, Qin Peng, Changchun Zeng . An integrative prognostic and immune analysis of PTPRD in cancer. Mathematical Biosciences and Engineering, 2022, 19(6): 5361-5379. doi: 10.3934/mbe.2022251 |
[4] | Guobing Lin, Cunming Zhang, Xuanyu Chen, Junwei Wang, Song Chen, Siyuan Tang, Tianqiang Yu . Identification of circulating miRNAs as novel prognostic biomarkers for bladder cancer. Mathematical Biosciences and Engineering, 2020, 17(1): 834-844. doi: 10.3934/mbe.2020044 |
[5] | Yuan Yang, Lingshan Zhou, Xi Gou, Guozhi Wu, Ya Zheng, Min Liu, Zhaofeng Chen, Yuping Wang, Rui Ji, Qinghong Guo, Yongning Zhou . Comprehensive analysis to identify DNA damage response-related lncRNA pairs as a prognostic and therapeutic biomarker in gastric cancer. Mathematical Biosciences and Engineering, 2022, 19(1): 595-611. doi: 10.3934/mbe.2022026 |
[6] | Sidan Long, Shuangshuang Ji, Kunmin Xiao, Peng Xue, Shijie Zhu . Prognostic and immunological value of LTB4R in pan-cancer. Mathematical Biosciences and Engineering, 2021, 18(6): 9336-9356. doi: 10.3934/mbe.2021459 |
[7] | Zekun Xin, Yang Li, Lingyin Meng, Lijun Dong, Jing Ren, Jianlong Men . Elevated expression of the MYB proto-oncogene like 2 (MYBL2)-encoding gene as a prognostic and predictive biomarker in human cancers. Mathematical Biosciences and Engineering, 2022, 19(2): 1825-1842. doi: 10.3934/mbe.2022085 |
[8] | Jihong Yang, Hao Xu, Congshu Li, Zhenhao Li, Zhe Hu . An explorative study for leveraging transcriptomic data of embryonic stem cells in mining cancer stemness genes, regulators, and networks. Mathematical Biosciences and Engineering, 2022, 19(12): 13949-13966. doi: 10.3934/mbe.2022650 |
[9] | Ahmed Hammad, Mohamed Elshaer, Xiuwen Tang . Identification of potential biomarkers with colorectal cancer based on bioinformatics analysis and machine learning. Mathematical Biosciences and Engineering, 2021, 18(6): 8997-9015. doi: 10.3934/mbe.2021443 |
[10] | Jianqiao Pan, Baoshan Ma, Xiaoyu Hou, Chongyang Li, Tong Xiong, Yi Gong, Fengju Song . The construction of transcriptional risk scores for breast cancer based on lightGBM and multiple omics data. Mathematical Biosciences and Engineering, 2022, 19(12): 12353-12370. doi: 10.3934/mbe.2022576 |
Transient receptor potential cation channel subfamily V member 1 (TRPV1) was considered to play pivotal roles in multiple cancers; however, the expression and clinical significance of the TRPV1 remain unclear, which were explored in this study.
The pan-cancer analysis was performed based on 10,236 samples in 32 cancers. Differential TRPV1 expression levels were detected in 12 cancers (p < 0.05). TRPV1 demonstrated its conspicuous prognosis significance and prediction effects for some cancers (e.g., lung adenocarcinoma), indicating its potential as a valuable and novel biomarker in treating and predicting cancers. TRPV1 expression was relevant to DNA methyltransferases, mismatch repair genes, tumor mutational burden, and microsatellite instability. TRPV1 expression was associated with the immune microenvironment of some cancers, and its roles in different cancers may be mediated by affecting various immune cells. Gene set enrichment analysis discloses the significant relevance of TRPV1 expression with a series of metabolic and immunoregulatory-related pathways.
This study provided a comprehensive workflow of the expression, clinical significance, and underlying mechanisms of TRPV1 in pan-cancer. TRPV1 may be an underlying biomarker for predicting and treating multiple cancer.
Cancer and cardiovascular disease are the two most common causes of death worldwide. The incidence and mortality of cardiovascular disease decreased significantly, while the incidence and mortality of cancer increased [1]. As predicted, 19.3 million cancer patients would be diagnosed, and nearly 10.0 million individuals would die of various cancers globally in 2020 [2]. Compared to traditional clinical management methods of cancers, such as surgical treatment, chemotherapy, and radiotherapy, targeted therapy shows increasingly prominent potential [3]. The significant progress of targeted therapy can be seen in some cancers (e.g., melanoma); however, quite a few patients with certain cancers (e.g., non-small-cell lung carcinoma) fail to benefit significantly from targeted therapy and have unfavorable prognoses [4,5]. Thus, more research on valuable biomarkers for cancer treatment and prediction is necessary.
Transient receptor potential cation channel subfamily V member 1 (TRPV1) protein is encoded by the gene located on chromosome 17p13 – TRPV1. TRPV1 is a transmembrane protein (a nonselective cation channel) associated with pain conduction, which stimulates various physical and chemical factors, thus promoting the cellular influx of calcium and sodium ions and resulting in cell death [6]. Increasing studies identified the pivotal roles of TRPV1 in multiple cancers [7]. For example, upregulated TRPV1 expression was detected in brain tumors and was relevant to tumor grading [8]. High-TRPV1 expression was discovered in breast cancer and suppressed the growth of certain types of breast cancer cells [9]. Indeed, TRPV1 demonstrated essential and complex factors in several biological processes, including proliferation, metastasis, and death of cancer cells [6]. Little is understood about the clinical value and mechanisms of TRPV1 in pan-cancer, and more efforts should focus on this vacancy.
