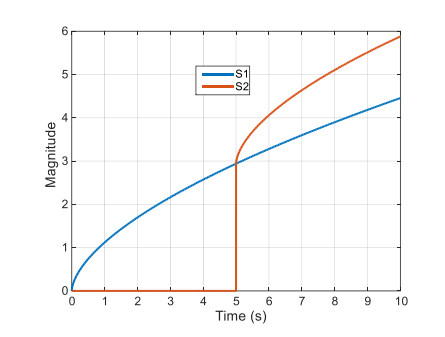
The rising energy prices and soaring environmental concerns have put an immense pressure on the wide usage of machining processes. The total power consumption during machining includes the power consumed by the machine itself and the power used to remove the material from the workpiece. An accurate prediction of energy consumption during the machining process is the basis for energy reduction. In this study, the specific cutting energy and surface finish for low and moderate-speed orthogonal machining of the aluminum alloy 2014 are evaluated. The measured values for the specific cutting energy and surface roughness are presented as maps on a grid, which is based on the machining parameters including the following: (1) cutting speed and (2) undeformed chip thickness. The specific cutting energy map depicts low energy consumption values of 0.52 J/mm3 for the aluminum alloy 2014 at medium speed machining. The roughness maps depict high roughness values at high cutting speeds. Both maps help in optimizing the machining process to achieve a required surface roughness with minimal energy consumption. A review of a specific cutting energy map demonstrates that energy consumption decreases by increasing the cutting speeds. The decrease in energy consumption at moderate speeds corresponds to the low cutting forces. This potentially happens as a result of thermal softening of the material caused by adiabatic heating. This subsequently leads to an increase in the machinability of the aluminum alloy 2014 at moderate cutting speeds. Furthermore, the decreasing chip thickness and increasing shear angle as a result of increasing the cutting speed confirms the increased machinability of the workpiece at moderate speeds.
Citation: Umer Shaukat, Scott Gohery, Tesfaye Molla. Energy consumption and surface roughness maps for low and moderate speed machining of Aluminum alloy 2014: An experimental study[J]. AIMS Materials Science, 2023, 10(4): 575-588. doi: 10.3934/matersci.2023032
[1] | Juan L. G. Guirao, Pshtiwan Othman Mohammed, Hari Mohan Srivastava, Dumitru Baleanu, Marwan S. Abualrub . Relationships between the discrete Riemann-Liouville and Liouville-Caputo fractional differences and their associated convexity results. AIMS Mathematics, 2022, 7(10): 18127-18141. doi: 10.3934/math.2022997 |
[2] | Shuqin Zhang, Jie Wang, Lei Hu . On definition of solution of initial value problem for fractional differential equation of variable order. AIMS Mathematics, 2021, 6(7): 6845-6867. doi: 10.3934/math.2021401 |
[3] | Khalid K. Ali, K. R. Raslan, Amira Abd-Elall Ibrahim, Mohamed S. Mohamed . On study the fractional Caputo-Fabrizio integro differential equation including the fractional q-integral of the Riemann-Liouville type. AIMS Mathematics, 2023, 8(8): 18206-18222. doi: 10.3934/math.2023925 |
[4] | Abdul Samad, Imran Siddique, Zareen A. Khan . Meshfree numerical approach for some time-space dependent order partial differential equations in porous media. AIMS Mathematics, 2023, 8(6): 13162-13180. doi: 10.3934/math.2023665 |
[5] | Ravi P. Agarwal, Snezhana Hristova . Stability of delay Hopfield neural networks with generalized proportional Riemann-Liouville fractional derivative. AIMS Mathematics, 2023, 8(11): 26801-26820. doi: 10.3934/math.20231372 |
[6] | Adisorn Kittisopaporn, Pattrawut Chansangiam . Approximate solutions of the 2D space-time fractional diffusion equation via a gradient-descent iterative algorithm with Grünwald-Letnikov approximation. AIMS Mathematics, 2022, 7(5): 8471-8490. doi: 10.3934/math.2022472 |
[7] | Deepak B. Pachpatte . On some ψ Caputo fractional Čebyšev like inequalities for functions of two and three variables. AIMS Mathematics, 2020, 5(3): 2244-2260. doi: 10.3934/math.2020148 |
[8] | Ravi Agarwal, Snezhana Hristova, Donal O'Regan . Integral presentations of the solution of a boundary value problem for impulsive fractional integro-differential equations with Riemann-Liouville derivatives. AIMS Mathematics, 2022, 7(2): 2973-2988. doi: 10.3934/math.2022164 |
[9] | Snezhana Hristova, Antonia Dobreva . Existence, continuous dependence and finite time stability for Riemann-Liouville fractional differential equations with a constant delay. AIMS Mathematics, 2020, 5(4): 3809-3824. doi: 10.3934/math.2020247 |
[10] | Erdal Bas, Ramazan Ozarslan . Theory of discrete fractional Sturm–Liouville equations and visual results. AIMS Mathematics, 2019, 4(3): 593-612. doi: 10.3934/math.2019.3.593 |
The rising energy prices and soaring environmental concerns have put an immense pressure on the wide usage of machining processes. The total power consumption during machining includes the power consumed by the machine itself and the power used to remove the material from the workpiece. An accurate prediction of energy consumption during the machining process is the basis for energy reduction. In this study, the specific cutting energy and surface finish for low and moderate-speed orthogonal machining of the aluminum alloy 2014 are evaluated. The measured values for the specific cutting energy and surface roughness are presented as maps on a grid, which is based on the machining parameters including the following: (1) cutting speed and (2) undeformed chip thickness. The specific cutting energy map depicts low energy consumption values of 0.52 J/mm3 for the aluminum alloy 2014 at medium speed machining. The roughness maps depict high roughness values at high cutting speeds. Both maps help in optimizing the machining process to achieve a required surface roughness with minimal energy consumption. A review of a specific cutting energy map demonstrates that energy consumption decreases by increasing the cutting speeds. The decrease in energy consumption at moderate speeds corresponds to the low cutting forces. This potentially happens as a result of thermal softening of the material caused by adiabatic heating. This subsequently leads to an increase in the machinability of the aluminum alloy 2014 at moderate cutting speeds. Furthermore, the decreasing chip thickness and increasing shear angle as a result of increasing the cutting speed confirms the increased machinability of the workpiece at moderate speeds.
