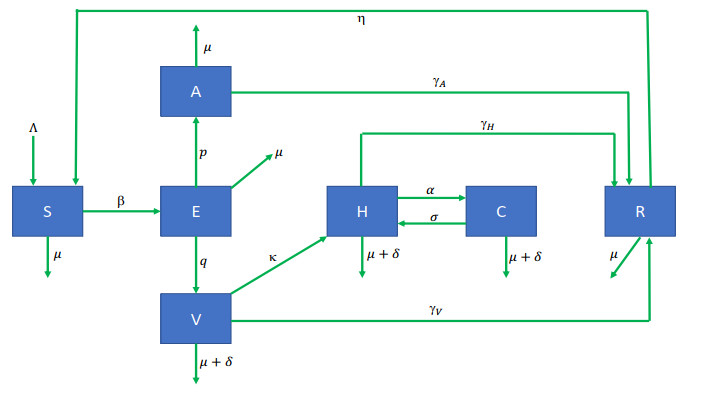
The aim of this work is to provide a new mathematical model that studies transmission dynamics of Coronavirus disease 2019 (COVID-19) caused by severe acute respiratory syndrome coronavirus 2 (SARS-CoV-2). The model captures the dynamics of the disease taking into consideration some measures and is represented by a system of nonlinear ordinary differential equations including seven classes, which are susceptible class (S), exposed class (E), asymptomatic infected class (A), severely infected class (V), hospitalized class (H), hospitalized class but in ICU (C) and recovered class (R). We prove positivity and boundedness of solutions, compute the basic reproduction number, and investigate asymptotic stability properties of the proposed model. As a consequence, dynamical properties of the model are established fully and some mitigation and prevention measures of COVID-19 outbreaks are also suggested. Furthermore, the model is fitted to COVID-19 confirmed cases in South Africa during the Omicron wave from November 27, 2021 to January 20, 2022 which helped determine the model parameters value for our numerical simulation. A set of numerical experiments using real data is conducted to support and illustrate the theoretical findings. Numerical simulation results show that fast waning of infection-induced immunity can increase the occurrence of outbreaks.
Citation: Oluwaseun F. Egbelowo, Justin B. Munyakazi, Manh Tuan Hoang. Mathematical study of transmission dynamics of SARS-CoV-2 with waning immunity[J]. AIMS Mathematics, 2022, 7(9): 15917-15938. doi: 10.3934/math.2022871
[1] | C. W. Chukwu, Fatmawati . Modelling fractional-order dynamics of COVID-19 with environmental transmission and vaccination: A case study of Indonesia. AIMS Mathematics, 2022, 7(3): 4416-4438. doi: 10.3934/math.2022246 |
[2] | Ahmed M. Elaiw, Amani S. Alsulami, Aatef D. Hobiny . Global properties of delayed models for SARS-CoV-2 infection mediated by ACE2 receptor with humoral immunity. AIMS Mathematics, 2024, 9(1): 1046-1087. doi: 10.3934/math.2024052 |
[3] | Tahir Khan, Fathalla A. Rihan, Muhammad Bilal Riaz, Mohamed Altanji, Abdullah A. Zaagan, Hijaz Ahmad . Stochastic epidemic model for the dynamics of novel coronavirus transmission. AIMS Mathematics, 2024, 9(5): 12433-12457. doi: 10.3934/math.2024608 |
[4] | A. M. Elaiw, A. S. Shflot, A. D. Hobiny . Stability analysis of SARS-CoV-2/HTLV-I coinfection dynamics model. AIMS Mathematics, 2023, 8(3): 6136-6166. doi: 10.3934/math.2023310 |
[5] | Maryam Amin, Muhammad Farman, Ali Akgül, Mohammad Partohaghighi, Fahd Jarad . Computational analysis of COVID-19 model outbreak with singular and nonlocal operator. AIMS Mathematics, 2022, 7(9): 16741-16759. doi: 10.3934/math.2022919 |
[6] | S. M. E. K. Chowdhury, J. T. Chowdhury, Shams Forruque Ahmed, Praveen Agarwal, Irfan Anjum Badruddin, Sarfaraz Kamangar . Mathematical modelling of COVID-19 disease dynamics: Interaction between immune system and SARS-CoV-2 within host. AIMS Mathematics, 2022, 7(2): 2618-2633. doi: 10.3934/math.2022147 |
[7] | Afnan Al Agha, Hakim Al Garalleh . Oncolysis by SARS-CoV-2: modeling and analysis. AIMS Mathematics, 2024, 9(3): 7212-7252. doi: 10.3934/math.2024351 |
[8] | Sara J Hamis, Fiona R Macfarlane . A single-cell mathematical model of SARS-CoV-2 induced pyroptosis and the effects of anti-inflammatory intervention. AIMS Mathematics, 2021, 6(6): 6050-6086. doi: 10.3934/math.2021356 |
[9] | Kai Zhang, Xinzhu Meng, Abdullah Khames Alzahrani . The ACE2 receptor protein-mediated SARS-CoV-2 infection: dynamic properties of a novel delayed stochastic system. AIMS Mathematics, 2024, 9(4): 8104-8133. doi: 10.3934/math.2024394 |
[10] | Muhammad Altaf Khan, Sajjad Ullah, Saif Ullah, Muhammad Farhan . Fractional order SEIR model with generalized incidence rate. AIMS Mathematics, 2020, 5(4): 2843-2857. doi: 10.3934/math.2020182 |
The aim of this work is to provide a new mathematical model that studies transmission dynamics of Coronavirus disease 2019 (COVID-19) caused by severe acute respiratory syndrome coronavirus 2 (SARS-CoV-2). The model captures the dynamics of the disease taking into consideration some measures and is represented by a system of nonlinear ordinary differential equations including seven classes, which are susceptible class (S), exposed class (E), asymptomatic infected class (A), severely infected class (V), hospitalized class (H), hospitalized class but in ICU (C) and recovered class (R). We prove positivity and boundedness of solutions, compute the basic reproduction number, and investigate asymptotic stability properties of the proposed model. As a consequence, dynamical properties of the model are established fully and some mitigation and prevention measures of COVID-19 outbreaks are also suggested. Furthermore, the model is fitted to COVID-19 confirmed cases in South Africa during the Omicron wave from November 27, 2021 to January 20, 2022 which helped determine the model parameters value for our numerical simulation. A set of numerical experiments using real data is conducted to support and illustrate the theoretical findings. Numerical simulation results show that fast waning of infection-induced immunity can increase the occurrence of outbreaks.
