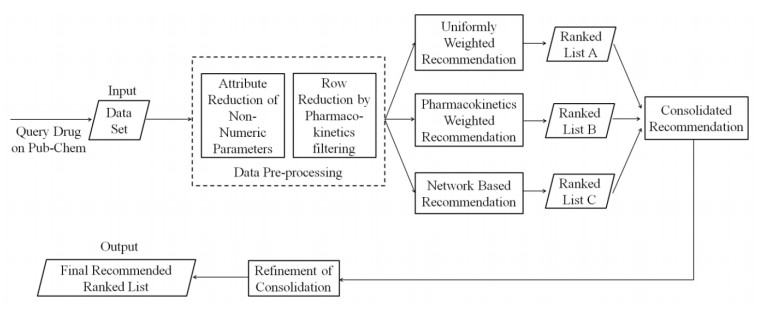
This paper investigates L2−L∞ control for memristive neural networks (MNNs) with a non-necessarily differentiable time-varying delay. The objective is to design an output-feedback controller to ensure the L2−L∞ stability of the considered MNN. A criterion on the L2−L∞ stability is proposed using a Lyapunov functional, the Bessel-Legendre inequality, and the convex combination inequality. Then, a linear matrix inequalities-based design scheme for the required output-feedback controller is developed by decoupling nonlinear terms. Finally, two examples are presented to verify the proposed L2−L∞ stability criterion and design method.
Citation: Jingya Wang, Ye Zhu. L2−L∞ control for memristive NNs with non-necessarily differentiable time-varying delay[J]. Mathematical Biosciences and Engineering, 2023, 20(7): 13182-13199. doi: 10.3934/mbe.2023588
[1] | Juan Zhou, Xiong Li, Yuanting Ma, Zejiu Wu, Ziruo Xie, Yuqi Zhang, Yiming Wei . Optimal modeling of anti-breast cancer candidate drugs screening based on multi-model ensemble learning with imbalanced data. Mathematical Biosciences and Engineering, 2023, 20(3): 5117-5134. doi: 10.3934/mbe.2023237 |
[2] | Saranya Muniyappan, Arockia Xavier Annie Rayan, Geetha Thekkumpurath Varrieth . DTiGNN: Learning drug-target embedding from a heterogeneous biological network based on a two-level attention-based graph neural network. Mathematical Biosciences and Engineering, 2023, 20(5): 9530-9571. doi: 10.3934/mbe.2023419 |
[3] | Meng Jiang, Bo Zhou, Lei Chen . Identification of drug side effects with a path-based method. Mathematical Biosciences and Engineering, 2022, 19(6): 5754-5771. doi: 10.3934/mbe.2022269 |
[4] | Silvia Martorano Raimundo, Hyun Mo Yang, Ezio Venturino . Theoretical assessment of the relative incidences of sensitive andresistant tuberculosis epidemic in presence of drug treatment. Mathematical Biosciences and Engineering, 2014, 11(4): 971-993. doi: 10.3934/mbe.2014.11.971 |
[5] | Abba B. Gumel, Baojun Song . Existence of multiple-stable equilibria for a multi-drug-resistant model of mycobacterium tuberculosis. Mathematical Biosciences and Engineering, 2008, 5(3): 437-455. doi: 10.3934/mbe.2008.5.437 |
[6] | Bo Zhou, Bing Ran, Lei Chen . A GraphSAGE-based model with fingerprints only to predict drug-drug interactions. Mathematical Biosciences and Engineering, 2024, 21(2): 2922-2942. doi: 10.3934/mbe.2024130 |
[7] | Rebeccah E. Marsh, Jack A. Tuszyński, Michael Sawyer, Kenneth J. E. Vos . A model of competing saturable kinetic processes with application to the pharmacokinetics of the anticancer drug paclitaxel. Mathematical Biosciences and Engineering, 2011, 8(2): 325-354. doi: 10.3934/mbe.2011.8.325 |
[8] | Chenhao Wu, Lei Chen . A model with deep analysis on a large drug network for drug classification. Mathematical Biosciences and Engineering, 2023, 20(1): 383-401. doi: 10.3934/mbe.2023018 |
[9] | Cristian Tomasetti, Doron Levy . An elementary approach to modeling drug resistance in cancer. Mathematical Biosciences and Engineering, 2010, 7(4): 905-918. doi: 10.3934/mbe.2010.7.905 |
[10] | Shizhen Huang, ShaoDong Zheng, Ruiqi Chen . Multi-source transfer learning with Graph Neural Network for excellent modelling the bioactivities of ligands targeting orphan G protein-coupled receptors. Mathematical Biosciences and Engineering, 2023, 20(2): 2588-2608. doi: 10.3934/mbe.2023121 |
This paper investigates L2−L∞ control for memristive neural networks (MNNs) with a non-necessarily differentiable time-varying delay. The objective is to design an output-feedback controller to ensure the L2−L∞ stability of the considered MNN. A criterion on the L2−L∞ stability is proposed using a Lyapunov functional, the Bessel-Legendre inequality, and the convex combination inequality. Then, a linear matrix inequalities-based design scheme for the required output-feedback controller is developed by decoupling nonlinear terms. Finally, two examples are presented to verify the proposed L2−L∞ stability criterion and design method.
Tuberculosis (TB), caused by the bacteria Mycobacterium tuberculosis (Mtb), is a major global health hazard, where the human mortality rate goes above 1 million per year. Surveys have revealed the existence of Mtb for more than four decades [1], and more importantly, it has developed various degrees of resistance to multiple anti tuberculosis drugs along this journey. Studies have also shown that [2] the pathways that led to these drug resistant Mtb strains were very complex and indicated that the bacteria acquired a step by step process, starting from a low degree mutation to a near complete drug resistance. The role of bacterial factors like persistence, compensatory evolution, fitness, hyper mutation on the evolution of drug resistance were also explored [3].
There are various resources which documented the drug resistant properties of Mtb by various types of databases [4]. For example, the TBDRMD database [5] reported polymorphisms at different codon regions of certain genes of H37Rv, a specific strain of Mtb, in response to specific anti tuberculosis drugs. The VFDB database [6] provided virulence factors and structural features of different strains of Mtb. Studies [4] stressed on the urgent need to rapidly discover new drugs which could tackle the mutating drug resistant strains of Mtb.
PubChem, an open chemistry database [7], provided an extensive resource on chemical structures, chemical and physical properties of a chemical compound (drug) as well as a comprehensive list of compounds (drugs) which were similar in nature. It was encouraging to know that a large number of growth inhibitors for Mtb were submitted [8] on PubChem after an elaborate process of experimentation and validation. This ensured that the list of similar drugs/compounds provided by PubChem, did not have any compounds that acted as inhibitors. Thus, for this paper, PubChem was chosen to be the resource which could give an initial list of drugs which had some kind of similarity with an existing anti tuberculosis drug.
The basic functional effectiveness of any drug depends heavily on the molecular properties that are crucial to its pharmacokinetics, including absorption, distribution, metabolism and excretion (ADME). The well-established Lipinski's rule of five [9] defined some boundaries on the chemical properties of the drugs which, when satisfied, made the drugs more functionally acceptable for human intake. This important aspect was emphasized in our proposed methodology, both during data pre-processing as well as during the actual recommendation process.
There were many instances found in the literature where machine learning techniques were used to suggest new drugs, using available datasets related to drug resistant Mtb. Reviews [4] have pointed out cheminformatcs tools that were used on several datasets related to drug resistant Mtb. For example, a cheminformatics data fusion approach [10] was followed by validation on datasets having cytotoxicity and tuberculosis data by Support Vector Machine and Bayesian models. Subsequently, Bayesian machine learning models were applied [11] on large datasets for rapid screening and prioritizing of compounds for anti-tubercular activities before in vitro testing. As both high throughput screening techniques and chemical datasets expanded [12], the needs to integrate and link the different datasets as well as consolidate the techniques by secure mechanisms were proposed. It has been reported that [13], popular machine learning techniques in cheminformatics can be useful in making the drug discovery process more efficient. This was due to the faster selection of filtered compounds for testing, when compared to the traditional in vitro screening for toxicity of compounds. This inference was also validated [14] when structure-activity relationships of a couple of Mtb enzymes were analysed both by in vitro screening as well as Naïve Bayesian model with smoothening. In another review [15], the relevance of the advancement of tools, including machine learning techniques, were highlighted with regard to development of anti-tuberculosis drugs.
Literature also revealed interesting approaches, other than throughput screening and cheminformatics tools, which were applied for drug discovery against drug resistant Mtb. Lipophilicity [16], along with structural peculiarities and energy depletion, in some cases, were reported to be more relevant than Lipinsky's rule of five, in the specific context of drug resistant TB. Reports [17] have summarised newer anti-tuberculosis drugs in different stages of clinical trials, along with their various methods of action. In another study [18[, the efficacy of Ethambutol (EMB), a popular anti-tuberculosis drug, was evaluated on how the drug affected both the cell wall integrity and the metabolism of the Mtb.
The review [15], mentioned before, even though acknowledged the effectiveness of compounds like EMB and INH as part of a multi-drug regimen against TB, it also underlined the critical need to come up with new anti-tuberculosis drugs, and suggested using repurposed drugs by exploring properties like genomics and crystallography. Reports [19] have also suggested that existing drugs meant for Parkinson's disease could be repositioned to treat drug resistant TB, by modelling protein-ligand interaction networks and exploring the bonding and docking properties. In the context of drug repurposing in general, reviews [20] classified them through two axes, drug based or disease based. The review also highlighted computational methods of repurposing benefitted both these axes. Studies [21] have also pointed out three actual repurposed drugs which were extensively used to treat drug resistant Mtb, when traditional combinations of anti-tuberculosis drugs were not effective. One study [22] looked at repurposing in general, by computational workflows, linking data availability with current trends in technology along with the associated algorithms. On similar lines, drug repositioning [23] was highlighted for associations between drug to target and target to disease. In an experimental study [24], Primaquine derivatives were shown to be effective as both anti-malarial and anti-tuberculosis agents. In one more recent study [25], a traditional anti-tuberculosis drug, AMC, and a repurposed drug, Diosmin, were used together to treat TB. Repurposing of drugs might be an effective way to deal with a current disease, however, the process would always require significant experimental studies. Network structure based approach, on the other hand, would stress more on the computational part to come up with candidates efficiently, which in turn could reduce the number of candidates for undergoing subsequent experimental studies.
