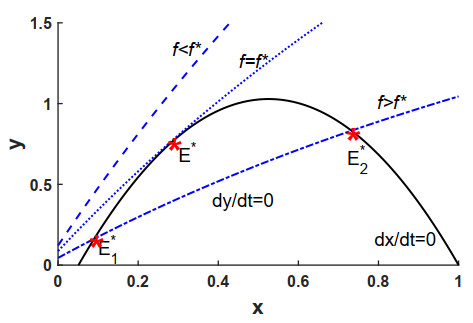
The Allee effect is an important mechanism in ecosystems and a realistic description of the interaction between species. The study of the predator-prey model with the Allee effect is of great significance to promote the development of marine ecology. In this work, three aspects of studies are presented: Modelling and analysis: a predator-prey fishery model with the Allee effect in prey and generalist predator is first established. The existence, type, and stability of the boundary equilibria as well as the number of interior equilibria of the proposed model are discussed. Parameter influence: the bifurcations in the predation system are analyzed by selecting the capture rate of prey by the predator and Allee threshold as key parameters, and the results show that the system will undergo saddle-node bifurcation and Bogdanov-Takens bifurcation of codimension at least 2 and 3. Control measures: a bilateral intervention strategy is adopted for the capture and protection of marine fish. The existence and stability of the order-1 periodic solution and the order-2 periodic solution of the control system are analyzed by using the differential equation geometry theory. Additionally, numerical simulations are carried out to verify the correctness of the conclusions, and illustrate the impact of the Allee effect and bilateral intervention on the ecosystem, which provides an effective method for modern fishery conservation and harvesting.
Citation: Yuan Tian, Yang Liu, Kaibiao Sun. Complex dynamics of a predator-prey fishery model: The impact of the Allee effect and bilateral intervention[J]. Electronic Research Archive, 2024, 32(11): 6379-6404. doi: 10.3934/era.2024297
[1] | Yichao Shao, Hengguo Yu, Chenglei Jin, Jingzhe Fang, Min Zhao . Dynamics analysis of a predator-prey model with Allee effect and harvesting effort. Electronic Research Archive, 2024, 32(10): 5682-5716. doi: 10.3934/era.2024263 |
[2] | Yujia Xiang, Yuqi Jiao, Xin Wang, Ruizhi Yang . Dynamics of a delayed diffusive predator-prey model with Allee effect and nonlocal competition in prey and hunting cooperation in predator. Electronic Research Archive, 2023, 31(4): 2120-2138. doi: 10.3934/era.2023109 |
[3] | Zhuo Ba, Xianyi Li . Period-doubling bifurcation and Neimark-Sacker bifurcation of a discrete predator-prey model with Allee effect and cannibalism. Electronic Research Archive, 2023, 31(3): 1405-1438. doi: 10.3934/era.2023072 |
[4] | Xin Du, Quansheng Liu, Yuanhong Bi . Bifurcation analysis of a two–dimensional p53 gene regulatory network without and with time delay. Electronic Research Archive, 2024, 32(1): 293-316. doi: 10.3934/era.2024014 |
[5] | Fengrong Zhang, Ruining Chen . Spatiotemporal patterns of a delayed diffusive prey-predator model with prey-taxis. Electronic Research Archive, 2024, 32(7): 4723-4740. doi: 10.3934/era.2024215 |
[6] | Jiange Dong, Xianyi Li . Bifurcation of a discrete predator-prey model with increasing functional response and constant-yield prey harvesting. Electronic Research Archive, 2022, 30(10): 3930-3948. doi: 10.3934/era.2022200 |
[7] | Mengting Sui, Yanfei Du . Bifurcations, stability switches and chaos in a diffusive predator-prey model with fear response delay. Electronic Research Archive, 2023, 31(9): 5124-5150. doi: 10.3934/era.2023262 |
[8] | Mengxin He, Zhong Li . Dynamic behaviors of a Leslie-Gower predator-prey model with Smith growth and constant-yield harvesting. Electronic Research Archive, 2024, 32(11): 6424-6442. doi: 10.3934/era.2024299 |
[9] | Yanhe Qiao, Hui Cao, Guoming Xu . A double time-delay Holling Ⅱ predation model with weak Allee effect and age-structure. Electronic Research Archive, 2024, 32(3): 1749-1769. doi: 10.3934/era.2024080 |
[10] | Ruizhi Yang, Dan Jin . Dynamics in a predator-prey model with memory effect in predator and fear effect in prey. Electronic Research Archive, 2022, 30(4): 1322-1339. doi: 10.3934/era.2022069 |
The Allee effect is an important mechanism in ecosystems and a realistic description of the interaction between species. The study of the predator-prey model with the Allee effect is of great significance to promote the development of marine ecology. In this work, three aspects of studies are presented: Modelling and analysis: a predator-prey fishery model with the Allee effect in prey and generalist predator is first established. The existence, type, and stability of the boundary equilibria as well as the number of interior equilibria of the proposed model are discussed. Parameter influence: the bifurcations in the predation system are analyzed by selecting the capture rate of prey by the predator and Allee threshold as key parameters, and the results show that the system will undergo saddle-node bifurcation and Bogdanov-Takens bifurcation of codimension at least 2 and 3. Control measures: a bilateral intervention strategy is adopted for the capture and protection of marine fish. The existence and stability of the order-1 periodic solution and the order-2 periodic solution of the control system are analyzed by using the differential equation geometry theory. Additionally, numerical simulations are carried out to verify the correctness of the conclusions, and illustrate the impact of the Allee effect and bilateral intervention on the ecosystem, which provides an effective method for modern fishery conservation and harvesting.
Predator-prey interactions have been an interesting and challenging issue that is frequently discussed in marine ecosystems, especially in fish populations. Predator-prey interactions are the most important component of ecology, determining various factors such as community composition, species behavior and dynamics. Mathematical modeling helps to provide insights into the dynamics of the system, which was investigated in the early pioneering work of Lotka[1] and Volterra[2]. In dynamical systems, continuity, equilibrium stability, bifurcation, and control problems are also often studied[3,4,5,6,7]. Traditionally, predators could be distinguished as specialists or generalists based on whether they ate only one or more types of prey. In general, a predator-prey model can be described by the following ordinary differential equation for both specialists and generalist predators[8]:
˙P=F(P)P−G(P,N)N,˙N=γυ(G(P,N))N+H(N)N, |
where P and N denote the prey and predator's population densities at moment t, respectively. F(⋅) and H(⋅) represent the growth of the species in the absence of the other one. G(⋅) is known as the functional response, which characterizes the average individual of prey consumed by a predator; γ represents the conversion rate from prey consumption to predator, υ(⋅) is a monotonically increasing function. For specialist predators, there is H(N)=−d<0; and for generalist predators, it is required that γυ(G(0,N))+H(N)>0. In literature, different type of functional responses were adopted to model different species, which can be prey dependent[9,10] or prey and predator dependent[11,12,13,14,15].
The classical view of population dynamics claims that the higher the population density, the lower the overall growth rate due to the competition for resources. The lower the density, the higher the overall growth rate. However, when the population density is low, Allee[16] introduced the opposite view that the lower the population density, the lower the overall growth rate, that is, the Allee effect. The Allee effect is a common phenomenon in marine populations[17,18,19]. When the population density is low, it may affect population development due to pairing restrictions, dispersal, habitat changes, cooperative foraging, cooperative defense, and predator saturation. Therefore, the study of the Allee effect on ecosystems has attracted the attention of many scholars. In general, the Allee effect can be represented by a multiplier of the form P-L[20,21,22], where L is the threshold for the Allee effect. When L<0, it is a weak Allee effect, and the Allee effect is always positive no matter how much the prey growth rate decreases. When L>0, it is a strong Allee effect. The strong Allee effect indicates that in order for the population to grow, the population size or density must be higher than L; otherwise, the population will die out. Scholars have analyzed the dynamics of the system with the Allee effect of the form P-L, and discussed the existence of the equilibrium of the system and various bifurcation phenomena such as saddle-node bifurcation and B-T bifurcation[23,24,25].
