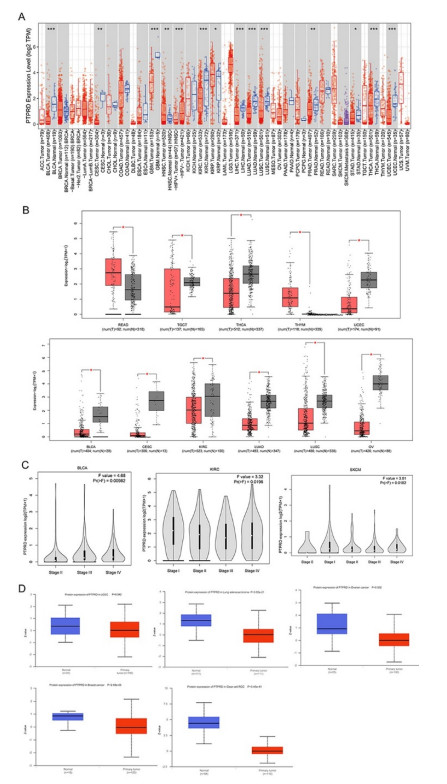
PTPRD plays an indispensable role in the occurrence of multiple tumors. However, pan-cancer analysis is unavailable. The purpose of this research was to preliminarily study its prognostic landscape across various tumors and investigate its relationship with immunotherapy. We exhibited the expression profile, survival analysis, and genomic alterations of PTPRD based on the TIMER, GEPIA, UALCAN, PrognoScan and cBioPortal database. The frequency of PTPRD mutation and its correlation with response to immunotherapy were evaluated using the cBioPortal database. The relationship between PTPRD and immune-cell infiltration was analyzed by the TIMER and TISIDB databases. A protein interaction network was constructed by the STRING database. GO and KEGG enrichment analysis was executed by the Metascape database. A correlation between PTPRD expression and prognosis was found in various cancers. Aberrant PTPRD expression was closely related to immune infiltration. In non-small cell lung cancer and melanoma, patients with PTPRD mutations had better overall survival with immune checkpoint inhibitors, and these patients had higher TMB scores. PTPRD mutation was involved in numerous biological processes, including immunological signaling pathways. A PTPRD protein interaction network was constructed, and genes that interacted with PTPRD were identified. Functional enrichment analysis demonstrated that a variety of GO biological processes and KEGG pathways associated with PTPRD were involved in the therapeutic mechanisms. These results revealed that PTPRD might function as a biomarker for prognosis and immune infiltration in cancers, throwing new light on cancer therapeutics.
Citation: Chunpei Ou, Qin Peng, Changchun Zeng. An integrative prognostic and immune analysis of PTPRD in cancer[J]. Mathematical Biosciences and Engineering, 2022, 19(6): 5361-5379. doi: 10.3934/mbe.2022251
[1] | Ying Liu, Xiao Ma, Linyuan Feng, Zhenhua Lin, Xianchun Zhou . An integrative pan-cancer analysis reveals the carcinogenic effects of NCAPH in human cancer. Mathematical Biosciences and Engineering, 2023, 20(1): 76-92. doi: 10.3934/mbe.2023005 |
[2] | Sidan Long, Shuangshuang Ji, Kunmin Xiao, Peng Xue, Shijie Zhu . Prognostic and immunological value of LTB4R in pan-cancer. Mathematical Biosciences and Engineering, 2021, 18(6): 9336-9356. doi: 10.3934/mbe.2021459 |
[3] | Jianpei Hu, Zengnan Mo . Dissection of tumor antigens and immune landscape in clear cell renal cell carcinoma: Preconditions for development and precision medicine of mRNA vaccine. Mathematical Biosciences and Engineering, 2023, 20(2): 2157-2182. doi: 10.3934/mbe.2023100 |
[4] | Xiaowei Zhang, Jiayu Tan, Xinyu Zhang, Kritika Pandey, Yuqing Zhong, Guitao Wu, Kejun He . Aggrephagy-related gene signature correlates with survival and tumor-associated macrophages in glioma: Insights from single-cell and bulk RNA sequencing. Mathematical Biosciences and Engineering, 2024, 21(2): 2407-2431. doi: 10.3934/mbe.2024106 |
[5] | Jun Wang, Mingzhi Gong, Zhenggang Xiong, Yangyang Zhao, Deguo Xing . Immune-related prognostic genes signatures in the tumor microenvironment of sarcoma. Mathematical Biosciences and Engineering, 2021, 18(3): 2243-2257. doi: 10.3934/mbe.2021113 |
[6] | Ye Hu, Meiling Wang, Kainan Wang, Jiyue Gao, Jiaci Tong, Zuowei Zhao, Man Li . A potential role for metastasis-associated in colon cancer 1 (MACC1) as a pan-cancer prognostic and immunological biomarker. Mathematical Biosciences and Engineering, 2021, 18(6): 8331-8353. doi: 10.3934/mbe.2021413 |
[7] | Jie Wang, Md. Nazim Uddin, Rehana Akter, Yun Wu . Contribution of endothelial cell-derived transcriptomes to the colon cancer based on bioinformatics analysis. Mathematical Biosciences and Engineering, 2021, 18(6): 7280-7300. doi: 10.3934/mbe.2021360 |
[8] | Dong-feng Li, Aisikeer Tulahong, Md. Nazim Uddin, Huan Zhao, Hua Zhang . Meta-analysis identifying epithelial-derived transcriptomes predicts poor clinical outcome and immune infiltrations in ovarian cancer. Mathematical Biosciences and Engineering, 2021, 18(5): 6527-6551. doi: 10.3934/mbe.2021324 |
[9] | Yuan Yang, Lingshan Zhou, Xi Gou, Guozhi Wu, Ya Zheng, Min Liu, Zhaofeng Chen, Yuping Wang, Rui Ji, Qinghong Guo, Yongning Zhou . Comprehensive analysis to identify DNA damage response-related lncRNA pairs as a prognostic and therapeutic biomarker in gastric cancer. Mathematical Biosciences and Engineering, 2022, 19(1): 595-611. doi: 10.3934/mbe.2022026 |
[10] | Yan He, Nannan Cao, Yanan Tian, Xuelin Wang, Qiaohong Xiao, Xiaojuan Tang, Jiaolong Huang, Tingting Zhu, Chunhui Hu, Ying Zhang, Jie Deng, Han Yu, Peng Duan . Development and validation of two redox-related genes associated with prognosis and immune microenvironment in endometrial carcinoma. Mathematical Biosciences and Engineering, 2023, 20(6): 10339-10357. doi: 10.3934/mbe.2023453 |
PTPRD plays an indispensable role in the occurrence of multiple tumors. However, pan-cancer analysis is unavailable. The purpose of this research was to preliminarily study its prognostic landscape across various tumors and investigate its relationship with immunotherapy. We exhibited the expression profile, survival analysis, and genomic alterations of PTPRD based on the TIMER, GEPIA, UALCAN, PrognoScan and cBioPortal database. The frequency of PTPRD mutation and its correlation with response to immunotherapy were evaluated using the cBioPortal database. The relationship between PTPRD and immune-cell infiltration was analyzed by the TIMER and TISIDB databases. A protein interaction network was constructed by the STRING database. GO and KEGG enrichment analysis was executed by the Metascape database. A correlation between PTPRD expression and prognosis was found in various cancers. Aberrant PTPRD expression was closely related to immune infiltration. In non-small cell lung cancer and melanoma, patients with PTPRD mutations had better overall survival with immune checkpoint inhibitors, and these patients had higher TMB scores. PTPRD mutation was involved in numerous biological processes, including immunological signaling pathways. A PTPRD protein interaction network was constructed, and genes that interacted with PTPRD were identified. Functional enrichment analysis demonstrated that a variety of GO biological processes and KEGG pathways associated with PTPRD were involved in the therapeutic mechanisms. These results revealed that PTPRD might function as a biomarker for prognosis and immune infiltration in cancers, throwing new light on cancer therapeutics.
