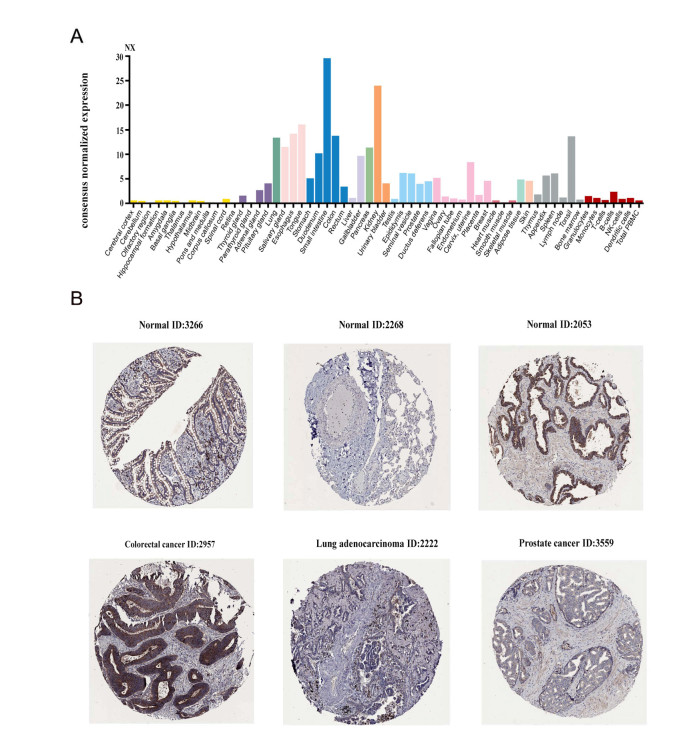
Metastasis-Associated in Colon Cancer 1(MACC1) is a validated biomarker for metastasis and is linked to survival. Although extensive experimental evidence indicates an association between MACC1 and diverse cancers, no pan-cancer analyses have yet been performed for this marker, and the role of MACC1 in immunology remains unknown.
In our study, we performed the analysis of MACC1 expression and its influence on prognosis using multiple databases, including TIMER2, GEPIA2, Kaplan-Meier plotter. MACC1 promoter methylation levels were evaluated using the UALCAN database. Based on the TCGA database, we explored the relationship between MACC1 and tumor mutational burden (TMB), microsatellite instability (MSI), immune checkpoints using the R programming language. We evaluated the association between MACC1 and immune infiltration via TIMER and UALCAN.
Our results revealed that abnormal DNA methylation may be an important cause for the different expression of MACC1 across cancer types. Meanwhile, we explored the potential oncogenic roles of MACC1 and found significant prognostic value. MACC1 may be related to T-cell function and the polarization of tumor-associated macrophages, especially in STAD and LGG. Its expression was associated with immune infiltration and was found to be closely related to immune checkpoint-associated genes, especially CD274 and SIGLEC15, indicating that MACC1 may be a potential immune therapeutic target for several malignancies. Our paper reveals for the first time the relationship between MACC1 and cancer immunology.
MACC1 might act as a predictor for the immune response in cancer patients, and could also represent a new potential immunotherapeutic target.
Citation: Ye Hu, Meiling Wang, Kainan Wang, Jiyue Gao, Jiaci Tong, Zuowei Zhao, Man Li. A potential role for metastasis-associated in colon cancer 1 (MACC1) as a pan-cancer prognostic and immunological biomarker[J]. Mathematical Biosciences and Engineering, 2021, 18(6): 8331-8353. doi: 10.3934/mbe.2021413
[1] | Sidan Long, Shuangshuang Ji, Kunmin Xiao, Peng Xue, Shijie Zhu . Prognostic and immunological value of LTB4R in pan-cancer. Mathematical Biosciences and Engineering, 2021, 18(6): 9336-9356. doi: 10.3934/mbe.2021459 |
[2] | Tao Huang . TRPV1 is a potential biomarker for the prediction and treatment of multiple cancers based on a pan-cancer analysis. Mathematical Biosciences and Engineering, 2022, 19(8): 8361-8379. doi: 10.3934/mbe.2022389 |
[3] | Chunpei Ou, Qin Peng, Changchun Zeng . An integrative prognostic and immune analysis of PTPRD in cancer. Mathematical Biosciences and Engineering, 2022, 19(6): 5361-5379. doi: 10.3934/mbe.2022251 |
[4] |
Shuoer Wang, Yidi Sun, Songjiao Zhao, Feng Wei, Gong Yang .
Breast conserving surgery (BCS) with adjuvant radiation therapy showed improved prognosis compared with mastectomy for early staged triple negative breast cancer patients . Mathematical Biosciences and Engineering, 2020, 17(1): 92-104. doi: 10.3934/mbe.2020005 |
[5] | Ying Liu, Xiao Ma, Linyuan Feng, Zhenhua Lin, Xianchun Zhou . An integrative pan-cancer analysis reveals the carcinogenic effects of NCAPH in human cancer. Mathematical Biosciences and Engineering, 2023, 20(1): 76-92. doi: 10.3934/mbe.2023005 |
[6] | Tongmeng Jiang, Pan Jin, Guoxiu Huang, Shi-Cheng Li . The function of guanylate binding protein 3 (GBP3) in human cancers by pan-cancer bioinformatics. Mathematical Biosciences and Engineering, 2023, 20(5): 9511-9529. doi: 10.3934/mbe.2023418 |
[7] | Jie Wang, Md. Nazim Uddin, Rehana Akter, Yun Wu . Contribution of endothelial cell-derived transcriptomes to the colon cancer based on bioinformatics analysis. Mathematical Biosciences and Engineering, 2021, 18(6): 7280-7300. doi: 10.3934/mbe.2021360 |
[8] | Shuyi Cen, Kaiyou Fu, Yue Shi, Hanliang Jiang, Jiawei Shou, Liangkun You, Weidong Han, Hongming Pan, Zhen Liu . A microRNA disease signature associated with lymph node metastasis of lung adenocarcinoma. Mathematical Biosciences and Engineering, 2020, 17(3): 2557-2568. doi: 10.3934/mbe.2020140 |
[9] | Yuan Yang, Lingshan Zhou, Xi Gou, Guozhi Wu, Ya Zheng, Min Liu, Zhaofeng Chen, Yuping Wang, Rui Ji, Qinghong Guo, Yongning Zhou . Comprehensive analysis to identify DNA damage response-related lncRNA pairs as a prognostic and therapeutic biomarker in gastric cancer. Mathematical Biosciences and Engineering, 2022, 19(1): 595-611. doi: 10.3934/mbe.2022026 |
[10] | Zekun Xin, Yang Li, Lingyin Meng, Lijun Dong, Jing Ren, Jianlong Men . Elevated expression of the MYB proto-oncogene like 2 (MYBL2)-encoding gene as a prognostic and predictive biomarker in human cancers. Mathematical Biosciences and Engineering, 2022, 19(2): 1825-1842. doi: 10.3934/mbe.2022085 |
Metastasis-Associated in Colon Cancer 1(MACC1) is a validated biomarker for metastasis and is linked to survival. Although extensive experimental evidence indicates an association between MACC1 and diverse cancers, no pan-cancer analyses have yet been performed for this marker, and the role of MACC1 in immunology remains unknown.
In our study, we performed the analysis of MACC1 expression and its influence on prognosis using multiple databases, including TIMER2, GEPIA2, Kaplan-Meier plotter. MACC1 promoter methylation levels were evaluated using the UALCAN database. Based on the TCGA database, we explored the relationship between MACC1 and tumor mutational burden (TMB), microsatellite instability (MSI), immune checkpoints using the R programming language. We evaluated the association between MACC1 and immune infiltration via TIMER and UALCAN.
Our results revealed that abnormal DNA methylation may be an important cause for the different expression of MACC1 across cancer types. Meanwhile, we explored the potential oncogenic roles of MACC1 and found significant prognostic value. MACC1 may be related to T-cell function and the polarization of tumor-associated macrophages, especially in STAD and LGG. Its expression was associated with immune infiltration and was found to be closely related to immune checkpoint-associated genes, especially CD274 and SIGLEC15, indicating that MACC1 may be a potential immune therapeutic target for several malignancies. Our paper reveals for the first time the relationship between MACC1 and cancer immunology.
MACC1 might act as a predictor for the immune response in cancer patients, and could also represent a new potential immunotherapeutic target.
Cancer is a major cause of death globally; incidence and mortality for this group of diseases are expected to increase in the coming years. A single cell with malignant characteristics acquired through epigenetic alterations can lead to carcinogenesis, and accumulated genetic mutations contribute to cancer progression [1,2]. Mutations at certain genomic locations result in the loss of gene function [3,4]. The significance of individual alterations in gene expression across diverse tumor types is still unclear. Moreover, the survival of patients with primary tumors is directly related to the occurrence of metastasis. Early and accurate identification of those at high risk of metastasis is vital for effective disease management, however, there are currently no clear molecular markers for this group. The identification of novel, reliable biomarkers with significant predictive value is therefore a priority.
The first identification of Metastasis Associated with Colon Cancer 1 (MACC1) has drawn great attention through analysis of genome-wide expression in all colorectal cancer patients [5]. Accumulated evidence has proved that MACC1 has a comprehensive function across many different solid cancer types and across many physiological and pathological processes including cell proliferation, migration, tumorigenesis and metastasis.
In recent years, the link between MACC1 expression and disease prognosis has been studied in diverse tumor types beyond colorectal cancer [5], such as hepatobiliary cancer [6], pancreatic cancer [7], lung cancer [8], ovarian cancer [9], breast cancer [10], glioblastoma [11]. In a pioneering study, we found that people with cancer who had a high MACC1 expression also had a survival rate of 15%, much lower than the 80% survival rate of those who had low MACC1 expression [5]. The laboratory evidence suggests that MACC1 overexpression accelerates the procession of benign adenoma into malignant tumors and increase hepatic metastases in xenograft models [12,13]. Previous meta-analysis results also confirmed that in multiple solid tumors, MACC1 expression was strongly related to prognosis, especially in digestive system neoplasms [14,15,16]. These data provide favorable evidence for MACC1 as a useful prognostic and predictive marker.
High-throughput molecular data for over 10,000 tumors is publicly available in online database, and many statistical methods for analysis have been established and validated in previous studies. Bioinformatics analyses are a cost-effective and relatively simple way to investigate gene function and provide new insight into potential biological mechanisms underlying tumor development and progression. In this study, we performed a comprehensive bioinformatic analysis of the role of MACC1 expression across 33 cancer types in the cancer genome atlas (TCGA) database. This is the first pan-cancer analysis to access multiple different aspects of gene expression and function, including DNA methylation, immune infiltration, genetic alteration, and relevant pathways. The insights gained from this study pave the way for subsequent, more specific investigations, and provide a novel overview into the underlying mechanisms of MACC1 involvement in tumor initiation and progression, and immune infiltrations.
The mRNA expression of MACC1 in normal tissue and protein expression in some major tissues (displayed by immunohistochemistry) were obtained from the Human Protein Atlas (HPA) databases (http://www.proteinatlas.org/) [17]. The antibodies used are HPA020103.
To explore the different MACC1 mRNA expressions between tumors and normal tissues, we used the "Gene-DE" module of TIMER2 (tumor immune estimation resource, Version 2) web (http://timer.cistrome.org/). TIMER2 is a web resource for analyzing immune infiltrates, and "Gene-DE" is a module for exploring the expression of differential genes in tumors compared to adjacent normal tissues [18].