There were some studies which looked at drug discovery, in general, from a graph oriented, network based approach. PROMISCOUS [26] was a database that had an extensive list of drugs annotated with protein-protein interactions (PPI) and drug-protein interactions. This study highlighted structural similarities among drugs, connected with PPI, which has the potential in the field of multi-pharmacology, and drug repositioning. Network analysis approaches [27] were studied in the context of drug discovery, and similarities were suggested in both social networks and biological networks. Incomplete network motifs of bi-cliques [28] were utilised in drug-target-disease networks to predict new drugs for one or more diseases. In one study, molecular interaction networks [29] were explored for lead identification in drug discovery pipeline. It also pointed out how this can help in exploring the emergence of drug resistance and drug repositioning. Reports [30] had also suggested the need to connect genome-based biological networks with anti-tuberculosis drug discovery to come up with potentially rational drugs. Surveys [31] had shown that network based computational approaches focussed on molecular interactions can address both drug repositioning, as well as drug combination. In case of finding drugs for Mtb, both of these (repositioning and combination) were very crucial for effective treatment of TB. A proteomic structural approach [32] was adopted for creation of clusters of pocket-similarity networks, or pocketomes, which consequently helped in finding sets of binding sites within Mtb. In a recent study [33], a drug-disease proximity measure was proposed by looking into the network neighbourhoods of both disease genes and drugs. This study revealed that effectiveness of most of the drugs was limited to small sub networks of disease genes.
In summary, the entire literature study repeatedly highlighted the importance of coming up with discovery of relevant and effective anti-tuberculosis drugs in a rapid manner. Majority of these studies acknowledged the importance of the pharmacokinetic/ADME properties while discovering the new drugs, while quite a few of them looked at the problem from the structural network point of view. With these issues in context, this work is aimed at providing a recommendation list of possible anti-tuberculosis drugs which were similar to a popular and existing tuberculosis drug. The proposed method incorporated an ensemble approach, where both pharmacokinetic properties as well as network properties of the chemical structures of the recommended drugs were given special attention. For this ensemble recommendation approach, literature showed that Borda Count [34] was a very well-established method and was used both in social science, as well as in machine learning domains. Studies [35] also showed that Borda Count can be effectively tailored for specific scenarios. In this context, the uniqueness of this effort was in the customisation of this popular ensemble ranking method, Borda Count, as well as utilising the network properties of the recommended drugs for the second time to fine tune the final recommendation list, and all these specific customisations were made to address the drug resistant property of the Mtb.
As a side note, artificial intelligence techniques [36], machine learning tools [37], with or without ensembles, as well as deep learning techniques [38] have been utilised very recently to tackle the covid-19 disease, with its continuously changing variants.
This section provided a basic introduction to the needs and a summary of techniques practised in discovering drugs against drug resistant Mtb. Section 2 details the methodology of this study along with our proposed ensemble recommendation system. Section 3 provides the experimental details for a popular anti-tuberculosis drug, and documents the results at each step of the process. Section 4 discusses and analyses the significance of our results, and finally, Section 5 highlights the conclusion and future possibilities.
The process of consolidating the recommendation list of proposed TB drugs from a given effective TB drug is shown by the block diagram in Figure 1. The process involved data pre-processing at the beginning, followed by the generation of three different recommendation lists (Uniform weighted, Pharmacokinetic weighted and Network weighted). These were then fed into an ensemble recommendation system resulting in a consolidated recommendation. The final step involved refinement of the consolidated ranking by utilising the Network weighted list of values.
The online PubChem database provided a list of drugs having similar characteristics for a TB drug given as a query. The dataset consisted of the list of drugs along with several attributes for each of these drugs. Since the objective of the research work dealt with providing recommendations by utilising different computational measures, a quantifiable approach was the basic requirement. In this context, data pre-processing was done on two fronts. The first filtering was done on numeric/non-numeric nature of the attributes, and the subsequent filtering was done on the pharmacokinetic values of the attributes. Additionally, for each of these pre-processing steps, each row/entry was checked for null values and missing values. Such entries, if found, were to be removed completely.
From the initial dataset, at first, all non-numeric attributes were removed. This paved the way for performing numerical computations.
The well-established pharmacokinetic properties of chemical drugs, provided by Lipinski's rule of five, Ghose Filter and Verber's rule, were applied to the relevant attribute values and those drugs whose attributes did not satisfy any of these rules, were removed. The combined rules are listed in Table 1.
Attribute Constraints | Filter Rule |
hbond donor count <=5 | Lipinski's Rule |
hbond receptor count <=10 | Lipinski's Rule |
180 <= Molecular weight <=480 | Lipinski's Rule and Ghose Filter (combined) |
-0.4 <= logP < 5 | Lipinski's Rule and Ghose Filter (combined) |
20 <=Total number of atoms <=70 | Ghose Filter |
Polar surface area <=140 | Verber's Rule |
Number of rotatable bonds <=10 | Verber's Rule |
Three different ranked lists were generated from the processed dataset. The objective was to explore multiple ways of finding k nearest neighbors/drugs for the given query drug, where the entries in the ranked recommendation lists were in ascending order of dissimilarity. This meant that the topmost drug on a particular list would be the closest to the queried drug, as per the chosen measure of evaluation, and consequently the bottommost (k th) drug on the list would be the farthest. Three separate ranked recommendation lists were generated using three different evaluation measures.
The first, and completely unbiased, ranking involved finding out the individual distances between each of the drugs in the processed dataset to the queried drug, and consequently storing the k nearest drugs. Mathematically, this process was similar to finding the Euclidean distance between vectors, and storing the k closest vectors, in sorted order, with respect to a single vector, represented by the queried drug. Each drug was represented by a vector of m numeric attributes. The formula for finding out the Euclidean dissimilarity between each of the suggested drugs and the queried drug is given in Eq (1).
UED(qd,rdi)=2√∑9j=1(qdj−rdij)2 | (1) |
UED(qd, rdi) stands for the uniform Euclidean distance between the vectors of the queried drug, qd,, and rdi, the ith suggested drug. Each of these vectors had nine numeric entries and qd1 to qd9 represents the numeric entries of the queried drug and subsequently rdi1 to rdi9 represents the numeric entries of the rdi drug.
The key characteristic of this evaluation measure was that each of the attributes was given equal weightage when distance/dissimilarity were calculated for each of the suggested drugs from the queried drug.
The second ranking used the same processed dataset to generate k nearest drugs and used the same Euclidean distance measure to find out the dissimilarity between each of the suggested drugs to the queried drugs. However, this method gave more weightage to a subset of the attributes while calculating the overall dissimilarity. These more important attributes (four in number) were the ones mentioned in Lipinsky's rule of five. Eq (2) shows the formula for calculating the dissimilarity, by incorporating the added weightage given to these more important attributes.
WED(qd,rdi)=Normalize(2√∑4j=1(29(qdpj−rdpij))2+2√∑5k=1(19(qdk−rdik))2) | (2) |
WED(qd, rdi) stands for the weighted Euclidean distance between the vectors of the queried drug, qd, , and rdi, the ith suggested drug. Each of these vectors had nine numeric entries, out of which four of them (qdp1, rdpi1 to qdp4, rdpi4) were given double the weightage, compared to the remaining five numeric attributes represented by qd1, rdi1 to qd5,rdi5. The total sum was then normalized for uniformity.
This weighted ranking approach gave more importance to the findings reported by researchers who had studied the pharmacokinetic properties of chemical drugs.
This paper introduces a new set of numeric attributes, which represents the network based characteristics of the chemical drugs. These network/graph based properties not only gave more insights into the chemical structures, by means of how the atoms were situated with respect to each other and how they were connected to each other, they also indicated the importance of an atom or a connection between two atoms to act as a bridge to connect to the remaining atoms of the network structure. This information, we believe, can be related with molecular bonding properties, which consequently determines the drug's ability to handle harmful bacterial antigens. The chosen network based attributes were ⅰ) average degree (DGA), ⅱ) average closeness (CLA), ⅲ) average node betweenness (NBA) and ⅳ) average edge betweenness (EBA). Eq (3) to Eq (6) shows the formula to calculate these values for a specific chemical drug structure.
DGA=(2∗E)/N | (3) |
CLA=(∑Ni=11∑Ny=1,y≠xid(y,xi))/N | (4) |
NBA=(∑s≠v≠tσ(v)stσst)/N | (5) |
EBA=(∑s≠e≠tλ(e)stλst)/N | (6) |
E stands for the total number of edges, and N stands for the total number of nodes in a network. In the case of CLA, d(y,xi) signifies the shortest distance between nodes y and xi. For NBA, σst represents the total number of shortest paths between the nodes s and t, while σ(v)st shows the ones among those which pass through the node y. Similarly, for EBA, λst generates the total number of shortest paths between the nodes s and t, while λ(e)st gives the ones which pass through the edge e.
This network based ranked recommendation list stored k nearest neighbours / drugs with respect to the queried drug, where the input was only the ids of the suggested drugs, and the four attributes (ⅰ to ⅳ). All these four numeric values were calculated from the graphical representations of the chemical drugs. The k nearest neighbours /drugs, in sorted order, with respect to the queried drug, with this customized dataset, were generated in the same manner as 3.2.1 using Eq (1) to calculate the dissimilarity.
The three different k-ranked recommendation lists were fed as input to the consolidated ranking system to generate a combined and consistent k-ranked recommendation list of drugs for a queried drug. The popular Borda ranking technique was initially chosen for this task, not only for its simple and effective way of ranking multiple ranked lists, but also for its unbiased way of giving weightage to a candidate even if it did not feature in the top k ranks in any of the ranked lists.
This paper proposes a customized version of Borda ranking to cater to the specific properties of the dataset. The ranking mechanism is explained in Table 2 as a demonstration. In the traditional Borda ranking system with three (A, B, C) evaluation measures, if C1, C2 and C3 were the chosen candidates, then the cells where C4 and C5 are placed (grey coloured cells), in the 3rd ranked position of evaluation measure B and C respectively, would be kept blank. This would have resulted in each of C1, C2 and C3 securing 6 points. However, the proposed modification looked at the absolute difference of ranking when lower ranked candidates were considered. In the case of Table 2, rank 4 and 5 (which were both lower than rank 3) resulted in Borda values of 0 and -1 respectively. Even though the proposed modification looked at lower ranks, the final result still looked at the top 3 ranks. The rankings generated by traditional Borda system and the rankings generated by our proposed modifications are highlighted in Table 2.