In much of the literature, time fractional models are defined using the Caputo definition [32,33,34,35,36], in which time fractional models are models described by fractional differential equations or pseudo state space descriptions. The Caputo definition is widely acclaimed because it makes it possible to define initial conditions that relate to the integer derivatives of the derived functions in the models considered. However, this paper shows that this definition does not take initial conditions properly into account if used to define a time fractional model.
The problem was analysed for the first time by Lorenzo and Hartley [1,2]. To take the past of the model into account in a convenient way in a finite interval, they introduced an initialization function. The idea of replacing the commonly used initial values by an initial function was further developed in [3]. In [4], the need to consider the "prehistories" before the initial instant of the derivate functions was shown, making it possible to address the initialisation of fractional visco-elastic equations to reach a unique solution. In [5,6], a counter example was used to demonstrate that initial conditions cannot be correctly taken into account in a dynamical model whether by Caputo or Riemann-Liouville definitions. This led to the conclusion in [7] that fractional derivative and time fractional model initializations are two distinct problems. Still using an initial time shifting method, counter examples were proposed in [8] to show similar initialisation problems with the Caputo definition for partial differential equations. A time shifting technique was also recently used in [9] to analyse a groundwater flow model with time Caputo or Riemann-Liouville fractional partial derivatives. The non-objectivity of these models was demonstrated in this paper. The authors in [9] did not address the problem of initialization, but this objectivity can be restored by also introducing an initialization function (instead of initial conditions).
As previously mentioned, several studies and several solutions have already been published on initialisation of fractional models, but many papers in which the initial conditions are taken into account incorrectly are also still published. Thus the novelties and the contributions of the paper are new demonstrations and new simulations that highlight how initialisations must be done with a time fractional model. Thus, in this paper, two examples are used to show that the Caputo definition does not enable initial conditions to be correctly handled when this definition is used to define a time fractional model. In the first example, the response of a simple model, assumed to be at rest, is calculated analytically on a given time interval. Then inside this interval, a second response is computed by considering initial conditions resulting from the first simulation, and ignoring the model past before the considered initial time. This is the initialisation currently found in the literature and this example shows that it is unable to ensure the correct model trajectories. In the second example, two different histories are generated that produce the same initial conditions for the model. This example shows that in spite of equal initial conditions, the model response is different, thus showing that all the model past must be taken into account to define its future. A similar analysis is also carried out with the Riemann-Liouville and the Grünwald-Letnikov's definitions, suggesting that other definitions should also be problematic. Note that all the analyzes carried out and conclusions obtained in this paper relate to models involving only time fractional derivatives and not space fractional derivatives as in [29,30,31].
The fractional integral of order ν, 0<ν<1, of a function y(t) is defined by [10]:
Iνt0y(t)=1Γ(ν)∫tt0y(τ)(t−τ)1−νdτ. | (1) |
Γ(.) being Euler's gamma function. From this definition, the Caputo derivative definition of order ν, 0<ν<1, of a function y(t) is defined by [11]:
CDνt0y(t)=I1−νt0(ddty(t))=1Γ(1−ν)∫tt01(t−τ)νdy(τ)dτdτ. | (2) |
Laplace transform applied to relation (2) reveals how initial conditions are associated to this definition:
L{CDνt0y(t)}=1s1−ν(sY(s)−y(t0)) = sνY(s)−y(t0). | (3) |
To demonstrate that Caputo definition is not able to take initial conditions correctly into account when used to define a time fractional model (a fractional differential equation or a pseudo state space description), the following model is considered
Dνy(t)=−ay(t)+u(t) 0<ν<1 a>0. | (4) |
In relation (4), Dν denotes the Caputo definition in this section but denotes the Riemann-Liouville or Grünwald-Letnikov definitions in the next section. Then, the following algorithm is used to study model (4).
Algorithm 1
1-Simulation on the time interval [0,t1] of the time fractional model (for instance model (4)) with null initial conditions (for t∈]−∞,0]). Let S1 denote this simulation.
2-Record the model output y(t) and the integer derivatives of y(t) (denoted y'(t), y''(t), ….) at time t0 such that 0<t0<t1.
3-Simulate the model again on [t0,t1], using y(t0), y'(t0), y''(t0) … as initial conditions. Let S2 denote this simulation.
4-Compare S1 and S2 on [t0,t1] and notice if they are different.
