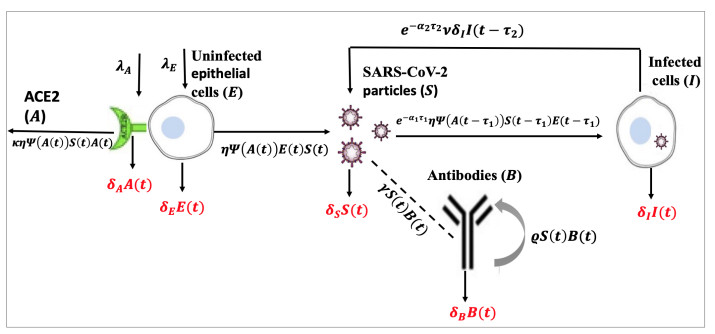
The coronavirus disease 2019 (COVID-19) is caused by a new coronavirus known as severe acute respiratory syndrome coronavirus 2 (SARS-CoV-2). SARS-CoV-2 infects the epithelial (target) cells by binding its spike protein, S, to the angiotensin-converting enzyme 2 (ACE2) receptor on the surface of epithelial cells. During the process of SARS-CoV-2 infection, ACE2 plays an important mediating role. In this work, we develop two models which describe the within-host dynamics of SARS-CoV-2 under the effect of humoral immunity, and considering the role of the ACE2 receptor. We consider two discrete (or distributed) delays: (ⅰ) Delay in the SARS-CoV-2 infection of epithelial cells, and (ⅱ) delay in the maturation of recently released SARS-CoV-2 virions. Five populations are considered in the models: Uninfected epithelial cells, infected cells, SARS-CoV-2 particles, ACE2 receptors and antibodies. We first address the fundamental characteristics of the delayed systems, then find all possible equilibria. On the basis of two threshold parameters, namely the basic reproduction number, ℜ0, and humoral immunity activation number, ℜ1, we prove the existence and stability of the equilibria. We establish the global asymptotic stability for all equilibria by constructing suitable Lyapunov functions and using LaSalle's invariance principle. To illustrate the theoretical results, we perform numerical simulations. We perform sensitivity analysis and identify the most sensitive parameters. The respective influences of humoral immunity, time delays and ACE2 receptors on the SARS-CoV-2 dynamics are discussed. It is shown that strong stimulation of humoral immunity may prevent the progression of COVID-19. It is also found that increasing time delays can effectively decrease ℜ0 and then inhibit the SARS-CoV-2 replication. Moreover, it is shown that ℜ0 is affected by the proliferation and degradation rates of ACE2 receptors, and this may provide worthy input for the development of possible receptor-targeted vaccines and drugs. Our findings may thus be helpful for developing new drugs, as well as for comprehending the dynamics of SARS-CoV-2 infection inside the host.
Citation: Ahmed M. Elaiw, Amani S. Alsulami, Aatef D. Hobiny. Global properties of delayed models for SARS-CoV-2 infection mediated by ACE2 receptor with humoral immunity[J]. AIMS Mathematics, 2024, 9(1): 1046-1087. doi: 10.3934/math.2024052
[1] | Kai Zhang, Xinzhu Meng, Abdullah Khames Alzahrani . The ACE2 receptor protein-mediated SARS-CoV-2 infection: dynamic properties of a novel delayed stochastic system. AIMS Mathematics, 2024, 9(4): 8104-8133. doi: 10.3934/math.2024394 |
[2] | A. M. Elaiw, A. S. Shflot, A. D. Hobiny . Stability analysis of SARS-CoV-2/HTLV-I coinfection dynamics model. AIMS Mathematics, 2023, 8(3): 6136-6166. doi: 10.3934/math.2023310 |
[3] | Afnan Al Agha, Hakim Al Garalleh . Oncolysis by SARS-CoV-2: modeling and analysis. AIMS Mathematics, 2024, 9(3): 7212-7252. doi: 10.3934/math.2024351 |
[4] | S. M. E. K. Chowdhury, J. T. Chowdhury, Shams Forruque Ahmed, Praveen Agarwal, Irfan Anjum Badruddin, Sarfaraz Kamangar . Mathematical modelling of COVID-19 disease dynamics: Interaction between immune system and SARS-CoV-2 within host. AIMS Mathematics, 2022, 7(2): 2618-2633. doi: 10.3934/math.2022147 |
[5] | Sara J Hamis, Fiona R Macfarlane . A single-cell mathematical model of SARS-CoV-2 induced pyroptosis and the effects of anti-inflammatory intervention. AIMS Mathematics, 2021, 6(6): 6050-6086. doi: 10.3934/math.2021356 |
[6] | Murugesan Sivashankar, Sriramulu Sabarinathan, Vediyappan Govindan, Unai Fernandez-Gamiz, Samad Noeiaghdam . Stability analysis of COVID-19 outbreak using Caputo-Fabrizio fractional differential equation. AIMS Mathematics, 2023, 8(2): 2720-2735. doi: 10.3934/math.2023143 |
[7] | Oluwaseun F. Egbelowo, Justin B. Munyakazi, Manh Tuan Hoang . Mathematical study of transmission dynamics of SARS-CoV-2 with waning immunity. AIMS Mathematics, 2022, 7(9): 15917-15938. doi: 10.3934/math.2022871 |
[8] | Maryam Amin, Muhammad Farman, Ali Akgül, Mohammad Partohaghighi, Fahd Jarad . Computational analysis of COVID-19 model outbreak with singular and nonlocal operator. AIMS Mathematics, 2022, 7(9): 16741-16759. doi: 10.3934/math.2022919 |
[9] | Chandan Maji, Fahad Al Basir, Debasis Mukherjee, Kottakkaran Sooppy Nisar, Chokkalingam Ravichandran . COVID-19 propagation and the usefulness of awareness-based control measures: A mathematical model with delay. AIMS Mathematics, 2022, 7(7): 12091-12105. doi: 10.3934/math.2022672 |
[10] | Tahir Khan, Fathalla A. Rihan, Muhammad Bilal Riaz, Mohamed Altanji, Abdullah A. Zaagan, Hijaz Ahmad . Stochastic epidemic model for the dynamics of novel coronavirus transmission. AIMS Mathematics, 2024, 9(5): 12433-12457. doi: 10.3934/math.2024608 |
The coronavirus disease 2019 (COVID-19) is caused by a new coronavirus known as severe acute respiratory syndrome coronavirus 2 (SARS-CoV-2). SARS-CoV-2 infects the epithelial (target) cells by binding its spike protein, S, to the angiotensin-converting enzyme 2 (ACE2) receptor on the surface of epithelial cells. During the process of SARS-CoV-2 infection, ACE2 plays an important mediating role. In this work, we develop two models which describe the within-host dynamics of SARS-CoV-2 under the effect of humoral immunity, and considering the role of the ACE2 receptor. We consider two discrete (or distributed) delays: (ⅰ) Delay in the SARS-CoV-2 infection of epithelial cells, and (ⅱ) delay in the maturation of recently released SARS-CoV-2 virions. Five populations are considered in the models: Uninfected epithelial cells, infected cells, SARS-CoV-2 particles, ACE2 receptors and antibodies. We first address the fundamental characteristics of the delayed systems, then find all possible equilibria. On the basis of two threshold parameters, namely the basic reproduction number, ℜ0, and humoral immunity activation number, ℜ1, we prove the existence and stability of the equilibria. We establish the global asymptotic stability for all equilibria by constructing suitable Lyapunov functions and using LaSalle's invariance principle. To illustrate the theoretical results, we perform numerical simulations. We perform sensitivity analysis and identify the most sensitive parameters. The respective influences of humoral immunity, time delays and ACE2 receptors on the SARS-CoV-2 dynamics are discussed. It is shown that strong stimulation of humoral immunity may prevent the progression of COVID-19. It is also found that increasing time delays can effectively decrease ℜ0 and then inhibit the SARS-CoV-2 replication. Moreover, it is shown that ℜ0 is affected by the proliferation and degradation rates of ACE2 receptors, and this may provide worthy input for the development of possible receptor-targeted vaccines and drugs. Our findings may thus be helpful for developing new drugs, as well as for comprehending the dynamics of SARS-CoV-2 infection inside the host.
The coronavirus disease 2019 (COVID-19) began in China in December 2019; it then turned into a global pandemic [1]. According to the World Health Organization report of August 27, 2023, there were over 770 million confirmed cases and 6.9 million deaths globally [2]. The world has also seen economic losses as a result of this sickness. COVID-19 originated from an infection with a virus called severe acute respiratory syndrome coronavirus 2 (SARS-CoV-2). It is a single-stranded RNA virus in the coronaviridae family. SARS-CoV-2 attacks the epithelial (target) cells by binding its spike protein, S, to the angiotensin-converting enzyme 2 (ACE2) receptor on the surface of epithelial cells [3,4]. ACE2 receptors of epithelial cells play a crucial role in cellular entry in humans, which may provide worthy input for the development of possible receptor-targeted vaccines and drugs [4,5]. Some of the symptoms that may appear in COVID-19 patients are like include headaches, fatigue, fever, myalgia, dry cough, nausea, abdominal pain, vomiting and diarrhea. Recognition of COVID-19 is essential because it enables the introduction of effective infection control measures and potentially helpful antiviral therapy. The adaptive immune response has an effective role in resisting and fighting viruses that attack the human body. B cells and cytotoxic T lymphocytes (CTLs) are two main players in the adaptive immune response. B cells generate antibodies to neutralize the SARS-CoV-2, while CTLs kill the epithelial cells infected by SARS-CoV-2.
Since the beginning of the spread of this disease, scientists and researchers from all fields have united their massive efforts to study and understand the mechanism between the virus and host cells in order to produce treatments and vaccines for this virus. Experimental evaluation of interactions between SARS-CoV-2, epithelial cells and immune cells can be difficult and expensive. Studying the dynamics of SARS-CoV-2 infection within the host by performing mathematical modeling may facilitate understanding of the dynamic behavior of the virus and its target cells, as well as immune cells. This type of study also helps in understanding the effectiveness of medications, whether individually or in combination. A within-host SARS-CoV-2 infection model with target-limited cells was given in [6]. Li et al. [7] included the production and death of the epithelial cells in a model of SARS-CoV-2 infection. Some biological processes were incorporated into the SARS-CoV-2 infection models by considering the effect of the immune response [8,9,10,11,12,13,14,15,16], drug therapies [17,18,19,20] and time delay [21]. In these works, the dynamics of ACE2 receptors of epithelial cells were not considered. In [22,23,24,25], the authors modeled the effect of the dipeptidyl peptidase 4 receptor on the Middle East respiratory syndrome coronavirus (MERS-CoV) infection. Chatterjee and Al Basir [26] presented a SARS-CoV-2 model with ACE2 receptors. The authors studied the local stability of equilibria. Lv and Ma [27] formulated a system of delay differential equations (DDEs) for SARS-CoV-2 infection, as mediated by ACE2 receptors, as follows:
Uninfected epithelial cells: ˙E(t)=Proliferation of epithelial cells⏞λE−Reduction of epithelial cells by SARS-CoV-2 and ACE2⏞ηΨ(A(t))E(t)S(t) −Natural death⏞δEE(t), | (1.1) |
Infected cells: ˙I(t)=Production of infected cells⏞e−α1τ1ηΨ(A(t−τ1))E(t−τ1)S(t−τ1)−Natural death⏞δII(t), | (1.2) |
SARS-CoV-2 particles: ˙S(t)=Production of SARS-CoV-2⏞δIνI(t)−Natural death⏞δSS(t), | (1.3) |
ACE2 receptors: ˙A(t)=Proliferation of ACE2 receptors⏞λA−Decrease in ACE2 receptors⏞κηΨ(A(t))A(t)S(t) −Degradation of ACE2 receptors⏞δAA(t). | (1.4) |
The variables E(t), I(t),S(t) and A(t) represent, respectively, the concentrations of per unit volume of uninfected epithelial cells, infected cells, SARS-CoV-2 particles and ACE2 receptors at time t. Ψ(A) is the probability of successful entry of the SARS-CoV-2 into the epithelial cell mediated by the ACE2 receptors. When the concentration of ACE2 receptor is lower (higher), then Ψ(A)∼0(∼1) [27]. Here, τ1 is the time from the SARS-CoV-2 particles making contact with uninfected epithelial cells to them becoming actively infected cells. The factor e−α1τ1 is the probability of survival of infected cells during the delay period of [t−τ1,t]. Note that the term ηΨ(A)ES denotes a decrease in uninfected epithelial cells (due to free virions), and the average number of ACE2 receptors carried by each uninfected epithelial cell is A/E. Therefore, the decrease in ACE2 receptors due to the decrease in uninfected epithelial cells (caused by free virions) is κηΨ(A)ES=κηΨ(A)ES×(A/E)=κηΨ(A)AS, where κ is a constant [27].
The model described by Eqs (1.1)–(1.4) does not take the immune system's response to SARS-CoV-2 infection into account. Furthermore, the model ignores the maturation delay and only takes into account one type of discrete-time (constant) delay, τ1. Therefore, our aim in this paper is to extend the model given by Eqs (1.1)–(1.4) by including the role of the humoral immune response and considering two classes of delays: (ⅰ) Delay in the SARS-CoV-2 infection of epithelial cells, and (ⅱ) delay in the maturation of recently released SARS-CoV-2 virions. In the first model, we consider discrete-time delays which are generalized in the second model by considering distributed-time delays. We first look into the fundamental characteristics of the DDEs; then, we find all equilibria and discuss their existence and global stability. We construct suitable Lyapunov functions and use LaSalle's invariance principle (LIP) to investigate the global asymptotic stability of all equilibria. We use numerical simulations to demonstrate the theoretical findings. Finally, we discuss the obtained results.