Mathematical modeling and analysis can provide us with characteristic properties of important phenomena and processes arising in real-world situations as well as predict the possible scenarios in reality (see, for example, [1,2,3,4,5,6,7,8,9,10,11,12]. In particular, the study of mathematical models describing infectious diseases has become one of the powerful and effective approaches to analyze, understand and predict transmission mechanisms as well as characteristics of infectious diseases. For many years, a great number of mathematical models of infectious diseases have been constructed and studied by many mathematicians, biologists, and epidemiologists (see, for example, [1,2,3,4,8,9,10] and references therein). Studying these models can provide us with suitable strategies for controlling and preventing diseases and for protecting the public health. In recent works [13,14,15] we have studied some epidemic models and their real-world applications.
In March 11, 2020, the World Health Organization (WHO) declared the Coronavirus disease 2019 (COVID-19) caused by severe acute respiratory syndrome coronavirus 2 (SARS-CoV-2) outbreak a global pandemic [16] and in terms of the number of people infected, deaths, and the unprecedented demand for healthcare services, COVID-19 had appeared in the top 10 worst infectious disease pandemic in history in just less than 2 years after the first case was detected. Similarly to the previous outbreak of other diseases, mathematical modeling and analysis of SARS-CoV-2 epidemic has strongly attracted the attention of many researchers with many useful applications in both theory and practice, especially in disease prevention. Consequently, a large number of mathematical models of COVID-19 corresponding to different strategies have been proposed analyzed by many infectious disease experts (see, for instance, [17,18,19,20,21,22,23,24,25,26,27,28,29,30,31,32,33,34,35] and references therein).
It is worth noting that the mathematical models of the COVID-19 transmission are constructed based on principles of mathematics of epidemics, strategies and measures for combating the epidemic. Therefore, strategies for confronting the COVID-19 epidemic of each country/region may lead to a different mathematical model. In other words, there can be many mathematical models of COVID-19 transmission depending on strategies and measures for confronting the COVID-19 epidemic. Motivated and inspired by the importance of mathematical models of epidemics, we provide in this work a new mathematical study for the transmission dynamics of SARS-CoV-2. More clearly, a new mathematical model describing transmission dynamics of SARS-CoV-2 epidemic is formulated and analyzed rigorously. This model is based on hypothesis that COVID-19 has threatened to collapse hospital and ICU services in most of nations of the world and it has affected the care programs for non-COVID-19 patients [36]. Hence, mathematical model designed to optimize predictions related to the need for hospitalization and ICU admission by COVID-19 patients will of great important. Mathematical model of COVID-19 dynamics represented by a system of nonlinear ordinary differential equations including seven classes, which are susceptible class (S), exposed class (E), asymptomatic infected class (A), severely infected class (V), hospitalized class (H), hospitalized class but in ICU (C) and recovered class (R). The positivity and boundedness of the new proposed model are established based on comparison principles for ODEs, and its basic reproduction number is calculated by using the approach developed in [37]. Next, the asymptotic stability properties of the model are investigated by the Lyapunov stability theory and the relationship between the basic reproduction number and global stability of disease free equilibrium. As an important consequence, dynamical properties of the model are established fully and some mitigation and prevention measures of COVID-19 outbreaks are also suggested. In addition, a set of numerical simulations using real data is reported to support and illustrate the theoretical results. The obtained results indicate that there is a good agreement between numerical simulation results and the theoretical ones. The plan of this work is as follows. The mathematical model is formulated in Section 2. Dynamics of the proposed models is analyzed in Section 3. Numerical experiments are reported in Section 4. The last section provides some remarks and conclusion.
In this section, we formulate mathematical model for transmission dynamics of COVID-19. In this model, the total population is divided into seven classes. The mathematical model transitions through the following classes: Susceptible (S), Exposed (E), asymptomatic (A), severely infected (V), hospitalized in general ward (H), hospitalized in ICU (C) and recovered (R). The model assume that recovered individuals experiences immunity waning, η [38]. The model has a constant recruitment rate Λ to the susceptible class, S. The transmission rate from asymptomatic, A, and severely infected individuals, V, to susceptible individuals, S is given by β with a modification parameters ϵ (0<ϵ<1) on β for the severely infected individuals since it generally assumed that the asymptomatic individuals are more infectious than the symptomatic individuals [20]. The asymptomatic, severely infected and hospitalized individuals transit to the recovered class at rate γA, γV and γH, respectively. It is assumed that those individuals severely infected, hospitalized in general ward and hospitalized in ICU can die due to COVID-19 disease at rate δ [39]. p and q is the rate at which exposed individual transit to asymptomatic and severely infected individuals, respectively. We considered demographic factor such as natural death in each of the class as rate μ. Severely infected individuals are hospitalized at rate κ and hospitalized individuals transit to ICU at rate, α while the those recovered from ICU return to the hospital general wards at rate, σ. In the model, we do not consider non-pharmaceutical intervention such as isolation or lockdowns.
Following Figure 1 and Table 1, the dynamics of COVID-19 transmission is modeled by the following system of nonlinear ordinary differential equations:
dSdt=Λ−μS−βAS−βES−βϵVS+ηR,dEdt=βAS+βES+βϵVS−pE−qE−μE,dAdt=pE−γAA−μA,dVdt=qE−κV−γVV−(μ+δ)V,dHdt=κV+σC−αH−γHH−(μ+δ)H,dCdt=αH−σC−(μ+δ)C,dRdt=γAA+γVV+γHH−μR−ηR, | (2.1) |
Symbol | Description |
S | Susceptible class |
E | Exposed class |
A | Asymptomatic infected class |
V | Severely infected class |
H | Hospitalized class |
C | Hospitalized class but in ICU |
R | Recovered class |
β | Rate at which infected individuals infect susceptibles |
p | Proportion of asymptomatic infected |
q | Proportion of severe infected |
κ | Proportion of severe progressing to hospitalise |
α | Proportion of hospitalise progressing to critical |
σ | Proportion of critical progressing to hospitalise |
γA | Recovery proportion of asymptomatic |
γV | Recovery proportion of mild |
γH | Recovery proportion of severe |
η | Rate of COVID-19 waning immunity |
ϵ | Infectious rate by the symptomatic individuals |
μ | Natural death rate |
δ | COVID-19 related death rate |
Λ | Recruitment rate |
subject to the initial data
S(0)>0,E(0)≥0,A(0)≥0,V(0)≥0,H(0)≥0,C(0)≥0,R(0)≥0. |
The parameter 0<ϵ<1 is a modification parameter since generally the asymptomatic individuals are assumed to be more infectious than the symptomatic individuals [20] and η is the rate at which those who recover from the disease become susceptible again over time [29].
