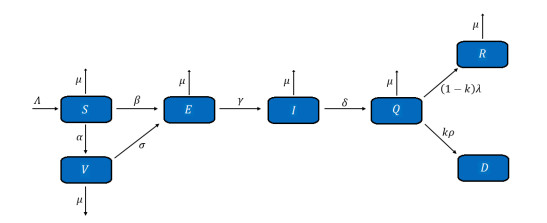
Several newly nonlinear models for describing dynamics of COVID-19 pandemic have been proposed and investigated in literature recently. In light of these models, we attempt to reveal the role of fractional calculus in describing the growth of COVID-19 dynamics implemented on Saudi Arabia's society over 107 days; from 17 Dec 2020 to 31 March 2021. Above is achieved by operating two fractional-order differential operators, Caputo and the Caputo-Fabrizio operators, instead of the classical one. One of expanded SEIR models is utilized for achieving our purpose. With the help of using the Generalized Euler Method (GEM) and Adams-Bashforth Method (ABM), the numerical simulations are performed respectively in view of the Caputo and Caputo-Fabrizio operators. Accordance with said, the stability analysis of the two proposed fractional-order models is discussed and explored in view of obtaining the equilibrium points, determining the reproductive number (R0) and computing the elasticity indices of R0. Several numerical comparisons reveal that the fractional-order COVID-19 models proposed in this work are better than that of classical one when such comparisons are performed between them and some real data collected from Saudi Arabia's society. This inference together with the cases predictions that could easily deduced from the proposed fractional-order models can allow primary decision makers and influencers to set the right plans and logic strategies that should be followed to face this pandemic.
Citation: Iqbal M. Batiha, Abeer A. Al-Nana, Ramzi B. Albadarneh, Adel Ouannas, Ahmad Al-Khasawneh, Shaher Momani. Fractional-order coronavirus models with vaccination strategies impacted on Saudi Arabia's infections[J]. AIMS Mathematics, 2022, 7(7): 12842-12858. doi: 10.3934/math.2022711
[1] | Muhammad Altaf Khan, Sajjad Ullah, Saif Ullah, Muhammad Farhan . Fractional order SEIR model with generalized incidence rate. AIMS Mathematics, 2020, 5(4): 2843-2857. doi: 10.3934/math.2020182 |
[2] | Sarkhel Akbar Mahmood, Pshtiwan Othman Mohammed, Dumitru Baleanu, Hassen Aydi, Yasser S. Hamed . Analysing discrete fractional operators with exponential kernel for positivity in lower boundedness. AIMS Mathematics, 2022, 7(6): 10387-10399. doi: 10.3934/math.2022579 |
[3] | E. Bonyah, C. W. Chukwu, M. L. Juga, Fatmawati . Modeling fractional-order dynamics of Syphilis via Mittag-Leffler law. AIMS Mathematics, 2021, 6(8): 8367-8389. doi: 10.3934/math.2021485 |
[4] | Muhammad Altaf Khan, Muhammad Ismail, Saif Ullah, Muhammad Farhan . Fractional order SIR model with generalized incidence rate. AIMS Mathematics, 2020, 5(3): 1856-1880. doi: 10.3934/math.2020124 |
[5] | Ihtisham Ul Haq, Nigar Ali, Hijaz Ahmad, Taher A. Nofal . On the fractional-order mathematical model of COVID-19 with the effects of multiple non-pharmaceutical interventions. AIMS Mathematics, 2022, 7(9): 16017-16036. doi: 10.3934/math.2022877 |
[6] | Murugesan Sivashankar, Sriramulu Sabarinathan, Vediyappan Govindan, Unai Fernandez-Gamiz, Samad Noeiaghdam . Stability analysis of COVID-19 outbreak using Caputo-Fabrizio fractional differential equation. AIMS Mathematics, 2023, 8(2): 2720-2735. doi: 10.3934/math.2023143 |
[7] | A. E. Matouk, Ismail Gad Ameen, Yasmeen Ahmed Gaber . Analyzing the dynamics of fractional spatio-temporal $ \mathrm{SEIR} $ epidemic model. AIMS Mathematics, 2024, 9(11): 30838-30863. doi: 10.3934/math.20241489 |
[8] | Hongyan Wang, Shaoping Jiang, Yudie Hu, Supaporn Lonapalawong . Analysis of drug-resistant tuberculosis in a two-patch environment using Caputo fractional-order modeling. AIMS Mathematics, 2024, 9(11): 32696-32733. doi: 10.3934/math.20241565 |
[9] | Shao-Wen Yao, Muhammad Farman, Maryam Amin, Mustafa Inc, Ali Akgül, Aqeel Ahmad . Fractional order COVID-19 model with transmission rout infected through environment. AIMS Mathematics, 2022, 7(4): 5156-5174. doi: 10.3934/math.2022288 |
[10] | Mohammad Sajid, Biplab Dhar, Ahmed S. Almohaimeed . Differential order analysis and sensitivity analysis of a CoVID-19 infection system with memory effect. AIMS Mathematics, 2022, 7(12): 20594-20614. doi: 10.3934/math.20221129 |
Several newly nonlinear models for describing dynamics of COVID-19 pandemic have been proposed and investigated in literature recently. In light of these models, we attempt to reveal the role of fractional calculus in describing the growth of COVID-19 dynamics implemented on Saudi Arabia's society over 107 days; from 17 Dec 2020 to 31 March 2021. Above is achieved by operating two fractional-order differential operators, Caputo and the Caputo-Fabrizio operators, instead of the classical one. One of expanded SEIR models is utilized for achieving our purpose. With the help of using the Generalized Euler Method (GEM) and Adams-Bashforth Method (ABM), the numerical simulations are performed respectively in view of the Caputo and Caputo-Fabrizio operators. Accordance with said, the stability analysis of the two proposed fractional-order models is discussed and explored in view of obtaining the equilibrium points, determining the reproductive number (R0) and computing the elasticity indices of R0. Several numerical comparisons reveal that the fractional-order COVID-19 models proposed in this work are better than that of classical one when such comparisons are performed between them and some real data collected from Saudi Arabia's society. This inference together with the cases predictions that could easily deduced from the proposed fractional-order models can allow primary decision makers and influencers to set the right plans and logic strategies that should be followed to face this pandemic.