We used the UALCAN database (http://ualcan.path.uab.edu/index.html database) to explore the MACC1 promoter methylation profile [19]. The UALCAN portal is a user-friendly web resource for analyzing cancer Omics data. P < 0.05 is considered a significant threshold.
The UALCAN database was also used to assess the relationship between MACC1 mRNA transcription level and pathological cancer stage, which was divided into 4 Stages (Stage 1, 2, 3, 4) [19]. P < 0.05 is considered a significant threshold.
The prognostic value of MACC1 mRNA expression in 33 cancers was visualized with two tools separately:GEPIA2(http://gepia2.cancer-pku.cn/), Kaplan-Meierplotter (http://kmplot.com/) databases [20]. This analysis was visualized using Kaplan-Meier cubes. GEPIA2 includes RNA sequencing expression data from 9736 tumors and 8587 normal samples from the TCGA and GTEx projects. The cutoff-high (50%) and cutoff-low (50%) values were used as expression thresholds to separate the high expression and low expression queues. We also used the Kaplan-Meier plotter database to evaluate these indicators, including overall survival (OS) and disease-free survival (DFS) in certain tumors. P < 0.05 was considered statistically significant.
The mRNA expression data of MACC1 mRNA expression data across 33 tumor types and normal tissues were downloaded from this TCGA database (https://portal.gdc.cancer.gov/). We computed TMB and MSI scores and analyzed the relationship between them and MACC1 using R programming language. TCGA is a comprehensive and web-based database that, as of 2018, has generated massive NGS data for more than 11,000 tumors across 33 cancer types. TMB can measure the number of mutations of a tumor genome and can predict the response to immunotherapy. MSI is a high mutation phenotype caused by frequent polymorphisms in short repeating DNA sequences and single nucleotide substitutions due to DNA mismatch repair (MMR) defects. MSI occurs frequently across tumors and can act as a predictor of sensitivity for immune-therapy [21,22]. In this analysis, the version of R language software was R-4.0.3. P-values less than 0.05 were considered statistically significant.
We extracted the expression values of 8 immune checkpoint-related genes, including SIGLEC15, IDO1, CD274, HAVCR2, PDCD1, CTLA4, LAG3 and PDCD1LG2, and observed the relationship between MACC1 mRNA expression and these genes. The results were presented as a heatmap. R-4.0.3 software was used for statistical analysis. P < 0.05 is taken as the significance threshold.
TIMER is a comprehensive online resource for analyzing the immune infiltrates across all TCGA cancers (https://cistrome.shinyapps.io/timer/) [23]. It is made up of seven modules, including gene, SCAN, mutation, survival, correlation, differential gene expression. First, we input MACC1 in the "Gene" module of TIMER and evaluated the correlation between infiltration levels of 6 immune cells and MACC1 expression, which involved B cells, CD4 + T cells, CD8 + T cells, Neutrophils, Macrophages, and Dendritic cells. We showed the expression level of MACC1 on X-axis and immune cell infiltration level on the Y-axis to plot the scatterplots. In the TIMER database, the expression level of MACC1 was adjusted to log2(TPM).
Secondly, the immunedeconv, an R package integrating six types of algorithms, was used to estimate the relationship of different infiltrating immune cell types and the MACC1 expression level of each tumor sample by R package. The results are presented as a heat map. P < 0.05 is considered statistically significant. The version of R software using in this article is R-4.0.3.
The relationship of MACC1 mRNA expression with immune cell-related markers of B-cells, CD8 + T cells, neutrophils, macrophages, dendritic cells NK cells, Th1 cells, Treg cells, and monocytes in tumors was estimated using the "correlation" module of the TIMER algorithm. Then we verified the correlation of MACC1 with immune cell markers in LGG and STAD and the P-values and partial correlation (Cor) values were obtained after tumor purity and the patient's age-adjusted Spearman's rank correlation test.
The cBio Cancer Genomics Portal (http://cbioportal.org) was used to analyze the frequency alteration of MACC1, including mutation, amplification, deep deletion, and multiple alterations. The cBioPortal database is an online resource to explore the multidimensional cancer genomics data [24].
We searched MACC1 in the SCAN module of TIMER, which includes deep deletions, arm-level deletions, normal alterations, arm-level gains, and high amplification [23]. In this module, we confirmed the association of the mRNA expression level of MACC1 and immune infiltration level with different somatic copy number alterations. A bilateral Wilcoxon rank-sum test was used to compare the infiltration levels of each SCNA category with normal. Box plots are used to display the distributions of each immune subpopulation of immune cells at different copy numbers. The significance threshold is set as P-value < 0.05.
MACC1 is located on the P-arm complementary chain of human chromosome 7 (7p21.1) (Figure S1A). The ZU5-DD architecture was observed in MACC1 and conserved among different species (e.g., Homo sapiens, Rattus norvegicus, etc.) The presence of carbon terminal death domains (DD) in MACC1, indicates that this gene is involved in the regulation of cell apoptosis (Figure S1B). We also use the phylogenetic tree date to demonstrate the evolutionary relationship of MACC1 among species (Figure S2).
First, we explored the mRNA expression profile of MACC1 in normal tissues. As shown in Figure 1A, based on a combination of HPA (human protein map), GTEx, and FANTOM5 (functional annotation of mammalian genome 5) data sets, MACC1 is highly expressed in the digestive system (such as the esophagus, tongue, colon), especially in the small intestine, relatively low expression in most other organs. The urinary system and reproductive system, including the kidney and uterus, also showed relatively high expression levels.
We accessed mRNA level as a proxy for MACC1 expression in various cancer types using data from TCGA database. MACC1 expression was observed in all tumor types, but the extent of expression was found to vary (Figure 2A). MACC1 expression in the tumor tissues of CHOL (cholangiocarcinoma), COAD (Colon adenocarcinoma), GBM (glioblastoma multiforme), KIRP (Kidney renal papillary cell carcinoma), LUAD (lung adenocarcinoma) (P < 0.001), ESCA (esophageal carcinoma) (P < 0.01), CESC (cervical and endocervical cancer), BLCA (bladder urothelial carcinoma) (P < 0.05) was much higher than the normal tissues. Additionally, compared to the adjacent normal tissues, MACC1 expression was significantly lower in HNSC (Head and neck squamous cell carcinoma), KICH (Kidney chromophobe), KIRC (Kidney renal clear cell carcinoma), LIHC (Liver hepatocellular carcinoma), LUSC (Lung squamous cell carcinoma), PARD (Prostate adenocarcinoma (P < 0.001), PCPG (Prostate adenocarcinoma) (P < 0.01). Furthermore, we selected some of the classic immunohistochemical images from HPA databases. These images demonstrated ubiquitous cytoplasmic staining for MACC1 protein, with low levels of staining in normal tissue and high levels of staining in cancer tissue, including in colorectal cancer and lung adenocarcinoma. Conversely, in prostate cancer, levels of MACC1 staining were lower than in corresponding normal prostate tissue (Figure 1B).
To better understand the relevance and underlying mechanisms of MACC1, we accessed the correlation between the expression level of MACC1 and methylation levels of MACC1 promoter in diverse cancers using ALCAN database (Figure 2B). Combined with the TCGA database, we selected several cancers which showed higher MACC1 expression compared to normal tissue, including BRCA, BLCA, LUAD, COAD, CHOL, CESE, LUSC, READ, TGCT, THCA, UCEC. And MACC1 promoter methylation levels were significantly lower in these cancer types than in corresponding normal tissue.
We analyzed the associated gene-drug interaction network using the comparative toxigenomics database (CTD) and visualized the results using Cytoscape. As shown in Figure S3, various drugs are predicted to affect the expression of MACC1. Interestingly, we found that histone deacetylase (HDAC) inhibitors, including Entinostat, Belinostat, and Panobinostat, may increase expression of MACC1. This finding provides novel avenues for therapeutic research.
Further correlation analyses of MACC1 mRNA expression level with pathological stage were performed for each tumor separately using the UALCAN database. The results showed that MACC1 expression was significantly different between pathological stages of 15 tumors compared to the normal tissues (Figure 3, Figure S4). First, we found that different pathological stages of 5 tumors showed lower MACC1 expression compared with normal tissues, including KICH, KIRC, KIRP, HNSC, LUSC. Stage IV samples of KICH, KIRC, KIRP were associated with significantly lower MACC1 expression than earlier stage, indicating that decreased MACC1 expression may indicate the disease has progressed in these patients. In contrast, in some tumors, its expression was found to be higher in patients with advanced cancer than in patients with earlier stage such as in CESC, ESCA, COAD, UCEC, THCA (Figure 3). In our study, MACC1 expression was found to be slightly increased in both Stages 3 and 4 versus Stage 2 (P < 0.001; P < 0.05). The result demonstrated that MACC1 expressed at higher levels in colon cancer of patients with metastasized compared to the tumor patients in the early stage.
The analysis of relationship between MACC1 expression and pathological stages was shown in 8 cancer types using UALCAN database, cervical and endocervical cancer (CESC), colon adenocarcinoma (COAD), thyroid carcinoma (THCA), uterine corpus endometrial carcinoma (UCEC), esophageal carcinoma (ESCA), kidney chromophobe (KICH), kidney renal clear cell carcinoma (KIRC), kidney renal papillary cell carcinoma (KIRP). The first layer asterisk above the error line indicates the comparison with normal group, and the layers layer asterisk above the remaining error line represents the comparison between the corresponding groups covered by the line. *P < 0.05, **P < 0.01, ***P < 0.001.
We analyzed the relationship between MACC1 expression status and the prognosis of patients across cancers. As shown in Figure 4A, stronger expression of MACC1 was related to poorer prognosis of overall survival (OS) in three cancer types: COAD (log-rank P = 0.0025), LGG (log-rank P = 6.9e-11), UVM (log-rank P = 0.019). In terms of DFS, the results revealed a correlation between high MACC1 expression and poor prognosis in COAD (log-rank P = 0.019), LGG (log-rank P = 2.6e-05), PAAD (log-rank P = 0.038). Notably, MACC1 was associated with greater OS in KIRC (log-rank P = 4.1e-07), KIRP (log-rank P = 0.008), PCPG (log-rank P = 0.018), SKCM (log-rank P = 0.0049) and greater DFS in KICH (log-rank P = 0.046), KIRC (log-rank P = 0.00023), KIRP (log-rank P = 0.0031).
Moreover, we investigated the correlation between MACC1 expression and prognosis across different cancer types in the Kaplan-Meier plotter database based on Affymetrix microarrays. We discovered that high MACC1 expression may be a predictor of poor prognosis of DFS (P = 5.8e-05) and OS (P = 0.0052) in ovarian cancer (Figure 4B). On the contrary, poorer prognosis in lung cancer and gastric cancer was significantly related to lower expression of MACC1 (OS: P = 2.9e-07; P = 0.0029, respectively). However, MACC1 expression had little impact on breast cancer according to the database.