Algorithm 1 is now applied to model (4) with a=0. The model is assumed to be at rest before t=0, and the input u(t) is assumed to be a Heaviside function H(t). In such conditions, relation (4) is equivalent to [11]
y(t)=y(t0)+Iνt0{H(t)} 0<ν<1. | (5) |
As a consequence, the simulation defined in Algorithm 1 provides the following solutions:
S1:y(t)=tνΓ(ν+1) 0<t<t1 | (6) |
S2:y(t)=(t−t0)νΓ(ν+1)+y(t0) t0<t<t1. | (7) |
Figure 1 proposes a comparison of S1 and S2 and reveals a difference, thus demonstrating that the Caputo definition does not correctly take initial conditions into account.
Another way to illustrate this result is to consider two different input signals {u}_{1}\left(t\right) and {u}_{2}\left(t\right) that create two different histories with:
{u}_{i}\left(t\right) = {A}_{i}H\left(t+{t}_{i}\right)-{A}_{i}H\left(t\right)~~ \text{with} ~~ {t}_{i} > 0 ,~~ i = \left\{\rm{1, 2}\right\} . | (8) |
The model is assumed to be at rest on t\in \left]-\infty, {t}_{i}\right] . A constraint is also imposed on these signals so that at t = 0 , the two resulting model outputs coincide:
{y}_{1}\left(0\right) = {y}_{2}\left(0\right)\text{.} | (9) |
The output {y}_{i}\left(t\right) is thus defined by:
{y}_{i}\left(t\right) = \frac{{A}_{i}}{a}\left(1-{E}_{\nu , 1}^{1}\left(-a{\left(t+{t}_{i}\right)}^{\nu }\right)\right)H\left(t+{t}_{i}\right)-\frac{{A}_{i}}{a}\left(1-{E}_{\nu , 1}^{1}\left(-a{t}^{\nu }\right)\right)H\left(t\right)\text{.} | (10) |
where {E}_{\alpha, \beta }^{\gamma }\left(z\right) is the Mittag-Leffler function defined by [12]:
{E}_{\alpha , \beta }^{\gamma }\left(z\right) = \sum _{k = 0}^{\infty }\frac{\rm{\Gamma }\left(\gamma +k\right)}{\rm{\Gamma }\left(\alpha k+\beta \right)\rm{\Gamma }\left(\gamma \right)}\frac{{z}^{k}}{k!}\text{.} | (11) |
Condition (9) thus leads to
\frac{{A}_{1}}{a}\left[\left(1-{E}_{\nu , 1}^{1}\left(-a{\left({t}_{1}\right)}^{\nu }\right)\right)-\left(1-{E}_{\nu , 1}^{1}\left(0\right)\right)\right] = \frac{{A}_{2}}{a}\left[\left(1-{E}_{\nu , 1}^{1}\left(-a{\left({t}_{2}\right)}^{\nu }\right)\right)-\left(1-{E}_{\nu , 1}^{1}\left(0\right)\right)\right] | (12) |
thus leading to the condition:
{A}_{1} = {A}_{2}\frac{1-{E}_{\nu , 1}^{1}\left(-a{\left({t}_{2}\right)}^{\nu }\right)}{1-{E}_{\nu , 1}^{1}\left(-a{\left({t}_{1}\right)}^{\nu }\right)}\text{.} | (13) |
With \nu = 0.4 , a = 1 , {t}_{1} = -8s , {t}_{2} = -2s , {A}_{2} = 5 and thus {A}_{1}\approx 4.17 , Figure 2 shows the signal inputs {u}_{1}\left(t\right) and {u}_{2}\left(t\right) used for the analysis and proposes a comparison of the resulting outputs. This figure shows that the two responses have the same values at t = 0 , but that the evolutions for t>0 are not the same. The information at t = 0 is thus not enough to predict the future of the model. All the past must be taken into account to predict the future of the model, which confirms that initialization as defined by the Caputo definition is not acceptable if used to define a time fractional model such as (4).
The previous section showed that the Caputo definition should no longer be used to define time fractional models such as (4). What about other definitions?