Throughout the ages, the world has witnessed the spread of various diseases. It claimed the lives of many, but soon faded by finding an effective and rapid vaccine. Recently, specifically March 11, 2020, the World Health Organization (WHO) announced the outbreak of the Corona pandemic globally [1]. During that period till now, the number of deaths has exceeded the barrier of 2 million deaths. The world was forced to follow a lot of precautionary laws such as imposing quarantine and using masks and sanitizers. Eventually, several vaccines of different efficacy were reached.
From the point of view of mathematicians, it was necessary to take slightly different approach and to have a mathematical model describing the development of COVID-19 disease and the effect of different vaccinations to ensure complete immunity, (see [2,3,4,5,6,7]). In fact, the Susceptible-Exposed-Infectious-Removed model (or simply SEIR model) is considered one of the most popular mathematical models that used to describe the pandemic dynamics and to estimate feasible infection scenarios. The SEIR model might be very helpful to evaluate the efficiency of several actions, such as lock-down, since the infectious disease outbreak. This model relies typically on a set of ordinary differential equations that take the population's amount into account subject to infection, the direction of persons who recover after infection over a given time, and the persons who unfortunately die.
The extended SEIR model that considered here involves seven classes: Susceptible, exposed, infectious, quarantined, recovered, deaths, and vaccinated classes. As a matter of fact, this model has been more recently established by Ghostine et al. in their paper [23]. All parameters' values are already estimated based on a real data collected for forecasting the COVID-19 pandemic in Saudi Arabia. However, this research focuses mainly on studying the effect of this model when converting its ordinary derivatives to its fractional-order case. In particular, the expanded SEIR models established in [23] will be fractionalized using two fractional-order differential operators; Caputo and Caputo-Fabrizio operators. The Generalized Euler Method (GEM) and Adams-Bashforth Method (ABM) are then implemented, respectively, to deal with the Caputo fractional-order COVID-19 model and the Caputo-Fabrizio fractional-order COVID-19 model, and consequently to clarify the interaction of the targeted vaccine with the disease and its immunological aspect. In summary, the role of fractional calculus in describing the growth of COVID-19 dynamics on Saudi Arabia's society from 17 Dec 2020 to 31 March 2021 will be clearly revealed, where the Saudi Minister of Health (Tawfiq Al-Rabiah) received the first dose of the available coronavirus vaccine at the beginning of that period. Besides, the equilibrium of the fractional-order models, the basic reproductive number R0 with its elasticity indices of the COVID-19 model will be also determined and documented in view of some mathematical analysis.
The main contribution that can be gained from performing this study is to provide an insight into the influence of fractional calculus in describing the growth of COVID-19 dynamics implemented on Saudi Arabia's society over 107 days; from 17 Dec 2020 to 31 March 2021. In particular, it will be revealed via certain numerical comparisons that the fractional-order COVID-19 models will be better than that of traditional one when such comparisons are performed between them and some real data collected from Saudi Arabia's society. This conclusion will undoubtedly enable mathematicians to predict the number of infected cases that may be correctly detected in the whole of society, and hence allow decision makers and influencers to set the right plans and logic strategies that should be followed to face this pandemic.
The rest of this paper is structured as follows: In the closest section, some of essential definitions, concepts and notions related to fractional calculus are inserted. Whereas in Section 3, an overview of the model configuration is given, as it reported in reference [23]. The fractional-order COVID-19 models are established in Section 4. Whereas some stability analysis related to the proposed models are discussed in Section 5. Finally, some comments and concluding remarks on what have been implemented in this research can be found in the conclusion section.
A set of essential definitions and basic facts related to the fractional calculus are addressed in this section for full explanation.
