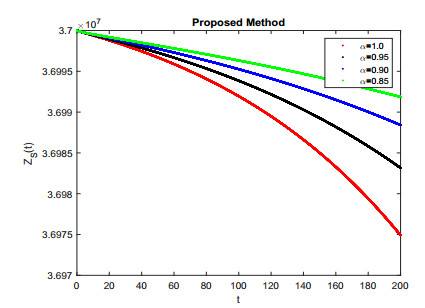
The SARS-CoV-2 virus pandemic remains a pressing issue with its unpredictable nature, and it spreads worldwide through human interaction. Current research focuses on the investigation and analysis of fractional epidemic models that discuss the temporal dynamics of the SARS-CoV-2 virus in the community. In this work, we choose a fractional-order mathematical model to examine the transmissibility in the community of several symptoms of COVID-19 in the sense of the Caputo operator. Sensitivity analysis of R0 and disease-free local stability of the system are checked. Also, with the assistance of fixed point theory, we demonstrate the existence and uniqueness of the system. In addition, numerically we solve the fractional model and presented some simulation results via actual estimation parameters. Graphically we displayed the effects of numerous model parameters and memory indexes. The numerical outcomes show the reliability, validation, and accuracy of the scheme.
Citation: Maryam Amin, Muhammad Farman, Ali Akgül, Mohammad Partohaghighi, Fahd Jarad. Computational analysis of COVID-19 model outbreak with singular and nonlocal operator[J]. AIMS Mathematics, 2022, 7(9): 16741-16759. doi: 10.3934/math.2022919
[1] | Muhammad Farman, Ali Akgül, Kottakkaran Sooppy Nisar, Dilshad Ahmad, Aqeel Ahmad, Sarfaraz Kamangar, C Ahamed Saleel . Epidemiological analysis of fractional order COVID-19 model with Mittag-Leffler kernel. AIMS Mathematics, 2022, 7(1): 756-783. doi: 10.3934/math.2022046 |
[2] | Iqbal M. Batiha, Abeer A. Al-Nana, Ramzi B. Albadarneh, Adel Ouannas, Ahmad Al-Khasawneh, Shaher Momani . Fractional-order coronavirus models with vaccination strategies impacted on Saudi Arabia's infections. AIMS Mathematics, 2022, 7(7): 12842-12858. doi: 10.3934/math.2022711 |
[3] | Aqeel Ahmad, Cicik Alfiniyah, Ali Akgül, Aeshah A. Raezah . Analysis of COVID-19 outbreak in Democratic Republic of the Congo using fractional operators. AIMS Mathematics, 2023, 8(11): 25654-25687. doi: 10.3934/math.20231309 |
[4] | Moh. Mashum Mujur Ihsanjaya, Nanang Susyanto . A mathematical model for policy of vaccinating recovered people in controlling the spread of COVID-19 outbreak. AIMS Mathematics, 2023, 8(6): 14508-14521. doi: 10.3934/math.2023741 |
[5] | Shao-Wen Yao, Muhammad Farman, Maryam Amin, Mustafa Inc, Ali Akgül, Aqeel Ahmad . Fractional order COVID-19 model with transmission rout infected through environment. AIMS Mathematics, 2022, 7(4): 5156-5174. doi: 10.3934/math.2022288 |
[6] | Oluwaseun F. Egbelowo, Justin B. Munyakazi, Manh Tuan Hoang . Mathematical study of transmission dynamics of SARS-CoV-2 with waning immunity. AIMS Mathematics, 2022, 7(9): 15917-15938. doi: 10.3934/math.2022871 |
[7] | Nadiyah Hussain Alharthi, Mdi Begum Jeelani . Analyzing a SEIR-Type mathematical model of SARS-COVID-19 using piecewise fractional order operators. AIMS Mathematics, 2023, 8(11): 27009-27032. doi: 10.3934/math.20231382 |
[8] | Muhammad Danish Zia, Esmail Hassan Abdullatif Al-Sabri, Faisal Yousafzai, Murad-ul-Islam Khan, Rashad Ismail, Mohammed M. Khalaf . A study of quadratic Diophantine fuzzy sets with structural properties and their application in face mask detection during COVID-19. AIMS Mathematics, 2023, 8(6): 14449-14474. doi: 10.3934/math.2023738 |
[9] | Rahat Zarin, Amir Khan, Aurangzeb, Ali Akgül, Esra Karatas Akgül, Usa Wannasingha Humphries . Fractional modeling of COVID-19 pandemic model with real data from Pakistan under the ABC operator. AIMS Mathematics, 2022, 7(9): 15939-15964. doi: 10.3934/math.2022872 |
[10] | CW Chukwu, S. Y. Tchoumi, Z. Chazuka, M. L. Juga, G. Obaido . Assessing the impact of human behavior towards preventative measures on COVID-19 dynamics for Gauteng, South Africa: a simulation and forecasting approach. AIMS Mathematics, 2024, 9(5): 10511-10535. doi: 10.3934/math.2024514 |
The SARS-CoV-2 virus pandemic remains a pressing issue with its unpredictable nature, and it spreads worldwide through human interaction. Current research focuses on the investigation and analysis of fractional epidemic models that discuss the temporal dynamics of the SARS-CoV-2 virus in the community. In this work, we choose a fractional-order mathematical model to examine the transmissibility in the community of several symptoms of COVID-19 in the sense of the Caputo operator. Sensitivity analysis of R0 and disease-free local stability of the system are checked. Also, with the assistance of fixed point theory, we demonstrate the existence and uniqueness of the system. In addition, numerically we solve the fractional model and presented some simulation results via actual estimation parameters. Graphically we displayed the effects of numerous model parameters and memory indexes. The numerical outcomes show the reliability, validation, and accuracy of the scheme.
In the 21st century, due to several applications of mathematical biology, researchers have shown exceptional interest in this sector. So, in the case of mathematical modeling, their vital concern is to express contagious diseases and control them. Brownlee [1] arranged a solid foundation of mathematical biology. For the spread of infection [2], he suggested a law with the help of probabilistic techniques. The implementation of fractional derivatives is helpful in the modeling of several problems of mathematical biology, like hepatitis, glucose, Ebola, HIV, etc. [3,4,5,6,7,8,9,10,11].