We formulate a system of DDEs for SARS-CoV-2 infection, as mediated by ACE2 receptors. We consider two discrete-time delays and the humoral immune response:
{˙E(t)=λE−ηΨ(A(t))E(t)S(t)−δEE(t),˙I(t)=e−α1τ1ηΨ(A(t−τ1))E(t−τ1)S(t−τ1)−δII(t),˙S(t)=e−α2τ2δIνI(t−τ2)−δSS(t)−γS(t)B(t),˙A(t)=λA−κηΨ(A(t))A(t)S(t)−δAA(t),˙B(t)=ϱS(t)B(t)−δBB(t), | (2.1) |
where B(t) denotes the concentration of antibodies at time t. The antibodies are stimulated at a rate of ϱSB, die at a rate of δBB and neutralize the SARS-CoV-2 particles at a rate of γSB. Here, τ2 is the maturation time of new virions. Factor e−α2τ2 represents the probability of survival of SARS-CoV-2 particles during their delay period of [t−τ2,t]. Usually, Ψ(A) is chosen as the classic Hill function: Ψ(A)=AnAns+An, where As is the half-saturation constant and n>0 is the Hill coefficient [27,28]. The function Ψ(A) is continuously differentiable on [0,∞) and strictly monotonically increasing. All parameters of model (2.1) are positive. A schematic representation of the model given by (2.1) is illustrated in Figure 1.
Let τ∗=max{τ1,τ2}, and consider the initial conditions for model (2.1) as follows:
E(θ)=ϕ1(θ), I(θ)=ϕ2(θ), S(θ)=ϕ3(θ), A(θ)=ϕ4(θ), B(θ)=ϕ5(θ),ϕi(θ)≥0, i=1,2,...,5, θ∈[−τ∗,0], | (2.2) |
where ϕi∈C([−τ∗,0],R≥0) is the Banach space of continuous functions mapping from [−τ∗,0] to R≥0 with the norm ‖ϕi‖=sup−τ∗≤θ≤0|ϕi(θ)| for ϕi∈C, i=1,2,...,5. We note that system (2.1) with the initial conditions given by Eq (2.2) has a unique solution [29].
This subsection proves the non-negativity and boundedness of the solutions of system (2.1).
Lemma 1. The solutions of model (2.1) with the initial conditions given by Eq (2.2) are non-negative and ultimately bounded.
Proof. We have that ˙E∣E=0=λE>0, ˙A∣A=0=λA>0 and ˙B∣B=0=0. Hence, E(t),A(t),B(t)≥0 for all t≥0 (see Proposition B.7 of [30]). From the second and third equations of system (2.1), we have
I(t)=e−δItϕ2(0)+e−α1τ1∫t0e−δI(t−θ)ηΨ(A(θ−τ1))E(θ−τ1)S(θ−τ1)dθ≥0,S(t)=e−∫t0(δS+γB(r))drϕ3(0)+e−α2τ2∫t0e−∫tθ(δS+γB(r))drδIνI(θ−τ2)dθ≥0 |
for all t∈[0,τ∗] [31]. Hence, by recursive argumentation, we obtain that I(t),S(t)≥0 for all t≥0. Hence, E,I,S,A and B are non-negative.
Now, we prove the ultimate boundedness of E(t), I(t), S(t), A(t) and B(t). From the first equation of system (2.1), we have that limt→∞supE(t)≤λEδE=ω1. To prove the ultimate boundedness of I(t), we define
Π1(t)=e−α1τ1E(t−τ1)+I(t). |
Then, we obtain
˙Π1(t)=e−α1τ1˙E(t−τ1)+˙I(t)=e−α1τ1λE−e−α1τ1ηΨ(A(t−τ1))E(t−τ1)S(t−τ1)−e−α1τ1δEE(t−τ1)+e−α1τ1ηΨ(A(t−τ1))E(t−τ1)S(t−τ1)−δII(t)=e−α1τ1λE−e−α1τ1δEE(t−τ1)−δII(t)≤λE−p1[e−α1τ1E(t−τ1)+I(t)]=λE−p1Π1(t), |
where p1=min{δE,δI}. Therefore, limt→∞supΠ1(t)≤λEp1=ω2. Since E(t)≥0 and I(t)≥0, then limt→∞supI(t)≤ω2. Now, let us define
Π2(t)=S(t)+γϱB(t). |
Then, we obtain
˙Π2(t)=˙S(t)+γϱ˙B(t)=e−α2τ2δIνI(t−τ2)−δSS(t)−γS(t)B(t)+γϱ[ϱS(t)B(t)−δBB(t)]=e−α2τ2δIνI(t−τ2)−δSS(t)−γδBϱB(t)≤δIνω2−p2[S(t)+γϱB(t)]=δIνω2−p2Π2(t), |
where p2=min{δS,δB}. Therefore, limt→∞supΠ2(t)≤δIνω2p2=ω3, and then limt→∞supS(t)≤ω3 and limt→∞supB(t)≤ϱγω3=ω5. Finally, from the fourth equation of system (2.1), we have that limt→∞supA(t)≤λAδA=ω4. Then, E,I,S,A and B are ultimately bounded.
From Lemma 1, we can establish that Γ={(E,I,S,A,B)∈C5≥0:‖E‖≤ω1, ‖I‖≤ω2, ‖S‖≤ω3, ‖A‖≤ω4, ‖B‖≤ω5} is positively invariant for system (2.1).
This subsection is a derivation of all equilibria of model (2.1) and the threshold parameters that determine the existence of the equilibria. First, we compute the basic infection reproduction number ℜ0 for system (2.1) by using the next-generation matrix method [32]. Define the matrices F and V as follows:
F=(0e−α1τ1ηΨ(A0)E000), V=(δI0−e−α2τ2δIνδS), |
where E0=λE/δE and A0=λA/δA. Then, ℜ0 can be derived as the spectral radius of FV−1, as follows:
ℜ0=e−α1τ1−α2τ2ηνΨ(A0)E0δS. | (2.3) |
Second, let Δ=(E,I,S,A,B) be any equilibrium of system (2.1); we have
0=λE−ηΨ(A)ES−δEE, | (2.4) |
0=e−α1τ1ηΨ(A)ES−δII, | (2.5) |
0=e−α2τ2δIνI−δSS−γSB, | (2.6) |
0=λA−κηΨ(A)SA−δAA, | (2.7) |
0=ϱSB−δBB. | (2.8) |
Equation (2.8) has two solutions, B=0 and S=δBϱ. When B=0, then, from Eq (2.6), we get
δII=δSνeα2τ2S. | (2.9) |
Substituting Eq (2.9) into Eq (2.5), we get
(e−α1τ1ηΨ(A)E−δSνeα2τ2)S=0, |
and then we have
S=0, or e−α1τ1ηΨ(A)E−δSνeα2τ2=0. |
If S=0, then, from Eqs (2.4), (2.5) and (2.7), we have that E=λE/δE, I=0 and A=λA/δA. Then, we obtain the uninfected equilibrium Δ0=(E0,0,0,A0,0).
If S≠0, then I≠0 and
e−α1τ1ηΨ(A)E=δSνeα2τ2. | (2.10) |
Therefore, we obtain
E=λE−eα1τ1δIIδE, S=e−α2τ2δIνIδS and A=λAδA+κeα1τ1δII/E. | (2.11) |
Substituting Eq (2.11) into Eq (2.5), we have
e−α1τ1ηΨ(λAδA+κeα1τ1δII/E)(λE−eα1τ1δIIδE)(e−α2τ2δIνIδS)−δII=0. |
Since I≠0, then
e−α1τ1ηΨ(λAδA+κeα1τ1δII/E)(λE−eα1τ1δIIδE)(e−α2τ2δIνδS)−δI=0. |
We define a function G(I) as follows:
G(I)=e−α1τ1−α2τ2(ηνδS)Ψ(λAδA+κeα1τ1δII/E)(λE−eα1τ1δIIδE)−1=0. |
We have
G(0)=ηνe−α1τ1−α2τ2δSΨ(λAδA)(λEδE)−1=ℜ0−1>0, if ℜ0>1, |
limI→λEδIe−α1τ1G(I)=−1<0, |
and
ddI[Ψ(λAδA+κeα1τ1δII/E)]=−eα1τ1κδIδEλAλE[δAλE+eα1τ1δII(κδE−δA)]2ΨI(λAδA+κeα1τ1δII/E)=Θ<0. |
So, we have
dG(I)dI=ηνe−α1τ1−α2τ2δS(λE−eα1τ1δIIδE)Θ−ηνδIe−α2τ2δSδEΨ(λAδA+κeα1τ1δII/E)<0. | (2.12) |
Then, there exists a unique I1∈(0,λEδIe−α1τ1) that satisfies that G(I1)=0.
Therefore, there exists a unique infected equilibrium without humoral immunity Δ1=(E1,I1,S1,A1,0) when ℜ0>1, where E1=λE−eα1τ1δII1δE∈(0,λEδE), S1=e−α2τ2δIνI1δS∈(0,λEνδSe−α1τ1−α2τ2) and A1=λAδA+κeα1τ1δII1/E1∈(0,λAδA).
If B≠0 and S=δBϱ, we therefore obtain
E=λE−eα1τ1δIIδE, A=λAδA+κeα1τ1δII/E, B=δSγ(e−α2τ2δIνϱIδSδB−1). | (2.13) |
Substituting Eq (2.13) into Eq (2.5), we obtain
e−α1τ1ηΨ(λAδA+κeα1τ1δII/E)(λE−eα1τ1δIIδE)(δBϱ)−δII=0. |
Define a function G∗(I) as follows:
G∗(I)=e−α1τ1ηΨ(λAδA+κeα1τ1δII/E)(λE−eα1τ1δIIδE)(δBϱ)−δII=0. |
We have
G∗(0)=e−α1τ1(ηδBϱ)Ψ(λAδA)(λEδE)>0, |
limI→λEδIe−α1τ1G∗(I)=−λEe−α1τ1<0. |
Moreover,
ddI[Ψ(λAδA+κeα1τ1δII/E)]=−eα1τ1κδIδEλAλE[δAλE+eα1τ1δII(κδE−δA)]2ΨI(λAδA+κeα1τ1δII/E)=Θ∗<0. |
So, we have
dG∗(I)dI=Θ∗e−α1τ1ηδBϱ(λE−eα1τ1δIIδE)−(ηδIδBϱδE)Ψ(λAδA+κeα1τ1δII/E)−δI<0. | (2.14) |
Hence, there exists a unique I2∈(0,λEδIe−α1τ1) that satisfies that G∗(I2)=0. Consequently, there exists a unique infected equilibrium with humoral immunity Δ2=(E2,I2,S2,A2,B2) when ℜ1>1, where E2=λE−eα1τ1δII2δE∈(0,λEδE), S2=δBϱ, A2=λAδA+κeα1τ1δII2/E2∈(0,λAδA) and B2=δSγ(ℜ1−1), where
ℜ1=e−α2τ2δIνϱI2δSδB. | (2.15) |
Here, ℜ1 represents the humoral immunity activation number.
We have that Ψ(A2)<Ψ(A0) and E2<E0. Therefore,
ℜ1=e−α2τ2δIνϱI2δSδB=e−α2τ2δIνϱδSδBe−α1τ1ηΨ(A2)E2S2δI=e−α1τ1−α2τ2νηΨ(A2)E2δS<e−α1τ1−α2τ2νηΨ(A0)E0δS=ℜ0. | (2.16) |
Now, we can state the following lemma:
Lemma 2. For system (2.1), there exist two threshold parameters ℜ0 and ℜ1 such that the following conditions hold:
(ⅰ) If ℜ0≤1, then the uninfected equilibrium Δ0=(E0,0,0,A0,0) is the only equilibrium.
(ⅱ) If ℜ1≤1<ℜ0, then there exists two equilibria, Δ0 and the infected equilibrium without humoral immunity Δ1=(E1,I1,S1,A1,0).
(ⅲ) If ℜ1>1, then there exist three equilibria, Δ0, Δ1 and the infected equilibrium with humoral immunity Δ2=(E2,I2,S2,A2,B2).
This subsection describes the use of the Lyapunov method to study the global asymptotic stability of the equilibria. We define a function Φ(x)=x−1−lnx. Clearly, Φ(1)=0 and Φ(x)≥0 for x>0. Let ˜Ωj be the largest invariant subset of
Ωj={(E,I,S,A,B):dΛjdt=0}, j=0,1,2, |
where Λj(E,I,S,A,B) is a Lyapunov function candidate. Denote (E,I,S,A,B)=(E(t),I(t),S(t),A(t),B(t)) and (Eτ,Iτ,Sτ,Aτ)=(E(t−τ),I(t−τ),S(t−τ),A(t−τ)). Subsequent to the studies of [33] and [44], we construct Lyapunov functions in the following theorems.
Theorem 1. For system (2.1), if ℜ0≤1, then Δ0 is globally asymptotically stable (G.A.S), and it is unstable when ℜ0>1.