The current study investigated the expression, clinical value, and underlying mechanisms of TRPV1 in pan-cancer. By using 10,236 samples from Cancer Cell Line Encyclopedia (CCLE) and The Cancer Genome Atlas (TCGA), the research provided an overview of TRPV1 expression in 32 cancers. Clinical relevance, clinical significance, and immune relevance of TRPV1 in multiple cancers were also evaluated, determining TRPV1 as a potential biomarker for cancers. Enrichment analyses were performed to explore the underlying molecular mechanisms of TRPV1 in pan-cancer, contributing to the understanding of the pathogenesis of some cancers.
Data on TRPV1 mRNA expression in various cancer cell lines of CCLE [10] was downloaded from Depmap Portal on November 16, 2021. Whole-genome RNA expression data of TCGA were obtained from the Xena database on November 17, 2021. For TCGA data, three types of samples—primary tumor, solid tissue normal, and primary blood-derived cancer peripheral blood—were considered for analyses in the present study. Cancers selected in the study and their sample numbers are shown in Supplementary Appendixes 1 and 2. Cancer with less than six samples (three cancer samples and three normal samples) was excluded in analyses of the expression and prediction value of TRPV1.
Tumor Immune Single-cell Hub (http://tisch.comp-genomics.org/home/) provides the single-cell RNA-seq data on 1,944,551 cells of 28 cancer types. Data in this database had been performed with quality control, clustering, and cell-type annotation, which were used to examine cell types of highly expressed TRPV1.
Three clinical parameters (age, gender, and American Joint Committee on Cancer stages) and four types of prognosis data—overall survival (OS), disease-specific survival (DSS), disease-free interval (DFI), and progression-free interval (PFI)—of TCGA were collected from the Xena database on November 17, 2021.
For evaluating the prognosis effects of TRPV1 in 32 cancers, univariate Cox regression analysis and Kaplan-Meier curves were applied to investigate the correlation of TRPV1 expression with OS, DSS, DFI, and PFI. Receiver operator characteristic curves were generated to determine whether TRPV1 can be identified as a potential marker for predicting cancers or not, which was judged by the value of the area under the curve (AUC). The greater the AUC value (the maximum value is 1), the more precisely TRPV1 expression predicted cancer.
A series of datasets of simple nucleotide variation (SNV) from TCGA, which had been processed by MuTect2 software [11], was downloaded from the GDC portal and visualized in Sanger Box (v3.0). DNA methyltransferases and mismatch repair (MMR) genes data were extracted from the TCGA cohort. Tumor mutational burden (TMB) and microsatellite instability (MSI) data of previous studies [12,13] were downloaded from Sanger Box (v3.0).
TIMER [14,15,16,17] and ESTIMATE [13,18,19,20] were two algorithms feasible to predict the immune microenvironment. TIMER was used to calculate the abundance of six types of immune cells, containing B cells, CD4+ T cells, CD8+ T cells, neutrophils, macrophages, and dendritic cells. Three ESTIMATE scores—stromal score, immune score, and estimated score—were generated to evaluate stromal cell infiltration levels, immune cell infiltration levels, and tumor purity. Relationships of TRPV1 expression with the immune microenvironment and a series of immune checkpoint genes (extracted from the TCGA cohort) expression were investigated in the study.
For studying potential mechanisms of TRPV1 in pan-cancer, TRPV1-related KEGG (Kyoto Encyclopedia of Genes and Genomes) signaling pathways were explored based on gene set enrichment analysis (GSEA) with the clusterProfiler package [21]. In the process, samples from the TCGA dataset were divided into the high-TRPV1 expression group and the low-TRPV1 expression group based on the median level of TRPV1 expression. Signaling pathways with a p-value less than 0.05 were selected in the study.
To further explore the mechanisms of TRPV1 in certain cancers, hub genes associated with TRPV1 expression were selected based on the weighted gene co-expression network analysis (WGCNA) by using the WGCNA package [22]. In this analysis: 1) all genes in the dataset (extracted from the TCGA dataset) for single cancer with a median absolute deviation of more than one were conserved for WGCNA; 2) the top 5000 genes ranked by the median absolute deviation values were classified into multiple modules associated with TRPV1 expression; 3) a scale-free network was determined with the minimum quantified soft threshold; 4) genes of the most significant module were prepared for identifying hub genes. Then, using the "degree" algorithm, hub genes of certain cancers were chosen in Cytoscape (v3.9.0).
CellMiner [23] collects a large of drugs and gene expression data. Using IC50 (half maximal inhibitory concentration) data from this database, drugs sensitive to cells with TRPV1 overexpression were selected with a p-value less than 0.01.
All data on TRPV1 gene expression levels were normalized with log2 (transcripts per million + 1) conversion. Wilcoxon rank-sum tests and Kruskal-Wallis tests were applied to identify the different expression levels of TRPV1. All correlation analyses in the study were performed based on Spearman's rank correlation coefficient. Processes of statistical analyses were performed in R (v4.1.0). If there was no particular explanation, a p-value less than 0.05 represented statistical significance in this study. Figure 1 shows an overview of the study.
Through CCLE data, TRPV1 expression was significantly different in 13 cancer cell lines (p < 0.05) (Figure 2A). Furthermore, based on TCGA data, the expression levels of TRPV1 in distinct cancer tissues were notably various from their normal tissues. In 20 cancers, TRPV1 was highly expressed in eight cancers – BLCA, HNSCC, KIRC, KIRP, LIHC, LUAD, LUSC, and STAD. On the contrary, TRPV1 was lowly expressed in four types of cancer – BRCA, COAD, GBM, and KICH (Figure 2B).