The Riemann-Liouville derivative of order \nu , 0 < \nu < 1 , of a function y\left(t\right) is defined by [11]:
{}_{RL}{\boldsymbol{D}}_{{t}_{0}}^{\nu }y\left(t\right) = \frac{1}{\rm{\Gamma }\left(1-\nu \right)}\frac{d}{dt}{\int }_{{t}_{0}}^{t}\frac{y\left(\tau \right)}{{\left(t-\tau \right)}^{\nu }}d\tau \text{.} | (14) |
Laplace transform applied to relation (14) reveals how initial conditions are associated to this definition:
\mathcal{L}\left\{{}_{RL}{\boldsymbol{D}}_{{t}_{0}}^{\nu }y\left(t\right)\right\} = \mathcal{L}\left\{\frac{d}{dt}\left(\frac{1}{\rm{\Gamma }\left(1-\nu \right)}{\int }_{{t}_{0}}^{t}\frac{y\left(\tau \right)}{{\left(t-\tau \right)}^{\nu }}d\tau \right)\right\} = s\frac{1}{{s}^{1-\nu }}Y\left(s\right)-{\left[{\boldsymbol{I}}_{{t}_{0}}^{1-\nu }y\left(t\right)\right]}_{t = {t}_{0}}\text{.} | (15) |
As a consequence, in [11,13], the initialisation of relation (4) is defined by
\frac{{d}^{\nu }}{{dt}^{\nu }}y\left(t\right) = -ay\left(t\right)+u\left(t\right) ~~{\left.{I}_{{t}_{0}}^{1-\nu }\left\{y\left(t\right)\right\}\right|}_{t = {t}_{0}} = {y}_{0} | (16) |
and thus the initialisation problem of relation (4) is equivalent to the integral equation
y\left(t\right) = \frac{{y}_{0}}{\rm{\Gamma }\left(\nu \right)}{\left(t-{t}_{0}\right)}^{\nu -1}+{I}_{{t}_{0}}^{\nu }\left\{-ay\left(t\right)+u\left(t\right)\right\}\text{.} | (17) |
Algorithm 1 is applied again to model (4) with a = 0 . The model is assumed to be at rest before t = 0 , and the input u\left(t\right) is assumed to be a Heaviside function H\left(t\right) . Algorithm 1 provides the following solutions:
{S}_{1}:y\left(t\right) = \frac{{t}^{\nu }}{\rm{\Gamma }\left(\nu +1\right)} ~~ 0 < t < {t}_{1} | (18) |
{S}_{2}:y\left(t\right) = \frac{{\left(t-{t}_{0}\right)}^{\nu }}{\rm{\Gamma }\left(\nu +1\right)} + \frac{{y}_{0}}{\rm{\Gamma }\left(\nu \right)}{\left(t-{t}_{0}\right)}^{\nu -1} ~~ {t}_{0} < t < {t}_{1} . | (19) |
Relation (19) seems to say that any value of {y}_{0} can be chosen, but whatever the value selected, for {S}_{2} y\left(t\right) tends toward infinity as t tends toward {t}_{0} if {y}_{0}\ne 0 whereas y\left(t\right) = {{t}_{0}}^{\nu }/\rm{\Gamma }\left(\nu +1\right) for {S}_{1} . The two simulations thus give different results. This is illustrated by Figure 3 for various values of {y}_{0} .
The Grünwald-Letnikov derivative of order \nu , 0 < \nu < 1 , of a function y\left(t\right) is defined by:
{}_{GL}{\boldsymbol{D}}_{{t}_{0}}^{\nu }y\left(t\right) = \underline{h\to 0}{\rm{lim}}\frac{1}{{h}^{\nu }}\sum _{0\le m < \infty }{\left(-1\right)}^{m}\left(\begin{array}{c}\nu \\ m\end{array}\right)f\left(t-mh\right) ~~ t > {t}_{0} | (20) |
with \left(\begin{array}{c}\nu \\ m\end{array}\right) = \frac{\rm{\Gamma }\left(\nu +1\right)}{m!\rm{\Gamma }\left(\nu -m+1\right)} = \frac{\nu \left(\nu -1\right)\left(\nu -2\right)\dots \left(\nu -m+1\right)}{m\left(m-1\right)\left(m-2\right)\dots \left(m-m+1\right)} .
This definition is often used in the literature as it provides a simple numerical scheme for fractional derivative implementation. In some research [14,15,16], these numerical schemes are used to solve the initialisation problem:
\begin{aligned} \boldsymbol{D}_{t_{0}}^{v} y(t) = -a y(t)+u(t) \quad 0 < v < 1 \quad a > 0 \quad \text { for } \quad t_{0} < v < T, \end{aligned} | (21) |
y\left({t}_{0}\right) = {y}_{0}\text{.} |
In this case, it is not the Grünwald-Letnikov derivative definition which is questionable, but the idea that a time fractional model can be initialized solely with information on the initial moment. From relation (20), it is possible to observe that variable m goes from 0 to infinity, and thus this definition is able to take into account the past of the derivative function, prior to {t}_{0} . In (21), the problem is the way the initial conditions are defined.
To illustrate this problem, Algorithm 1 is applied to model (4) with a = 1 . The model is assumed to be at rest before t = 0 , and the input u\left(t\right) is assumed to be a Heaviside function H\left(t\right) . In such conditions, the simulation {S}_{1} defined in Algorithm 1 provides the following solution:
{S}_{1}:y\left(t\right) = \frac{{t}^{\nu }}{\rm{\Gamma }\left(\nu +1\right)} ~~ 0 < t < {t}_{1} . | (22) |
Simulation {S}_{2} is done using the Grünwald-Letnikov formula (20) and provides
{S}_{2}:y\left(t\right) = \frac{\frac{1}{{h}^{\nu }}\sum _{1\le m < \infty }{\left(-1\right)}^{m}\left(\begin{array}{c}\nu \\ m\end{array}\right)y\left(t-mh\right)+H\left(t\right)}{\frac{1}{{h}^{\nu }}+1} ~~ t > {t}_{0} . | (23) |
This simulation is done under two conditions:
- {S}_{21}: by taking into account all the past of the model (all the values of y\left(t\right) on t\in \left[0, {t}_{0}\right] , provided by {S}_{1} )
- {S}_{22}: by considering only an initial condition at {t}_{0} (value of y\left(t\right) at {t}_{0} provided by {S}_{1} ).
The comparison of the three simulations is done in Figure 4 and reveals that the Grünwald-Letnikov definition produces an exact solution provided that all the past of the model is taken into account.
Relation (23) is particularly interesting because it shows that a time fractional model (here a fractional integrator) is represented by an infinite difference equation, and therefore an initialization of all its terms is necessary for a prediction of the output y\left(t\right).