Proof. Define
Λ0=E0Φ(EE0)+eα1τ1I+eα1τ1+α2τ2νS+E0κA0(A−A0−∫AA0Ψ(A0)Ψ(ξ)dξ)+γeα1τ1+α2τ2ϱνB+∫tt−τ1ηΨ(A(s))E(s)S(s)ds+eα1τ1δI∫tt−τ2I(s)ds. | (2.17) |
We note that Λ0(E,I,S,A,B)>0 for all E,I,S,A,B>0 and Λ0(E0,0,0,A0,0)=0. We evaluate dΛ0dt along the solutions of system (2.1) as follows:
dΛ0dt=(1−E0E)˙E+eα1τ1˙I+eα1τ1+α2τ2ν˙S+E0κA0(1−Ψ(A0)Ψ(A))˙A+γeα1τ1+α2τ2ϱν˙B+ddt∫tt−τ1ηΨ(A(s))E(s)S(s)ds+eα1τ1δIddt∫tt−τ2I(s)ds. |
Using system (2.1), we get
dΛ0dt=(1−E0E)[λE−ηΨ(A)ES−δEE]+eα1τ1[e−α1τ1ηΨ(Aτ1)Eτ1Sτ1−δII]+eα1τ1+α2τ2ν[e−α2τ2δIνIτ2−δSS−γSB]+E0κA0(1−Ψ(A0)Ψ(A))[λA−κηΨ(A)SA−δAA]+γeα1τ1+α2τ2ϱν[ϱSB−δBB]+ηΨ(A)ES−ηΨ(Aτ1)Eτ1Sτ1+eα1τ1δII−eα1τ1δIIτ2. |
Collecting terms, we get
dΛ0dt=(1−E0E)[λE−δEE]+ηΨ(A)E0S−eα1τ1+α2τ2νδSS+ηΨ(A0)E0S−ηΨ(A0)E0S+E0κA0(1−Ψ(A0)Ψ(A))[λA−δAA]−E0A0(Ψ(A)−Ψ(A0))ηSA−γδBeα1τ1+α2τ2ϱνB=(E−E0E)[λE−δEE]+(ηΨ(A0)E0−eα1τ1+α2τ2νδS)S+ηE0S(Ψ(A)−Ψ(A0))+E0κA0Ψ(A)(Ψ(A)−Ψ(A0))[λA−δAA]−E0A0(Ψ(A)−Ψ(A0))ηSA−γδBeα1τ1+α2τ2ϱνB. |
Using the equilibrium condition of λE=δEE0, as well as λA=δAA0, we get
dΛ0dt=−δE(E−E0)2E+δSeα1τ1+α2τ2ν(ℜ0−1)S+ηE0S(Ψ(A)−Ψ(A0))A0A0+δAE0κA0Ψ(A)(Ψ(A)−Ψ(A0))(A0−A)−ηE0A0S(Ψ(A)−Ψ(A0))A−γδBeα1τ1+α2τ2ϱνB=−δE(E−E0)2E+δSeα1τ1+α2τ2ν(ℜ0−1)S+(ηE0SA0+δAE0κA0Ψ(A))(Ψ(A)−Ψ(A0))(A0−A)−γδBeα1τ1+α2τ2ϱνB. |
Since Ψ(A) is strictly monotonically increasing, then (Ψ(A)−Ψ(A0))(A0−A)≤0. Therefore, dΛ0dt≤0 for all E,S,A,B>0 when ℜ0≤1. In addition, dΛ0dt=0 when E=E0, A=A0 and S=B=0. Solutions of system (2.1) converge to ˜Ω0, which contains elements [37]. Since ˜Ω0 is invariant with respect to (2.1), on ˜Ω0, we have
0=˙S=e−α1τ1δIνI⟹I=0 for all t. |
Therefore, ˜Ω0={Δ0} and, applying the LIP (see [29,39]), we obtain that Δ0 is G.A.S.
To show the instability of Δ0, we calculate the characteristic equation of system (2.1) at Δ0 as follows:
0=(c+δE)(c+δB)[c3+(δI+δS+δA)c2+(δSδA+δI(δS+δA)−ηe−(α1+c)τ1−(α2+c)τ2δIνΨ(A0)E0)c+δIδSδA−ηe−(α1+c)τ1−(α2+c)τ2δIνδAΨ(A0)E0]. |
Define a function where T(c) as follows:
T(c)=c3+(δI+δS+δA)c2+(δSδA+δI(δS+δA)−ηe−(α1+c)τ1−(α2+c)τ2δIνΨ(A0)E0)c+δIδSδA−ηe−(α1+c)τ1−(α2+c)τ2δIνδAΨ(A0)E0, |
which is continuous on [0,∞). We have
T(0)=δIδSδA(1−ℜ0)<0, when ℜ0>1,limc→∞T(c)=∞. |
Hence, T(c) has a positive real root and Δ0 is unstable.
To confirm the result on the dynamics of Δ1, we require additional assumptions [38]:
S1≤δBϱ. | (A) |
Theorem 2. Consider system (2.1) and suppose that assumption (A) is satisfied and ℜ1≤1<ℜ0; then, Δ1 is G.A.S.
Proof. Define
Λ1=e−α1τ1E1Φ(EE1)+I1Φ(II1)+eα2τ2νS1Φ(SS1)+e−α1τ1E1κA1(A−A1−∫AA1Ψ(A1)Ψ(ξ)dξ)+γeα2τ2νϱB+δII1∫tt−τ1Φ(Ψ(A(s))E(s)S(s)Ψ(A1)E1S1)ds+δII1∫tt−τ2Φ(I(s)I1)ds. | (2.18) |
Note that Λ1(E,I,S,A,B)>0 for all E,I,S,A,B>0 and Λ1(E1,I1,S1,A1,0)=0. We evaluate dΛ1dt as follows:
dΛ1dt=e−α1τ1(1−E1E)˙E+(1−I1I)˙I+eα2τ2ν(1−S1S)˙S+e−α1τ1E1κA1(1−Ψ(A1)Ψ(A))˙A+γeα2τ2νϱ˙B+δII1ddt∫tt−τ1Φ(Ψ(A(s))E(s)S(s)Ψ(A1)E1S1)ds+δII1ddt∫tt−τ2Φ(I(s)I1)ds. |
Using system (2.1), we get
dΛ1dt=e−α1τ1(1−E1E)[λE−ηΨ(A)ES−δEE]+(1−I1I)[e−α1τ1ηΨ(Aτ1)Eτ1Sτ1−δII]+eα2τ2ν(1−S1S)[e−α2τ2δIνIτ2−δSS−γSB]+e−α1τ1E1κA1(1−Ψ(A1)Ψ(A))[λA−κηΨ(A)SA−δAA]+γeα2τ2νϱ[ϱSB−δBB]+δII1[Ψ(A)ESΨ(A1)E1S1−Ψ(Aτ1)Eτ1Sτ1Ψ(A1)E1S1]+δII1ln(Ψ(Aτ1)Eτ1Sτ1Ψ(A)ES)+δII1[II1−Iτ2I1]+δII1ln(Iτ2I). |
Collecting terms, we get
dΛ1dt=e−α1τ1(1−E1E)[λE−δEE]−ηe−α1τ1Ψ(A)ES+ηe−α1τ1Ψ(A)E1S+e−α1τ1ηΨ(Aτ1)Eτ1Sτ1−e−α1τ1ηΨ(Aτ1)Eτ1Sτ1I1I+δII1−eα2τ2δSνS−δIIτ2S1S+eα2τ2δSνS1+eα2τ2γνS1B+e−α1τ1E1κA1(1−Ψ(A1)Ψ(A))×[λA−δAA]−e−α1τ1E1A1ηSA(Ψ(A)−Ψ(A1))−γδBeα2τ2νϱB+δII1Ψ(A)ESΨ(A1)E1S1−δII1Ψ(Aτ1)Eτ1Sτ1Ψ(A1)E1S1+δII1ln(Ψ(Aτ1)Eτ1Sτ1Ψ(A)ES)+δII1ln(Iτ2I). |
Using the equilibrium condition for Δ1, i.e.,
λE=ηΨ(A1)E1S1+δEE1, δII1=e−α1τ1ηΨ(A1)E1S1,δSS1=e−α2τ2δIνI1, λA=κηΨ(A1)S1A1+δAA1, | (2.19) |
we obtain
dΛ1dt=−δEe−α1τ1(E−E1)2E+4δII1−δII1E1E+e−α1τ1ηΨ(A)E1S−δII1Ψ(Aτ1)Eτ1Sτ1I1Ψ(A1)E1S1I−e−α1τ1ηΨ(A1)E1S−δII1Iτ2S1I1S+(γeα2τ2νS1−γδBeα2τ2νϱ)B+e−α1τ1δAE1κA1Ψ(A)(Ψ(A)−Ψ(A1))(A1−A)−δII1Ψ(A1)Ψ(A)−e−α1τ1ηE1A1(Ψ(A)−Ψ(A1))SA+δII1ln(Ψ(Aτ1)Eτ1Sτ1Ψ(A)ES)+δII1ln(Iτ2I)=−δEe−α1τ1(E−E1)2E+4δII1−δII1E1E+e−α1τ1ηE1S(Ψ(A)−Ψ(A1))−δII1Ψ(Aτ1)Eτ1Sτ1I1Ψ(A1)E1S1I−δII1Iτ2S1I1S+(γeα2τ2νS1−γδBeα2τ2νϱ)B+e−α1τ1δAE1κA1Ψ(A)(Ψ(A)−Ψ(A1))(A1−A)−δII1Ψ(A1)Ψ(A)−e−α1τ1ηE1A1(Ψ(A)−Ψ(A1))×SA+δII1ln(Ψ(Aτ1)Eτ1Sτ1Ψ(A)ES)+δII1ln(Iτ2I)=−δEe−α1τ1(E−E1)2E+4δII1−δII1E1E+e−α1τ1ηE1SA1(Ψ(A)−Ψ(A1))(A1−A)−δII1Ψ(Aτ1)Eτ1Sτ1I1Ψ(A1)E1S1I−δII1Iτ2S1I1S+γeα2τ2ν[S1−S2]B+e−α1τ1δAE1κA1Ψ(A)(Ψ(A)−Ψ(A1))(A1−A)−δII1Ψ(A1)Ψ(A)+δII1ln(Ψ(Aτ1)Eτ1Sτ1Ψ(A)ES)+δII1ln(Iτ2I). |
Using the equalities
ln(Ψ(Aτ1)Eτ1Sτ1Ψ(A)ES)=ln(Ψ(Aτ1)Eτ1Sτ1I1Ψ(A1)E1S1I)+ln(Ψ(A1)Ψ(A))+ln(IS1I1S)+ln(E1E),ln(Iτ2I)=ln(Iτ2S1I1S)+ln(I1SIS1), |
we obtain
dΛ1dt=−δEe−α1τ1(E−E1)2E−δII1Φ(E1E)−δII1Φ(Ψ(Aτ1)Eτ1Sτ1I1Ψ(A1)E1S1I)−δII1Φ(Iτ2S1I1S)−δII1Φ(Ψ(A1)Ψ(A))+γeα2τ2ν(S1−δBϱ)B+[e−α1τ1δAE1κA1Ψ(A)+e−α1τ1ηSE1A1](Ψ(A)−Ψ(A1))(A1−A). | (2.20) |
We have that (Ψ(A)−Ψ(A1))(A1−A)≤0, and, from Assumption (A), we have that S1−δBϱ≤0. Thus, dΛ1dt≤0 for all E,I,S,A,B>0. In addition, dΛ1dt=0 when E=E1, A=A1, B=0 and
Iτ2S1I1S=Ψ(Aτ1)Eτ1Sτ1I1Ψ(A1)E1S1I=1. | (2.21) |
All solutions of system (2.1) are attracted to ˜Ω1. Since ˜Ω1 is invariant with respect to (2.1), on ˜Ω1, we have
0=˙E=λE−ηΨ(A1)E1S−δEE1⟹S(t)=S1 for any t, |
and, from Eq (2.21), we get that I(t)=Iτ2=I1 for any t. Therefore, ˜Ω1={Δ1}, and by applying the LIP, we obtain that Δ1 is G.A.S.
Theorem 3. Consider system (2.1) and let ℜ1>1; then, Δ2 is G.A.S.