Single-cell RNA-Seq data from Tumor Immune Single-cell Hub were utilized to investigate TRPV1 expression in cell types of the five eights cancers (no TRPV1 expression data can be found for BLCA, KIRP, and STAD) with upregulated TRPV1 expression. As a result, conspicuously elevated expression of TRPV1 can be detected in various cell types for different cancers (CD4 T cells for HNSCC, plasma for KIRC, proliferating T cells for LIHC, and monocytes or macrophages for non-small-cell lung carcinoma [for LUAD and LUSC]) (Supplementary Appendix 3).
Upregulated TRPV1 expression was determined at advanced stages in ACC, while it was found at lower stages in BLCA, KIRP, LIHC, LUAD, and STAD (Figure 2C). Compared to the young, the elderly (≥ 65 years old) patients with LUAD tend to have reduced TRPV1 expression levels (Figure 2C). For BLCA and KIRC, the differential expression levels of TRPV1 were detected in various gender groups (Figure 2C).
TRPV1 expression has different prognostic values in various cancers. In OS, high TRPV1 expression predicted poor overall survival for patients with ACC and PRAD, while it was associated with a favorable prognosis for patients with BLCA, HNSCC, PAAD, and READ (Figure 3A). The same situation can be observed in DSS, and TRPV1 also played a protective role for KIRP patients and a risk role for patients with MESO and THCA (Figure 3C). Analyses of the Kaplan-Meier curves consistently supported the results in univariate Cox analysis (Figures 3B and D). In several cancers, upregulated TRPV1 expression also demonstrated its correlations with unfavorable DFI (LUAD and PRAD) (Figures 4A and C) and PFI (ACC, PCPG, and PRAD) (Figures 4B and D), and both favorable DFI and PFI for patients with PAAD (Figure 4).
TRPV1 expression showed different predictive abilities in various cancers. In KICH, the effect of TRPV1 expression on identifying cancer tissues and non-cancer tissues was significant (AUC = 0.941). In BLCA, GBM, and LUAD, TRPV1 expression made it feasible to identify these cancer tissues (AUC > 0.7), while such an effect for the rest 16 cancers was not conscious (AUC < 0.7) (Figure 5A). In an overview of the 20 types of cancers, AUC for TRPV1 expression was equal to 0.75 (95 % CI: 0.71–0.78) (Figure 5B). Therefore, TRPV1 expression had prediction significance for cancers, suggesting its potential for screening some cancers, especially KICH, BLCA, GBM, and LUAD.
Among the four types of mutations detected, the missense mutation was the most common, and the splice site was the rarest form of TRPV1's SNVs. Moreover, UCEC had the highest frequency of TRPV1's SNV (4.6 %) (Figure 5C).
In almost all 32 cancers, TRPV1 was conspicuously related to three DNA methyltransferases. The positive expression correlation between TRPV1 and DNMT3B was the most significant in DLBC (Spearman's ρ = 0.806, p < 0.001). A conspicuous positive relevance between TRPV1 and some methyltransferases was also observed in LAML (DNMT3A) and UVM (all of DNMT1, DNMT3A, and DNMT3B) (Figure 6A). In addition to CHOL, PAAD, and READ, expression associations (mainly positive) of TRPV1 with at least one MMR gene were detected in 29 cancers (Figure 6B).
As shown in Figure 6C, in MESO and THYM, TRPV1 expression was positively correlated with TMB; in BRCA and PAAD, TRPV1 expression was negatively relevant to TMB (Figure 6C). A significantly negative relationship between TRPV1 expression and MSI was observed in DLBC and LIHC. In contrast, weak correlations between TRPV1 expression and MSI can be detected in BLCA, GBM, LGG, LUAD, LUSC, PRAD, SARC, STAD, and THCA (Figure 6D).
According to the TIMER algorithm, TRPV1 was significantly associated with infiltration levels of six immune cells in DLBC, CHOL, and SKCM (Figure 7A). TRPV1 expression was positively related to B cell infiltration levels in DLBC (Spearman's ρ = 0.63, p < 0.05), and negatively correlated with dendritic cell infiltration levels in CHOL (Spearman's ρ = −0.39, p = 0.018). A positive correlation between TRPV1 expression and the infiltration levels of neutrophils and macrophages could be detected in SKCM (Spearman's ρ > 0.30, p < 0.05) (Figure 7A). Based on the ESTIMATE algorithm, the three cancers with the most significant negative correlations between TRPV1 and the three ESTIMATE scores were CHOL, LAML, and LGG (Figure 7B). Thus, TRPV1 expression was associated with the immune microenvironment of some cancers, although its roles in different cancers may be mediated by affecting various immune cells.
In seven cancers – DLBC, LAML, OV, READ, SKCM, THYM, and UVM, TRPV1 expression was found to be positively associated with almost a third (15/46) of the immune checkpoint genes included in the study (Spearman's ρ > 0.2, p < 0.05, Figure 7C).
Association of TRPV1 with at least three KEGG signaling pathways were detected in four cancers —ACC, BLCA, GBM, and OV. It can be seen from Figure 8A that TRPV1 was significantly relevant to a series of metabolic-related pathways (e.g., "ARACHIDONIC ACID METABOLISM" and "MATURITY ONSET DIABETES OF THE YOUNG") and immunoregulatory-related pathways (e.g., "AUTOIMMUNE THYROID DISEASE" and "CHEMOKINE SIGNALING PATHWAY") (Figure 8A). Furthermore, a notable tumor-related pathway ("HEDGEHOG SIGNALING PATHWAY") was also detected in ACC (Figure 8A).