Fish is a kind of important ecological resources. In view of the fish resources development problems, scholars studied population behavior by adding harvest items on the basis of continuous systems. However, fishing activities are not continuous, so continuous dynamic systems cannot accurately describe the actual fishing process. In the process of fish harvesting, the periodic harvesting of fish is a kind of human-controlled behavior that can be described by an impulse differential equation, and it has been found that the impulse differential equation is more accurate in describing and portraying the dynamical behavior of the population[26]. The theory of semi-continuous dynamic systems has been widely used in modeling research on pest management modeling[27,28,29,30,31]. Based on the analysis of the literature, due to the fact that impulsive differential systems (semi-continuous dynamical systems) have the characteristics of both continuous and discrete dynamical systems, there are some studies applying the theory to the development of fish populations in deterministic environments[32,33,34,35,36] and uncertain environment[37,38,39]. In addition, most of the studies considered fish harvesting or fish protection only (unilateral control); in this study, a bilateral state control[40,41,42] is considered, and a predator-prey model for conservation and harvesting of two fish species is developed by constructing a semi-continuous dynamical system. When designing the state feedback control strategy, the number of objective fish was used as the state variable for feedback control. On the one hand, when the number of prey fish is small, the Allee effect will lead to their extinction, which will lead to the lack of enough food for prey fish and destroy the balance of the ecosystem. Therefore, it is necessary to release a certain amount of prey fish when the number of prey fish decreases to a certain threshold. When the number of prey fish is high, it is necessary to catch prey fish from the economic point of view. Since the fishing behavior will also lead to the harvesting of some predator fish, in order to maintain the ecological balance and avoid the extinction of predator fish caused by the fishing behavior, it is necessary to release a certain amount of predator fish larvae at the same time. Based on the above two aspects, we propose a bilateral control strategy to maintain the population size of the two species in a suitable range.
This paper considers a predator-prey model in which the predator is a generalist and the growth of the prey is affected by the Allee effect. The structure is as follows: In Section Ⅱ, we describe the fishery model, non-negativity, persistent survivability and discuss the existence and stability of equilibria of the model. In Section Ⅲ, the bifurcation dynamics of the model are discussed using bifurcation theory. In Section Ⅳ, based on this model, we analyze the model of marine fish harvesting and conservation with bilateral controls. The existence and stability of the order-1 and order-2 periodic solutions of the system are analyzed by using the geometry theory of differential equations. In the fifth section, we performed numerical simulations using MATLAB to verify the correctness of the results.
In this paper, we present a predator-prey model in which predators are generalists, prey growth rates are logical and subject to strong Allee effects, and the functional response is a Holling-Ⅰ type, while the conversion from prey consumption to predator species is saturated,
{dPdT=rP(1−PK)(P−L)−APN,dNdT=e(AP1+BP)N+(s1+fN−d)N, | (2.1) |
where p(T), N(T) denote the prey and predator's densities at the moment of T; K denotes the prey's environmental holding capacity; L is the threshold of the prey's Allee effect; r and s represent the intrinsic growth rates of prey and predator, respectively; A is the capture rate of prey by the predator; e is the efficiency with which prey is converted to predator; B is the half-saturation constant; f is the intensity of predator density dependence, and d is the predator mortality rate. Since the predators are generalist, it requires that eA≤Bd, s>d, and all parameters of model (2.1) are positive.
To facilitate the analysis, let x=PK, y=N, t=rKT, α=ArK, β=LK, γ=eAr, δ=BK, s1=srK, d1=drK. Then system (2.1) is simplified to system (2.2):
{dxdt=x(1−x)(x−β)−αxy,dydt=γxy1+δx+(s11+fy−d1)y, | (2.2) |
and from the biological point of consideration, the model (2.2) is limited in the region
Ω={(x,y)∈R2+|0≤x≤1,y≥0}. |
On the other hand, as a renewable resource, fish species are closely related to human life. In order to maintain the balance of the ecosystem during the fishing process, we consider a fish stock control strategy with a combination of fishing and investment. First, to avoid the distinction of prey fish caused by the Allee effect, a quantity (η) of juvenile prey fish is released when the prey density declines to the level x=h1. But at a higher level x=h2, a proportion a of prey fish together with a proportion b of predator fish will also be caught for economic purposes, and simultaneously, a quantity τ of juvenile predator fish is released into the system to maintain the level of predator fish. Based on the control measures, the model can be described as follows:
{dxdt=x(1−x)(x−β)−αxydydt=γxy1+δx+(s11+fy−d1)y}h1<x<h2,Δx=ηΔy=0}x=h1,Δx=−axΔy=−by+τ}x=h2, | (2.3) |
where η, a, b, τ are all positive, and a,b∈(0,1).
For a given planar model
{dxdt=f1(x,y),dydt=f2(x,y)ω(x,y)≠0,Δx=I1(x,y),Δy=I2(x,y)ω(x,y)=0, | (2.4) |
Definition 1 (Order-k periodic solution[32,33,36]) The solution ˜z(t)=(˜x(t),˜y(t)) is called periodic if there exists m(⩾1) satisfying ˜zm=˜z0. Furthermore, ˜z is an order-k T-periodic solution with k≜min{j|1≤j≤m,˜zj=˜z0}.
Lemma 1 (Analogue of Poincaré Criterion[32,33,36]). The order-k T-periodic solution z(t)=(ξ(t),η(t))T is orbitally asymptotically stable if |μq|<1, where
μk=k∏j=1Δjexp(∫T0[∂f1∂x+∂f2∂y](ξ(t),η(t))dt), |
with
Δj=f+1(∂I2∂y∂ω∂x−∂I2∂x∂ω∂y+∂ω∂x)+f+2(∂I1∂x∂ω∂y−∂I1∂y∂ω∂x+∂ω∂y)f1∂ω∂x+f2∂ω∂y, |
f+1=f1(ξ(θ+j),η(θ+j)), f+2=f2(ξ(θ+j),η(θ+j)) and f1, f2, ∂I1∂x, ∂I1∂y, ∂I2∂x, ∂I2∂y, ∂ω∂x, ∂ω∂y are calculated at (ξ(θj),η(θj)).
In this section, the bounded-ness of the solution for Model (2.2) is discussed. Moreover, the existence, type, and local stability of the equilibrium as well as the bifurcation properties are verified.
Define
g1(x,y)=(1−x)(x−β)−αy,f1(x,y)=xg1(x,y); |
g2(x,y)=γx1+δx+(s11+fy−d1),f2(x,y)=yg2(x,y). |
Theorem 1. The solution of Model (2.2) with non-negative initial values will remain non-negative for all time and is bounded on R2+.
Proof. By Eq (2.2), it can be obtained that
x(t)=x(0)exp[∫t0g1(x(s),y(s))ds],y(t)=y(0)exp[∫t0g2(x(s),y(s))ds], |
for all t≥0, as long as x(0) and y(0) are non-negative, then x(t) and y(t) are also non-negative.
Next, we define a function u(t)=γαx(t)+y(t). Then
˙u=γα˙x+˙y=γα[x(1−x)(x−β)−αxy]+γxy1+δx+(s11+fy−d1)y≤γα[x(1−x)(x−β)−αxy]+γxy+(s11+fy−d1)y≤γ(1−x)(x−β)αx+s1f−d1y≤[γα((1−β)24+d1)+s1f]−d1u, |
which implies that
u(t)≤1d1[γα((1−β)24+d1)+s1f]+(u0−1d1[γα((1−β)24+d1)+s1f])e−d1t, |
so long as u0=γαx0+y0 is bounded, u(t) is bounded in Ω. To sum up, any solution of Model (2.2) starting with a non-negative bounded initial condition is non-negative and bounded in Ω.
Obviously, four equilibria always exist
O(0,0),E1(0,1f(s1−d1d1)),E2(β,0),E3(1,0). |
Define
y1(x)=1α(1−x)(x−β), | (3.1) |
y2(x)=1f[s1δ(1δ+x)d1−(γ−d1δ)x−1] | (3.2) |
and denote ¯γ1≜d1δ. Due to the assumptions eA≤Bd and s>d, then γ<¯γ1, i.e., y2(x)<s1δ¯γ1−γ. The positional relationship between y1(x) and y2(x) for different cases is shown in Figure 1.
Let y1(x)=y2(x). Then it has
a1x3+a2x2+a3x+a4=:g(x)=0, | (3.3) |
where
a1=f(δd1−γ)>0,a2=f[(γ−δd1)(β+1)+d1],a3=α[δ(s1−d1)+γ]+f[β(δd1−γ)−d1(β+1)],a4=α(s1−d1)+βfd1>0. |
Define
¯α≜fβ,¯δ1≜d1(1+β)s1β,¯δ2≜fd1(1+β)fβd1+α(s1−d1),¯δ3≜(1+β)fd1αs1, |
¯γ2≜αδ(s1−d1)+fd1(βδ−(1+β))fβ−α,xd≜√a22−3a1a3−a23a1,ρ≜g(xd). |
Theorem 2. For any of the following cases: C1) α<¯α, δ<¯δ2, γ<¯γ1; C2) α<¯α, ¯δ2<δ<¯δ3, ¯γ2<γ<¯γ1; C3) α>¯α, δ<¯δ2, 0<γ<−¯γ2, if ρ<0 holds, there exists two interior equilibria in Model (2.2); if ρ=0, there exists a unique interior equilibrium in Model (2.2); if ρ>0, there doesn't exists interior equilibrium in Model (2.2).