Evaluation Measures | Rank of Candidates | ||||
1 | 2 | 3 | 4 | 5 | |
A | C2 | C3 | C1 | C5 | C4 |
B | C1 | C2 | C4 | C3 | C5 |
C | C3 | C1 | C5 | C2 | C4 |
![]() |
|||||
Note: C1 to C5 are the candidates and A, B, C are the three evaluation measures, and the objective is to get the top three candidates. The upper table shows the ranks of the 5 candidates for each of the evaluation measures. The bottom left table shows the ranks of C1, C2, C3 by the traditional Borda ranking, while the bottom right table shows the same by our customized Borda ranking. The grey coloured cells represent locations where traditional Borda ranking would have kept blank, and would have given C3 and C2 1 point each, respectively. |
The consolidated ranked recommendation list provided k nearest neighbors/drugs with respect to the queried drugs by incorporating three kinds of evaluation measures. This paper specifically looked at one Tuberculosis (TB) drug in the query and consequently generated the k nearest recommended drugs. The queried TB drug, although being quite effective, was not perfect, as limited polymorphisms were reported by certain genes of a specific Mtb strain. This indicated that the effectiveness of the drug might reduce drastically in the future once the Mtb strain gradually becomes drug resistant to that specific drug. Therefore, an effective way to recommend other drugs could be to look for similar drugs, i.e., drugs that are high up in the ranked recommendation list, but not those drugs which are extremely similar, or identical, in terms of the attribute values. In this specific context, this paper proposes a unique refinement on the consolidated recommendation, by revisiting the network based recommendation list, and removing those topmost recommended drugs from the consolidated ranked recommendation list which had identical network structure based attribute values when compared with the queried drug. This meant that drugs which had almost identical chemical structure might not be an effective recommended drug in the long run, while top recommended drugs which are similar, but did not have identical network structures were viable suggestions.
The consolidated ranked k nearest neighbor recommendation list was thus refined by removing those drug entries which had identical attribute values with respect to the queried drug in the network based ranked recommendation list.
The proposed methodology is detailed by the steps given below.
Input: ID[n x c]
//A dataset "ID", with "n" number of tuples and "c" number of columns. Each tuple represents a drug along with its "c" properties. The dataset was provided by PubChem in response to a queried drug
Output: FR[fn x 2]
//A table "FR" having "fn" number of tuples and 2 columns, the first column being the unique numeric (cid) code of the drug and the second column representing the consolidated rank of the drug, 1 being the highest rank
Begin
Step 1: ID'[n x num] ← ID[n x c]
delete column i if i is non-numerical
// ID[n x c] is reduced to ID'[n x num] (num < c), where num represents the numeric attributes of the drug. For our experiment, num = 10, consisting of the cid and nine numeric attributes.
Step 2: D[n' x num] ← ID'[n x num]
delete row i if i contains entries which do not satisfy Table 1
// ID'[n x num] is reduced to D[n' x num] (n' < n), after tuples were removed because these tuples (drugs) did not satisfy the pharmacokinetic properties, as specified in Table 1.
Step 3a: A[tn, 3] ← D[n' x num]
for all i < - 1 to tn rows
A[i, 1] < - cid of ith drug
A[i, 2] < - UDS of ith drug
A[i, 3] < - UR of ith drug
//A[tn, 3] is acquired from D[n' x num], by calculating uniform weighted dissimilarity using Eq (1). The first column in A corresponds to the cid of the recommended drug, the second column gave the uniform dissimilarity score (UDS in Table 3) and the third column gave the dissimilarity rank (UR in Table 3). tn represented the top number of drugs that was asked for.
Step 3b: B[tn, 3] ← D[n' x num]
for all i < - 1 to tn rows
B[i, 1] < - cid of ith drug
B[i, 2] < - WDS of ith drug
B[i, 3] < - WR of ith drug
//B[tn, 3] is acquired from D[n' x num], by calculating pharmacokinetic weighted dissimilarity using Eq (2). The first column in B corresponds to the cid of the recommended drug, the second column gave the weighted dissimilarity score (WDS in Table 4) and the third column gave the dissimilarity rank (WR in Table 4). tn represented the top number of drugs asked for.
Step 3c: C[tn, 3] ← D[n' x 4]
for all i < - 1 to tn rows
C[i, 1] < - cid of ith drug
C[i, 2] < - NWDS of ith drug
C[i, 3] < - NWR of ith drug
//C[tn, 3] is acquired from D[n' x 4], by calculating four Network based dissimilarity features for each of the tuples. The four features (DGA, CLA, NBA, EBA) were calculated using Eq (3-6). The final dissimilarity of each of these four attributed vectors from the queried drug, cid 14052, was calculated using Eq (1). The first column in C corresponds to the cid of the recommended drug, the second column gave the Network based dissimilarity score (NWDS in Table 5) and the third column gave the dissimilarity rank (NWR in Table 5). tn represented the top number of drugs that was asked for.
Step 4: F[tn x 3]←Ensemble ranking (A[tn, 2], B[tn, 2], C[tn, 2]) by customised Borda
for all i < - 1 to tn rows
F[i, 1] < - cid of ith drug
F[i, 2] < - MBV of ith drug
F[i, 3] < - CR of ith drug
//F corresponds to a table which holds the ⅰ) cid, ⅱ) customised Borda Value (MBV in Table 6) and ⅲ)customised Borda rank (CR in Table 6) for tn number of tuples (drugs). The inputs were the datasets A, B and C, with tn entries and only two columns, the ⅰ) cid and the corresponding ⅱ) dissimilarity ranks (UR, WR and NWR respectively).
Step 5: FR[fn x 2] ← F[tn x 3]
j < -1
for all i < - 1 to tn rows
If (C[i, 2] not equal to 0)
FR[j, 1] < - cid of ith drug
FR[j, 2] < - RR of ith drug
j < - j+1
// FR represents the final recommendation table with fn number of entries and 2 columns. The columns being ⅰ) cid and ⅱ) final recommendation rank (RR in Table 6). The value fn ( <= tn) corresponds to the number of drugs which are still there after filtering is done on the table F by entries in table C. If any entry in the second column of C was zero, then that corresponding cid tuple entry is removed from the FR table.
End
The online PubChem database was used to gather the initial dataset for this effort. The dataset provided a list of chemical structures (and their details) that was similar to a queried chemical structure. The queried chemical structure was EMB (Ethambutol), an effective TB drug used for analysing drug resistant properties of H37Rv strain of Mycobacterium Tuberculosis, and this was gathered from VFDB and TBDRMD datasets. These datasets revealed that EMB was a drug of choice for many proteins on the H37Rv strain of Mtb as it resulted in either zero or extremely low number of polymorphisms. In response to the queried TB drug, EMB, PubChem generated a dataset having a dimension of 352 x 20, signifying 352 similar drugs/chemical structures, each having 20 attributes. The 20 attributes consisted of nine numeric attributes, namely ⅰ) molecular weight, ⅱ) polar area, ⅲ) complexity, ⅳ) log p, ⅴ) heavy count, ⅵ) hbond donor, ⅶ) hbond acceptor, ⅷ) rotatable bonds and ⅸ) annotation hit count. There were 13 non-numeric attributes like the cid, molecular formula, name of component, synonym of component, inchikey, meshheading etc. For our calculations, only the cid and the nine numeric attributes were kept and the remaining attributes were pruned as the first part of data pre-processing, which reduced the dataset to be 352 x 10. Thereafter, pharmacokinetic filtering was applied as the second part of data pre-processing, which involved with the values in six (ⅰ, ⅱ, ⅳ, ⅵ, ⅶ and ⅶ) of the numeric attributes. The filtering was done as per rules described in Table 1, and the final pre-processed dataset, D, came out with a dimension of 236 x 10. None of the entries generated from the query had any missing values or null values, hence, no pruning took place on these conditions.
The cleaned dataset D was first utilised to generate the uniform weighted ranked recommendation list. For the experiments conducted, we chose the top 15 (k = 15) chemical structures / drugs which were similar to the queried drug, EMB, having a cid of 14052. The uniform weighted ranked recommendation list is shown in Table 3. This was calculated using Eq. (1), where k-nearest neighbours/drugs of 14052 were listed with the dissimilarity values and the corresponding ranks, the 1st rank being the one with the least dissimilarity.
PubChem Sl No. | cid | mw | Polar area | complexity | xlogp | heavycnt | hbonddonor | hbondacc | rotbonds | annothitcnt | Dissimilarity Score (UDS) | Uniform Rank (UR) |
14052 | 204.31 | 64.5 | 109 | -0.1 | 14 | 4 | 4 | 9 | 15 | |||
1 | 3279 | 204.31 | 64.5 | 109 | 0 | 14 | 4 | 4 | 9 | 15 | 0.1 | 1 |
2 | 162045 | 204.31 | 64.5 | 123 | 0.4 | 14 | 4 | 4 | 9 | 0 | 20.5243758 | 6 |
3 | 465436 | 204.31 | 64.5 | 109 | 0 | 14 | 4 | 4 | 9 | 1 | 14.0003571 | 4 |
4 | 469919 | 188.31 | 35.5 | 105 | 0 | 13 | 2 | 3 | 8 | 1 | 36.2768521 | 13 |
5 | 469924 | 202.34 | 35.5 | 129 | 0 | 14 | 2 | 3 | 8 | 1 | 38.0380191 | 14 |
6 | 469926 | 218.34 | 64.5 | 120 | 0 | 15 | 4 | 4 | 10 | 1 | 22.7123513 | 7 |
7 | 469930 | 218.34 | 64.5 | 145 | 0 | 15 | 4 | 4 | 9 | 3 | 40.4703706 | 15 |
8 | 469973 | 219.32 | 81.8 | 147 | 0 | 15 | 4 | 5 | 9 | 1 | 46.5467518 | 16 |
9 | 469988 | 204.31 | 64.5 | 128 | 0 | 14 | 4 | 4 | 7 | 2 | 23.1086564 | 8 |
10 | 469989 | 232.36 | 64.5 | 149 | 0 | 16 | 4 | 4 | 9 | 1 | 50.8607167 | 18 |
11 | 469990 | 204.31 | 64.5 | 109 | 0 | 14 | 4 | 4 | 9 | 1 | 14.0003571 | 4 |
12 | 469991 | 232.36 | 64.5 | 145 | 0 | 16 | 4 | 4 | 9 | 3 | 47.2314779 | 17 |
13 | 469996 | 204.31 | 44.7 | 123 | 0 | 14 | 2 | 4 | 9 | 0 | 28.5840865 | 9 |
14 | 470016 | 192.3 | 45.3 | 96.5 | 0 | 13 | 4 | 4 | 7 | 0 | 29.9856649 | 11 |
15 | 470071 | 204.31 | 64.5 | 109 | 0 | 14 | 4 | 4 | 9 | 6 | 9.00055554 | 2 |
16 | 470072 | 204.31 | 64.5 | 109 | 0 | 14 | 4 | 4 | 9 | 6 | 9.00055554 | 2 |
17 | 470075 | 188.31 | 44.3 | 109 | 0 | 13 | 3 | 3 | 8 | 1 | 29.3947274 | 10 |
18 | 470076 | 188.31 | 44.3 | 121 | 0 | 13 | 3 | 3 | 7 | 1 | 31.7970124 | 12 |
The dataset D was again used to generate the pharmacokinetic weighted ranked recommendation list, where the top k ( = 15) nearest neighbours/drugs of 14052 were listed (in Table 4) with the dissimilarity values and the corresponding ranks, the 1st rank being the one with the least dissimilarity. The dissimilarity was calculated using Eq (2), by a Euclidean distance measure, where more weightage was given to four of the attributes (mw, xlogp, hbond donor count, hbond acceptor count).
