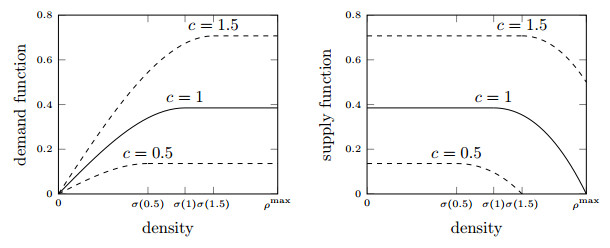
In this paper, we illustrate how second order traffic flow models, in our case the Aw-Rascle equations, can be used to reproduce empirical observations such as the capacity drop at merges and solve related optimal control problems. To this aim, we propose a model for on-ramp junctions and derive suitable coupling conditions. These are associated to the first order Godunov scheme to numerically study the well-known capacity drop effect, where the outflow of the system is significantly below the expected maximum. Control issues such as speed and ramp meter control are also addressed in a first-discretize-then-optimize framework.
Citation: Oliver Kolb, Simone Göttlich, Paola Goatin. Capacity drop and traffic control for a second order traffic model[J]. Networks and Heterogeneous Media, 2017, 12(4): 663-681. doi: 10.3934/nhm.2017027
[1] | Oliver Kolb, Simone Göttlich, Paola Goatin . Capacity drop and traffic control for a second order traffic model. Networks and Heterogeneous Media, 2017, 12(4): 663-681. doi: 10.3934/nhm.2017027 |
[2] | Mohamed Benyahia, Massimiliano D. Rosini . A macroscopic traffic model with phase transitions and local point constraints on the flow. Networks and Heterogeneous Media, 2017, 12(2): 297-317. doi: 10.3934/nhm.2017013 |
[3] | Benjamin Seibold, Morris R. Flynn, Aslan R. Kasimov, Rodolfo R. Rosales . Constructing set-valued fundamental diagrams from Jamiton solutions in second order traffic models. Networks and Heterogeneous Media, 2013, 8(3): 745-772. doi: 10.3934/nhm.2013.8.745 |
[4] | Paola Goatin, Chiara Daini, Maria Laura Delle Monache, Antonella Ferrara . Interacting moving bottlenecks in traffic flow. Networks and Heterogeneous Media, 2023, 18(2): 930-945. doi: 10.3934/nhm.2023040 |
[5] | Bertrand Haut, Georges Bastin . A second order model of road junctions in fluid models of traffic networks. Networks and Heterogeneous Media, 2007, 2(2): 227-253. doi: 10.3934/nhm.2007.2.227 |
[6] | Caterina Balzotti, Maya Briani, Benedetto Piccoli . Emissions minimization on road networks via Generic Second Order Models. Networks and Heterogeneous Media, 2023, 18(2): 694-722. doi: 10.3934/nhm.2023030 |
[7] | Tong Li . Qualitative analysis of some PDE models of traffic flow. Networks and Heterogeneous Media, 2013, 8(3): 773-781. doi: 10.3934/nhm.2013.8.773 |
[8] | Michael Herty, J.-P. Lebacque, S. Moutari . A novel model for intersections of vehicular traffic flow. Networks and Heterogeneous Media, 2009, 4(4): 813-826. doi: 10.3934/nhm.2009.4.813 |
[9] | Edward S. Canepa, Alexandre M. Bayen, Christian G. Claudel . Spoofing cyber attack detection in probe-based traffic monitoring systems using mixed integer linear programming. Networks and Heterogeneous Media, 2013, 8(3): 783-802. doi: 10.3934/nhm.2013.8.783 |
[10] | Felisia Angela Chiarello, Harold Deivi Contreras, Luis Miguel Villada . Nonlocal reaction traffic flow model with on-off ramps. Networks and Heterogeneous Media, 2022, 17(2): 203-226. doi: 10.3934/nhm.2022003 |
In this paper, we illustrate how second order traffic flow models, in our case the Aw-Rascle equations, can be used to reproduce empirical observations such as the capacity drop at merges and solve related optimal control problems. To this aim, we propose a model for on-ramp junctions and derive suitable coupling conditions. These are associated to the first order Godunov scheme to numerically study the well-known capacity drop effect, where the outflow of the system is significantly below the expected maximum. Control issues such as speed and ramp meter control are also addressed in a first-discretize-then-optimize framework.