This remark could also apply to the Caputo and Riemann-Liouville definitions which would lead to their reformulations with integrals on the interval \left]-\rm{\infty }, \rm{ }t\right] as suggested in [27],
The need to take into account the all past of a time fractional model and not just the knowledge of its pseudo state at a single point in the past can be demonstrated quite simply on relation (4) (a particular case of fractional differential equation or of pseudo state space description). Contrary to what relation (4) might suggest, Figure 5 highlights that the implementation of fractional differential equations does not explicitly involve the fractional differentiation operator but the fractional order integration operator {\boldsymbol{I}}^{\boldsymbol{\nu }} . Thus in practice, it is not necessary to specify which particular definition is used for {\boldsymbol{D}}^{\boldsymbol{\nu }} in equation (4). Moreover, even if the system is assumed to have zero initial conditions at t = 0 , namely if the system is supposed at rest ( u\left(t\right) = y\left(t\right) = 0 , \forall t < 0 ), it is important to note that y\left(t\right) cannot be considered as a state for the time fractional model and that all the past of y\left(t\right) is required to compute the model evolution.
To better illustrate such a concept, a simple time fractional model is used: a fractional integrator supposed at rest at t = 0 . The corresponding block diagram is shown in Figure 6.
For an integer integrator, \nu = 1 , relation (4) is really a state space description. At {t}_{1}>0 , state y\left(t\right) can be computed if the input between 0 and {t}_{1} is known:
y\left({t}_{1}\right) = {\int }_{0}^{{t}_{1}}\underline{x}\left(\tau \right)d\tau = {y}_{1} = cst\text{.} | (24) |
Values of y\left(t\right) at later times than {t}_{1} are given by:
y(t)=\int_{0}^{t} \underline{x}(\tau) d \tau=\underbrace{\int_{0}^{t_{1}} \underline{x}(\tau) d \tau}_{y_{1}=c s t}+\int_{t_{1}}^{t} \underline{x}(\tau) d \tau, \quad t > t_{1}. | (25) |
Thus, y\left(t\right) can be computed if \underline{x}\left(t\right) is known within {t}_{1} and t . Integrator output at time {t}_{1} thus summarizes the whole model past. y\left(t\right) is really the state of the dynamic model, in agreement with the definition given in [26].
Let us apply the same reasoning to the fractional integrator case of order \nu . From the definition of fractional integration, value of y\left(t\right) at {t}_{1}>0 can be computed if the input between t = 0 and {t}_{1} is known:
y\left({t}_{1}\right) = \frac{1}{\rm{\Gamma }\left(\nu \right)}{\int }_{0}^{{t}_{1}}{\left({t}_{1}-\tau \right)}^{\nu -1}\underline{x}\left(\tau \right)d\tau = {y}_{1} = cst\text{.} | (26) |
Variable y\left(t\right) , \forall t>{t}_{1} , is thus given by:
y\left(t_{1}\right)=\frac{1}{\Gamma(v)} \int_{0}^{t}(t-\tau)^{v-1} \underline{x}(\tau) d \tau=\underbrace{\frac{1}{\Gamma(v)} \int_{0}^{t_{1}}(t-\tau)^{v-1} \underline{x}(\tau) d \tau}_{\alpha(t) \neq y_{1}}+\frac{1}{\Gamma(v)} \int_{t_{1}}^{t}(t-\tau)^{v-1} \underline{x}(\tau) d \tau. | (27) |
Two notable differences can be highlighted with respect to the integer case. First, term \alpha \left(t\right) in equation (27) is not a constant but depends on the considered time t . Moreover, even if {y}_{1} = y\left({t}_{1}\right) is known, it is not enough to compute \alpha \left(t\right) . Output y\left(t\right) of the fractional integrator is thus not a state. The same analysis can be held for the general case of a pseudo state description or a fractional differential equation.
Beyond discussions on the concept of state, computation of \alpha \left(t\right) in relation (27) whatever time t , requires to know y\left(t\right) \forall t\in \left[0..{t}_{1}\right] , thus all the model past. This clearly shows that knowledge of y\left(t\right) at a unique point of the past is not enough.
Fractional operators and the resulting time fractional models are known for their memory property. However, for the following two reasons, many studies proposed in the literature seem to ignore this property when the model initialization problem is considered:
-they use the Caputo definition that involves only integer derivatives of the derivate function at the initial time,
-they use other definitions but initialization is done by taking only an initial value for the initial time into consideration.
This kind of initialization means that the operator or model memory exists everywhere on the time axis, except at the initial time. This is not consistent. Memory is an intrinsic property that exists all the time and that is proved in this paper with very simple examples. If from a mathematical point of view, most of the fractional derivative definitions encountered in the literature [17] are not problematic, this paper shows that the Caputo and Riemann-Liouville definitions are not able to ensure a proper initialization when used in a model definition. The paper also shows that this problem is not encountered with the Grünwald-Letnikov definition, provided that all the past of the model (from t\to -\infty ) is taken into account. And this is precisely one of the drawbacks of time fractional models that induces a physical inconsistency and many analysis problems [17].