PubChem Sl No. | cid | mw | Polar area | complexity | xlogp | heavycnt | hbonddonor | hbondacc | rotbonds | annothitcnt | Weighted Dissimilarity Score (WDS) | Weighted Rank (WR) |
14052 | 204.31 | 64.5 | 109 | -0.1 | 14 | 4 | 4 | 9 | 15 | |||
1 | 3279 | 204.31 | 64.5 | 109 | 0 | 14 | 4 | 4 | 9 | 15 | 0.015385 | 1 |
2 | 162045 | 204.31 | 64.5 | 123 | 0.4 | 14 | 4 | 4 | 9 | 0 | 1.580203 | 6 |
3 | 465436 | 204.31 | 64.5 | 109 | 0 | 14 | 4 | 4 | 9 | 1 | 1.077033 | 4 |
4 | 469919 | 188.31 | 35.5 | 105 | 0 | 13 | 2 | 3 | 8 | 1 | 3.524252 | 14 |
5 | 469924 | 202.34 | 35.5 | 129 | 0 | 14 | 2 | 3 | 8 | 1 | 2.952848 | 11 |
6 | 469926 | 218.34 | 64.5 | 120 | 0 | 15 | 4 | 4 | 10 | 1 | 2.558665 | 9 |
7 | 469930 | 218.34 | 64.5 | 145 | 0 | 15 | 4 | 4 | 9 | 3 | 3.631228 | 15 |
8 | 469973 | 219.32 | 81.8 | 147 | 0 | 15 | 4 | 5 | 9 | 1 | 4.103347 | 16 |
9 | 469988 | 204.31 | 64.5 | 128 | 0 | 14 | 4 | 4 | 7 | 2 | 1.777639 | 7 |
10 | 469989 | 232.36 | 64.5 | 149 | 0 | 16 | 4 | 4 | 9 | 1 | 5.410515 | 18 |
11 | 469990 | 204.31 | 64.5 | 109 | 0 | 14 | 4 | 4 | 9 | 1 | 1.077033 | 4 |
12 | 469991 | 232.36 | 64.5 | 145 | 0 | 16 | 4 | 4 | 9 | 3 | 5.212213 | 17 |
13 | 469996 | 204.31 | 44.7 | 123 | 0 | 14 | 2 | 4 | 9 | 0 | 2.214904 | 8 |
14 | 470016 | 192.3 | 45.3 | 96.5 | 0 | 13 | 4 | 4 | 7 | 0 | 2.807313 | 10 |
15 | 470071 | 204.31 | 64.5 | 109 | 0 | 14 | 4 | 4 | 9 | 6 | 0.692479 | 2 |
16 | 470072 | 204.31 | 64.5 | 109 | 0 | 14 | 4 | 4 | 9 | 6 | 0.692479 | 2 |
17 | 470075 | 188.31 | 44.3 | 109 | 0 | 13 | 3 | 3 | 8 | 1 | 3.113323 | 12 |
18 | 470076 | 188.31 | 44.3 | 121 | 0 | 13 | 3 | 3 | 7 | 1 | 3.250016 | 13 |
The dataset D had a list of drugs (cids) which were similar to EMB (14052) as per PubChem query. PubChem also provided 2-dimensional chemical structures of these cids. This paper, as a novelty, looked into four network based properties (average degree, average closeness, average node betweenness and average edge betweenness) of each of these chemical structures and found out how each of them were dissimilar from the chemical structural properties of popular TB drug EMB. The dissimilarity was calculated by the Euclidean distance measure and consequently the network weighted ranked recommendation list was generated for the k ( = 15) nearest neighbours/drugs as shown in Table 5.
Pub Rank | cid | Avg degree | Avg closeness | Avg Nbetween | Avg Ebetween | N/w Weighted Dissimilarity Score (NWDS) | N/w Weighted Rank (NWR) |
14052 | 1.833 | 0.030437 | 11.667 | 18.7272 | |||
1 | 3279 | 1.8571 | 0.025008 | 14.2857 | 22.3846 | 4.49830799 | 9 |
2 | 162045 | 1.833 | 0.030437 | 11.667 | 18.7272 | 0 | 1 |
3 | 465436 | 1.8461 | 0.02755192 | 12.923 | 20.5 | 2.1726794 | 6 |
4 | 469919 | 1.7778 | 0.0511514 | 6.222 | 11.5 | 9.04897348 | 13 |
5 | 469924 | 1.8182 | 0.0358856 | 9.5454 | 16 | 3.45529378 | 7 |
6 | 469926 | 1.8571 | 0.025008 | 14.2857 | 22.3846 | 4.49830799 | 9 |
7 | 469930 | 1.875 | 0.0194469 | 19.1875 | 28.4667 | 12.3051886 | 15 |
8 | 469973 | 1.875 | 0.020659 | 17.6875 | 28.8667 | 11.7922746 | 14 |
9 | 469988 | 1.8889 | 0.016112 | 23.7778 | 34.1765 | 19.6304783 | 17 |
10 | 469989 | 1.8889 | 0.016112 | 23.7778 | 34.1765 | 19.6304783 | 17 |
11 | 469990 | 1.8571 | 0.025008 | 14.2857 | 22.3846 | 4.49830799 | 9 |
12 | 469991 | 1.8889 | 0.0167056 | 22.5556 | 32.8823 | 17.8586612 | 16 |
13 | 469996 | 1.8182 | 0.037331 | 9 | 15.4 | 4.26420161 | 8 |
14 | 470016 | 1.7333 | 0.011509 | 8.6 | 15.2307 | 4.6521285 | 12 |
15 | 470071 | 1.833 | 0.030437 | 11.667 | 18.7272 | 0 | 1 |
16 | 470072 | 1.833 | 0.030437 | 11.667 | 18.7272 | 0 | 1 |
17 | 470075 | 1.846 | 0.028601 | 12.1538 | 19.6667 | 1.05820927 | 4 |
18 | 470076 | 1.8333 | 0.031711 | 10.9167 | 17.9091 | 1.1100628 | 5 |
After generating the three different ranked recommendation lists (Table 3, 4 and 5), a consolidated ranked list was prepared by using a modified Borda ranking technique. The unique modification to the traditional Borda technique is explained in Section 3.3. For our experiment, we chose the top 15 drugs/chemical structures for the consolidated list, thus rank 16, 17 and 18 had a value of 0, -1 and -2 respectively. The 'Consolidated Rank' column of Table 6 shows the result of this modified Borda ranking.
Sl. No. | cid | Uniform Rank (UR) | Weighted Rank (WR) | N/w Rank (NWR) | Modified Borda Value(MBV) | Consolidated Rank (CR) | Refined Recommendation (RR) |
1 | 3279 | 1 | 1 | 9 | 37 | 3 | 1 |
2 | 162045 | 6 | 6 | 1 | 35 | 4 | |
3 | 465436 | 4 | 4 | 6 | 34 | 5 | 2 |
4 | 469919 | 13 | 14 | 13 | 8 | 14 | 11 |
5 | 469924 | 14 | 11 | 7 | 16 | 11 | 8 |
6 | 469926 | 7 | 9 | 9 | 23 | 7 | 4 |
7 | 469930 | 15 | 15 | 15 | 3 | 15 | 12 |
8 | 469973 | 16 | 16 | 14 | 2 | 16 | 13 |
9 | 469988 | 8 | 7 | 17 | 16 | 12 | 9 |
10 | 469989 | 18 | 18 | 17 | -5 | 18 | 15 |
11 | 469990 | 4 | 4 | 9 | 31 | 6 | 3 |
12 | 469991 | 17 | 17 | 16 | -2 | 17 | 14 |
13 | 469996 | 9 | 8 | 8 | 23 | 8 | 5 |
14 | 470016 | 11 | 10 | 12 | 15 | 13 | 10 |
15 | 470071 | 2 | 2 | 1 | 43 | 1 | |
16 | 470072 | 2 | 2 | 1 | 43 | 2 | |
17 | 470075 | 10 | 12 | 4 | 22 | 9 | 6 |
18 | 470076 | 12 | 13 | 5 | 18 | 10 | 7 |
Note: "Consolidated Rank" column ranked the drugs as per the customized Borda ranking, and "Refined Recommendation" provided the final recommendation ranks after revisiting Network Based dissimilarities. The grey coloured entries showed the ranks of the cids which were below the rank of 15. Our proposed modification to the Borda ranking incorporated these ranks also. The violet entries signified drugs which had identical network based attribute properties to the queried drug, EMB with cid 14092. |
The final list of suggested drugs with their corresponding ranks is listed in the "Refined Recommendation" column of Table 6. According to our proposed recommendation mechanism, the chemical compound Myambutol, having cid 3279, was top ranked among the suggested chemical structures, which could be studied as an alternative to the queried drug, EMB, with cid 14052. The network based properties of both 14052 and 3279 are shown in Figure 2 and Figure 3 respectively. The circles represent atoms and the edges represent the connections between the atoms. The Nitrogen, Hydrogen and Oxygen atoms are represented as N, H and O respectively, followed by a serial number, while the other atoms are represented by a serial number only. The size of a circle is proportional to the value for that atom for a particular attribute, signifying that a bigger value of the specific attribute resulted in a bigger sized circle.