Proof. Consider
Λ2=e−α1τ1E2Φ(EE2)+I2Φ(II2)+eα2τ2νS2Φ(SS2)+e−α1τ1E2κA2(A−A2−∫AA2Ψ(A2)Ψ(ξ)dξ)+γeα2τ2νϱB2Φ(BB2)+δII2∫tt−τ1Φ(Ψ(A(s))E(s)S(s)Ψ(A2)E2S2)ds+δII2∫tt−τ2Φ(I(s)I2)ds. | (2.22) |
We note that Λ2(E,I,S,A,B)>0 for all E,I,S,A,B>0 and Λ2(E2,I2,S2,A2,B2)=0. We calculate dΛ2dt as follows:
dΛ2dt=e−α1τ1(1−E2E)˙E+(1−I2I)˙I+eα2τ2ν(1−S2S)˙S+e−α1τ1E2κA2(1−Ψ(A2)Ψ(A))˙A+γeα2τ2νϱ(1−B2B)˙B+δII2ddt∫tt−τ1Φ(Ψ(A(s))E(s)S(s)Ψ(A2)E2S2)ds+δII2ddt∫tt−τ2Φ(I(s)I2)ds. |
From system (2.1), we get
dΛ2dt=e−α1τ1(1−E2E)[λE−ηΨ(A)ES−δEE]+(1−I2I)[e−α1τ1ηΨ(Aτ1)Eτ1Sτ1−δII]+eα2τ2ν(1−S2S)[e−α2τ2δIνIτ2−δSS−γSB]+e−α1τ1E2κA2(1−Ψ(A2)Ψ(A))[λA−κηΨ(A)SA−δAA]+γeα2τ2νϱ(1−B2B)[ϱSB−δBB]+δII2[Ψ(A)ESΨ(A2)E2S2−Ψ(Aτ1)Eτ1Sτ1Ψ(A2)E2S2]+δII2ln(Ψ(Aτ1)Eτ1Sτ1Ψ(A)ES)+δII2[II2−Iτ2I2]+δII2ln(Iτ2I). |
Collecting terms, we get
dΛ2dt=e−α1τ1(1−E2E)[λE−δEE]−ηe−α1τ1Ψ(A)ES+ηe−α1τ1Ψ(A)E2S+e−α1τ1ηΨ(Aτ1)Eτ1Sτ1−e−α1τ1ηΨ(Aτ1)Eτ1Sτ1I2I+δII2−eα2τ2δSνS−δIIτ2S2S+eα2τ2δSνS2+γeα2τ2νS2B+e−α1τ1E2κA2(1−Ψ(A2)Ψ(A))[λA−δAA]−e−α1τ1E2A2(Ψ(A)−Ψ(A2))ηSA−γeα2τ2δBνϱB−γeα2τ2νSB2+γeα2τ2δBνϱB2+δII2Ψ(A)ESΨ(A2)E2S2−δII2Ψ(Aτ1)Eτ1Sτ1Ψ(A2)E2S2+δII2ln(Ψ(Aτ1)Eτ1Sτ1Ψ(A)ES)+δII2ln(Iτ2I). |
Using the equilibrium condition for Δ2, i.e.,
λE=ηΨ(A2)E2S2+δEE2, δII2=e−α1τ1ηΨ(A2)E2S2, δSS2=e−α2τ2δIνI2−γS2B2, λA=κηΨ(A2)S2A2+δAA2, S2=δBϱ, |
we obtain
dΛ2dt=−δEe−α1τ1(E−E2)2E+4δII2−δII2E2E+e−α1τ1ηΨ(A)E2S−δII2Ψ(Aτ1)Eτ1Sτ1I2Ψ(A2)E2S2I−e−α1τ1ηΨ(A2)E2S−δII2Iτ2S2I2S−δII2Ψ(A2)Ψ(A)+e−α1τ1δAE2κA2Ψ(A)(Ψ(A)−Ψ(A2))(A2−A)−e−α1τ1E2A2ηSA(Ψ(A)−Ψ(A2))+δII2ln(Ψ(Aτ1)Eτ1Sτ1Ψ(A)ES)+δII2ln(Iτ2I)=−δEe−α1τ1(E−E2)2E+4δII2−δII2E2E+e−α1τ1ηE2S(Ψ(A)−Ψ(A2))−δII2Ψ(Aτ1)Eτ1Sτ1I2Ψ(A2)E2S2I−δII2Iτ2S2I2S−δII2Ψ(A2)Ψ(A)+e−α1τ1δAE2κA2Ψ(A)(Ψ(A)−Ψ(A2))(A2−A)−e−α1τ1E2A2ηSA(Ψ(A)−Ψ(A2))+δII2ln(Ψ(Aτ1)Eτ1Sτ1Ψ(A)ES)+δII2ln(Iτ2I). |
Using the equalities
ln(Ψ(Aτ1)Eτ1Sτ1Ψ(A)ES)=ln(Ψ(Aτ1)Eτ1Sτ1I2Ψ(A2)E2S2I)+ln(Ψ(A2)Ψ(A))+ln(IS2I2S)+ln(E2E),ln(Iτ2I)=ln(Iτ2S2I2S)+ln(I2SIS2), |
we obtain
dΛ2dt=−δEe−α1τ1(E−E2)2E−δII2Φ(E2E)−δII2Φ(Ψ(Aτ1)Eτ1Sτ1I2Ψ(A2)E2S2I)−δII2Φ(Iτ2S2I2S)−δII2Φ(Ψ(A2)Ψ(A))+[e−α1τ1δAE2κA2Ψ(A)+e−α1τ1ηSE2A2]×(Ψ(A)−Ψ(A2))(A2−A). | (2.23) |
If ℜ1>1, we get that dΛ2dt≤0 for all E,I,S,A>0. Further, dΛ2dt=0 when E=E2, A=A2 and
Iτ2S2I2S=Ψ(Aτ1)Eτ1Sτ1I2Ψ(A2)E2S2I=1. | (2.24) |
Trajectories of system (2.1) converge to ˜Ω2, where E=E2 and A=A2; then,
0=˙E=λE−ηΨ(A2)E2S−δEE2⟹S(t)=S2 for any t, |
and, from Eq (2.24), we get that I(t)=Iτ2=I2 for any t. Moreover, the third equation of system (2.1) yields
0=˙S=e−α2τ2δIνI2−δSS2−γS2B⟹B(t)=B2 for any t. |
Hence, ˜Ω2={Δ2} and the LIP implies that Δ2 is G.A.S.
In the previous section, we assumed that the time between the virus entering the cell and the production of new immature virions (τ1) is fixed for each cell. Moreover, the maturation time (τ2) for each virus is fixed. Several viral infection models were developed by taking into account the time delay as a random variable drawn from the probability distribution function in order to avoid such an (biologically implausible) assumption (see, e.g., [34,35,36]). In this section, we study a SARS-CoV-2 infection model with distributed-time delay. It is worth pointing out that the distributed delay is one of various time delays, and it is more general than discrete delay. In various nonlinear systems, other types of time delays have been examined, including proportional delay [40], time-varying delay [45,46] and state-dependent delay [47].
We formulate a SARS-CoV-2 infection system with two kinds of distributed delays as follows:
{˙E=λE−ηΨ(A)ES−δEE,˙I=η∫h10f1(τ)e−α1τΨ(Aτ)EτSτdτ−δII,˙S=δIν∫h20f2(τ)e−α2τIτdτ−δSS−γSB,˙A=λA−κηΨ(A)AS−δAA,˙B=ϱSB−δBB. | (3.1) |
Here, τ is a random variable from a probability distributed function fi(τ) over the interval [0,hi], where hi is the limit superior of the delay period, and i=1,2. The factor f1(τ)e−α1τ represents the probability that uninfected epithelial cells contacted by the SARS-CoV-2 at time t−τ survive τ time units and become infected at time t. The factor f2(τ)e−α2τ is the probability that an immature SARS-CoV-2 at time t−τ survives τ time units to become mature SARS-CoV-2 at time t. The function fi(τ) satisfies the following conditions:
fi(τ)>0, ∫hi0fi(τ)dτ=1, ∫hi0fi(τ)eℓτdτ<∞, where ℓ>0,i=1,2. | (3.2) |
Let χi(τ)=fi(τ)e−αiτ and ζi=∫hi0χi(τ)dτ; thus, 0<ζi≤1, i=1,2. Because fi(τ) is a general distribution, it is possible to model a variety of delay distributions by using model (3.1). The initial conditions for model (3.1) are the same as those given by Eq (2.2), where τ∗=max{h1,h2}.
This subsection proves the non-negativity and boundedness of the solutions of system (3.1).
Lemma 3. Solutions of model (3.1) with the initial conditions given by Eq (2.2) are non-negative and ultimately bounded.
Proof. We have that ˙E∣E=0=λE>0, ˙A∣A=0=λA>0 and ˙B∣B=0=0. Thus, E(t)≥0, A(t)≥0 and B(t)≥0 for all t≥0 (see Proposition B.7 of [30]). In addition, we have
I(t)=e−δItϕ2(0)+η∫t0∫h10χ1(τ)Ψ(A(θ−τ))E(θ−τ)S(θ−τ)e−δI(t−θ)dτdθ≥0,S(t)=e−∫t0(δS+γB(r))drϕ3(0)+δIν∫t0∫h20χ2(τ)I(θ−τ)e−∫tθ(δS+γB(r))drdτdθ≥0 |
for all t∈[0,τ∗] [31]. Hence, by recursive argumentation, we get that I(t),S(t)≥0 for all t≥0. Hence, E,I,S,A and B are non-negative.
Now, we prove that E, I, S, A and B are all ultimately bounded. From the first equation of system (3.1) we have that limt→∞supE(t)≤λEδE=ω1. To investigate the ultimate boundedness of I, we define
Π1=∫h10χ1(τ)Eτdτ+I. |
Then, we obtain
˙Π1=∫h10χ1(τ)˙E(t−τ)+˙I=∫h10χ1(τ){λE−ηΨ(Aτ)EτSτ−δEEτ}dτ+η∫h10χ1(τ)Ψ(Aτ)EτSτdτ−δII=λE∫h10χ1(τ)dτ−δE∫h10χ1(τ)Eτdτ−δII≤λEζ1−p1[∫h10χ1(τ)Eτ+I]≤λE−p1[∫h10χ1(τ)Eτ+I]=λE−p1Π1, |
where p1=min{δE,δI}.
It follows that limt→∞supΠ1(t)≤λEp1=ω2. Since E≥0 and I≥0, then limt→∞supI(t)≤ω2. Now, let us define Π2=S+γϱB. Then, we obtain
˙Π2=˙S+γϱ˙B=δIν∫h20χ2(τ)Iτdτ−δSS−γSB+γϱ(ϱSB−δBB)=δIν∫h20χ2(τ)Iτdτ−δSS−γδBϱB≤δIνω2ζ2−p2[S+γϱB]≤δIνω2−p2[S+γϱB]=δIνω2−p2Π2, |
where p2=min{δS,δB}. Hence, limt→∞supΠ2(t)≤δIνω2p2=ω3. Since S≥0 and B≥0, then limt→∞supS(t)≤ω3 and limt→∞supB(t)≤ϱγω3=ω5. Finally, from the fourth equation of system (3.1), we have limt→∞supA(t)≤λAδA=ω4. Then, E,I,S,A and B are ultimately bounded.
From Lemma 3, we can demonstrate that Γ={(E,I,S,A,B)∈C5≥0:‖E‖≤ω1, ‖I‖≤ω2, ‖S‖≤ω3, ‖A‖≤ω4, ‖B‖≤ω5} is positively invariant for system (3.1).
First, we compute the basic reproduction number ¯ℜ0 for system (3.1). Define ˉF and ˉV as follows:
ˉF=(0ηζ1Ψ(A0)E000), ˉV=(δI0−ζ2δIνδS), |
where E0=λE/δE and A0=λA/δA. Then, ¯ℜ0 can be computed as the spectral radius of ˉFˉV−1, as follows:
¯ℜ0=ηνζ1ζ2Ψ(A0)E0δS. | (3.3) |
Second, let Δ=(E,I,S,A,B) be any equilibrium of system (3.1) such that
0=λE−ηΨ(A)ES−δEE, | (3.4) |
0=ηζ1Ψ(A)ES−δII, | (3.5) |
0=δIνζ2I−δSS−γSB, | (3.6) |
0=λA−κηΨ(A)SA−δAA, | (3.7) |
0=ϱSB−δBB. | (3.8) |
Equation (3.8) has two solutions, B=0 and S=δBϱ. When B=0, then, from Eq (3.6), we get
δII=δSνζ2S. | (3.9) |
Substituting Eq (3.9) into Eq (3.5), we obtain
[ηζ1Ψ(A)E−δSνζ2]S=0, | (3.10) |
and then we have
S=0, or ηζ1Ψ(A)E−δSνζ2=0. |
If S=0, then, from Eqs (3.4), (3.5) and (3.7), we have that E=λE/δE, I=0 and A=λA/δA. Then, we obtain the uninfected equilibrium Δ0=(E0,0,0,A0,0).
If S≠0, then I≠0 and
ζ1ηΨ(A)E=δSνζ2. | (3.11) |
Therefore, we obtain
E=λE−ζ−11δIIδE, S=ζ2δIνIδS and A=λAδA+κζ−11δII/E. | (3.12) |
Substituting Eq (3.12) into Eq (3.5), we have
ζ1ηΨ(λAδA+κζ−11δII/E)(λE−ζ−11δIIδE)(ζ2δIνIδS)−δII=0. |
Since I≠0, then
ζ1ηΨ(λAδA+κζ−11δII/E)(λE−ζ−11δIIδE)(ζ2δIνδS)−δI=0. |
We define a function G1(I) as follows:
G1(I)=ζ1ζ2(ηνδS)Ψ(λAδA+κζ−11δII/E)(λE−ζ−11δIIδE)−1=0. |
We have
G1(0)=ηνζ1ζ2δSΨ(λAδA)(λEδE)−1=ˉℜ0−1>0 for ˉℜ0>1 |
limI→λEδIζ1G1(I)=−1<0 |
and
ddI[Ψ(λAδA+κζ−11δII/E)]=−ζ−11κδIδEλAλE[δAλE+ζ−11δII(κδE−δA)]2ΨI(λAδA+κζ−11δII/E)=Θ1<0. |
So, we have
dG1(I)dI=ηνζ1ζ2δS(λE−ζ−11δIIδE)Θ1−ηνδIζ2δSδEΨ(λAδA+κζ−11δII/E)<0. | (3.13) |
Hence, there exists a unique I1∈(0,λEδIζ1) satisfying that G1(I1)=0.
Therefore, there exists a unique infected equilibrium without humoral immunity Δ1=(E1,I1,S1,A1,0) when ˉℜ0>1, where E1=λE−ζ−11δII1δE∈(0,λEδE), S1=ζ2δIνI1δS∈(0,λEνδSζ1ζ2) and A1=λAδA+κζ−11δII1/E1∈(0,λAδA).
If B≠0 and S=δBϱ, we therefore obtain
E=λE−ζ−11δIIδE, A=λAδA+κζ−11δII/E, B=δSγ(ζ2δIνϱIδSδB−1). | (3.14) |
Substituting Eq (3.14) into Eq (3.5), we obtain
ζ1ηΨ(λAδA+κζ−11δII/E)(λE−ζ−11δIIδE)(δBϱ)−δII=0. |
Define a function G∗1(I) as follows:
G∗1(I)=ζ1ηΨ(λAδA+κζ−11δII/E)(λE−ζ−11δIIδE)(δBϱ)−δII=0. |
We have
G∗1(0)=ζ1(ηδBϱ)Ψ(λAδA)(λEδE)>0, |
limI→λEδIζ1G∗1(I)=−λEζ1<0. |
Moreover,
ddI[Ψ(λAδA+κζ−11δII/E)]=−ζ−11κδIδEλAλE[δAλE+ζ−11δII(κδE−δA)]2ΨI(λAδA+κζ−11δII/E)=Θ∗1<0. |
So, we have
dG∗1(I)dI=Θ∗1ζ1ηδBϱ(λE−ζ−11δIIδE)−(ηδIδBϱδE)Ψ(λAδA+κζ−11δII/E)−δI<0. | (3.15) |
Then, there exists a unique I2∈(0,λEδIζ1) such that G∗1(I2)=0. It follows that there exists a unique infected equilibrium with humoral immunity Δ2=(E2,I2,S2,A2,B2) when ˉℜ1>1, where E2=λE−ζ−11δII2δE∈(0,λEδE), S2=δBϱ, A2=λAδA+κζ−11δII2/E2∈(0,λAδA) and B2=δSγ(ˉℜ1−1), where
ˉℜ1=ζ2δIνϱI2δSδB. | (3.16) |
Here, ˉℜ1 represents the antibody activation number.