Within a few years, several techniques were used to investigate the dynamics of COVID-19. Among these, Caputo [12], Caputo-Fabrizio [13] and Atangana-Baleanu-Caputo (ABC) [14] are the focus of attention around the world. [15,16,17,18,19] Some applications of these were helpful in several issues. Also, over the last few months, numerous infection models have been constructed and discussed based on various fractional-order operators to study the dynamic behavior and control of new COVID-19 cases. In [20], with the help of the famous Caputo Fabrizio derivative, a new transmission model for COVID-19 dynamics was established. In [21], the authors developed a fractional-order model with the ABC operator. In [22], to see the effect of lockdown, Atangana presented a model with the assistance of the fractal fractional operator. Vaccines play a vital role in decreasing the growth of COVID-19, and their effects can be seen in [23]. In [24], Akgül et al. analyzed the differential equation model in the context of COVID-19. Further, they determined its stability with fractal-fraction derivative. Din et al. [25] proceed Akgül's work to solve the problems of Hepatitis B. In [26], the authors qualitatively used the stability theory of DEs and the basic reproduction number, which represents an epidemic index obtained from the so-called maximum eigenvalues of the next-generation matrix. They presented a mathematical model that calculates the results, and it turns out to be beneficial to public health initiatives. [27,28,29] Various fractional operators associated with the infection COVID-19 have been proposed to work the dynamics of fractional epidemic models. [30] Atangana et al. suggested a model and extended it to the scope of nonlocal operators. Moreover, simulations were performed for different non-integer numbers. In [31], the authors initially considered the classical integer model and then generalized it by introducing the Caputo fractional derivative. Further, for the case of simulation, they used the generalized Adams-Bashforth-Moulton scheme.
An inverse problem for the heat equation in two dimensional space with the Robin boundary condition that involves a new fractional derivative, namely, the Atangana-Baleanu approach with non-local and non-singular kernel was considered in [37]. Futher, a numerical algorithm in the reproducing kernel Hilbert space (RKHS) approach was applied to the inverse source problem for the diffusion equation in a time-space fractional sense, where determinations of state variables and source parameters subject to initial boundary and over-determination conditions are the main goal. [38] dealt with two types of inverse problems for diffusion equations involving Caputo fractional derivatives in time and the fractional Sturm-Liouville operator for space. An inverse source problem for fractional diffusion equation that containing fractional derivative with non-singular and non-local kernel, namely, the Atangana-Baleanu-Caputo fractional derivative, was studied in [39].
The manuscript is arranged as follows: In Section 2, some basic notions related to the Caputo derivative are described. In Section 3, we formulated COVID-19 model. We analyze the positivity and local stability of the system in Section 4. Moving on, in Section 5, we proved some useful results related to existence and uniqueness of the model. We develop numerical schemes in Section 6. Further, we discuss simulations in Section 7. The last segment consists of a conclusion.
In this section, some primary notions are described that are helpful for analyzing the system.
Definition 2.1. [32] A Caputo derivative of fractional order ϱ∈(0,1), for the function Z can be defined as
∁Dϱt(z(t))=1Γ(p−ϱ)∫t0zp(ρ)(t−ρ)ϱ−p+1]dρ,p=[ϱ]+1. |
Definition 2.2. [32] The integral operator of fractional order ϱ∈(0,1) corresponding to the Caputo fractional derivative is defined as
∁Iϱt(z(t))=1Γ(ϱ)∫t0(t−ρ)ϱ−1z(ρ)dρ. |
Lemma 2.3. [12] Suppose S(⋏)∈C(0,T), the result of the fractional differential equation
{∁Dp0⊏(⋏)=S(⋏),⋏∈(0,T),⊏(0)=S0, |
is given by
I(⋏)=p∑m=0Lm⋏m+1Γ(ν)∫⋏0(⋏−ρ)ν−1S(ρ)dρ, |
where Lm∈R,m=0,1,2,...,p.
Lemma 2.4. [14,25,33] For any arbitrary am∈R,m=0,1,2,...,p−1, the following result holds:
Iϱ[∁Dϱt⋏(t)]=⋏(t)+a0+a1t+a2t2+...+ap−1tp−1, |
where p=[ϱ]+1, and [ϱ] represents the integer part of ϱ.
Lemma 2.5. [25] Suppose ⋏∈ACl[0,T],ϱ>0, and l=[ϱ]. Then, the following condition is satisfied:
Iϱ[∁Dϱt⋏(∧)]=⋏(∧)−l−1∑m=0Dm⋏(a)m!(t−a)m. |
Lemma 2.6. [14,25] From the above Lemma, 2.5, the outcome Dϱ⋏(∧)=x(t),l−1<ϱ<l, is
⋏(∧)=Iϱx(t)+b0+b1t+b1t2+...+bl−1tl−1, |
where bm∈R.
Definition 2.7. [14] If
∁Dϱt⋏(t)=Z(t,⋏(t)), |
then
⋏(tm+1)=⋏(tm)+z(tm,⋏m)kΓ(ϱ)[2kϱtϱm+1−tϱ+1m+1ϱ+1+hϱtϱm−tϱ+1ϱ]+z(tm−1,⋏m−1)kΓ(ϱ)[kϱtϱm+1−tϱ+1m+1ϱ+1+tϱmϱ+1]+Rϱm(t). |
Here, Rϱm(t) is the remainder.
Theorem 2.1. [32,33] Let B:Y→G be compact and continuous, where G is a Banach space. If the set
F={⋏∈G:⋏=nB⋏,n∈(0,1)}, |
is bounded, then we can say that B has a unique fixed point.
The epidemiological compartment concept is one that we support [34] that takes into consideration the distinction among people with extreme symptoms, mild symptoms, and without symptoms. We can split the model into nine parts at time t: Susceptible ZS(t), exposed ZE(t), severe symptoms of infectious individuals, ZIss(t), mild symptoms infectious individuals ZIms(t), infectious but asymptomatic individuals ZIa(t), hospitalized ZH(t), intensive care unit class ZIcu(t), recovery with immunity ZR(t) and the class of death ZD(t).