Proof. Clearly, the existence of an interior equilibrium is equivalent to that of a positive root of Eq (3.3) in the interval (0, 1). Since
g(0)=a4>0,g(1)=a1+a2+a3+a4=α[(δ+1)(s1−d1)+γ]>0, |
and
g′(x)=3a1x2+2a2x+a3, | (3.4) |
then for any of the cases C1)–C3), there is a3<0. Moreover, g′(0)<0, g′(xd)=0, g″(xd)>0 and
g′(1)=(1−β)f(δd1−γ)+(1−β)d1f+α[δ(s1−d1)+γ]>0, |
so that for x∈(0,xd), g′(x)<0, for x∈(xd,0), g′(x)>0. If ρ<0, Eq (3.3) has two distinct positive roots x∗i∈(0,1), i=1,2. Denote y∗i=y1(x∗i), i=1,2. Then two interior equilibria exist in Model (2.2), denoted by E∗1(x∗1,y∗1) and E∗2(x∗2,y∗2). If ρ=0, Eq (3.3) has a unique positive root x∗=xd∈(0,1), then a unique positive equilibrium exists in Model (2.2), denoted by E∗(x∗,y1(x∗); when ρ>0, Eq (3.3) does not have positive root, and thus there does not exist interior equilibrium in Model (2.2).
For any equilibrium ¯E(¯x,¯y), there is
J(¯E)=[−3¯x2+2(β+1)¯x−β−α¯y−α¯xγ¯y(1+δ¯x)2γ¯x1+δ¯x+s1(1+f¯y)2−d1], |
its characteristic equation is
λ2−TR(J(¯E))λ+DET(J(¯E))=0, |
where
TR(J(¯E))=−3¯x2+2(β+1)¯x−β−α¯y+γ¯x1+δ¯x+s1(1+f¯y)2−d1, |
DET(J(¯E))=[−3¯x+2(β+1)¯x−β−α¯y][γ¯x1+δ¯x+s1(1+f¯y)2−d1]+α¯x(γ¯y(1+δ¯x)2). |
1) Boundary equilibria
At O(0,0), there is
J(O)=[−β00s1−d1], |
since λ1=−β<0, λ2=s1−d1>0, then O is an unstable higher-order singularity.
At E1(0,1f(s1−d1d1)), there is
J(E1)=[−β−αf(s1−d1d1)0γf(s1−d1d1)0], |
since λ1=0, λ2=−αf(s1−d1d1)<0, so that E1 is locally stable.
At E2(β,0), there is
J(E2)=[β(1−β)−αβ0γβ1+δβ+s1−d1], |
since λ1=β(1−β)>0, λ2=γβ1+δβ+s1−d1>0, then E2 is unstable.
At E3(1,0), there is
J(E3)=[β−1−α0γ1+δ+s1−d1], |
since λ1=β−1<0, λ2=γ1+δ+s1−d1>0, then E3 is unstable.
2) Interior equilibrium
At E∗(x∗,y∗), there are g1(x∗,y∗)=0 and g2(x∗,y∗)=0, then
J(E∗)=[(1+β)x∗−2(x∗)2−αx∗γy∗(1+δx∗)2−s1fy∗(1+fy∗)2], |
thus,
DET(J(E))=[x∗y∗∂g1∂y∂g2∂y(dy1dx−dy2dx)](x∗,y∗), |
where x∗y∗∂g1∂y∂g2∂y|x∗,y∗>0, then the sign of DET(J(E∗)) is identical to that of dy1dx|x=x∗−dy2dx|x=x∗. Next, it will discuss the sign of dy1dx|x=x∗−dy2dx|x=x∗ for different cases in Theorem 2.
ⅰ) When ρ<0 holds, two interior equilibria E∗1(x∗1,y∗1) and E∗2(x∗2,y∗2) with 0<x∗1<x∗2<1 exists in Model (2.2), as illustrated in Figure 2(a). It can be easily checked that
SIGN(J(E∗1))=[+−+−],SIGN(J(E∗2))=[−−+−]. |
Besides, at E∗1, there is dy1dx|x=x∗1>dy2dx|x=x∗1, thus
DET(J(E∗1))=[xy∂f1∂y∂f2∂y(dy1dx−dy2dx)](x∗1,y∗1)<0, |
i.e., E∗1 is unstable. Similarly, at E∗2, there is dy1dx|x=x∗2<dy2dx|x=x∗2, thus
(λ1+λ2)|(x∗2,y∗2)=TR(J(E∗2))<0, |
(λ1λ2)|(x∗2,y∗2)=DET(J(E∗2))=[xy∂f1∂y∂f2∂y(dy1dx−dy2dx)](x∗2,y∗2)>0, |
i.e., E∗2 is a locally asymptotically stable node.
ⅱ) When ρ=0 holds, system (2.2) has a unique interior equilibrium E∗(x∗,y∗), as shown in Figure 2(b). Let X=x−x∗,Y=y−y∗, then E∗ is converted to the origin O(0,0), and the model is written as
{dXdt=a11X+a12Y+A1X2+A2XY,dYdt=a21X+a22Y+B1X2+B2XY+B3Y2+P3(X,Y), | (3.5) |
where,
a11=(1+β)x∗−2(x∗)2,a12=−αx∗,a21=γy∗(1+δx∗)2,a22=−s1fy∗(1+fy∗)2,A1=β+1−3x∗,A2=−α2,B1=−γδy∗(1+δx∗)3,B2=γ(1+δx∗)2,B3=−s1f(1+fy∗)3 |
and P3(X,Y) is a function of (X,Y) with degree of three or higher. The Jacobian matrix at E∗ is
J(E∗)=[a11a12a21a22], |
thus
DET(J(E∗))=a11a22−a12a21=0,TR(J(E∗))=a11+a22. |
a) If TR(J(E∗))=a11+a22=0, then λ1=λ2=0. Make the transformation
(XY)=(a110a211)(x1y1), |
then Model (3.5) is converted into the following standard form:
{dx1dt=ˉa12y1+ˉA1x21+ˉA2x1y1,dy1dt=ˉB1x21+ˉB2x1y1+ˉB3y21+ˉP3(x1,y1), |
where
ˉa12=a12a11,ˉA1=a11A1+a21A2,ˉA2=A2, |
ˉB1=a211B1+a11a21(B2−A1)+a221(B3−A2),ˉB2=a11B2+a21(2B3−A2),ˉB3=B3, |
and ˉP3(X,Y) is a function of three or more degrees about (X,Y).
Let τ=ˉa12t. For convenience, t is still used to represent τ, then it has
{dx1dt=y1+˜A1x21+˜A2x1y1,dy1dt=˜B1x21+˜B2x1y1+˜B3y21+˜P3(x1,y1), | (3.6) |
where
˜Ai=ˉAiˉa12,i=1,2,˜Bj=ˉBjˉa12,j=1,2,3. |
and ˜P3(X,Y) is a function of (X,Y) with three or higher degree. Model (3.6) can be transformed into the following form[43]:
{dx1dt=y1,dy1dt=˜B1x21+(˜B2+2˜A1)x1y1+˜P′3(x1,y1), |
and if ˜B1≠0, then E∗ is a cusp point.
Meanwhile, if ˜B2+2˜A1≠0, E∗ is a cusp of codimension two. If ˜B2+2˜A1=0, E∗ is a cusp with at least codimension three.
b) If TR(J(E∗))=a11+a22≠0, then λ1=0, λ2≠0. Make the transformation
(XY)=(a22a11−a21a21)(x1y1), |
system (3.5) is converted into the following standard form
{dx1dt=A′1x21+A′2x1y1+A′y21,dy1dt=a′22y1+B′1x21+B′2x1y1+B′3y21+P′3(x1,y1), |
where
A′1=a21a222A1−a221a22A2−a11a222B1+a11a21a22B2−a11a221B3a21(a11+a22),A′2=2a211a22+a221a22A2−a11a221A2−2a211a22B1−a11a21a22B2+a11a221B2+2a11a221B3a21(a11+a22),A′3=a211a21A1+a11a221A2−a311B1−a211a21B2−a11a221B3a21(a11+a22),B′1=a21a222A1−a221a22A2+a322B1−a21a222B2+a221a22B3a21(a11+a22),B′2=2a11a21a22A2+a221a22A2−a11a221A2+2a11a222B1+a21a222B2−a11a21a22B2−2a221a22B3a21(a11+a22),B′3=a211a21A1+a11a221A2+a11a222B1+a11a21a22B2+a221a22B3a21(a11+a22). |
Next, introduce a new variable τ=a′22t (for convenience, is represented by t), then
{dx1dt=ˆA1x21+ˆA2x1y1+ˆA3y21,dy1dt=y1+ˆB1x21+ˆB2x1y1+ˆB3y21+ˆP3(x1,y1), |
where, ˆAi=A′ia′22,ˆBi=B′ia′22,i=1,2,3.