The description of vehicular traffic flow based on systems of conservation or balance laws has been proposed by many researchers during the last decades, see for example [9,30] and the references therein. In contrast to first order traffic models consisting only of one scalar equation for the traffic density, second order models are characterized by a second equation for the evolution of traffic mean velocity. Among these, Aw and Rascle [2] proposed a model that overcomes the drawbacks of previous models, and is capable to capture some traffic characteristics linked to the anticipation behavior of drivers. Greenberg [12] presented an extension of the Aw-Rascle model including a relaxation term towards a preferred (or equilibrium) velocity. Since then many contributions have appeared on theoretical and numerical investigations [1,3,4,10], networked problems [8,13,15,16,26], and more recently phenomenological and data driven approaches [7,17,21,24,29].
In this work, we present results concerning the coupling of interlinked road networks to on-ramps. It is known that in such situations capacity drops occur for high traffic densities, see e.g. [13,17,21,23,29]. Capacity drop means that the measured outflow of the system is smaller than the expected maximal flow if the system is well utilized. The difference might be up to 10% or even higher [17,29]. This can be explained by a less efficient driving style when exiting a congested zone (upstream the on-ramp), which reduces the downstream flow compared to free-flow conditions [30].
The coupling of on-ramps to roads is similar to the modeling of a merge [18]. However, in contrast to the majority of already existing approaches, here we assume that the on-ramp is given by an ordinary differential equation, see [6,11]. For second order models, the challenge here is to find appropriate conditions to ensure the conservation of mass and momentum flow. Once these coupling conditions are defined, they can be integrated in a finite-volume type numerical scheme to compute the evolution of traffic conditions on the network. We use a first-order Godunov scheme combined with a time splitting to resolve the relaxation term. In particular, we observe that the model is able to reproduce the capacity drop effect for increasing inflows, similar to the study made in [13] and in contrast to [23], where the phenomenon is only observed for a short time period.
Furthermore, our approach gives the opportunity to consider questions of optimal control in a first-discretize-then-optimize framework. Typical control issues arising in the on-ramp context are speed limit and ramp metering. These kind of traffic flow control problems have been mainly studied in the context of first-order models based on the Lighthill-Whitham-Richards (LWR) equations [22], see e.g. [5,11,14,25]. To the best of our knowledge, speed limit and ramp meter control has been not considered for the Aw-Rascle model so far. We discuss the numerical results obtained from the adjoint calculus and compare them to the LWR equations.
The outline is as follows: In Section 2 we present the Aw-Rascle model with relaxation term and the coupling conditions at on-ramps. Our discretization scheme will be introduced in Section 3. Numerical results concerning the capacity drop and optimal control issues can be found in Section 4. Comparisons to the solution of the LWR model are also given for all experiments.
We introduce the modeling equations given by the Aw-Rascle (AR) model [2] including a relaxation term as originally introduced in [12]. The model consists of a
In this work, our focus is on the coupling of the Aw-Rascle model to on-ramps whose dynamics are modeled by ordinary differential equations. We will derive appropriate coupling conditions that allow for the definition of boundary conditions at junctions. As we will see in the numerical experiments, our modeling approach covers the capacity drop effect and allows to solve optimal control problems such as speed limit and ramp metering, cf. Section 4.3.
We briefly recall the Aw-Rascle traffic flow model [2] and explain how it can be extended to the context of networks. Traffic states are described by the density
Given some initial state
∂tρi+∂x(ρivi)=0, | (1) |
∂t(ρiwi)+∂x(ρiviwi)=−ρivi−Vi(ρi)δ, | (2) |
wi=vi+pi(ρi), | (3) |
or in conservative form with
∂t(ρiyi)+∂x(yi−ρipi(ρi)(yi−ρipi(ρi))yiρi)=(0−(yi−ρipi(ρi))−ρiVi(ρi)δ)⏟=gi(ρi,yi), | (4) |
where
We consider the preferential velocity depending on the density,
Vi(ρ)=vmaxi(1−ρρmaxi), | (5) |
and the pressure function (as in [13,23])
pi(ρ)=vrefiγi(ρρmaxi)γi | (6) |
equipped with maximal density
Note that above and in the following, we use
Di(ρ,c,t)={(c−pi(ρ,t))ρif ρ≤σi(c,t),(c−pi(σi(c,t),t))σi(c,t)if ρ≥σi(c,t), | (7) |
Si(ρ,c,t)={(c−pi(σi(c,t),t))σi(c,t)if ρ≤σi(c,t),(c−pi(ρ,t))ρif ρ≥σi(c,t), | (8) |
where
σi(c,t)=ρmaxi(cγivrefi(t)(1+γi))1γi | (9) |
is the sonic point on the curve
In the following, we present the serial coupling between single roads as well as the coupling with an on-ramp. In general, for well-posed coupling conditions in networks of hyperbolic conservation laws, one considers (half-)Riemann problems defining the set of admissible states for each road at the junction, i.e., states such that all waves produced by the Riemann problem have negative/positive speed if the considered road is an incoming/outgoing road (cf. [13]). Under these constraints, the flow at the junction is maximized, where additional conditions have to be posed to achieve uniqueness of the solution. Those conditions as well as the flow maximization are already included in the following description.