Definition 2.1. [8] (Riemann-Liouville integrator) Suppose f∈L1[a,b], the Riemann-Liouville fractional-order integral operator is defined by:
Jqf(t)=1Γ(q)∫ta (t−x)q−1f(x)dx, | (2.1) |
where q∈R+, a≤t≤b.
Definition 2.2. [9] (Caputo Differentiator) Suppose 0≤n−1<q<n∈Z+, the Caputo fractional-order derivative operator is defined by:
Dqf(t)=1Γ(n−q)∫ta (t−x)n−q−1f(n)(x)dx. | (2.2) |
Definition 2.3. [10] (Caputo-Fabrizio Differentiator) Suppose 0<q<1 and L(q) is the normalized function in which L(0)=L(1)=1. The Caputo-Fabrizio fractional-order differential operator Dq∗ of a function f∈H1(a,b) is defined by:
Dq∗f(t)=L(q)1−q∫ta f′(x)exp(−qt−x1−q)dx. | (2.3) |
If f∉H1(a,b), then such operator is defined by:
Dq∗f(t)=qL(q)1−q∫tα (f(t)−f(x))exp(−qt−x1−q)dx. | (2.4) |
Remark 2.1. [11] If μ=1−qq∈[0,∞), (i.e. q=11+μ∈[0,1]), then (2.3) could be rewritten in the following form:
Dq∗f(t)=L(q)μ∫tα f′(x)exp(−t−xμ)dx, | (2.5) |
where L(0) = L(∞)=1 and
limμ→01μexp(−t−xμ)=δ(x−t). | (2.6) |
Definition 2.4. [12,13] (Caputo-Fabrizio integrator) The corresponding Caputo-Fabrizio fractional-order integral operator Jq∗ of the operator Dq∗ for a function f can be expressed as follows:
Jq∗f(t)=2(1−q)(2−q)L(q)+2q(2−q)L(q)∫t0 f(x)dx, | (2.7) |
where 0<q<1 and t≥0.
Remark 2.2. [12,13] From Definition 2.4, we can get the following confirmation:
L(q)=2(2−q), for 0<q<1. | (2.8) |
Based on Remark 2.2, modern version of the parameter has been created as:
Jq∗f(t)=2(1−q)(2−q)L(q)+2q(2−q)L(q)∫t0 f(x)dx, | (2.9) |
where 0<q<1.
When studying and analyzing the spread out of infectious diseases and the ability to control them, mathematical modeling come as an effective tool that could be employed to provide better support of control strategies development which should be followed to face these diseases. Several studies of SEIR epidemiological models, which are commonly used for the COVID-19 pandemic, have made minor or major changes to the classical SEIR model. Extremely significant mathematical models have been developed to study the dynamics transmission of the COVID-19 pandemic, and to illustrate their relations by structuring them into inputs and outputs, which, in their role, can show the pandemic's impact on the whole population. The compartment diagram of the expanded SEIR model established in [23] can be shown in Figure 1. It exhibits the growth of COVID-19 dynamics on Saudi Arabia's society from 17 Dec 2020 to 31 March 2021, where the population has been divided into the following classes: Susceptible (S), vaccinated (V), exposed (E), infected (I), recovered (R), quarantined (Q), and dead (D).
In order to gain more depth in understanding these model and their impact on reality, it is useful to set the initial values of the model's states (S0,V0,E0,I0,R0,D0) as well as all the parameters' values. In particular, the initial values of the model's states can be listed, as reported in [14,15,18], in Table 1, where S0=ℵ−V0−E0−I0−R0−D0, so that ℵ represents the size of population. On the other hand, the parameters' values of the model at hand can be shown in Table 2 [23].
Variable of compartment | Value |
ℵ | 3.4218×107 |
E(0) | 0 |
V(0) | 1 |
I(0) | 174 |
R(0) | 208 |
Q(0) | 10 |
D(0) | 10 |
Parameters | Description | Initial Value | References |
Λ | New births and new residents | 2300 | [14] |
β1 | Transmission rate before intervention | 8.58×10−9 | assumed |
β2 | Transmission rate during and after intervention | 3.43×10−9 | assumed |
α | Vaccination rate | 3.5×10−4 | [15] |
μ | Natural death rate | 3×10−5 | [14] |
1/γ | Incubation period | 5.5 days | [16] |
σ | Vaccine inefficacy | 0.05 | [17] |
1/δ | Infection time | 3.8 days | [16] |
κ | Case fatality rate | 0.014 | [18] |
1/λ | Recovery time | 10 days | [16] |
1/ρ | Time until death | 15 days | [16] |
Generally, the Ordinary Differential Equations (ODEs) applied to expound the extended SEIR model with the vaccination impact can be given by the following system [23]:
dSdt=Λ−(βI(t)−α−μ)S(t),dEdt=(βS(t)+σβV(t))I(t)−(γ+μ)E(t),dIdt=μE(t)−(δ+μ)I(t),dQdt=δI(t)−((1−κ)λ+ρκ+μ)Q(t),dRdt=(1−κ)λQ(t)−μR(t),dDdt=ρκQ(t),dVdt=αS(t)−(σβI(t)+μ)V(t), | (3.1) |
where t≥0, and the initial conditions S(0)=S0, V(0)=V0, I(0)=I0, Q(0)=Q0, D(0)=D0, E(0)=E0 and R(T)=R0.