The prevalence and mortality of cancer are rising worldwide, making cancer the most common cause of mortality in many countries. The American Cancer Society estimates that 1,898,160 new cancer cases and 608,570 cancer deaths are likely to occur in the United States in 2021 [1]. Therefore, identifying a new target for optimizing cancer treatment is an immediate and severe global demand. The personalized medicine strategy may strengthen the therapeutic benefit by identifying the tumor-specific target or tumor-associated characteristics [2]. Although cancers share common features, there is currently no one-size-fits-all solution to the disease. With advances in genetics and cancer genome research, we recognize that cancers are heterogeneous and vary greatly both in origin and genetic alteration [3,4]. Meanwhile, commonalities, such as driver alterations, pathways, mutational signatures, immune signatures, microbial signatures and pan-cancer studies revealed the potential for targeting common features across different cancer types using the same therapeutic strategies [5]. Therefore, pan-cancer research is valuable in understanding the role of genes in cancer.
Protein tyrosine phosphatase receptor type D (PTPRD) is a member of the protein tyrosine phosphatase (PTP) family, several members of which can regulate a variety of cellular processes, including cell proliferation, differentiation, cell cycle, and malignant transformation [6]. The PTPRD gene is located on chromosome 9p, and its canonical model contains 1912 amino acids, with an estimated mass of 215 kDa. PTPRD is a receptor-type PTP with an extracellular region, which is composed of three Ig-like and eight fibronectin type Ⅲ-like domains, a single transmembrane segment, and two tandem intracytoplasmic catalytic domains, which possess tyrosine-protein phosphatase 1 domain (D1) and tyrosine-protein phosphatase 2 domain (D2). The physiological function of PTPRD depends primarily on its D1 domain, which facilitates the phosphate interaction of cytoplasmic proteins, while the D2 domain can mediate the function of the D1 domain. Recently, the relationship between PTPRD and tumorigenesis has received growing attention. PTPRD participates in multiple signaling pathways, including β-catenin/ T-cell factor (TCF), signal transduction and activation of transcription 3 (STAT3), extracellular-signal-regulated kinase (ERK), and insulin signaling pathway [7,8]. The PTPRD expression levels were down-regulated in colon cancer, and PTPRD regulated the colon cancer cell adhesion and migration in cooperation with β-catenin/TCF signaling [9]. PTPRD deficiency was linked with aggressive behavior in gastric cancer, and PTPRD inactivation facilitated angiogenesis and metastasis of gastric cancer via the upregulation of CXCL8 [10]. These results suggested that PTPRD was involved in the invasion and progression of a variety of tumors. However, PTPRD has not been intensively investigated in the pan-cancer.
Immune checkpoint inhibitors (ICIs) have redefined therapies for various types of cancer. However, despite the remarkable performance of ICIs, long-lasting therapeutic responses differ among patients [11]. It has recently been found that high microsatellite instability (MSI-H), PD-L1 expression, tumor mutation burden (TMB), gene expression profiles (GEPs), tumor immune microenvironment (TIME), and some specific gene mutations are associated with immunotherapy response [12,13,14]. Even those biomarkers that have been identified and validated have certain clinical implementation restrictions. For instance, 44–50% of high TMB or high PD-L1 expression advanced non-small-cell lung cancer (NSCLC) patients had no response to nivolumab plus ipilimumab, while almost 12–15% of low TMB or low PD-L1 expression patients obtained a partial or complete response in the CheckMate 568 (NCT02659059) study [15]. Therefore, there is an urgent need for predictive biomarkers of response to ICIs. Outlining the mechanisms of tumor development and immune infiltration will lay the foundation for future clinical progress. PTPRD restricts STAT3 phosphorylation to suppress the activation of STAT3, contributing to the inhibition of PD-L1 expression, which is involved in the immune response of malignant tumors [7,16]. Accordingly, PTPRD has potential predictive significance for immunotherapy [7]. However, the underlying features and mechanisms of PTPRD in pan-cancer immunology remain unclear.
In this study, we intensively investigated PTPRD expression and association with cancer patient prognosis using databases such as TIMER, GEPIA2, UALCAN, PrognoScan. Moreover, the relationship between PTPRD mutation and immunotherapy was further explored using the cBioPortal database, and a survival advantage could be observed in PTPRD mutated patients who obtained ICIs. Additionally, we analyzed the association of PTPRD and tumor microenvironments through the TIMER and TISIDB database, indicating that PTPRD is a potential predictive biomarker for immunotherapy. The results illustrated the potential role of PTPRD and provided the association and underlying mechanisms between PTPRD and tumor-immune behaviors across multiple cancer types.
To explore the differential gene expression of PTPRD between tumor and normal tissue, pan-cancer analysis of PTPRD mRNA expression in 32 tumor types from The Cancer Genome Atlas (TCGA) database was performed using the "Diff Exp" module of Tumor Immune Estimation Resource (TIMER; https://cistrome.shinyapps.io/timer/) database [17]. Moreover, the "Expression analysis" module of the Gene Expression Profiling Interactive Analysis (GEPIA2; http://gepia2.cancer-pku.cn/#analysis) database was used to profile the tissue-wise expression of PTPRD in different cancer types using the data from TCGA and Genotype-Tissue Expression (GTEx) database. The threshold was set according to the following values: P-value cutoff = 0.01, log2FC (fold change) cutoff = 1, and "Match TCGA normal and GTEx data". We profile the expression of PTPRD in different cancer stages using log2 [TPM (Transcripts per million) + 1] transformed expression data to obtain violin plots. The UALCAN portal (http://ualcan.path.uab.edu/analysis-prot.html) provided the protein expression analysis option for PTPRD using data from the Clinical proteomic tumor analysis consortium (CPTAC) dataset [18].