The considered coupling and boundary conditions can be given in terms of mass flow
1-to-1 junction. We use index 1 for the ingoing road, index 2 for the outgoing road, and consider the data
Flow maximization at the junction over all admissible states leads to
q1=q2=˜q=min{D1(ρ1,w1,t),S2(˜ρ2,w1,t)}, | (10) |
where
{v2(U,t)=v2(U2,t)}and{w2(U,t)=w1}, | (11) |
or
˜ρ2=ρmax2(max(γ2vref2(t)(w1−v2(U2,t)),0))1γ2. | (12) |
Then,
On-ramp at a 1-to-1 junction. We consider a 1-to-1 junction with an on-ramp. Here, we use index 1 for the ingoing road, index 2 for the on-ramp, index 3 for the outgoing road, and consider the data
For the demand
D2(l,t)=u2(t){fmax2if l>0,min{fin2(t),fmax2}if l=0, | (13) |
where
To get a unique solution, we assign the priority parameter
q1=min{D1(ρ1,w1,t),max{PS3(˜ρ3,w1,t),S3(˜ρ3,w1,t)−D2(l2,t)}}, | (14) |
q2=min{D2(l2,t),max{(1−P)S3(˜ρ3,w1,t),S3(˜ρ3,w1,t)−D1(ρ1,w1,t)}}, | (15) |
q3=q1+q2, | (16) |
where
{v3(U,t)=v3(U3,t)}and{w3(U,t)=w1}, | (17) |
or
Again, the density
˜ρ3=ρmax3(max(γ3vref3(t)(w1−v3(U3,t)),0))1γ3. | (18) |
As before, the momentum flow is computed by multiplication of
The evolution of the queue at the on-ramp is given by
dl2(t)dt=fin2(t)−q2, | (19) |
where we typically start with empty queues, i.e.,
On-ramp at origin. We consider an on-ramp at a vertex with only one outgoing road. We use index 1 for the on-ramp with queue length
Similar to above we apply
D1(l,t)=u1(t){fmax1if l>0,min{fin1(t),fmax1}if l=0 | (20) |
for the demand at the on-ramp, where
In this case there is no value for
U1=(ρ,ρ˜w) such that ρV2(ρ,t)=D1(l,t), | (21) |
where
ρ±=ρmax22±√(ρmax2)24−ρmax2D1(l,t)vmax2(t). | (22) |
Here we choose
D2(ρ−,˜w,t)=D1(l,t). | (23) |
Finally, we apply
q1=q2=˜q=min{D1(l1,t),S2(˜ρ2,˜w,t)} | (24) |
with
{v2(U,t)=v2(U2,t)}and{w2(U,t)=˜w}, | (25) |
or
Similar to above, the evolution of the queue at the on-ramp is given by
dl1(t)dt=fin1(t)−q1. | (26) |
Outflow conditions. At nodes with only one ingoing road (with index 1) we consider absorbing boundary conditions up to a given maximum flow rate
q1=min{D1(ρ1,w1,t),fout1(t)}. | (27) |
The momentum flow is given by
For the numerical solution of the described model, a finite number of time points
The underlying balance law (4) is discretized with a fractional step method: We use a first order Godunov scheme for the flux term and an implicit Euler method for the relaxation term. Due to the fundamental idea of the Godunov scheme, the computation of the actual states at junctions is not necessary and the coupling will be given in terms of fluxes only.Note that in the following
On each road
U0i,j−0.5=1ΔxijΔxi∫(j−1)ΔxiUi(x,0)dx. | (28) |
Then, for
˜Un+1i,j−0.5=Uni,j−0.5−ΔtΔxi(Fni,j−Fni,j−1),(transport) | (29) |
Un+1i,j−0.5=˜Un+1i,j−0.5+Δtg(Un+1i,j−0.5).(relaxation term) | (30) |
The flux terms
Fni,j=(qni,jwni,j−0.5qni,j) | (31) |
with
qni,j=min(Di(ρni,j−0.5,wni,j−0.5,tn),Si(˜ρni,j,wni,j−0.5,tn)), | (32) |
where
{vi(U,tn)=vni,j+0.5}and{wi(U,tn)=wni,j−0.5}, | (33) |
or
We complete the numerical scheme by the description of the coupling and boundary conditions according to the model equations from Section 2.2. As already mentioned, we only need the coupling fluxes here and not the actual states at the junctions.