In this section, we aim to propose two new versions of the extended SEIR model given in [23]. These two versions will be established by operating the Caputo and the Caputo-Fabrizio fractional-order operators instead of the ordinary operator applied to the model at hand. Below, these two versions are clearly listed.
● COVID-19 model in Caputo sense
dqSdt=Λ−(βI(t)−α−μ)S(t),dqEdt=(βS(t)+σβV(t))I(t)−(γ+μ)E(t),dqIdt=μE(t)−(δ+μ)I(t),dqQdt=δI(t)−((1−κ)λ+ρκ+μ)Q(t),dqRdt=(1−κ)λQ(t)−μR(t),dqDdt=ρκQ(t),dqVdt=αS(t)−(σβI(t)+μ)V(t). | (4.1) |
● COVID-19 model in Caputo-Fabrizio sense
dq∗Sdt=Λ−(βI(t)−α−μ)S(t),dq∗Edt=(βS(t)+σβV(t))I(t)−(γ+μ)E(t),dq∗Idt=μE(t)−(δ+μ)I(t),dq∗Qdt=δI(t)−((1−κ)λ+ρκ+μ)Q(t),dq∗Rdt=(1−κ)λQ(t)−μR(t),dq∗Ddt=ρκQ(t),dq∗Vdt=αS(t)−(σβI(t)+μ)V(t). | (4.2) |
Of course, the above two fractional-order models are established subject to the initial conditions given in Table 1. It is worth noting that the GEM has been successfully employed to solve the fractional-order system (4.1), while the ABM has confirmed its ability in providing an efficient numerical solution of system (4.2). The reader may understand the merits of these two methods and the modality to use them in various researches by referring to the references [19,20]. However, in order to see the numerical solutions of system (4.1) and system (4.2), the GEM and ABM are respectively implemented to produce Figures 2 and 3 according to different values of α. Actually, these two simulations represent the size of all classes over the time for system (4.1) and system (4.2) in view of different values of α.
For the purpose of investigating the fractional-order COVID-19 models, certain numerical comparisons are carried out between the two vector-valued solutions of systems (4.1) and (4.2). Such comparisons are performed by considering the initial values provided in Table 1, and the same values of parameters provided in Table 2. The whole numerical simulations of these comparisons are exhibited in Figure 4. The executed graphs compare the number of each class of the whole compartments' classes based on simulating all dynamics of the two proposed systems (system (4.1) and system (4.2)) from 0 to 500 day. It should be mentioned that the behaviour of the two proposed systems' dynamics are likewise and close in terms of results. Overall, there is a steadily increase of the recovered and vaccinated people over the period given. On contrary, there is a steadily decrease of susceptible people over the same period. For quarantined, infectious and exposed cases, it can be observed that such cases began with a few persons and then climbed dramatically to the top in the middle of the period. From that point, they have fluctuated in gradual downward trends to same of beginning at the end of period.
This section is devoted to exploring some mathematical aspects related to fractional-order of the COVID-19 model with its two types Caputo operator (4.1) and Caputo-Fabrizio operator (4.2) and deducing results of the stability analysis. Which is represented in discussing the equilibrium points and the non-negative solution such system, calculating the basic reproductive of and its elasticity indices.
In order to obtain epidemiological stability of the COVID-19 model, it is necessary to determine its equilibrium points. In fact, there are generally two types of equilibrium points of the epidemiological models. The first one is the Disease-Free Equilibrium (TDFE) point that typically occurs when assuming I=0, and equating the left-hand side of the considered system to zero. For instance, one can deduce the following point:
TDFE=(Λμ+α,0,0,0,0,0,αΛμ+α). | (5.1) |
On the other hand, the second type of equilibrium points is the Endemic Equilibrium (TEE) point. Such point can be yielded by setting I≠0, and then equating the left-hand side of the system to zero too. In particular, the TEE point of the system at hand can be expressed as follows:
TEE=(S∗,E∗,I∗,Q∗,D∗,V∗), | (5.2) |
where
E∗=FγI∗, Q∗=δGI∗, S∗=ΛβI∗+N, V∗=ΛγβI+Λαγ−MF(βI+N)Iγμ(βI+N), | (5.3) |
N=α+μ,M=γ+μ, F=δ+μ, G=μ+λ(1−κ)+ρκ,α2I∗2+α1I∗+α0=0, | (5.4) |
and where
α0=μNMF(1−R0), α2=μNMF+μMF+Λγβ2σ, α3=βMF. | (5.5) |
In light of obtaining equilibrium points of the system at hand, we can find that the so-called basic reproductive number R0, which can be commonly fruitful in guiding various monitor strategies to face the pandemic in all. This is, actually, what we will discuss and present in the upcoming subsection.