We have that Ψ(A2)<Ψ(A0) and E2<E0. Therefore,
ˉℜ1=ζ2δIνϱI2δSδB=ζ2δIνϱδSδBζ1ηΨ(A2)E2S2δI=ζ1ζ2νηΨ(A2)E2δS<ζ1ζ2νηΨ(A0)E0δS=ˉℜ0. | (3.17) |
Now, we can state the following lemma:
Lemma 4. For system (3.1), there exist two threshold parameters ˉℜ0 and ˉℜ1 such that the following conditions hold:
(ⅰ) If ˉℜ0≤1, then the uninfected equilibrium Δ0=(E0,0,0,A0,0) is the unique equilibrium.
(ⅱ) If ˉℜ1≤1<ˉℜ0, then there exists two equilibria, Δ0 and the infected equilibrium without humoral immunity Δ1=(E1,I1,S1,A1,0).
(ⅲ) If ˉℜ1>1, then there exist three equilibria, Δ0, Δ1 and the infected equilibrium with humoral immunity Δ2=(E2,I2,S2,A2,B2).
This subsection proves the global stability of the equilibria of model (3.1) by using the Lyapunov method. Let ˉ˜Ωj be the largest invariant subset of
ˉΩj={(E,I,S,A,B):dˉΛjdt=0}, j=0,1,2, |
where ˉΛj(E,I,S,A,B) is a Lyapunov function candidate. Subsequent to the studies of [33,34,36], we construct Lyapunov functions in the following theorems.
Theorem 4. Consider system (3.1) and let ˉℜ0≤1; then, Δ0 is G.A.S. Moreover, if ˉℜ0>1, then Δ0 is unstable.
Proof. Define
ˉΛ0=ζ1E0Φ(EE0)+I+1νζ2S+ζ1E0κA0(A−A0−∫AA0Ψ(A0)Ψ(ξ)dξ)+γϱνζ2B+η∫h10χ1(τ)∫tt−τΨ(A(s))E(s)S(s)dsdτ+δIζ2∫h20χ2(τ)∫tt−τI(s)dsdτ. | (3.18) |
We note that ˉΛ0(E,I,S,A,B)>0 for all E,I,S,A,B>0 and ˉΛ0(E0,0,0,A0,0)=0. We evaluate dˉΛ0dt as follows:
dˉΛ0dt=ζ1(1−E0E)˙E+˙I+1νζ2˙S+ζ1E0κA0(1−Ψ(A0)Ψ(A))˙A+γϱνζ2˙B+ηddt∫h10χ1(τ)∫tt−τΨ(A(s))E(s)S(s)dsdτ+δIζ2ddt∫h20χ2(τ)∫tt−τI(s)dsdτ. |
Using system (3.1), we get
dˉΛ0dt=ζ1(1−E0E)[λE−ηΨ(A)ES−δEE]+η∫h10χ1(τ)Ψ(Aτ)EτSτdτ−δII+1νζ2[δIν∫h20χ2(τ)Iτdτ−δSS−γSB]+ζ1E0κA0(1−Ψ(A0)Ψ(A))[λA−κηΨ(A)SA−δAA]+γϱνζ2[ϱSB−δBB]+η∫h10χ1(τ)[Ψ(A)ES−Ψ(Aτ)EτSτ]dτ+δIζ2∫h20χ2(τ)[I−Iτ]dτ. |
Collecting terms, we get
dˉΛ0dt=ζ1(1−E0E)[λE−δEE]+ηζ1Ψ(A)E0S−δSνζ2S+ηζ1Ψ(A0)E0S−ηζ1Ψ(A0)E0S+ζ1E0κA0(1−Ψ(A0)Ψ(A))[λA−δAA]−ζ1E0A0(Ψ(A)−Ψ(A0))ηSA−γδBϱνζ2B=ζ1(E−E0E)[λE−δEE]+(ηζ1Ψ(A0)E0−δSνζ2)S+ηζ1E0S(Ψ(A)−Ψ(A0))+ζ1E0κA0Ψ(A)(Ψ(A)−Ψ(A0))[λA−δAA]−ζ1E0A0(Ψ(A)−Ψ(A0))ηSA−γδBϱνζ2B. |
Using the equilibrium condition λE=δEE0, as well as λA=δAA0, we get
dˉΛ0dt=−ζ1δE(E−E0)2E+δSνζ2(νζ1ζ2ηΨ(A0)E0δS−1)S+ηζ1E0S(Ψ(A)−Ψ(A0))A0A0+ζ1δAE0κA0Ψ(A)(Ψ(A)−Ψ(A0))(A0−A)−ηζ1E0A0S(Ψ(A)−Ψ(A0))A−γδBϱνζ2B=−ζ1δE(E−E0)2E+δSνζ2(¯ℜ0−1)S+(ηζ1E0SA0+ζ1δAE0κA0Ψ(A))×(Ψ(A)−Ψ(A0))(A0−A)−γδBϱνζ2B. |
Since ˉℜ0≤1 and (Ψ(A)−Ψ(A0))(A0−A)≤0, then dˉΛ0dt≤0 for all E,S,A,B>0. In addition, dˉΛ0dt=0 when E=E0, A=A0 and S=B=0. Trajectories of system (3.1) converge to ˉ˜Ω0, where S=0 and ˙S=0. The third equation of system (3.1) gives
0=˙S=δIν∫h20χ2(τ)Iτdτ⟹I(t)=0 for all t. |
Therefore, ˉ˜Ω0={Δ0} and by using the LIP, we obtain that Δ0 is G.A.S.
To show the instability of Δ0, we calculate the characteristic equation of system (3.1) at Δ0 as follows:
0=(c+δE)(c+δB)[c3+(δI+δS+δA)c2+(δSδA+δI(δS+δA)−η¯ζ1¯ζ2δIνΨ(A0)E0)c+δIδSδA−η¯ζ1¯ζ2δIνδAΨ(A0)E0], |
where ¯ζi=∫hi0fi(τ)e−(c+αi)τdτ,i=1,2. Define a function ˉT(c) as follows:
ˉT(c)=c3+(δI+δS+δA)c2+(δSδA+δI(δS+δA)−η¯ζ1¯ζ2δIνΨ(A0)E0)c+δIδSδA−η¯ζ1¯ζ2δIνδAΨ(A0)E0, |
which is continuous on [0,∞). We have
ˉT(0)=δIδSδA(1−ˉℜ0)<0 when ˉℜ0>1,limc→∞ˉT(c)=∞; |
this shows that ˉT(c) has a positive real root; therefore, Δ0 is unstable.
Theorem 5. Consider system (3.1) and suppose that Assumption (A) is satisfied and ˉℜ1≤1<ˉℜ0; then, Δ1 is G.A.S.
Proof. Define
ˉΛ1=ζ1E1Φ(EE1)+I1Φ(II1)+1νζ2S1Φ(SS1)+ζ1E1κA1(A−A1−∫AA1Ψ(A1)Ψ(ξ)dξ)+γϱνζ2B+ηΨ(A1)E1S1∫h10χ1(τ)∫tt−τΦ(Ψ(A(s))E(s)S(s)Ψ(A1)E1S1)dsdτ+δIζ2I1∫h20χ2(τ)∫tt−τΦ(I(s)I1)dsdτ. | (3.19) |
Clearly, ˉΛ1(E,I,S,A,B)>0 for all E,I,S,A,B>0 and ˉΛ1(E1,I1,S1,A1,0)=0. We obtain dˉΛ1dt as follows:
dˉΛ1dt=ζ1(1−E1E)˙E+(1−I1I)˙I+1νζ2(1−S1S)˙S+ζ1E1κA1(1−Ψ(A1)Ψ(A))˙A+γϱνζ2˙B+ηΨ(A1)E1S1ddt∫h10χ1(τ)×∫tt−τΦ(Ψ(A(s))E(s)S(s)Ψ(A1)E1S1)dsdτ+δIζ2I1ddt∫h20χ2(τ)∫tt−τΦ(I(s)I1)dsdτ. |
Using system (3.1), we get
dˉΛ1dt=ζ1(1−E1E)[λE−ηΨ(A)ES−δEE]+(1−I1I)[η∫h10χ1(τ)Ψ(Aτ)EτSτdτ−δII]+1νζ2(1−S1S)[δIν∫h20χ2(τ)Iτdτ−δSS−γSB]+ζ1E1κA1(1−Ψ(A1)Ψ(A))[λA−κηΨ(A)SA−δAA]+γϱνζ2[ϱSB−δBB]+ηΨ(A1)E1S1∫h10χ1(τ)[Ψ(A)ESΨ(A1)E1S1−Ψ(Aτ)EτSτΨ(A1)E1S1+ln(Ψ(Aτ)EτSτΨ(A)ES)]dτ+δIζ2I1∫h20χ2(τ)[II1−IτI1+ln(IτI)]dτ. |
Collecting terms, we get
dˉΛ1dt=ζ1(1−E1E)[λE−δEE]+ζ1ηΨ(A)E1S−η∫h10χ1(τ)Ψ(Aτ)EτSτI1Idτ+δII1−δSνζ2S−δIζ2∫h20χ2(τ)IτS1Sdτ+δSνζ2S1+γνζ2S1B+ζ1E1κA1(1−Ψ(A1)Ψ(A))×[λA−δAA]−ζ1E1A1ηSA(Ψ(A)−Ψ(A1))−γδBϱνζ2B+ηΨ(A1)E1S1×∫h10χ1(τ)ln(Ψ(Aτ)EτSτΨ(A)ES)dτ+δIζ2I1∫h20χ2(τ)ln(IτI)dτ. |
Using the equilibrium condition for Δ1, i.e.,
λE=ηΨ(A1)E1S1+δEE1, δII1=ηζ1Ψ(A1)E1S1,δSS1=δIνζ2I1, λA=κηΨ(A1)S1A1+δAA1, |
we obtain
dˉΛ1dt=−ζ1δE(E−E1)2E+4δII1−δII1E1E+ζ1ηΨ(A)E1S−δIζ1I1∫h10χ1(τ)Ψ(Aτ)EτSτI1Ψ(A1)E1S1Idτ−ζ1ηΨ(A1)E1S−δIζ2I1∫h20χ2(τ)IτS1I1Sdτ+(γνζ2S1−γδBϱνζ2)B+ζ1δAE1κA1Ψ(A)(Ψ(A)−Ψ(A1))×(A1−A)−δII1Ψ(A1)Ψ(A)−ηζ1E1A1(Ψ(A)−Ψ(A1))SA+δIζ1I1∫h10χ1(τ)ln(Ψ(Aτ)EτSτΨ(A)ES)dτ+δIζ2I1∫h20χ2(τ)ln(IτI)dτ=−ζ1δE(E−E1)2E+4δII1−δII1E1E+ηζ1E1S(Ψ(A)−Ψ(A1))−δIζ1I1∫h10χ1(τ)Ψ(Aτ)EτSτI1Ψ(A1)E1S1Idτ−δIζ2I1∫h20χ2(τ)IτS1I1Sdτ+γνζ2(S1−δBϱ)B+ζ1δAE1κA1Ψ(A)(Ψ(A)−Ψ(A1))(A1−A)−δII1Ψ(A1)Ψ(A)−ηζ1E1A1(Ψ(A)−Ψ(A1))SA+δIζ1I1∫h10χ1(τ)ln(Ψ(Aτ)EτSτΨ(A)ES)dτ+δIζ2I1∫h20χ2(τ)ln(IτI)dτ. |
Using the equalities
ln(Ψ(Aτ)EτSτΨ(A)ES)=ln(Ψ(Aτ)EτSτIiΨ(Ai)EiSiI)+ln(Ψ(Ai)Ψ(A))+ln(ISiIiS)+ln(EiE),ln(IτI)=ln(IτSiIiS)+ln(IiSISi), i=1,2, | (3.20) |
we obtain
dˉΛ1dt=−ζ1δE(E−E1)2E−δII1[Φ(E1E)+1ζ1∫h10χ1(τ)Φ(Ψ(Aτ)EτSτI1Ψ(A1)E1S1I)dτ+1ζ2∫h20χ2(τ)Φ(IτS1I1S)dτ+Φ(Ψ(A1)Ψ(A))]+γνζ2(S1−δBϱ)B+[ζ1δAE1κA1Ψ(A)+ηζ1E1SA1](Ψ(A)−Ψ(A1))(A1−A). | (3.21) |
We have that (Ψ(A)−Ψ(A1))(A1−A)≤0, and, from Assumption (A), we have that S1−δBϱ≤0. It follows that dˉΛ1dt≤0 for all E,I,S,A,B>0. In addition, dˉΛ1dt=0 when E=E1, A=A1, B=0 and
IτS1I1S=Ψ(Aτ)EτSτI1Ψ(A1)E1S1I=1 for almost all τ∈[0,τ∗]. | (3.22) |
All solutions of system (3.1) are attracted to ˉ˜Ω1. Since ˉ˜Ω1 is invariant with respect to (3.1), on ˉ˜Ω1, we have
0=˙E=λE−ηΨ(A1)E1S−δEE1⟹S(t)=S1 for any t, |
and, from Eq (3.22), we get that I(t)=Iτ=I1 for any t. Therefore, ˉ˜Ω1={Δ1}, and by applying the LIP, we obtain that Δ1 is G.A.S.
Theorem 6. For system (3.1), let ˉℜ1>1; then, Δ2 is G.A.S.