1-to-1 junction. As before, we use index 1 for the ingoing road and index 2 for the outgoing road. According to (10) we apply for
˜qn=min{D1(ρn1,Nx1−0.5,wn1,Nx1−0.5,tn),S2(˜ρn2,0,wn1,Nx1−0.5,tn)}, | (34) |
where
{v2(U,tn)=vn2,0.5}and{w2(U,tn)=wn1,Nx1−0.5}, | (35) |
or
Fn1,Nx1=Fn2,0=(˜qnwn1,Nx1−0.5˜qn). | (36) |
On-ramp at a 1-to-1 junction. We use index 1 for the ingoing road, index 2 for the on-ramp and index 3 for the outgoing road. According to (13) we apply
˜D2(l,t)=u2(t)min{fin2(t)+lΔt,fmax2} | (37) |
for the demand at the on-ramp. Then, using the abbreviations
dn1=D1(ρn1,Nx1−0.5,wn1,Nx1−0.5,tn), | (38) |
dn2=˜D2(ln2,tn), | (39) |
sn3=S3(˜ρn3,0,wn1,Nx1−0.5,tn), | (40) |
we get from (14) and (15)
qn1=min{d1,max{Ps3,s3−d2}}, | (41) |
qn2=min{d2,max{(1−P)s3,s3−d1}}, | (42) |
qn3=qn1+qn2, | (43) |
where
{v3(U,tn)=vn3,0.5}and{w3(U,tn)=wn1,Nx1−0.5}, | (44) |
or
Fn1,Nx1=(qn1wn1,Nx1−0.5qn1),Fn3,0=(qn3wn1,Nx1−0.5qn3) | (45) |
and apply
ln+12=ln2+Δt(fin2(tn)−qn2) | (46) |
for the update of the queue length via the explicit Euler scheme. Note that we always achieve a non-negative queue length by our choice of the demand function (37). Alternatively, the time step
On-ramp at origin. We use index 1 for the on-ramp and index 2 for the road. Similar to above we apply
˜D1(l,t)=u1(t)min{fin1(t)+lΔt,fmax1} | (47) |
for the demand at the on-ramp. Next, following the idea introduced in Section 2.2, we compute
ρn1,−=ρmax22−√(ρmax2)24−ρmax2˜D1(ln1,tn)vmax2(tn) | (48) |
and
˜qn=min{˜D1(ln1,tn),S2(˜ρn2,0,˜wn,tn)} | (49) |
with
{v2(U,t)=vn2,0.5}and{w2(U,tn)=˜wn}, | (50) |
or
Fn2,0=(˜qn˜wn˜qn) | (51) |
and apply
ln+11=ln1+Δt(fin1(tn)−˜qn) | (52) |
for the update of the queue length. Again, non-negativity of the queue length is ensured by our choice of the demand function (47).
Outflow conditions. At nodes with only one ingoing road (with index 1) we apply according to (27)
Fn1,Nx1=(qn1wn1,Nx1−0.5qn1) | (53) |
with
qn1=min{D1(ρn1,Nx1−0.5,wn1,Nx1−0.5,tn),fout1(tn)}. | (54) |
In the next section, we present a numerical study to show the characteristics of our modeling approach. We also comment on already existing examples from the literature, pointing out differences and similarities.
Our numerical results emphasize on three different scenarios that our approach is able to deal with from a modeling and computational point of view. First, we show that, for a single road, the Aw-Rascle (AR) model with relaxation term numerically converges to the Lighthill-Whitham-Richards (LWR) model as expected [12]. The second example considers the capacity drop for a 1-to-1 junction with on-ramp. We will see that this phenomenon is not covered by the classical LWR model. The third example tackles speed control and coordinated ramp metering control issues. It will turn out that the control strategies considered individually do not lead to the best possible travel times, while the combination of both lead to satisfying results.