If ˆA1≠0 then E∗ is unstable. Meanwhile, E∗ is a saddle node of attraction.
To sum up, the following result hold.
Theorem 3. For Model (2.2), 1) O(0,0) is unstable, E1(0,1f(s1−d1d1)) is locally stable, E2(β,0), E3(1,0) is unstable; 2) For the interior equilibrium, when ρ>0, E∗1(x∗1,y∗1) is a saddle (unstable), and E∗2(x∗2,y∗2) is a stable node; when ρ=0, if TR(J(E∗))=0 and ˜B1≠0, E∗(x∗,y∗) is a cusp of codimension two in case of ˜B2+2˜A1≠0, and a cusp with at least codimension three in case of ˜B2+2˜A1=0; If TR(J(E∗))≠0 and ˆA1≠0, E∗(x∗,y∗) is an attractive saddle node.
Let α=α0 satisfy
DET(J(E∗))=(λ1λ2)|(x∗,y∗)=0,TR(J(E∗))=−(λ1+λ2)|(x∗,y∗)≠0. |
Denote
ξ1=2(x∗)2−(1+β)x∗,ξ2=−γy∗(1+δx∗)2,ω1=αx∗,ω2=s1fy∗(1+fy∗)2 |
and define
Φ2Δ=2ω21ξ2x∗−2γδy∗(1+δx∗)3ω21ξ1−2s1f2(1+fy∗)3ξ31. |
Theorem 4. Let the parameters of Model (2.2) satisfy TR(J(E∗))≠0 and ˆA1≠0. If Φ2≠0, system (2.2) undergoes a saddle node bifurcation near E∗(x∗,y∗) when α=α0.
Proof. At E∗(x∗,y∗), there is
J(E∗)=(−ξ1−ω1−ξ2−ω2). |
Let V=(V1,V2)T (W=(W1,W2)T) be the eigenvector corresponding to the zero eigenvalue of J(E∗) ((J(E∗))T). Then V=(ω1,−ξ1)T, W=(−ξ2,ξ1)T. Let g=(g1,g2)T. Then
gα(E∗,α0)=∂g∂α(E∗,α0)=(−y∗0). |
and
D2g(E∗,α0)(V,V)=(∂2g1∂x2V21+2∂2g1∂x∂yV1V2+∂2g1∂y2V22∂2g2∂x2V21+2∂2g2∂x∂yV1V2+∂2g2∂y2V22)=(−2ω21−2γδ(1+δx∗)3ω21−2s1f2(1+fy∗)3ξ21). |
Since
Φ1=WTgα(E∗,α0)=−x∗ξ1≠0 |
and
Φ2=WT[D2g(E∗,α0)(V,V)]=2ω21ξ2−2γδ(1+δx∗)3ω21ξ1−2s1f2(1+fy∗)3ξ31≠0, |
then according to Sotomayor's theorem[44], Model (2.2) undergoes a saddle-node bifurcation near E∗(x∗,y∗) when α=α0.
Remark 1. For Model (2.2), it can be concluded that for a lager α, the interior equilibrium does not exist. When α decreases to α=α0, a unique equilibrium exists in the system. As α decreases below α0, the system undergoes a saddle-node bifurcation at the interior equilibrium E∗, giving rise to two interior equilibria E∗1 and E∗2.
According to Theorem 3, when ρ=0, TR(J(E∗))=0, ˜B1≠0 and ˜B2+2˜A1≠0, Model (2.2) to undergo a Bogdanov-Takens bifurcation of codimension two near E∗ when (α,β)=(α0,β0). Next, it will show the universal unfolding of the Bodmanov-Takens bifurcation of codimension two under parameter perturbation when α and β are taken as bifurcation parameters.
Define
b01=x∗(1−x∗)(x∗−ϵ2)−ϵ1x∗y∗,b11=[1+(β+ϵ2)]x∗−2(x∗)2,b12=−(α+ϵ1)x∗,b10=0,b21=γy∗(1+δx∗)2,b21=−s1fy∗(1+fy∗)2,E1=(β+ϵ2)+1−3x∗,E2=−(α+ϵ1)2,F1=−γδy∗(1+δx∗)3,F2=γ(1+δx∗)2,F3=−s1f(1+fy∗)3,H1=−b212b10+b01b12b22−b201F3b12,H2=b201E22+b212b10E22+b312b21−b11b212b22+b01b212F2b212,H3=b11b12−b01E2+b12b22−2b10F3b12,H4=−b01b12E1E2−b01b11E22−b212b22E1−b212F1+b11b212F2−b211b12F3b212,H5=2b212E21−b11b12E2−2b01E22+b212F2−2b11b12F3b212,H6=E2+F3b12,J1=H1,J2=H2−2H1H6,J3=H3J4=H1H26−2H2H6+H4,J5=H5−H3H6, |
K1=−J1J4,K2=−J2J4,K3=−J3√−J4,K4=−J5√−J4,L1=K1+K224,L2=K3+K2K42,L3=K4,O1=−L1L43,O2=−L2L3, |
Let (ϵ1,ϵ2) be a parameter vector in a small neighborhood of (0,0). Then
Theorem 5. Let the parameters of Model (2.2) satisfy ρ=0, TR(J(E∗))=0, ˜B1≠0, ˜B2+2˜A1≠0 and |∂(O1,O2)∂(ϵ1,ϵ2)|≠0. When (α,β) varies in the neighborhood of (α0,β0), Model (2.2) changes in the small neighborhood of E∗(x∗,y∗), and a codimensional 2 Bogdanov-Takens branching occurs.
Proof. Consider the perturbation system
{dxdt=x(1−x)[x−(γ+ϵ2)]−(α+ϵ1)xy≜F(x,y),dydt=γxy1+δx+(s11+fy−d1)y≜G(x,y), |
For (α,β)=(α0,β0), there is DET(J(E∗))=0,TR(J(E∗))=0. With the transformation x1=x−x∗, y1=y−y∗, we can obtain
{dx1dt=b01+b11x1+b12y1+E1x21+E2x1y1,dy1dt=b10+b21x1+b22y1+F1x21+F2x1y1+F3y21+N3(x1,y1), | (4.1) |
where N3(x1,y1,ϵ1,ϵ2)∈C∞.
Make the following transformation:
{x2=x1,y2=b01+b11x1+b12y1+E1x21+E2x1y1, |
then Model (4.1) is converted to
{dx2dt=y2,dy2dt=H1+H2x2+H3y2+H5x22+H5x2y2+H6y22+N′3(x2,y2,ϵ1,ϵ2), |
where N′3(x2,y2,ϵ1,ϵ2)∈C∞ with coefficients depending smoothly on ϵ1,ϵ2.
Next, introduce the variable τ, denoted as dt=(1−H6x2)dτ (still denote τ as t), then
{dx2dt=(1−H6x2)y2,dy2dt=(1−H6x2)(H1+H2x2+H3y2+H4x22+H5x2y2+H6y22+N′3(x2,y2,λ1,λ2)), |
Let x3=x2,y3=(1−H6x2)y2, then the above system of equations is transformed into
{dx3dt=y3,dy3dt=J1+J2x3+J3y3+J4x23+J5x3y3+˜N3(x3,y3,ϵ1,ϵ2), |
where ˜N3(x3,y3,ϵ1,ϵ2)∈C∞ with coefficients depending smoothly on ϵ1,ϵ2.
(ⅰ) When J4<0, the following transformations are applied to the variables:
x4=x3,y4=y3√−J4,τ=√−J4t, |
still denote τ as t, there is
{dx4dt=y4,dy4dt=K1+K2x4+K3y4−x24+K4x4y4+M3(x4,y4,ϵ1,ϵ2), |
where M3(x4,y4,ϵ1,ϵ2)∈C∞ with at least third order.
Let x5=x4−K22, y5=y4, and obtain
{dx5dt=y5,dy5dt=L1+L2y5−x25+L3x5y5+M′3(x5,y5,ϵ1,ϵ2), |
where M′3(x5,y5,ϵ1,ϵ2)∈C∞ with at least third order.
If set J5≠0, then L3≠0. Define new variables: x6=−L23x5, y6=L33y5, τ=−tL3, and denote x6 by x, y6 by y, and τ by t, which yields that
{dxdt=y,dydt=O1+O2y+x2+xy+˜M3(x,y,λ1,λ2), | (4.2) |
where ˜N3(x2,y2,ϵ1,ϵ2)∈C∞ with at least third order, and O1, O2 can be represented by ϵ1 and ϵ2.