Table 1 revealed the correlation of MACC1 and TMB, MSI, which can predict the immunotherapy efficacy. As shown in Figure 5A, we found TMB was negatively related to MACC1 expression in BRCA, KICH, LIHC, LUAD, PRAD, UCS, UCEC, and COAD (P < 0.0.05), but positively in KIRP, ESCA, HNSC, LGG, and PAAD (P < 0.05). Additionally, MSI was also found to be negatively associated with MACC1 expression levels in UCEC (P < 0.001) and UCS (P < 0.05), but positively associated with expression of this gene in OV, READ, STAD, TGCT (P < 0.01) (Figure 5B)
Cancer | TMB | MSI | ||
Correlation | P-value | Correlation | P-value | |
ACC | -0.142 | 0.21873 | 0.071 | 0.53964 |
BLCA | 0.027 | 0.59282 | -0.003 | 0.94940 |
BRCA | -0.073 | 0.02169 | -0.009 | 0.76597 |
CESC | 0.046 | 0.57506 | -0.026 | 0.65448 |
CHOL | -0.199 | 0.24307 | 0.242 | 0.15427 |
COAD | -0.293 | 2.48E-08 | 0.077 | 0.11088 |
DLBC | -0.295 | 0.07668 | -0.28 | 0.05424 |
ESCA | 0.367 | 3.82E-06 | 0.139 | 0.07896 |
GBM | 0.058 | 0.48026 | 0.037 | 0.65588 |
HNSC | 0.153 | 0.00070 | -0.015 | 0.73890 |
KICH | -0.251 | 0.04581 | 0.14 | 0.26538 |
KIRC | -0.033 | 0.53559 | 0.079 | 0.14706 |
KIRP | 0.128 | 0.03413 | 0.021 | 0.72721 |
LAML | -0.161 | 0.09980 | -0.051 | 0.59316 |
LGG | 0.343 | 9.75E-15 | -0.072 | 0.11118 |
LIHC | -0.141 | 0.01563 | 0.001 | 0.97814 |
LUAD | -0.173 | 9.99E-05 | 0.03 | 0.49973 |
LUSC | 0.007 | 0.88178 | -0.039 | 0.38478 |
MESO | -0.146 | 0.19437 | 0.01 | 0.93062 |
OV | -0.1 | 0.11796 | 0.142 | 0.01827 |
PAAD | 0.348 | 7.28E-06 | -0.046 | 0.54665 |
PCPG | 0.043 | 0.57813 | -0.1 | 0.18305 |
PRAD | -0.096 | 0.03472 | -0.023 | 0.60271 |
READ | -0.176 | 0.05380 | 0.195 | 0.01670 |
SARC | 0.064 | 0.34035 | -0.004 | 0.94713 |
SKCM | 0.032 | 0.48829 | 0.035 | 0.45072 |
STAD | 0.101 | 0.05260 | 0.136 | 0.00869 |
TGCT | 0.038 | 0.66762 | 0.28 | 0.00106 |
THCA | -0.019 | 0.68334 | -0.016 | 0.71447 |
THYM | 0.092 | 0.32419 | 0.012 | 0.89740 |
UCEC | -0.253 | 7.33E-09 | -0.2 | 3.04E-06 |
UCS | -0.272 | 0.04268 | -0.294 | 0.02812 |
UVM | -0.181 | 0.13749 | 0.06 | 0.62210 |
To find potential patients who can benefit from this treatment, we selected several potential immune checkpoints to explore whether there is a relationship between the immune escape and MACC1 expression, including SIGLEC15, TIGIT, CD274, HAVCR2, PDCD1, CTLA4, LAG3, and PDCD1LG2. As shown in Figure 5C, expression of all immune checkpoint-associated genes was related to MACC1 expression in LGG, PRAD, SKCM, UVM. Moreover, most of the genes found closely with MACC1 expression except for one gene in GBM, LIHC, SARC, TGCT. Most cancers showed a significant correlation with the immune checkpoint. Our analyses in STAD revealed that MACC1 expression is correlated with that of HAVCR2, LAG3, SIGLEC15, TIGIT. Interestingly, SIGLEC15 has a strong association with MACC1 expression in 21 types of cancer.
To explore the relationship between MACC1 expression and immune infiltration, we further analyzed it with the online TIMER database in 33 cancer types. The results revealed that MACC1 expression was significant correlated with the level of B cell infiltration in 21 types of cancer, and significantly associated with infiltrating levels of CD8+ T cells in 19 types of cancer, CD4+ T cells in 18 types, macrophages in 19 types, neutrophils in 19 types, and dendritic cells in 16 types (Figure 6, Figure S5).
According to the correlations between MACC1 expression and the level of immune-infiltration in various types of cancer, we next selected certain types of cancer in which higher MACC1 expression levels shown significantly worse prognoses according to the TCGA database, such as LGG. And our analysis of TIMER data showed that MACC1 expression was positively relation with immune infiltration. For example, as shown in Figure 6, MACC1 was positively associated with B cell (r = 0.278, P = 6.43e-10), CD8+ T cell (r = 0.336, P = 4.76e-14), CD4+T cell (r = 0.332, P = 9.95e-14), macrophages (r = 0.457, P = 9.49e-26), neutrophil (r = 0.447, P = 1.15e-24) and dendritic cell (r = 0.446, P = 1.22e-24) immune infiltration levels of LGG. In contrast, negative correlations with infiltrating levels of CD8+ T cell (r = -0.179, P = 5.23e-04), CD4 + T cell (r = -0.105, P = 4.39e-02), macrophages (r = -0.229, P = 8.49e-06), neutrophil (r = -0.147, P = 4.60e-03) and dendritic cell (r = -0.24, P = 2.98e-06) were observed in STAD (stomach adenocarcinoma), in which MACC1 expression is associated with better prognosis. We also used TIMER algorithms to analyze this relationship (Figure S6A). Collectively, these results were in line with expectations and strongly suggested that MACC1 plays a vital role in tumor immunity, especially in these tumors.
Additionally, we used XCELL algorithms to elucidate the correlation between MACC1 and diverse infiltrating lymphocytes across 33 cancer types. In this analysis, 64 types of cells were involved using XCELL algorithms, including adaptive and innate immune cells, hematopoietic progenitor cells, epithelial cells, and extracellular matrix cells. As shown in Figure S6B, we found the relationship between MACC1 expression and immune infiltration differed between cancer types. But the results are consistent with TIMER databases in most cancers.
The relationship between MACC1 and six immune cells (B-cells, CD4+T-cells, CD8+T-cells, macrophages, neutrophils, and dendritic cells) in LGG and STAD.
Given that MACC1 expression was found to be associated with immune cell infiltration, we investigated the relationship between MACC1 expression and the representative immune markers of several immune cells, including B cells, CD8+ T cells, T cells (general), macrophages, M1 macrophages, M2 macrophages, tumor-associated macrophages, monocytes, natural killer cells, neutrophils, dendritic cell, and diverse functional T cells. Expression levels of MACC1 were found to be strongly correlated with the most immune markers. In LGG, 41 types of immune markers were positively correlated with MACC1 expression, and in STAD, 30 types of immune markers showed negative correlations of MACC1, consistent with the above results (Table 2). The same results were observed after the adjustment for tumor purity and age. Taken together, the patient with higher MACC1 expression levels may have a poorer prognosis and a significant positive correlation with immune infiltration and most sets of immune markers. Specifically, the results revealed that CD86, CCL2, IL10 of TAMs, CD14 of Monocyte, CD163, VSIG4, MS4A4A of M2 have significant associations with MACC1 expression in LGG and STAD (P < 0.001). Most importantly, we found a significant correlation between MACC1 expression and the regulation of several markers of T cells, such as CD8A of CD8+Tcell, CD3D, CD3E, CD2 of T cell (general), CCR10, AHR of Th22, was observed in LGG and STAD. These results suggested that there was a potential mechanism where MACC1 may regulate T cell functions. Meanwhile, it worth noting that several makers of Treg and T cell exhaustion were closely related to MACC1 expressions, such as STAT5B, CCR8, TGFβ1, CTLA4, LAG3, GZMB. These immune marker genes played a key role in T cell exhaustion, indicating that MACC1 may be involved in the immune escape.
Cell type | Gene marker | LGG | STAD | ||||||||||
None | purity | age | none | purity | age | ||||||||
cor | p | cor | p | cor | p | cor | p | cor | p | cor | p | ||
B cell | CD19 | 0.3000 | *** | 0.2731 | *** | 0.3308 | *** | -0.2446 | *** | -0.2210 | *** | -0.2344 | *** |
CD38 | -0.1206 | ** | -0.1334 | ** | -0.0614 | 0.1650 | -0.1218 | * | -0.1125 | * | -0.1314 | ** | |
CD79A | 0.2047 | *** | 0.2286 | *** | 0.2361 | *** | -0.2327 | *** | -0.2031 | *** | -0.2319 | *** | |
CD8+Tcell | CD8A | 0.3461 | *** | 0.3538 | *** | 0.3323 | *** | -0.1703 | *** | -0.1571 | ** | -0.1673 | *** |
CD8B | 0.2227 | *** | 0.2247 | *** | 0.2090 | *** | -0.2275 | *** | -0.2373 | *** | -0.2213 | *** | |
T cell (general) | CD3D | 0.5183 | *** | 0.5214 | *** | 0.5079 | *** | -0.1984 | *** | -0.1814 | *** | -0.1983 | *** |
CD3E | 0.5515 | *** | 0.5604 | *** | 0.5402 | *** | -0.1893 | *** | -0.1697 | *** | -0.1884 | *** | |
CD2 | 0.5662 | *** | 0.5709 | *** | 0.5525 | *** | -0.1619 | *** | -0.1397 | ** | -0.1647 | *** | |
TAM | CD80 | 0.4756 | *** | 0.4671 | *** | 0.4877 | *** | 0.0169 | 0.7309 | 0.0371 | 0.4719 | -0.0046 | 0.9257 |
CD86 | 0.4007 | *** | 0.3920 | *** | 0.4453 | *** | -0.1517 | ** | -0.1464 | ** | -0.1633 | *** | |
CCL2 | 0.3665 | *** | 0.3644 | *** | 0.3858 | *** | -0.2900 | *** | -0.3056 | *** | -0.2811 | *** | |
IL10 | 0.3796 | *** | 0.3629 | *** | 0.4065 | *** | -0.0967 | * | -0.1058 | * | -0.1062 | * | |
Tfh | CXCR5 | 0.2636 | *** | 0.2575 | *** | 0.2734 | *** | -0.1894 | *** | -0.1612 | ** | -0.1727 | *** |
ICOS | 0.5196 | *** | 0.5134 | *** | 0.5120 | *** | -0.0699 | 0.1553 | -0.0359 | 0.4854 | -0.0851 | 0.0873 | |
BCL6 | -0.0110 | 0.8024 | -0.0276 | 0.5473 | 0.0273 | 0.5371 | -0.2195 | *** | -0.2198 | *** | -0.2121 | *** | |
Th1 | STAT1 | 0.4891 | *** | 0.4811 | *** | 0.4764 | *** | 0.0860 | 0.0801 | 0.1013 | * | 0.0809 | 0.1041 |
IFNG | 0.2944 | *** | 0.2919 | *** | 0.2905 | *** | -0.0324 | 0.5105 | -0.0079 | 0.8785 | -0.0478 | 0.3377 | |
Th2 | CCR3 | 0.3355 | *** | 0.3410 | *** | 0.3375 | *** | -0.0348 | 0.4800 | -0.0143 | 0.7809 | -0.0410 | 0.4111 |
GATA3 | 0.5002 | *** | 0.5044 | *** | 0.4711 | *** | -0.1644 | *** | -0.1553 | ** | -0.1607 | ** | |
STAT5A | 0.4410 | *** | 0.4281 | *** | 0.4601 | *** | 0.0104 | 0.8324 | 0.0189 | 0.7145 | 0.0034 | 0.9461 | |
Th9 | TGFBR2 | 0.4199 | *** | 0.4017 | *** | 0.4561 | *** | 0.0672 | 0.1718 | 0.0622 | 0.2273 | 0.0781 | 0.1168 |
IRF4 | 0.1375 | *** | 0.1175 | *** | 0.1855 | *** | -0.1395 | *** | -0.1135 | ** | -0.1550 | *** | |
Th17 | IL21R | 0.1629 | *** | 0.1686 | *** | 0.1717 | *** | -0.1651 | *** | -0.1387 | ** | -0.1696 | *** |