It is evident from Table 6 that three chemical compounds, namely 162045, 470071 and 470072, were omitted from the final recommendation list ('Refined Recommendation' column). This was due to the fact that these three chemical compounds had no difference with EMB, and consequently held the 1st recommendation rank jointly, based on the chosen network based properties, as shown in Table 5. The motivation for their omission in the Refined Recommendation was to give importance to similar drugs/chemical structures, but not to those which had almost identical chemical structures. The reason was inferred from the fact that although EMB was an effective drug against the H37Rv strain of Mtb due to the very low number of polymorphisms, the complex drug resistance properties of Mtb [1,2] would eventually make an existing drug, like EMB, to be ineffective in due course of time. Hence, finding an alternative drug with almost identical chemical structure as the current one would also be ineffective. However, a recommended drug which had minor changes in the chemical structure, when compared to an existing effective drug, could be effective in dealing with a mutated strain of Mtb. This drug recommendation, needless to say, should also adhere to the pharmacokinetic limitations or boundaries. These boundaries might have limited the search space for candidate drugs / compounds and consequently could have restricted the degree of effectiveness. However, adhering to the boundaries would ensure that the bioavailability of the candidates would not be compromised. This could be significant for dealing with lethal bacteria like Mtb.
The literature revealed that several promising studies were conducted [27,29,31,33] which focused on the chemical/drug from the structural network point of view only and consequently came up with new drugs or existing drugs which could be repurposed. On the other hand, the recommended drug had to satisfy basic pharmacokinetic properties for effective utilization by the human body. In this context, this study attempted to provide a balanced approach by giving due weightage to both these aspects by generating two additional recommendation lists, one with emphasis on network centric similarities and the other with emphasis on pharmacokinetic related similarities. Consequently, the customized ensemble ranking was aimed at providing an elegant way to come up with a consolidated ranking which took into account all these aspects.
Interestingly, the effect of the network structure for some of the drugs, shown in Table 6, was significant in their corresponding consolidated ranking. The drug with cid no 469924 had lower ranking (WR = 14) in pharmacokinetic properties, but had higher ranking (NWR = 7) in network properties. This resulted in the compound to get a somewhat higher ranking (RR = 8) in the consolidated ranking. Similar findings can be observed for cid 470075 (WR = 12, NWR = 4, RR = 6) and cid 470076 (WR = 13, NWR = 5, RR = 7).
The final list of suggested drugs (from the last column in Table 6) had 15 entries, even though Table 3, Table 4 and table 5 showed 18 entries. These 3 additional entries were utilised in our modified Borda ranking technique. These additional entries were also helpful because after the final refinement of recommendation, where three chemical structures were omitted, it was still able to suggest 15 chemical structures at the end.
There were other experimental studies which reported the use of compounds in dealing with tuberculosis which coincided with the results generated by the computational approach of this paper. The compound with cid 469926 was applied in an antituberculosis and antimicrobial experimental study [39]. The cid 469990 was useful in an experimental study on the cell wall core of Mtb [40]. Additionally, looking at the repurposing effectiveness of the generated results, the same cid 469990 was also useful in an experimental study which dealt with human cancer cell lines [41].
The effort was aimed at generating a list of possible TB drugs by using an ensemble of ranking mechanisms in which one of the ranking mechanisms included a novel way of looking at the chemical structures of the drugs and evaluating them based on network based attributes, and another ranking mechanism stressed on pharmacokinetic compatibility. The effort also proposed unique modifications on a popular ensemble ranking technique to suit our specific requirement. The results dealt with providing established chemical structures along with their ranks, which could possibly be used as alternatives to EMB, a popular drug used for H37Rv strain of TB. It is believed that the incorporation of the pharmacokinetic properties as well as network based properties in the ensemble ranking mechanism would make the proposed recommendations logical, practical and effective.
The proposed methodology can be applied not only for TB drugs, but to any other drugs, provided they are recorded in the online PubChem database. From the pharmacokinetic point of view, more studies can be done to verify that the recommendation does not produce an unwanted inhibitory or toxic effect on the biological system. On the other hand, while evaluating network based properties of the chemical structures, four popular properties were looked at. One can also look at more network properties as well as graph isomorphism properties to find out the level of similarity/dissimilarity among chemical structures of drugs.
The authors take this opportunity to thank the management of VIT for providing the facilities and encouragement to carry out this work.
The authors have declared that no conflicts of interest exist.
[1] |
Z. Zhang, X. Liu, D. Zhou, C. Lin, J. Chen, H. Wang, Finite-time stabilizability and instabilizability for complex-valued memristive neural networks with time delays, IEEE Trans. Syst. Man Cybern. Syst., 48 (2017), 2371–2382. https://doi.org/10.1109/TSMC.2017.2754508 doi: 10.1109/TSMC.2017.2754508
![]() |
[2] |
A. Wu, Z. Zeng, Exponential stabilization of memristive neural networks with time delays, IEEE Trans. Neural Networks Learn. Syst., 23 (2012), 1919–1929. https://doi.org/10.1109/TNNLS.2012.2219554 doi: 10.1109/TNNLS.2012.2219554
![]() |
[3] |
R. Li, X. Gao, J. Cao, Quasi-state estimation and quasi-synchronization control of quaternion-valued fractional-order fuzzy memristive neural networks: Vector ordering approach, Appl. Math. Comput., 362 (2019), 124572. https://doi.org/10.1016/j.amc.2019.124572 doi: 10.1016/j.amc.2019.124572
![]() |
[4] |
J. Zhang, X. Ma, Y. Li, Q. Gan, C. Wang, Synchronization in fixed/preassigned-time of delayed fully quaternion-valued memristive neural networks via non-separation method, Commun. Nonlinear Sci. Numer. Simul., 113 (2022), 106581. https://doi.org/10.1016/j.cnsns.2022.106581 doi: 10.1016/j.cnsns.2022.106581
![]() |
[5] |
N. Yang, Y. Yu, S. Zhong, X. Wang, K. Shi, J. Cai, Exponential synchronization of stochastic delayed memristive neural networks via a novel hybrid control, Neural Networks, 131 (2020), 242–250. https://doi.org/10.1016/j.neunet.2020.07.034 doi: 10.1016/j.neunet.2020.07.034
![]() |
[6] | Z. Yan, X. Huang, J. Cao, Variable-sampling-period dependent global stabilization of delayed memristive neural networks based on refined switching event-triggered control, Sci. China Inf. Sci., 63 (2020), 212201. |
[7] | X. Wu, S. Liu, H. Wang, Asymptotic stability and synchronization of fractional delayed memristive neural networks with algebraic constraints, Commun. Nonlinear Sci. Numer. Simul., 114 (2022), 106694. |
[8] |