For the model (2.1), we denote the total population by N, i.e,
N(t):=S(t)+E(t)+A(t)+V(t)+H(t)+C(t)+R(t),t≥0. |
By using comparison theorems for ODEs [40], it is easy to establish the positivity and boundedness of the model as follows.
Theorem 1 (Positivity and boundedness of solutions). The set
R7+:={(S,E,A,V,H,I,R)∈R7|S,E,A,V,H,I,R≥0} |
is a positively invariant set of the model (2.1). Furthermore, we have the following estimate
lim supt→∞N(t)≤Λμ. |
Proof. First, it follows from (2.1) that
dSdt|S=0=Λ+ηR>0,dEdt|E=0=βAS+βϵVS≥0,dAdt|A=0=pE≥0,dVdt|V=0=qE≥0,dHdt|H=0=κV+σC≥0,dCdt|C=0=αH≥0,dRdt|R=0=γAA+γVV+γHH≥0. |
By [40,Theorem B.7], we deduce that the set R7+ is a positively invariant set of the model (2.1).
Next, from (2.1) we obtain
dNdt=Λ−μN−δV−δH−δC≤Λ−μN. |
By using a comparison theorem for ODEs [40,Theorem B.1], we obtain
lim supt→∞N(t)≤Λμ. |
The proof is complete.
In this section, we will analyze dynamical properties of the model (2.1). As a consequence of Theorem 1, we only need to study dynamics of the model (2.1) on a feasible set defined by
Ω={(S,E,A,V,H,C,R)∈R7+|S+E+A+V+H+C+R≤Λμ}. | (3.1) |
We solve the following non-linear algebraic system in order to determine equilibria of the model (2.1):
Λ−μS−βAS−βES−βϵVS+ηR=0,βAS+βES+βϵVS−pE−qE−μE=0,pE−γAA−μA=0,qE−κV−γVV−(μ+δ)V=0,κV+σC−αH−γHH−(μ+δ)H=0,αH−σC−(μ+δ)C=0,γAA+γVV+γHH−μR−ηR=0. | (3.2) |
For convenience, we introduce the following notations:
τ1:=σ+μ+δα,τ2:=(α+γH+μ+δ)τ1−σκ,τ3:=κ+γV+μ+δqτ2,τ4:=pγA+μτ3,τ5:=γAτ4+γVτ2+γHτ1μ+η,τ6:=(p+q+μ)τ3−ητ5. | (3.3) |
It follows from the 6th, 5th, 4th, 3rd and 7th equations of the system (3.2) that
H=τ1C,V=τ2C,E=τ3C,A=τ4C,R=τ5C. | (3.4) |
On the other hand, adding side-by-side the 1st and 2nd equations of (3.2) we obtain
Λ−μS=τ6C. | (3.5) |
Combining (3.4), (3.5) with the 1st equation of (3.2) we have an equation for C
C[τ6−β(Λ−τ6Cμ)(ϵτ2+τ3+τ4)+ητ5]=0. | (3.6) |
The Eq (3.6) always possesses a trivial solution C=0. In this case, we have
E0=A0=V0=H0=C0=R0=0,S0=Λμ, |
which corresponds to a unique disease-free equilibrium point.
Lemma 1. The model (2.1) always possesses a disease-free equilibrium (DFE) point given by
E0=(S0,E0,A0,V0,H0,C0,R0)=(Λμ,0,0,0,0,0,0). | (3.7) |
We now use the approach developed by van den Driessche and Watmough [37] to compute the basic reproduction of the model. For this purpose, we set X=(E,A,V,H,C,R,S) and rewrite the model (2.1) in the matrix form
dXdt=F(X)−V(X), |
where
X=(EAVHCRS),F=(βES+βSA+βϵSV000000),V=((p+q+μ)E−pE+(γA+μ)A−qE+(κ+γV+μ+δ)V−κV−σC+(μ+δ+α+γH)H−αH+(σ+μ+δ)C−γAA−γVV−γVH+μR+ηR−Λ+μS+βSE+βSA+βϵSV−ηR). |
At the equilibrium point E0, we obtain
F=(βΛμβΛμβϵΛμ000000),V=(p+q+μ00−pμ+γA0−q0κ+μ+δ+γV). |
Consequently, the basic reproduction number of the model can be computed by
R0=ρ(FV−1)=βΛμ(p+q+μ)+βΛpμ(p+q+μ)(γA+μ)+βΛqϵμ(p+q+μ)(κ+γV+μ+δ), | (3.8) |
which is the number of secondary SARS-CoV-2 infections caused by one infectious individual during the infectious period in a completely susceptible population [32].
We now determine disease-endemic equilibrium (DEE) points by reconsidering (3.6). By simple algebraic manipulation, we obtain the non-trivial solution
C∗=Λτ6−(τ6+ητ5)μβτ6(ϵτ2+τ3+τ4). | (3.9) |
It is easy to verify that C∗>0 if and only if R0>1. In this case, we obtain a unique (positive) DEE point by using (3.4) and (3.5).
Lemma 2. If R0>1, then the model (2.1) has a unique DEE point E∗=(S∗,E∗,A∗,V∗,H∗,C∗,R∗), where C∗ is given by (3.9), E∗,A∗,V∗,H∗,R∗ are given by (3.4) and S∗ is given by (3.5).
Combining Lemmas 1 and 2, we have the following result.
Theorem 2. The model (2.1) always possess a DFE point E0 for all values of the parameters, whereas, the unique DEE point E∗ exists if and only if R0>1.
In this subsection, we analyze asymptotic stability of the model (2.1). For convenience, let us denote
ζ1:=p+q+μ,ζ2:=γA+μ,ζ3:=κ+γV+μ+δ,ζ4:=γV+δ+κ. |
Theorem 3 (Local stability of the DFE point). The DFE point of the model (2.1) is locally asymptotically stable if R0<1 and unstable if R0>1.
Proof. The Jacobian matrix of the model (2.1) evaluating at the DFE point is given by
J(E0)=(−μ−βΛμ−βΛμ−βϵΛμ00η0βΛμ−ζ1βΛμβϵΛμ0000p−ζ200000q0−ζ3000000κ−(α+γH+μ+δ)σ00000α−(σ+μ+δ)000γAγVγH0−(μ+η)). |
We now determine eigenvalues of J(E0). Since J(E0) is a block matrix, it is sufficient to determine eigenvalues of the three following sub-matrices
J0=(−μ), |
and
J1=(βΛμ−ζ1βΛμβϵΛμp−ζ20q0−ζ3), | (3.10) |
and
J2=(−(α+γH+μ+δ)σ0α−(σ+μ+δ)0γH0−(μ+η)). | (3.11) |
The matrix J0 has only one eigenvalue −μ<0. Meanwhile, the matrix J2 has an eigenvalue is −(μ+η)<0, and the remaining eigenvalues are the ones of the sub-matrix
J′2=(−(α+γH+μ+δ)σα−(σ+μ+δ)). | (3.12) |
Since det(J′2)>0 and tr(J′2)<0, real parts of two eigenvalues of J′2 are all negative. Consequently, real parts of three eigenvalues of J2 are all negative.