We consider a single road as depicted in Figure 6 with length
We consider a simulation time of
fin(t)={4000+800cos(4πtT)for 0≤t≤T2,4800otherwise |
as inflow profile at the origin ''in'' (in
Figure 7 shows density and velocity profiles on the whole road after 36 seconds. As expected, the solution of the Aw-Rascle model with relaxation term tends towards the LWR model for decreasing relaxation parameter
Now we turn our attention to study the capacity drop effect, cf. [13]. We will see that increasing the inflow into the network depicted in Figure 8 will at a certain point lead to a decreasing outflow. Thus, a significant difference between the effective and maximal possible outflow occurs in the Aw-Rascle model. Conversely, this effect is not captured by the classical LWR model. This is due to the different modeling of the supply function. In the congested regime, the value of the supply function is at its maximum in the LWR model and therefore no capacity drop occurs. However, in the AR model, the supply function additionally depends on the ''incoming'' value of
We consider two roads of 1 km length with an on-ramp in between, see Figure 8. On both roads we use
Table 1 and Figure 9 show the simulation results for the AR and the LWR model (with
inflow at on-ramp in | outflow AR in | outflow LWR in | ||||
desired | actual | |||||
500 | 500 | 47.6 | 73.6 | 77.1 | 4000 | 4000 |
1000 | 1000 | 47.6 | 73.6 | 77.1 | 4500 | 4500 |
1500 | 1500 | 156.4 | 13.1 | 50.9 | 3554 | 4500 |
2000 | 1764 | 160.2 | 11.0 | 50.6 | 3527 | 4500 |
2500 | 1764 | 160.2 | 11.0 | 50.6 | 3527 | 4500 |
1000 | 1000 | 148.0 | 17.8 | 51.6 | 3629 | 4500 |
500 | 500 | 137.2 | 23.8 | 52.8 | 3762 | 4000 |
We begin with the interpretation of the results obtained from the AR model: Obviously, up to an inflow of
Interestingly, even when the desired inflow at the on-ramp is lowered again, the original state for the same values of
Note that compared to the existing literature the capacity drop is investigated for different situations. In [13] no relaxation term is considered but a similar result is obtained.
The construction principle for the capacity drop is the same (decreasing value of
road | length | initial density | |||
road1 | 2 | 180 | 100 | 100 | 50 |
road2 | 1 | 180 | 50 | 100 | 50 |
road3 | 1 | 180 | 50 | 100 | 50 |
road4 | 2 | 180 | 100 | 100 | 50 |
AR, | AR, | LWR | |
no control | 1871.7 | 1871.7 | 834.9 |
ramp metering only | 1325.3 | 1325.3 | 834.9 |
speed control only | 1122.8 | 872.6 | 834.9 |
both control types | 814.5 | 818.4 | 834.9 |
Finally, considering the same scenario with the LWR model (see [11] for the details), one observes that the outflow increases with the accumulated desired inflows until it reaches the maximum capacity of
For optimization purposes, we are finally interested in controlling maximal speed limits as well as on-ramp inflows to minimize the total travel time. As already introduced in equations (13) and (20), the ramp metering rate
1.
2.
For our investigations, we consider the road network depicted in Figure 10 (adapted from [14]) with the road parameters of Table 2. The exponents in the pressure function are
We consider a time horizon of
∑roads iT∫0Li∫0ρi(x,t)dxdt + ∑on-ramps jT∫0lj(t)dt, | (55) |
given an upper bound of 100 vehicles in the queue of the on-ramp. To solve this optimization task, we apply a first-discretize-then-optimize approach and adjoint calculus, see also [11]. Thus, for given control decisions (speed limits and metering rates), the discretization scheme described in Section 3 is always used to evaluate the objective function (55) (using the trapezoidal rule for quadrature). Further, sensitivity/gradient information with respect to the control decisions is computed based on the same discretization (we refer to [19,20] for more details). Finally, the SQP solver DONLP2 [27,28] is used for the optimization of the control decisions. The applied discretization parameters are
Table 3 shows the total travel times for the different models with and without optimization. The resulting queues for the AR model with scaling of the pressure function (
Note that the results for the model without scaling of the pressure function (
Figure 13 exemplarily shows where the improvement in total travel time comes from in the case of coordinated ramp metering and speed control in the model with scaling of the pressure function: The outflow of the system (plot on the right) in the optimized scenario is permanently above the outflow of the uncontrolled system until it drops to the low inflow level after two hours. Figure 14 shows the applied (optimal) controls.