(ⅱ) When J4>0, the following transformations are applied
x4=x3,y4=y3√J4,τ=√J4t, |
still denote τ by t, it has
{dx4dt=y4,dy4dt=K′1+K′2x4+K′3y4+x24+K′4x4y4+N3(x4,y4,ϵ1,ϵ2), |
where N3(x4,y4,ϵ1,ϵ2)∈C∞ with at least third order, and
K′1=J1J4,K′2=J2J4,K′3=J3√J4,K′4=J5√J4. |
Let x5=x4+K′2/2,y5=y4. Then it has
{dx5dt=y5,dy5dt=L′1+L′2y5+x25+L′3x5y5+N′3(x5,y5,ϵ1,ϵ2), |
where N′3(x5,y5,ϵ1,ϵ2)∈C∞ with at least third order, and
L′1=K′1−K′224,L′2=K′3−K′2K′42,L′3=K′4. |
If set J5≠0, then L′3≠0. Define new variables: x6=L′32x5, y6=L′33y5, τ=t/L′3, and still denote x6 by x, y6 by y, τ by t, which yields that
{dxdt=y,dydt=O′1+O′2y+x2+xy+˜N3(x2,y2,ϵ1,ϵ2), | (4.3) |
where O′1=L′1L′34,O′2=L′2L′3, and ϵ1, ϵ2 can be represented by O′1, O′2.
For convenience of discussion, O′1, O′2 is still denoted by O1,O2. When |∂(O1,O2)∂(ϵ1,ϵ2)|≠0, Models (4.2) and (4.3) are the cardinal folds of the Bogdanov-Takens bifurcation[44], when (α,β) varies in the vicinity of (α0,β0), Model (2.2) undergoes a codimension 2 Bogdanov-Takens bifurcation in a small neighborhood of E∗(x∗,y∗).
It only focuses on the case of ρ<0 in Theorem 3. For Model (2.3), there are
M1={(x,y)∈R2+:x=h1,y≥0},M2={(x,y)∈R2+:x=h2,y≥0}, |
N1={(x,y)∈R2+:x=h1+η,y≥0},N2={(x,y)∈R2+:x=(1−a)h2,y≥τ}. |
Denote l1=1α(1−x)(x−β) as the prey isocline, l2=(s1−d1)(1+δx)−γxf[d1+(d1δ−γ)x] as the predator's isocline, l3, l4, l5, l6 as the saddle point separatrix of E∗1 in different directions. The intersection point between l1 and N2 is denoted by A3. The intersection point between l4 and N2 is denoted by M. The trajectory from A3 intersects M2 at the point B3. Define ¯τ2≜yM−(1−b)yB3. Denote
Ω1={(x,y)|0≤x≤x∗1,y≥0},Ω2={(x,y)|x∗1≤x≤1,y≥0}. |
Definition 2 (Successor function). For a point S∈N1, if the trajectory from S intersects M1, then denote the intersection point by S−∈M1. Under the impulse effect, the point S− is mapped to S+∈N1. In such a case, we can define fISOR1: N1→R, S→fISOR1(S)≜yS+−yS. If the trajectory from S intersects M2, then denote the intersection point by S−. Under the impulse effect, the point S− is mapped to S+∈N2. If the trajectory from S+ intersects M1, denote the intersection point by S+−∈M1. Under the impulse effect, the point S+− is mapped to S++∈N1. In such cases, we can define fIISOR1: N1→R, S→fIISOR1(S)≜yS++−yS. Similarly, For a point S′∈N2, define fISOR2: N2→R, S′→fISOR2(S′)≜yS′+−yS′.
Theorem 6. For system (2.3) with model parameters satisfying any one of C1)–C3) and ρ>0, if D-1) h1+η<x∗1<(1−a)h2<h2<x∗2, y2(h1)≥y1(h1+η) and τ<¯τ2, an order-1 periodic solution exists in each Ωi, i=1,2; if D-2) h1<(1−a)h2<h1+η<x∗1<h2<x∗2 and y2(h1)≥y1(h1+η), an order-1 periodic solution exists in Ω1; if D-3) h1<x∗1<h1+η<(1−a)h2<h2<x∗2 and τ<¯τ2, an order-1 periodic solution exists in Ω2.
Proof. For case D-1) h1+η<x∗1<(1−a)h2<h2<x∗2 and y2(h1)≥y1(h1+η), denote the intersection point between l2 and N1 by A0. Select a point A1∈N1 above A0; the trajectory from A1 intersects M1 at B1. Under the impulse effect, the point B1 is mapped to A+1∈N1. Since g1(xA1,yA1)<0, g2(xA1,yA1)<0, then we have fISOR1(A1)=yA+1−yA1=yB1−yA1<0. Besides, denote the intersection between l1 and N1 by A2. Since g1(xA2,yA2)=0, g2(xA2,yA2)>0 and g1(xS,yS)<0 for S∈U(A1,ϵ) with xS<h1+η and yS<yA1, then we have fISOR1(A2)=yA+2−yA2=yB2−yA2>0 due to y2(h1)≥y1(h1+η). The continuity of fISOR1 implies that a point S∈¯A1A2 exists so that fISOR1(S)=0, i.e., an order-1 periodic solution exists in Ω1 (Figure 3(a)). Similarly, it can be proved that an order-1 periodic solution exists in Ω1 for case D-2).
Under the impulse effect, B3 is mapped to A3+∈N2, where yA3+=(1−b)yB3+τ. Define ¯τ1≜yA3−(1−b)yB3.
ⅰ) If τ=¯τ1, then fISOR2(A3)=yA3+−yA3=0, i.e., an order-1 periodic solution exists in Ω1 (Figure 3(a)).
ⅱ) If τ<¯τ1, then fISOR2(A3)=yA3+−yA3<0. On the other hand, for A4((1−a)h2,τ), there is fISOR2(A4)=yA4+−yA4>0. The continuity of fISOR2 implies that a point S′∈¯A3A4 exists so that fISOR2(S′)=0, i.e., an order-1 periodic solution exists in Ω2 (Figure 3(b)).
ⅲ) If ¯τ1<τ<¯τ2, then fISOR2(A3)=yA3+−yA3>0. According to the trend of the trajectory and the fact that any two trajectories cannot be intersected, select D∈N2 above and sufficiently close to the A3, then fISOR2(D)=yD+−yD>0. On the other hand, for C=A+3, there is fISOR2(C)=yC+−yC<yA+3−yC=0. The continuity of fISOR2 implies that a point S′∈¯CD exists so that fISOR2(S′)=0, i.e., an order-1 periodic solution exists in Ω2 (Figure 3(c)).
Similarly, system (2.3) has an order-1 periodic solution in Ω2 for case D-3).
To sum up, an order-1 periodic solution exists in Model (2.3) for case D-1).
Let zi(t)=(ϕi(t),φi(t)) (k−1)Ti≤t≤kTi, k∈N be the order-1 periodic solution in Ωi, i=1,2. For z1(t)=(ϕ1(t),φ1(t)) (k−1)T1≤t≤kT1, denote
ϕ1(T+1)=ϕ1(T1)+η=h1+η,φ1(T+1)=φ1(T1)=δ1. |
For z2(t)=(ϕ2(t),φ2(t)) (k−1)T2≤t≤kT2, denote
ϕ2(T+2)=(1−a)ϕ2(T2)=(1−a)h2,φ2(T+2)=(1−b)φ2(T2)+τ=δ2. |
Theorem 7. For the model parameters with any one of C1)–C3), ρ>0 and D-1), zi(t)=(ϕi(t),φi(t)) (k−1)Ti≤t≤kTi is orbitally asymptotically stable if μi<1, where
μ1=|[1−(h1+η)][(h1+η)−β]−αδ1(1−h1)(h1−β)−αδ1|exp{∫T10+[ϕ1(t)(β+1−2ϕ1(t))−s1fφ1(t)(1+fφ1(t))2]dt},μ2=|{[1−(1−a)h2][(1−a)h2−β]−αδ2}(δ2−τ)δ2[(1−b)(1−h2)(h2−β)−α(δ2−τ)]|Λ(t) |
with Λ(t)=exp{∫T20+[ϕ2(t)(β+1−2ϕ2(t))−s1fφ2(t)(1+fφ2(t))2]dt}.