On the other hand, thanks to Schur-Cohn criterion (see [1]) it is easy to verify that if R0<1 then real parts of all the eigenvalues of J1 are negative. Hence, the local asymptotic stability of E0 is proved.
We now study the global stability of the DEE point based on the approach constructed by Castillo-Chavez et al. [41]. For this reason, consider a system of ODEs of the form
dxdt=F(x,I),dIdt=G(x,I),G(x,0)=0, | (3.13) |
where x∈Rm denotes (its components) the number of uninfected individuals and I∈Rn denotes (its components) the number of infected individuals including latent, infectious, etc. Let U0=(x∗,0) be the disease-free equilibrium and R0 be the basic reproduction number of this system.
The conditions (H1) and (H2) below must be met to guarantee local asymptotic stability.
(H1) For dxdt=F(x,0), x∗ is globally asymptotically stable (g.a.s.).
(H2) G(x,I)=AI−ˆG(x,I), ˆG(x,I)≥0 for (x,I)∈Ω,
where A=DIG(x∗,0) is an M-matrix (the off diagonal elements of A are nonnegative) and Ω is the region where the model makes biological sense.
Corollary 1 ([41]). The fixed point U0=(x∗,0) is a globally asymptotic stable (g.a.s.) equilibrium of (3.13) provided that R0<1 (l.a.s.) and that assumptions (H1) and (H2) are satisfied.
Theorem 4 (Global asymptotic stability of the DFE point). The DFE point E0 of the model (2.1) is globally asymptotically stable if R0<1.
Proof. First, we rewrite the model (2.1) in the form (3.13) by setting x=S and I=(E,A,V,H,C,R). Then, the DFE point becomes U0=(x∗,0)=(Λ/μ,0) and the system dx/dt=F(x,0) becomes
dSdt=Λ−μS. |
This equation has a unique equilibrium point x∗=Λ/μ, which is globally asymptotically stable. Therefore, the condition (H1) is satisfied.
We now verify the condition (H2). For the model (2.1), we have
G(x,I)=(βAS+βES+βϵVS−pE−qE−μEpE−γAA−μAqE−κV−γVV−(μ+δ)VκV+σC−αH−γHH−(μ+δ)HαH−σC−(μ+δ)CγAA+γVV+γHH−μR−ηR), |
and
DIG(x∗,0)=(βΛμ−ζ1βΛμβϵΛμ000p−ζ20000q0−ζ300000κ−α−γH−(μ+δ)σ0000α−(σ+μ+δ)00γAγVγH0−(μ+η)). |
Clearly, all the off diagonal elements of A:=G(x,I) are non-negative. Furthermore, we have
ˆG=AI−G(x,I)=(βE(Λμ−S)+βA(Λμ−S)+βϵV(Λμ−S)00000), | (3.14) |
which implies that ˆG(x,I)≥0 for all (x,I)∈Ω. Therefore, the conditions (H1) and (H2) are satisfied. By Corollary 1 the global stability of the DEE point is obtained. The proof is complete.
Remark 1. In order to investigate the local stability of J(E∗), we consider the Jacobian of the model (2.1) at E∗, which is given by
![]() |
Note that the DEE point exists if and only if R0>1. Since J(E∗) is very complicated, it is very difficult to have a format proof which shows that all the eigenvalues of J(E∗) have negative real parts. However, based on existing results on the asymptotic stability of mathematical models arising in biology, ecology and epidemiology (see, for instance, [1,2,3,4,8,9,10]), we can conjecture that the DEE point is not only locally asymptotically stable but also globally asymptotically stable if R0>1. Furthermore, in numerical examples performed in the next section, we will see that E∗ is asymptotically stable when R0>1. The asymptotic stability analysis of the DEE point is an interesting mathematical problem but in real-world applications, our main objective is to control the parameters by suitable strategies such that R0≤1. In this case, the DFE point is globally stable, i.e, the disease is eradicated.
Sensitivity analysis studies how uncertainty in the output of a model can be apportioned to different source of uncertainty in the model input. It is commonly used to determine the robustness of model predictions to parameter values. In this study, the normalized forward sensitivity index of R0 will be computed to identify parameters that have a high influence on R0. This can be used to improve intervention strategies. The normalized forward sensitivity index of a variable to a parameter is the ratio of the relative change in the variable to the relative change in the parameter [19].
Definition 1. (see [19]) The normalized forward sensitivity index of a variable, L, that depends differentiably on a parameter, u, is defined as:
ΥLu=uL∂L∂u. |
The formula (1) evaluates the sensitivity of an outcome to all input variables. Given the basic reproduction number R0 above, we derive the sensitivity index of R0 with respect to each parameters in R0 as follows.
ΥR0β=βR0∂R0∂β=1,ΥR0Λ=ΛR0∂R0∂Λ=1,ΥR0p=pR0∂R0∂p=−(((ϵ−1)q+γA)μ+(qϵ+δ+γV+κ)γA−q(γV+δ+κ))p(μ2+(qϵ+δ+γA+γV+κ+p)μ+(qϵ+δ+γV+κ)γA+pζ4)ζ1,ΥR0q=qR0∂R0∂q=q((ϵ−1)μ2+((ϵ−1)γA+(ϵ−1)p−γV−δ−κ)μ+(ϵp−δ−γV−κ)γA−pζ4)(μ2+(qϵ+δ+γA+γV+κ+p)μ+(qϵ+δ+γV+κ)γA+pζ4)ζ1,ΥR0γA=γAR0∂R0∂γA=−γAp(κ+γV+μ+δ)(μ2+(qϵ+δ+γA+γV+κ+p)μ+(qϵ+δ+γV+κ)γA+pζ4)(γA+μ),ΥR0γV=γVR0∂R0∂γV=−γVqϵ(γA+μ)(μ2+(qϵ+δ+γA+γV+κ+p)μ+(qϵ+δ+γV+κ)γA+pζ4)ζ3,ΥR0κ=κR0∂R0∂κ=−κqϵ(γA+μ)(μ2+(qϵ+δ+γA+γV+κ+p)μ+(qϵ+δ+γV+κ)γA+pζ4)ζ3,ΥR0δ=δR0∂R0∂δ=−δqϵ(γA+μ)(μ2+(qϵ+δ+γA+γV+κ+p)μ+(qϵ+δ+γV+κ)γA+pζ4)ζ3,ΥR0ϵ=ϵR0∂R0∂ϵ=qϵ(γA+μ)μ2+(qϵ+δ+γA+γV+κ+p)μ+(qϵ+δ+γV+κ)γA+pζ4. |
Table 2 provides sensitivity indices of R0 with respect to parameters given in Set 1 of Table 3.