STAT3 | 0.4223 | *** | 0.4037 | *** | 0.4368 | *** | 0.0377 | 0.4438 | 0.0411 | 0.4253 | 0.0229 | 0.6456 | |
Th22 | CCR10 | 0.2110 | *** | 0.2097 | *** | 0.1851 | *** | -0.2561 | *** | -0.2566 | *** | -0.2481 | *** |
AHR | 0.3579 | *** | 0.3476 | *** | 0.4009 | *** | 0.3871 | *** | 0.3893 | *** | 0.3783 | *** | |
Treg | CCR8 | 0.2271 | *** | 0.2324 | *** | 0.2116 | *** | -0.0113 | 0.8190 | -0.0006 | 0.9914 | -0.0193 | 0.6987 |
STAT5B | -0.0385 | 0.3833 | -0.0326 | 0.4767 | -0.0150 | 0.7343 | 0.0207 | 0.6746 | 0.0162 | 0.7536 | 0.0218 | 0.6623 | |
TGFB1 | 0.3335 | *** | 0.3140 | *** | 0.3851 | *** | -0.1751 | *** | -0.1639 | ** | -0.1820 | *** | |
T cell (exhaustion) | CTLA4 | 0.3479 | *** | 0.3383 | *** | 0.3469 | *** | -0.1141 | * | -0.0915 | 0.0751 | -0.1315 | ** |
LAG3 | 0.2768 | *** | 0.2954 | *** | 0.2914 | *** | -0.1936 | *** | -0.1853 | *** | -0.2056 | *** | |
GZMB | 0.3564 | *** | 0.3813 | *** | 0.3255 | *** | -0.1001 | * | -0.0799 | 0.1206 | -0.1170 | * | |
NK | XCL1 | 0.4235 | *** | 0.4168 | *** | 0.4331 | *** | -0.2295 | *** | -0.2335 | *** | -0.2277 | *** |
KIR3DL1 | 0.1350 | *** | 0.1403 | *** | 0.1393 | *** | -0.1605 | *** | -0.1570 | *** | -0.1715 | *** | |
CD7 | 0.5055 | *** | 0.5146 | *** | 0.5177 | *** | -0.2073 | *** | -0.1951 | *** | -0.2199 | *** | |
Monocyte | CD14 | 0.3595 | *** | 0.3379 | *** | 0.4062 | *** | -0.1944 | *** | -0.2080 | *** | -0.1967 | *** |
Macrophage | CD68 | 0.4314 | *** | 0.4167 | *** | 0.4650 | *** | 0.0576 | 0.2415 | 0.0596 | 0.2473 | 0.0493 | 0.3221 |
M1 | NOS2 | -0.0084 | 0.8491 | -0.0129 | 0.7786 | -0.0245 | 0.5800 | 0.2512 | *** | 0.2618 | *** | 0.2277 | *** |
I5 | 0.3861 | *** | 0.3806 | *** | 0.4182 | *** | 0.0122 | 0.8042 | 0.0107 | 0.8351 | 0.0195 | 0.6961 | |
M2 | CD163 | 0.5021 | *** | 0.4900 | *** | 0.5125 | *** | -0.0821 | 0.0950 | -0.0853 | 0.0972 | -0.1056 | * |
VSIG4 | 0.2379 | *** | 0.2028 | *** | 0.2878 | *** | -0.1702 | *** | -0.1820 | *** | -0.1758 | *** | |
MS4A4A | 0.4084 | *** | 0.3912 | *** | 0.4383 | *** | -0.1663 | *** | -0.1762 | *** | -0.1705 | *** | |
Neutrophil | MPO | 0.0085 | 0.8481 | 0.0009 | 0.9841 | -0.0446 | 0.3129 | -0.1189 | * | -0.1039 | * | -0.1216 | * |
CCR7 | 0.3712 | *** | 0.3739 | *** | 0.3610 | *** | -0.1862 | *** | -0.1691 | *** | -0.1792 | *** | |
DC | CD1C | 0.3017 | *** | 0.2972 | *** | 0.3212 | *** | -0.1579 | ** | -0.1580 | ** | -0.1367 | ** |
*P < 0.05, **P < 0.01, ***P < 0.001. Tfh, follicular helper T cell; Th, T helper cell; Treg, regulatory T cell; TAM, tumor associated macrophage; NK, natural killer cell; DC, dendritic cell; None, correlation without adjustment; Purity, correlation adjusted for tumor purity; Cor, R value of Spearman's correlation. |
Here we analyzed genetic alterations in MACC1 in different tumor samples from TCGA cohort. The results revealed the main type of genetic alteration of MACC1 were missense mutation and amplification. And the highest frequency of MACC1 alteration (>8%) occurred in patients with melanoma tumors, in which "mutation" was the predominant type of alteration. The "amplification" was the dominant type in people with esophageal squamous cell carcinoma, sarcoma, seminoma, pancreatic adenocarcinoma, and pheochromocytoma (Figure 7A).
We also investigated genetic alteration of MACC1 across different cancers. Furthermore, we analyzed whether the MACC1 copy number variations impact the level of immune infiltrates, including six types of immune cells. As shown in Figure 7B, deletion and amplification of MACC1 were both found to be correlated with the lower levels of immune infiltrates in STAD and LUSC. In SKCM and HNSC, lower levels of six immune cells are mainly associated with the amplification of MACC1. Conversely, in LUAD and HNSC-HPV neg, deletion of MACC1 was related to the same results.
To our knowledge, this is the first pan-cancer analysis of MACC1, providing novel insights into expression pattern, function, association with immune cell infiltration, and disease prognosis, which together form a solid basis for further research into diagnostic and therapeutic strategies. And the results of our analysis can help to prioritize cancer types for further clinical trials and experimental studies.
Metastasis-associated in colon cancer-1 (MACC1) was first identified through genome-wide expression analyses in 2009. Furthermore, they also revealed that MACC1 can induce cell proliferation, invasion, migration, and regulate transcription of genes involved in the metastasis process [5]. Jan et al. demonstrated that MACC1 was significantly correlation with the key transcription genes in more than half of the cancer types in the big data analysis, including receptor tyrosine kinase MET, hepatocyte growth factor (HGF) and recombinant Matrix Metalloproteinase 7 (MMP7) [25]. In addition, the meta-analysis results suggested MACC1 was correlation with regional invasion and distant metastasis [26,27]. MACC1 is a significant biomarker for the prognosis of colorectal cancer, but it is also applicable to various other forms of solid cancer. It has been proved to be a predictor of many solid cancers, such as lung, breast, and pancreatic cancer. Pan-cancer analysis displays the similarities and differences between genomic and cellular changes in different tumor types and provides new insights into cancer prevention and treatment targets [28]. However, the role of MACC1 in a full spectrum of tumors has not been identified.
According to our gene homologous and phylogenetic tree analysis in our study, the protein structure of MACC1 was found to be conserved, indicating that the normal physiological function of MACC1 may be similar among species. This provides new ideas for understanding the role in the pathogenesis of different tumors.
In this study, MACC1 was differentially expressed in tumor versus normal tissues, and its expression varied across diverse cancer types. These results suggest that MACC1 may play different roles in different cancers. Nevertheless, this was a preliminary assumption. To verify the role of MACC1 in tumors, we performed prognostic analyses in cancers with different MACC1 expression levels, which revealed that higher MACC1 expression was correlated with better prognoses in KIRC, SKCM, KIRP, but worse prognoses in COAD, LGG, UVM, PAAD. Our Kaplan-Meier plotter analyses showed that high level of MACC1 expression was correlated with better prognosis in lung and gastric cancer, but poor prognosis in ovarian cancer. In addition, wang et al demonstrated that high expression of MACC1 predicts a worse prognosis of gynecologic cancers and breast cancer. But further studies with larger sample sizes are needed to validate the role of MACC1 in gynecologic cancers [29]. As a predictor for metastasis formation in colon cancer, we future investigated the relationship between clinical phenotypes and the expression level of MACC1. A previous study examined the expression of MACC1 in 52 clinical specimens with different pathological stages in colorectal carcinoma using qRT-PCR [30]. The results are consistent with ours, MACC1 expression was higher in patients with advanced colon cancer. The trend was also observed in CESE, ESCA, UCEC, THCA; however, MACC1 expression was higher in the early-stage KIRP, KIRC, and KICH compared with later stage disease. These results suggest that the role of MACC1 varies between cancer types, and may serve as an indicator of disease progression in some cancers and as an early warning in others. Cancer is a highly heterogeneous set of disease; emerging evidence suggests that differences in signal transduction driven by the same oncogene may lead to different clinical outcomes depending on the tumor type, which may help to explain our findings.[31] For example, the BRAF inhibitor is effective in people with melanoma who have a BRAF-driven mutation, but not in those with colorectal cancer [32,33]. Gene function may vary between different cancers and genetic changes in the same cancer may have different outcomes. Our results provide evidence for MACC1 as an independent prognostic factor, but that the underlying mechanisms for its role and prognostic value in different cancer types require further study.
The transformation of normal cells into cancer cell mostly involves proteomics, transcriptomics, epigenetics, and somatic acquired genetic changes [34]. Epigenetic modification within the genome, including methylation, can affect disease progression. A previous study demonstrated that hypomethylation can cause genome instability and lead to gene activation [35]. An important characteristic of many tumors is the hypomethylation of oncogene promoters, and hypermethylation of tumor suppressor gene promoters. Abnormal DNA methylation can be used as an important molecular marker for tumor diagnosis, classification, and treatment [36,37]. And we found that MACC1 expression varies across cancer types and is significantly correlated with DNA methylation status [38]. Previous studies show that demethylation may activate the expression of the tumor genes, resulting in up-regulation or instability in the chromosomal structure. Hypermethylation may affects the cell cycle by silencing genes resulting in down-regulation and inhibition of DNA damage repair [37]. Most cancer types in this study were associated with a high expression level of MACC1 and corresponding low methylation levels of MACC1 promoters compared with normal tissue, supporting both previous research and the role of MACC1 as a potential biomarker. In our study, we have confirmed this theory. In most cancers, with a high expression level of MACC1, the methylation levels of MACC1 promoters are lower than in normal tissue. Above all, we can speculate that there is a certain relationship between increased MACC1 expression levels and methylation in cancer, suggesting that MACC1 is an attempt to identify the role of biomarkers.