dZSdt=−¯βZs(t)N(ZIss(t)+ZIms(t)+ZIa(t)),dZEdt=¯βZs(t)N(ZIss(t)+ZIms(t)+ZIa(t))−¯kZE(t),dZIssdt=¯k¯p1ZE(t)−¯hZIss(t),dZImsdt=¯k¯p2ZE(t)−¯γ3ZIms(t),dZIadt=¯k(1−¯p1−¯p2)ZE(t)−¯γ3ZIa(t),dZHdt=¯h¯q1ZIss(t)−ZH(t),dZIcudt=¯h(1−¯q1)ZIss(t)−ZIcu(t),dZRdt=¯γ3ZIms(t)+¯γ3ZIa(t)+(1−¯δ1)ZH(t)+(1+¯γ1)ZIcu(t),dZDdt=¯δ1ZH(t)+¯γ1)ZIcu(t), | (3.1) |
with initial condition
ZS(0)≥0,ZE(0)≥0,ZIss(0)≥(0),ZIms≥0,ZIa(0)≥0,ZH(0)≥0, |
ZIcu(0)≥0,ZR(0)=0,ZD(0)=0. |
The human-to-human transmission coefficient per unit time per person is ¯β in this case. The rate at which an individual departs the exposed clan by becoming contagious is represented by ¯k. An incubation period is defined as a time of 1/¯k. The parameter ¯p1 represents the likelihood that an individual will leave an exposed compartment ZE and become infected with severe symptoms ZIss. ¯p2 is the probability that an exposed person will become infected with moderate symptoms is called ZIms and 1−¯p1−¯p2 is the likelihood that exposed people joined an asymptomatic clan. ZIa. ¯h represents the pace at which a person exits the compartment ZIss while ¯q1 is the probability at which a person in ZIss went to the compartment H of hospitalized individuals. The recovery rate of persons with minor symptoms and asymptomatic people who are not hospitalized is ¯γ3. The death rate of hospitalized patients H who do not receive intensive care is ¯δ1. ¯γ1 is the death rate of hospitalized patients with intensive care ZIcu.
We apply the Caputo derivative to the COVID-19 model
∁DαtZS=−¯βZs(t)N(ZIss(t)+ZIms(t)+ZIa(t)),∁DαtZE=¯βZs(t)N(ZIss(t)+ZIms(t)+ZIa(t))−¯kZE(t),∁DαtZIss=¯k¯p1ZE(t)−¯hZIss(t),∁DαtZIms=¯k¯p2ZE(t)−¯γ3ZIms(t),∁DαtZIa=¯k(1−¯p1−¯p2)ZE(t)−¯γ3ZIa(t),∁DαtZH=¯h¯q1ZIss(t)−ZH(t),∁DαtZIcu=¯h(1−¯q1)ZIss(t)−ZIcu(t),∁DαtZR=¯γ3ZIms(t)+¯γ3ZIa(t)+(1−¯δ1)ZH(t)+(1+¯γ1)ZIcu(t),∁DαtZD=¯δ1ZH(t)+¯γ1)ZIcu(t), | (3.2) |
with initial condition
ZS(0)≥0,ZE(0)≥0,ZIss(0)≥(0),ZIms≥0,ZIa(0)≥0,ZH(0)≥0,ZIcu(0)≥0, |
ZR(0)=0,ZD(0)=0. |
Theorem 4.1. The solution of the proposed model (3.2) along initial conditions is unique and bounded in R9+.
Proof. In system (3.2), we can get its existence and uniqueness on the time interval (0,∞). Afterwards, we need to show that the non-negative region R9+ is a positively invariant region. From model (3.2), we find
∁DαtZS(t)|ZS=0=−¯βZs(t)N(ZIss(t)+ZIms(t)+ZIa(t)),∁DαtZE(t)|ZE=0=¯βZs(t)N(ZIss(t)+ZIms(t)+ZIa(t))−¯kZE(t),∁DαtZIss(t)|ZIss=0=¯k¯p1ZE(t)−¯hZIss(t),∁DαtZIms(t)ZIms=0=¯k¯p2ZE(t)−¯γ3ZIms(t),∁DαtZIa(t)|ZIa=0=¯k(1−¯p1−¯p2)ZE(t)−¯γ3ZIa(t),∁DαtZH(t)|ZH=0=¯h¯q1ZIss(t)−ZH(t),∁DαtZIcu(t)|ZIcu=0=¯h(1−¯q1)ZIss(t)−ZIcu(t),∁DαtZR(t)|ZR=0=¯γ3ZIms(t)+¯γ3ZIa(t)+(1−¯δ1)ZH(t)+(1+¯γ1)ZIcu(t),∁DαtZD(t)|ZD=0=¯δ1ZH(t)+¯γ1)ZIcu(t). | (4.1) |
If ZS(0),ZE(0),ZIss(0)ZIms,ZIa(0),ZH(0),ZIcu(0),ZR(0),ZD(0)∈R9+, then according to Eq (4.1), the solution cannot escape from the hyperplanes. Also, on each hyperplane bounding the non-negative orthant, the vector field points into R9+, i.e., the domain R9+ is a positively invariant set.
For the system (3.2), the disease-free equilibrium is given by ZS=N,ZE=ZIss=ZIms=ZIa=ZH=ZIcu=ZR=ZD=0). Further, to figure out the reproduction number R0 of the proposed system, we use the next-generation approach defined in [35,36]. Thus,
F=(0¯β¯β¯β00000000000000000000000000000000) | (4.2) |
and
V=(−¯k00000¯k¯p1−¯h0000¯k¯p20−¯γ3000¯k(1−¯p1−¯p2)00−¯γ3000¯h¯q100−100¯h(1−¯q1)000−1) | (4.3) |
Hence,
R0=FV−1=¯β(¯p1¯h+1−¯p1¯γ3). | (4.4) |
We investigate the sensitivity of R0 by taking the partial derivatives of R0 for the involved parameters as follows:
∂R0¯β=¯p1¯h+1−¯p1¯γ3>0,∂R0∂¯p1=¯β¯h>0,∂R0∂¯h=−¯β¯p1¯h2<0,∂R0∂¯γ3=¯β(¯p1−1)¯γ23>0. |
We see that change in parameter R0 is very sensitive. Here, ¯β,¯p1,¯γ3 are growing while ¯h is decreasing. Hence, from the above analysis, we can say that prevention is better to control the disease.
Theorem 4.2. If R0<1, the disease-free equilibrium (E∗) of the system (3.2) is locally stable, and it is unstable if R0>1.