Epidemiology defines the basic reproductive number R0 as the number of infections caused by the first disease case, that appeared in an appointed population where assume everyone is susceptible to infection. The importance of R0 lies in knowing the rapidity of the spread of the emerging disease among the inhabitants and the proportion of the population to be immunized. To be precise, the population spread of the epidemic will occur when R0>1, where it is difficult to control. The method to numerate the basic reproductive ratio by finding the spectral radius of the next generation matrix Y (i.e. R0=ρ(Y)). The matrix Y is a multiplication of F by W−1, where:
F=[∂Fi(TDFE )∂tj] and W=[∂Wi(TDFE )∂tj], | (5.6) |
where Fi refers to the stream of freshly infected cases into compartment tj, and Wi refers to the entering/leaving streams connected with tj, for i,j=1,2,3,...,m such that m is the total of compartments demonstrated in the model. Based on the aforesaid argument, one might calculate R0 for any the fractional-order models ((4.1) or (4.2)) by first obtaining the two primary matrices F and W. These matrices have the following forms:
F=[0β(S0+σV0)00] and W=[μ+γ0−γμ+δ]. | (5.7) |
In this regard, the basic reproductive number R0 can be then calculated to be as follows:
R0=βγΛ(μ+ασ)μ(μ+γ)(μ+δ)(μ+α). | (5.8) |
With the help of the above resultant relation, it is possible to estimate the epidemiological situation in the community as a whole [21,22], where the value of R0 is deemed very important in medical sense. In particular, an epidemic is predictable climb up if R0>1, and to end if R0<1 [21]. Along the same lines, the aforementioned discussion lays the foundation to explore further result connected with the local stability analysis of the TDFE point. This result is stated and derived below.
Theorem 5.1. The disease-free equilibrium point TDFE of the systems (4.1) and (4.2) is locally stable if R0<1, and unstable if R0>1.
Proof. It is possible to express systems (4.1) and (4.2) more clearly with the Jacobin matrix by deleting the equation expressing the death compartment (D) where its importance appears only in the following differential equation:
D=N−S−E−I−Q−R−V. | (5.9) |
The Jacobian matrix of the reduced model at TDFE point is then given by:
J(TDFE)=(−N0−βS00000−Mβ(S0+σW0)0000γ−F00000δ−G00000λ(1−κ)−μ0α0−σβW000−μ). | (5.10) |
The eigenvalues of the above matrix will be then as:
λ1=−N,λ2=−G,λ3=λ4=−μ, |
λ5=−12(M+F+√(M−F)2+4MFR0), |
λ6=−12(M+F−√(M−F)2+4MFR0). |
Obviously, we have λi<0, ∀i=1,2,3,4. At the same time, if one assumes R0<1, then λ5<0, and λ6<0. Thus, due to all eigenvalues of the matrix are negative, then the TDFE point is locally asymptotically stable. On the contrary, if one assumes R0>1, then we have λ5<0 and λ6>0, which leads to assert that the TDFE point is locally asymptotically unstable.
In order to evaluate the performance of the two fractional-order systems (systems (4.1) and (4.2)) and their impacts, we will make certain predictions through using such proposed systems based on some numerical comparisons that have been performed between their dynamics and some real data collected from Saudi Arabia's society over 107 days; from 17 Dec 2020 to 31 March 2021 [14,15,18]. Actually, these numerical comparisons are deemed one of the main focuses of this research, as they clearly show the agreement of the numbers of people recovering from the COVID-19 disease with the results of the two fractional-order epidemiological models proposed previously. In other words, Figure 5 reveals that the fractional-order COVID-19 models given in systems (4.1) and (4.2) are better than the traditional system (3.1) especially when we make a comparison between their dynamics and certain real data collected throughout the aforesaid period. In particular, we note that those data aggregate and come closer to the recovering cases curve for systems (4.1) and (4.2) more than they aggregate and come closer to the recovering cases curve for system (3.1). It means that the fractional-order systems (4.1) and (4.2) formulated at α=0.9 have proved their efficiency in describing the dynamics of the recovery cases against the integer-order system (3.1). This outcome will undoubtedly enable specialists to predict the number of infected cases that may be correctly detected in the whole of society, and hence allow decision makers and influencers to set the right plans and logic strategies that should be followed to face this pandemic.
Among the measures of control that have an effect on the transmission of the disease are the so-called sensitivity and elasticity indices. The sensitivity index of R0 with respect to a parameter τ is ∂R0∂σ, whereas the elasticity index of R0, which measures the relative change of R0 with respect to τ, denoted by χR0τ, and defined as:
χR0τ=∂R0∂ττR0. | (5.11) |
As a result of an explanation, the sign of the elasticity indicator shows a decrease or increase in R0. By knowing the value of R0, it is easy to infer and accurately calculate the elasticity index of each parameter. The magnitude of the elasticity indices depends generally on the parameter values found in the expression of R0. These values can be mostly determined by adopting certain methods of estimation. According to the parameters' values presented in Table 2, the baseline parameters' values and their corresponding elasticity indices of R0, which are calculated based on the relation (5.11), are reported in Table 3. For further illustration, these indices can be shown, at the same time, in the bar graph given in Figure 6.