Gene regulatory network analysis was common for further identifying hub genes in specific classification (e.g., clinical features and gene expression) [24,25]. To explore the hub genes significantly associated with TRPV1 in ACC, BLCA, GBM, and OV, WGCNA was performed. For ACC, six outlying specimens were removed, and the remaining were used to carry out WGCNA (Supplementary Appendix 4A). A scale-free network was established with the soft threshold equaling 4 (Supplementary Appendix 4B). The hierarchical clustering identified eight modules (Supplementary Appendix 4C). The module strongly correlated with TRPV1 expression was the "brown" module (Supplementary Appendix 4D). In the brown" module, genes with high module membership generally had elevated gene significance (Supplementary Appendix 4E). Ultimately, CDCA8 was identified as the hub gene via the WGCNA weight score and "degree" algorithm (Supplementary Appendix 4F). Similarly, hub genes were determined for BLCA, GBM, and OV; in detail, HID1 for BLCA (Supplementary Appendixes 5A–F), LY6G5B for GBM (Supplementary Appendixes 6A–F), and three genes (EFR3B, TMSB15B, and EFS) for OV (Supplementary Appendixes 7A–F).
This study selected drugs sensitive to highly expressed TRPV1 cells from 574 drugs validated by clinical trials and 218 drugs approved by the American Food and Drug Administration. As a result, IC50 values of 34 drugs were associated with TRPV1 expression (p < 0.01; Supplementary Appendix 8). Among these drugs, three (I-BET-762, DOLASTATIN 10, and ABBV-075) were sensitive to TRPV1, as they had lower IC50 values for high-TRPV1 expression cells (p < 0.01; Figure 8B).
The current study provided a comprehensive workflow of the expression, clinical significance, and underlying mechanisms of TRPV1 in pan-cancer. Using 10,236 samples, differential TRPV1 expression levels were detected in multiple cancers. TRPV1 demonstrated its conspicuous prognosis significance and prediction effects for some cancers, indicating its potential as a valuable and potential biomarker in treating and predicting cancers. TRPV1 expression was relevant to DNA methyltransferases, MMR, TMB, and MSI. TRPV1 expression was associated with the immune microenvironment of some cancers, and its roles in different cancers may be mediated by affecting various immune cells. GSEA discloses the significant relevance of TRPV1 expression with a series of metabolic and immunoregulatory-related pathways.
TRPV1 demonstrated different expression levels and prognosis significance in various cancers. Previously, Han et al. [26,27] identified overexpression of TRPV1 and its risk factor for prognosis in both cervical cancer and epithelial ovarian cancer. On the contrary, Gao et al. [28] discovered decreased TRPV1 expression in gastric cancer and the association of high-TRPV1 expression with a favorable prognosis. Different TRPV1 expression levels were detected in 13 cancer cell lines in my study. The expression levels of TRPV1 in distinct cancer tissues varied from their normal tissues: upregulated in BLCA, HNSCC, KIRC, KIRP, LIHC, LUAD, LUSC, and STAD, while downregulated in BRCA, COAD, GBM, and KICH. For certain cancers with elevated TRPV1 expression, high gene expression of TRPV1 was mainly found in immune cells, including CD4 T cells, plasma, proliferating T cells, and monocytes or macrophages. Furthermore, TRPV1 expression had conspicuous prognosis values in numerous cancers. Both univariate Cox regression and Kaplan-Meier curves revealed that: (1) TRPV1 expression was related to the inferior OS of patients with ACC and PRAD and good OS of patients with BLCA, HNSCC, PAAD, and READ. (2) For DSS, TRPV1 expression was associated with unfavorable prognosis of patients with ACC, MESO, PRAD, and THCA, and favorable prognosis of patients with BLCA, HNSCC, KIRP, PAAD, and READ. (3) The gene represented a risk factor for DFI of LUAD and PRAD and a protective role for DFI of PAAD. (4) In PFI, TRPV1 expression also acted as a negative factor for patients with ACC, PCPG, and PRAD and a positive role in PAAD patients' prognosis. Taken together, TRPV1 expression was closely relevant to the prognosis of multiple cancers, and it played distinct roles in various cancers.
TRPV1 may be a potential predictor of tumor status. Through AUC values, the significant effects of TRPV1 expression on identifying cancer tissues and their control tissues were detected in several cancers, including BLCA, GBM, KICH, and LUAD. Thus, TRPV1 can be considered an underlying marker for predicting and screening these cancers. To my best knowledge, such a finding has not been previously revealed, and more research focusing on the novel discovery should be performed in the future.
Genome heterogeneity may be an important factor resulting in the different roles of TRPV1 in various cancers. My study discussed the relationships of TRPV1 expression with SNV, DNA methyltransferases, MMR, TMB, and MSI. Missense mutation enables a polypeptide chain encoded by the corresponding gene to lose its original function, and abnormal functions of quite a few proteins result from missense mutations. A typical example is that the wild-type p53 protein has an anti-cancer effect, whereas the missense mutant p53 plays a role in promoting cancer [29,30]. Bosson et al. [31] revealed that TRPV1 missense mutation could alter the reactivity of TRPV1 protein to its natural agonist, suggesting that TRPV1 missense mutation may affect specific processes of cells. Based on my work, missense mutations in TRPV1 are not rare in pan-cancer, indicating that SNVs deserve further study. Similarly, different roles of TRPV1 in various tumors may also be attributed to different statuses of DNA methyltransferases, MMR, TMB, and MSI [32,33,34], with the fact that significant associations of TRPV1 expression with DNA methyltransferases expression and MMR genes expression were demonstrated in my study.
