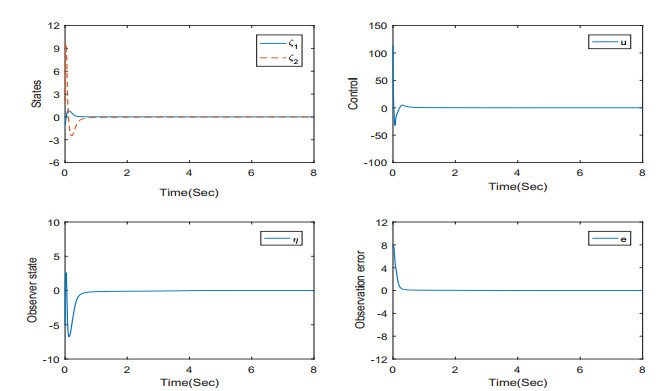
In the field of state-of-the-art object detection, the task of object localization is typically accomplished through a dedicated subnet that emphasizes bounding box regression. This subnet traditionally predicts the object's position by regressing the box's center position and scaling factors. Despite the widespread adoption of this approach, we have observed that the localization results often suffer from defects, leading to unsatisfactory detector performance. In this paper, we address the shortcomings of previous methods through theoretical analysis and experimental verification and present an innovative solution for precise object detection. Instead of solely focusing on the object's center and size, our approach enhances the accuracy of bounding box localization by refining the box edges based on the estimated distribution at the object's boundary. Experimental results demonstrate the potential and generalizability of our proposed method.
Citation: Peng Zhi, Haoran Zhou, Hang Huang, Rui Zhao, Rui Zhou, Qingguo Zhou. Boundary distribution estimation for precise object detection[J]. Electronic Research Archive, 2023, 31(8): 5025-5038. doi: 10.3934/era.2023257
[1] | Jingya Wang, Ye Zhu . L2−L∞ control for memristive NNs with non-necessarily differentiable time-varying delay. Mathematical Biosciences and Engineering, 2023, 20(7): 13182-13199. doi: 10.3934/mbe.2023588 |
[2] | Kseniia Kravchuk, Alexander Vidybida . Non-Markovian spiking statistics of a neuron with delayed feedback in presence of refractoriness. Mathematical Biosciences and Engineering, 2014, 11(1): 81-104. doi: 10.3934/mbe.2014.11.81 |
[3] | Tianqi Yu, Lei Liu, Yan-Jun Liu . Observer-based adaptive fuzzy output feedback control for functional constraint systems with dead-zone input. Mathematical Biosciences and Engineering, 2023, 20(2): 2628-2650. doi: 10.3934/mbe.2023123 |
[4] | Gonzalo Robledo . Feedback stabilization for a chemostat with delayed output. Mathematical Biosciences and Engineering, 2009, 6(3): 629-647. doi: 10.3934/mbe.2009.6.629 |
[5] | Linni Li, Jin-E Zhang . Input-to-state stability of stochastic nonlinear system with delayed impulses. Mathematical Biosciences and Engineering, 2024, 21(2): 2233-2253. doi: 10.3934/mbe.2024098 |
[6] | Xiaoxiao Dong, Huan Qiao, Quanmin Zhu, Yufeng Yao . Event-triggered tracking control for switched nonlinear systems. Mathematical Biosciences and Engineering, 2023, 20(8): 14046-14060. doi: 10.3934/mbe.2023627 |
[7] | Tong Guo, Jing Han, Cancan Zhou, Jianping Zhou . Multi-leader-follower group consensus of stochastic time-delay multi-agent systems subject to Markov switching topology. Mathematical Biosciences and Engineering, 2022, 19(8): 7504-7520. doi: 10.3934/mbe.2022353 |
[8] | Dong Xu, Xinling Li, Weipeng Tai, Jianping Zhou . Event-triggered stabilization for networked control systems under random occurring deception attacks. Mathematical Biosciences and Engineering, 2023, 20(1): 859-878. doi: 10.3934/mbe.2023039 |
[9] | Yue Song, Yi Zhang, Song Yang, Na Li . Investigation on stability and controller design for singular bio-economic systems with stochastic fluctuations. Mathematical Biosciences and Engineering, 2021, 18(3): 2991-3005. doi: 10.3934/mbe.2021150 |
[10] | Xiaofeng Chen . Double-integrator consensus for a switching network without dwell time. Mathematical Biosciences and Engineering, 2023, 20(7): 11627-11643. doi: 10.3934/mbe.2023516 |
In the field of state-of-the-art object detection, the task of object localization is typically accomplished through a dedicated subnet that emphasizes bounding box regression. This subnet traditionally predicts the object's position by regressing the box's center position and scaling factors. Despite the widespread adoption of this approach, we have observed that the localization results often suffer from defects, leading to unsatisfactory detector performance. In this paper, we address the shortcomings of previous methods through theoretical analysis and experimental verification and present an innovative solution for precise object detection. Instead of solely focusing on the object's center and size, our approach enhances the accuracy of bounding box localization by refining the box edges based on the estimated distribution at the object's boundary. Experimental results demonstrate the potential and generalizability of our proposed method.