Parameter | Elasticity index | Numerical value |
Λ | χR0Λ | 1 |
β | χR0β | 1 |
α | χR0α | −0.552632 |
μ | χR0μ | −0.447647 |
γ | χR0γ | 1.64973×10−4 |
δ | χR0δ | −0.999886 |
σ | χR0σ | −0.368421 |
In light of the above drawings and results, it appears notably that the two parameters Λ and β are the most influential in calculating R0. That is, the higher the values of these two parameters, the higher the number of people infected with COVID-19. In contrast, the parameter δ is appeared as the least influential. To see how such three parameters impact on R0, we plot Figure 7. In particular, based on Figure 7(a, b), we can observe that when the two parameter's values of λ and β are increased, the value of R0 will be so, indicating that the ratios of the new births and new resident as well as the ratio of the transmission before intervention are the most impactful on the society in terms of they have an ability to yield an pandemic in all. On the contrary, when the value of the parameter δ is decreased, the value of R0 will be so, as exhibited in Figure 7(c). This, actually, indicates that the ratio of infection time is the slightest impactful on the society.
In this work, two newly nonlinear fractional-order COVID-19 models have been proposed and investigated to describe the growth of COVID-19 dynamics implemented on Saudi Arabia's society over 107 days; from 17 Dec 2020 to 31 March 2021. In particular, these two models have been proposed in view of the Caputo and the Caputo-Fabrizio operators. The Generalized Euler Method (GEM) and the Adams-Bashforth Method (ABM) have been successfully implemented to simulate the dynamics of the established models, where the stability analysis of the such models has been discussed and exmined in light of finding the equilibrium points, determining the reproductive number (R0) and computing the elasticity indices of R0. It has been demonstrated, through some numerical comparisons, that the fractional-order COVID-19 models are better than the classical one due to what these models have presented in comparison with some real data collected from Saudi Arabia's society. In summary, the proposed fractional-order models allow specialists to predict the growth of the COVID-19 dynamics more efficiency than the integer-order one allows. This conclusion can enable primary decision makers and influencers to set the right plans and logic strategies that should be followed to face this pandemic.
All authors declare that they have no conflicts of interest.
[1] | Coronavirus Disease (COVID-19) Outbreak Situation, World Health Organization (WHO), 2020. Available from: https://www.who.int/emergencies/diseases/novel-coronavirus-2019. |
[2] | R. B. Albadarneh, I. M. Batiha, A. Ouannas, S. Momani, Modeling COVID-19 pandemic outbreak using fractional-order systems, Int. J. Math. Comput. Sci., 16 (2021), 1405–1421. |
[3] |
A. Moussaoui, P. Auger, Prediction of confinement effects on the number of COVID-19 outbreak in Algeria, Math. Model. Nat. Phenom., 15 (2020), 1–15. https://doi.org/10.1051/mmnp/2020028 doi: 10.1051/mmnp/2020028
![]() |
[4] | F. Farooq, J. Khan, M. U. G. Khan, Effect of Lockdown on the spread of COVID-19 in Pakistan, arXiv, 2020. Available from: https://arXiv.org/abs/2005.09422. |
[5] |
A. M. Ramos, M. Vela-Pérez, M. R. Ferrández, A. B. Kubik, B. Ivorra, Modeling the impact of SARS-CoV-2 variants and vaccines on the spread of COVID-19, Commun. Nonlinear Sci. Numer. Simul., 102 (2021), 105937. https://doi.org/10.1016/j.cnsns.2021.105937 doi: 10.1016/j.cnsns.2021.105937
![]() |
[6] |
M. A. Acuña-Zegarra, S. Díaz-Infante, D. Baca-Carrasco, D. Olmos-Liceaga, COVID-19 optimal vaccination policies: A modeling study on efficacy, natural and vaccine-induced immunity responses, Math. Biosci., 337 (2021), 108614. https://doi.org/10.1016/j.mbs.2021.108614 doi: 10.1016/j.mbs.2021.108614
![]() |
[7] |
C. A. Varotsos, V. F. Krapivin, A new model for the spread of COVID-19 and the improvement of safety, Saf. Sci., 132 (2020), 104962. https://doi.org/10.1016/j.ssci.2020.104962 doi: 10.1016/j.ssci.2020.104962