Immunotherapy is an important treatment option for people with cancer these years. However, only a small proportion of people benefit from immune checkpoint inhibitors. Therefore, the exploration of promising biomarkers is a clinical priority. Whether there is a relationship between MACC1 and immunity has attracted our attention. The adaptive immune system can recognize cancer by non-autoantigens associated with somatic mutations. TMB levels influence the generation of immunogenic peptides, which in turn affect the patient's response to immune checkpoint inhibitors. And genomic instability is a new feature of cancer and is closely related to cancer development, progression and metastasis [39]. Microsatellite instability (MSI) level can affect TMB level, which is a crucial index to predict tumorigenesis. And both TMB and MSI can be used to predict the potential efficacy of immunotherapy. It is important to explore the relationship between MACC1 expression and TMB levels in cancer patients. In our study, an association between TMB and MACC1 was observed in 14 types of cancer and between MSI and MACC1 in six types of cancer. A previous study found that MACC1 works with mismatch repair (MMR) to predict the response to adjuvant chemotherapy. And MACC1 stratifies colon cancer patients with poor pMMR status and tumor patients with pMMR/MACC1-low tumors have similar outcomes as dMMR patients. This further suggests a potential functional link between MACC1 and genomic instability [40].
Importantly, we further explored the relationship between immune infiltration and MACC1 expression in selected cancers based on our prognostic results. The tumor microenvironment (TME) has been the focus of recent oncology research, which is mainly is mainly composed of tumor-infiltrating lymphocytes (TILs; B cells and T cells), dendritic cells, neutrophils, and macrophages [41,42]. Immune cells, as a double-edged sword, play a role in promoting or suppressing cancer in different situations [43]. On the one hand, the tumor microenvironment not only contributes to tumor genesis, development, metastasis, and tumor immune tolerance. Extensive researches have proved that tumor-associated macrophages and other inflammatory cells can promote angiogenesis and many tumor cells can evade the surveillance of immune cells through a variety of mechanisms. On the other hand, some inflammatory cells, notably T cells, have inhibitory properties, monitoring and destroying tumor cells and are associated with a favorable prognosis in many tumor types [44]. In our study, we found that MACC1 expression were inversely correlated with immune infiltration (especially, the CD4+ T cell and CD8+ T cell, macrophages, neutrophils, dendritic cells), in which MACC1 expression is related to better prognosis. In contrast, in other cancer types, the level of immune infiltration was positively correlated with MACC1 expression. These results revealed a strong correlation between MACC1 and immune infiltration, but that this relationship varied between cancer types. However, MACC1 may lead to tumorigenesis or inhibit tumor progression by changing the state of tumor-infiltrating lymphocytes. As an initial investigation of the potential mechanism underlying this process, the correlation between MACC1 expression and that of immune cell markers was further assessed. First, gene markers of TAMs such as CCL2, CD86, and IL-10 showed correlations with MACC1 expression. Meanwhile, M2 macrophage markers such as VSIG4 and MS4A4A were strongly related to MACC1 expression. These results demonstrated that MACC1 expression may be related to the polarization of tumor-associated macrophages. Moreover, another interesting aspect of our study is that the potential regulating role of MACC1 in T cell function. T cells are an important component of adaptive immunity and have become a focus of immunotherapy owing to their capacity for specific antigen recognition and tumor destruction [45]. In our study, a significant correlation can be observed between MACC1 expression and T helper cells (Th22), T cell (general). As we all know, the immune system prevents tumor progression mainly by activating T cells and macrophages. However, as the tumor progresses, the immune infiltration will transform to promote tumor progression and inhibit immune cell-mediated cytotoxicity. Identifying and characterizing interactions between the immune system and the tumor is crucial for both reducing drug resistance and developing new cancer treatments. These results may provide new insights into the tumorigenesis of MACC1 and immune infiltration; however, further research is needed to clarify the exact mechanism for this. In addition, our analysis of some common immune checkpoints, such as SIGLEC15, TIGIT, CD274, HAVCR2, PDCD1, CTLA4, LAG3, and PDCD1LG2, demonstrated that these were associated with most of the cancers; Tong and his colleagues verified that MACC1 can regulate PDL1 expression in gastric cancer cells. And the positive results suggest that MACC1 may be a therapeutic target for immunotherapy [46]. In particular, SIGLEC15 may be a potential treatment option for patients who are not likely to benefit from anti-PD1 therapy [47]. Overall, our study confirmed the predictive value of MACC1 as a metastatic biomarker. And alterations in the expression of MACC1 may promote tumor progression by influencing the levels of various cancer marker genes; this process could potentially be prevented by targeting the expression or function of MACC1 as a therapeutic strategy, limiting or even preventing metastasis.
Although in our pan-cancer analysis, the role of MACC1 expression has been extensively explored in various genetic and biological processes, including methylation, prognosis, immune infiltration, etc.; however, there are some limitations. First, multiple analyses based on diverse databases can provide large amounts of data. But the discrepancies in results may be a reflection in data collection approaches, data heterogeneous to a certain extent, and underlying mechanisms pertinent to different biological properties. Second, our analysis is based on various databases, and interpretation of our analysis results is inherently limited by this approach. Therefore, experimental studies are needed to elucidate the mechanism of action of MACC1 and further clinical trials are needed to validate possible therapeutic interventions in the process of transition into the clinic. In the future, there will be more researches to validate the highlights of our bioinformatics analysis.
In conclusion, our pan-cancer analysis for the first time revealed that the expression of MACC1 was statistically significant with clinical prognosis, pathological stage, immune infiltration, DNA methylation, cell infiltration of multiple tumors, and drug targets, etc., contributing to a more comprehensive understanding of the role of MACC1 in tumorigenesis, and the identification of potential therapeutic targets. More importantly, we explored that MACC1 may closely related to cancer immunity.
This work was supported by the National Natural Science Foundation of China (No.81071127, 81471751, 81673762).
The authors declare that they have no competing interests.
The study analyzed publicly available data sets, which can be found in The Cancer Genome Atlas (https://portal.gdc.cancer.gov), GEO database (https://www.ncbi.nlm.nih.gov/geo), cbioportal (http://www.cbioportal.org), Oncomine database (https://www.oncomine.org/resource/main.html).
[1] |
L. A. Salas, K. C. Johnson, D. C. Koestler, D. E. O'Sullivan, B. C. Christensen, Integrative epigenetic and genetic pan-cancer somatic alteration portraits, Epigenetics, 12 (2017), 561-574. doi: 10.1080/15592294.2017.1319043
![]() |
[2] |
S. Xie, C. Shen, M. Tan, M. Li, X. Song, C. Wang, Systematic analysis of gene expression alterations and clinical outcomes of adenylate cyclase-associated protein in cancer, Oncotarget, 8 (2017), 27216-27239. doi: 10.18632/oncotarget.16111
![]() |
[3] |
M. W. Ball, M. A. Gorin, C. G. Drake, H. J. Hammers, M. E. Allaf, The Landscape of Whole-genome Alterations and Pathologic Features in Genitourinary Malignancies: An Analysis of the Cancer Genome Atlas, Eur. Urol. Focus, 3 (2017), 584-589. doi: 10.1016/j.euf.2017.01.007
![]() |
[4] | J. Fridlyand, A. M. Snijders, B. Ylstra, H. Li, A. Olshen, R. Segraves, et al., Breast tumor copy number aberration phenotypes and genomic instability, BMC Cancer, 6 (2016), 96. |