In many industrial applications, due to the ubiquity of stochastic noise and nonlinear [1,2], real systems are often modelled by stochastic differential equations, which attracts researchers to pay more and more attention to the control of stochastic systems. Using the state-feedback, a closed-loop pole can be arbitrarily configured to improve the performance of the control systems. Therefore, some scholars research the problem of state-feedback stabilization for stochastic systems, e.g., reference [3] focuses on the cooperative control problem of multiple nonlinear systems perturbed by second-order moment processes in a directed topology. Reference [4] considers the case where the diffusion term and the drift term are unknown parameters for stochastic systems with strict feedback. Reference [5] studies stochastic higher-order systems with state constraints and [6] discusses output constrained stochastic systems with low-order and high-order nonlinear and stochastic inverse dynamics. However, it is often difficult to obtain all the state variables of the system directly, it is unsuitable for direct measurement or the measurement equipment is limited in economy and practicality, so the physical implementation of state-feedback is difficult. One of the solutions to this difficulty is to reconstruct the state of the system. At this time, scholars use an observer to investigate the output-feedback stabilization, e.g., reference [7] investigates the prescribed-time stability problem of stochastic nonlinear strict-feedback systems. Reference [8] focuses on stochastic strict feedback systems with sensor uncertainties. In addition, based on output-feedback, for nonlinear multiagent systems, a distributed output-feedback tracking controller is proposed in [9].
It should be noted that all of the above results [7,8,9], Markovian switching is not considered in the design of output-feedback controller. However, as demonstrated by [10], switching system is a complex hybrid system, which consists of a series of subsystems and switching rules that coordinate the order of each subsystem. In real life, due to the aging of internal components, high temperature, sudden disturbance of external environment, operator error and other inevitable factors, the structure of many systems changes suddenly. Such systems can be reasonably modelled as differential equation with Markovian switching, see [11,12]. Recently, references [13] and [14] discuss the adaptive tracking problem and output tracking problem with Markovian switching respectively. Besides, as shown in [15], the power of the system changes because of factors such as the aging of the springs inside the boiler-turbine unit. Therefore, the research on the stability of stochastic nonlinear systems with time-varying powers has important practical significance. Reference [16] investigates the optimality and stability of high-order stochastic systems with time-varying powers. However, these results do not address the output-feedback stabilization for higher-order stochastic systems with both Markovian switching and time-varying powers.
Based on these discussions, we aim to resolve the output-feedback stabilization for higher-order stochastic nonlinear systems with both Markovian switching and time-varying powers. The main contributions and characteristics of this paper are two-fold:
1) The system model we take into account is more applicable than the existing results [7,8,9] and [12,13,14]. Different from the previous results [7,8,9], the stochastic system with Markovian switching is studied in this paper. Unlike previous studies in [12,13,14], we investigate the power is time-varying. The simultaneous existence of the Markov process and time-varying order makes the controller design process more complicated and difficult. More advanced stochastic analysis techniques are needed.
2) We propose a new observer. The existence of Markovian switching and nondifferentiable time-varying power makes the observer constructed in [7,8,9] invalid. We use the time-varying power's bounds to construct a new observer, which can effectively observe the unmeasurable state and can deal with the nonlinear growth rate, while the existing observer can only deal with constant growth rate.
The rest of this paper is listed as follows. The problem is formulated in Section 2. In Section 3, an output-feedback controller is designed. Section 4 is the stability analysis. A simulation is given in Section 5. The conclusions are collected in Section 6.
Notations: R2 denotes the 2-dimensional space and the set of nonnegative real numbers is represented by R+. X denotes the matrix or vector, its transpose is represented by XT. |X| denotes the Euclidean norm of a vector X. When X is square, Tr{X} denotes its trace. The set of all functions with continuous ith partial derivatives is represented by Ci. Let C2,1(R2×R+×S;R+) represent all nonnegative functions V on R2×R+×S which are C2 in x and C1 in t.
This paper studies the output-feedback stabilization for stochastic nonlinear systems with both Markovian switching and time-varying powers described by:
dζ1=[ζ2]m(t)dt,dζ2=[u]m(t)dt+fTγ(t)(ˉζ2)dω,y=ζ1, | (2.1) |
where ζ=ˉζ2=(ζ1,ζ2)T∈R2, y∈R and u∈R are the system state, control output and the input, respectively. The state ζ2 is unmeasurable. The function m(t):R+→R+ is continuous and bounded, which satisfies 1≤m_≤m(t)≤ˉm with m_ and ˉm being constants. The powers sign function [⋅]α is defined as [⋅]α:=sign(⋅)|⋅|α with α∈(0,+∞). The functions fγ(t) is assumed to be smooth, and for all t≥0, the locally Lipschitz continuous in x uniformly. fγ(t)(t,0)=0. ω is an r−dimensional standard Wiener process, which is defined on the complete probability space (Ω,F,Ft,P) with the filtration Ft satisfying the general conditions. γ(t) is a homogeneous Markov process on the probability space taking values in a space S={1,2,...,N}, which the generator Γ=(λij)N×N given by
Pij(t)=P{γ(t+s)=i|γ(s)=j}={λijt+o(t)ifi≠j,1+λijt+o(t)ifi=j, | (2.2) |
where λij>0 is the transition rate from i to j if i≠j while λii=−ΣNj=1,i≠jλij for any s,t≥0. Suppose the Markov process γ(t) is irrelevant to the ω(t).
To implement the controller design, we need the following assumption.
Assumption 2.1. There exists a non-negative smooth function ˜f(ζ1) such that
|fγ(t)(ˉζ2)|≤(|ζ1|m(t)+12+|ζ2|m(t)+12)˜f(ζ1). | (2.3) |
Remark 2.1. As we know, the existing results for stochastic systems with time-varying powers (e.g., [16]), neither the state-feedback control nor the output-feedback control, has considered Markovian switching. However, the structure of many physical systems in the actual system often mutates, which makes it necessary to study systems with both Markovain switching and time-varying powers. Therefore, compared with [16], the model we consider is more practical and more general.