Proof. Define
ˉΛ2=ζ1E2Φ(EE2)+I2Φ(II2)+1νζ2S2Φ(SS2)+ζ1E2κA2(A−A2−∫AA2Ψ(A2)Ψ(ξ)dξ)+γϱνζ2B2Φ(BB2)+ηΨ(A2)E2S2∫h10χ1(τ)∫tt−τΦ(Ψ(A(s))E(s)S(s)Ψ(A2)E2S2)dsdτ+δIζ2I2∫h20χ2(τ)∫tt−τΦ(I(s)I2)dsdτ. | (3.23) |
Obviously, ˉΛ2(E,I,S,A,B)>0 for all E,I,S,A,B>0 and ˉΛ2(E2,I2,S2,A2,B2)=0. We calculate dˉΛ2dt as follows:
dˉΛ2dt=ζ1(1−E2E)˙E+(1−I2I)˙I+1νζ2(1−S2S)˙S+ζ1E2κA2(1−Ψ(A2)Ψ(A))˙A+γϱνζ2(1−B2B)˙B+ηΨ(A2)E2S2ddt∫h10χ1(τ)∫tt−τΦ(Ψ(A(s))E(s)S(s)Ψ(A2)E2S2)dsdτ+δIζ2I2ddt∫h20χ2(τ)∫tt−τΦ(I(s)I2)dsdτ. |
From system (3.1), we get
dˉΛ2dt=ζ1(1−E2E)[λE−ηΨ(A)ES−δEE]+(1−I2I)[η∫h10χ1(τ)Ψ(Aτ)EτSτdτ−δII]+1νζ2(1−S2S)[δIν∫h20χ2(τ)Iτdτ−δSS−γSB]+ζ1E2κA2(1−Ψ(A2)Ψ(A))[λA−κηΨ(A)SA−δAA]+γϱνζ2(1−B2B)[ϱSB−δBB]+ηΨ(A2)E2S2∫h10χ1(τ)[Ψ(A)ESΨ(A2)E2S2−Ψ(Aτ)EτSτΨ(A2)E2S2+ln(Ψ(Aτ)EτSτΨ(A)ES)]dτ+δIζ2I2∫h20χ2(τ)dτ[II2−IτI2+ln(IτI)]dτ. |
Collecting terms, we get
dˉΛ2dt=ζ1(1−E2E)[λE−δEE]+ηζ1Ψ(A)E2S−η∫h10χ1(τ)Ψ(Aτ)EτSτI2Idτ+δII2−δSνζ2S−δIζ2×∫h20χ2(τ)IτS2Sdτ+δSνζ2S2+γνζ2S2B+ζ1E2κA2(1−Ψ(A2)Ψ(A))[λA−δAA]−ζ1E2A2(Ψ(A)−Ψ(A2))ηSA−γδBϱνζ2B−γνζ2SB2+γδBϱνζ2B2+ηΨ(A2)E2S2×∫h10χ1(τ)ln(Ψ(Aτ)EτSτΨ(A)ES)dτ+δIζ2I2∫h20χ2(τ)ln(IτI)dτ. |
Using the equilibrium condition for Δ2, i.e.,
λE=ηΨ(A2)E2S2+δEE2, δII2=ηζ1Ψ(A2)E2S2,δSS2=δIνζ2I2−γS2B2, λA=κηΨ(A2)S2A2+δAA2, S2=δBϱ, |
we obtain
dˉΛ2dt=−δEζ1(E−E2)2E+4δII2−δII2E2E+ζ1ηΨ(A)E2S−δIζ1I2∫h10χ1(τ)Ψ(Aτ)EτSτI2Ψ(A2)E2S2Idτ−ηζ1Ψ(A2)E2S−δIζ2I2∫h20χ2(τ)IτS2I2Sdτ+ζ1δAE2κA2Ψ(A)(Ψ(A)−Ψ(A2))(A2−A)−δII2Ψ(A2)Ψ(A)−ζ1E2A2ηSA(Ψ(A)−Ψ(A2))+δIζ1I2∫h10χ1(τ)×ln(Ψ(Aτ)EτSτΨ(A)ES)dτ+δIζ2I2∫h20χ2(τ)ln(IτI)dτ=−δEζ1(E−E2)2E+4δII2−δII2E2E+ζ1ηE2S(Ψ(A)−Ψ(A2))−δIζ1I2∫h10χ1(τ)Ψ(Aτ)EτSτI2Ψ(A2)E2S2Idτ−δIζ2I2∫h20χ2(τ)IτS2I2Sdτ+ζ1δAE2κA2Ψ(A)(Ψ(A)−Ψ(A2))(A2−A)−δII2Ψ(A2)Ψ(A)−ζ1E2A2ηSA×(Ψ(A)−Ψ(A2))+δIζ1I2∫h10χ1(τ)ln(Ψ(Aτ)EτSτΨ(A)ES)dτ+δIζ2I2∫h20χ2(τ)ln(IτI)dτ. |
Applying the equalities of (3.20) for i=2, we obtain
dˉΛ2dt=−δEζ1(E−E2)2E−δII2[Φ(E2E)+1ζ1∫h10χ1(τ)Φ(Ψ(Aτ)EτSτI2Ψ(A2)E2S2I)dτ+1ζ2∫h20χ2(τ)Φ(IτS2I2S)+Φ(Ψ(A2)Ψ(A))]+[ζ1δAE2κA2Ψ(A)+ζ1ηSE2A2]×(Ψ(A)−Ψ(A2))(A2−A). | (3.24) |
If ˉℜ1>1, we get that dˉΛ2dt≤0 for all E,I,S,A>0. Further, dˉΛ2dt=0 when E=E2, A=A2 and
IτS2I2S=ˉΨ(Aτ)EτSτI2Ψ(A2)E2S2I=1 for almost all τ∈[0,τ∗]. | (3.25) |
All solutions of system (3.1) are attracted to ˉ˜Ω2. Since ˉ˜Ω2 is invariant with respect to (3.1), on ˉ˜Ω2, we have
0=˙E=λE−ηΨ(A2)E2S−δEE2⟹S(t)=S2 for any t, |
and, from Eq (3.25), we get that I(t)=Iτ=I2 for any t. The third equation of system (3.1) yields
0=˙S=δIνζ2I2−γS2B−δSS2⟹B(t)=B2 for any t. |
Hence, ˉ˜Ω2={Δ2} and, by utilizing the LIP, we get that Δ2 is G.A.S.
Let us compare our proposed model (2.1) and the model given by Eqs (1.1)–(1.4), which was studied in [27]. We consider the administration of a treatment to inhibit the virus replication with a drug efficacy ϵI∈[0,1] [43]. Then, model (2.1) becomes
{˙E(t)=λE−ηΨ(A(t))E(t)S(t)−δEE(t),˙I(t)=e−α1τ1ηΨ(A(t−τ1))E(t−τ1)S(t−τ1)−δII(t),˙S(t)=(1−ϵI)e−α2τ2δIνI(t−τ2)−δSS(t)−γS(t)B(t),˙A(t)=λA−κηΨ(A(t))A(t)S(t)−δAA(t),˙B(t)=ϱS(t)B(t)−δBB(t). | (4.1) |
The basic reproduction number of system (4.1) is given by
ℜϵI0=(1−ϵI)e−α1τ1−α2τ2ηνΨ(A0)E0δS=(1−ϵI)ℜ0, | (4.2) |
where ℜ0 is the basic reproduction number of system (2.1) (i.e., there is no treatment). Assume that ℜ0>1; then, the uninfected equilibrium Δ0 for system (2.1) is unstable. Now, we want to determine the range of medication efficacy, ϵI, that stabilizes system (4.1)'s equilibrium Δ0 and makes ℜϵI0≤1:
1≥ϵI≥ϵminI=ℜ0−1ℜ0. | (4.3) |
On the other hand, the model given by Eqs (1.1)–(1.4) under the effect of treatment becomes
{˙E(t)=λE−ηΨ(A(t))E(t)S(t)−δEE(t),˙I(t)=e−α1τ1ηΨ(A(t−τ1))E(t−τ1)S(t−τ1)−δII(t),˙S(t)=(1−ϵI)δIνI(t)−δSS(t),˙A(t)=λA−κηΨ(A(t))A(t)S(t)−δAA(t), | (4.4) |
and the basic reproduction number of system (4.4) is given by
ˆℜϵI0=(1−ϵI)e−α1τ1ηνΨ(A0)E0δS=(1−ϵI)ˆℜ0, |
where ˆℜ0 is the basic reproduction number of the system given by Eqs (1.1)–(1.4), which is assumed to be ˆℜ0>1. We determine the drug efficacy ϵI that makes ˆℜϵI0≤1 and stabilizes the uninfected equilibrium, ˉΔ0, of system (4.4) as follows:
1≥ϵI≥ˆϵminI=ˆℜ0−1ˆℜ0. | (4.5) |
Since τ2>0, then
ℜ0=e−α1τ1−α2τ2ηνΨ(A0)E0δS<e−α1τ1ηνΨ(A0)E0δS=ˆℜ0. |
It follows from Eqs (4.3) and (4.5) that ϵminI<ˆϵminI. As a result, adding the maturation delay τ2 to the system will lessen the amount of medication required to stabilize it at the uninfected equilibrium Δ0 and eradicate SARS-CoV-2 from the body. Thus, designing overflow antiviral medications will result from neglecting the maturation delay in SARS-CoV-2 infection models.
When we look at our proposed model (2.1) and the model given by Eqs (1.1)–(1.4), we can see that our model admits three equilibria, uninfected equilibrium (Δ0): infected equilibrium without humoral immunity (Δ1) and infected equilibrium with humoral immunity (Δ2). On the other hand, the model given by Eqs (1.1)–(1.4) admits only two equilibria:
(ⅰ) Uninfected equilibrium, ˉΔ0=(E0,0,0,A0), where the SARS-CoV-2 infection is cleared.
(ⅱ) Infected equilibrium ˉΔ1=(E1,I1,S1,A1), where the SARS-CoV-2 infection is present.
This shows that ignoring the effect of humoral immunity in the SARS-CoV-2 infection model may not accurately describe SARS-CoV-2 infection. Thus, our proposed models are more relevant as a tool to describe the within-host dynamics of SARS-CoV-2 infection than the model presented in [27].
The above comparisons underscore the significance of including both the humoral response and maturation delay in the SARS-CoV-2 infection paradigm.
In this section, we describe the numerical simulation for model (2.1) to illustrate the theoretical findings. We performed sensitivity analysis for the model. We demonstrate here the effect of humoral immunity and time delays on the SARS-CoV-2 dynamics. The system of DDEs were solved numerically by using the dde23 solver in MATLAB version R2022a. Table 1 contains the values of some parameters of model (2.1). The other values were chosen just for numerical purposes. We chose the function Ψ as Ψ(A)=AnAns+An [27,28]. Then ℜ0, given by Eq (2.3) becomes
ℜ0=e−α1τ1−α2τ2ηνE0δSAn0Ans+An0. | (5.1) |
Parameter | Value | Parameter | Value | Parameter | Value | Parameter | Value |
λE | 5 | ϱ | Varied | δE | 0.1 | δB | 0.1 |
η | Varied | As | 50 | δI | 0.1 | α2 | 1 |
ν | 20 | α1 | 1 | δS | 0.1 | τ2 | Varied |
γ | 0.04 | τ1 | Varied | λA | 1 | n | 1 |
κ | 0.3 | δA | 0.1 |
To show the global stability of the equilibria of system (2.1), we applied the following three initial conditions:
C1:(E(θ),I(θ),S(θ),A(θ),B(θ))=(20,2,3,6,1),C2:(E(θ),I(θ),S(θ),A(θ),B(θ))=(30,4,5,8,2),C3:(E(θ),I(θ),S(θ),A(θ),B(θ))=(45,6,8,9.5,3), |
where θ∈[−max{τ1,τ2},0]. Here, we set τ1=τ2=0.9 and selected the values of η and ϱ as follows:
State 1. (Stability of Δ0) η=0.003 and ϱ=0.001. These values give ℜ0=0.826494<1. Figure 2 shows that the trajectories tend to the equilibrium Δ0=(50,0,0,10,0) for all initial conditions C1–C3. This shows that Δ0 is G.A.S according to Theorem 1. In this state, SARS-CoV-2 particles are eventually cleared.
State 2. (Stability of Δ1) η=0.01 and ϱ=0.001. With such a selection, we obtain that ℜ1=0.923507<1<2.75498=ℜ0 and S1=88.157<δBϱ=0.10.001=100. The equilibrium point Δ1 exists with Δ1=(23.3341,10.8416,88.157,7.44692,0). Figure 3 shows that the trajectories tend, eventually, to Δ1 for all initial conditions, and this is in agreement with Theorem 2. This state describes an infected individual when humoral immunity is not activated.
State 3. (Stability of Δ2) η=0.01 and ϱ=0.005. This gives ℜ1=1.65788>1. The numerical results show that Δ2=(38.1854,4.8035,20,9.1506,2.3824) exists. Figure 4 displays that the trajectories converge eventually to Δ2 for all initial conditions and this is consistent with Theorem 3. This state describes an infected individual with active humoral immunity.
We show the effects of time delays τ1 and τ2 on solutions of the system, as well as the stability of Δ0. We can see from Eq (5.1) that ℜ0 is reduced by increasing the delay parameters τ1 and τ2 when all other parameters are fixed. Therefore, the stability of Δ0 can significantly be changed based on τ1 and τ2. Let us fix η=0.003, ϱ=0.01 and vary τ1 and τ2 as follows:
D1: τ1=τ2=0,
D2: τ1=τ2=0.79,
D3: τ1=τ2=1,
D4: τ1=τ2=2.
Further, we consider the following initial condition:
C4:(E(θ),I(θ),S(θ),A(θ),B(θ))=(48,1.5,6,9.8,5), θ∈[−max{τ1,τ2}.0]. |
Assume that τ=τ1=τ2; then, ℜ0, in the case of n=1, is given by
ℜ0=e−(α1+α2)τηνλEλAδS(AsδEδA+λAδE). | (5.2) |
We see that ℜ0 is a decreasing function of τ. Let τcr be such that ℜ0(τcr)=1. Consequently,
ℜ0≤1 for all τ≥τcr. |
Hence, Δ0 is G.A.S when τ≥τcr=0.804719. Therefore, we have the following cases:
(ⅰ) If τ≥τcr, then ℜ0≤1 and Δ0 is G.A.S. Therefore, when τ is large enough, then Δ0 can be stabilized.