[5] | U. Stein, W. Walther, F. Arlt, H. Schwabe, J. Smith, I. Fichtner, et al., MACC1, a newly identified key regulator of HGF-MET signaling, predicts colon cancer metastasis, Nat. Med., 15 (2009), 59-67. |
[6] | A. Shirahata, W. Fan, K. Sakuraba, K. Yokomizo, T. Goto, H. Mizukami, et al., MACC 1 as a Marker for Vascular Invasive Hepatocellular Carcinoma, Anticancer Res., 31 (2011), 777-780. |
[7] |
G. Wang, M. X. Kang, W. J. Lu, Y. Chen, B. Zhang, Y. L. Wu, MACC1: A potential molecule associated with pancreatic cancer metastasis and chemoresistance, Oncol. Lett, 4 (2012), 783-791. doi: 10.3892/ol.2012.784
![]() |
[8] |
H. Shimokawa, H. Uramoto, T. Onitsuka, C. Guang, T. Hanagiri, T. Oyama, et al., Overexpression of MACC1 mRNA in lung adenocarcinoma is associated with postoperative recurrence, J. Thorac. Cardiovasc Surg., 141 (2011), 895-898. doi: 10.1016/j.jtcvs.2010.09.044
![]() |
[9] |
R. T. Zhang, H. R. Shi, Z. M. Chen, Q. H. Wu, F. Ren, H. L. Huang, Effects of metastasis-associated in colon cancer 1 inhibition by small hairpin RNA on ovarian carcinoma OVCAR-3 cells, J. Exp. Clin. Can. Res., 30 (2011), 83. doi: 10.1186/1756-9966-30-83
![]() |
[10] |
Y. B. Huang, H. Z. Zhang, J. C. Cai, L. S. Fang, J. H. Wu, C. S. Ye, et al., Overexpression of MACC1 and Its significance in human Breast Cancer Progression, Cell Biosci., 3 (2013), 16. doi: 10.1186/2045-3701-3-16
![]() |
[11] |
T. H. Yang, M. Kon, J. H. Hung, C. Delisi, Combinations of newly confirmed Glioma-Associated loci link regions on chromosomes 1 and 9 to increased disease risk, BMC Med. Genom., 4 (2011), 63. doi: 10.1186/1755-8794-4-63
![]() |
[12] |
J. Barbazan, Y. Dunkel, H. Y. Li, U. Nitsche, K. P. Janssen, K. Messer, et al., Prognostic Impact of Modulators of G proteins in Circulating Tumor Cells from Patients with Metastatic Colorectal Cancer, Sci. Rep.-Uk, 6 (2016), 22112. doi: 10.1038/srep22112
![]() |
[13] |
A. Pichorner, U. Sack, D. Kobelt, I. Kelch, F. Arlt, J. Smith, et al., In vivo imaging of colorectal cancer growth and metastasis by targeting MACC1 with shRNA in xenografted mice, Clin. Exp. Metastas., 29 (2012), 573-583. doi: 10.1007/s10585-012-9472-6
![]() |
[14] | Z. Wu, R. Zhou, Y. Su, S. Li, Y. Liao, W. Liao, Prognostic Value of MACC1 in Digestive System Neoplasms: A Systematic Review and Meta-Analysis., BioMed. Res. Int., 2015 (2015), 252043. |
[15] |
G. Wang, Z. Fu, D. Li, MACC1 overexpression and survival in solid tumors: a meta-analysis, Tumour. Biol., 36 (2015), 1055-1065. doi: 10.1007/s13277-014-2736-9
![]() |
[16] |
Y. Jin, K. Zhou, W. Zhao, R. Han, X. Huo, F. Yang, et al., Clinicopathological and prognostic significance of metastasis-associated in colon cancer-1 in gastric cancer: A meta-analysis, Int. J. Biol. Markers, 34 (2019), 27-32. doi: 10.1177/1724600818813634
![]() |
[17] |
M. Uhlen, L. Fagerberg, B. M. Hallstrom, C. Lindskog, P. Oksvold, A. Mardinoglu, et al., Proteomics., Tissue-based map of the human proteome, Science, 347 (2015), 1260419. doi: 10.1126/science.1260419
![]() |
[18] | T. Li, J. Fu, Z. Zeng, D. Cohen, J. Li, Q. Chen, et al., TIMER2.0 for analysis of tumor-infiltrating immune cells, Nucleic Acids Res., 48 (2020), W509-W514. |
[19] |
D. S. Chandrashekar, B. Bashel, S. A. Balasubramanya, C. J. Creighton, I. P. Rodrigues, B. V. Chakravarthi, et al., UALCAN: A Portal for Facilitating Tumor Subgroup Gene Expression and Survival Analyses, Neoplasia, 19 (2017), 649-658. doi: 10.1016/j.neo.2017.05.002
![]() |
[20] |
Z. Tang, B. Kang, C. Li, T. Chen, Z. Zhang, GEPIA2: an enhanced web server for large-scale expression profiling and interactive analysis, Nucleic Acids Res., 47 (2019), W556-W560. doi: 10.1093/nar/gkz430
![]() |
[21] |
M. Yarchoan, A. Hopkins, E. M. Jaffee, Tumor Mutational Burden and Response Rate to PD-1 Inhibition, N Eng. J. Med., 377 (2017), 2500-2501. doi: 10.1056/NEJMc1713444
![]() |
[22] | T. Krieger, I. Pearson, J. Bell, J. Doherty, P. Robbins, Targeted literature review on use of tumor mutational burden status and programmed cell death ligand 1 expression to predict outcomes of checkpoint inhibitor treatment, Diagn. Pathol., 15 (2010), 6. |
[23] | T. W. Li, J. Y. Fan, B. B. Wang, N. Traugh, Q. M. Chen, J. S. Liu, et al., TIMER: A Web Server for Comprehensive Analysis of Tumor-Infiltrating Immune Cells, Cancer Res., 77 (2019), E108-E110. |
[24] |
E. Cerami, J. Gao, U. Dogrusoz, B. E. Gross, S. O. Sumer, B. A. Aksoy, et al., The cBio cancer genomics portal: an open platform for exploring multidimensional cancer genomics data, Cancer Discov., 2 (2012), 401-404. doi: 10.1158/2159-8290.CD-12-0095
![]() |
[25] |
J. Budczies, K. Kluck, W. Walther, Decoding and targeting the molecular basis of MACC1-driven metastatic spread: Lessons from big data mining and clinical-experimental approaches, Semin. Cancer Biol., 60 (2020), 365-379. doi: 10.1016/j.semcancer.2019.08.010
![]() |
[26] | D. W. Sun, Y. Y. Zhang, Y. Qi, G. Q. Liu, Y. G. Chen, J. Ma, et al., Prognostic and clinicopathological significance of MACC1 expression in hepatocellular carcinoma patients: a meta-analysis, Int. J. Clin. Exp. Med., 8 (2015), 4769-4777. |
[27] |
Y. Zhao, C. Dai, M. Wang, H. F. Kang, S. Lin, P. T. Yang, et al., Clinicopathological and prognostic significance of metastasis-associated in colon cancer-1 (MACC1) overexpression in colorectal cancer: a meta-analysis, Oncotarget, 7 (2016), 62966-62975. doi: 10.18632/oncotarget.11287
![]() |
[28] |
F. X. Schaub, V. Dhankani, A. C. Berger, M. Trivedi, A. B. Richardson, R. Shaw, et al., Pan-cancer Alterations of the MYC Oncogene and Its Proximal Network across the Cancer Genome Atlas, Cell Syst., 6 (2018), 282. doi: 10.1016/j.cels.2018.03.003
![]() |
[29] | L. J. Wang, L. Y. Fan, H. Y. Xu, H. Y. Jiang, Prognostic significance of the expression of metastasis-associated in colon cancer-1 in gynecologic cancers and breast cancer A protocol for systematic review and meta-analysis, Medicine, 100 (2021). |
[30] | A. Shirahata, K. Shinmura, Y. Kitamura, K. Sakuraba, K. Yokomizo, T. Goto, et al., MACC1 as a Marker for Advanced Colorectal Carcinoma, Anticancer Res., 30(2010), 2689-2692. |
[31] |
A. Stewart, E. A. Coker, S. Palsterl, A. Georgiou, A. R. Minchom, S. Carreira, et al., Differences in Signaling Patterns on PI3K Inhibition Reveal Context Specificity in KRAS-Mutant Cancers, Mol. Cancer Ther., 18 (2019), 1396-1404. doi: 10.1158/1535-7163.MCT-18-0727
![]() |
[32] |
P. B. Chapman, A. Hauschild, C. Robert, J. B. Haanen, P. Ascierto, J. Larkin, et al., Improved Survival with Vemurafenib in Melanoma with BRAF V600E Mutation., New Eng. J. Med., 364 (2011), 2507-2516. doi: 10.1056/NEJMoa1103782
![]() |
[33] | U. J. M. Mey, C. Renner, R. von Moos, Vemurafenib in combination with cobimetinib in relapsed and refractory extramedullary multiple myeloma harboring the BRAF V600E mutation, Hematol. Oncol., 35 (2017), 890-893. |
[34] | D. Subramaniam, R. Thombre, A. Dhar, S. Anant, DNA methyltransferases: a novel target for prevention and therapy, Front. Oncol., 4 (2014), 80. |
[35] |
H. Heyn, M. Esteller, DNA methylation profiling in the clinic: applications and challenges, Nat. Rev. Genet., 13 (2012), 679-692. doi: 10.1038/nrg3270
![]() |
[36] |
M. R. Hassler, W. Pulverer, R. Lakshminarasimhan, E. Redl, J. Hacker, G. D. Garland, et al., Insights into the Pathogenesis of Anaplastic Large-Cell Lymphoma through Genome-wide DNA Methylation Profiling, Cell Rep., 17 (2016), 596-608. doi: 10.1016/j.celrep.2016.09.018
![]() |
[37] |
T. Hinoue, D. J. Weisenberger, C. P. E. Lange, H. Shen, H. M. Byun, D. V. D. Berg, et al., Genome-scale analysis of aberrant DNA methylation in colorectal cancer, Genome Res., 22 (2012), 271-282. doi: 10.1101/gr.117523.110
![]() |
[38] |
N. Bethge, R. A. Lothe, H. Honne, K. Andresen, G. Troen, M. Eknaes, et al., Colorectal cancer DNA methylation marker panel validated with high performance in non-hodgkin lymphoma, Epigenetics, 9 (2014), 428-436. doi: 10.4161/epi.27554
![]() |
[39] |
D. Hanahan, R. A. Weinberg, Hallmarks of Cancer: The Next Generation, Cell, 144 (2011), 646-674. doi: 10.1016/j.cell.2011.02.013
![]() |
[40] |
U. P. Rohr, P. Herrmann, K. Ilm, H. Zhang, S. Lohmann, A. Reiser, et al., Prognostic value of MACC1 and proficient mismatch repair status for recurrence risk prediction in stage II colon cancer patients: the BIOGRID studies, Ann. Oncol., 28 (2017), 1869-1875. doi: 10.1093/annonc/mdx207
![]() |
[41] |