Remark 2.2. In Assumption 2.1, we can see that the power m(t) is time-varying and the growth rate ˜f(ζ1) is a nonlinear function. When m(t)=1 and ˜f(ζ1) is a constant, Assumption 2.1 is a linear growth condition. However, we consider that ˜f(ζ1) is a nonlinear function, which includes the constant case as a special case. The growth condition of Assumption 2.1 is broader than the linear growth condition. The time-varying power m(t) makes the design in [7,8,9] for time-invariant power invalid. In addition, the nonlinear growth rate ˜f(ζ1) makes the design in [7,8,9,17,18] for constant growth rate fail. A new design scheme should be proposed.
In this section, we develop an output-feedback controller design for system (2.1). The process is divided into two steps:
∙ Firstly, we assume that all states are measurable and develop a state-feedback controller using backstepping technique.
∙ Secondly, we construct a reduced-order observer with a dynamic gain, and design an output-feedback controller.
In this part, under Assumption 2.1, our objective is to develop a state-feedback controller design for the system (2.1).
Step 1. Introducing the coordinate transformation ξ1=ζ1 and choosing V1=14ξ41, by using the infinitesimal generator defined in section 1.8 of [11], we have
LV1≤ξ31[ζ2]m(t)+IIV1≤ξ31([ζ2]m(t)−[ζ∗2]m(t))+ξ31[ζ∗2]m(t)+IIV1. | (3.1) |
If we choose ζ∗2 as
ζ∗2=−c1/m_1ξ1:=−α1ξ1, | (3.2) |
we get
ξ31[ζ∗2]m(t)=−αm(t)1ξm(t)+31≤−c1|ξ1|m(t)+3, | (3.3) |
where α1=c1/m_1≥1 is a constant with c1≥1 being a design parameter.
Substituting (3.3) into (3.1) yields
LV1≤−c1|ξ1|m(t)+3+ξ31([ζ2]m(t)−[ζ∗2]m(t))+IIV1. | (3.4) |
Step 2. Introducing the coordinate transformation ξ2=ζ2−ζ∗2, and using Itô's differentiation rule, we get
dξ2=([u]m(t)−∂ζ∗2∂ζ1[ζ2]m(t))dt+fTγ(t)(ˉζ2)dω. | (3.5) |
Choose V2=V1+14ξ42. From (3.4) and (3.5), we obtain
LV2≤−c1|ξ1|m(t)+3+ξ31([ζ2]m(t)−[ζ∗2]m(t))+ξ32[u]m(t)−ξ32∂ζ∗2∂ζ1[ζ2]m(t)+32ξ22|fTγ(t)(ˉζ2)|2+IIV2. | (3.6) |
By (3.2) and using Lemma 1 in [19], we have
ξ31([ζ2]m(t)−[ζ∗2]m(t))≤ˉm(2ˉm−2+2)(|ξ1|3|ξ2|m(t)+αˉm−11|ξ1|m(t)+2|ξ2|). | (3.7) |
By using Lemma 2.1 in [20], we get
ˉm(2+2ˉm−2)|ξ1|3|ξ2|m(t)≤16|ξ1|3+m(t)+β211|ξ2|3+m(t),ˉm(2+2ˉm−2)αˉm−11|ξ1|m(t)+2|ξ2|≤16|ξ1|3+m(t)+β212|ξ2|3+m(t), | (3.8) |
where
β211=ˉmm_+3(ˉm(2+2ˉm−2))3+ˉmm_(3+m_18)−3ˉm,β212=1m_+3(ˉm(2+2ˉm−2)αˉm−11)ˉm+3(m_+36(ˉm+2))−(ˉm+2). | (3.9) |
Substituting (3.8) into (3.7) yields
ξ31([ζ2]m(t)−[ζ∗2]m(t))≤13|ξ1|3+m(t)+β21|ξ2|3+m(t), | (3.10) |
where β21=β211+β212 is a positive constant.
By (3.2) and using Lemma 5 in [21], we get
|ζ2|m(t)=|ξ2+ζ∗2|m(t)≤(|ξ2|+|α1ξ1|)m(t)≤2ˉm−1(|ξ2|m(t)+|α1ξ1|m(t))≤2ˉm−1αˉm1(|ξ2|m(t)+|ξ1|m(t)), | (3.11) |
which means that
|ζ1|m(t)+|ζ2|m(t)≤φ1(|ξ2|m(t)+|ξ1|m(t)), | (3.12) |
where φ1=2ˉm−1αˉm1+1≥0 is a constant.
By (3.11) and using Lemma 1 in [19], we have
ξ32∂ζ∗2∂ζ1[ζ2]m(t)≤|ξ32||∂ζ∗2∂ζ1|2ˉm−1αˉm1(|ξ2|m(t)+|ξ1|m(t))≤2ˉm−1αˉm1|∂ζ∗2∂ζ1|(|ξ2|m(t)+3+|ξ32||ξ1|m(t)). | (3.13) |
By using Lemma 2.1 in [20], we get
2ˉm−1αˉm1|∂ζ∗2∂ζ1||ξ32||ξ1|m(t)≤13|ξ1|3+m(t)+β221(ζ1)|ξ2|3+m(t), | (3.14) |
where
β221(ζ1)=3m_+3(2ˉm−1αˉm1|∂ζ∗2∂ζ1|)ˉm+33(m_+33ˉm)−ˉm3. | (3.15) |
Substituting (3.14) into (3.13) yields
ξ32∂ζ∗2∂ζ1[ζ2]m(t)≤13|ξ1|3+m(t)+β22(ζ1)|ξ2|3+m(t), | (3.16) |
where β22(ζ1)=2ˉm−1αˉm1|∂ζ∗2∂ζ1|+β221(ζ1) is a smooth function irrelevant to m(t).