What are the possible solutions? One solution can be to add an initialization function to the definition of the model. This is what was proposed by Lorenzo and Hartley [1,2]. Yet again however, it requires the knowledge of all the model past (from t\to -\infty ). Another solution consists in introducing new kernels for the definition of fractional integration as in [19]. But the goal would not be to solve only a singularity problem as in [19], but to reach a finite memory length as was done for instance in [20]. Note that while it was claimed in [21] that this class of kernels was too restrictive, it is linked to the problem analyzed in this paper: the inability of the Caputo definition to take into account initial conditions properly if used to define a time fractional model [22]. The other solution is to introduce new solutions for fractional behavior modeling, without the drawbacks associated to time fractional models [18]:
-distributed time delay models [23];
-non-linear models [24];
-partial differential equation with spatially varying coefficients [25].
All the conclusions presented in this paper can they be extended to models involving space fractional derivatives as in [29,30,31]? As shown in [28], whatever the variable on which the derivative relates, a fractional model remains a doubly infinite dimensional model and as such requires an infinite amount of information for its initialization. The question remains open, however, the authors will seek to answer it in their future work.
[1] | Morinaga M (2018) A Quantum Approach to Alloy Design An Exploration of Material Design and Development Based Upon Alloy Design Theory and Atomization Energy Method, Elsevier. |
[2] | Campbell FC (2006) Manufacturing Technology for Aerospace Structural Materials, Elsevier. https://doi.org/10.1016/B978-185617495-4/50011-1 |
[3] |
Tan Z, Pang B, Oliveira JP, et al. (2022) Effect of S-curve laser power for power distribution control on laser oscillating welding of 5A06 aluminum alloy. Opt Laser Technol 149: 107909. https://doi.org/10.1016/j.optlastec.2022.107909 doi: 10.1016/j.optlastec.2022.107909
![]() |
[4] |
Zheng M, Yang J, Xu J, et al. (2023) Interfacial microstructure and strengthening mechanism of dissimilar laser al/steel joint via a porous high entropy alloy coating. J Mater Res Technol 23: 3997–4011. https://doi.org/10.1016/j.jmrt.2023.02.040 doi: 10.1016/j.jmrt.2023.02.040
![]() |
[5] | Torenbeek E (2013) Advanced Aircraft Design: Conceptual Design, Analysis and Optimization of Subsonic Civil Airplanes, John Wiley and Sons, Ltd. https://doi.org/10.1002/9781118568101 |
[6] |
Yoon HS, Kim ES, Kim MS, et al. (2015) Towards greener machine tools-A review on energy saving strategies and technologies. Renew Sustain Energy Rev 48: 870–891. https://doi.org/10.1016/j.rser.2015.03.100 doi: 10.1016/j.rser.2015.03.100
![]() |
[7] | Mead I (2017) International Energy Outlook 2017. Available from: https://www.eia.gov/pressroom/presentations/mead_91417.pdf |
[8] |
Zhao G, Hou C, Qiao J, et al. (2016) Energy consumption characteristics evaluation method in turning. Adv Mech Eng 8: 168781401668073. https://doi.org/10.1177/1687814016680737 doi: 10.1177/1687814016680737
![]() |
[9] |
Li W, Kara S (2011) An empirical model for predicting energy consumption of manufacturing processes: a case of turning process. Proc Inst Mech Eng Part B 225: 643–652. https://doi.org/10.1177/2041297511398541 doi: 10.1177/2041297511398541
![]() |
[10] |
Dahmus JB, Gutowski TG (2004) An environmental analysis of machining. IMECE04 2004 ASME International Mechanical Engineering Congress and Exposition, Anaheim, California, USA, 2004: 643–652. https://doi.org/10.1115/IMECE2004-62600 doi: 10.1115/IMECE2004-62600
![]() |
[11] | Gutowski T, Dahmus J, Thiriez A (2006) Electrical energy requirements for manufacturing processes. 13th CIRP International Conference on Life Cycle Engineering, Leuven, Belgium, 31: 623–638. |
[12] |
Goncharenko AV (2018) Aeronautical and aerospace material and structural damages to failures: Theoretical concepts. Int J Aerosp Eng 2018: 1–7. https://doi.org/10.1155/2018/4126085 doi: 10.1155/2018/4126085
![]() |
[13] | Groover MP (2010) Fundamentals of Modern Manufacturing: Materials, Processes, and Systems, Wiley. |
[14] |
Li L, Yan J, Xing Z (2013) Energy requirements evaluation of milling machines based on thermal equilibrium and empirical modelling. J Clean Prod 52: 113–121. https://doi.org/10.1016/j.jclepro.2013.02.039 doi: 10.1016/j.jclepro.2013.02.039
![]() |
[15] |
Benedicto E, Carou D, Rubio EM (2017) Technical, economic and environmental review of the lubrication/cooling systems used in machining processes. Procedia Eng 184: 99–116. https://doi.org/10.1016/j.proeng.2017.04.075 doi: 10.1016/j.proeng.2017.04.075