Z. Wang, K. Song, W. X. Zhao, Z. M. Zhao, Dendritic cells in tumor microenvironment promoted the neuropathic pain via paracrine inflammatory and growth factors, Bioengineered, 11 (2020), 661-678. doi: 10.1080/21655979.2020.1771068
![]() |
[42] |
S. Ostrand-Rosenberg, Immune surveillance: a balance between protumor and antitumor immunity, Curr. Opin. Genet. Dev., 18 (2008), 11-18. doi: 10.1016/j.gde.2007.12.007
![]() |
[43] |
C. Murdoch, M. Muthana, S. B. Coffelt, C. E. Lewis, The role of myeloid cells in the promotion of tumour angiogenesis, Nat. Rev. Cancer, 8 (2008), 618-631. doi: 10.1038/nrc2444
![]() |
[44] |
P. Sharma, S. Hu-Lieskovan, J. A. Wargo, A. Ribas, Primary, Adaptive, and Acquired Resistance to Cancer Immunotherapy, Cell, 168 (2017), 707-723. doi: 10.1016/j.cell.2017.01.017
![]() |
[45] | B. V. Kumar, T. J. Connors, D. L. Farber, Human T Cell Development, Localization, and Function throughout Life, Immunity, 48 (2018), 202-213. |
[46] |
G. Tong, B. Cheng, J. Li, X. Wu, Q. Nong, L. He, et al., MACC1 regulates PDL1 expression and tumor immunity through the c-Met/AKT/mTOR pathway in gastric cancer cells, Cancer Med., 8 (2019), 7044-7054. doi: 10.1002/cam4.2542
![]() |
[47] |
J. Wang, J. W. Sun, L. N. Liu, D. B. Flies, X. X. Nie, M. Toki, et al., Siglec-15 as an immune suppressor and potential target for normalization cancer immunotherapy, Nat. Med., 25 (2019), 656. doi: 10.1038/s41591-019-0374-x
![]() |
![]() |
![]() |
1. | Xiaotao Qian, Yongxin Zhao, Tengyue Zhang, Pingsheng Fan, Downregulation of MACC1 facilitates the reversal effect of verapamil on the chemoresistance to active metabolite of irinotecan in human colon cancer cells, 2022, 8, 24058440, e11294, 10.1016/j.heliyon.2022.e11294 | |
2. | Ivan Izonin, Nataliya Shakhovska, Special issue: informatics & data-driven medicine-2021, 2022, 19, 1551-0018, 9769, 10.3934/mbe.2022454 | |
3. | Bi-Xia Liu, Jing Yang, Chunyan Zeng, Youxiang Chen, Nabiha Missaoui, MACC1 Correlates with Tumor Progression and Immune Cell Infiltration of Colon Adenocarcinoma and is Regulated by the lncRNA ZFAS1/miR-642a-5p Axis, 2022, 2022, 1687-8469, 1, 10.1155/2022/8179208 | |
4. | Mengmeng Lv, Yunjuan Jiao, Bowen Yang, Mengchen Ye, Wenyu Di, Wei Su, Jiateng Zhong, MACC1 as a Potential Target for the Treatment and Prevention of Breast Cancer, 2023, 12, 2079-7737, 455, 10.3390/biology12030455 | |
5. | Shuke Ge, Xu Sun, Limin Sang, Min Zhang, Xubo Yan, Qi Ju, Xuefeng Ma, Meng Xu, Curcumin inhibits malignant behavior of colorectal cancer cells by regulating M2 polarization of tumor‐associated macrophages and metastasis associated in colon cancer 1 (MACC1) expression, 2023, 102, 1747-0277, 1202, 10.1111/cbdd.14330 | |
6. | Sebastian Torke, Wolfgang Walther, Ulrike Stein, Immune Response and Metastasis—Links between the Metastasis Driver MACC1 and Cancer Immune Escape Strategies, 2024, 16, 2072-6694, 1330, 10.3390/cancers16071330 | |
7. | Paul Curtis Schöpe, Sebastian Torke, Dennis Kobelt, Benedikt Kortüm, Christoph Treese, Malti Dumbani, Nazli Güllü, Wolfgang Walther, Ulrike Stein, MACC1 revisited – an in-depth review of a master of metastasis, 2024, 12, 2050-7771, 10.1186/s40364-024-00689-4 | |
8. | Buze Chen, Ke'er Jiang, Haihong Wang, Lu Miao, Xiaoman Lin, Qing Chen, Li Jing, Xiaoyuan Lu, NOTCH Pathway Genes in Ovarian Cancer: Clinical Significance and Associations with Immune Cell Infiltration, 2023, 28, 2768-6701, 10.31083/j.fbl2809220 |
Cancer | TMB | MSI | ||
Correlation | P-value | Correlation | P-value | |
ACC | -0.142 | 0.21873 | 0.071 | 0.53964 |
BLCA | 0.027 | 0.59282 | -0.003 | 0.94940 |
BRCA | -0.073 | 0.02169 | -0.009 | 0.76597 |
CESC | 0.046 | 0.57506 | -0.026 | 0.65448 |
CHOL | -0.199 | 0.24307 | 0.242 | 0.15427 |
COAD | -0.293 | 2.48E-08 | 0.077 | 0.11088 |
DLBC | -0.295 | 0.07668 | -0.28 | 0.05424 |
ESCA | 0.367 | 3.82E-06 | 0.139 | 0.07896 |
GBM | 0.058 | 0.48026 | 0.037 | 0.65588 |
HNSC | 0.153 | 0.00070 | -0.015 | 0.73890 |
KICH | -0.251 | 0.04581 | 0.14 | 0.26538 |
KIRC | -0.033 | 0.53559 | 0.079 | 0.14706 |
KIRP | 0.128 | 0.03413 | 0.021 | 0.72721 |
LAML | -0.161 | 0.09980 | -0.051 | 0.59316 |
LGG | 0.343 | 9.75E-15 | -0.072 | 0.11118 |
LIHC | -0.141 | 0.01563 | 0.001 | 0.97814 |
LUAD | -0.173 | 9.99E-05 | 0.03 | 0.49973 |
LUSC | 0.007 | 0.88178 | -0.039 | 0.38478 |
MESO | -0.146 | 0.19437 | 0.01 | 0.93062 |
OV | -0.1 | 0.11796 | 0.142 | 0.01827 |
PAAD | 0.348 | 7.28E-06 | -0.046 | 0.54665 |
PCPG | 0.043 | 0.57813 | -0.1 | 0.18305 |
PRAD | -0.096 | 0.03472 | -0.023 | 0.60271 |
READ | -0.176 | 0.05380 | 0.195 | 0.01670 |
SARC | 0.064 | 0.34035 | -0.004 | 0.94713 |
SKCM | 0.032 | 0.48829 | 0.035 | 0.45072 |
STAD | 0.101 | 0.05260 | 0.136 | 0.00869 |
TGCT | 0.038 | 0.66762 | 0.28 | 0.00106 |
THCA | -0.019 | 0.68334 | -0.016 | 0.71447 |
THYM | 0.092 | 0.32419 | 0.012 | 0.89740 |
UCEC | -0.253 | 7.33E-09 | -0.2 | 3.04E-06 |
UCS | -0.272 | 0.04268 | -0.294 | 0.02812 |
UVM | -0.181 | 0.13749 | 0.06 | 0.62210 |
Cell type | Gene marker | LGG | STAD | ||||||||||
None | purity | age | none | purity | age | ||||||||
cor | p | cor | p | cor | p | cor | p | cor | p | cor | p | ||
B cell | CD19 | 0.3000 | *** | 0.2731 | *** | 0.3308 | *** | -0.2446 | *** | -0.2210 | *** | -0.2344 | *** |
CD38 | -0.1206 | ** | -0.1334 | ** | -0.0614 | 0.1650 | -0.1218 | * | -0.1125 | * | -0.1314 | ** | |
CD79A | 0.2047 | *** | 0.2286 | *** | 0.2361 | *** | -0.2327 | *** | -0.2031 | *** | -0.2319 | *** | |
CD8+Tcell | CD8A | 0.3461 | *** | 0.3538 | *** | 0.3323 | *** | -0.1703 | *** | -0.1571 | ** | -0.1673 | *** |
CD8B | 0.2227 | *** | 0.2247 | *** | 0.2090 | *** | -0.2275 | *** | -0.2373 | *** | -0.2213 | *** | |
T cell (general) | CD3D | 0.5183 | *** | 0.5214 | *** | 0.5079 | *** | -0.1984 | *** | -0.1814 | *** | -0.1983 | *** |
CD3E | 0.5515 | *** | 0.5604 | *** | 0.5402 | *** | -0.1893 | *** | -0.1697 | *** | -0.1884 | *** | |
CD2 | 0.5662 | *** | 0.5709 | *** | 0.5525 | *** | -0.1619 | *** | -0.1397 | ** | -0.1647 | *** | |
TAM | CD80 | 0.4756 | *** | 0.4671 | *** | 0.4877 | *** | 0.0169 | 0.7309 | 0.0371 | 0.4719 | -0.0046 | 0.9257 |
CD86 | 0.4007 | *** | 0.3920 | *** | 0.4453 | *** | -0.1517 | ** | -0.1464 | ** | -0.1633 | *** | |
CCL2 | 0.3665 | *** | 0.3644 | *** | 0.3858 | *** | -0.2900 | *** | -0.3056 | *** | -0.2811 | *** | |
IL10 | 0.3796 | *** | 0.3629 | *** | 0.4065 | *** | -0.0967 | * | -0.1058 | * | -0.1062 | * | |
Tfh | CXCR5 | 0.2636 | *** | 0.2575 | *** | 0.2734 | *** | -0.1894 | *** | -0.1612 | ** | -0.1727 | *** |
ICOS | 0.5196 | *** | 0.5134 | *** | 0.5120 | *** | -0.0699 | 0.1553 | -0.0359 | 0.4854 | -0.0851 | 0.0873 | |
BCL6 | -0.0110 | 0.8024 | -0.0276 | 0.5473 | 0.0273 | 0.5371 | -0.2195 | *** | -0.2198 | *** | -0.2121 | *** | |
Th1 | STAT1 | 0.4891 | *** | 0.4811 | *** | 0.4764 | *** | 0.0860 | 0.0801 | 0.1013 | * | 0.0809 | 0.1041 |
IFNG | 0.2944 | *** | 0.2919 | *** | 0.2905 | *** | -0.0324 | 0.5105 | -0.0079 | 0.8785 | -0.0478 | 0.3377 | |
Th2 | CCR3 | 0.3355 | *** | 0.3410 | *** | 0.3375 | *** | -0.0348 | 0.4800 | -0.0143 | 0.7809 | -0.0410 | 0.4111 |
GATA3 | 0.5002 | *** | 0.5044 | *** | 0.4711 | *** | -0.1644 | *** | -0.1553 | ** | -0.1607 | ** | |
STAT5A | 0.4410 | *** | 0.4281 | *** | 0.4601 | *** | 0.0104 | 0.8324 | 0.0189 | 0.7145 | 0.0034 | 0.9461 | |
Th9 | TGFBR2 | 0.4199 | *** | 0.4017 | *** | 0.4561 | *** | 0.0672 | 0.1718 | 0.0622 | 0.2273 | 0.0781 | 0.1168 |
IRF4 | 0.1375 | *** | 0.1175 | *** | 0.1855 | *** | -0.1395 | *** | -0.1135 | ** | -0.1550 | *** | |
Th17 | IL21R | 0.1629 | *** | 0.1686 | *** | 0.1717 | *** | -0.1651 | *** | -0.1387 | ** | -0.1696 | *** |
STAT3 | 0.4223 | *** | 0.4037 | *** | 0.4368 | *** | 0.0377 | 0.4438 | 0.0411 | 0.4253 | 0.0229 | 0.6456 | |
Th22 | CCR10 | 0.2110 | *** | 0.2097 | *** | 0.1851 | *** | -0.2561 | *** | -0.2566 | *** | -0.2481 | *** |
AHR | 0.3579 | *** | 0.3476 | *** | 0.4009 | *** | 0.3871 | *** | 0.3893 | *** | 0.3783 | *** | |
Treg | CCR8 | 0.2271 | *** | 0.2324 | *** | 0.2116 | *** | -0.0113 | 0.8190 | -0.0006 | 0.9914 | -0.0193 | 0.6987 |
STAT5B | -0.0385 | 0.3833 | -0.0326 | 0.4767 | -0.0150 | 0.7343 | 0.0207 | 0.6746 | 0.0162 | 0.7536 | 0.0218 | 0.6623 | |
TGFB1 | 0.3335 | *** | 0.3140 | *** | 0.3851 | *** | -0.1751 | *** | -0.1639 | ** | -0.1820 | *** | |
T cell (exhaustion) | CTLA4 | 0.3479 | *** | 0.3383 | *** | 0.3469 | *** | -0.1141 | * | -0.0915 | 0.0751 | -0.1315 | ** |
LAG3 | 0.2768 | *** | 0.2954 | *** | 0.2914 | *** | -0.1936 | *** | -0.1853 | *** | -0.2056 | *** | |
GZMB | 0.3564 | *** | 0.3813 | *** | 0.3255 | *** | -0.1001 | * | -0.0799 | 0.1206 | -0.1170 | * | |