By (3.12), using Assumption 2.1 and Lemma 1 in [19], we get
32ξ22|fTγ(t)(ˉζ2)|2≤3˜f2(ζ1)|ξ2|2(|ζ1|m(t)+1+|ζ2|m(t)+1)≤3˜f2(ζ1)φ2(|ξ2|m(t)+3+|ξ2|2|ξ1|m(t)+1), | (3.17) |
where φ2=2ˉmαˉm+11+1≥0 is a constant.
From Lemma 2.1 in [20], we obtain
3˜f2(ζ1)φ2|ξ2|2|ξ1|m(t)+1≤13|ξ1|m(t)+3+β231(ζ1)|ξ2|m(t)+3, | (3.18) |
where
β231(ζ1)=2m_+3(3˜f2(ζ1)φ2)ˉm+32(m_+33(ˉm+1))−ˉm+12. | (3.19) |
Substituting (3.18) into (3.17) yields
32ξ22|fTγ(t)(ˉζ2)|2≤13|ξ1|m(t)+3+β23(ζ1)|ξ2|m(t)+3, | (3.20) |
where β23(ζ1)=3˜f2(ζ1)φ2+β231(ζ1)≥0 is a smooth function irrelevant to m(t).
By using (3.6), (3.10), (3.16) and (3.20), we obtain
LV2≤−(c1−1)|ξ1|m(t)+3+ξ32([u]m(t)−[x∗3]m(t))+ξ32[x∗3]m(t)+β2(ζ1)|ξ2|m(t)+3+IIV2, | (3.21) |
where β2(ζ1)=β21(ζ1)+β22(ζ1)+β23(ζ1) is a smooth function irrelevant to m(t).
Constructing the virtual controller as
x∗3=−(c2+β2(ζ1))1m_:=−α2(ζ1)ξ2, | (3.22) |
we have
ξ32[x∗3]m(t)=−αm(t)2(ζ1)ξm(t)+32≤−(c2+β2(ζ1))ξm(t)+32, | (3.23) |
where c2>0 is a constant and α2(ζ1)≥0 is a smooth function irrelevant to m(t).
Substituting (3.23) into (3.21) yields
LV2≤−(c1−1)|ξ1|m(t)+3−c2|ξ2|m(t)+3+ξ32([u]m(t)−[x∗3]m(t))+IIV2. | (3.24) |
In this part, we first design a reduced-order observer with a dynamic gain, then we design an output-feedback controller.
Since ζ2 are unmeasurable, we construct the following observer
dη=([u]m(t)−∂L(ζ1)∂ζ1[η+L(ζ1)]m(t))dt, | (3.25) |
where L(ζ1) is a smooth function, and ∂L(ζ1)∂ζ1>0 is irrelevant to m(t).
Defining e=ζ2−L(ζ1)−η and by the construction of the observer, we have
de=∂L(ζ1)∂ζ1([η+L(ζ1)]m(t)−[ζ2]m(t))dt+fTγ(t)(ˉζ2)dω. | (3.26) |
Choose U=14e4. From (3.26), we get
LU=e3∂L(ζ1)∂ζ1([η+L(ζ1)]m(t)−[ζ2]m(t))+32e2|fTγ(t)(ˉζ2)|2+IIU. | (3.27) |
By definition of e and lemma 2.2 in [22], we have
e3∂L(ζ1)∂ζ1([η+L(ζ1)]m(t)−[ζ2]m(t))≤−12ˉm−1∂L(ζ1)∂ζ1em(t)+3. | (3.28) |
From (3.12), (3.17) and Assumption 2.1, we get
32e2|fTγ(t)(ˉζ2)|2≤3˜f2(ζ1)|e|2(|ζ1|m(t)+1+|ζ2|m(t)+1)≤3˜f2(ζ1)φ2(|e|2|ξ2|m(t)+1+|e|2|ξ1|m(t)+1). | (3.29) |
By using Lemma 2.1 in [20], we have
3˜f2(ζ1)φ2|e|2|ξ1|1+m(t)≤|ξ1|3+m(t)+β31(ζ1)|e|3+m(t),3˜f2(ζ1)φ2|e|2|ξ2|1+m(t)≤12|ξ2|3+m(t)+β32(ζ1)|e|3+m(t), | (3.30) |
where
β31(ζ1)=2m_+3(3˜f2(ζ1)φ2)ˉm+32(m_+3ˉm+1)−ˉm+12,β32(ζ1)=2m_+3(3˜f2(ζ1)φ2)ˉm+32(3+m_2(1+ˉm))−1+ˉm2. | (3.31) |
Substituting (3.30) into (3.29) yields
32e2|fTγ(t)(ˉζ2)|2≤|ξ1|m(t)+3+12|ξ2|m(t)+3+β3(ζ1)|e|m(t)+3, | (3.32) |
where β3(ζ1)=β31(ζ1)+β32(ζ1)≥0 is a smooth function irrelevant to m(t).