Proof. For (3.2), we have
{ZR(t)=¯γ3∫t0ZIms(s)+¯γ3∫t0ZIa(s)+(1−¯δ1)∫t0ZH(S),ZD(t)=¯δ1∫t0¯ZH(S)+¯γ1∫t0¯ZIcu(S). | (4.5) |
The total population N is constant, so we have
ZS(t)=N−[E+ZIss+ZIms+ZIa+ZH+ZIcu+ZR+ZD]. |
Thus, for the system (3.2), we can find the local stability through the variables ZE,ZIss,ZIms,ZIa,ZH and ZIcu. Therefore, the system is:
∁DαtZE=¯βZs(t)N(ZIss(t)+ZIms(t)+ZIa(t))−¯kZE(t),∁DαtZIss=¯k¯p1ZE(t)−¯hZIss(t),∁DαtZIms=¯k¯p2ZE(t)−¯γ3ZIms(t),∁DαtZIa=¯k(1−¯p1−¯p2)ZE(t)−¯γ3ZIa(t),∁DαtZH=¯h¯q1ZIss(t)−ZH(t),∁DαtZIcudt=¯h(1−¯q1)ZIss(t)−ZIcu(t). | (4.6) |
The Jacobian matrix J(E∗) for the above system is:
J(E∗)=(−¯k¯β¯β¯β00¯k¯p1−¯h0000¯k¯p20−¯γ3000¯k(1−¯p1−¯p2)00−¯γ3000¯h¯q100−100¯h(1−¯q1)000−1) | (4.7) |
The two eigenvalues are λ∗1=λ∗2=−1. For the remaining, we have
P∗(λ∗)=l0λ4∗+l1λ3∗+l2λ2∗+l3λ∗+l4=0, | (4.8) |
where
l0=1,l1=¯h+¯k+2¯γ3,l2=¯h¯k−¯k¯β+¯γ23+2¯hγ3+2¯k¯γ3,l3=−¯h¯k¯β−¯h¯k¯β¯p1+¯h¯γ32+¯k¯γ32+2¯h¯k¯γ3−¯k¯β¯γ3−¯k¯β¯γ3¯p1,l4=¯h¯k¯γ32−¯k¯p1¯β¯γ32−¯h¯k¯β¯γ3+¯h¯k¯β¯p1¯γ3. | (4.9) |
If R0<1, then all the states are fulfilled, which shows system (3.2) is locally stable and disease will decrease. If R0>1, the last state is not fulfilled, which shows system (3.2) is unstable, and disease can be increase.
For the sake of the required goal, here we set up the states for the existence and uniqueness of the system. Consider the functions:
∧1(t,ZS,ZE,ZIss,ZIms,ZIa,ZH,ZIcu,ZR,ZD)=−¯βZs(t)N(ZIss(t)+ZIms(t)+ZIa(t)),∧2(t,ZS,ZE,ZIss,ZIms,ZIa,ZH,ZIcu,ZR,ZD)=¯βZs(t)N(ZIss(t)+ZIms(t)+ZIa(t))−¯kZE(t),∧3(t,ZS,ZE,ZIss,ZIms,ZIa,ZH,ZIcu,ZR,ZD)=¯k¯p1ZE(t)−¯hZIss(t),∧4(t,ZS,ZE,ZIss,ZIms,ZIa,ZH,ZIcu,ZR,ZD)=¯k¯p2ZE(t)−¯γ3ZIms(t),∧5(t,ZS,ZE,ZIss,ZIms,ZIa,ZH,ZIcu,ZR,ZD)=¯k(1−¯p1−¯p2)ZE(t)−¯γ3ZIa(t),∧6(t,ZS,ZE,ZIss,ZIms,ZIa,ZH,ZIcu,ZR,ZD)=¯h¯q1ZIss(t)−ZH(t),∧7(t,ZS,ZE,ZIss,ZIms,ZIa,ZH,ZIcu,ZR,ZD)=¯h(1−¯q1)ZIss(t)−ZIcu(t),∧8(t,ZS,ZE,ZIss,ZIms,ZIa,ZH,ZIcu,ZR,ZD)=¯γ3ZIms(t)+¯γ3ZIa(t)+(1−¯δ1)ZH(t)+(1+¯γ1)ZIcu(t),∧9(t,ZS,ZE,ZIss,ZIms,ZIa,ZH,ZIcu,ZR,ZD)=¯δ1ZH(t)+¯γ1)ZIcu(t). | (5.1) |
Consider the Banach space, C[0,T]=B, having norm
‖ϱ‖=supt∈[0,T][|ZS(t)|+|ZE(t)|+|ZIss(t)|+|ZIms(t)|+|ZIa(t)|+|ZH(t)|+|ZIcu(t)|+|ZR(t)|+|ZD(t)|], |
where
⋏(t)={ZS(t)ZE(t)ZIss(t)ZIms(t)ZIa(t),ZH(t)ZIcu(t)ZR(t)ZD(t)⋏0(t)={ZS0ZE0ZIss0ZIms0ZIa0,ZH0ZIcu0ZR0ZD0 |
℘(t,⋏(t))={∧1(t,ZS,ZE,ZIss,ZIms,ZIa,ZH,ZIcu,ZR,ZD)∧2(t,ZS,ZE,ZIss,ZIms,ZIa,ZH,ZIcu,ZR,ZD)∧3(t,ZS,ZE,ZIss,ZIms,ZIa,ZH,ZIcu,ZR,ZD)∧4(t,ZS,ZE,ZIss,ZIms,ZIa,ZH,ZIcu,ZR,ZD)∧5(t,ZS,ZE,ZIss,ZIms,ZIa,ZH,ZIcu,ZR,ZD).∧6(t,ZS,ZE,ZIss,ZIms,ZIa,ZH,ZIcu,ZR,ZD)∧7(t,ZS,ZE,ZIss,ZIms,ZIa,ZH,ZIcu,ZR,ZD)∧8(t,ZS,ZE,ZIss,ZIms,ZIa,ZH,ZIcu,ZR,ZD)∧9(t,ZS,ZE,ZIss,ZIms,ZIa,ZH,ZIcu,ZR,ZD) | (5.2) |
From (5.2), we can represent the system (3.2) as
{∁Dp0⋏(t)=℘(t,⋏(t)),t∈[0,T],⋏(0)=⋏0. | (5.3) |
From Lemma 1, Eq (5.3) can be written as:
⋏(t)=⋏(0)+∫t0(t−ρ)ϱ−1Γ(ϱ)℘(ρ,⋏(ρ))dρ,t∈[0,T]. | (5.4) |
We assume the following hypothesis.
∙(h1)ThereexistsconstantsZK,ZM>0
|℘(t,⋏(t))|≤ZK|⋏|p+ZM. |
∙(h2)Foreach⋏,¯⋏,existsaconstantZL>0
|℘(t,⋏)|−|℘(t,¯⋏|≤ZL‖⋏−¯⋏‖. |
For an operator ⋄:B⟶B,
⋄⋏(t)=⋏0+∫t0(t−ρ)ϱ−1Γ(ϱ)℘(ρ,⋏(ρ))dρ. | (5.5) |
Theorem 5.1. or the authentication of Eq (5.3), suppose our hypotheses are true. Then, it has at least one solution h1,h2 are true. Then, it has at least one solution.