Parameter | Sensitivity Index |
β | 1 |
Λ | 1 |
p | -0.181 |
q | -0.096 |
γA | -0.698 |
γV | -0.010 |
κ | -0.013 |
ϵ | 0.023 |
Parameter | Value | Source | Parameter | Value | Source |
β | 0.034 | assumed | μ | 164.13×365 | Estimated |
p | 0.1760 | [18,19] | δ | 1.7826×10−5 | [18,19] |
q | 0.0240 | [18,19] | Λ=μ×N(0) | 2533.8 | Estimated |
κ | 0.08 | [18,19] | η | 0.008 | [29] |
σ | 0.2 | [18,19] | ϵ | 0.5 | [42] |
α | 0.15 | [18,19] | γH | 0.047 | fitted |
γA | 0.07 | fitted | R0 | 0.61 | Computed |
γV | 0.061 | fitted |
Parameter | Value | Source | Parameter | Value | Source |
β | 0.34 | fitted | μ | 164.13×365 | Estimated |
p | 0.1760 | [18,19] | δ | 1.7826×10−5 | [18,19] |
q | 0.0240 | [18,19] | Λ=μ×N(0) | 2533.8 | Estimated |
κ | 0.08 | [18,19] | η | 0.008 | [29] |
σ | 0.2 | [18,19] | ϵ | 0.5 | [42] |
α | 0.15 | [18,19] | γH | 0.047 | fitted |
γA | 0.07 | fitted | R0 | 6.11 | Computed |
γV | 0.061 | fitted |
The implication of the above investigation for intervention strategy is that decreasing β or Λ by 10% decreases R0 by 10%. Also, the most negative sensitive parameter is the proportion of asymptomatic infected, γA, with ΥR0p=−0.698. If γA increase by 10%, then the basic reproduction number R0 decreases approximately 7%. We have not included the sensitivity index related to death rates due to ethical reasons. Therefore, compliance with COVID-19 mitigation measures such as social distancing, wearing a face mask, and washing hands is needed to reduce the transmission parameter β; furthermore, the spread of COVID-19 infection can be reduced by quarantining infected people and/or by using COVID-19 vaccines.
In this section, we provide the biological parameters of the proposed COVID-19 model (2.1) using the confirmed COVID-19 cases in South Africa during the Omicron wave period from November 27, 2021 to January 20, 2022 [43]. Using the using Poisson maximum likelihood and the dataset, we estimate parameter values in the model.
The initial value of the total population of South Africa based on the data is N(0)=59310000 and the average life span is 164.13 years. The fitting result to the reported data through our model is compared in Figure 2. Hence, it can be seen that the simulation model is quite in accordance with the actual data. We perform some numerical experiments with initial condition given in Table 5. For this purpose, we utilize the classical fourth order Runge-Kutta (RK4) method with step-size h=10−4 to simulate the model (2.1). In addition, the following set of initial data will be also used in numerical simulations.
S(0) | E(0) | A(0) | V(0) | H(0) | C(0) | R(0) | N(0) | Ref |
29675142 | 21968562 | 2861104 | 715275 | 572220 | 114444 | 3403253 | 59310000 | [44] |
Example 1 (The dynamics of the transmission model when R0<1). Consider the model (2.1) with the parameters given in Table 3. This scenario is to show the dynamic of the model when R0<1.
In this example, R0<1 and hence, the DFE point E0=(Λμ,0,0,0,0,0,0) is globally asymptotically stable. This implies that the epidemic will be eradicated. The solutions of the model (2.1) are depicted in Figure 3. It is clear that the DFE point is globally asymptotically stable. Therefore, the validity of Theorems 3 and 4 is supported.
Example 2 (The dynamics of the transmission model when R0>1). Consider the model (2.1) with the parameters given in Table 4. This scenario is to show the dynamic of the model when R0>1.
In this case R0>1 and therefore, the DEE point E∗ is locally asymptotically stable. The solutions of the model are depicted in Figure 4. It is clear that the DEE point is locally asymptotically stable. This is a evidence supporting the comments in Remark 1. Also, we can observe that the DEE point may be not only locally stable but also globally stable. Based on this, it is reasonable to conjecture the global asymptotic stability of the DEE point as reported in Remark 1.
Figure 5 shows that variable duration of immunity has impact on the dynamics of the diseases. If there is relatively quick loss of immunity, accumulation of susceptible individual may results in a disproportionately large outbreak in a subsequent wave. in Figure 5, when η=0.0111, which corresponded to the fasted loss of immunity in all the η considered, we observed accumulation of more susceptible individuals and low number of recovered individuals. Figure 6(a) shows that an increase in the transmission rate will increase the R0 while Figure 6(b) shows that the values of R0 can be kept below 1 as long as the values of transmission rate do not exceed a certain threshold (β≈0.11).
In this work, a mathematical model of the transmission dynamics of SARS-CoV-2 epidemic has been formulated and its dynamical properties have been investigated rigorously. By the rigorous mathematical analyses, we have computed the basic reproduction number R0, proved the positivity and boundedness of solutions and established the asymptotic stability properties of the DFE and DEE points. It was proved that the DFE point is globally asymptotically stable if R0≤1, whereas, the DEE point is locally asymptotically stable if R0>1. The normalized sensitivity index of R0 has been considered. We adopted the Poisson maximum likelihood to estimate and fit the model parameters using the reported active cases of COVID-19 in South Africa. The results indicate that the use of Poisson maximum likelihood yields a good fit as shown in Figure 2. Also, we have conducted a set of numerical experiments with with estimated parameters to support and illustrate the constructed theoretical results.
The constructed model and theoretical analysis not only provide us with a new observation of the transmission dynamics of COVID-19 but also suggest effective disease prevention measures. More clearly, an important consequence obtained from the theoretical analysis is that we determined the basic reproduction number R0 and identified the parameters that influence the R0. Based on this, some mitigation and preventative measures of COVID-19 outbreaks have been suggested. These mitigation and preventative measures can be useful in real situations and consistence with the WHO's recommendations.