To investigate the relationship between PTPRD expression and survival across various types of cancers, the survival analysis was performed using the "survival analysis" module of GEPIA2 based on the TCGA and GTEx database. Group cutoff was set as the median. Additionally, the PrognoScan database (http://dna00.bio.kyutech.ac.jp/PrognoScan/index.html) was used to assess the prognostic value across a wide collection of accessible microarray datasets from the Gene Expression Omnibus (GEO) database. The significance threshold was Cox P-value < 0.05 and N > 100 [19].
The cBioPortal (https://www.cbioportal.org/) database was applied to analyze and visualize the genomic data. "TCGA PanCancer Atlas Studies" containing 10,967 samples from 32 studies was selected to query the genetic alteration features of PTPRD, and the "Cancer Types Summary" module exhibited the alteration frequency, mutation type, and cancer types [20]. Additionally, genomic and survival data containing 2185 samples from four studies were selected to explore the relationship between PTPRD mutation and immunotherapy, and the association of PTPRD mutation with clinical attributes was assessed in the selected cases [21,22,23,24].
TIMER database containing 10,897 samples from the TCGA database across 32 cancer types was applied to systematically analyze immune infiltrates with a deconvolution statistical method to deduce tumor-infiltrating immune cells (TIICs) abundance from gene expression profiles. We explore the relationship between PTPRD expression and the TIICs abundance, including macrophages, CD4+ T cells, B cells, neutrophils, CD8+ T cells, and dendritic cells. P-values and partial correlation (cor) values were calculated utilizing a purity-adjusted Spearman rank correlation test. The association between PTPRD mutation and TIICs, including dendritic cells (DCs), B cells, CD8+ T cells, M1 macrophages, T cells (general), TAMs, natural killer (NK) cells, T-helper 2 (Th2) cells, follicular helper T (Tfh) cells, M2 macrophages, monocytes, T-helper 1 (Th1) cells, neutrophils, Tregs, T-helper 17 (Th17) cells, and exhausted T cells, were further analyzed using the data from TCGA database [17,25,26]. Additionally, the association between PTPRD mutation and infiltrating immune cells was further investigated by TISIDB (http://cis.hku.hk/TISIDB/index.php) database, which integrated data from the TCGA database [27].
GEPIA database was applied to obtain genes that had a similar expression pattern with PTPRD expression based on the data from the TCGA database, including 9736 tumors and 8587 normal samples, and the top 100 genes were selected based on the correlation coefficient using the Spearman method. Moreover, the correlation analysis was conducted between PTPRD expression and the top 5 gene expression in multiple cancer types and tissues using the Pearson method [28].
The STRING (http://string-db.org) database was applied to exhibit a protein-protein interaction network for PTPRD protein. The hub genes were screened with the following criteria: no more than 50 interactors; low confidence (0.150) as the minimum required interaction score [29].
The systematic and integrative functional enrichment analysis was provided by Metascape (http://metascape.org/gp/index.html#/main/step1) database. The 100 PTPRD-correlated targeting genes from the gene correlation analysis and the identified 50 PTPRD-correlated targeting proteins from the PPI network analysis were placed into the Metascape database for Gene Ontology (GO) and Kyoto Encyclopedia of Genes and Genomes (KEGG) pathways enrichment analysis [30].
The Wilcoxon test was applied to assess the expression differences of PTPRD between tumor and normal tissues. Overall survival (OS) was evaluated using Kaplan–Meier method and log-rank tests. The association of PTPRD expression and immune infiltration level was assessed by the Spearman rank correlation test. The correlation of PTPRD mutation and clinical attributes was determined by Kruskal Wallis Test or Chi-squared Test. The p-values < 0.05 were considered statistically significant.
To determine the expression differences of PTPRD in tumor tissues and normal tissues, PTPRD mRNA levels in more than 10,000 tumor and normal tissue samples across 23 cancer types from the TCGA database were evaluated using the TIMER database (Table S1). As shown in Figure 1A, PTPRD was downregulated in lung adenocarcinoma (LUAD), uterine corpus endometrial carcinoma (UCEC), kidney renal clear cell carcinoma (KIRC), bladder carcinoma (BLCA), head and neck carcinoma (HNSC) HPV+, liver hepatocellular carcinoma (LIHC), lung squamous carcinoma (LUSC), glioblastoma multiforme (GBM), thyroid carcinoma (THCA), prostate adenocarcinoma (PRAD), cervical squamous carcinoma (CESC), and stomach adenocarcinoma (STAD) compared with the adjacent normal tissues. PTPRD was upregulated in head and neck carcinoma (HNSC), and kidney renal papillary cell carcinoma (KIRP) compared to the normal tissues. Moreover, we further explored PTPRD expression across 33 cancer types using the GEPIA2 database, containing the TCGA and GTEx data. As shown in Figure 1B, PTPRD expression was lower in BLCA, CESC, KIRC, LUAD, LUSC, ovarian serous cystadenocarcinoma (OV), THCA, UCEC, and testicular germ cell tumors (TGCT) compared with the normal tissues, and PTPRD expression was higher in rectum adenocarcinoma (READ) and thymoma (THYM). Moreover, a significant correlation between PTPRD expression and the pathological stages was observed using the GEPIA2 database in multiple cancers, including BLCA, KIRC, and skin cutaneous melanoma (SKCM) (Figure 1C). These findings suggested that PTPRD might perform diverse functions in cancer progression. Also, the UALCAN database was applied to analyze PTPRD protein expression across tumor and normal cases in the CPTAC datasets, and the results showed that lower protein expression of PTPRD was observed in UCEC, LUAD, clear cell renal cell carcinoma (ccRCC), ovarian cancer, and breast cancer compared with adjacent normal tissues (Figure 1D).
To investigate the prognostic value of PTPRD across multiple cancers from the TCGA project, the impact of PTPRD expression on survival was evaluated using the GEPIA2 database. As shown in Figure 2A, low PTPRD expression was associated with a better OS (overall survival) for STAD, uveal melanoma (UVM), SKCM and BLCA, while low PTPRD expression was related to the poor OS for READ and brain lower-grade glioma (LGG). Low PTPRD expression was linked to a better DFS (disease-free survival) for UVM and KIRP, while low PTPRD expression was related to poor DFS for KIRP. To further investigate the prognostic potential of PTPRD in different tumors, the PrognoScan database was used to assess the relationship between PTPRD expression and prognosis of patients with different cancers based on the data retrieved from Gene Expression Omnibus (GEO) datasets (Table S2). The results exhibited that PTPRD expression affected the survival of breast cancer, ovarian cancer, lung cancer, and liposarcoma. As shown in Figure 2B, the cohort (GSE8894, N = 138, P = 0.029) of lung cancer patients with low PTPRD expression exhibited a poorer relapse-free survival. The cohort (GSE30929, N = 140, P = 0.003) containing 140 samples of liposarcoma showed that low PTPRD expression was observably associated with improved distant recurrence-free survival (Figure 2C). Similarly, the GSE26712 (N = 185, P = 0.002) and GSE9891 (N = 278, P = 0.001) cohorts containing 185 and 278 ovarian cancer cases, respectively, showed that low PTPRD expression was significantly associated with improved overall survival (Figure 2D, E). Additionally, patients with low PTPRD expression had poorer disease-specific survival in the GSE3494-GPL96 cohort (N = 236, P = 0.003) (Figure 2F), and patients with low PTPRD expression was correlated with improved distant metastasis-free survival in the GSE2034 cohort (N = 286, P = 0.030) (Figure 2G). Therefore, it is conceivable that PTPRD expression may have an impact on the prognosis of multiple cancers.