Proof. We validate it in the following four steps:
S1.Initially, we show that operator, ⋄ is continuous. For that, consider an operator ℘ is a continuous, so that ℘(ρ,⋏(ρ)) is continuous as well. Further, if for ⋏,⋏m∈G there exist ⋏n⟶⋏ then we have ⋄⋏n⟶⋄⋏. Suppose
‖⋄⋏n⟶⋄⋏‖=maxt∈[0,T]|∫t0(t−ρ)ϱ−1Γ(ϱ)℘m(ρ,⋏m(ρ))dρ−∫t0(t−ρ)ϱ−1Γ(ϱ)℘(ρ,⋏(ρ))dρ|,=maxt∈[0,T]|∫t0(t−ρ)ϱ−1Γ(ϱ)||℘m(ρ,⋏m(ρ))dρ−℘(ρ,⋏(ρ))|dρ,≤T1Γ(ϱ+1)‖℘m−℘‖⟶0asm⟶∞. | (5.6) |
Thus, ℘ is continuous, so ⋄ is continuous.
S2.In this step, we show that ⋄ is bounded. For this, we consider that ⋄ fulfills the growth condition:
‖⋄⋏‖=maxt∈[0,T]|⋏0+1Γ(ϱ)∫t0(t−ρ)ϱ−1℘(ρ,⋏(ρ))dρ|,≤|⋏0|+maxt∈[0,T]|1Γ(ϱ)∫t0|(t−ρ)ϱ−1|℘(ρ,⋏(ρ))dρ|,≤|⋏0|+T1Γ(ϱ+1)[ZK⋏0‖p+ZM]. | (5.7) |
Hence, ⋄ is bounded.
S3.Furthermore, we show that ⋄ is equi-continuous. For this, consider t2≤t1=[0,T]. Then,
|⋄⋏(t1)−⋄⋏(t2)|=|1Γ(ϱ)∫t0(t1−ρ)ϱ−1℘(ρ,⋏(ρ))dρ−1Γ(ϱ)∫t0(t2−ρ)ϱ−1℘(ρ,⋏(ρ))dρ|,≤|[1Γ(ϱ)∫t0(t1−ρ)ϱ−1−1Γ(ϱ)∫t0(t2−ρ)ϱ−1]℘(ρ,⋏(ρ))dρ|,≤T1Γ(ϱ+1)[ZK‖⋏‖p+ZM][t1−t2]. | (5.8) |
Thus, by using the theorem of Arzela-Ascoli, it is compact
S4.At the final stage, we show that set E is bounded. For this, consider that for all t∈[0,T], we have ⋏∈E and then
‖⋏‖=m‖⋄⋏‖≤m[T1Γ(ϱ+1)[ZK‖⋏‖p+ZM]. | (5.9) |
So, it is bounded, and by following the outcomes of Schauder's fixed point theorem, ⋄ has at least one solution.
Theorem 5.2. If TϱZKΓ(ϱ+1)<1, then Eq (5.3) has a distinct solution.
Proof. Suppose ⋏,¯⋏∈G and then
‖⋄⋏−⋄¯⋏‖=maxt∈[0,T]∫t0|(t1−ρ)ϱ−1Γ(ϱ)|℘(ρ,⋏(ρ))dρ−℘(ρ,¯⋏(ρ))|dρ,≤TϱZK⋄Γ(ϱ+1)‖⋏−¯⋏‖. | (5.10) |
Therefore, the fixed point is distinct and has a single outcome.
In this part, our focus is to implement the operators on the proposed model. Further, we restore the classical operators with the power-law kernel and implement the variable version. For the power-law kernel
∁DαtZS=−¯βZs(t)N(ZIss(t)+ZIms(t)+ZIa(t)),∁DαtZE=¯βZs(t)N(ZIss(t)+ZIms(t)+ZIa(t))−¯kZE(t),∁DαtZIss=¯k¯p1ZE(t)−¯hZIss(t),∁DαtZIms=¯k¯p2ZE(t)−¯γ3ZIms(t),∁DαtZIa=¯k(1−¯p1−¯p2)ZE(t)−¯γ3ZIa(t),∁DαtZH=¯h¯q1ZIss(t)−ZH(t),∁DαtZIcu=¯h(1−¯q1)ZIss(t)−ZIcu(t),∁DαtZR=¯γ3ZIms(t)+¯γ3ZIa(t)+(1−¯δ1)ZH(t)+(1+¯γ1)ZIcu(t),∁DαtZD=¯δ1ZH(t)+¯γ1)ZIcu(t). | (6.1) |
For clarification, we represent the model as;
∁DαtZS=ZS1(t,ZS,ZE,ZIss,ZIms,ZIa,ZH,ZIcu,ZR,ZD),∁DαtZE=ZE1(t,ZS,ZE,ZIss,ZIms,ZIa,ZH,ZIcu,ZR,ZD),∁DαtZIss=ZIss1(t,ZS,ZE,ZIss,ZIms,ZIa,ZH,ZIcu,ZR,ZD),∁DαtZIms=ZIms1(t,ZS,ZE,ZIss,ZIms,ZIa,ZH,ZIcu,ZR,ZD),∁DαtZIa=ZIa1(t,ZS,ZE,ZIss,ZIms,ZIa,ZH,ZIcu,ZR,ZD),∁DαtZH=ZH1(t,ZS,ZE,ZIss,ZIms,ZIa,ZH,ZIcu,ZR,ZD),∁DαtZIcu=ZIcu1(t,ZS,ZE,ZIss,ZIms,ZIa,ZH,ZIcu,ZR,ZD),∁DαtZR=ZR1(t,ZS,ZE,ZIss,ZIms,ZIa,ZH,ZIcu,ZR,ZD),∁DαtZD=ZD1(t,ZS,ZE,ZIss,ZIms,ZIa,ZH,ZIcu,ZR,ZD), | (6.2) |
where
ZS1(t,ZS,ZE,ZIss,ZIms,ZIa,ZH,ZIcu,ZR,ZD)=−¯βZs(t)N(ZIss(t)+ZIms(t)+ZIa(t)),ZE1(t,ZS,ZE,ZIss,ZIms,ZIa,ZH,ZIcu,ZR,ZD)=¯βZs(t)N(ZIss(t)+ZIms(t)+ZIa(t))−¯kZE(t),ZIss1(t,ZS,ZE,ZIss,ZIms,ZIa,ZH,ZIcu,ZR,ZD)=¯k¯p1ZE(t)−¯hZIss(t),ZIms1(t,ZS,ZE,ZIss,ZIms,ZIa,ZH,ZIcu,ZR,ZD)=¯k¯p2ZE(t)−¯γ3ZIms(t),ZIa1(t,ZS,ZE,ZIss,ZIms,ZIa,ZH,ZIcu,ZR,ZD)=¯k(1−¯p1−¯p2)ZE(t)−¯γ3ZIa(t),ZH1(t,ZS,ZE,ZIss,ZIms,ZIa,ZH,ZIcu,ZR,ZD)=¯h¯q1ZIss(t)−ZH(t),ZIcu1(t,ZS,ZE,ZIss,ZIms,ZIa,ZH,ZIcu,ZR,ZD)=¯h(1−¯q1)ZIss(t)−ZIcu(t),ZR1(t,ZS,ZE,ZIss,ZIms,ZIa,ZH,ZIcu,ZR,ZD)=¯γ3ZIms(t)+¯γ3ZIa(t)+(1−¯δ1)ZH(t)+(1+¯γ1)ZIcu(t),ZD1(t,ZS,ZE,ZIss,ZIms,ZIa,ZH,ZIcu,ZR,ZD)=¯δ1ZH(t)+¯γ1)ZIcu(t). | (6.3) |