Proof. For system (2.3), there are
f1(x,y)=x(1−x)(x−β)−αxy,f2(x,y)=γxy1+δx+(s11+fy−d1)y, |
and ω1(x,y)=x−h1,I11(x,y)=η,I21(x,y)=0, So it can be concluded that
∂f1(x,y)∂x=˙xx+x(β+1−2x),∂f2(x,y)∂x=˙yy−s1fy(1+fy)2, |
∂ω1(x,y)∂x=1,∂ω1(x,y)∂y=∂I11(x,y)∂x=∂I11(x,y)∂y=∂I21(x,y)∂x=∂I21(x,y)∂y=0. |
Denote f1+≜f1(ϕ1(T+1),φ1(T+1)),f2+≜f2(ϕ1(T+1),φ1(T+1)). Then by Lemma 1, there are
κ1=(∂I21∂x⋅∂ω1∂x−∂β1∂x⋅∂ω1∂y+∂ω1∂x)f1++(∂I11∂x⋅∂ω1∂y−∂I11∂y⋅∂ω1∂x+∂ω1∂y)f2+∂ω1∂xf1+∂ω1∂yf2=(h1+η)[1−(h1+η)][(h1+η)−β]−α(h1+η)δ1h1(1−h1)(h1−β)−αh1δ1 |
and
μ1=|κ1|exp[∫T10+(∂f1∂x+∂f2∂y)dt]=|[1−(h1+η)][(h1+η)−β]−αδ1(1−h1)(h1−β)−αδ1|exp{∫T10+[ϕ1(t)(β+1−2ϕ1(t))−s1fφ1(t)(1+fφ1(t))2]dt}. |
If μ1<1, the order-1 periodic solution z1(t)=(ϕ1(t),φ1(t)) (k−1)T1≤t≤kT1 is orbitally asymptotically stable.
Similarly, for the order-1 periodic solution z2(t)=(ϕ2(t),φ2(t)) (k−1)T2≤t≤kT2, there is
μ2=|{[1−(1−a)h2][(1−a)h2−β]−αδ2}(δ2−τ)δ2[(1−b)(1−h2)(h2−β)−α(δ2−τ)]|Λ(t), |
where Λ(t)≜exp{∫T20+[ϕ2(t)(β+1−2ϕ2(t))−s1fφ2(t)(1+fφ2(t))2]dt}. By Lemma 1, if μ2<1, the order-1 periodic solution z2(t)=(ϕ2(t),φ2(t)) (k−1)T2≤t≤kT2 is orbitally asymptotically stable. The proof is completed.
Here only the case for h1<(1−a)h2<x∗1<h1+η<h2<x∗2 is considered. Let G1(h1+η,yG1) be the intersection point between l1 and N1, G2(h1,yG2) be the intersection point between l2 and M1. The trajectory that starts with G1 intersects M2 at B1(h2,yB1). Define ¯τ3≜yG1−(1−b)yB1.
Theorem 8. For the model parameters with any one of C1)-C3), ρ>0 and D-4) h1<(1−a)h2<x∗1<h1+η<h2<x∗2, if byG2≤τ≤min{yG2,¯τ3} holds, an order-2 periodic solution exists in system (2.3).
Proof. For G1∈N1, the trajectory starting from G1 intersects with M2 at B1. Then B1 is mapped to B″1∈N2, and next intersects with M1 at ˆB1, and then ˆB1 is mapped to G+1 under impulse effect. Since τ<¯τ3, then yB″1=(1−b)yB1+τ<yG1. Since g1(B″1)<0 and g2(B″1)<0, it has yˆB1<yB″1, then yG+1=yˆB1<yB″1=(1−b)yB1+τ<yG1, i.e., fIISOR1(G1)=yG+1−yG1<0.
On the other hand, since τ≤yG2, then G2∈N1. The trajectory starting from A1∈N1 with yA1=yG2 intersects with M2 at A−1, and then A−1 is mapped to A+1. Then it intersects with M1 at A+−1, and next A+−1 is mapped to A++1. Since yA+1=(1−b)yA−1+τ>(1−b)yG2+τ>yG2, so fIISOR1(A1)=yA++1−yA1=yA+−1−yG2>0.
The continuity of fIISOR1 implies that a point S∈¯A1G1⊂N1 exists so that fIISOR1(S)=0, i.e., an order-2 periodic solution exists (Figure 4(a)).
Similarly, a point S′∈¯A2B2⊂N2 exists so that fIISOR2(S′)=0, i.e., an order-2 periodic solution exists (Figure 4(b)).
Let z3(t)=(ϕ3(t),φ3(t)) (k−1)(T1+T2)≤t≤k(T1+T2), k∈N be the order-2 periodic solution. Denote
ϕ3(0)=h1+η,ϕ3(T1)=h2,ϕ3(T+1)=(1−a)h2,ϕ3(T1+T2)=h1,ϕ3((T1+T2)+)=h1+η, |
φ3(0)=δ3,φ3(T1)=δ4,φ3(T+1)=(1−b)δ4+τ,φ3(T1+T2)=δ3,φ3((T1+T2)+)=δ3. |
Define
Θ0≜δ4(1−b)δ4+τγ1γ3γ2γ4, |
where
γ1≜(1−h1−η)(h1+η−β)−αδ3,γ2≜(1−h2)(h2−β)−αδ4,γ3≜(1−(1−a)h2)((1−a)h2−β)−α((1−b)δ4+τ),γ4≜(1−h1)(h1−β)−αδ3. |
Theorem 9. For the model parameters with any one of C1)–C3), ρ>0 and D-4) h1<(1−a)h2<x∗1<h1+η<h2<x∗2, and byG2≤τ≤min{yG2,¯τ3}, if
μ3=Θ0exp{∫T1+T20+[ϕ3(t)(β+1−2ϕ3(t))−s1fφ3(t)(1+fφ3(t))2]dt}<1, |
then z3(t)=(ϕ3(t),φ3(t)) (k−1)(T1+T2)≤t≤k(T1+T2) is orbitally asymptotically stable.
Proof. For convenience, denote the intersection point between z3(t)=(ϕ3(t),φ3(t)) and N1 (M2, N2, M1) by L1(h1+η,δ3) (L2(h2,δ4), L3((1−a)h2,(1−b)δ4+τ), L4(h1,δ3)). Then, according to analogue of Poincaré Criterion, there are
κ1=f1(L3)f1(L2)=(1−a)h3[(1−(1−a)h2)((1−a)h2−β)−α((1−b)δ4+τ)]h2[(1−h2)(h2−β)−αδ4], |
κ2=f1(L1)f1(L4)=(h1+η)[(1−h1−η)(h1+η−β)−αδ3]h1[(1−h1)(h1−β)−αδ3 |
and
∫T1+T20+(∂f1∂x+∂f2∂y)dt=ln(h2h1+η)+ln(δ4δ3)+ln(h1(1−a)h2)+ln(δ3(1−b)δ4+τ)+∫T1+T20+[ϕ3(t)(β+1−2ϕ3(t))−s1fφ3(t)(1+fφ3(t))2]dt. |
Then
μ3=|κ1κ2|exp(∫T1+T20+(∂f1∂x+∂f2∂y)dt)=δ4(1−b)δ4+τγ1γ3γ2γ4exp(∫T1+T20+[ϕ3(t)(β+1−2ϕ3(t))−s1fφ3(t)(1+fφ3(t))2]dt). |
If μ<1, then z3(t)=(ϕ3(t),φ3(t)) (k−1)(T1+T2)≤t≤k(T1+T2) is orbitally asymptotically stable.
For system (2.2) with model parameters α=0.09, β=0.15, γ=0.6, δ=0.9, s1=1.12, d1=1.1, f=0.21, there is ρ<0, then two interior equilibria exist in the system, and the phase diagram is presented in Figure 5(a); For the model parameters α=0.219, β=0.051, γ=0.6, δ=0.9, s1=1.12, d1=1.1, f=0.21, there is ρ=0, then a unique equilibrium exists in system (2.2), which is a sharp point. The phase diagram of the system (2.2) for such a case is presented in Figure 5(b). Moreover, system (2.2) undergoes a Bogdanov-Takens bifurcation of codimension two in a very small neighborhood of the unique interior equilibrium.
While for system (2.2) with model parameters α=0.09, β=0.29, γ=0.6, δ=0.9, s1=1.12, d1=1.1, f=0.21, there is also ρ=0, in such case a unique equilibrium exists in system (2.2), which is a saddle node, and the phase diagram is presented in Figure 5(c). System (2.2) undergoes a saddle-node bifurcation of codimension one in a very small neighborhood of the interior equilibrium.
For system (2.2) with model parameters α=0.09, β=0.35,γ=0.6, δ=0.9, s1=1.12, d1=1.1, f=0.21, there is ρ>0. In such a case, system (2.2) does not have interior equilibrium, and the phase diagram of the system (2.2) is presented in Figure 5(d).
For system (2.2) with parameters β=0.051, γ=0.6, δ=0.9, s1=1.12, d1=1.1, f=0.21, the bifurcation diagrams of the residual dimension 1 for the system (2.2) are shown in Figure 6(a), (b) when α is selected as the bifurcation parameter. The result shows that for larger α, the interior equilibrium does not exist. When α decreases to α=α0, a unique equilibrium exists in the system. As α decreases below α0, the system undergoes a saddle-node bifurcation at the interior equilibrium E∗, giving rise to two interior equilibria E∗1 and E∗2.