It has been broadly recognized that genomic alteration is closely linked to tumorigenesis. To investigate the genomic aberration of PTPRD in pan-cancer, a combined study containing 10,967 samples from 32 studies was extracted from the TCGA database for the genetic alteration analysis (Table S3). The mutation frequency and genetic alteration type of PTPRD were explored in various cancers (Figure 3A). The mutation frequency of PTPRD was 9.5% (1045/10950) in the TCGA pan-cancer cohort with stomach adenocarcinoma (25.2%) ranking first followed by skin cutaneous melanoma (25.0%), lung adenocarcinoma (23.1%), and head and neck squamous cell carcinoma (19.1%). The genetic alteration profiling of PTPRD exhibited that its deep deletion was one of the most significant factors for alteration in stomach adenocarcinoma, cholangiocarcinoma, testicular germ cell tumors, head and neck squamous cell carcinoma, lung squamous cell carcinoma, bladder urothelial carcinoma, and lung adenocarcinoma. As shown in Figure 3B, PTPRD mutations have abundant types of mutation alteration, including missense, nonsense, silent mutation, insertion or deletion, duplication, and frameshift mutation, and G203E, S431L, R705Q, and L1053I sites were observed many times. Moreover, the association between genetic aberrations of PTPRD and the survival prognosis of cases with various types of tumors has been investigated, and the results exhibited that kidney renal clear cell carcinoma cases with altered PTPRD mutations had worse prognosis in disease-specific (P = 1.780e-5), progression-free (P = 3.899e-4) and overall (P = 6.366e-4) survival, but not disease-free (P = 0.595) survival, compared with cases with unaltered PTPRD (Figure 3C–E).
A combined cohort of 2185 immune checkpoint blockade (ICB)-treated patients (249 from Dana Farber Cancer Institute (DFCI) and 1936 from Memorial Sloan Kettering Cancer Center (MSKCC)) were further used to investigate the relationship between PTPRD mutation and response to ICB therapy (Table S4). A comparatively high proportion of PTPRD mutation cases occurred in patients with melanoma (20.59% of 471 cases) and non-small cell lung cancer (12.48% of 681 cases) (Figure 4A). Moreover, a significant difference in the number of coexisting mutations between patients with PTPRD mutation type and PTPRD wild type. For instance, PTPRD mutation coexisted more mutated NF1 (36.84% VS. 8.89%), PTPRT (34.65% VS. 8.02%), GRIN2A (28.95 VS. 5.88%), PAK5 (25.55% VS. 4.70%) and FLT1 (21.49% VS. 3.88%) (Figure 4B). TP53, NF1, PTPRT, TERT, PREX2, KMT2D, GRIN2A, KMT2C, FAT1, PAK5 mutations are high-frequency mutations that occur in both PTPRD mutation and PTPRD wild group (Figure 4C).
In the cohort of ICB-treated non-small cell lung cancer (N = 406), the OS of the PTPRD-mutant patients (N = 49) was better than that of those without the PTPRD mutation (P = 0.011) (Figure 4D). Significant differences were found in the distribution of TMB Score (N = 350, P < 10e-10), mutation count (N = 673, P < 10e-10), mutation rate (N = 240, P = 3.78e-10), smoker (N = 296, P = 6.74e-8), and RECIST (N = 56, P = 0.002) between the PTPRD-mutation and PTPRD-wildtype groups in the ICB-treated non-small cell lung cancer cohort (Figure 4E). The ICB-treated non-small cell lung cancer patients with PTPRD mutation have higher TMB scores, more mutation counts, and an increased mutation rate. Moreover, the proportion of former smokers (10.26% VS. 9.73%), ever smokers (82.05% VS. 62.65%) and current smokers (5.13% VS. 4.67%) in ICB-treated non-small cell lung cancer patients with PTPRD mutation is higher than that of patients without PTPRD mutation (Figure 4F).
For the melanoma patients (N = 471) who received immune checkpoint inhibitors, better OS could be observed in the PTPRD-mutation group (N = 97, P = 0.005) (Figure 4G). In a subgroup of 471 melanoma patients from the ICB-treated cohort, patients with PTPRD mutation had more mutation counts, and TMB score was significantly higher in PTPRD-mutated patients compared with that in PTPRD-wildtype patients (Figure 4H).
Immune infiltration was closely associated with the occurrence and development of cancer. Therefore, the relationship between PTPRD expression and the level of immune cell infiltration in various cancers of TCGA was evaluated by investigating the coefficient of PTPRD expression and immune infiltration level using the TIMER database. The findings revealed that PTPRD expression had significant correlations with tumor purity in multiple cancers, including UVM, LUAD, and LUSC. In UVM, the PTPRD expression level was negatively correlated with neutrophil (r = -0.263, P = 0.021), and positively correlated with CD8+ T cells (r = 0.251, P = 0.028) (Figure 5A). In LUAD, PTPRD expression was positively correlated with CD4+ T cells (r = 0.256, P = 1.16e-08), macrophages (r = 0.362, P = 1.86e-16), neutrophils (r = 0.287, P = 1.30e-10), and dendritic cells (r = 0.302, P = 9.47e-12) (Figure 5B). In LUSC, PTPRD expression was positively correlated with macrophages (r = 0.174, P = 1.28e-04) (Figure 5C). Moreover, we assessed the relationship between PTPRD mutation and immune cell infiltration level in various cancers of TCGA using the TIMER database, and the results showed that the mutated PTPRD status was correlated with high infiltrating levels of CD4 + T cells (P = 0.026) in SKCM (Figure 5D). Additionally, the TISIDB database was used to further evaluate the abundance of tumor-infiltrating lymphocytes (TILs) and PTPRD mutation, and the results showed that PTPRD mutation was positively correlated with memory B cell abundance (P = 0.015), and negatively correlated with plasmacytoid dendritic cell (P = 0.009) in LUAD (Figure 5E). Therefore, the findings revealed that PTPRD was closely linked with immune infiltration.