As shown in Table 3, the uncontrolled case is already optimal for the LWR model, i.e., no ramp metering or speed control is required. Accordingly, we take a look at the influence of the relaxation parameter
Table 4 shows the simulation and optimization results for a wide choice of
no control | opt. control, | opt. control, | |
2199.4 | 868.8 | 953.4 | |
2137.1 | 860.1 | 856.3 | |
1871.7 | 814.5 | 818.4 | |
731.4 | 731.4 | 731.4 | |
725.9 | 725.9 | 725.9 |
First, one observes larger total travel times in the case of smaller values for
In this work, we have set up coupling conditions for the Aw-Rascle model linked to on-ramps described by ordinary differential equations. Applying a first order Godunov scheme, the presented approach allows for numerical investigations of the capacity drop effect and optimal control problems.
Future work will include extensions to on-ramps that are described by first order traffic models such as the LWR model. We will derive appropriate coupling conditions and focus on theoretical properties.
[1] |
Derivation of continuum traffic flow models from microscopic follow-the-leader models. SIAM Journal on Applied Mathematics (2002) 63: 259-278. ![]() |
[2] |
Resurrection of ''second order" models of traffic flow. SIAM Journal on Applied Mathematics (2000) 60: 916-938. ![]() |
[3] |
A model for the formation and evolution of traffic jams. Archive for Rational Mechanics and Analysis (2008) 187: 185-220. ![]() |
[4] |
Transport-equilibrium schemes for computing contact discontinuities in traffic flow modeling. Communications in Mathematical Sciences (2007) 5: 533-551. ![]() |
[5] |
Traffic Regulation via Controlled Speed Limit. SIAM Journal on Control and Optimization (2017) 55: 2936-2958. ![]() |
[6] |
A PDE-ODE model for a junction with ramp buffer. SIAM Journal on Applied Mathematics (2014) 74: 22-39. ![]() |
[7] |
Comparative model accuracy of a data-fitted generalized Aw-Rascle-Zhang model. Networks and Heterogeneous Media (2014) 9: 239-268. ![]() |
[8] |
Traffic flow on a road network using the Aw-Rascle model. Communications in Partial Differential Equations (2006) 31: 243-275. ![]() |
[9] | M. Garavello and B. Piccoli, Traffic Flow on Networks, Springfield, MO: American Institute of Mathematical Sciences (AIMS), 2006. |
[10] |
The Aw-Rascle vehicular traffic flow model with phase transitions. Mathematical and Computer Modelling (2006) 44: 287-303. ![]() |
[11] |
Speed limit and ramp meter control for traffic flow networks. Engineering Optimization (2016) 48: 1121-1144. ![]() |
[12] |
Extensions and amplifications of a traffic model of Aw and Rascle. SIAM Journal on Applied Mathematics (2001) 62: 729-745. ![]() |
[13] |
A second order model of road junctions in fluid models of traffic networks. Networks and Heterogeneous Media (2007) 2: 227-253. ![]() |
[14] |
Optimal coordination of variable speed limits to suppress shock waves. IEEE Transactions on Intelligent Transportation Systems (2005) 6: 102-112. ![]() |
[15] |
Optimization criteria for modelling intersections of vehicular traffic flow. Networks and Heterogeneous Media (2006) 1: 275-294. ![]() |
[16] |
Coupling conditions for a class of second-order models for traffic flow. SIAM Journal on Mathematical Analysis (2006) 38: 595-616. ![]() |
[17] |
A kinematic wave theory of capacity drop. Transportation Research Part B: Methodological (2015) 81: 316-329. ![]() |
[18] |
On the distribution schemes for determining flows through a merge. Transportation Research Part B: Methodological (2003) 37: 521-540. ![]() |