(ⅱ) If τ<τcr, then ℜ0>1 and Δ0 will be unstable.
Figure 5 shows the effect of time delay on the system's trajectories. It is clear that, as τ is increased, the population of the uninfected epithelial cells and ACE2 receptors are increased, while the populations of infected epithelial cells, SARS-CoV-2 particles and antibodies are decreased.
This subsection addresses the effect of the stimulated rate constant ϱ on the dynamics of system (2.1). We fix the parameters η=0.01 and τ1=τ2=0.9 and vary the parameter ϱ as follows: ϱ=0.001, ϱ=0.003, ϱ=0.005 and ϱ=0.007. Further, we consider the following initial condition:
C5:(E(θ),I(θ),S(θ),A(θ),B(θ))=(35,6,30,9,2), θ∈[−0.9,0]. |
The impact of humoral immunity on SARS-CoV-2 infection can be seen in Figure 6. We observe that, as ϱ is increased, the concentrations of uninfected epithelial cells, antibodies and ACE2 receptors are increased, while concentrations of infected cells and SARS-CoV-2 particles are decreased. We note that ℜ0 does not depend on the humoral immune parameters; therefore, humoral immunity plays the role of controlling the infection, but not clearing it. This may help to develop drug therapies with the ability to stimulate the humoral response.
Sensitivity analysis is crucial in pathology and epidemiology when modeling complex interactions [41]. Sensitivity analysis can help us to assess how well we are able to prevent the progression of the disease between hosts and within the host. Three techniques may be used to determine sensitivity indices: Directly by direct differentiation, with the use of a Latin hypercube sampling technique or by linearizing the system and resolving the resultant equations [41,42]. With the use of direct differentiation, the indices in this study may be stated analytically. When variables fluctuate according to the parameters, one may get the sensitivity index by using partial derivatives. The normalized forward sensitivity index of ℜ0 is written in terms of the parameter m:
Sm=mℜ0∂ℜ0∂m. | (5.3) |
Using the values given in Table 1 and η=0.003, ϱ=0.001 and τ1=τ2=0.9, we present the sensitivity index Sm in Table 2 and Figure 7. Obviously, λE, η, λA and ν have positive indices. Clearly, λE, η and ν have the most positive sensitivity index. In this state, there is a positive relationship between the progression of COVID-19 and the parameters λE, η, λA and ν when all other parameters are fixed. The parameters δE, δS, δA, τ1, τ2, α1, α2 and As have negative indices, meaning that, when the values of these parameters rise, the value of ℜ0 declines. Obviously, n, δE and δS have the most negative sensitivity index.
m | Sm | m | Sm | m | Sm | m | Sm |
λE | 1 | δA | −0.833 | η | 1 | α2 | −0.9 |
ν | 1 | τ1 | −0.9 | δE | −1 | λA | 0.833 |
δS | −1 | τ2 | −0.9 | α1 | −0.9 | As | −0.833 |
n | −1.3412 |
Since the beginning of the outbreak of SARS-CoV-2 at the end of 2019, many researchers have formulated and developed mathematical models to characterize the dynamics of the virus within the host. Most of these models neglect the role of ACE2 receptors in the infection. In this paper, we studied two SARS-CoV-2 infection models which describe the within-host dynamics of SARS-CoV-2 by considering the role of ACE2 receptors. The effects of humoral immunity and time delays on the SARS-CoV-2 infection was included.
The model admits three equilibrium points, as follows:
● The uninfected equilibrium, Δ0, usually exists. Moreover, Δ0 is G.A.S when ℜ0≤1, and it is unstable otherwise. In this state, the number of SARS-CoV-2 particles eventually converges to 0 and the COVID-19 patient will recover. Different control strategies can be applied to realize
ℜ0=e−α1τ1−α2τ2ηνλEλAδS(AsδEδA+λAδE)≤1. | (6.1) |
These strategies are provided for example:
(ⅰ) Reducing the parameter η as (1−ϵB)η by applying treatment to block the virus binding with drug efficacy ϵB∈[0,1] [43].
(ⅱ) Reducing the parameter ν as (1−ϵI)ν by using treatment to inhibit the virus replication with drug efficacy ϵI∈[0,1] [43].
(ⅲ) Enlarging the length of delay periods τ1 and τ2 [44].
(ⅳ) Inhibiting the proliferation rate of ACE2 receptors, λA [27].
(ⅴ) Increasing the degradation rate of ACE2 receptors, δA [27].
We observe that model (2.1) may be seen as a nonlinear control system with drug efficacies (e.g., ϵB and ϵI) serving as the control inputs when medicines are used. Then, a variety of control design techniques, including feedback control [49], model predictive control [50,51] and optimal control [19,48], may be applied.
● The infected equilibrium without humoral immunity, Δ1, exists when ℜ0>1. Further, Δ1 is G.A.S when ℜ1≤1<ℜ0 and S>δB/ϱ. In this case, the infection is present, but with an inactive immune response. The reason for this is that the amount of viruses present in the body is small, that, is S≤δB/ϱ, and it may be insufficient to activate the immune system's reaction.
● The infected equilibrium with humoral immunity, Δ2, exists and is G.A.S when ℜ0>1. In this case, the amount of viruses present in the body is sufficient to activate (i.e., S>δB/ϱ) the immune system's reaction.
The main limitation of our research is that we were not able to use real data from COVID-19 patients to estimate the values of the model's parameters. The following are the reasons: (ⅰ) Real data from infected people are still lacking; (ⅱ) comparing our findings to a small number of real studies may not be very accurate; (ⅲ) it is challenging to collect real data from patients who are SARS-CoV-2-infected; and (ⅳ) conducting experiments to obtain real data is outside the scope of this study.
In this paper, we studied two SARS-CoV-2 infection models which describe the within-host dynamics of SARS-CoV-2 by considering the role of ACE2 receptors. The effect of humoral immunity on the SARS-CoV-2 infection was included. Two time-delays were incorporated: (ⅰ) Delay in the SARS-CoV-2 infection of epithelial cells, and (ⅱ) delay in the maturation of recently released SARS-CoV-2 virions. In the first model, we consider discrete-time delays, which are generalized in the second model by considering distributed-time delays. We first showed the fundamental properties of the solutions, non-negativity and boundedness. Then, we established that the models have three equilibria. On the basis of the two threshold parameters, ℜ0 and ℜ1, we proved the existence and global stability of the equilibria. We constructed suitable Lyapunov functions and used the LIP to prove the global asymptotic stability of the three equilibria. We solved the model numerically, presented the results graphically and found agreement between the numerical and theoretical findings. We discussed the respective impacts of humoral immunity, time delay and ACE2 receptors on the SARS-CoV-2 dynamics. We showed that humoral immunity plays the role of controlling the infection, but it does not ultimately clear SARS-CoV-2 particles. Further, increasing the time delay length can significantly decrease ℜ0 and then inhibit COVID-19 progression. This opens the door for the creation of some treatments that will prolong the delay period. We discussed the mediated effect of the ACE2 receptors. We found that ℜ0 is affected by the proliferation and degradation rates of ACE2 receptors, and this may serve as worthy insight for the development of possible receptor-targeted vaccines and drugs. Finally, we performed the sensitivity analysis to establish how the values of the model's parameters affect ℜ0.
Our suggested model may be expanded in several ways by incorporating (ⅰ) latently infected cells [6], (ⅱ) immune response delay [10], (ⅲ) the CTL response, the other component of the adaptive immune response [12], (ⅳ) stochastic interactions [52,53], (ⅴ) reaction diffusion [16,54] and (ⅵ) immunologic memory by formulation of the model using fractional differential equations [48]. By assuming that the generic functions provide the production/stimulation, infection and clearance rates of compartments, our models can be made more widely applicable [16]. In future work, we will examine the modeling and analysis of coinfections between two SARS-CoV-2 variants, such as Omicron and Delta [55,56]. It is possible to direct future research to incorporate the impact of vaccinations and antiviral medications into the model. We also want to compare the outcomes with data from infected patients.
The authors declare that they have not used artificial intelligence (AI) tools in the creation of this article.
This research work was funded by Institutional Fund Projects under grant no. (IFPIP: 1103-130-1443). The authors gratefully acknowledge the technical and financial support provided by the Ministry of Education and King Abdulaziz University, DSR, Jeddah, Saudi Arabia.
The authors declare no conflict of interest.
[1] |
E. Vermisoglou, D. Panáček, K. Jayaramulu, M. Pykal, I. Frébort, M. Kolář, et al., Human virus detection with graphenebased materials, Biosens. Bioelectron., 166 (2020), 112436. https://doi.org/10.1016/j.bios.2020.112436 doi: 10.1016/j.bios.2020.112436
![]() |
[2] | World Health Organization (WHO), Coronavirus disease (COVID-19), weekly epidemiological update, 2023. Available from: https://www.who.int/publications/m/item/weekly-epidemiological-update-on-covid-19–-1-september-2023. |
[3] |
C. B. Jackson, M. Farzan, B. Chen, H. Choe, Mechanisms of SARS-CoV-2 entry into cells, Nat. Rev. Mol. Cell Bio., 23 (2022), 3–20. https://doi.org/10.1038/s41580-021-00418-x doi: 10.1038/s41580-021-00418-x
![]() |
[4] |
P. Zhou, X. L. Yang, X. G. Wang, B. Hu, L. Zhang, W. Zhang, et al., A pneumonia outbreak associated with a new coronavirus of probable bat origin, Nature, 579 (2020), 270–273. https://doi.org/10.1038/s41586-020-2012-7 doi: 10.1038/s41586-020-2012-7
![]() |
[5] |
Y. Wan, J. Shang, R. Graham, R. S. Baric, F. Li, Receptor recognition by the novel coronavirus from Wuhan: An analysis based on decade-long structural studies of SARS coronavirus, J. Virol., 94 (2020). https://doi.org/10.1128/jvi.00127-20 doi: 10.1128/jvi.00127-20
![]() |
[6] |
E. A. H. Vargas, J. X. V. Hernandez, In-host mathematical modelling of COVID-19 in humans, Ann. Rev. Control, 50 (2020), 448–456. https://doi.org/10.1016/j.arcontrol.2020.09.006 doi: 10.1016/j.arcontrol.2020.09.006
![]() |
[7] |
C. Li, J. Xu, J. Liu, Y. Zhou, The within-host viral kinetics of SARS-CoV-2, Math. Biosci. Eng., 17 (2020), 2853–2861. https://doi.org/10.3934/mbe.2020159 doi: 10.3934/mbe.2020159
![]() |
[8] |
R. Ke, C. Zitzmann, D. D. Ho, R. M. Ribeiro, A. S. Perelson, In vivo kinetics of SARS-CoV-2 infection and its relationship with a person's infectiousness, P. Nat. A. Sci., 118 (2021), e2111477118. https://doi.org/10.1073/pnas.2111477118 doi: 10.1073/pnas.2111477118
![]() |
[9] |
M. Sadria, A. T. Layton, Modeling within-host SARS-CoV-2 infection dynamics and potential treatments, Viruses, 13 (2021), 1141. https://doi.org/10.3390/v13061141 doi: 10.3390/v13061141
![]() |
[10] |
I. Ghosh, Within host dynamics of SARS-CoV-2 in humans: Modeling immune responses and antiviral treatments, SN Comput. Sci., 2 (2021), 482. https://doi.org/10.1007/s42979-021-00919-8 doi: 10.1007/s42979-021-00919-8
![]() |
[11] |
S. Q. Du, W. Yuan, Mathematical modeling of interaction between innate and adaptive immune responses in COVID-19 and implications for viral pathogenesis, J. Med. Virol., 92 (2020), 1615–1628. https://doi.org/10.1002/jmv.25866 doi: 10.1002/jmv.25866
![]() |
[12] |
K. Hattaf, N. Yousfi, Dynamics of SARS-CoV-2 infection model with two modes of transmission and immune response, Math. Biosci. Eng., 17 (2020), 5326–5340. https://doi.org/10.3934/mbe.2020288 doi: 10.3934/mbe.2020288
![]() |
[13] |