For the Caputo fractal fractional derivative, consider
{∁Dα,τ0⋏(t)=℘(t,⋏(t)),t∈[0,T],⋏(0)=⋏0. | (6.4) |
By applying the fractional integral with power law kernel, we can write the above expression as:
⋏(t)=⋏(0)+∫t0(t−ϱ)τ−1Γ(ϱ)ϱ1−α℘(ϱ,⋏(ϱ))dϱ. | (6.5) |
At tσ+1=(σ+1)Δt, we get
⋏(t)=⋏(0)+∫tσ+10(tσ+1−ϱ)τ−1Γ(ϱ)ϱ1−α℘(ϱ,⋏(ϱ))dϱ. | (6.6) |
For clarity, we take
Q(t,⋏(t))=℘(t,⋏(t))tτ−1. | (6.7) |
We also have
⋏(tσ+1)=⋏(0)+σ∑m=2∫tl+1l(tσ+1−ϱ)τ−1Γ(ϱ)ϱ1−αQ(ϱ,⋏(ϱ))dϱ. | (6.8) |
Replacing them in the above equation and substituting Q(t,⋏(t))=℘(t,⋏(t))tτ−1, we get the numerical scheme
⋏(tσ+1)=(Δt)τΓ(τ+1)α∑m=2t1−σm−2℘(tm−2,⋏m−Δt℘(tm,⋏m)−Δt℘(tm−1,⋏m−Δt℘(tm,⋏m)))×[(σ−m+1)τ−(σ−m)τ]+(Δt)τΓ(τ+2)σ∑m=2[t1−αm−1℘(tm−1,⋏m−Δt℘(tm,⋏m)−t1−αm−2℘(tm−2,⋏m−Δt℘(tm,⋏m)−Δt℘(tm−1,⋏m−Δt℘(tm,⋏m))×[(σ−m+1)τ(σ−m+3+2τ)−(σ−m)τ(σ−m+3+3τ)]+(Δt)τ2Γ(τ+3)σ∑m=2[t1−αm℘(tm,⋏m−2Δt1−αm−1℘(tm−1,⋏m)−℘(tm,⋏mΔ)+t1−αm−2℘(tm−2,⋏m−Δt℘(tm,⋏m))−Δt℘(tm−1,⋏m−Δt℘(tm,⋏m)))]×[(σ−m+1)τ[2(σ−m)2+(3τ+10)(σ−m)+2τ2+9τ+12]−(σ−m)τ[2(σ−m)2+(5τ+10)(σ−m)+6τ2+18τ+12]]. |
The plot plays a dominant role in realizing the behavior of the simulation of the model with the Caputo operator. We plot, for different values of α=0.85,0.9,0.95,1.0, all nine compartments of the model in Figures 1–9. In Figures 1 and 6, we can see that the plot of susceptible and hospitalized people are decreasing with the passage of time. We see that the number of exposed ones and population having no symptoms both are increasing as shown in Figure 2. In the cases of people with severe and mild symptoms, their numbers initially go down rapidly and then rise. Similarly, the number of people in the intensive care unit also goes down rapidly. At certain value, plot moves smoothly, which means minor patients are in severe condition, as shown in Figures 3–7. Lastly, computational results of recovers and death populations are shown in Figures 8 and 9. The results of the nonlinear system memory were also detected with the help of fractional value results. Results provides a better way in case you want to control the disease without defining other parameters.
The spread of COVID-19 infections is not only for both human health, but also a serious threat to the economy. Although the amount of exercise and the number of reports on the possibility of this new pandemic possibility control are published in recent years. In this study, we have formulated a new mathematical model to analyze the complex transfer dynamics of COVID-19 under the Caputo operator. The model has been studied qualitatively as well as quantitatively. For the suggested system, positivity, uniqueness, and boundedness for solution are proven. The proposed compartment model is prescribed by a classic differential equation first with an integer order. Treatment classes are suitable for model construction. We determined that our obtained results are effective for the proposed model. Some theoretical results were also discussed for the model.
The authors declare no conflict of interest.