To further investigate the potential mechanisms of PTPRD, we conducted a PPI network of PTPRD based on the top 50 PTPRD-related genes with the STRING database (Table S5). 51 nodes and 227 edges were obtained in the PPI network (avg. local clustering coefficient: 0.801, PPI enrichment p-value: < 1.0e-16), and the combined score of SLITRK1, IL1RAPL1, SLITRK2, IRS1, and PPFIA1 with PTPRD is > 0.5 in the PPI network (Figure 6A). Based on the GEPIA2 database, the expression levels of SLITRK1, IL1RAPL1, SLITRK2, and PPFIA1 were positively correlated with PTPRD (Figure 6B). The findings indicated that SLITRK1, IL1RAPL1, SLITRK2, and PPFIA1 were closely related to the modulation and function of PTPRD.
To further explore the underlying mechanism of PTPRD in tumorigenesis, we identified the targeting PTPRD-binding proteins and the PTPRD expression-correlated genes for functional enrichment analysis. We gathered a total of 50 PTPRD-binding proteins using the STRING database, which was validated by experimental evidence (Figure 6A). Moreover, we used the GEPIA2 database to integrate all TCGA tumor expression data and obtained the top 100 genes related to PTPRD expression (Table S6). As shown in Figure 7A, PTPRD were significantly positively correlated with GATS (GATS, stromal antigen 3 opposite strand) (R = 0.58, P < 0.001), NRXN1 (neurexin 1) (R = 0.58, P < 0.001), QKI (QKI, KH domain containing, RNA binding) (R = 0.59, P < 0.001), RP11 (re-mRNA processing factor 31) (R = 0.58, P < 0.001), and AS1 (prostaglandin D2 receptor (DP)) (R = 0.75, P < 0.001). The top 50 PTPRD-binding proteins were obtained from the PTPRD-target network which was constructed by the STRING database, and the top 100 genes related to PTPRD were screened from the GEPIA2 database. As shown in Figure 7B, the overlapping target related to PTPRD was SLITRK2 (SLIT and NTRK-like family, member 2) by drawing a Venn diagram. The corresponding heatmap data showed a positive correlation between PTPRD and SLITRK2 in various cancers (Figure 7C). We combined the top 50 PTPRD-binding proteins and the top 100 genes related to PTPRD to perform KEGG and GO enrichment analyses using the metascape database. The GO enrichment analysis suggested that most of these genes are related to the pathways or cellular biology of RNA metabolisms, such as neuron projection morphogenesis, synapse organization, presynapse, axon, positive regulation of nervous system development, and others (Figure 7D). The KEGG enrichment analysis indicated that cell adhesion molecules, foxo signaling pathway, AGE-RAGE signaling pathway in diabetic complications, Wnt signaling pathway, GABAergic synapse, hedgehog signaling pathway, neurotrophin signaling pathway, and adherens junction might be involved in the effect of PTPRD on tumor pathogenesis (Figure 7E).
PTPRD has recently been identified as a potential therapeutic target due to the high prevalence of PTPRD alterations across multiple cancers. The levels of PTPRD mRNA expression were dramatically decreased in liver cirrhosis and HCC cases and were typically increased in healthy liver cases [31]. PTPRD developed both deletion and mutation in several malignancies, and PTPRD deactivation was related to many genetic and epigenetic alterations [6]. However, the underlying molecular mechanisms of PTPRD are still largely unclear. Our research offers insights into the underlying role of PTPRD in tumor immunology and its function as a tumor biomarker. Previous studies have illustrated that PTPRD participated in various signaling pathways, including PTPRD/STAT3/JAK, PTPRD/Wnt/β-catenin/TCF, PTPRD-CXCL8 axis, PTPRD/PI3K/Akt/mTOR, PTPRD/PD-1/PD-L1 axis [7,32,33,34]. Consistent with these findings, our study revealed that PTPRD might modulate cancer-related signaling pathways, such as the foxo signaling pathway, Wnt signaling pathway, Hedgehog signaling pathway, and neurotrophin signaling pathway. Therefore, PTPRD may be a hopeful therapeutic target across various cancers.
PTPRD, which was involved in the development of glioblastoma multiforme as well as several cancers, could serve as a tumor suppressor. PTPRD suppression appeared in over 50% of glioblastoma multiforme tumors, and reduced expression of PTPRD demonstrated poor prognosis in patients with glioma [6]. PTPRD mutation was associated with STAT3 activation in HNSCC. However, mRNA expression levels of PTPRD were not related to STAT3 overactivation in HNSCC, suggesting that PTPRD mutation, but not hypermethylation or gene copy number alterations, might be used as a predictive biomarker of sensitivity to STAT3 inhibitors in HNSCC [35]. Furthermore, PTPRD deletion was observed to be associated with a worse prognosis in patients with gastric cancer. Silencing PTPRD remarkably facilitated the proliferation, invasion, and migration of gastric cancer cells via phosphorylating STAT3, suggesting that silencing PTPRD might be an underlying therapeutic target in gastric cancer [36]. Also, heterozygous loss of PTPRD cooperated with Cdkn2a deletion to induce tumorigenesis in glioblastoma. The expression of chemokines, such as CCL2, CCL6, CCL12, and CXCL14, and the polarization of M2 macrophages increased in PTPRD heterozygous tumor cells, indicating that heterozygous PTPRD loss triggered immune activities and affected the macrophage response [37]. STAT3 has recently appeared as an attractive therapeutic target for various cancers due to its vital role in carcinogenesis. Numerous signaling molecules were involved in STAT3 activation, including ligand binding to growth factor receptors, G-protein coupled receptors (GPCRs), cytokine receptors, toll-like receptors (TLRs), and non-receptor tyrosine kinases. Moreover, IL-6 stimulated PTPRD by hindering miR-34a to suppress the overactivation of IL-6/STAT3 signaling in breast cancer [38]. Additionally, STAT3 has also been displayed to bind to the PD-L1 promoter for transcriptional modulation of PD-L1 expression, which can facilitate tumor immune evasion [37,39]. These results showed that the function of PTPRD was varied in various types of cancer.
We observed that the proportion of PTPRD mutation was over 15% in patients with stomach adenocarcinoma, skin cutaneous melanoma, lung adenocarcinoma, head and neck squamous cell carcinoma, and uterine corpus endometrial carcinoma. Moreover, the deep deletion variations of PTPRD were widespread in head and neck squamous cell carcinoma, lung squamous cell carcinoma, bladder urothelial carcinoma, lung adenocarcinoma, and other cancers, which was consistent with the tumor suppressor role of PTPRD in multi-cancer cancers [7,36]. Notably, the relationship between PTPRD mutation and response to immunotherapy in pan-cancer was investigated, and the results showed that the OS of the PTPRD-mutant patients was better than that of those without the PTPRD mutation in the cohort of ICB-treated non-small cell lung cancer and melanoma. Previous studies have proved that TMB scores could be applied as prognostic indicators for immunotherapy [40,41]. A recent study found that PTPRD mutation is associated with TMB distribution in early NSCLC, suggesting that PTPRD mutation may be a predictor of TMB [42]. In this study, we observed that non-small cell lung cancer and melanoma patients with PTPRD mutation had higher TMB scores, consistent with patients with PTPRD mutation or higher TMB scores were more likely to benefit from immunotherapy. Studies in lung cancer patients have demonstrated a stronger response to PD-1 inhibitors in smokers than in non-smokers [43]. Consistently, PTPRD mutations accounted for a higher proportion of lung cancer smokers in our study, indicating that these patients may be more likely to benefit from immunotherapy. A further prospective study is warranted to affirm the predictive role of PTPRD in immunotherapy.