In the current model, we incorporate infection-induced waning immunity. However, promising development directions for model (2.1) are to consider it along with vaccine waning immunity and optimal control strategies. Especially, in the context that the vaccination is being promoted in all countries, the study of the impact of vaccines, waning immunity and optimal control strategies are very important. This can provide us with effective vaccination strategies as well as disease prevention.
The proposed model is not exhaustive and can be extended in various ways. In the near future, we intend to develop this work to construct new mathematical models for transmission dynamics of SARS-CoV-2 epidemic and study practical applications of the proposed model. Also, pharmaceutical and non-pharmaceutical interventions, and optimal control strategies will be considered for the SARS-CoV-2 epidemic model.
The first author, Oluwaseun F. Egbelowo, acknowledges support from the DSI-NRF Centre of Excellence in Mathematical and Statistical Sciences (CoE-MaSS), South Africa. Opinions expressed and conclusions arrived at are those of the authors and are not necessarily to be attributed to the CoE-MaSS. Justin Munyakazi's research was supported by the National Research Foundation of South Africa. The authors wish to thank the editor and the anonymous reviewers for their time and for providing comments and suggestions that have helped improve the quality of this paper.
The authors declare that there is no conflict of interest regarding the publication of this paper.
[1] | L. J. S. Allen, Introduction to mathematical biology, Pearson, 2016. |
[2] | F. Brauer, C. Castillo-Chavez, Z. Feng, Mathematical models in epidemiology, 1 Ed., Springer, 2019. |
[3] |
F. Brauer, Mathematical epidemiology: Past, present, and future, Infect. Dis. Model., 2 (2017), 113–127. https://doi.org/10.1016/j.idm.2017.02.001 doi: 10.1016/j.idm.2017.02.001
![]() |
[4] | F. Brauer, Compartmental models in epidemiology, In: Mathematical epidemiology, Springer, Berlin, Heidelberg, 2008. https://doi.org/10.1007/978-3-540-78911-6_2 |
[5] |
N. C. Grassly, C. Fraser, Mathematical models of infectious disease transmission, Nat. Rev. Microbiol., 6 (2008), 477–487. https://doi.org/10.1038/nrmicro1845 doi: 10.1038/nrmicro1845
![]() |
[6] | K. Hattaf, H. Dutta, Mathematical modelling and analysis of infectious diseases, Springer, 2020. https://doi.org/10.1007/978-3-030-49896-2 |
[7] |
S. Tyagi, S. C. Martha, S. Abbas, A. Debbouch, Mathematical modeling and analysis for controlling the spread of infectious diseases, Chaos, Solitons Fract., 144 (2021), 110707. https://doi.org/10.1016/j.chaos.2021.110707 doi: 10.1016/j.chaos.2021.110707
![]() |
[8] | M. Y. Li, An introduction to mathematical modeling of infectious diseases, 1 Ed., Springer, 2018. |
[9] | M. Martcheva, An introduction to mathematical epidemiology, 1 Ed., Springer, 2015. |
[10] | M. A. Nowak, R. M. May, Viral dynamics, Oxford: Oxford University Press, 2000. |
[11] |
Y. Xie, Z. Wang, Transmission dynamics, global stability and control strategies of a modified SIS epidemic model on complex networks with an infective medium, Math. Comput. Simul., 188 (2021), 23–34. https://doi.org/10.1016/j.matcom.2021.03.029 doi: 10.1016/j.matcom.2021.03.029
![]() |
[12] |
Y. Xie, Z. Wang, A ratio-dependent impulsive control of an SIQS epidemic model with non-linear incidence, Appl. Math. Comput., 423 (2022), 127018. https://doi.org/10.1016/j.amc.2022.127018 doi: 10.1016/j.amc.2022.127018
![]() |
[13] |
M. T. Hoang, O. F. Egbelowo, Nonstandard finite difference schemes for solving an SIS epidemic model with standard incidence, Rend. Circ. Mat. Palermo, Ser. II, 69 (2020), 753–769. https://doi.org/10.1007/s12215-019-00436-x doi: 10.1007/s12215-019-00436-x
![]() |
[14] |
M. T. Hoang, O. F. Egbelowo, On the global asymptotic stability of a hepatitis B epidemic model and its solutions by nonstandard numerical schemes, Bol. Soc. Mat. Mex., 26 (2020), 1113–1134. https://doi.org/10.1007/s40590-020-00275-2 doi: 10.1007/s40590-020-00275-2
![]() |
[15] | M. T. Hoang, O. F. Egbelowo, Dynamics of a fractional-order hepatitis b epidemic model and its solutions by nonstandard numerical schemes, In: K. Hattaf, H. Dutta, Mathematical modelling and analysis of infectious diseases, Studies in Systems, Decision and Control, Springer, 2020. https://doi.org/10.1007/978-3-030-49896-2_5 |
[16] | WHO Director-General's opening remarks at the media briefing on COVID19-March 2020, 2020. Available from: https://www.who.int/director-general/speeches/detail/who-director-general-s-opening-remarks-at-the-media-briefing-on-covid-19---20-march-2020. |
[17] |
A. Anirudh, Mathematical modeling and the transmission dynamics in predicting the Covid-19-What next in combating the pandemic, Infect. Dis. Model., 5 (2020), 366–374. https://doi.org/10.1016/j.idm.2020.06.002 doi: 10.1016/j.idm.2020.06.002
![]() |
[18] |
M. Kimathi, S. Mwalili, V. Ojiambo, D. K. Gathungu, Age-structured model for COVID-19: Effectiveness of social distancing and contact reduction in Kenya, Infect. Dis. Model., 6 (2021), 15–23. https://doi.org/10.1016/j.idm.2020.10.012 doi: 10.1016/j.idm.2020.10.012
![]() |
[19] |