Z. Yan, X. Huang, Y. Fan, J. Xia, H. Shen, Threshold-function-dependent quasi-synchronization of delayed memristive neural networks via hybrid event-triggered control, IEEE Trans. Syst. Man Cybern. Syst., 51 (2021), 6712–6722. https://doi.org/10.1109/TSMC.2020.2964605 doi: 10.1109/TSMC.2020.2964605
![]() |
[9] | S. Murugesan, Y. C. Liu, Finite-time resilient control for networked control systems with multiple cyber-attacks: Memory/adaptive event-triggered scheme, Int. J. Adapt. Control Signal Process., 36 (2022), 901–925. |
[10] |
W. Tai, D. Gao, A. Zhao, J. Zhou, X. Wang, Weight learning for H∞ stabilization of uncertain switched neural networks with external disturbance and reaction-diffusion, Int. J. Adapt. Control Signal Process., 37 (2023), 1015–1029. https://doi.org/10.1002/acs.3558 doi: 10.1002/acs.3558
![]() |
[11] |
M. Sathishkumar, R. Sakthivel, F. Alzahrani, B. Kaviarasan, Y. Ren, Mixed H∞ and passivity-based resilient controller for nonhomogeneous Markov jump systems, Nonlinear Anal. Hybrid Syst., 31 (2019), 86–99. https://doi.org/10.1016/j.mattod.2019.06.003 doi: 10.1016/j.mattod.2019.06.003
![]() |
[12] |
J. Zhou, J. Dong, S. Xu, Asynchronous dissipative control of discrete-time fuzzy Markov jump systems with dynamic state and input quantization, IEEE Trans. Fuzzy Syst., 2023 (2023), forthcoming. https://doi.org/10.1109/TFUZZ.2023.3271348 doi: 10.1109/TFUZZ.2023.3271348
![]() |
[13] |
X. Meng, G. Zhang, Q. Zhang, Robust adaptive neural network integrated fault-tolerant control for underactuated surface vessels with finite-time convergence and event-triggered inputs, Math. Biosci. Eng., 20 (2022), 2131–2156. https://doi.org/10.3934/mbe.2023099 doi: 10.3934/mbe.2023099
![]() |
[14] |
R. Saravanakumar, Y. Hoon Joo, Network-based robust exponential fuzzy control for uncertain systems, Math. Methods Appl. Sci., 2023 (2023), forthcoming. https://doi.org/10.1002/mma.8943 doi: 10.1002/mma.8943
![]() |
[15] |
Y. Cao, N. Liu, C. Zhang, T. Zhang, Z.-F. Luo, Synchronization of multiple reaction–diffusion memristive neural networks with known or unknown parameters and switching topologies, Knowl. Based Syst., 254 (2022), 109595. https://doi.org/10.1016/j.knosys.2022.109595 doi: 10.1016/j.knosys.2022.109595
![]() |
[16] | I. Ghous, J. Lu, Z. Duan, H∞ stabilization problem for memristive neural networks with time-varying delays, Inf. Sci., 607 (2022), 27–43. |
[17] |
Z. Yan, D. Zuo, T. Guo, J. Zhou, Quantized H∞ stabilization for delayed memristive neural networks, Neural Comput. Appl., 2023 (2023), forthcoming. https://doi.org/10.1007/s00521-023-08510-3 doi: 10.1007/s00521-023-08510-3
![]() |
[18] |
W. Tai, X. Li, J. Zhou, S. Arik, Asynchronous dissipative stabilization for stochastic Markov-switching neural networks with completely-and incompletely-known transition rates, Neural Networks, 161 (2023), 55–64. https://doi.org/10.1016/j.neunet.2023.01.039 doi: 10.1016/j.neunet.2023.01.039
![]() |
[19] |
C. Deng, D. Zhang, G. Feng, Resilient practical cooperative output regulation for mass with unknown switching exosystem dynamics under DoS attacks, Automatica, 139 (2022), 110172. https://doi.org/10.1016/j.automatica.2022.110172 doi: 10.1016/j.automatica.2022.110172
![]() |
[20] | S. Dong, L. Liu, G. Feng, M. Liu, Z. G. Wu, R. Zheng, Cooperative output regulation quadratic control for discrete-time heterogeneous multiagent Markov jump systems, IEEE Trans. Cybern., 52 (2022), 9882–9892. |
[21] | J. Gu, H. Wang, W. Li, Output-feedback stabilization for stochastic nonlinear systems with Markovian switching and time-varying powers, Math. Biosci. Eng., 19 (2022), 11 071–11 085. |
[22] |
T. Yu, L. Liu, Y. J. Liu, Observer-based adaptive fuzzy output feedback control for functional constraint systems with dead-zone input, Math. Biosci. Eng., 20 (2023), 2628–2650. https://doi.org/10.3934/mbe.2023123 doi: 10.3934/mbe.2023123
![]() |
[23] | Z. Yan, C. Sang, M. Fang, J. Zhou, Energy-to-peak consensus for multi-agent systems with stochastic disturbances and Markovian switching topologies, Trans. Inst. Measure. Control, 40 (2018), 4358–4368. |
[24] |
J. Zhou, D. Xu, W. Tai, C. K. Ahn, Switched event-triggered H∞ security control for networked systems vulnerable to aperiodic DoS attacks, IEEE Trans. Network Sci. Eng., 2023 (2023), forthcoming. https://doi.org/10.1109/TNSE.2023.3243095 doi: 10.1109/TNSE.2023.3243095
![]() |
[25] |
Y. Liu, J. Li, F. Fang, J. H. Park, Further studies on sampled-data consensus of multi-agent systems with communication delays, IEEE Trans. Signal Inf. Process. Networks, 8 (2022), 920–931. https://doi.org/10.1109/TSIPN.2022.3220153 doi: 10.1109/TSIPN.2022.3220153
![]() |
[26] |
E. Arslan, G. Narayanan, M. S. Ali, S. Arik, S. Saroha, Controller design for finite-time and fixed-time stabilization of fractional-order memristive complex-valued bam neural networks with uncertain parameters and time-varying delays, Neural Networks, 130 (2020), 60–74. https://doi.org/10.1016/j.neunet.2020.06.021 doi: 10.1016/j.neunet.2020.06.021
![]() |
[27] |
R. Saravanakumar, G. Rajchakit, M. S. Ali, Z. Xiang, Y. H. Joo, Robust extended dissipativity criteria for discrete-time uncertain neural networks with time-varying delays, Neural Comput. Appl., 30 (2018), 3893–3904. https://doi.org/10.1007/s00521-017-2974-z doi: 10.1007/s00521-017-2974-z
![]() |
[28] |
L. He, W. Wu, J. Zhou, G. Yao, Input-to-state stable synchronization for delayed Lurie systems via sampled-data control, Discrete Contin. Dyn. Syst., 28 (2023), 1553–1570. https://doi.org/10.3934/dcdsb.2022135 doi: 10.3934/dcdsb.2022135
![]() |
[29] | X. Huang, J. Jia, Y. Fan, Z. Wang, J. Xia, Interval matrix method based synchronization criteria for fractional-order memristive neural networks with multiple time-varying delays, J. Franklin Inst., 357 (2020), 1707–1733. |
[30] |
L. Yao, Z. Wang, X. Huang, Y. Li, Q. Ma, H. Shen, Stochastic sampled-data exponential synchronization of Markovian jump neural networks with time-varying delays, IEEE Trans. Neural Networks Learn. Syst., 34 (2023), 909–920. https://doi.org/10.1109/TNNLS.2021.3103958 doi: 10.1109/TNNLS.2021.3103958
![]() |
[31] |
R. Vadivel, P. Hammachukiattikul, N. Gunasekaran, R. Saravanakumar, H. Dutta, Strict dissipativity synchronization for delayed static neural networks: An event-triggered scheme, Chaos Solitons Fractals, 150 (2021), 111212. https://doi.org/10.1016/j.chaos.2021.111212 doi: 10.1016/j.chaos.2021.111212
![]() |
[32] | Y. Ni, Z. Wang, Y. Fan, X. Huang, H. Shen, Memory-based event-triggered control for global synchronization of chaotic Lur'e systems and its application, IEEE Trans. Syst. Man Cybern. Syst., 53 (2023), 1920–1931. |
[33] |
G. Zhang, Z. Zeng, D. Ning, Novel results on synchronization for a class of switched inertial neural networks with distributed delays, Inf. Sci., 511 (2020), 114–126. https://doi.org/10.1016/j.ins.2019.09.048 doi: 10.1016/j.ins.2019.09.048
![]() |
[34] |
Z. Wang, S. Ding, Z. Huang, H. Zhang, Exponential stability and stabilization of delayed memristive neural networks based on quadratic convex combination method, IEEE Trans. Neural Networks Learn. Syst., 27 (2015), 2337–2350. https://doi.org/10.1109/TNNLS.2015.2485259 doi: 10.1109/TNNLS.2015.2485259
![]() |
[35] |
X. Yang, D. W. Ho, Synchronization of delayed memristive neural networks: robust analysis approach, IEEE Trans. Cybern., 46 (2015), 3377–3387. https://doi.org/10.1109/TCYB.2015.2505903 doi: 10.1109/TCYB.2015.2505903
![]() |
[36] |
A. Seuret, F. Gouaisbaut, Hierarchy of LMI conditions for the stability analysis of time-delay systems, Syst. Control Lett., 81 (2015), 1–7. https://doi.org/10.1016/j.sysconle.2015.03.007 doi: 10.1016/j.sysconle.2015.03.007
![]() |