[19] | O. Kolb, Simulation and Optimization of Gas and Water Supply Networks, PhD thesis, TU Darmstadt, 2011. |
[20] |
O. Kolb and J. Lang, Simulation and continuous optimization, in "Mathematical Optimization of Water Networks" (eds. A. Martin, K. Klamroth, J. Lang, G. Leugering, A. Morsi, M. Oberlack, M. Ostrowski and R. Rosen), Springer Basel, 162 (2012), 17-33. doi: 10.1007/978-3-0348-0436-3_2
![]() |
[21] |
Capacity drops at merges: New analytical investigations. Transportation Research Part C: Emerging Technologies (2016) 62: 171-181. ![]() |
[22] |
On kinematic waves. Ⅱ. A theory of traffic flow on long crowded roads. Royal Society of London Proceedings Series A (1955) 229: 317-345. ![]() |
[23] |
Second-order model and capacity drop at merge. Transportation Research Record: Journal of the Transportation Research Board (2012) 2315: 25-34. ![]() |
[24] |
Second-order models and traffic data from mobile sensors. Transportation Research Part C: Emerging Technologies (2015) 52: 32-56. ![]() |
[25] |
Adjoint-based optimization on a network of discretized scalar conservation laws with applications to coordinated ramp metering. Journal of Optimization Theory and Applications (2015) 167: 733-760. ![]() |
[26] |
Balanced vehicular traffic at a bottleneck. Mathematical and Computer Modelling (2009) 49: 689-702. ![]() |
[27] |
A new technique for inconsistent QP problems in the SQP method. Mathematical Methods of Operations Research (1998) 47: 355-400. ![]() |
[28] |
An SQP method for general nonlinear programs using only equality constrained subproblems. Mathematical Programming (1998) 82: 413-448. ![]() |
[29] |
Empirical observations of capacity drop in freeway merges with ramp control and integration in a first-order model. Transportation Research Part C: Emerging Technologies (2013) 30: 161-177. ![]() |
[30] |
M. Treiber and A. Kesting, Traffic Flow Dynamics, Data, models and simulation, Translated by Treiber and Christian Thiemann, Springer, Heidelberg, 2013. doi: 10.1007/978-3-642-32460-4
![]() |
1. | Felisia A. Chiarello, Jan Friedrich, Paola Goatin, Simone Göttlich, Micro-Macro Limit of a Nonlocal Generalized Aw-Rascle Type Model, 2020, 80, 0036-1399, 1841, 10.1137/20M1313337 | |
2. | Giulia Piacentini, Paola Goatin, Antonella Ferrara, Traffic control via moving bottleneck of coordinated vehicles, 2018, 51, 24058963, 13, 10.1016/j.ifacol.2018.07.003 | |
3. | Nikolaos Bekiaris-Liberis, Argiris I. Delis, PDE-Based Feedback Control of Freeway Traffic Flow via Time-Gap Manipulation of ACC-Equipped Vehicles, 2021, 29, 1063-6536, 461, 10.1109/TCST.2020.2974148 | |
4. | Silvia Siri, Cecilia Pasquale, Simona Sacone, Antonella Ferrara, Freeway traffic control: A survey, 2021, 130, 00051098, 109655, 10.1016/j.automatica.2021.109655 | |
5. | Simone Göttlich, Michael Herty, Salissou Moutari, Jennifer Weissen, Second-Order Traffic Flow Models on Networks, 2021, 81, 0036-1399, 258, 10.1137/20M1339908 | |
6. | Alexandre Bayen, Maria Laura Delle Monache, Mauro Garavello, Paola Goatin, Benedetto Piccoli, 2022, Chapter 4, 978-3-030-93014-1, 73, 10.1007/978-3-030-93015-8_4 | |
7. | M. L. Delle Monache, C. Pasquale, M. Barreau, R. Stern, 2022, New frontiers of freeway traffic control and estimation, 978-1-6654-6761-2, 6910, 10.1109/CDC51059.2022.9993221 | |
8. | Jan Friedrich, Simone Göttlich, Maximilian Osztfalk, Network models for nonlocal traffic flow, 2022, 56, 2822-7840, 213, 10.1051/m2an/2022002 | |