This study initially explored the role of PTPRD in different tumors. Abnormal expression of PTPRD has been observed in several cancer types, and PTPRD expression may be closely related to prognosis in breast cancer, non-small cell lung cancer, liposarcoma, and ovarian cancer. PTPRD mutations are frequently detected in lung cancer and melanoma, which may be positively associated with immune checkpoint inhibitor therapy. Additionally, PTPRD may perform a crucial function in tumorigenesis as a promising molecular target, thus strengthening the comprehension of immunopathogenesis and facilitating the likelihood of discovery and development of new targeted therapeutics.
Notably, some questions remain unanswered. PTPRD expression exhibited prognostic value in some datasets of breast, non-small cell lung, and ovarian cancer. However, we also noticed that PTPRD expression did not show prognostic value in other datasets of these cancers. This also means that the prognostic value of PTPRD expression needs to be further explored, especially in breast cancer, non-small cell lung cancer, and ovarian cancer. Although protein-protein interaction network analysis, immune infiltration analysis, and functional enrichment analysis were carried out for PTPRD, the function of PTPRD is largely unknown. This study preliminarily revealed that PTPRD mutation is closely related to immunotherapy in some types of cancer. The relationship between PTPRD expression and immunotherapy is also worthy of further exploration. Different roles of PTPRD in different cancer types may be related to tumor heterogeneity and deserve further exploration.
This work was supported by the National Natural Science Foundation of China (81660755); and the Science and Technology Project of Shenzhen of China (JCYJ20170307160524377 and JCYJ20190808162605484).
The authors declare that they have no competing interests.
[1] |
R. L. Siegel, K. D. Miller, H. E. Fuchs, A. Jemal, Cancer Statistics, 2021, CA Cancer J. Clin., 71 (2021), 7-33. https://doi.org/10.3322/caac.21654 doi: 10.3322/caac.21654
![]() |
[2] |
P. Krzyszczyk, A. Acevedo, E. J. Davidoff, L. M. Timmins, I. Marrero-Berrios, M. Patel, et al., The growing role of precision and personalized medicine for cancer treatment, Technology, 6 (2018), 79-100. https://doi.org/10.1142/S2339547818300020 doi: 10.1142/S2339547818300020
![]() |
[3] |
The Cancer Genome Atlas Research Network, J. N. Weinstein, E. A. Collisson, G. B. Mills, K. R. Shaw, B. A. Ozenberger, et al., The cancer genome atlas pan-cancer analysis project, Nat. Genet., 45 (2013), 1113-1120. https://doi.org/10.1038/ng.2764 doi: 10.1038/ng.2764
![]() |
[4] |
J. Liu, T. Lichtenberg, K. A. Hoadley, L. M. Poisson, A. J. Lazar, A. D. Cherniack, et al., An integrated TCGA pan-cancer clinical data resource to drive high-quality survival outcome analytics, Cell, 173 (2018), 400-416. https://doi.org/10.1158/1538-7445.AM2018-3287 doi: 10.1158/1538-7445.AM2018-3287
![]() |
[5] |
F. Chen, M. C. Wendl, M. A. Wyczalkowski, M. H. Bailey, Y. Li, L. Ding, Moving pan-cancer studies from basic research toward the clinic, Nat. Cancer, 2 (2021), 879-890. https://doi.org/10.1038/s43018-021-00250-4 doi: 10.1038/s43018-021-00250-4
![]() |
[6] |
S. Veeriah, C. Brennan, S. Meng, B. Singh, J. A. Fagin, D. B. Solit, et al., The tyrosine phosphatase PTPRD is a tumor suppressor that is frequently inactivated and mutated in glioblastoma and other human cancers, Proc. Natl. Acad. Sci., 106 (2009), 9435-9440. https://doi.org/10.1073/pnas.0900571106 doi: 10.1073/pnas.0900571106
![]() |
[7] |
X. Huang, F. Qin, Q. Meng, M. Dong, Protein tyrosine phosphatase receptor type D (PTPRD)-mediated signaling pathways for the potential treatment of hepatocellular carcinoma: a narrative review, Ann. Transl. Med., 8 (2020), 1192. https://doi.org/10.21037/atm-20-4733 doi: 10.21037/atm-20-4733
![]() |
[8] |
G. R. Uhl, M. J. Martinez, PTPRD: neurobiology, genetics, and initial pharmacology of a pleiotropic contributor to brain phenotypes, Ann. N. Y. Acad. Sci., 1451 (2019), 112-129. https://doi.org/10.1111/nyas.14002 doi: 10.1111/nyas.14002
![]() |
[9] |
K. Funato, Y. Yamazumi, T. Oda, T. Akiyama, Tyrosine phosphatase PTPRD suppresses colon cancer cell migration in coordination with CD44, Exp. Ther. Med., 2 (2011), 457-463. https://doi.org/10.3892/etm.2011.231 doi: 10.3892/etm.2011.231
![]() |
[10] |
W. J. Bae, J. M. Ahn, H. E. Byeon, S. Kim, D. Lee, PTPRD-inactivation-induced CXCL8 promotes angiogenesis and metastasis in gastric cancer and is inhibited by metformin, J. Exp. Clin. Cancer Res., 38 (2019), 484. https://doi.org/10.1186/s13046-019-1469-4 doi: 10.1186/s13046-019-1469-4
![]() |
[11] |
K. Onoi, Y. Chihara, J. Uchino, T. Shimamoto, Y. Morimoto, M. Iwasaku, et al., Immune checkpoint inhibitors for lung cancer treatment: A review, J. Clin. Med., 9 (2020). https://doi.org/10.3390/jcm9051362 doi: 10.3390/jcm9051362
![]() |
[12] |
A. M. Goodman, E. S. Sokol, G. M. Frampton, S. M. Lippman, R. Kurzrock, Microsatellite-stable tumors with high mutational burden benefit from immunotherapy, Cancer Immunol. Res., 7 (2019), 1570-1573. https://doi.org/10.1158/2326-6066.CIR-19-0149 doi: 10.1158/2326-6066.CIR-19-0149
![]() |
[13] |