For system (2.3) with model parameters α=0.1, β=0.15, γ=0.6, δ=0.9, s1=1.12, d1=1.1, f=0.21, the control parameters: h1=0.07, η=0.1, h2=0.6, a=0.35, b=0.38, τ=0.51, an order-1 periodic solution can be formed in both Ω1 and Ω2 (Theorem 6), as presented in Figure 7(a); For model parameters α=0.1, β=0.15, γ=0.6, δ=0.9, s1=1.12, d1=1.1, f=0.21 and control parameters h1=0.07, η=0.128, h2=0.44, a=0.7, b=0.08, τ=0.51, an order-1 periodic solution can be formed in Ω1 (Theorem 6), as presented in Figure 7(b); while for control parameters h1=0.14, η=0.16, h2=0.6, a=0.25, b=0.38, τ=0.51, an order-1 periodic solution can be formed (Theorem 6), as presented in Figure 7(c).
For system (2.3) with given model parameters α=0.09, β=0.215, γ=0.6, δ=0.9, s1=1.12, d1=1.1, f=0.21 and control parameters h1=0.07, η=0.1, h2=0.4, a=0.65, b=2, τ=3, an order-2 periodic solution can be formed in system (2.3) (Theorem 3.2), as presented in Figure 8(a); while for the control parameters h1=0.07, η=0.4, h2=0.68, a=0.65, b=0.85, τ=0.5, a different order-2 periodic solution can be formed in system (2.3) (Theorem 8), as presented in Figure 8(b).
Considering that the Allee effect is an important mechanism in ecosystems and a realistic description of the interaction between species, we presented a model of prey-predator system with prey's Allee effect and generalist predator in the context of fish resources (Models (2.1) or (2.2)). We investigated the dynamic properties of Model (2.2) such as the type and stability of the boundary equilibria as well as the existence and stability of the interior equilibrium in detail (Theorems 1–3, Figure 5).
To show the influence of the parameters on the dynamics of Model (2.2), we analyzed the bifurcations in the predation system by selecting the capture rate of prey by predator and Allee threshold as key parameters. We showed that Model (2.2) will undergo a saddle-node bifurcation as changing of the capture rate α (Figure 6), and undergo a Bogdanov-Takens bifurcation of codimension at least 2 and 3 as changing of (α,β).
To achieve sustainable and efficient exploitation of fish stocks, we adopted a bilateral intervention strategy, i.e., to avoid the distinction of prey fish caused by the Allee effect, releasing juvenile prey fish is adopted at a lower-level x=h1; while for economic purposes, capturing both prey and predator fish is adopted at a higher-level x=h2. We obtained the conditions for the existence and stability of the order-1 periodic solution (Theorems 6, 7, Figure 7) and order-2 periodic solution (Theorems 8, 9, Figure 8) of the control system (2.3). The results showed that the extinction can be prevented by control even when the prey density is low, while in the case of the prey density increasing to a certain extent, fishing activities can be taken in a periodic way (periodic solution) to obtain the fish resources. Therefore, as long as the fish stocks are properly managed, the number of fish stocks can be controlled within an appropriate range, and the sustainable development and utilization of biological resources can be realized.
The authors declare they have not used Artificial Intelligence (AI) tools in the creation of this article.
The work was supported by the National Natural Science Foundation of China (No. 11401068).
The authors declare that they have no known competing financial interests or personal relationships that could have appeared to influence the work reported in this paper.
[1] |
A. J. Lotka, Eelements of physical Biology, Am. J. Public Health, 21 (1926), 341–343. https://doi.org/10.2307/2298330 doi: 10.2307/2298330
![]() |
[2] |
V. Volterra, Fluctuations in the abundance of a species considered mathematically, Nature, 118 (1926), 558–560. https://doi.org/10.1038/119012b0 doi: 10.1038/119012b0
![]() |
[3] |
L. Nie, Z. Teng, H. Lin, J. Peng, The dynamics of a Lotka-Volterra predator-prey model with state dependent impulsive harvest for predator, Biosystems, 98 (2009), 67–72. https://doi.org/10.1016/j.biosystems.2009.06.001 doi: 10.1016/j.biosystems.2009.06.001
![]() |
[4] |
M. X. Chen, R. C. Wu, Dynamics of a harvested predator-prey model with predator-taxis, Bull. Malay. Math. Sci. Soc., 46 (2023), 76. https://doi.org/10.1007/s40840-023-01470-w doi: 10.1007/s40840-023-01470-w
![]() |
[5] |
X. R. Yan, Y. Tian, K. B. Sun, Effects of additional food availability and pulse control on the dynamics of a Holling-(p+1) type pest-natural enemy model, Electron. Res. Arch., 31 (2023), 6454–6480. https://doi.org/10.3934/era.2023327 doi: 10.3934/era.2023327
![]() |
[6] |
M. X. Chen, Y. Liu, C. R. Tian, Bifurcations of a single species model with spatial memory environment, Appl. Math. Lett. 154 (2024), 109110. https://doi.org/10.1016/j.aml.2024.109110 doi: 10.1016/j.aml.2024.109110
![]() |
[7] |
X. R. Yan, Y. Tian, K. B. Sun, Dynamic analysis of a delayed pest-natural enemy model: Triple effects of non-monotonic functional response, additional food supply and habitat complexity, Int. J. Biomath., (2024), 2450062. https://doi.org/10.1142/S1793524524500621 doi: 10.1142/S1793524524500621
![]() |
[8] |
A. Erbach, F. Lutscher, G. Seo, Bistability and limit cycles in generalist predator-prey dynamics, Ecol. Complex., 14 (2013), 48–55. https://doi.org/10.1016/j.ecocom.2013.02.005 doi: 10.1016/j.ecocom.2013.02.005
![]() |
[9] |
C. S. Holling, The components of predation as revealed by a study of small-mammal predation of the european pine sawfly, Can. Entomol., 91 (1959), 293–320. https://doi.org/10.4039/Ent91293-5 doi: 10.4039/Ent91293-5
![]() |
[10] |
C. S. Holling, Some characteristics of simple types of predation and parasitism, Can. Entomol., 91 (1959), 385–398. https://doi.org/10.4039/Ent91385-7 doi: 10.4039/Ent91385-7
![]() |
[11] |
R. Arditi, L. R. Ginzburg, Coupling in predator-prey dynamics: ratio-dependence, J. Theor. Biol., 139 (1989), 311–326. https://doi.org/10.1016/S0022-5193(89)80211-5 doi: 10.1016/S0022-5193(89)80211-5
![]() |
[12] |
J. R. Beddington, Mutual interference between parasites or predators and its effect on searching efficiency, J. Anim. Ecol., 44 (1975), 331–340. https://doi.org/10.2307/3866 doi: 10.2307/3866
![]() |
[13] |
R. S. Cantrell, C. Cosner, On the dynamics of predator-prey models with the Beddington-Deangelis functional response, J. Math. Anal. Appl., 257 (2001), 206–222. https://doi.org/10.1006/jmaa.2000.7343 doi: 10.1006/jmaa.2000.7343
![]() |
[14] |
Y. Kuang, E. Beretta, Global qualitative analysis of a ratio-dependent predator-prey system, J. Math. Biol., 36 (1998), 389–406. https://doi.org/10.1007/s002850050105 doi: 10.1007/s002850050105
![]() |
[15] |
D. Xiao, S. Ruan, Global dynamics of a ratio-dependent predator-prey system, J. Math. Biol., 43 (2001), 268–290. https://doi.org/10.1007/s002850100097 doi: 10.1007/s002850100097
![]() |
[16] | W. C. Allee, Animal Aggregations: A Study in General Sociology, The University of Chicago Press, 1931. https://doi.org/10.2307/2961735 |
[17] |
J. Gascoigne, R. Lipcius, Allee effects in marine systems, Mar. Ecol. Prog. Ser., 269 (2004), 49–59. https://doi.org/10.3354/meps269049 doi: 10.3354/meps269049
![]() |
[18] |
P. Aguirre, A general class of predation models with multiplicative Allee effect, Nonlinear Dyn., 78 (2014), 629–648. https://doi.org/10.1007/s11071-014-1465-3 doi: 10.1007/s11071-014-1465-3