![]() |
[16] | Kopac J, Pusavec F, Krolczyk G (2015) Cryogenic machining, surface integrity and machining performance. Arch Mater Sci Eng 71: 83–93. |
[17] |
Krolczyk GM, Maruda RW, Krolczyk JB, et al. (2019) Ecological trends in machining as a key factor in sustainable production—A review. J Clean Prod 218: 601–615. https://doi.org/10.1016/j.jclepro.2019.02.017 doi: 10.1016/j.jclepro.2019.02.017
![]() |
[18] |
Saleem W, Zain-ul-abdein M, Ijaz H, et al. (2017) Computational analysis and artificial neural network optimization of dry turning parameters-AA2024-T351. Appl Sci 7: 642. https://doi.org/10.3390/app7060642 doi: 10.3390/app7060642
![]() |
[19] |
Do TV, Hsu QC (2016) Optimization of minimum quantity lubricant conditions and cutting parameters in hard milling of AISI H13 steel. Appl Sci 6: 83. https://doi.org/10.3390/app6030083 doi: 10.3390/app6030083
![]() |
[20] |
Dhokia V, Newman ST, Imani-Asrai R (2012) An initial study of the effect of using liquid nitrogen coolant on the surface roughness of inconel 718 nickel-based alloy in CNC milling. Procedia CIRP 3: 121–125. https://doi.org/10.1016/j.procir.2012.07.022 doi: 10.1016/j.procir.2012.07.022
![]() |
[21] |
Syed AK, Zhang X, Moffatt JE, et al. (2017) Effect of temperature and thermal cycling on fatigue crack growth in aluminium reinforced with GLARE bonded crack retarders. Int J Fatigue 98: 53–61. https://doi.org/10.1016/j.ijfatigue.2017.01.018 doi: 10.1016/j.ijfatigue.2017.01.018
![]() |
[22] |
Caruso S, Rotella G, Del Prete A, et al. (2019) Finite element modeling of microstructural changes in hard machining of SAE 8620. Appl Sci 10: 121. https://doi.org/10.3390/app10010121 doi: 10.3390/app10010121
![]() |
[23] |
Meng X, Lin Z, Wang F (2013) Investigation on corrosion fatigue crack growth rate in 7075 aluminum alloy. Mater Des 51: 683–687. https://doi.org/10.1016/j.matdes.2013.04.097 doi: 10.1016/j.matdes.2013.04.097
![]() |
[24] |
Warsi SS, Jaffery SHI, Ahmad R, et al. (2018) Development of energy consumption map for orthogonal machining of Al 6061-T6 alloy. P I Mech Eng B-J Eng 232: 2510–2522. https://doi.org/10.1177/0954405417703424 doi: 10.1177/0954405417703424
![]() |
[25] |
Draganescu F, Gheorghe M, Doicin CV (2003) Models of machine tool efficiency and specific consumed energy. J Mater Process Technol 141: 9–15. https://doi.org/10.1016/S0924-0136(02)00930-5 doi: 10.1016/S0924-0136(02)00930-5
![]() |
[26] |
Pawade RS, Sonawane HA, Joshi SS (2009) An analytical model to predict specific shear energy in high-speed turning of Inconel 718. Int J Mach Tools Manuf 49: 979–990. https://doi.org/10.1016/j.ijmachtools.2009.06.007 doi: 10.1016/j.ijmachtools.2009.06.007
![]() |
[27] |
Balogun VA, Mativenga PT (2014) Impact of un-deformed chip thickness on specific energy in mechanical machining processes. J Clean Prod 69: 260–268. https://doi.org/10.1016/j.jclepro.2014.01.036 doi: 10.1016/j.jclepro.2014.01.036
![]() |
[28] |
Warsi SS, Agha MH, Ahmad R, et al. (2019) Sustainable turning using multi-objective optimization: a study of Al 6061 T6 at high cutting speeds. Int J Adv Manuf Technol 100: 843–855. https://doi.org/10.1007/s00170-018-2759-2 doi: 10.1007/s00170-018-2759-2
![]() |
[29] |
Ghosh CS, Rao PV (2018) Specific cutting energy modeling for turning nickel-based Nimonic 90 alloy under MQL condition. Int J Mech Sci 146–147: 25–38. https://doi.org/10.1016/j.ijmecsci.2018.07.033 doi: 10.1016/j.ijmecsci.2018.07.033
![]() |
[30] |
Yao Y, Zhu H, Huang C, et al. (2019) On the relations between the specific cutting energy and surface generation in micro-milling of maraging steel. Int J Adv Manuf Technol 104: 585–598. https://doi.org/10.1007/s00170-019-03911-y doi: 10.1007/s00170-019-03911-y
![]() |
[31] |
Liu ZJ, Sun DP, Lin CX, et al. (2016) Multi-objective optimization of the operating conditions in a cutting process based on low carbon emission costs. J Clean Prod 124: 266–275. https://doi.org/10.1016/j.jclepro.2016.02.087 doi: 10.1016/j.jclepro.2016.02.087
![]() |
[32] |
Bhattacharya A, Das S, Majumder P, et al. (2009) Estimating the effect of cutting parameters on surface finish and power consumption during high speed machining of AISI 1045 steel using Taguchi design and ANOVA. Prod Eng 3: 31–40. https://doi.org/10.1007/s11740-008-0132-2 doi: 10.1007/s11740-008-0132-2
![]() |
[33] |
Bhushan RK (2013) Optimization of cutting parameters for minimizing power consumption and maximizing tool life during machining of Al alloy SiC particle composites. J Clean Prod 39: 242–254. https://doi.org/10.1016/j.jclepro.2012.08.008 doi: 10.1016/j.jclepro.2012.08.008