Similar to genome heterogeneity, the immune microenvironment may also affect the roles of TRPV1 in cancers. Increasing evidence supported that TRPV1 participated in pivotal immune cell functions [6]. TRPV1 expression was detected in several immune cells, including T cells, macrophages, dendritic cells, and natural killer cells [35]. Bertin et al. [36] identified the expression of two critical molecules of CD4+ T cells activation — NFAT and NFκB, was downregulated in TRPV1 knockout mice, demonstrating the essential role of TRPV1 in T-cells activating. In my study focusing on pan-cancer, TRPV1 expression was positively related to B cell infiltration levels in DLBC and negatively correlated with dendritic cell infiltration levels in CHOL. A positive correlation between TRPV1 expression and the infiltration levels of neutrophils and macrophages could be detected in SKCM. Negative correlations of TRPV1 expression with three ESTIMATE scores were also discovered in certain cancers including CHOL, LAML, and LGG. Furthermore, TRPV1 expression was positively associated with the expression of quite a few immune checkpoint genes in several cancers – DLBC, LAML, OV, READ, SKCM, THYM, and UVM, suggesting its potential in treatment concerning immune checkpoint blockades.
The mechanisms of TRPV1 in the tumorigenesis and development of cancers remained complex and needed to be further explored. TRPV1 was considered a nonselective cation ion channel (another known function was identified as a nociceptive stimuli receptor [6,37]) and involved in cellular processes: activation of TRPV1 promoted the flow of calcium and sodium ions into cells; subsequently, excessive calcium and sodium ions in the cells led to cell death [38,39]. Indeed, increasingly studies have shown that the mechanism of TRPV1 in cancer is not only here but also involves many aspects of the tumor microenvironment (e.g., extracellular mechanism, angiogenesis, immune regulation) [6,36,40], indicating the complexity of the mechanism of TRPV1 in cancer. Based on the GSEA in my study, TRPV1 was relevant to several metabolic-related pathways (e.g., "AUTOIMMUNE THYROID DISEASE"), immunoregulatory-related pathways (e.g., "CHEMOKINE SIGNALING PATHWAY"), and tumor-related pathways (e.g., "HEDGEHOG SIGNALING PATHWAY"), to some extent implying the potential molecular mechanisms of TRPV1 in pan-cancer. Furthermore, hub genes associated with TRPV1 expression in ACC, BLCA, GBM, and OV were also explored based on WGCNA (i.e., CDCA8 for ACC, HID1 for BLCA, LY6G5B for GBM, and EFR3B, TMSB15B, and EFS for OV.). Previously, CDCA8 has been reported to represent aggressive progression and poor prognosis of ACC [41], and HID1 has been determined as a cancer marker for BLCA development [42]. However, the role of LY6G5B in GBM and the roles of EFR3B, TMSB15B, and EFS in OV have not been reported before; the mechanisms of these hub genes in ACC, BLCA, GBM, and OV also remain unclear, which still need more research to confirm.
Three drugs—I-BET-762, DOLASTATIN 10, and ABBV-075—may target TRPV1, as their IC50 values were negatively related to TRPV1 expression, and low IC50 values of them can be detected in high-TRPV1 expression cells. However, more pharmacological experiments should be performed to verify this finding.
Additionally, the current study provides some ideas for similar clinical investigation: 1) based on pan-cancer data, differentially expressed genes in a variety of tumors can be screened from thousands of genes; 2) using the existing clinical information, the clinical significance of differentially expressed genes can be explored, including prognostic correlation, prediction of tumor status analysis; 3) statistical analysis reveals whether a gene is associated with the immune microenvironment of several tumors and multiple immune checkpoints, to clarify whether the gene has the potential as a target for immunotherapy; 4) the potential molecular mechanism of a gene can be explored by methods such as GSEA; 5) drugs that may target a specific gene can be selected by drug sensitivity analysis. Based on these ideas, a "virtual laboratory" may be helpful for further pharmacological and clinical studies can be constructed.
Some limitations in my study should be concerned. The current research is a retrospective study, and multicenter samples need to be collected for prospective studies to confirm further the expression and clinical significance of TRPV1 in pan-cancer. There are deficiencies in the analysis of the potential mechanism of TRPV1 in pan-cancer. First, the results of underlying mechanism analysis may be biased due to the lack of control of potential confounding factors in carrying out mechanism-related research. Second, due to the lack of data (e.g., ion channel activity state), this study failed to further clarify the potential mechanism of TRPV1 in pan-cancer through machine learning based on big data (e.g., constructing deterministic models) and molecular biology experiments. Third, in addition to the current statistical analysis, future in vivo and in vitro experiments are necessary to clarify the molecular mechanism of TRPV1 in various cancers.
Herein, the present study initially provided an overview of the expression, clinical value, and underlying mechanisms of TRPV1 in numerous cancers. The study revealed TRPV1's potential as a novel biomarker for predicting and treating multiple cancer.
The datasets for this study can be found in the Depmap Portal at https://depmap.org/portal/download/, Xena database (for TCGA data) at http://xena.ucsc.edu/, and Sanger Box 3.0 at http://vip.sangerbox.com/.
The author has no conflicts of interest to declare.