NK | XCL1 | 0.4235 | *** | 0.4168 | *** | 0.4331 | *** | -0.2295 | *** | -0.2335 | *** | -0.2277 | *** |
KIR3DL1 | 0.1350 | *** | 0.1403 | *** | 0.1393 | *** | -0.1605 | *** | -0.1570 | *** | -0.1715 | *** | |
CD7 | 0.5055 | *** | 0.5146 | *** | 0.5177 | *** | -0.2073 | *** | -0.1951 | *** | -0.2199 | *** | |
Monocyte | CD14 | 0.3595 | *** | 0.3379 | *** | 0.4062 | *** | -0.1944 | *** | -0.2080 | *** | -0.1967 | *** |
Macrophage | CD68 | 0.4314 | *** | 0.4167 | *** | 0.4650 | *** | 0.0576 | 0.2415 | 0.0596 | 0.2473 | 0.0493 | 0.3221 |
M1 | NOS2 | -0.0084 | 0.8491 | -0.0129 | 0.7786 | -0.0245 | 0.5800 | 0.2512 | *** | 0.2618 | *** | 0.2277 | *** |
I5 | 0.3861 | *** | 0.3806 | *** | 0.4182 | *** | 0.0122 | 0.8042 | 0.0107 | 0.8351 | 0.0195 | 0.6961 | |
M2 | CD163 | 0.5021 | *** | 0.4900 | *** | 0.5125 | *** | -0.0821 | 0.0950 | -0.0853 | 0.0972 | -0.1056 | * |
VSIG4 | 0.2379 | *** | 0.2028 | *** | 0.2878 | *** | -0.1702 | *** | -0.1820 | *** | -0.1758 | *** | |
MS4A4A | 0.4084 | *** | 0.3912 | *** | 0.4383 | *** | -0.1663 | *** | -0.1762 | *** | -0.1705 | *** | |
Neutrophil | MPO | 0.0085 | 0.8481 | 0.0009 | 0.9841 | -0.0446 | 0.3129 | -0.1189 | * | -0.1039 | * | -0.1216 | * |
CCR7 | 0.3712 | *** | 0.3739 | *** | 0.3610 | *** | -0.1862 | *** | -0.1691 | *** | -0.1792 | *** | |
DC | CD1C | 0.3017 | *** | 0.2972 | *** | 0.3212 | *** | -0.1579 | ** | -0.1580 | ** | -0.1367 | ** |
*P < 0.05, **P < 0.01, ***P < 0.001. Tfh, follicular helper T cell; Th, T helper cell; Treg, regulatory T cell; TAM, tumor associated macrophage; NK, natural killer cell; DC, dendritic cell; None, correlation without adjustment; Purity, correlation adjusted for tumor purity; Cor, R value of Spearman's correlation. |
Cancer | TMB | MSI | ||
Correlation | P-value | Correlation | P-value | |
ACC | -0.142 | 0.21873 | 0.071 | 0.53964 |
BLCA | 0.027 | 0.59282 | -0.003 | 0.94940 |
BRCA | -0.073 | 0.02169 | -0.009 | 0.76597 |
CESC | 0.046 | 0.57506 | -0.026 | 0.65448 |
CHOL | -0.199 | 0.24307 | 0.242 | 0.15427 |
COAD | -0.293 | 2.48E-08 | 0.077 | 0.11088 |
DLBC | -0.295 | 0.07668 | -0.28 | 0.05424 |
ESCA | 0.367 | 3.82E-06 | 0.139 | 0.07896 |
GBM | 0.058 | 0.48026 | 0.037 | 0.65588 |
HNSC | 0.153 | 0.00070 | -0.015 | 0.73890 |
KICH | -0.251 | 0.04581 | 0.14 | 0.26538 |
KIRC | -0.033 | 0.53559 | 0.079 | 0.14706 |
KIRP | 0.128 | 0.03413 | 0.021 | 0.72721 |
LAML | -0.161 | 0.09980 | -0.051 | 0.59316 |
LGG | 0.343 | 9.75E-15 | -0.072 | 0.11118 |
LIHC | -0.141 | 0.01563 | 0.001 | 0.97814 |
LUAD | -0.173 | 9.99E-05 | 0.03 | 0.49973 |
LUSC | 0.007 | 0.88178 | -0.039 | 0.38478 |
MESO | -0.146 | 0.19437 | 0.01 | 0.93062 |
OV | -0.1 | 0.11796 | 0.142 | 0.01827 |
PAAD | 0.348 | 7.28E-06 | -0.046 | 0.54665 |
PCPG | 0.043 | 0.57813 | -0.1 | 0.18305 |
PRAD | -0.096 | 0.03472 | -0.023 | 0.60271 |
READ | -0.176 | 0.05380 | 0.195 | 0.01670 |
SARC | 0.064 | 0.34035 | -0.004 | 0.94713 |
SKCM | 0.032 | 0.48829 | 0.035 | 0.45072 |
STAD | 0.101 | 0.05260 | 0.136 | 0.00869 |
TGCT | 0.038 | 0.66762 | 0.28 | 0.00106 |
THCA | -0.019 | 0.68334 | -0.016 | 0.71447 |
THYM | 0.092 | 0.32419 | 0.012 | 0.89740 |
UCEC | -0.253 | 7.33E-09 | -0.2 | 3.04E-06 |
UCS | -0.272 | 0.04268 | -0.294 | 0.02812 |
UVM | -0.181 | 0.13749 | 0.06 | 0.62210 |
Cell type | Gene marker | LGG | STAD | ||||||||||
None | purity | age | none | purity | age | ||||||||
cor | p | cor | p | cor | p | cor | p | cor | p | cor | p | ||
B cell | CD19 | 0.3000 | *** | 0.2731 | *** | 0.3308 | *** | -0.2446 | *** | -0.2210 | *** | -0.2344 | *** |
CD38 | -0.1206 | ** | -0.1334 | ** | -0.0614 | 0.1650 | -0.1218 | * | -0.1125 | * | -0.1314 | ** | |
CD79A | 0.2047 | *** | 0.2286 | *** | 0.2361 | *** | -0.2327 | *** | -0.2031 | *** | -0.2319 | *** | |
CD8+Tcell | CD8A | 0.3461 | *** | 0.3538 | *** | 0.3323 | *** | -0.1703 | *** | -0.1571 | ** | -0.1673 | *** |
CD8B | 0.2227 | *** | 0.2247 | *** | 0.2090 | *** | -0.2275 | *** | -0.2373 | *** | -0.2213 | *** | |
T cell (general) | CD3D | 0.5183 | *** | 0.5214 | *** | 0.5079 | *** | -0.1984 | *** | -0.1814 | *** | -0.1983 | *** |
CD3E | 0.5515 | *** | 0.5604 | *** | 0.5402 | *** | -0.1893 | *** | -0.1697 | *** | -0.1884 | *** | |
CD2 | 0.5662 | *** | 0.5709 | *** | 0.5525 | *** | -0.1619 | *** | -0.1397 | ** | -0.1647 | *** | |
TAM | CD80 | 0.4756 | *** | 0.4671 | *** | 0.4877 | *** | 0.0169 | 0.7309 | 0.0371 | 0.4719 | -0.0046 | 0.9257 |
CD86 | 0.4007 | *** | 0.3920 | *** | 0.4453 | *** | -0.1517 | ** | -0.1464 | ** | -0.1633 | *** | |
CCL2 | 0.3665 | *** | 0.3644 | *** | 0.3858 | *** | -0.2900 | *** | -0.3056 | *** | -0.2811 | *** | |
IL10 | 0.3796 | *** | 0.3629 | *** | 0.4065 | *** | -0.0967 | * | -0.1058 | * | -0.1062 | * | |
Tfh | CXCR5 | 0.2636 | *** | 0.2575 | *** | 0.2734 | *** | -0.1894 | *** | -0.1612 | ** | -0.1727 | *** |
ICOS | 0.5196 | *** | 0.5134 | *** | 0.5120 | *** | -0.0699 | 0.1553 | -0.0359 | 0.4854 | -0.0851 | 0.0873 | |
BCL6 | -0.0110 | 0.8024 | -0.0276 | 0.5473 | 0.0273 | 0.5371 | -0.2195 | *** | -0.2198 | *** | -0.2121 | *** | |
Th1 | STAT1 | 0.4891 | *** | 0.4811 | *** | 0.4764 | *** | 0.0860 | 0.0801 | 0.1013 | * | 0.0809 | 0.1041 |
IFNG | 0.2944 | *** | 0.2919 | *** | 0.2905 | *** | -0.0324 | 0.5105 | -0.0079 | 0.8785 | -0.0478 | 0.3377 | |
Th2 | CCR3 | 0.3355 | *** | 0.3410 | *** | 0.3375 | *** | -0.0348 | 0.4800 | -0.0143 | 0.7809 | -0.0410 | 0.4111 |
GATA3 | 0.5002 | *** | 0.5044 | *** | 0.4711 | *** | -0.1644 | *** | -0.1553 | ** | -0.1607 | ** | |
STAT5A | 0.4410 | *** | 0.4281 | *** | 0.4601 | *** | 0.0104 | 0.8324 | 0.0189 | 0.7145 | 0.0034 | 0.9461 | |
Th9 | TGFBR2 | 0.4199 | *** | 0.4017 | *** | 0.4561 | *** | 0.0672 | 0.1718 | 0.0622 | 0.2273 | 0.0781 | 0.1168 |
IRF4 | 0.1375 | *** | 0.1175 | *** | 0.1855 | *** | -0.1395 | *** | -0.1135 | ** | -0.1550 | *** | |
Th17 | IL21R | 0.1629 | *** | 0.1686 | *** | 0.1717 | *** | -0.1651 | *** | -0.1387 | ** | -0.1696 | *** |
STAT3 | 0.4223 | *** | 0.4037 | *** | 0.4368 | *** | 0.0377 | 0.4438 | 0.0411 | 0.4253 | 0.0229 | 0.6456 | |
Th22 | CCR10 | 0.2110 | *** | 0.2097 | *** | 0.1851 | *** | -0.2561 | *** | -0.2566 | *** | -0.2481 | *** |
AHR | 0.3579 | *** | 0.3476 | *** | 0.4009 | *** | 0.3871 | *** | 0.3893 | *** | 0.3783 | *** | |
Treg | CCR8 | 0.2271 | *** | 0.2324 | *** | 0.2116 | *** | -0.0113 | 0.8190 | -0.0006 | 0.9914 | -0.0193 | 0.6987 |
STAT5B | -0.0385 | 0.3833 | -0.0326 | 0.4767 | -0.0150 | 0.7343 | 0.0207 | 0.6746 | 0.0162 | 0.7536 | 0.0218 | 0.6623 | |
TGFB1 | 0.3335 | *** | 0.3140 | *** | 0.3851 | *** | -0.1751 | *** | -0.1639 | ** | -0.1820 | *** | |
T cell (exhaustion) | CTLA4 | 0.3479 | *** | 0.3383 | *** | 0.3469 | *** | -0.1141 | * | -0.0915 | 0.0751 | -0.1315 | ** |
LAG3 | 0.2768 | *** | 0.2954 | *** | 0.2914 | *** | -0.1936 | *** | -0.1853 | *** | -0.2056 | *** | |
GZMB | 0.3564 | *** | 0.3813 | *** | 0.3255 | *** | -0.1001 | * | -0.0799 | 0.1206 | -0.1170 | * | |
NK | XCL1 | 0.4235 | *** | 0.4168 | *** | 0.4331 | *** | -0.2295 | *** | -0.2335 | *** | -0.2277 | *** |
KIR3DL1 | 0.1350 | *** | 0.1403 | *** | 0.1393 | *** | -0.1605 | *** | -0.1570 | *** | -0.1715 | *** | |
CD7 | 0.5055 | *** | 0.5146 | *** | 0.5177 | *** | -0.2073 | *** | -0.1951 | *** | -0.2199 | *** | |
Monocyte | CD14 | 0.3595 | *** | 0.3379 | *** | 0.4062 | *** | -0.1944 | *** | -0.2080 | *** | -0.1967 | *** |
Macrophage | CD68 | 0.4314 | *** | 0.4167 | *** | 0.4650 | *** | 0.0576 | 0.2415 | 0.0596 | 0.2473 | 0.0493 | 0.3221 |
M1 | NOS2 | -0.0084 | 0.8491 | -0.0129 | 0.7786 | -0.0245 | 0.5800 | 0.2512 | *** | 0.2618 | *** | 0.2277 | *** |
I5 | 0.3861 | *** | 0.3806 | *** | 0.4182 | *** | 0.0122 | 0.8042 | 0.0107 | 0.8351 | 0.0195 | 0.6961 | |
M2 | CD163 | 0.5021 | *** | 0.4900 | *** | 0.5125 | *** | -0.0821 | 0.0950 | -0.0853 | 0.0972 | -0.1056 | * |
VSIG4 | 0.2379 | *** | 0.2028 | *** | 0.2878 | *** | -0.1702 | *** | -0.1820 | *** | -0.1758 | *** | |
MS4A4A | 0.4084 | *** | 0.3912 | *** | 0.4383 | *** | -0.1663 | *** | -0.1762 | *** | -0.1705 | *** | |
Neutrophil | MPO | 0.0085 | 0.8481 | 0.0009 | 0.9841 | -0.0446 | 0.3129 | -0.1189 | * | -0.1039 | * | -0.1216 | * |
CCR7 | 0.3712 | *** | 0.3739 | *** | 0.3610 | *** | -0.1862 | *** | -0.1691 | *** | -0.1792 | *** | |
DC | CD1C | 0.3017 | *** | 0.2972 | *** | 0.3212 | *** | -0.1579 | ** | -0.1580 | ** | -0.1367 | ** |
*P < 0.05, **P < 0.01, ***P < 0.001. Tfh, follicular helper T cell; Th, T helper cell; Treg, regulatory T cell; TAM, tumor associated macrophage; NK, natural killer cell; DC, dendritic cell; None, correlation without adjustment; Purity, correlation adjusted for tumor purity; Cor, R value of Spearman's correlation. |