D. Sha, Z. Jin, J. Budczies, K. Kluck, A. Stenzinger, F. A. Sinicrope, Tumor mutational burden as a predictive biomarker in solid tumors, Cancer Discov., 10 (2020), 1808-1825. https://doi.org/10.1158/2159-8290.CD-20-0522 doi: 10.1158/2159-8290.CD-20-0522
![]() |
[14] |
Z. Lu, H. Chen, X. Jiao, W. Zhou, W. Han, S. Li, et al., Prediction of immune checkpoint inhibition with immune oncology-related gene expression in gastrointestinal cancer using a machine learning classifier, J. Immunother. Cancer, 8 (2020). https://doi.org/10.1136/jitc-2020-000631 doi: 10.1136/jitc-2020-000631
![]() |
[15] |
N. Ready, M. D. Hellmann, M. M. Awad, G. A. Otterson, M. Gutierrez, J. F. Gainor, et al., First-line nivolumab plus ipilimumab in advanced non-small-cell lung cancer (CheckMate 568): outcomes by programmed death ligand 1 and tumor mutational burden as biomarkers, J. Clin. Oncol., 37 (2019), 992-1000. https://doi.org/10.1200/JCO.18.01042 doi: 10.1200/JCO.18.01042
![]() |
[16] |
D. A. Solomon, J. S. Kim, J. C. Cronin, Z. Sibenaller, T. Ryken, S. A. Rosenberg, et al., Mutational inactivation of PTPRD in glioblastoma multiforme and malignant melanoma, Cancer Res., 68 (2008), 10300-10306. https://doi.org/10.1158/0008-5472.CAN-08-3272 doi: 10.1158/0008-5472.CAN-08-3272
![]() |
[17] |
T. Li, J. Fu, Z. Zeng, D. Cohen, J. Li, Q. Chen, et al., TIMER2.0 for analysis of tumor-infiltrating immune cells, Nucleic Acids Res., 48 (2020), W509-W514. https://doi.org/10.1093/nar/gkaa407 doi: 10.1093/nar/gkaa407
![]() |
[18] |
D. S. Chandrashekar, B. Bashel, S. A. H. Balasubramanya, C. J. Creighton, I. Ponce-Rodriguez, B. Chakravarthi, et al., UALCAN: a portal for facilitating tumor subgroup gene expression and survival analyses, Neoplasia, 19 (2017), 649-658. https://doi.org/10.1016/j.neo.2017.05.002 doi: 10.1016/j.neo.2017.05.002
![]() |
[19] |
H. Mizuno, K. Kitada, K. Nakai, A. Sarai, PrognoScan: a new database for meta-analysis of the prognostic value of genes, BMC Med. Genomics, 2 (2009), 18. https://doi.org/10.1186/1755-8794-2-18 doi: 10.1186/1755-8794-2-18
![]() |
[20] |
J. Gao, B. A. Aksoy, U. Dogrusoz, G. Dresdner, B. Gross, S. O. Sumer, et al., Integrative analysis of complex cancer genomics and clinical profiles using the cBioPortal, Sci. Signal, 6 (2013). https://doi.org/10.1126/scisignal.2004088 doi: 10.1126/scisignal.2004088
![]() |
[21] |
R. M. Samstein, C. H. Lee, A. N. Shoushtari, M. D. Hellmann, R. Shen, Y. Y. Janjigian, et al., Tumor mutational load predicts survival after immunotherapy across multiple cancer types, Nat. Genet., 51 (2019), 202-206. https://doi.org/10.1038/s41588-018-0312-8 doi: 10.1038/s41588-018-0312-8
![]() |
[22] |
D. Miao, C. A. Margolis, N. I. Vokes, D. Liu, A. Taylor-Weiner, S. M. Wankowicz, et al., Genomic correlates of response to immune checkpoint blockade in microsatellite-stable solid tumors, Nat. Genet., 50 (2018), 1271-1281. https://doi.org/10.1038/s41588-018-0200-2 doi: 10.1038/s41588-018-0200-2
![]() |
[23] |
H. Rizvi, F. Sanchez-Vega, K. La, W. Chatila, P. Jonsson, D. Halpenny, et al., Molecular determinants of response to anti-programmed cell death (PD)-1 and anti-programmed death-ligand 1 (PD-L1) blockade in patients with non-small-cell lung cancer profiled with targeted next-generation sequencing, J. Clin. Oncol., 36 (2018), 633-641. https://doi.org/10.1200/JCO.2017.75.3384 doi: 10.1200/JCO.2017.75.3384
![]() |
[24] |
N. A. Rizvi, M. D. Hellmann, A. Snyder, P. Kvistborg, V. Makarov, J. J. Havel, et al., Mutational landscape determines sensitivity to PD-1 blockade in non-small cell lung cancer, Science, 348 (2015), 124-128. https://doi.org/10.1126/science.aaa1348 doi: 10.1126/science.aaa1348
![]() |
[25] |
T. Li, J. Fan, B. Wang, N. Traugh, Q. Chen, J. S. Liu, et al., TIMER: a web server for comprehensive analysis of tumor-infiltrating immune cells, Cancer Res., 77 (2017), e108-e110. https://doi.org/10.1158/0008-5472.CAN-17-0307 doi: 10.1158/0008-5472.CAN-17-0307
![]() |
[26] |
B. Li, E. Severson, J. C. Pignon, H. Zhao, T. Li, J. Novak, et al., Comprehensive analyses of tumor immunity: implications for cancer immunotherapy, Genome Biol., 17 (2016), 174. https://doi.org/10.1186/s13059-016-1028-7 doi: 10.1186/s13059-016-1028-7
![]() |
[27] |
B. Ru, C. N. Wong, Y. Tong, J. Y. Zhong, S. S. W. Zhong, W. C. Wu, et al., TISIDB: an integrated repository portal for tumor-immune system interactions, Bioinformatics, 35 (2019), 4200-4202. https://doi.org/10.1093/bioinformatics/btz210 doi: 10.1093/bioinformatics/btz210
![]() |
[28] |
Z. Tang, C. Li, B. Kang, G. Gao, C. Li, Z. Zhang, GEPIA: a web server for cancer and normal gene expression profiling and interactive analyses, Nucleic Acids Res., 45 (2017), W98-W102. https://doi.org/10.1093/nar/gkx247 doi: 10.1093/nar/gkx247
![]() |
[29] |
D. Szklarczyk, A. L. Gable, D. Lyon, A. Junge, S. Wyder, J. Huerta-Cepas, et al., STRING v11: protein-protein association networks with increased coverage, supporting functional discovery in genome-wide experimental datasets, Nucleic Acids Res., 47 (2019), D607-D613. https://doi.org/10.1093/nar/gky1131 doi: 10.1093/nar/gky1131
![]() |
[30] |
Y. Zhou, B. Zhou, L. Pache, M. Chang, A. H. Khodabakhshi, O. Tanaseichuk, et al., Metascape provides a biologist-oriented resource for the analysis of systems-level datasets, Nat. Commun., 10 (2019), 1523. https://doi.org/10.1038/s41467-019-09234-6 doi: 10.1038/s41467-019-09234-6