Substituting (3.28), (3.32) into (3.27) yields
LU≤|ξ1|m(t)+3+12|ξ2|m(t)+3−(12ˉm−1∂L(ζ1)∂ζ1−β3(ζ1))|e|m(t)+3+IIU. | (3.33) |
Since ζ2 is unmeasurable, replace ζ2 in virtual controller x∗3 with η+L(ζ1), and we can get the controller as follows
u=−α2(ζ1)(η+L(ζ1)+α1ζ1). | (3.34) |
By (3.22), (3.24) and (3.34), we obtain
LV2≤−(c1−1)|ξ1|m(t)+3−c2|ξ2|m(t)+3+ξ32αˉm2(ζ1)([ξ2]m(t)−[ξ2−e]m(t))+IIV2. | (3.35) |
By using Lemma 1 in [19], we have
ξ32αˉm2(ζ1)([ξ2]m(t)−[ξ2−e]m(t))≤αˉm2(ζ1)ˉm(2ˉm−2+2)(|ξ2|3|e|m(t)+|e||ξ2|m(t)+2). | (3.36) |
By using Lemma 2.1 in [20], we get
αˉm2(ζ1)ˉm(2ˉm−2+2)|ξ2|3|e|m(t)≤14|ξ2|3+m(t)+β41(ζ1)|e|3+m(t),αˉm2(ζ1)ˉm(2ˉm−2+2)|e||ξ2|2+m(t)≤14|ξ2|3+m(t)+β42(ζ1)|e|3+m(t), | (3.37) |
where
β41(ζ1)=ˉmm_+3(αˉm2(ζ1)ˉm(2ˉm−2+2))ˉm+3m_(m_+312)−3ˉm,β42(ζ1)=1m_+3(αˉm2(ζ1)ˉm(2ˉm−2+2))ˉm+3(m_+34(ˉm+2))−(ˉm+2). | (3.38) |
Substituting (3.37) into (3.36) yields
ξ32αˉm2(ζ1)([ξ2]m(t)−[ξ2−e]m(t))≤12|ξ2|3+m(t)+β4(ζ1)|e|3+m(t), | (3.39) |
where β4(ζ1)=β41(ζ1)+β42(ζ1)≥0 is a smooth function irrelevant to m(t).
By using (3.39) and (3.35), we have
LV2≤−(c1−1)|ξ1|m(t)+3−(c2−12)|ξ2|m(t)+3+β4(ζ1)|e|m(t)+3+IIV2. | (3.40) |
Choosing V(ξ1,ξ2,e)=V2(ξ1,ξ2)+U(e), by (3.33) and (3.40), we obtain
LV≤−(c1−2)|ξ1|m(t)+3−(c2−1)|ξ2|m(t)+3−(12ˉm−1∂L(ζ1)∂ζ1−β3(ζ1)−β4(ζ1))|e|m(t)+3+IIV. | (3.41) |
Let
L(ζ1)=12ˉm−1(c3ζ1+∫ζ10(β3(s)+β4(s))ds), | (3.42) |
and the controller as
u=−α2(ζ1)(η+12ˉm−1(c3ζ1+∫ζ10(β3(s)+β4(s))ds)+α1ζ1), | (3.43) |
where c3>0 is a design parameter.
By using (3.41) and (3.42), we can obtain
LV≤−(c1−2)|ξ1|m(t)+3−(c2−1)|ξ2|m(t)+3−c3|e|m(t)+3+IIV. | (3.44) |
Remark 3.1. If m(t) is time-invariant and the growth rate is a constant rather than a smooth function, such as those in [7,8,9], from (3.32) and (3.39), β3 and β4 are constants irrelevant to ζ1. Then, the dynamic gain L(ζ) is a linear function of ζ1. We can design L(ζ1)=cζ1 by choosing the right parameter c to make LV in (3.41) negative definite. However, in this paper, the growth rate ˜f(ζ1) is a nonnegative smooth function and the m(t) is time-varying and non-differentiable, which makes the deducing of the dynamic gain much more difficult. To solve this problem, we introduce two constants m_ and ˉm, which are reasonably used in the design process, see (3.7) and (3.11). In this way, the dynamic gain (3.42) can be designed irrelevant to m(t), which is crucial to assure the effectiveness of the observer and controller. This is one of the main innovations of this paper.
In this section, for the closed-loop system (2.1), (3.25) and (3.43), we first give a lemma, which is useful to prove the system has a unique solution. Then, we present the main results of the stability analysis.
Lemma 4.1. For ζ∈R, the function g(ζ)=[ζ]m(t) satisfies the locally Lipschitz condition.
Proof. If ζ=0, we can get
h′+(0)=limζ→0+h(ζ)−h(0)ζ=0,h′−(0)=limζ→0−h(ζ)−h(0)ζ=0. | (4.1) |
Then, we have
dhdζ|ζ=0=h′+(0)=h′−(0)=0, | (4.2) |
thus, h(ζ) is differentiable function in ζ=0 and so meets the locally Lipschitz condition in ζ=0.
As ζ>0, we get
h(ζ)=[ζ]m(t)=ζm(t). | (4.3) |
For m(t)≥1, h(ζ) is differentiable function in ζ>0, so meets the locally Lipschitz condition in ζ>0. Similarly, as ζ<0, the conclusion is valid.
Therefore, the conclusion holds for ζ∈R.
Next, we give the stability results.
Theorem 4.1. Under Assumption 2.1, for the system (2.1), using the observer (3.25) and controller (3.43) with
ci>3−i,i=1,2,3, | (4.4) |
we can get
1) For each ζ(t0)=ζ0∈R2 and γ(t0)=i0∈S, the closed-loop system has an almost surely unique solution on [0,+∞);
2) For any ζ0∈R2 and i0∈S, the closed-loop system is almost surely regulated to the equilibrium at the origin.
Proof. By (2.1), (3.25), (3.43) and using Lemma 4.1, we can conclude that the closed-loop system satisfies the locally Lipschitz condition. By (3.2), (3.22), (3.25) and (3.42), we can get that ξ1,ξ2,η are bounded, which implies that ζ1 is bounded, which means that
VR=inft≥t0,|ζ|>RV(ζ(t))→∞⟺R→∞. | (4.5) |
Through the verification of the controller development process, we choose appropriate design parameters ci to satisfy (4.4), and we can get IIV=0. For each l>0, the first exit time is defined as
σl=inf{t:t≥t0,|ζ(t)|≥l}. | (4.6) |
When t≥t0, choose tl=min{σl,t}. We can obtain that bounded |ζ(t)| on interval [t0,tl] a.s., which means that V(ζ) is bounded in the interval [t0,tl] a.s. By using (3.44), we can get that LV is bounded in the interval [t0,tl] a.s. By using Lemma 1.9 in [11], (3.44) and (4.4), we can obtain
EV(ζ(tl))≤EV(ζ(t0)). | (4.7) |
By (4.5), (4.7) and using Lemma 1 in [23], we can obtain conclusion (1).