[1] |
J. Brownlee, Certain considerations on the causation and course of epidemics, P. Roy. Soc. Med., 2 (1909), 243–258. http://dx.doi.org/10.1177/003591570900201307 doi: 10.1177/003591570900201307
![]() |
[2] |
J. Brownlee, The mathematical theory of random migration and epidemic distribution, P. Roy. Soc. Edinb., 31 (1912), 262–289. http://dx.doi.org/10.1017/S0370164600025116 doi: 10.1017/S0370164600025116
![]() |
[3] |
L. Frunzo, R. Garra, A. Giusti, V. Luongo, Modeling biological systems with an improved fractional Gompertz law, Commun. Nonlinear Sci., 74 (2019), 260–267. http://dx.doi.org/10.1016/j.cnsns.2019.03.024 doi: 10.1016/j.cnsns.2019.03.024
![]() |
[4] |
I. Zada, M. Naeem Jan, N. Ali, D. Alrowail, K. S. Nisar, G. Zaman, Mathematical analysis of hepatitis B epidemic model with optimal control, Adv. Differ. Equ., 2012 (2021), 451. https://doi.org/10.1186/s13662-021-03607-2 doi: 10.1186/s13662-021-03607-2
![]() |
[5] |
M. Farman, A. Ahmad, M. U. Saleem, A. Hafeez, A mathematical analysis and modelling of hepatitis B model with non-integer time fractional derivative, Commun. Math. Appl., 10 (2019), 571–584. https://doi.org/10.26713/cma.v10i3.1154 doi: 10.26713/cma.v10i3.1154
![]() |
[6] |
M. H. Alshehri, F. Z. Duraihem, A. Alalyani, A. Saber, A Caputo (discretization) fractional-order model of glucose-insulin interaction: Numerical solution and comparisons with experimental data, J. Taibah Univ. Sci., 15 (2021), 26–36. https://doi.org/10.1080/16583655.2021.1872197 doi: 10.1080/16583655.2021.1872197
![]() |
[7] |
M. U. Saleem, M. Farman, A. Ahmad, H. Ehsan, M. O. Ahmad, A Caputo Fabrizio fractional order model for control of glucose in insulintherapies for diabetes, Ain Shams Eng. J., 11 (2020), 1309–1316. https://doi.org/10.1016/j.asej.2020.03.006 doi: 10.1016/j.asej.2020.03.006
![]() |
[8] |
A. Ahmad, M. Farman, F. Muhammad, P. A. Naik, A. Akgül, N. Zafar, et al., Modeling and numerical investigation of fractional-order bovine babesiosis disease, Numer. Meth. Part. Differ. D. E., 37 (2021), 1946–1964. https://doi.org/10.1002/num.22632 doi: 10.1002/num.22632
![]() |
[9] |
A. Raza, M. Farman, A. Akgül, S. Iqbal, A. Ahmad, Simulation and numerical solution of fractional order ebola virus model with novel technique, Bio. Eng. J., 7 (2020), 194–207. https://doi.org/10.3934/bioeng.2020017 doi: 10.3934/bioeng.2020017
![]() |
[10] |
A. Ahmad, M. Farman, A. Akgül, N. Bukhari, S. Imtiaz, Mathematical analysis and numerical simulation of co-infection of TB-HIV, Arab J. Basic Appl. Sci., 27 (2020), 431–441. https://doi.org/10.1080/25765299.2020.1840771 doi: 10.1080/25765299.2020.1840771
![]() |
[11] |
S. W. Yao, M. Farman, M. Amin, M. İnç, A. Akgül, A. Ahmad, Fractional order COVID-19 model with transmission rout infected through environment, AIMS Math., 7 (2022), 5156–5174. https://doi.org/10.3934/math.2022288 doi: 10.3934/math.2022288
![]() |
[12] | I. Podlubny, Fractional differential equations: An introduction to fractional derivatives, fractional differential equations, to methods of their solution and some of their applications, Elsevier, 198 (1998). |
[13] |
M. Caputo, M. Fabrizio, A new definition of fractional derivative without singular kernel, Prog. Fract. Diff. Appl., 1 (2015), 73–85. http://dx.doi.org/10.12785/pfda/010201 doi: 10.12785/pfda/010201
![]() |
[14] |
A. Atangana, D. Baleanu, New fractional derivatives with nonlocal and non-singular kernel: Theory and application to heat transfer model, Therm. Sci., 20 (2016), 763–769. https://doi.org/10.2298/TSCI160111018A doi: 10.2298/TSCI160111018A
![]() |
[15] |
S. Ullah, M. A. Khan, M. Farooq, Z. Hammouch, D. Baleanu, A fractional model for the dynamics of tuberculosis infection using caputo-fabrizio derivative, Discrete Contin. Dyn.-S, 13 (2020), 975–993. http://dx.doi.org/10.3934/dcdss.2020057 doi: 10.3934/dcdss.2020057
![]() |
[16] |
M. Abdullah, A. Ahmad, N. Raza, M. Farman, M. O. Ahmad, Approximate solution and analysis of smoking epidemic model with caputo fractional derivatives, Int. J. Appl. Comput. Math., 4 (2018), 112. https://doi.org/10.1007/s40819-018-0543-5 doi: 10.1007/s40819-018-0543-5
![]() |
[17] |
S. Kumar, A. Ahmadian, R. Kumar, D. Kumar, J. Singh, D. Baleanu, et al., An efficient numerical method for fractional sir epidemic model of infectious disease by using Bernstein wavelets, Mathematics, 8 (2020), 558. https://doi.org/10.3390/math8040558 doi: 10.3390/math8040558
![]() |
[18] |
M. A. Khan, M. Azizah, S. Ullah, A fractional model for the dynamics of competition between commercial and rural banks in Indonesia, Chaos Soliton. Fract., 122 (2019), 32–46. https://doi.org/10.1016/j.chaos.2019.02.009 doi: 10.1016/j.chaos.2019.02.009
![]() |
[19] |
S. Kumar, S. Ghosh, B. Samet, E. F. D. Goufo, An analysis for heat equations arises in diffusion process using new Yang-Abdel-Aty-Cattani fractional operator, Math. Methods Appl. Sci., 43 (2020), 6062–6080. https://doi.org/10.1002/mma.6347 doi: 10.1002/mma.6347
![]() |
[20] |
D. Baleanu, H. Mohammadi, S. Rezapour, A fractional differential equation model for the covid-19 transmission by using the Caputo-Fabrizio derivative, Adv. Differ. Equ., 2020 (2020), 299. https://doi.org/10.1186/s13662-020-02762-2 doi: 10.1186/s13662-020-02762-2
![]() |
[21] |
M. A. Khan, A. Atangana, Modeling the dynamics of novel coronavirus (2019-nCov) with fractional derivative, Alex. Eng. J., 59 (2020), 2379–2389. https://doi.org/10.1016/j.aej.2020.02.033 doi: 10.1016/j.aej.2020.02.033