![]() |
[31] |
T. Acun, K. Demir, E. Oztas, D. Arango, M. C. Yakicier, PTPRD is homozygously deleted and epigenetically downregulated in human hepatocellular carcinomas, OMICS, 19 (2015), 220-229. https://doi.org/10.1089/omi.2015.0010 doi: 10.1089/omi.2015.0010
![]() |
[32] |
H. Tomita, F. Cornejo, B. Aranda-Pino, C. L. Woodard, C. C. Rioseco, B. G. Neel, et al., The protein tyrosine phosphatase receptor delta regulates developmental neurogenesis, Cell Rep., 30 (2020), 215-228. https://doi.org/10.1016/j.celrep.2019.11.033 doi: 10.1016/j.celrep.2019.11.033
![]() |
[33] |
H. C. Hsu, N. Lapke, S. J. Chen, Y. J. Lu, R. S. Jhou, C. Y. Yeh, et al., PTPRT and PTPRD deleterious mutations and deletion predict bevacizumab resistance in metastatic colorectal cancer patients, Cancers (Basel), 10 (2018). https://doi.org/10.3390/cancers10090314 doi: 10.3390/cancers10090314
![]() |
[34] |
G. R. Uhl, M. J. Martinez, P. Paik, A. Sulima, G. H. Bi, M. R. Iyer, et al., Cocaine reward is reduced by decreased expression of receptor-type protein tyrosine phosphatase D (PTPRD) and by a novel PTPRD antagonist, Proc. Natl. Acad. Sci., 115 (2018), 11597-11602. https://doi.org/10.1073/pnas.1720446115 doi: 10.1073/pnas.1720446115
![]() |
[35] |
N. D. Peyser, Y. Du, H. Li, V. Lui, X. Xiao, T. A. Chan, et al., Loss-of-function PTPRD mutations lead to increased STAT3 activation and sensitivity to STAT3 inhibition in head and neck cancer, PLoS One, 10 (2015), e0135750. https://doi.org/10.1371/journal.pone.0135750 doi: 10.1371/journal.pone.0135750
![]() |
[36] |
L. Wu, L. Gao, D. Kong, H. Xue, Loss of tyrosine phosphatase delta promotes gastric cancer progression via signal transducer and activator of transcription 3 pathways, Dig. Dis. Sci., 64 (2019), 3164-3172. https://doi.org/10.1007/s10620-019-05637-z doi: 10.1007/s10620-019-05637-z
![]() |
[37] |
B. Ortiz, A. W. Fabius, W. H. Wu, A. Pedraza, C. W. Brennan, N. Schultz, et al., Loss of the tyrosine phosphatase PTPRD leads to aberrant STAT3 activation and promotes gliomagenesis, Proc. Natl. Acad. Sci., 111 (2014), 8149-8154. https://doi.org/10.1073/pnas.1401952111 doi: 10.1073/pnas.1401952111
![]() |
[38] |
F. Zhang, B. Wang, T. Qin, L. Wang, Q. Zhang, Y. Lu, et al., IL-6 induces tumor suppressor protein tyrosine phosphatase receptor type D by inhibiting miR-34a to prevent IL-6 signaling overactivation, Mol. Cell Biochem., 473 (2020). https://doi.org/10.1007/s11010-020-03803-w doi: 10.1007/s11010-020-03803-w
![]() |
[39] |
L. Ding, X. Chen, X. Xu, Y. Qian, G. Liang, F. Yao, et al., PARP1 suppresses the transcription of PD-L1 by poly(ADP-Ribosyl) ating STAT3, Cancer Immunol. Res., 7 (2019), 136-149. https://doi.org/10.1158/2326-6066.CIR-18-0071 doi: 10.1158/2326-6066.CIR-18-0071
![]() |
[40] |
J. Mo, X. Hu, L. Gu, B. Chen, P. A. Khadaroo, Z. Shen, et al., Smokers or non-smokers: who benefits more from immune checkpoint inhibitors in treatment of malignancies? An up-to-date meta-analysis, World J. Surg. Oncol., 18 (2020), 15. https://doi.org/10.1186/s12957-020-1792-4 doi: 10.1186/s12957-020-1792-4
![]() |
[41] |
G. T. Gibney, L. M. Weiner, M. B. Atkins, Predictive biomarkers for checkpoint inhibitor-based immunotherapy, Lancet Oncol., 17 (2016), e542-e551. https://doi.org/10.1016/S1470-2045(16)30406-5 doi: 10.1016/S1470-2045(16)30406-5
![]() |
[42] |
Y. Qiu, L. Liu, H. Yang, H. Chen, Q. Deng, D. Xiao, et al., Integrating histologic and genomic characteristics to predict tumor mutation burden of early-stage non-small-cell lung cancer, Front. Oncol., 10 (2020), 608989. https://doi.org/10.3389/fonc.2020.608989 doi: 10.3389/fonc.2020.608989
![]() |
[43] |
J. Norum, C. Nieder, Tobacco smoking and cessation and PD-L1 inhibitors in non-small cell lung cancer (NSCLC): a review of the literature, ESMO Open, 3 (2018), e000406. https://doi.org/10.1136/esmoopen-2018-000406 doi: 10.1136/esmoopen-2018-000406
![]() |
![]() |
![]() |
1. | Sophie Orton, Rebecca Karkia, Denis Mustafov, Seley Gharanei, Maria Braoudaki, Alice Filipe, Suzana Panfilov, Sayeh Saravi, Nabeel Khan, Ioannis Kyrou, Emmanouil Karteris, Jayanta Chatterjee, Harpal S. Randeva, In Silico and In Vitro Mapping of Receptor-Type Protein Tyrosine Phosphatase Receptor Type D in Health and Disease: Implications for Asprosin Signalling in Endometrial Cancer and Neuroblastoma, 2024, 16, 2072-6694, 582, 10.3390/cancers16030582 | |
2. | Yu Matsui, Ayako Imai, Hironori Izumi, Misato Yasumura, Teruhiko Makino, Tadamichi Shimizu, Makoto Sato, Hisashi Mori, Tomoyuki Yoshida, Cancer‐associated point mutations within the extracellular domain of PTPRD affect protein stability and HSPG interaction, 2024, 38, 0892-6638, 10.1096/fj.202302279RR | |
3. | Shuxian Ma, Xuze Li, Wenhao Wu, Pei Zhang, Yanjie Yang, Lining Huang, Qian Wan, Screening and identification of hub genes of scar physique via weighted gene co-expression network analysis, 2023, 102, 0025-7974, e36077, 10.1097/MD.0000000000036077 | |
4. | Min Yuan, Wei Feng, Haolun Ding, Yaning Yang, Xu Steven Xu, Discovery of mutations predictive of survival benefit from immunotherapy in first-line NSCLC: A retrospective machine learning study of IMpower150 liquid biopsy data, 2025, 189, 00104825, 109964, 10.1016/j.compbiomed.2025.109964 |