[1] |
F. Bray, M. Laversanne, E. Weiderpass, I. Soerjomataram, The ever-increasing importance of cancer as a leading cause of premature death worldwide, Cancer, 127 (2021), 3029-3030. https://doi.org/10.1002/cncr.33587 doi: 10.1002/cncr.33587
![]() |
[2] |
H. Sung, J. Ferlay, R. L. Siegel, M. Laversanne, I. Soerjomataram, A. Jemal, et al., Global cancer statistics 2020: GLOBOCAN estimates of incidence and mortality worldwide for 36 cancers in 185 countries, CA Cancer J. Clin., 71 (2021), 209-249. https://doi.org/10.3322/caac.21660 doi: 10.3322/caac.21660
![]() |
[3] |
W. Chen, Z. Sun, L. Lu, Targeted engineering of medicinal chemistry for cancer therapy: Recent advances and perspectives, Angew Chem. Int. Ed. Engl., 60 (2021), 5626-5643. https://doi.org/10.1002/anie.201914511 doi: 10.1002/anie.201914511
![]() |
[4] |
J. Berk-Krauss, J. A. Stein, J. Weber, D. Polsky, A. C. Geller, New Systematic Therapies and Trends in Cutaneous Melanoma Deaths Among US Whites, 1986-2016, Am. J. Public Health, 110 (2020), 731-733. https://doi.org/10.2105/AJPH.2020.305567 doi: 10.2105/AJPH.2020.305567
![]() |
[5] |
P. A. Ott, E. Elez, S. Hiret, D. W. Kim, A. Morosky, S. Saraf, et al., Pembrolizumab in patients with extensive-stage small-cell lung cancer: results from the phase Ib KEYNOTE-028 study, J. Clin. Oncol., 35 (2017), 3823-3829. https://doi.org/10.1200/JCO.2017.72.5069 doi: 10.1200/JCO.2017.72.5069
![]() |
[6] |
L. Li, C. Chen, C. Chiang, T. Xiao, Y. Chen, Y. Zhao, et al., The impact of TRPV1 on cancer pathogenesis and therapy: A systematic review, Int. J. Biol. Sci, . 17 (2021), 2034-2049. https://doi.org/10.7150/ijbs.59918 doi: 10.7150/ijbs.59918
![]() |
[7] |
K. Zhai, A. Liskova, P. Kubatka, D. Busselberg, Calcium entry through TRPV1: A potential target for the regulation of proliferation and apoptosis in cancerous and healthy cells, Int. J. Mol. Sci., 21 (2020). https://doi.org/10.3390/ijms21114177 doi: 10.3390/ijms21114177
![]() |
[8] |
K. Stock, J. Kumar, M. Synowitz, S. Petrosino, R. Imperatore, E. S. Smith, et al., Neural precursor cells induce cell death of high-grade astrocytomas through stimulation of TRPV1, Nat. Med., 18 (2012), 1232-1238. https://doi.org/10.1038/nm.2827 doi: 10.1038/nm.2827
![]() |
[9] |
L. V. Weber, K. Al-Refae, G. Wolk, G. Bonatz, J. Altmuller, C. Becker, et al., Expression and functionality of TRPV1 in breast cancer cells, Breast Cancer (Dove Med Press), 8 (2016), 243-252. https://doi.org/10.2147/BCTT.S121610 doi: 10.2147/BCTT.S121610
![]() |
[10] |
M. Ghandi, F. W. Huang, J. Jane-Valbuena, G. V. Kryukov, C. C. Lo, E. R. McDonald, et al., Next-generation characterization of the Cancer Cell Line Encyclopedia, Nature, 569 (2019), 503-508. https://doi.org/10.1038/s41586-019-1186-3 doi: 10.1038/s41586-019-1186-3
![]() |
[11] |
R. Beroukhim, C. H. Mermel, D. Porter, G. Wei, S. Raychaudhuri, J. Donovan, et al., The landscape of somatic copy-number alteration across human cancers, Nature, 463 (2010), 899-905. https://doi.org/10.1038/nature08822 doi: 10.1038/nature08822
![]() |
[12] |
T. Li, J. Fan, B. Wang, N. Traugh, Q. Chen, J. S. Liu, et al., TIMER: A web server for comprehensive analysis of tumor-infiltrating immune cells, Cancer Res., 77 (2017), e108-e110. https://doi.org/10.1158/0008-5472.CAN-17-0307 doi: 10.1158/0008-5472.CAN-17-0307
![]() |
[13] |
K. Yoshihara, M. Shahmoradgoli, E. Martinez, R. Vegesna, H. Kim, W. Torres-Garcia, et al., Inferring tumour purity and stromal and immune cell admixture from expression data, Nat. Commun., 4 (2013), 2612. https://doi.org/10.1038/ncomms3612 doi: 10.1038/ncomms3612
![]() |
[14] |
T. Li, J. Fu, Z. Zeng, D. Cohen, J. Li, Q. Chen, et al., TIMER2.0 for analysis of tumor-infiltrating immune cells, Nucleic Acids Res., 48 (2020), W509-W514. https://doi.org/10.1093/nar/gkaa407 doi: 10.1093/nar/gkaa407
![]() |
[15] |
J. Hu, B. Othmane, A. Yu, H. Li, Z. Cai, X. Chen, et al., 5mC regulator-mediated molecular subtypes depict the hallmarks of the tumor microenvironment and guide precision medicine in bladder cancer, BMC Med., 19 (2021), 289. https://doi.org/10.1186/s12916-021-02163-6 doi: 10.1186/s12916-021-02163-6
![]() |
[16] |