From (3.44), (4.5), by using Theorem 2.1 in [24], we can prove conclusion (2).
In this section, a simulation example is given to show the availability of the control method.
Study the stabilization for system with two modes. The Markov process γ(t) belongs to the space S={1,2} with generator Γ=(λij)2×2 given by λ11=2,λ12=−2,λ21=−1 and λ22=1. We have π1=13,π2=23. When γ(t)=1, the systems can be written as
dζ1=[ζ2]32+12sintdt,dζ2=[u]32+12sintdt+ζ1sinζ2dω,y=ζ1, | (5.1) |
where m(t)=32+12sint,m_=1,ˉm=2. When γ(t)=2, the systems are described by
dζ1=[ζ2]2+sintdt,dζ2=[u]2+sintdt+12ζ21sinζ2dω,y=ζ1, | (5.2) |
where m(t)=2+sint,m_=1,ˉm=3. Clearly, system (5.1) and (5.2) satisfy Assumption 2.1.
According to the above design process, when γ(t)=1, the observer is constructed as
dη=([u]32+12sint−∂L(ζ1)∂ζ1[η+L(ζ1)]32+12sint)dt, | (5.3) |
and the control is
u=−(c2+4ζ21)(η+L(ζ1)+c1ζ1), | (5.4) |
where L(ζ1)=12(c3ζ1+6ζ21).
When γ(t)=2, the observer is constructed as
dη=([u]2+sint−∂L(ζ1)∂ζ1[η+L(ζ1)]2+sint)dt, | (5.5) |
and the control is
u=−(c2+4ζ1+12ζ21)(η+L(ζ1)+c1ζ1), | (5.6) |
where L(ζ1)=14(c3ζ1+20ζ1+4ζ21).
For simulation, we select c1=6,c2=6,c3=5, and the initial conditions as ζ1(0)=−1,ζ2(0)=2,η(0)=−5. We can obtain Figure 1, which illustrates that the signals of the closed-loop system (ζ1,ζ2,u,η,e) converge to zero. Specifically, the states and controller of the closed-loop system converge to zero. The observation error also converges to zero, which means that our constructed observer and controller are efficient. Figure 2 illustrates the jump of Markov process γ(t) in 1 and 2.
Remark 5.1. It can be observed from the example that there are time-varying powers and Markovian switching in systems (5.1) and (5.2). For the output-feedback control of the system (5.1) and (5.2), the method in [7,8,9] fails since they can only deal with time-invariant powers without Markovian switching. To solve the difficulties caused by time-varying powers, we introduce constants 1, 2, and 1, 3 so that the design of the observer and controller is irrelevant to the power. This is one of the characteristics of our controller and observer design scheme (5.3)–(5.6).
We investigate the output-feedback stabilization for stochastic nonlinear systems with both Markovian switching and time-varying powers in this paper. Compared with existing work, the system model considered in this paper is more general because it studies the time-varying power and Markovian switching, simultaneously. To achieve stabilization, we first design a state observer with a dynamic gain and an output-feedback controller, then use advanced stochastic analysis techniques to prove that the closed-loop system has an almost surely unique solution and the states are regulated to the origin almost surely. Even though there is no Markovian switching, the results in this paper are also new in the sense that we consider nonlinear growth rate, which is much more general than constant growth rate cases in [7,8,9].
There are many related problems to be considered, such as how to extend the result to impulsive systems [25,26,27] and systems with arbitrary order.
This work is funded by Shandong Province Higher Educational Excellent Youth Innovation team, China (No. 2019KJN017), and Shandong Provincial Natural Science Foundation for Distinguished Young Scholars, China (No. ZR2019JQ22).
The authors declare there is no conflict of interest.
[1] |
R. Kaur, S. Singh, A comprehensive review of object detection with deep learning, Digital Signal Process., 132 (2023), 103812. https://doi.org/10.1016/j.dsp.2022.103812 doi: 10.1016/j.dsp.2022.103812
![]() |
[2] |
P. Jiang, D. Ergu, F. Liu, Y. Cai, B. Ma, A Review of Yolo algorithm developments, Proc. Comput. Sci., 199 (2022), 1066–1073. https://doi.org/10.1016/j.procs.2022.01.135 doi: 10.1016/j.procs.2022.01.135
![]() |
[3] |
W. Liu, G. Wu, F. Ren, X. Kang, DFF-ResNet: An insect pest recognition model based on residual networks, Big Data Min. Anal., 3 (2020), 300–310. https://doi.org/10.26599/BDMA.2020.9020021 doi: 10.26599/BDMA.2020.9020021
![]() |
[4] |
A. Mughees, L. Tao, Multiple deep-belief-network-based spectral-spatial classification of hyperspectral images, Tsinghua Sci. Technol., 24 (2019), 183–194. https://doi.org/10.26599/TST.2018.9010043 doi: 10.26599/TST.2018.9010043
![]() |
[5] | T. Y. Lin, M. Maire, S. Belongie, J. Hays, P. Perona, D. Ramanan, et al., Microsoft COCO: Common objects in context, in European Conference on Computer Vision, (2014), 740–755. https://doi.org/10.1007/978-3-319-10602-1_48 |