[37] |
P. Park, J. W. Ko, C. Jeong, Reciprocally convex approach to stability of systems with time-varying delays, Automatica, 47 (2011), 235–238. https://doi.org/10.1016/j.automatica.2010.10.014 doi: 10.1016/j.automatica.2010.10.014
![]() |
[38] |
S. Xu, J. Lam, A survey of linear matrix inequality techniques in stability analysis of delay systems, Int. J. Syst. Sci., 39 (2008), 1095–1113. https://doi.org/10.1080/00207720802300370 doi: 10.1080/00207720802300370
![]() |
[39] | S. Boyd, L. El Ghaoui, E. Feron, V. Balakrishnan, Linear Matrix Inequalities in System and Control Theory, SIAM, 1994. |
[40] |
J. Zhou, J. H. Park, Q. Ma, Non-fragile observer-based H∞ control for stochastic time-delay systems, Appl. Math. Comput., 291 (2016), 69–83. https://doi.org/10.1016/j.amc.2016.06.024 doi: 10.1016/j.amc.2016.06.024
![]() |
[41] |
M. Solak, O. Faydasicok, S. Arik, A general framework for robust stability analysis of neural networks with discrete time delays, Neural Networks, 162 (2023), 186–198. https://doi.org/10.1016/j.neunet.2023.02.040 doi: 10.1016/j.neunet.2023.02.040
![]() |
1. | Sasha E. Larsen, Jesse H. Erasmus, Valerie A. Reese, Tiffany Pecor, Jacob Archer, Amit Kandahar, Fan-Chi Hsu, Katrina Nicholes, Steven G. Reed, Susan L. Baldwin, Rhea N. Coler, An RNA-Based Vaccine Platform for Use against Mycobacterium tuberculosis, 2023, 11, 2076-393X, 130, 10.3390/vaccines11010130 | |
2. | Junli Li, Dong Liu, Xiaochi Li, Jiazheng Wei, Weixin Du, Aihua Zhao, Miao Xu, RNA vaccines: The dawn of a new age for tuberculosis?, 2025, 21, 2164-5515, 10.1080/21645515.2025.2469333 |
Attribute Constraints | Filter Rule |
hbond donor count <=5 | Lipinski's Rule |
hbond receptor count <=10 | Lipinski's Rule |
180 <= Molecular weight <=480 | Lipinski's Rule and Ghose Filter (combined) |
-0.4 <= logP < 5 | Lipinski's Rule and Ghose Filter (combined) |
20 <=Total number of atoms <=70 | Ghose Filter |
Polar surface area <=140 | Verber's Rule |
Number of rotatable bonds <=10 | Verber's Rule |
Evaluation Measures | Rank of Candidates | ||||
1 | 2 | 3 | 4 | 5 | |
A | C2 | C3 | C1 | C5 | C4 |
B | C1 | C2 | C4 | C3 | C5 |
C | C3 | C1 | C5 | C2 | C4 |
![]() |
|||||
Note: C1 to C5 are the candidates and A, B, C are the three evaluation measures, and the objective is to get the top three candidates. The upper table shows the ranks of the 5 candidates for each of the evaluation measures. The bottom left table shows the ranks of C1, C2, C3 by the traditional Borda ranking, while the bottom right table shows the same by our customized Borda ranking. The grey coloured cells represent locations where traditional Borda ranking would have kept blank, and would have given C3 and C2 1 point each, respectively. |
PubChem Sl No. | cid | mw | Polar area | complexity | xlogp | heavycnt | hbonddonor | hbondacc | rotbonds | annothitcnt | Dissimilarity Score (UDS) | Uniform Rank (UR) |
14052 | 204.31 | 64.5 | 109 | -0.1 | 14 | 4 | 4 | 9 | 15 | |||
1 | 3279 | 204.31 | 64.5 | 109 | 0 | 14 | 4 | 4 | 9 | 15 | 0.1 | 1 |
2 | 162045 | 204.31 | 64.5 | 123 | 0.4 | 14 | 4 | 4 | 9 | 0 | 20.5243758 | 6 |
3 | 465436 | 204.31 | 64.5 | 109 | 0 | 14 | 4 | 4 | 9 | 1 | 14.0003571 | 4 |
4 | 469919 | 188.31 | 35.5 | 105 | 0 | 13 | 2 | 3 | 8 | 1 | 36.2768521 | 13 |
5 | 469924 | 202.34 | 35.5 | 129 | 0 | 14 | 2 | 3 | 8 | 1 | 38.0380191 | 14 |
6 | 469926 | 218.34 | 64.5 | 120 | 0 | 15 | 4 | 4 | 10 | 1 | 22.7123513 | 7 |
7 | 469930 | 218.34 | 64.5 | 145 | 0 | 15 | 4 | 4 | 9 | 3 | 40.4703706 | 15 |
8 | 469973 | 219.32 | 81.8 | 147 | 0 | 15 | 4 | 5 | 9 | 1 | 46.5467518 | 16 |
9 | 469988 | 204.31 | 64.5 | 128 | 0 | 14 | 4 | 4 | 7 | 2 | 23.1086564 | 8 |
10 | 469989 | 232.36 | 64.5 | 149 | 0 | 16 | 4 | 4 | 9 | 1 | 50.8607167 | 18 |
11 | 469990 | 204.31 | 64.5 | 109 | 0 | 14 | 4 | 4 | 9 | 1 | 14.0003571 | 4 |
12 | 469991 | 232.36 | 64.5 | 145 | 0 | 16 | 4 | 4 | 9 | 3 | 47.2314779 | 17 |
13 | 469996 | 204.31 | 44.7 | 123 | 0 | 14 | 2 | 4 | 9 | 0 | 28.5840865 | 9 |
14 | 470016 | 192.3 | 45.3 | 96.5 | 0 | 13 | 4 | 4 | 7 | 0 | 29.9856649 | 11 |
15 | 470071 | 204.31 | 64.5 | 109 | 0 | 14 | 4 | 4 | 9 | 6 | 9.00055554 | 2 |
16 | 470072 | 204.31 | 64.5 | 109 | 0 | 14 | 4 | 4 | 9 | 6 | 9.00055554 | 2 |
17 | 470075 | 188.31 | 44.3 | 109 | 0 | 13 | 3 | 3 | 8 | 1 | 29.3947274 | 10 |
18 | 470076 | 188.31 | 44.3 | 121 | 0 | 13 | 3 | 3 | 7 | 1 | 31.7970124 | 12 |
PubChem Sl No. | cid | mw | Polar area | complexity | xlogp | heavycnt | hbonddonor | hbondacc | rotbonds | annothitcnt | Weighted Dissimilarity Score (WDS) | Weighted Rank (WR) |
14052 | 204.31 | 64.5 | 109 | -0.1 | 14 | 4 | 4 | 9 | 15 | |||
1 | 3279 | 204.31 | 64.5 | 109 | 0 | 14 | 4 | 4 | 9 | 15 | 0.015385 | 1 |
2 | 162045 | 204.31 | 64.5 | 123 | 0.4 | 14 | 4 | 4 | 9 | 0 | 1.580203 | 6 |
3 | 465436 | 204.31 | 64.5 | 109 | 0 | 14 | 4 | 4 | 9 | 1 | 1.077033 | 4 |
4 | 469919 | 188.31 | 35.5 | 105 | 0 | 13 | 2 | 3 | 8 | 1 | 3.524252 | 14 |
5 | 469924 | 202.34 | 35.5 | 129 | 0 | 14 | 2 | 3 | 8 | 1 | 2.952848 | 11 |
6 | 469926 | 218.34 | 64.5 | 120 | 0 | 15 | 4 | 4 | 10 | 1 | 2.558665 | 9 |
7 | 469930 | 218.34 | 64.5 | 145 | 0 | 15 | 4 | 4 | 9 | 3 | 3.631228 | 15 |
8 | 469973 | 219.32 | 81.8 | 147 | 0 | 15 | 4 | 5 | 9 | 1 | 4.103347 | 16 |
9 | 469988 | 204.31 | 64.5 | 128 | 0 | 14 | 4 | 4 | 7 | 2 | 1.777639 | 7 |
10 | 469989 | 232.36 | 64.5 | 149 | 0 | 16 | 4 | 4 | 9 | 1 | 5.410515 | 18 |
11 | 469990 | 204.31 | 64.5 | 109 | 0 | 14 | 4 | 4 | 9 | 1 | 1.077033 | 4 |
12 | 469991 | 232.36 | 64.5 | 145 | 0 | 16 | 4 | 4 | 9 | 3 | 5.212213 | 17 |
13 | 469996 | 204.31 | 44.7 | 123 | 0 | 14 | 2 | 4 | 9 | 0 | 2.214904 | 8 |
14 | 470016 | 192.3 | 45.3 | 96.5 | 0 | 13 | 4 | 4 | 7 | 0 | 2.807313 | 10 |
15 | 470071 | 204.31 | 64.5 | 109 | 0 | 14 | 4 | 4 | 9 | 6 | 0.692479 | 2 |
16 | 470072 | 204.31 | 64.5 | 109 | 0 | 14 | 4 | 4 | 9 | 6 | 0.692479 | 2 |
17 | 470075 | 188.31 | 44.3 | 109 | 0 | 13 | 3 | 3 | 8 | 1 | 3.113323 | 12 |
18 | 470076 | 188.31 | 44.3 | 121 | 0 | 13 | 3 | 3 | 7 | 1 | 3.250016 | 13 |
Pub Rank | cid | Avg degree | Avg closeness | Avg Nbetween | Avg Ebetween | N/w Weighted Dissimilarity Score (NWDS) | N/w Weighted Rank (NWR) |
14052 | 1.833 | 0.030437 | 11.667 | 18.7272 | |||
1 | 3279 | 1.8571 | 0.025008 | 14.2857 | 22.3846 | 4.49830799 | 9 |
2 | 162045 | 1.833 | 0.030437 | 11.667 | 18.7272 | 0 | 1 |
3 | 465436 | 1.8461 | 0.02755192 | 12.923 | 20.5 | 2.1726794 | 6 |
4 | 469919 | 1.7778 | 0.0511514 | 6.222 | 11.5 | 9.04897348 | 13 |
5 | 469924 | 1.8182 | 0.0358856 | 9.5454 | 16 | 3.45529378 | 7 |
6 | 469926 | 1.8571 | 0.025008 | 14.2857 | 22.3846 | 4.49830799 | 9 |
7 | 469930 | 1.875 | 0.0194469 | 19.1875 | 28.4667 | 12.3051886 | 15 |
8 | 469973 | 1.875 | 0.020659 | 17.6875 | 28.8667 | 11.7922746 | 14 |
9 | 469988 | 1.8889 | 0.016112 | 23.7778 | 34.1765 | 19.6304783 | 17 |
10 | 469989 | 1.8889 | 0.016112 | 23.7778 | 34.1765 | 19.6304783 | 17 |
11 | 469990 | 1.8571 | 0.025008 | 14.2857 | 22.3846 | 4.49830799 | 9 |
12 | 469991 | 1.8889 | 0.0167056 | 22.5556 | 32.8823 | 17.8586612 | 16 |
13 | 469996 | 1.8182 | 0.037331 | 9 | 15.4 | 4.26420161 | 8 |
14 | 470016 | 1.7333 | 0.011509 | 8.6 | 15.2307 | 4.6521285 | 12 |
15 | 470071 | 1.833 | 0.030437 | 11.667 | 18.7272 | 0 | 1 |
16 | 470072 | 1.833 | 0.030437 | 11.667 | 18.7272 | 0 | 1 |
17 | 470075 | 1.846 | 0.028601 | 12.1538 | 19.6667 | 1.05820927 | 4 |
18 | 470076 | 1.8333 | 0.031711 | 10.9167 | 17.9091 | 1.1100628 | 5 |
Sl. No. | cid | Uniform Rank (UR) | Weighted Rank (WR) | N/w Rank (NWR) | Modified Borda Value(MBV) | Consolidated Rank (CR) | Refined Recommendation (RR) |
1 | 3279 | 1 | 1 | 9 | 37 | 3 | 1 |
2 | 162045 | 6 | 6 | 1 | 35 | 4 | |
3 | 465436 | 4 | 4 | 6 | 34 | 5 | 2 |