O. Iyiola, B. Oduro, T. Zabilowicz, B. Iyiola, D. Kenes, System of time fractional models for COVID-19: Modeling, analysis and solutions, Symmetry, 13 (2021), 787. https://doi.org/10.3390/sym13050787 doi: 10.3390/sym13050787
![]() |
[20] |
M. Kinyili, J. B. Munyakazi, A. Y. A. Mukhtar, Assessing the impact of vaccination on COVID-19 in South Africa using mathematical modeling, Appl. Math. Inf. Sci., 15 (2021), 701–716. https://doi.org/10.18576/amis/150604 doi: 10.18576/amis/150604
![]() |
[21] |
V. S. Panwar, P. S. Sheik Uduman, J. F. Gomez-Aguilar, Mathematical modeling of coronavirus disease COVID-19 dynamics using CF and ABC non-singular fractional derivatives, Chaos, Solitons Fract., 145 (2021), 110757. https://doi.org/10.1016/j.chaos.2021.110757 doi: 10.1016/j.chaos.2021.110757
![]() |
[22] |
R. K. Rai, S. Khajanchi, P. K. Tiwari, E. Venturino, A. K. Misra, Impact of social media advertisements on the transmission dynamics of COVID-19 pandemic in India, J. Appl. Math. Comput., 68 (2022), 19–44. https://doi.org/10.1007/s12190-021-01507-y doi: 10.1007/s12190-021-01507-y
![]() |
[23] |
A. ul Rehman, R. Singh, P. Agarwal, Modeling, analysis and prediction of new variants of COVID-19 and dengue co-infection on complex network, Chaos, Solitons Fract., 150 (2021), 111008. https://doi.org/10.1016/j.chaos.2021.111008 doi: 10.1016/j.chaos.2021.111008
![]() |
[24] |
M. Serhani, H. Labbardi, Mathematical modeling of COVID-19 spreading with asymptomatic infected and interacting peoples, J. Appl. Math. Comput., 66 (2021), 1–20. https://doi.org/10.1007/s12190-020-01421-9 doi: 10.1007/s12190-020-01421-9
![]() |
[25] | Y. Guo, T. Li, Modeling and dynamic analysis of novel coronavirus pneumonia (COVID-19) in China, J. Appl. Math. Comput., 2021. https://doi.org/10.1007/s12190-021-01611-z |
[26] |
S. He, Y. Peng, K. Sun, SEIR modeling of the COVID-19 and its dynamics, Nonlinear Dyn., 101 (2020), 1667–1680. https://doi.org/10.1007/s11071-020-05743-y doi: 10.1007/s11071-020-05743-y
![]() |
[27] |
S. Annas, M. I. Pratama, M. Rifandi, W. Sanusi, S. Side, Stability analysis and numerical simulation of SEIR model for pandemic COVID-19 spread in Indonesia, Chaos, Solitons Fract., 139 (2020), 110072. https://doi.org/10.1016/j.chaos.2020.110072 doi: 10.1016/j.chaos.2020.110072
![]() |
[28] |
B. A. Baba, B. Bilgehan, Optimal control of a fractional order model for the COVID-19 pandemic, Chaos, Solitons Fract., 144 (2021), 110678. https://doi.org/10.1016/j.chaos.2021.110678 doi: 10.1016/j.chaos.2021.110678
![]() |
[29] |
D. Dwomoh, S. Iddi, B. Adu, J. M. Aheto, K. M. Sedzro, J. Fobil, et al., Mathematical modeling of COVID-19 infection dynamics in Ghana: Impact evaluation of integrated government and individual level interventions, Infect. Dis. Model., 6 (2021), 381–397. https://doi.org/10.1016/j.idm.2021.01.008 doi: 10.1016/j.idm.2021.01.008
![]() |
[30] |
M. Higazy, Novel fractional order SIDARTHE mathematical model of COVID-19 pandemic, Chaos, Solitons Fract., 138 (2020), 110007. https://doi.org/10.1016/j.chaos.2020.110007 doi: 10.1016/j.chaos.2020.110007
![]() |
[31] |
M. M. Hikal, M. M. A. Elsheikh, W. K. Zahra, Stability analysis of COVID-19 model with fractional-order derivative and a delay in implementing the quarantine strategy, J. Appl. Math. Comput., 68 (2021), 295–321. https://doi.org/10.1007/s12190-021-01515-y doi: 10.1007/s12190-021-01515-y
![]() |
[32] |
J. M. Tchuenche, N. Dube, C. P. Bhunu, R. J. Smith, C. T. Bauch, The impact of media coverage on the transmission dynamics of human influenza, BMC Public Health, 11 (2011), 1–16. https://doi.org/10.1186/1471-2458-11-S1-S5 doi: 10.1186/1471-2458-11-S1-S5
![]() |
[33] |
A. R. Tuite, D. N. Fisman, A. L. Greer, Mathematical modelling of COVID-19 transmission and mitigation strategies in the population of Ontario, Canada, CMAJ, 192 (2020), E497–E505. https://doi.org/10.1503/cmaj.200476 doi: 10.1503/cmaj.200476
![]() |
[34] | M. Wang, Y. Hu, L. Wu, Dynamic analysis of a SIQR epidemic model considering the interaction of environmental differences, J. Appl. Math. Comput., 2021. https://doi.org/10.1007/s12190-021-01628-4. |
[35] |
Z. Zhang, A. Zeb, O. F. Egbelowo, V. S. Erturk, Dynamics of a fractional order mathematical model for COVID-19 epidemic, Adv. Differ. Equ., 2020 (2020), 1–16. https://doi.org/10.1186/s13662-020-02873-w doi: 10.1186/s13662-020-02873-w
![]() |
[36] |
J. M. Garrido, D. Martínez-Rodríguez, F. Rodríguez-Serrano, J. M. Pérez-Villares, A. Ferreiro-Marzal, M. M. Jiménez-Quintana, et al., Mathematical model optimized for prediction and health care planning for COVID-19, Med. Intensiva, 46 (2022), 248–258. https://doi.org/10.1016/j.medin.2021.02.014 doi: 10.1016/j.medin.2021.02.014
![]() |
[37] |
P. van den Driessche, J. Watmough, Reproduction numbers and sub-threshold endemic equilibria for compartmental models of disease transmission, Math. Biosci., 180 (2002), 29–48. https://doi.org/10.1016/S0025-5564(02)00108-6 doi: 10.1016/S0025-5564(02)00108-6
![]() |
[38] |
S. Xia, K. Duan, Y. Zhang, D. Zhao, H. Zhang, Z. Xie, et al., Effect of an inactivated vaccine against SARS-CoV-2 on safety and immunogenicity outcomes: Interim analysis of 2 randomized clinical trials, JAMA, 324 (2020), 951–960. https://doi.org/10.1001/jama.2020.15543 doi: 10.1001/jama.2020.15543
![]() |
[39] |
G. Gonzalez-Parra, A. J. Arenas, Nonlinear dynamics of the introduction of a new SARS-CoV-2 variant with different infectiousness, Mathematics, 9 (2021), 1564. https://doi.org/10.3390/math9131564 doi: 10.3390/math9131564