[6] |
O. Russakovsky, J. Deng, H. Su, J. Krause, S. Satheesh, S. Ma, et al., ImageNet large scale visual recognition challenge, Int. J. Comput. Vis., 115 (2015), 211–252. https://doi.org/10.1007/s11263-015-0816-y doi: 10.1007/s11263-015-0816-y
![]() |
[7] |
Y. Fan, D. Ni, H. Ma, HyperDB: a hyperspectral land class database designed for an image processing system, Tsinghua Sci. Technol., 22 (2017), 112–118. https://doi.org/10.1109/TST.2017.7830901 doi: 10.1109/TST.2017.7830901
![]() |
[8] |
M. Everingham, S. M. A. Eslami, L. Van Gool, C. K. I. Williams, J. Winn, A. Zisserman, The PASCAL visual object classes challenge: A retrospective, Int. J. Comput. Vis., 111 (2015), 98–136. https://doi.org/10.1007/s11263-014-0733-5 doi: 10.1007/s11263-014-0733-5
![]() |
[9] |
S. Ren, K. He, R. Girshick, J. Sun, Faster R-CNN: Towards real-time object detection with region proposal networks, IEEE Trans. Pattern Anal. Mach. Intell., 39 (2017), 1137–1149. https://doi.org/10.1109/TPAMI.2016.2577031 doi: 10.1109/TPAMI.2016.2577031
![]() |
[10] | T. Y. Lin, P. Goyal, R. Girshick, K. He, P. Dollár, Focal loss for dense object detection, in 2017 IEEE International Conference on Computer Vision (ICCV), (2017), 2999–3007. https://doi.org/10.1109/ICCV.2017.324 |
[11] | X. Zhou, D. Wang, P. Krähenbühl, Objects as points, preprint, arXiv: 1904.07850. |
[12] |
K. He, G. Gkioxari, P. Dollár, R. Girshick, Mask R-CNN, IEEE Trans. Pattern Anal. Mach. Intell., 42 (2020), 386–397. https://doi.org/10.1109/TPAMI.2018.2844175 doi: 10.1109/TPAMI.2018.2844175
![]() |
[13] | M. Chen, F. Bai, Z. Gerile, Special object detection based on Mask RCNN, in 2021 17th International Conference on Computational Intelligence and Security (CIS), (2021), 128–132. https://doi.org/10.1109/CIS54983.2021.00035 |
[14] |
Z. Ou, Z. Wang, F. Xiao, B. Xiong, H. Zhang, M. Song, et al., AD-RCNN: Adaptive dynamic neural network for small object detection, IEEE Int. Things J., 10 (2023), 4226–4238. https://doi.org/10.1109/JIOT.2022.3215469 doi: 10.1109/JIOT.2022.3215469
![]() |
[15] |
L. Yang, Y. Xu, S. Wang, C. Yang, Z. Zhang, B. Li, et al., PDNet: Toward better one-stage object detection with prediction decoupling, IEEE Trans. Image Process., 31 (2022), 5121–5133. https://doi.org/10.1109/TIP.2022.3193223 doi: 10.1109/TIP.2022.3193223
![]() |
[16] | J. Redmon, S. Divvala, R. Girshick, A. Farhadi, You only look once: Unified, real-time object detection, in 2016 IEEE Conference on Computer Vision and Pattern Recognition (CVPR), (2016), 770–778. https://doi.ieeecomputersociety.org/10.1109/CVPR.2016.91 |
[17] | W. Liu, D. Anguelov, D. Erhan, C. Szegedy, S. Reed, C. Y. Fu, et al., SSD: Single shot multiBox detector, in European Conference on Computer Vision, (2016), 21–37. https://doi.org/10.1007/978-3-319-46448-0_2 |
[18] | J. Redmon, A. Farhadi, YOLOv3: An incremental improvement, preprint, arXiv: 1804.02767. |
[19] |
G. Wang, J. Wu, B. Tian, S. Teng, L. Chen, D. Cao, et al., CenterNet3D: An anchor free object detector for point cloud, IEEE Trans. Intell. Transp. Syst., 23 (2022), 12953–12965. https://doi.org/10.1109/TITS.2021.3118698 doi: 10.1109/TITS.2021.3118698
![]() |
[20] | Z. Tian, C. Shen, H. Chen, T. He, FCOS: Fully convolutional one-stage object detection, in 2019 IEEE/CVF International Conference on Computer Vision (ICCV), (2019), 9626–9635. https://doi.ieeecomputersociety.org/10.1109/ICCV.2019.00972 |
[21] |
H. Law, J. Deng, CornerNet: Detecting objects as paired keypoints, Int. J. Comput. Vis., 128 (2020), 642–656. https://doi.org/10.1007/s11263-019-01204-1 doi: 10.1007/s11263-019-01204-1
![]() |
[22] |
J. Canny, A computational approach to edge detection, IEEE Trans. Pattern Anal. Mach. Intell., 8 (1986), 679–698. https://doi.org/10.1109/TPAMI.1986.4767851 doi: 10.1109/TPAMI.1986.4767851
![]() |
[23] |
D. Marr, E. Hildreth, Theory of edge detection, Proc. R. Soc. Lond. B, 207 (1980), 187–217. https://doi.org/10.1098/rspb.1980.0020 doi: 10.1098/rspb.1980.0020
![]() |
[24] |
J. Kittler, On the accuracy of the Sobel edge detector, Image Vis. Comput., 1 (1983), 37–42. https://doi.org/10.1016/0262-8856(83)90006-9 doi: 10.1016/0262-8856(83)90006-9
![]() |
[25] |
D. R. Martin, C. C. Fowlkes, J. Malik, Learning to detect natural image boundaries using local brightness, color, and texture cues, IEEE Trans. Pattern Anal. Mach. Intell., 26 (2004), 530–549. https://doi.org/10.1109/TPAMI.2004.1273918 doi: 10.1109/TPAMI.2004.1273918
![]() |
[26] |