![]() |
[19] |
C. Arancibia-Ibarra, J. Flores, G. Pettet, P. Heijster, A holling-tanner predator-prey model with strong allee effect, Int. J Bifurcat. Chaos, 29 (2019), 1930032. https://doi.org/10.1142/S0218127419300325 doi: 10.1142/S0218127419300325
![]() |
[20] |
Y. N. Zeng, P. Yu, Multistable states in a predator-prey model with generalized Holling type Ⅲ functional response and a strong Allee effect, Commun. Nonlinear Sci., 131 (2024), 107846. https://doi.org/10.1016/j.cnsns.2024.107846 doi: 10.1016/j.cnsns.2024.107846
![]() |
[21] |
P. J. Pal, T. S. Saha, Qualitative analysis of a predator-prey system with double Allee effect in prey, Chaos Soliton Fract., 73 (2015), 36–63. https://doi.org/10.1016/j.chaos.2014.12.007 doi: 10.1016/j.chaos.2014.12.007
![]() |
[22] |
A. Arsie, C. Kottegoda, C. H. Shan, A predator-prey system with generalized Holling type Ⅳ functional response and Allee effects in prey, J Differ. Equations, 309 (2022), 704–740. https://doi.org/10.1016/j.jde.2021.11.041 doi: 10.1016/j.jde.2021.11.041
![]() |
[23] |
E. González-Olivares, J. Mena-Lorca, A. Rojas-Palma, J. D. Flores, Dynamical complexities in the Leslie-Gower predator-prey model as consequences of the Allee effect on prey, Appl. Math. Model., 35 (2011), 366–381. https://doi.org/10.1016/j.apm.2010.07.001 doi: 10.1016/j.apm.2010.07.001
![]() |
[24] |
M. Sen, M. Banerjee, A. Morozov, Bifurcation analysis of a ratio-dependent prey-predator model with the Allee effect, Ecol. Complexity, 11 (2012) 12–27. https://doi.org/10.1016/j.ecocom.2012.01.002 doi: 10.1016/j.ecocom.2012.01.002
![]() |
[25] |
D. Sen, S. Ghorai, S. Sharma, M. Banerjee, Allee effect in prey's growth reduces the dynamical complexity in prey-predator model with generalist predator, Appl. Math. Modell., 91 (2020), 768–790. https://doi.org/10.1016/j.apm.2020.09.046 doi: 10.1016/j.apm.2020.09.046
![]() |
[26] |
J. J. Nieto, D. O'Regan, Variational approach to impulsive differential equations, Nonlinear Anal.-Real., 10 (2009), 680–690. https://doi.org/10.1016/j.nonrwa.2007.10.022 doi: 10.1016/j.nonrwa.2007.10.022
![]() |
[27] |
B. Liu, Y. Zhang, L. Chen, The dynamical behaviors of a Lotka-Volterra predator-prey model concerning integrated pest management, Nonlinear Anal.-Real., 6 (2005), 227–243. https://doi.org/10.1016/j.nonrwa.2004.08.001 doi: 10.1016/j.nonrwa.2004.08.001
![]() |
[28] |
X. Y. Song, Y. F. Li, Dynamic behaviors of the periodic predator-prey model with modified Leslie-Gower Holling-type Ⅱ schemes and impulsive effect, Nonlinear Anal.-Real., 9 (2008), 64–79. https://doi.org/10.1016/j.nonrwa.2006.09.004 doi: 10.1016/j.nonrwa.2006.09.004
![]() |
[29] |
S. Tang, W. Pang, R. A. Cheke, J. H. Wu, Global dynamics of a state-dependent feedback control system, Adv. Differ. Equations, 2015 (2015), 1–70. https://doi.org/10.1186/s13662-015-0661-x doi: 10.1186/s13662-015-0661-x
![]() |
[30] |
Q. Zhang, B. Tang, T. Cheng, S. Tang, Bifurcation analysis of a generalized impulsive Kolmogorov model with applications to pest and disease control, SIAM J. Appl. Math., 80 (2020), 1796–1819. https://doi.org/10.1137/19M1279320 doi: 10.1137/19M1279320
![]() |
[31] |
Y. Tian, X. R. Yan, K. B. Sun, Dual effects of additional food supply and threshold control on the dynamics of a Leslie-Gower model with pest herd behavior, Chaos Soliton Fract., 185 (2024), 115163. https://doi.org/10.1016/j.chaos.2024.115163 doi: 10.1016/j.chaos.2024.115163
![]() |
[32] |
H. Li, Y. Tian, Dynamic behavior analysis of a feedback control predator-prey model with exponential fear effect and Hassell-Varley functional response, J Franklin. I., 360 (2023), 3479–3498. https://doi.org/10.1016/j.jfranklin.2022.11.030 doi: 10.1016/j.jfranklin.2022.11.030
![]() |
[33] |
Y. Tian, Y. Gao, K. B. Sun, Global dynamics analysis of instantaneous harvest fishery model guided by weighted escapement strategy, Chaos Soliton Fract., 164 (2022), 112597. https://doi.org/10.1016/j.chaos.2022.112597 doi: 10.1016/j.chaos.2022.112597
![]() |
[34] |
Y. Tian, Y. Gao, K. B. Sun, Qualitative analysis of exponential power rate fishery model and complex dynamics guided by a discontinuous weighted fishing strategy, Commun. Nonlinear Sci., 118 (2023), 107011. https://doi.org/10.1016/j.cnsns.2022.107011 doi: 10.1016/j.cnsns.2022.107011
![]() |
[35] |
Y. Tian, C. X. Li, J. Liu, Non-smooth competitive systems and complex dynamics induced by linearly dependent feedback control, Nonlinear Anal, -Hybri., 51 (2024), 101442. https://doi.org/10.1016/j.nahs.2023.101442 doi: 10.1016/j.nahs.2023.101442
![]() |
[36] |
Y. Tian, H. Li, K. Sun, Complex dynamics of a fishery model: Impact of the triple effects of fear, cooperative hunting and intermittent harvesting, Math. Comput. Simulat., 218 (2024), 31–48. https://doi.org/10.1016/j.matcom.2023.11.024 doi: 10.1016/j.matcom.2023.11.024
![]() |
[37] |
Y. Tian, H. Guo, K. B. Sun, Complex dynamics of two prey–predator harvesting models with prey refuge and interval-valued imprecise parameters, Math. Meth. Appl. Sci., 46 (2023), 14278–14298. https://doi.org/10.1002/mma.9319 doi: 10.1002/mma.9319
![]() |
[38] |
H. Guo, Y. Tian, K. B. Sun, X. Y. Song, Study on dynamic behavior of two fishery harvesting models: effects of variable prey refuge and imprecise biological parameters, J. Appl. Math. Comput., 69 (2023), 4243–4268. https://doi.org/10.1007/s12190-023-01925-0 doi: 10.1007/s12190-023-01925-0
![]() |
[39] |
Y. Tian, C. X. Li, J. Liu, Complex dynamics and optimal harvesting strategy of competitive harvesting models with interval-valued imprecise parameters. Chaos Soliton Fract., 167 (2023), 113084. https://doi.org/10.1016/j.chaos.2022.113084 doi: 10.1016/j.chaos.2022.113084
![]() |
[40] |
J. Xu, M. Z. Huang, X. Y. Song, Dynamical analysis of a two-species competitive system with state feedback impulsive control, Int. J Biomath., 13 (2020), 2050007. https://doi.org/10.1142/S1793524520500072 doi: 10.1142/S1793524520500072
![]() |
[41] |
M. Zhang, Y. Zhao, X. Y. Song, Dynamics of bilateral control system with state feedback for price adjustment strategy, Int. J Biomath., 14 (2021), 2150031. https://doi.org/10.1142/S1793524521500315 doi: 10.1142/S1793524521500315
![]() |
[42] |
J. Xu, M. Z. Huang, X. Y. Song, Dynamics of a guanaco–sheep competitive system with unilateral and bilateral control, Nonlinear Dyn., 107 (2022), 3111–3126. https://doi.org/10.1007/s11071-021-07128-1 doi: 10.1007/s11071-021-07128-1
![]() |
[43] | L. Perko, Differential Equations and Dynamical System, New York: Springer, 1996. |
[44] | J. Sotomayor, Generic Bifurcations of Dynamical Systems, New York: Academic Press, 1973. https://doi.org/10.1016/B978-0-12-550350-1.50047-3 |
1. | Xinrui Yan, Yuan Tian, Kaibiao Sun, Dynamic analysis of additional food provided non-smooth pest-natural enemy models based on nonlinear threshold control, 2025, 1598-5865, 10.1007/s12190-024-02318-7 | |
2. | Yuan Tian, Xinlu Tian, Xinrui Yan, Jie Zheng, Kaibiao Sun, Complex dynamics of non-smooth pest-natural enemy Gomportz models with a variable searching rate based on threshold control, 2025, 33, 2688-1594, 26, 10.3934/era.2025002 | |
3. | Xinrui Yan, Yuan Tian, Kaibiao Sun, Hybrid Effects of Cooperative Hunting and Inner Fear on the Dynamics of a Fishery Model With Additional Food Supplement, 2025, 0170-4214, 10.1002/mma.10805 |