S. Fu, B. Gong, S. Wang, Q. Chen, Y. Liu, C. Zhuang, et al., Prognostic value of long noncoding rna dleu2 and its relationship with immune infiltration in kidney renal clear cell carcinoma and liver hepatocellular carcinoma, Int. J. Gen. Med., 14 (2021), 8047-8064. https://doi.org/10.2147/IJGM.S336428 doi: 10.2147/IJGM.S336428
![]() |
[17] |
W. Li, J. A. Ma, X. Sheng, C. Xiao Screening of CXC chemokines in the microenvironment of ovarian cancer and the biological function of CXCL10, World J. Surg. Oncol., 19 (2021), 329. https://doi.org/10.1186/s12957-021-02440-x doi: 10.1186/s12957-021-02440-x
![]() |
[18] |
Z. Zhuang, H. Cai, H. Lin, B. Guan, Y. Wu, Y. Zhang, et al., Development and validation of a robust pyroptosis-related signature for predicting prognosis and immune status in patients with colon cancer, J. Oncol., 2021 (2021), 5818512. https://doi.org/10.1155/2021/5818512 doi: 10.1155/2021/5818512
![]() |
[19] |
Y. Wang, Y. Tian, S. Liu, Z. Wang, Q. Xing, Prognostic value and immunological role of AXL gene in clear cell renal cell carcinoma associated with identifying LncRNA/RBP/AXL mRNA networks, Cancer Cell Int., 21 (2021), 625. https://doi.org/10.1186/s12935-021-02322-y doi: 10.1186/s12935-021-02322-y
![]() |
[20] |
Y. Zhu, Y. Zhou, H. Jiang, Z. Chen, B. Lu, Analysis of core genes for colorectal cancer prognosis based on immune and stromal scores, PeerJ, 9 (2021), e12452. https://doi.org/10.7717/peerj.12452 doi: 10.7717/peerj.12452
![]() |
[21] |
G. Yu, L. G. Wang, Y. Han, Q. Y. He, clusterProfiler: an R package for comparing biological themes among gene clusters, OMICS, 16 (2012), 284-287. https://doi.org/10.1089/omi.2011.0118 doi: 10.1089/omi.2011.0118
![]() |
[22] |
P. Langfelder, S. Horvath WGCNA: An R package for weighted correlation network analysis, BMC Bioinform., 9 (2008), 559. https://doi.org/10.1186/1471-2105-9-559 doi: 10.1186/1471-2105-9-559
![]() |
[23] |
W. C. Reinhold, M. Sunshine, H. Liu, S. Varma, K. W. Kohn, J. Morris, et al., CellMiner: A web-based suite of genomic and pharmacologic tools to explore transcript and drug patterns in the NCI-60 cell line set, Cancer Res., 72 (2012), 3499-3511. https://doi.org/10.1158/0008-5472.CAN-12-1370 doi: 10.1158/0008-5472.CAN-12-1370
![]() |
[24] |
J. Luo, L. Wu, D. Liu, Z. Xiong, L. Wang, X. Qian, et al., Gene regulatory network analysis identifies key genes and regulatory mechanisms involved in acute myocardial infarction using bulk and single cell RNA-seq data, Math. Biosci. Eng., 18 (2021), 7774-7789. https://doi.org/10.3934/mbe.2021386 doi: 10.3934/mbe.2021386
![]() |
[25] |
J. Cheng, J. Zhang, Z. Wu, X. Sun, Inferring microenvironmental regulation of gene expression from single-cell RNA sequencing data using scMLnet with an application to COVID-19, Brief Bioinform., 22 (2021), 988-1005. https://doi.org/10.1093/bib/bbaa327 doi: 10.1093/bib/bbaa327
![]() |
[26] |
G. H. Han, D. B. Chay, S. Nam, H. Cho, J. Y. Chung, J. H. Kim, Prognostic significance of Transient Receptor Potential Vanilloid Type 1 (TRPV1) and Phosphatase and Tension Homolog (PTEN) in epithelial ovarian cancer, Cancer Genom. Proteom., 17 (2020), 309-319. https://doi.org/10.21873/cgp.20191 doi: 10.21873/cgp.20191
![]() |
[27] |
G. H. Han, D. B. Chay, S. Nam, H. Cho, J. Y. Chung, J. H. Kim, The combination of Transient Receptor Potential Vanilloid Type 1 (TRPV1) and Phosphatase and Tension Homolog (PTEN) is an effective prognostic biomarker in cervical cancer, Int. J. Gynecol. Pathol., 40 (2021), 214-223. https://doi.org/10.1097/PGP.0000000000000677 doi: 10.1097/PGP.0000000000000677
![]() |
[28] |
N. Gao, F. Yang, S. Chen, H. Wan, X. Zhao, H. Dong, The role of TRPV1 ion channels in the suppression of gastric cancer development, J. Exp. Clin. Cancer Res., 39 (2020), 206. https://doi.org/10.1186/s13046-020-01707-7 doi: 10.1186/s13046-020-01707-7
![]() |
[29] |
M. C. Liebl, T. G. Hofmann, The Role of p53 Signaling in Colorectal Cancer, Cancers (Basel), 13 (2021). https://doi.org/10.3390/cancers13092125 doi: 10.3390/cancers13092125
![]() |
[30] |
V. J. N. Bykov, S. E. Eriksson, J. Bianchi, K. G. Wiman, Targeting mutant p53 for efficient cancer therapy, Nat. Rev. Cancer, 18 (2018), 89-102. https://doi.org/10.1038/nrc.2017.109 doi: 10.1038/nrc.2017.109
![]() |
[31] |
C. Bosson, J. Rendu, L. Pelletier, A. Abriat, A. Chatagnon, J. Brocard, et al., Variations in the TRPV1 gene are associated to exertional heat stroke, J. Sci. Med. Sport, 23 (2020), 1021-1027. https://doi.org/10.1016/j.jsams.2020.04.018 doi: 10.1016/j.jsams.2020.04.018