P. Arbeláez, M. Maire, C. Fowlkes, J. Malik, Contour detection and hierarchical image segmentation, IEEE Trans. Pattern Anal. Mach. Intell., 33 (2011), 898–916. https://doi.org/10.1109/TPAMI.2010.161 doi: 10.1109/TPAMI.2010.161
![]() |
[27] | J. J. Lim, C. L. Zitnick, P. Dollár, Sketch tokens: A learned mid-level representation for contour and object detection, in 2013 IEEE Conference on Computer Vision and Pattern Recognitionn, (2013), 3158–3165. https://doi.org/10.1109/CVPR.2013.406 |
[28] | P. Dollár, C. L. Zitnick, Structured forests for fast edge detection, in 2013 IEEE International Conference on Computer Vision, (2013), 1841–1848. https://doi.org/10.1109/ICCV.2013.231 |
[29] | S. Xie, Z. Tu, Holistically-nested edge detection, in 2015 IEEE International Conference on Computer Vision (ICCV), (2015), 1395–1403. https://doi.org/10.1109/ICCV.2015.164 |
[30] | G. Bertasius, J. Shi, L. Torresani, DeepEdge: A multi-scale bifurcated deep network for top-down contour detection, in 2015 IEEE Conference on Computer Vision and Pattern Recognition (CVPR), (2015), 4380–4389. https://doi.org/10.1109/CVPR.2015.7299067 |
[31] | W. Shen, X. Wang, Y. Wang, X. Bai, Z. Zhang, DeepContour: A deep convolutional feature learned by positive-sharing loss for contour detection, in 2015 IEEE Conference on Computer Vision and Pattern Recognition (CVPR), (2015), 3982–3991. https://doi.org/10.1109/CVPR.2015.7299024 |
[32] | Z. Huang, L. Huang, Y. Gong, C. Huang, X. Wang, Mask scoring R-CNN, in 2019 IEEE/CVF Conference on Computer Vision and Pattern Recognition (CVPR), (2019), 6402–6411. https://doi.org/10.1109/CVPR.2019.00657 |
[33] | K. Chen, J. Pang, J. Wang, Y. Xiong, X. Li, S. Sun, et al., Hybrid task cascade for instance segmentation, in 2019 IEEE/CVF Conference on Computer Vision and Pattern Recognition (CVPR), (2019), 4974–4983. https://doi.org/10.1109/CVPR.2019.00511 |
[34] | C. L. Zitnick, P. Dollár, Edge boxes: Locating object proposals from edges, in European Conference on Computer Vision, (2014), 391–405. https://doi.org/10.1007/978-3-319-10602-1_26 |
[35] | J. Dai, H. Qi, Y. Xiong, Y. Li, G. Zhang, H. Hu, et al., Deformable convolutional networks, in 2017 IEEE International Conference on Computer Vision (ICCV), (2017), 764–773. https://doi.org/10.1109/ICCV.2017.89 |
[36] | Y. Kim, T. Kim, B. N. Kang, J. Kim, D. Kim, BAN: Focusing on boundary context for object detection, in Asian Conference on Computer Vision, (2018), 555–570. https://doi.org/10.1007/978-3-030-20876-9_35 |
[37] | J. Wang, W. Zhang, Y. Cao, K. Chen, J. Pang, T. Gong, et al., Side-aware boundary localization for more precise object detection, in European Conference on Computer Vision, (2020), 403–419. https://doi.org/10.1007/978-3-030-58548-8_24 |
[38] | C. Y. Fu, W. Liu, A. Ranga, A. Tyagi, A. C. Berg, DSSD: Deconvolutional single shot detector, preprint, arXiv: 1701.06659. |
[39] | R. Araki, T. Onishi, T. Hirakawa, T. Yamashita, H. Fujiyoshi, MT-DSSD: Deconvolutional single shot detector using multi task learning for object detection, segmentation, and grasping detection, in 2020 IEEE International Conference on Robotics and Automation (ICRA), (2020), 10487–10493. https://doi.org/10.1109/ICRA40945.2020.9197251 |
[40] | C. Y. Fu, M. Shvets, A. C. Berg, RetinaMask: Learning to predict masks improves state-of-the-art single-shot detection for free, preprint, arXiv: 1901.03353. |
[41] | R. K. Meleppat, M. V. Matham, L. K. Seah, Optical frequency domain imaging with a rapidly swept laser in the 1300nm bio-imaging window, in International Conference on Optical and Photonic Engineering, (2015), 721–729. https://doi.org/10.1117/12.2190530 |
[42] |
R. K. Meleppat, C. R. Fortenbach, Y. Jian, E. S. Martinez, K. Wagner, B. S. Modjtahedi, et al., In Vivo Imaging of Retinal and Choroidal Morphology and Vascular Plexuses of Vertebrates Using Swept-Source Optical Coherence Tomography, Transl. Vis. Sci. Technol., 11 (2022), 11. https://doi.org/10.1167/tvst.11.8.11 doi: 10.1167/tvst.11.8.11
![]() |
[43] | H. Huang, Research on Object Detection Based on Improved MASK R-CNN, Master's degree, Lanzhou University in Lanzhou, 2021. https://doi.org/10.27204/d.cnki.glzhu.2021.001818 |
1. | Xinling Li, Xueli Qin, Zhiwei Wan, Weipeng Tai, Chaos synchronization of stochastic time-delay Lur'e systems: An asynchronous and adaptive event-triggered control approach, 2023, 31, 2688-1594, 5589, 10.3934/era.2023284 | |
2. | Jingya Wang, Ye Zhu, L2−L∞ control for memristive NNs with non-necessarily differentiable time-varying delay, 2023, 20, 1551-0018, 13182, 10.3934/mbe.2023588 |