![]() |
[40] | H. L. Smith, P. E. Waltman, The theory of the chemostat: Dynamics of microbial competition, Cambridge University Press, 2008. |
[41] | C. Castillo-Chavez, Z. Feng, W. Huang, On the computation of R0 and its role in global stability, Mathematical Approaches for Emerging and Re-emerging Infection Diseases: An Introduction, 125 (2002), 31–65. |
[42] |
E. A. Iboi, C. N. Ngonghala, A. B. Gumel, Will an imperfect vaccine curtail the COVID-19 pandemic in the U.S., Infect. Dis. Model., 5 (2020), 510–524. https://doi.org/10.1016/j.idm.2020.07.006 doi: 10.1016/j.idm.2020.07.006
![]() |
[43] | World Health Organization. Available from: https://covid19.who.int/region/afro/country/za. |
[44] | South Africa Coronavirus. Available from: https://sacoronavirus.co.za. |
1. | Victoria May P. Mendoza, Renier Mendoza, Youngsuk Ko, Jongmin Lee, Eunok Jung, Managing bed capacity and timing of interventions: a COVID-19 model considering behavior and underreporting, 2022, 8, 2473-6988, 2201, 10.3934/math.2023114 | |
2. | Itsuki Nishimura, Md. Rajib Arefin, Yuichi Tatsukawa, Shinobu Utsumi, Md. Anowar Hossain, Jun Tanimoto, Social dilemma analysis on vaccination game accounting for the effect of immunity waning, 2023, 171, 09600779, 113426, 10.1016/j.chaos.2023.113426 | |
3. | D. Calvetti, E. Somersalo, Post-pandemic modeling of COVID-19: Waning immunity determines recurrence frequency, 2023, 365, 00255564, 109067, 10.1016/j.mbs.2023.109067 |
Symbol | Description |
S | Susceptible class |
E | Exposed class |
A | Asymptomatic infected class |
V | Severely infected class |
H | Hospitalized class |
C | Hospitalized class but in ICU |
R | Recovered class |
β | Rate at which infected individuals infect susceptibles |
p | Proportion of asymptomatic infected |
q | Proportion of severe infected |
κ | Proportion of severe progressing to hospitalise |
α | Proportion of hospitalise progressing to critical |
σ | Proportion of critical progressing to hospitalise |
γA | Recovery proportion of asymptomatic |
γV | Recovery proportion of mild |
γH | Recovery proportion of severe |
η | Rate of COVID-19 waning immunity |
ϵ | Infectious rate by the symptomatic individuals |
μ | Natural death rate |
δ | COVID-19 related death rate |
Λ | Recruitment rate |
Parameter | Sensitivity Index |
β | 1 |
Λ | 1 |
p | -0.181 |
q | -0.096 |
γA | -0.698 |
γV | -0.010 |
κ | -0.013 |
ϵ | 0.023 |
Parameter | Value | Source | Parameter | Value | Source |
β | 0.034 | assumed | μ | 164.13×365 | Estimated |
p | 0.1760 | [18,19] | δ | 1.7826×10−5 | [18,19] |
q | 0.0240 | [18,19] | Λ=μ×N(0) | 2533.8 | Estimated |
κ | 0.08 | [18,19] | η | 0.008 | [29] |
σ | 0.2 | [18,19] | ϵ | 0.5 | [42] |
α | 0.15 | [18,19] | γH | 0.047 | fitted |
γA | 0.07 | fitted | R0 | 0.61 | Computed |
γV | 0.061 | fitted |
Parameter | Value | Source | Parameter | Value | Source |
β | 0.34 | fitted | μ | 164.13×365 | Estimated |
p | 0.1760 | [18,19] | δ | 1.7826×10−5 | [18,19] |
q | 0.0240 | [18,19] | Λ=μ×N(0) | 2533.8 | Estimated |
κ | 0.08 | [18,19] | η | 0.008 | [29] |
σ | 0.2 | [18,19] | ϵ | 0.5 | [42] |
α | 0.15 | [18,19] | γH | 0.047 | fitted |
γA | 0.07 | fitted | R0 | 6.11 | Computed |
γV | 0.061 | fitted |
S(0) | E(0) | A(0) | V(0) | H(0) | C(0) | R(0) | N(0) | Ref |
29675142 | 21968562 | 2861104 | 715275 | 572220 | 114444 | 3403253 | 59310000 | [44] |
Symbol | Description |
S | Susceptible class |
E | Exposed class |
A | Asymptomatic infected class |
V | Severely infected class |
H | Hospitalized class |
C | Hospitalized class but in ICU |
R | Recovered class |
β | Rate at which infected individuals infect susceptibles |
p | Proportion of asymptomatic infected |
q | Proportion of severe infected |
κ | Proportion of severe progressing to hospitalise |
α | Proportion of hospitalise progressing to critical |
σ | Proportion of critical progressing to hospitalise |
γA | Recovery proportion of asymptomatic |
γV | Recovery proportion of mild |
γH | Recovery proportion of severe |
η | Rate of COVID-19 waning immunity |
ϵ | Infectious rate by the symptomatic individuals |
μ | Natural death rate |
δ | COVID-19 related death rate |
Λ | Recruitment rate |
Parameter | Sensitivity Index |
β | 1 |
Λ | 1 |
p | -0.181 |
q | -0.096 |
γA | -0.698 |
γV | -0.010 |
κ | -0.013 |
ϵ | 0.023 |
Parameter | Value | Source | Parameter | Value | Source |
β | 0.034 | assumed | μ | 164.13×365 | Estimated |
p | 0.1760 | [18,19] | δ | 1.7826×10−5 | [18,19] |
q | 0.0240 | [18,19] | Λ=μ×N(0) | 2533.8 | Estimated |
κ | 0.08 | [18,19] | η | 0.008 | [29] |
σ | 0.2 | [18,19] | ϵ | 0.5 | [42] |
α | 0.15 | [18,19] | γH | 0.047 | fitted |
γA | 0.07 | fitted | R0 | 0.61 | Computed |
γV | 0.061 | fitted |
Parameter | Value | Source | Parameter | Value | Source |
β | 0.34 | fitted | μ | 164.13×365 | Estimated |
p | 0.1760 | [18,19] | δ | 1.7826×10−5 | [18,19] |
q | 0.0240 | [18,19] | Λ=μ×N(0) | 2533.8 | Estimated |
κ | 0.08 | [18,19] | η | 0.008 | [29] |
σ | 0.2 | [18,19] | ϵ | 0.5 | [42] |
α | 0.15 | [18,19] | γH | 0.047 | fitted |
γA | 0.07 | fitted | R0 | 6.11 | Computed |
γV | 0.061 | fitted |
S(0) | E(0) | A(0) | V(0) | H(0) | C(0) | R(0) | N(0) | Ref |
29675142 | 21968562 | 2861104 | 715275 | 572220 | 114444 | 3403253 | 59310000 | [44] |