9. | Joshua Buli, Yulong Xing, A discontinuous Galerkin method for the Aw-Rascle traffic flow model on networks, 2020, 406, 00219991, 109183, 10.1016/j.jcp.2019.109183 | |
10. | Giacomo Dimarco, Andrea Tosin, Mattia Zanella, Kinetic Derivation of Aw–Rascle–Zhang-Type Traffic Models with Driver-Assist Vehicles, 2022, 186, 0022-4715, 10.1007/s10955-021-02862-7 | |
11. | Jie Qi, Shurong Mo, Miroslav Krstic, Delay-Compensated Distributed PDE Control of Traffic With Connected/Automated Vehicles, 2023, 68, 0018-9286, 2229, 10.1109/TAC.2022.3174032 | |
12. | Oliver Kolb, Guillaume Costeseque, Paola Goatin, Simone Göttlich, Pareto-Optimal Coupling Conditions for the Aw--Rascle--Zhang Traffic Flow Model at Junctions, 2018, 78, 0036-1399, 1981, 10.1137/17M1136900 | |
13. | Jennifer Weissen, Oliver Kolb, Simone Göttlich, A combined first and second order model for a junction with ramp buffer, 2022, 8, 2426-8399, 349, 10.5802/smai-jcm.90 | |
14. | Huan Yu, Miroslav Krstic, 2022, Chapter 1, 978-3-031-19345-3, 1, 10.1007/978-3-031-19346-0_1 | |
15. | Shurong Mo, Nailong Wu, Jie Qi, Anqi Pan, Zhiguang Feng, Huaicheng Yan, Yueying Wang, Proximal policy optimization learning based control of congested freeway traffic, 2023, 0143-2087, 10.1002/oca.3068 | |
16. | Michael Herty, Niklas Kolbe, Data‐driven models for traffic flow at junctions, 2024, 0170-4214, 10.1002/mma.10053 | |
17. | Wei Chen, Shumo Cui, Kailiang Wu, Tao Xiong, Bound-Preserving OEDG Schemes for Aw–Rascle–Zhang Traffic Models on Networks, 2024, 00219991, 113507, 10.1016/j.jcp.2024.113507 |
inflow at on-ramp in | outflow AR in | outflow LWR in | ||||
desired | actual | |||||
500 | 500 | 47.6 | 73.6 | 77.1 | 4000 | 4000 |
1000 | 1000 | 47.6 | 73.6 | 77.1 | 4500 | 4500 |
1500 | 1500 | 156.4 | 13.1 | 50.9 | 3554 | 4500 |
2000 | 1764 | 160.2 | 11.0 | 50.6 | 3527 | 4500 |
2500 | 1764 | 160.2 | 11.0 | 50.6 | 3527 | 4500 |
1000 | 1000 | 148.0 | 17.8 | 51.6 | 3629 | 4500 |
500 | 500 | 137.2 | 23.8 | 52.8 | 3762 | 4000 |
road | length | initial density | |||
road1 | 2 | 180 | 100 | 100 | 50 |
road2 | 1 | 180 | 50 | 100 | 50 |
road3 | 1 | 180 | 50 | 100 | 50 |
road4 | 2 | 180 | 100 | 100 | 50 |
AR, | AR, | LWR | |
no control | 1871.7 | 1871.7 | 834.9 |
ramp metering only | 1325.3 | 1325.3 | 834.9 |
speed control only | 1122.8 | 872.6 | 834.9 |
both control types | 814.5 | 818.4 | 834.9 |
no control | opt. control, | opt. control, | |
2199.4 | 868.8 | 953.4 | |
2137.1 | 860.1 | 856.3 | |
1871.7 | 814.5 | 818.4 | |
731.4 | 731.4 | 731.4 | |
725.9 | 725.9 | 725.9 |
inflow at on-ramp in | outflow AR in | outflow LWR in | ||||
desired | actual | |||||
500 | 500 | 47.6 | 73.6 | 77.1 | 4000 | 4000 |
1000 | 1000 | 47.6 | 73.6 | 77.1 | 4500 | 4500 |
1500 | 1500 | 156.4 | 13.1 | 50.9 | 3554 | 4500 |
2000 | 1764 | 160.2 | 11.0 | 50.6 | 3527 | 4500 |
2500 | 1764 | 160.2 | 11.0 | 50.6 | 3527 | 4500 |
1000 | 1000 | 148.0 | 17.8 | 51.6 | 3629 | 4500 |
500 | 500 | 137.2 | 23.8 | 52.8 | 3762 | 4000 |
road | length | initial density | |||
road1 | 2 | 180 | 100 | 100 | 50 |
road2 | 1 | 180 | 50 | 100 | 50 |
road3 | 1 | 180 | 50 | 100 | 50 |
road4 | 2 | 180 | 100 | 100 | 50 |
AR, | AR, | LWR | |
no control | 1871.7 | 1871.7 | 834.9 |
ramp metering only | 1325.3 | 1325.3 | 834.9 |
speed control only | 1122.8 | 872.6 | 834.9 |
both control types | 814.5 | 818.4 | 834.9 |
no control | opt. control, | opt. control, | |
2199.4 | 868.8 | 953.4 | |
2137.1 | 860.1 | 856.3 | |
1871.7 | 814.5 | 818.4 | |
731.4 | 731.4 | 731.4 | |
725.9 | 725.9 | 725.9 |