![]() |
[8] |
R. B. Albadarneh, I. M. Batiha, A. Adwai, N. tahat, A. K. Alomari, Numerical approach of Riemann-Liouville fractional derivative operator, Int. J. Electr. Comput. Eng., 11 (2021), 5367–5378. http://doi.org/10.11591/ijece.v11i6.pp5367-5378 doi: 10.11591/ijece.v11i6.pp5367-5378
![]() |
[9] |
R. B. Albadarneh, I. M. Batiha, A. K. Alomari, N. Tahat, Numerical approach for approximating the Caputo fractional-order derivative operator, AIMS Math., 6 (2021), 12743–12756. https://doi.org/10.3934/math.2021735 doi: 10.3934/math.2021735
![]() |
[10] |
M. Caputo, M. Fabrizio, On the notion of fractional derivative and applications to the hysteresis phenomena, Meccanica, 52 (2017), 3043–3052. https://doi.org/10.1007/s11012-017-0652-y doi: 10.1007/s11012-017-0652-y
![]() |
[11] | J. Losada, J. J. Nieto, Properties of a new fractional derivative without singular Kernel, Progr. Fract. Differ. Appl., 1 (2015), 87–92. |
[12] |
K. Altaf, A. Atangana, Dynamics of Ebola disease in the framework of different fractional derivatives, Entropy, 21 (2019), 303. https://doi.org/10.3390/e21030303 doi: 10.3390/e21030303
![]() |
[13] |
S. A. Khan, K. Shah, G. Zaman, F. Jarad, Existence theory and numerical solutions to smoking model under Caputo-Fabrizio fractional derivative, Chaos, 29 (2019), 013128. https://doi.org/10.1063/1.5079644 doi: 10.1063/1.5079644
![]() |
[14] | Saudi Ministry of Health, Saudi Ministry of Health, 2020. Available from: https://www.moh.gov.sa/en/Ministry/Statistics/ Indicator/Pages/Indicator-1440.aspx. |
[15] | Saudi Health Council, Saudi Health Council, 2021. Available from: https://coronamap.sa. |
[16] | G. Evensen, J. Amezcua, M. Bocquet, A. Carrassi, A. Farchi, A. Fowler, et al., An international assessment of the COVID-19 pandemic using ensemble data assimilation, medRxiv, 2020. https://doi.org/10.1101/2020.06.11.20128777 |
[17] |
F. P. Polack, S. J. Thomas, N. Kitchin, J. Absalon, A. Gurtman, S. Lockhart, et al., Safety and efficacy of the BNT162b2 mRNA COVID-19 vaccine, N. Engl. J. Med., 29 (2020), 2603–2615. https://doi.org/10.1056/NEJMoa2034577 doi: 10.1056/NEJMoa2034577
![]() |
[18] | Saudi Center for Diseases Prevention and Control, 2020. Available from: https://covid19.cdc.gov.sa/daily-updates. |
[19] |
Y. Y. Yameni Noupoue, Y. Tandoǧdu, M. Awadalla, On numerical techniques for solving the fractional logistic differential equation, Adv. Differ. Equ., 108 (2019), 108. https://doi.org/10.1186/s13662-019-2055-y doi: 10.1186/s13662-019-2055-y
![]() |
[20] |
J. Peinado, J. Ibáñez, E. Arias, V. Hernández, Adams-Bashforth and Adams-Moulton methods for solving differential Riccati equations, Comput. Math. Appl., 60 (2010), 3032–3045. https://doi.org/10.1016/j.camwa.2010.10.002 doi: 10.1016/j.camwa.2010.10.002
![]() |
[21] |
P. van den Driessche, J. Watmough, eproduction numbers and sub-threshold endemic equilibria for compartmental models of disease transmission, Math. Biosci., 108 (2002), 29–48. https://doi.org/10.1016/S0025-5564(02)00108-6 doi: 10.1016/S0025-5564(02)00108-6
![]() |
[22] |
O. Diekmann, J. A. P Heesterbeek, J. A. J. Metz, On the definition and the computation of the basic reproduction ratio R0 in models for infectious diseases in heterogeneous populations, J. Math. Biol., 28 (1990), 365–382. https://doi.org/10.1007/BF00178324 doi: 10.1007/BF00178324
![]() |
[23] |
R. Ghostine, M. Gharamti, S. Hassrouny, I. Hoteit, An extended SEIR model with vaccination for forecasting the COVID-19 pandemicin Saudi Arabia using an ensembleKalman filter, Mathematics, 9 (2021), 636. https://doi.org/10.3390/math9060636 doi: 10.3390/math9060636
![]() |
1. | Isra Al-Shbeil, Noureddine Djenina, Ali Jaradat, Abdallah Al-Husban, Adel Ouannas, Giuseppe Grassi, A New COVID-19 Pandemic Model including the Compartment of Vaccinated Individuals: Global Stability of the Disease-Free Fixed Point, 2023, 11, 2227-7390, 576, 10.3390/math11030576 | |