J. Mondal, P. Samui, A. N. Chatterjee, Dynamical demeanour of SARS-CoV-2 virus undergoing immune response mechanism in COVID-19 pandemic, Eur. Phys. J.-Spec. Top., 231 (2022), 3357–3370. https://doi.org/10.1140/epjs/s11734-022-00437-5 doi: 10.1140/epjs/s11734-022-00437-5
![]() |
[14] |
A. E. S. Almoceraa, G. Quiroz, E. A. H. Vargas, Stability analysis in COVID-19 within-host model with immune response, Commun. Nonlinear Sci., 95 (2021), 105584. https://doi.org/10.1016/j.cnsns.2020.105584 doi: 10.1016/j.cnsns.2020.105584
![]() |
[15] |
A. M. Elaiw, A. J. Alsaedi, A. D. Hobiny, S. Aly, Stability of a delayed SARS-CoV-2 reactivation model with logistic growth and adaptive immune response, Physica A, 616 (2023), 128604. https://doi.org/10.1016/j.physa.2023.128604 doi: 10.1016/j.physa.2023.128604
![]() |
[16] |
P. Wu, X. Wang, Z. Feng, Spatial and temporal dynamics of SARS-CoV-2: Modeling, analysis and simulation, Appl. Math. Model., 113 (2023), 220–240. https://doi.org/10.1016/j.apm.2022.09.006 doi: 10.1016/j.apm.2022.09.006
![]() |
[17] |
A. Gonçalves, J. Bertrand, R. Ke, E. Comets, X. D. Lamballerie, D. Malvy, et al., Timing of antiviral treatment initiation is critical to reduce SARS-CoV-2 viral load, CPT-Pharmacomet. Syst., 9 (2020), 509–514. https://doi.org/10.1002/psp4.12543 doi: 10.1002/psp4.12543
![]() |
[18] |
P. Abuin, A. Anderson, A. Ferramosca, E. A. H. Vargas, A. H. Gonzalez, Characterization of SARS-CoV-2 dynamics in the host, Ann. Rev. Control, 50 (2020), 457–468. https://doi.org/10.1016/j.arcontrol.2020.09.008 doi: 10.1016/j.arcontrol.2020.09.008
![]() |
[19] |
B. Chhetri, V. M. Bhagat, D. K. K. Vamsi, V. S. Ananth, D. B. Prakash, R. Mandale, et al., Within-host mathematical modeling on crucial inflammatory mediators and drug interventions in COVID-19 identifies combination therapy to be most effective and optimal, Alex. Eng. J., 60 (2021), 2491–2512. https://doi.org/10.1016/j.aej.2020.12.011 doi: 10.1016/j.aej.2020.12.011
![]() |
[20] |
T. Song, Y. Wang, X. Gu, S. Qiao, Modeling the within-host dynamics of SARS-CoV-2 infection based on antiviral treatment, Mathematics, 11 (2023), 3485. https://doi.org/10.3390/math11163485 doi: 10.3390/math11163485
![]() |
[21] |
A. M. Elaiw, A. J. Alsaedi, A. D. Al Agha, A. D. Hobiny, Global stability of a humoral immunity COVID-19 model with logistic growth and delays, Mathematics, 10 (2022), 1857. https://doi.org/10.3390/math10111857 doi: 10.3390/math10111857
![]() |
[22] |
S. Tang, W. Ma, P. Bai, A novel dynamic model describing the spread of the MERS-CoV and the expression of dipeptidyl peptidase 4, Comput. Math. Method. M., 2017 (2017), 5285810. https://doi.org/10.1155/2017/5285810 doi: 10.1155/2017/5285810
![]() |
[23] |
T. Keyoumu, W. Ma, K. Guo, Existence of positive periodic solutions for a class of in-host MERS-CoV infection model with periodic coefficients, AIMS Math., 7 (2021), 3083–3096. https://doi.org/10.3934/math.2022171 doi: 10.3934/math.2022171
![]() |
[24] |
T. Keyoumu, K. Guo, W. Ma, Periodic oscillation for a class of in-host MERS-CoV infection model with CTL immune response, Math. Biosci. Eng., 19 (2022), 12247–12259. https://doi.org/10.3934/mbe.2022570 doi: 10.3934/mbe.2022570
![]() |
[25] |
T. Keyoumu, W. Ma, K. Guo, Global stability of a MERS-CoV infection model with CTL immune response and intracellular delay, Mathematics, 11 (2023), 1066. https://doi.org/10.3390/math11041066 doi: 10.3390/math11041066
![]() |
[26] |
A. N. Chatterjee, F. Al Basir, A model for SARS-CoV-2 infection with treatment, Comput. Math. Method. M., 2020 (2020), 1352982. https://doi.org/10.1155/2020/1352982 doi: 10.1155/2020/1352982
![]() |
[27] |
J. Lv, W. Ma, Global asymptotic stability of a delay differential equation model for SARS-CoV-2 virus infection mediated by ACE2 receptor protein, Appl. Math. Lett., 142 (2023), 108631. https://doi.org/10.1016/j.aml.2023.108631 doi: 10.1016/j.aml.2023.108631
![]() |
[28] |
N. Bairagi, D. Adak, Global analysis of HIV-1 dynamics with Hill type infection rate and intracellular delay, Appl. Math. Model., 38 (2014), 5047–5066. https://doi.org/10.1016/j.apm.2014.03.010 doi: 10.1016/j.apm.2014.03.010
![]() |
[29] | Y. Kuang, Delay differential equations with applications in population dynamics, Boston: Academic Press, 1993. |
[30] | H. L. Smith, P. Waltman, The theory of the chemostat: Dynamics of microbial competition, Cambridge University Press, 1995. |
[31] |
X. Yang, L. Chen, J. Chen, Permanence and positive periodic solution for the single-species nonautonomous delay diffusive models, Comput. Math. Appl., 32 (1996), 109–116. https://doi.org/10.1016/0898-1221(96)00129-0 doi: 10.1016/0898-1221(96)00129-0
![]() |
[32] |
P. V. D. Driessche, J. Watmough, Reproduction numbers and sub-threshold endemic equilibria for compartmental models of disease transmission, Math. Biosci., 180 (2002), 29–48. https://doi.org/10.1016/S0025-5564(02)00108-6 doi: 10.1016/S0025-5564(02)00108-6
![]() |
[33] |
A. Korobeinikov, Global properties of basic virus dynamics models, B. Math. Biol., 66 (2004), 879–883. https://doi.org/10.1016/j.bulm.2004.02.001 doi: 10.1016/j.bulm.2004.02.001
![]() |
[34] |
R. Xu, Global dynamics of an HIV-1 infection model with distributed intracellular delays, Comput. Math. Appl., 61 (2011), 2799–2805. https://doi.org/10.1016/j.camwa.2011.03.050 doi: 10.1016/j.camwa.2011.03.050
![]() |
[35] |
R. V. Culshaw, S. Ruan, G. Webb, A mathematical model of cell-to-cell spread of HIV-1 that includes a time delay, J. Math. Biol., 46 (2003), 425–444. https://doi.org/10.1007/s00285-002-0191-5 doi: 10.1007/s00285-002-0191-5
![]() |
[36] |
Y. Nakata, Global dynamics of a cell mediated immunity in viral infection models with distributed delays, J. Math. Anal. Appl., 375 (2011), 14–27. https://doi.org/10.1016/j.jmaa.2010.08.025 doi: 10.1016/j.jmaa.2010.08.025
![]() |
[37] | J. K. Hale, S. M. V. Lunel, Introduction to functional differential equations, New York: Springer-Verlag, 1993. |
[38] |
H. Yang, J. Wei, Analyzing global stability of a viral model with general incidence rate and cytotoxic T lymphocytes immune response, Nonlinear Dynam., 82 (2015), 713–722. https://doi.org/10.1007/s11071-015-2189-8 doi: 10.1007/s11071-015-2189-8
![]() |
[39] | H. K. Khalil, Nonlinear systems, 3 Eds., Upper Saddle River: Prentice Hall, 2002. |
[40] |
C. Huang, B. Liu, C. Qian, J. Cao, Stability on positive pseudo almost periodic solutions of HPDCNNs incorporating D operator, Math. Comput. Simulat., 190 (2021), 1150–1163. https://doi.org/10.1016/j.matcom.2021.06.027 doi: 10.1016/j.matcom.2021.06.027
![]() |
[41] |
S. Marino, I. B. Hogue, C. J. Ray, D. E. Kirschner, A methodology for performing global uncertainty and sensitivity analysis in systems biology, J. Theor. Biol., 254 (2008), 178–196. https://doi.org/10.1016/j.jtbi.2008.04.011 doi: 10.1016/j.jtbi.2008.04.011
![]() |
[42] |
A. Khan, R. Zarin, G. Hussain, N. A. Ahmad, M. H. Mohd, A. Yusuf, Stability analysis and optimal control of covid-19 with convex incidence rate in Khyber Pakhtunkhawa (Pakistan), Results Phys., 20 (2021), 103703. https://doi.org/10.1016/j.jtbi.2008.04.011 doi: 10.1016/j.jtbi.2008.04.011
![]() |
[43] |
I. Al-Darabsah, K. L. Liao, S. Portet, A simple in-host model for COVID-19 with treatments: Model prediction and calibration, J. Math. Biol., 86 (2023), 20. https://doi.org/10.1007/s00285-022-01849-6 doi: 10.1007/s00285-022-01849-6
![]() |
[44] |
G. Huang, Y. Takeuchi, W. Ma, Lyapunov functionals for delay differential equations model of viral infections, SIAM J. Appl. Math., 70 (2010), 2693–2708. https://doi.org/10.1137/090780821 doi: 10.1137/090780821
![]() |
[45] |
W. Guo, Q. Zhang, X. Li, M. Ye, Finite-time stability and optimal impulsive control for age-structured HIV model with time-varying delay and Lévy noise, Nonlinear Dynam., 106 (2021), 3669–3696. https://doi.org/10.1007/s11071-021-06974-3 doi: 10.1007/s11071-021-06974-3
![]() |
[46] |
B. Liu, Global exponential stability for BAM neural networks with time-varying delays in the leakage terms, Nonlinear Anal.-Real, 14 (2013), 559–566. https://doi.org/10.1016/j.nonrwa.2012.07.016 doi: 10.1016/j.nonrwa.2012.07.016
![]() |
[47] |
A. Rezounenko, Continuous solutions to a viral infection model with general incidence rate, discrete state-dependent delay, CTL and antibody immune responses, Electronic J. Qual. Theo., 2016 (2016), 1–15. http://doi.org/10.14232/ejqtde.2016.1.79 doi: 10.14232/ejqtde.2016.1.79
![]() |
[48] |
A. N. Chatterjee, F. Al Basir, M. A. Almuqrin, J. Mondal, I. Khan, SARS-CoV-2 infection with lytic and nonlytic immune responses: A fractional order optimal control theoretical study, Results Phys., 26 (2021), 104260. https://doi.org/10.1016/j.rinp.2021.104260 doi: 10.1016/j.rinp.2021.104260
![]() |
[49] |
H. T. Banks, H. D. Kwon, J. A. Toivanen, H. T. Tran, A state-dependent Riccati equation-based estimator approach for HIV feedback control, Optim. Contr. Appl. Met., 27 (2006), 93–121. https://doi.org/10.1002/oca.773 doi: 10.1002/oca.773
![]() |
[50] |
A. M. Elaiw, X. Xia, HIV dynamics: Analysis and robust multirate MPC-based treatment schedules, J. Math. Anal. Appl., 359 (2009), 285–301. https://doi.org/10.1016/j.jtbi.2008.04.011 doi: 10.1016/j.jtbi.2008.04.011
![]() |
[51] |
T. Péni, B. Csutak, G. Szederkényi, G. Röst, Nonlinear model predictive control with logic constraints for COVID-19 management, Nonlinear Dynam., 102 (2020), 1965–1986. https://doi.org/10.1007/s11071-020-05980-1 doi: 10.1007/s11071-020-05980-1
![]() |
[52] |
L. Gibelli, A. M. Elaiw, M. A. Alghamdi, A. M. Althiabi, Heterogeneous population dynamics of active particles: Progression, mutations, and selection dynamics, Math. Mod. Meth. Appl. S., 27 (2017), 617–640. https://doi.org/10.1142/S0218202517500117 doi: 10.1142/S0218202517500117
![]() |
[53] |
W. Wang, Mean-square exponential input-to-state stability of stochastic fuzzy delayed Cohen-Grossberg neural networks, J. Exp. Theor. Artif. In., 2023, 1–14. https://doi.org/10.1080/0952813X.2023.2165725 doi: 10.1080/0952813X.2023.2165725
![]() |
[54] |
A. M. Elaiw, A. D. AlAgha, Analysis of a delayed and diffusive oncolytic M1 virotherapy model with immune response, Nonlinear Anal.-Real, 55 (2020), 103116. https://doi.org/10.1016/j.nonrwa.2020.103116 doi: 10.1016/j.nonrwa.2020.103116
![]() |
[55] |
N. Bellomo, D. Burini, N. Outada, Multiscale models of Covid-19 with mutations and variants, Netw. Heterog. Media, 17 (2022), 293–310. https://doi.org/10.3934/nhm.2022008 doi: 10.3934/nhm.2022008
![]() |
[56] |
R. J. Rockett, J. Draper, M. Gall, E. M. Sim, A. Arnott, J. E. Agius, et al., Co-infection with SARS-CoV-2 Omicron and Delta variants revealed by genomic surveillance, Nat. Commun., 13 (2022), 2745. https://doi.org/10.1038/s41467-022-30518-x doi: 10.1038/s41467-022-30518-x
![]() |
1. | Saeed Ahmad, Wedad Albalawi, Nadir Omer, Control of scabies fluctuation during COVID-19 pandemic, 2025, 110, 11100168, 193, 10.1016/j.aej.2024.10.004 |
Parameter | Value | Parameter | Value | Parameter | Value | Parameter | Value |
λE | 5 | ϱ | Varied | δE | 0.1 | δB | 0.1 |
η | Varied | As | 50 | δI | 0.1 | α2 | 1 |
ν | 20 | α1 | 1 | δS | 0.1 | τ2 | Varied |
γ | 0.04 | τ1 | Varied | λA | 1 | n | 1 |
κ | 0.3 | δA | 0.1 |
m | Sm | m | Sm | m | Sm | m | Sm |
λE | 1 | δA | −0.833 | η | 1 | α2 | −0.9 |
ν | 1 | τ1 | −0.9 | δE | −1 | λA | 0.833 |
δS | −1 | τ2 | −0.9 | α1 | −0.9 | As | −0.833 |
n | −1.3412 |
Parameter | Value | Parameter | Value | Parameter | Value | Parameter | Value |
λE | 5 | ϱ | Varied | δE | 0.1 | δB | 0.1 |
η | Varied | As | 50 | δI | 0.1 | α2 | 1 |
ν | 20 | α1 | 1 | δS | 0.1 | τ2 | Varied |
γ | 0.04 | τ1 | Varied | λA | 1 | n | 1 |
κ | 0.3 | δA | 0.1 |
m | Sm | m | Sm | m | Sm | m | Sm |
λE | 1 | δA | −0.833 | η | 1 | α2 | −0.9 |
ν | 1 | τ1 | −0.9 | δE | −1 | λA | 0.833 |
δS | −1 | τ2 | −0.9 | α1 | −0.9 | As | −0.833 |
n | −1.3412 |