![]() |
[34] |
Kosaraju S, Chandraker S(2015) Taguchi analysis on cutting force and surface roughness in turning MDN350 steel. Mater Today Proc 2: 3388–3393. https://doi.org/10.1016/j.matpr.2015.07.313 doi: 10.1016/j.matpr.2015.07.313
![]() |
[35] |
Warsi SS, Jaffery SHI, Ahmad R, et al. (2018) Development and analysis of energy consumption map for high-speed machining of Al 6061-T6 alloy. Int J Adv Manuf Technol 96: 91–102. https://doi.org/10.1007/s00170-018-1588-7 doi: 10.1007/s00170-018-1588-7
![]() |
[36] | Find your way to gain an edge in steel turning, Sandvik Coromant. Available from: https://www.sandvik.coromant.com/en-us/campaigns/gain-an-edge-in-steel-turning. |
[37] |
Iqbal SA, Mativenga PT, Sheikh MA (2009) A comparative study of the tool-chip contact length in turning of two engineering alloys for a wide range of cutting speeds. Int J Adv Manuf Technol 42: 30–40. https://doi.org/10.1007/s00170-008-1582-6 doi: 10.1007/s00170-008-1582-6
![]() |
[38] | Shaw MC (2005) Metal Cutting Principles, 2nd Eds., Oxford University Press. https://www.scribd.com/document/344789895/Shaw-Milton-C-Metal-Cutting-Principles# |
[39] |
Trent EM (1988) Metal cutting and the tribology of seizure: Ⅰ seizure in metal cutting. Wear 128: 29–45. https://doi.org/10.1016/0043-1648(88)90251-7 doi: 10.1016/0043-1648(88)90251-7
![]() |
[40] | Childs T, Maekawa K, Obikawa T, et al. (2000) Metal Machining, Elsevier. |
[41] |
Abukhshim NA, Mativenga PT, Sheikh MA (2006) Heat generation and temperature prediction in metal cutting: A review and implications for high speed machining. Int J Mach Tools Manuf 46: 782–800. https://doi.org/10.1016/j.ijmachtools.2005.07.024 doi: 10.1016/j.ijmachtools.2005.07.024
![]() |
[42] | Schmid SR, Kalpakjian S (2015) Manufacturing Processes for Engineering Materials, 5th Eds., Pearson Education India. |
[43] |
Giridhar D, Vijayaraghavan L, Krishnamurthy R (2010) Micro-grooving studies on alumina ceramic material. Mater Manuf Process 25: 1148–1159. https://doi.org/10.1080/10426914.2010.502952 doi: 10.1080/10426914.2010.502952
![]() |
[44] |
Atkins AG (2003) Modelling metal cutting using modern ductile fracture mechanics: quantitative explanations for some longstanding problems. Int J Mech Sci 45: 373–396. https://doi.org/10.1016/S0020-7403(03)00040-7 doi: 10.1016/S0020-7403(03)00040-7
![]() |
[45] |
Akram S, Jaffery SHI, Khan M, et al. (2018) Numerical and experimental investigation of Johnson-Cook material models for aluminum (Al 6061-T6) alloy using orthogonal machining approach. Adv Mech Eng 10: 168781401879779. https://doi.org/10.1177/1687814018797794 doi: 10.1177/1687814018797794
![]() |
1. | Vincent Tartaglione, Christophe Farges, Jocelyn Sabatier, Fractional Behaviours Modelling with Volterra Equations: Application to a Lithium-Ion Cell and Comparison with a Fractional Model, 2022, 6, 2504-3110, 137, 10.3390/fractalfract6030137 | |
2. | Juan A. López-Villanueva, Pablo Rodríguez-Iturriaga, Luis Parrilla, Salvador Rodríguez-Bolívar, A compact model of the ZARC for circuit simulators in the frequency and time domains, 2022, 153, 14348411, 154293, 10.1016/j.aeue.2022.154293 | |
3. | Nezha Maamri, Jean-Claude Trigeassou, A Plea for the Integration of Fractional Differential Systems: The Initial Value Problem, 2022, 6, 2504-3110, 550, 10.3390/fractalfract6100550 | |
4. | Jocelyn Sabatier, 2022, Chapter 1, 978-3-031-04382-6, 1, 10.1007/978-3-031-04383-3_1 | |
5. | Zdeněk Biolek, Dalibor Biolek, Viera Biolková, Zdeněk Kolka, Extended Higher-Order Elements with Frequency-Doubled Parameters: The Hysteresis Loops Are Always of Type II, 2023, 23, 1424-8220, 7179, 10.3390/s23167179 | |
6. | Abdelkader Moumen, Abdelaziz Mennouni, Mohamed Bouye, Contributions to the Numerical Solutions of a Caputo Fractional Differential and Integro-Differential System, 2024, 8, 2504-3110, 201, 10.3390/fractalfract8040201 | |
7. | Reny George, Fahad Al-shammari, Mehran Ghaderi, Shahram Rezapour, On the boundedness of the solution set for the \psi -Caputo fractional pantograph equation with a measure of non-compactness via simulation analysis, 2023, 8, 2473-6988, 20125, 10.3934/math.20231025 | |
8. | Shabnam Tashakori, Andres San-Millan, Vahid Vaziri, Sumeet S. Aphale, Fast Parameter Identification of the Fractional-Order Creep Model, 2024, 13, 2076-0825, 534, 10.3390/act13120534 | |
9. | Hacen Serrai, Brahim Tellab, A generalized contraction mapping applied for existence results for ordinary hybrid version of a generalized Sturm–Liouville–Langevin equations under \Psi -Hilfer fractional-order derivative, 2025, 74, 0009-725X, 10.1007/s12215-024-01175-4 |