2. | Victoria May P. Mendoza, Renier Mendoza, Youngsuk Ko, Jongmin Lee, Eunok Jung, Managing bed capacity and timing of interventions: a COVID-19 model considering behavior and underreporting, 2022, 8, 2473-6988, 2201, 10.3934/math.2023114 | |
3. | Amer Dababneh, Noureddine Djenina, Adel Ouannas, Giuseppe Grassi, Iqbal M. Batiha, Iqbal H. Jebril, A New Incommensurate Fractional-Order Discrete COVID-19 Model with Vaccinated Individuals Compartment, 2022, 6, 2504-3110, 456, 10.3390/fractalfract6080456 | |
4. | Mohammed Subhi Hadi, Bülent Bilgehan, Fractional COVID-19 Modeling and Analysis on Successive Optimal Control Policies, 2022, 6, 2504-3110, 533, 10.3390/fractalfract6100533 | |
5. | Victoria May P. Mendoza, Renier Mendoza, Jongmin Lee, Eunok Jung, Adjusting non-pharmaceutical interventions based on hospital bed capacity using a multi-operator differential evolution, 2022, 7, 2473-6988, 19922, 10.3934/math.20221091 | |
6. | Abdallah Al-Husban, Noureddine Djenina, Rania Saadeh, Adel Ouannas, Giuseppe Grassi, A New Incommensurate Fractional-Order COVID 19: Modelling and Dynamical Analysis, 2023, 11, 2227-7390, 555, 10.3390/math11030555 | |
7. | Iqbal M. Batiha, Ahmad Obeidat, Shameseddin Alshorm, Ahmed Alotaibi, Hajid Alsubaie, Shaher Momani, Meaad Albdareen, Ferjeni Zouidi, Sayed M. Eldin, Hadi Jahanshahi, A Numerical Confirmation of a Fractional-Order COVID-19 Model’s Efficiency, 2022, 14, 2073-8994, 2583, 10.3390/sym14122583 | |
8. | Nabila Barrouk, Iqbal M. Batiha, Adel Ouannas, An investigation of global existence of the solution of fractional reaction-diffusion system , 2023, Accepted, 1450-5444, 10.30755/NSJOM.15045 | |
9. | Shaher Momani, Noureddine Djenina, Adel Ouannas, Iqbal M. Batiha, Stability Results for Nonlinear Fractional Differential Equations with Incommensurate Orders, 2024, 58, 24058963, 286, 10.1016/j.ifacol.2024.08.204 | |
10. | Noureddine Djenina, Adel Ouannas, 2023, Chapter 9, 978-3-031-37969-7, 147, 10.1007/978-3-031-37970-3_9 | |
11. | U. K. Nwajeri, S. E. Fadugba, E. O. Ohaeri, K. I. Oshinubi, R. R. Ogunrinde, R. B. Ogunrinde, Co-dynamic Model of Drug Trafficking and Money Laundering Coupled with Fractional Derivative, 2023, 9, 2349-5103, 10.1007/s40819-023-01539-x | |
12. | R.P. Chauhan, Anil Kumar, Sunil Kumar, 2025, 9780443288142, 81, 10.1016/B978-0-44-328814-2.00010-2 |
Parameters | Description | Initial Value | References |
Λ | New births and new residents | 2300 | [14] |
β1 | Transmission rate before intervention | 8.58×10−9 | assumed |
β2 | Transmission rate during and after intervention | 3.43×10−9 | assumed |
α | Vaccination rate | 3.5×10−4 | [15] |
μ | Natural death rate | 3×10−5 | [14] |
1/γ | Incubation period | 5.5 days | [16] |
σ | Vaccine inefficacy | 0.05 | [17] |
1/δ | Infection time | 3.8 days | [16] |
κ | Case fatality rate | 0.014 | [18] |
1/λ | Recovery time | 10 days | [16] |
1/ρ | Time until death | 15 days | [16] |
Parameter | Elasticity index | Numerical value |
Λ | χR0Λ | 1 |
β | χR0β | 1 |
α | χR0α | −0.552632 |
μ | χR0μ | −0.447647 |
γ | χR0γ | 1.64973×10−4 |
δ | χR0δ | −0.999886 |
σ | χR0σ | −0.368421 |
Variable of compartment | Value |
ℵ | 3.4218×107 |
E(0) | 0 |
V(0) | 1 |
I(0) | 174 |
R(0) | 208 |
Q(0) | 10 |
D(0) | 10 |
Parameters | Description | Initial Value | References |
Λ | New births and new residents | 2300 | [14] |
β1 | Transmission rate before intervention | 8.58×10−9 | assumed |
β2 | Transmission rate during and after intervention | 3.43×10−9 | assumed |
α | Vaccination rate | 3.5×10−4 | [15] |
μ | Natural death rate | 3×10−5 | [14] |
1/γ | Incubation period | 5.5 days | [16] |
σ | Vaccine inefficacy | 0.05 | [17] |
1/δ | Infection time | 3.8 days | [16] |
κ | Case fatality rate | 0.014 | [18] |
1/λ | Recovery time | 10 days | [16] |
1/ρ | Time until death | 15 days | [16] |
Parameter | Elasticity index | Numerical value |
Λ | χR0Λ | 1 |
β | χR0β | 1 |
α | χR0α | −0.552632 |
μ | χR0μ | −0.447647 |
γ | χR0γ | 1.64973×10−4 |
δ | χR0δ | −0.999886 |
σ | χR0σ | −0.368421 |