![]() |
[32] |
X. He, C. Xu, Immune checkpoint signaling and cancer immunotherapy, Cell Res., 30 (2020), 660-669. https://doi.org/10.1038/s41422-020-0343-4 doi: 10.1038/s41422-020-0343-4
![]() |
[33] |
M. Greally, J. F. Chou, W. K. Chatila, M. Margolis, M. Capanu, J. F. Hechtman, et al., Clinical and molecular predictors of response to immune checkpoint inhibitors in patients with advanced esophagogastric cancer, Clin. Cancer Res., 25 (2019), 6160-6169. https://doi.org/10.1158/1078-0432.CCR-18-3603 doi: 10.1158/1078-0432.CCR-18-3603
![]() |
[34] |
C. M. Fares, E. M. Van Allen, C. G. Drake, J. P. Allison, S. Hu-Lieskovan, Mechanisms of resistance to immune checkpoint blockade: Why does checkpoint inhibitor immunotherapy not work for all patients?, Am. Soc. Clin. Oncol. Educ. Book, 39 (2019), 147-164. https://doi.org/10.1200/EDBK_240837 doi: 10.1200/EDBK_240837
![]() |
[35] |
J. K. Bujak, D. Kosmala, I. M. Szopa, K. Majchrzak, P. Bednarczyk, Inflammation, Cancer and Immunity-Implication of TRPV1 Channel, Front. Oncol., 9 (2019), 1087. https://doi.org/10.3389/fonc.2019.01087 doi: 10.3389/fonc.2019.01087
![]() |
[36] |
S. Bertin, Y. Aoki-Nonaka, P. R. de Jong, L. L. Nohara, H. Xu, S. R. Stanwood, et al., The ion channel TRPV1 regulates the activation and proinflammatory properties of CD4(+) T cells, Nat. Immunol., 15 (2014), 1055-1063. https://doi.org/10.1038/ni.3009 doi: 10.1038/ni.3009
![]() |
[37] |
K. Zhang, D. Julius, Y. Cheng, Structural snapshots of TRPV1 reveal mechanism of polymodal functionality, Cell, 184 (2021), 5138-5150. https://doi.org/10.1016/j.cell.2021.08.012 doi: 10.1016/j.cell.2021.08.012
![]() |
[38] |
L. Pecze, B. Viskolcz, Z. Olah, Molecular surgery concept from bench to bedside: A focus on TRPV1+ pain-sensing neurons, Front. Physiol., 8 (2017), 378. https://doi.org/10.3389/fphys.2017.00378 doi: 10.3389/fphys.2017.00378
![]() |
[39] |
L. Pecze, W. Blum, T. Henzi, B. Schwaller, Endogenous TRPV1 stimulation leads to the activation of the inositol phospholipid pathway necessary for sustained Ca(2+) oscillations, Biochim. Biophys. Acta, 1863 (2016), 2905-2915. https://doi.org/10.1016/j.bbamcr.2016.09.013 doi: 10.1016/j.bbamcr.2016.09.013
![]() |
[40] |
N. Erin, Role of sensory neurons, neuroimmune pathways, and transient receptor potential vanilloid 1 (TRPV1) channels in a murine model of breast cancer metastasis, Cancer Immunol. Immunother, 69 (2020), 307-314. https://doi.org/10.1007/s00262-019-02463-0 doi: 10.1007/s00262-019-02463-0
![]() |
[41] |
X. Tian, W. Xu, Y. Wang, A. Anwaier, H. Wang, F. Wan, et al., Identification of tumor-infiltrating immune cells and prognostic validation of tumor-infiltrating mast cells in adrenocortical carcinoma: results from bioinformatics and real-world data, Oncoimmunology, 9 (2020), 1784529. https://doi.org/10.1080/2162402X.2020.1784529 doi: 10.1080/2162402X.2020.1784529
![]() |
[42] |
R. de Matos Simoes, S. Dalleau, K. E. Williamson, F. Emmert-Streib, Urothelial cancer gene regulatory networks inferred from large-scale RNAseq, Bead and Oligo gene expression data, BMC Syst. Biol., 9 (2015), 21. DOI:10.1186/s12918-015-0165-z doi: 10.1186/s12918-015-0165-z
![]() |
![]() |
![]() |
![]() |
![]() |
1. | Arpad Szallasi, “ThermoTRP” Channel Expression in Cancers: Implications for Diagnosis and Prognosis (Practical Approach by a Pathologist), 2023, 24, 1422-0067, 9098, 10.3390/ijms24109098 | |
2. | Subramanyam R. Chinreddy, Nicole Tendayi Mashozhera, Badraldeen Rashrash, Gerardo Flores-Iga, Padma Nimmakayala, Gerald R. Hankins, Robert T. Harris, Umesh K. Reddy, Unraveling TRPV1’s Role in Cancer: Expression, Modulation, and Therapeutic Opportunities with Capsaicin, 2024, 29, 1420-3049, 4729, 10.3390/molecules29194729 | |
3. | Sara J. Bonvini, Michael A. Wortley, Maria G. Belvisi, 2024, 9780443186530, 257, 10.1016/B978-0-443-18653-0.00030-7 | |
4. | Xin Liu, Mengying Zhang, Chongyang He, Shubing Jia, Rongwu Xiang, Yijia Xu, Mingyi Zhao, Research focus and thematic trends of transient receptor potential vanilloid member 1 research: a bibliometric analysis of the global publications (1990–2023), 2023, 0028-1298, 10.1007/s00210-023-02709-w |