![]() |
[22] |
A. Atangana, Modelling the spread of COVID-19 with new fractal-fractional operators: Can the lockdown save mankind before vaccination? Chaos Soliton. Fract., 136 (2020). https://doi.org/10.1016/j.chaos.2020.109860 doi: 10.1016/j.chaos.2020.109860
![]() |
[23] |
M. Amin, M. Farman, A. Akgül, R. T. Alqahtani, Effect of vaccination to control COVID-19 with fractal fractional operator, Alex. Eng. J., 61 (2022), 3551–3557. http://dx.doi.org/10.1016/j.aej.2021.09.006 doi: 10.1016/j.aej.2021.09.006
![]() |
[24] | A. Akgül, N. Ahmed, A. Raza, Z. Iqbal, M. Rafiq, D. Baleanu, et al., New applications related to COVID-19, Results Phys., 20 (2021), 1–6. |
[25] |
A. Din, Y. Li, F. M. Khan, Z. U. Khan, P. Liu, On analysis of fractional order mathematical model of hepatitis b using Atangana-Baleanu Caputo ABC derivative, Fractals, 30 (2021), 224001. https://doi.org/10.1142/S0218348X22400175 doi: 10.1142/S0218348X22400175
![]() |
[26] |
O. J. Peter, S. Qureshi, A. Yusuf, M. Al-shomrani, A. A. Idowu, A new mathematical model of COVID-19 using real data from Pakistan, Results Phys., 24 (2021), 104098. https://doi.org/10.1016/j.rinp.2021.104098 doi: 10.1016/j.rinp.2021.104098
![]() |
[27] |
A. S. Alshomrani, M. Z. Ullah, D. Baleanu, Caputo SIR model for COVID-19 under optimized fractional order, Adv. Differ. Equ., 2021 (2021), 185. https://doi.org/10.1186/s13662-021-03345-5 doi: 10.1186/s13662-021-03345-5
![]() |
[28] |
D. Baleanu, A. Jajarmi, H. Mohammadi, S. Rezapour, A new study on the mathematical modeling of human liver with Caputo-Fabrizio fractional derivative, Chaos Soliton. Fract., 134 (2020), 1–7. https://doi.org/10.1016/j.chaos.2020.109705 doi: 10.1016/j.chaos.2020.109705
![]() |
[29] |
A. Hussain, D. Baleanu, M. Adeel, Existence of solution and stability for the fractional-order novel coronavirus (nCoV-2019) model, Adv. Differ. Equ., 2020 (2020), 384. https://doi.org/10.1186/s13662-020-02845-0 doi: 10.1186/s13662-020-02845-0
![]() |
[30] |
A. Atangana, S. I. Araz, Mathematical model of COVID-19 spread in Turkey and South Africa: Theory, methods, and applications, Adv. Differ. Equ., 2020 (2020), 659. https://doi.org/10.1186/s13662-020-03095-w doi: 10.1186/s13662-020-03095-w
![]() |
[31] |
A. A. Khan, R. Amin, S. Ullah, W. Sumelka, M. Altanji, Numerical simulation of a Caputo fractional epidemic model for the novel coronavirus with the impact of environmental transmission, Alex. Eng. J., 61 (2022), 5083–5095. https://doi.org/10.1016/j.aej.2021.10.008 doi: 10.1016/j.aej.2021.10.008
![]() |
[32] |
P. J. Liu, T. Munir, T. Cui, A. Din, P. Wu, Mathematical assessment of the dynamics of the tobacco smoking model: An application of fractional theory, AIMS Math., 7 (2022), 7143–7165. https://doi.org/10.3934/math.2022398 doi: 10.3934/math.2022398
![]() |
[33] |
A. Atangana, K. M. Owolabi, New numerical approach for fractional differential equations, Math. Model. Nat. Pheno., 13 (2018). https://doi.org/10.1051/mmnp/2021039 doi: 10.1051/mmnp/2021039
![]() |
[34] |
M. Amouch, N. Karim, Modeling the dynamic of COVID-19 with different types of transmissions, Chaos Soliton. Fract., 150 (2021), 111188. https://doi.org/10.1016/j.chaos.2021.111188 doi: 10.1016/j.chaos.2021.111188
![]() |
[35] |
O. Diekmann, J. A. P. Heesterbeek, J. A. J. Metz, On the definition and the computation of the basic reproduction ratio R 0 in models for infectious diseases in heterogeneous populations, J. Math. Biol., 28 (1990), 365–382. https://doi.org/10.1007/BF00178324 doi: 10.1007/BF00178324
![]() |
[36] |
P. van den Driessche, J. Watmough, Reproduction numbers and sub-threshold endemic equilibria for compartmental models of disease transmission, Math. Biosci., 180 (2022), 29–48. https://doi.org/10.1016/S0025-5564(02)00108-6 doi: 10.1016/S0025-5564(02)00108-6
![]() |
[37] |
S. Djennadi, N. Shawagfeh, O. A. Arqub, A numerical algorithm in reproducing kernel-based approach for solving the inverse source problem of the time-space fractional diffusion equation, Part. Diff. Equ. Appl. Math., 4 (2021), 100164. https://doi.org/10.1016/j.padiff.2021.100164 doi: 10.1016/j.padiff.2021.100164
![]() |
[38] |
S. Djennadi, N. Shawagfeh, O. A. Arqub, A fractional Tikhonov regularization method for an inverse backward and source problems in the time-space fractional diffusion equations, Chaos Soliton. Fract., 150 (2021), 111127. https://doi.org/10.1016/j.chaos.2021.111127 doi: 10.1016/j.chaos.2021.111127
![]() |
[39] |
S. Djennadi, N. Shawagfeh, M. Inc, M. S. Osman, J. F. Gómez-Aguilar, O. A. Arqub, The Tikhonov regularization method for the inverse source problem of time fractional heat equation in the view of ABC-fractional technique, Phys. Scripta, 96 (2021). https://doi.org/10.1088/1402-4896/ac0867 doi: 10.1088/1402-4896/ac0867
![]() |
1. | Ali Hasan, Ali Akgül, Muhammad Farman, Faryal Chaudhry, Muhammad Sultan, Manuel De la Sen, Epidemiological Analysis of Symmetry in Transmission of the Ebola Virus with Power Law Kernel, 2023, 15, 2073-8994, 665, 10.3390/sym15030665 | |
2. | Muhammad Farman, Saba Jamil, Muhammad Bilal Riaz, Muhammad Azeem, Muhammad Umer Saleem, Numerical and quantitative analysis of HIV/AIDS model with modified Atangana-Baleanu in Caputo sense derivative, 2023, 66, 11100168, 31, 10.1016/j.aej.2022.11.034 | |
3. | Saba Jamil, Muhammad Farman, Ali Akgül, Qualitative and quantitative analysis of a fractal fractional HIV/AIDS model, 2023, 76, 11100168, 167, 10.1016/j.aej.2023.06.021 |