4 | 469919 | 13 | 14 | 13 | 8 | 14 | 11 |
5 | 469924 | 14 | 11 | 7 | 16 | 11 | 8 |
6 | 469926 | 7 | 9 | 9 | 23 | 7 | 4 |
7 | 469930 | 15 | 15 | 15 | 3 | 15 | 12 |
8 | 469973 | 16 | 16 | 14 | 2 | 16 | 13 |
9 | 469988 | 8 | 7 | 17 | 16 | 12 | 9 |
10 | 469989 | 18 | 18 | 17 | -5 | 18 | 15 |
11 | 469990 | 4 | 4 | 9 | 31 | 6 | 3 |
12 | 469991 | 17 | 17 | 16 | -2 | 17 | 14 |
13 | 469996 | 9 | 8 | 8 | 23 | 8 | 5 |
14 | 470016 | 11 | 10 | 12 | 15 | 13 | 10 |
15 | 470071 | 2 | 2 | 1 | 43 | 1 | |
16 | 470072 | 2 | 2 | 1 | 43 | 2 | |
17 | 470075 | 10 | 12 | 4 | 22 | 9 | 6 |
18 | 470076 | 12 | 13 | 5 | 18 | 10 | 7 |
Note: "Consolidated Rank" column ranked the drugs as per the customized Borda ranking, and "Refined Recommendation" provided the final recommendation ranks after revisiting Network Based dissimilarities. The grey coloured entries showed the ranks of the cids which were below the rank of 15. Our proposed modification to the Borda ranking incorporated these ranks also. The violet entries signified drugs which had identical network based attribute properties to the queried drug, EMB with cid 14092. |
Attribute Constraints | Filter Rule |
hbond donor count <=5 | Lipinski's Rule |
hbond receptor count <=10 | Lipinski's Rule |
180 <= Molecular weight <=480 | Lipinski's Rule and Ghose Filter (combined) |
-0.4 <= logP < 5 | Lipinski's Rule and Ghose Filter (combined) |
20 <=Total number of atoms <=70 | Ghose Filter |
Polar surface area <=140 | Verber's Rule |
Number of rotatable bonds <=10 | Verber's Rule |
Evaluation Measures | Rank of Candidates | ||||
1 | 2 | 3 | 4 | 5 | |
A | C2 | C3 | C1 | C5 | C4 |
B | C1 | C2 | C4 | C3 | C5 |
C | C3 | C1 | C5 | C2 | C4 |
![]() |
|||||
Note: C1 to C5 are the candidates and A, B, C are the three evaluation measures, and the objective is to get the top three candidates. The upper table shows the ranks of the 5 candidates for each of the evaluation measures. The bottom left table shows the ranks of C1, C2, C3 by the traditional Borda ranking, while the bottom right table shows the same by our customized Borda ranking. The grey coloured cells represent locations where traditional Borda ranking would have kept blank, and would have given C3 and C2 1 point each, respectively. |
PubChem Sl No. | cid | mw | Polar area | complexity | xlogp | heavycnt | hbonddonor | hbondacc | rotbonds | annothitcnt | Dissimilarity Score (UDS) | Uniform Rank (UR) |
14052 | 204.31 | 64.5 | 109 | -0.1 | 14 | 4 | 4 | 9 | 15 | |||
1 | 3279 | 204.31 | 64.5 | 109 | 0 | 14 | 4 | 4 | 9 | 15 | 0.1 | 1 |
2 | 162045 | 204.31 | 64.5 | 123 | 0.4 | 14 | 4 | 4 | 9 | 0 | 20.5243758 | 6 |
3 | 465436 | 204.31 | 64.5 | 109 | 0 | 14 | 4 | 4 | 9 | 1 | 14.0003571 | 4 |
4 | 469919 | 188.31 | 35.5 | 105 | 0 | 13 | 2 | 3 | 8 | 1 | 36.2768521 | 13 |
5 | 469924 | 202.34 | 35.5 | 129 | 0 | 14 | 2 | 3 | 8 | 1 | 38.0380191 | 14 |
6 | 469926 | 218.34 | 64.5 | 120 | 0 | 15 | 4 | 4 | 10 | 1 | 22.7123513 | 7 |
7 | 469930 | 218.34 | 64.5 | 145 | 0 | 15 | 4 | 4 | 9 | 3 | 40.4703706 | 15 |
8 | 469973 | 219.32 | 81.8 | 147 | 0 | 15 | 4 | 5 | 9 | 1 | 46.5467518 | 16 |
9 | 469988 | 204.31 | 64.5 | 128 | 0 | 14 | 4 | 4 | 7 | 2 | 23.1086564 | 8 |
10 | 469989 | 232.36 | 64.5 | 149 | 0 | 16 | 4 | 4 | 9 | 1 | 50.8607167 | 18 |
11 | 469990 | 204.31 | 64.5 | 109 | 0 | 14 | 4 | 4 | 9 | 1 | 14.0003571 | 4 |
12 | 469991 | 232.36 | 64.5 | 145 | 0 | 16 | 4 | 4 | 9 | 3 | 47.2314779 | 17 |
13 | 469996 | 204.31 | 44.7 | 123 | 0 | 14 | 2 | 4 | 9 | 0 | 28.5840865 | 9 |
14 | 470016 | 192.3 | 45.3 | 96.5 | 0 | 13 | 4 | 4 | 7 | 0 | 29.9856649 | 11 |
15 | 470071 | 204.31 | 64.5 | 109 | 0 | 14 | 4 | 4 | 9 | 6 | 9.00055554 | 2 |
16 | 470072 | 204.31 | 64.5 | 109 | 0 | 14 | 4 | 4 | 9 | 6 | 9.00055554 | 2 |
17 | 470075 | 188.31 | 44.3 | 109 | 0 | 13 | 3 | 3 | 8 | 1 | 29.3947274 | 10 |
18 | 470076 | 188.31 | 44.3 | 121 | 0 | 13 | 3 | 3 | 7 | 1 | 31.7970124 | 12 |
PubChem Sl No. | cid | mw | Polar area | complexity | xlogp | heavycnt | hbonddonor | hbondacc | rotbonds | annothitcnt | Weighted Dissimilarity Score (WDS) | Weighted Rank (WR) |
14052 | 204.31 | 64.5 | 109 | -0.1 | 14 | 4 | 4 | 9 | 15 | |||
1 | 3279 | 204.31 | 64.5 | 109 | 0 | 14 | 4 | 4 | 9 | 15 | 0.015385 | 1 |
2 | 162045 | 204.31 | 64.5 | 123 | 0.4 | 14 | 4 | 4 | 9 | 0 | 1.580203 | 6 |
3 | 465436 | 204.31 | 64.5 | 109 | 0 | 14 | 4 | 4 | 9 | 1 | 1.077033 | 4 |
4 | 469919 | 188.31 | 35.5 | 105 | 0 | 13 | 2 | 3 | 8 | 1 | 3.524252 | 14 |
5 | 469924 | 202.34 | 35.5 | 129 | 0 | 14 | 2 | 3 | 8 | 1 | 2.952848 | 11 |
6 | 469926 | 218.34 | 64.5 | 120 | 0 | 15 | 4 | 4 | 10 | 1 | 2.558665 | 9 |
7 | 469930 | 218.34 | 64.5 | 145 | 0 | 15 | 4 | 4 | 9 | 3 | 3.631228 | 15 |
8 | 469973 | 219.32 | 81.8 | 147 | 0 | 15 | 4 | 5 | 9 | 1 | 4.103347 | 16 |
9 | 469988 | 204.31 | 64.5 | 128 | 0 | 14 | 4 | 4 | 7 | 2 | 1.777639 | 7 |
10 | 469989 | 232.36 | 64.5 | 149 | 0 | 16 | 4 | 4 | 9 | 1 | 5.410515 | 18 |
11 | 469990 | 204.31 | 64.5 | 109 | 0 | 14 | 4 | 4 | 9 | 1 | 1.077033 | 4 |
12 | 469991 | 232.36 | 64.5 | 145 | 0 | 16 | 4 | 4 | 9 | 3 | 5.212213 | 17 |
13 | 469996 | 204.31 | 44.7 | 123 | 0 | 14 | 2 | 4 | 9 | 0 | 2.214904 | 8 |
14 | 470016 | 192.3 | 45.3 | 96.5 | 0 | 13 | 4 | 4 | 7 | 0 | 2.807313 | 10 |
15 | 470071 | 204.31 | 64.5 | 109 | 0 | 14 | 4 | 4 | 9 | 6 | 0.692479 | 2 |
16 | 470072 | 204.31 | 64.5 | 109 | 0 | 14 | 4 | 4 | 9 | 6 | 0.692479 | 2 |
17 | 470075 | 188.31 | 44.3 | 109 | 0 | 13 | 3 | 3 | 8 | 1 | 3.113323 | 12 |
18 | 470076 | 188.31 | 44.3 | 121 | 0 | 13 | 3 | 3 | 7 | 1 | 3.250016 | 13 |
Pub Rank | cid | Avg degree | Avg closeness | Avg Nbetween | Avg Ebetween | N/w Weighted Dissimilarity Score (NWDS) | N/w Weighted Rank (NWR) |
14052 | 1.833 | 0.030437 | 11.667 | 18.7272 | |||
1 | 3279 | 1.8571 | 0.025008 | 14.2857 | 22.3846 | 4.49830799 | 9 |
2 | 162045 | 1.833 | 0.030437 | 11.667 | 18.7272 | 0 | 1 |
3 | 465436 | 1.8461 | 0.02755192 | 12.923 | 20.5 | 2.1726794 | 6 |
4 | 469919 | 1.7778 | 0.0511514 | 6.222 | 11.5 | 9.04897348 | 13 |
5 | 469924 | 1.8182 | 0.0358856 | 9.5454 | 16 | 3.45529378 | 7 |
6 | 469926 | 1.8571 | 0.025008 | 14.2857 | 22.3846 | 4.49830799 | 9 |
7 | 469930 | 1.875 | 0.0194469 | 19.1875 | 28.4667 | 12.3051886 | 15 |
8 | 469973 | 1.875 | 0.020659 | 17.6875 | 28.8667 | 11.7922746 | 14 |
9 | 469988 | 1.8889 | 0.016112 | 23.7778 | 34.1765 | 19.6304783 | 17 |
10 | 469989 | 1.8889 | 0.016112 | 23.7778 | 34.1765 | 19.6304783 | 17 |
11 | 469990 | 1.8571 | 0.025008 | 14.2857 | 22.3846 | 4.49830799 | 9 |
12 | 469991 | 1.8889 | 0.0167056 | 22.5556 | 32.8823 | 17.8586612 | 16 |
13 | 469996 | 1.8182 | 0.037331 | 9 | 15.4 | 4.26420161 | 8 |
14 | 470016 | 1.7333 | 0.011509 | 8.6 | 15.2307 | 4.6521285 | 12 |
15 | 470071 | 1.833 | 0.030437 | 11.667 | 18.7272 | 0 | 1 |
16 | 470072 | 1.833 | 0.030437 | 11.667 | 18.7272 | 0 | 1 |
17 | 470075 | 1.846 | 0.028601 | 12.1538 | 19.6667 | 1.05820927 | 4 |
18 | 470076 | 1.8333 | 0.031711 | 10.9167 | 17.9091 | 1.1100628 | 5 |
Sl. No. | cid | Uniform Rank (UR) | Weighted Rank (WR) | N/w Rank (NWR) | Modified Borda Value(MBV) | Consolidated Rank (CR) | Refined Recommendation (RR) |
1 | 3279 | 1 | 1 | 9 | 37 | 3 | 1 |
2 | 162045 | 6 | 6 | 1 | 35 | 4 | |
3 | 465436 | 4 | 4 | 6 | 34 | 5 | 2 |
4 | 469919 | 13 | 14 | 13 | 8 | 14 | 11 |
5 | 469924 | 14 | 11 | 7 | 16 | 11 | 8 |
6 | 469926 | 7 | 9 | 9 | 23 | 7 | 4 |
7 | 469930 | 15 | 15 | 15 | 3 | 15 | 12 |
8 | 469973 | 16 | 16 | 14 | 2 | 16 | 13 |
9 | 469988 | 8 | 7 | 17 | 16 | 12 | 9 |
10 | 469989 | 18 | 18 | 17 | -5 | 18 | 15 |
11 | 469990 | 4 | 4 | 9 | 31 | 6 | 3 |
12 | 469991 | 17 | 17 | 16 | -2 | 17 | 14 |
13 | 469996 | 9 | 8 | 8 | 23 | 8 | 5 |
14 | 470016 | 11 | 10 | 12 | 15 | 13 | 10 |
15 | 470071 | 2 | 2 | 1 | 43 | 1 | |
16 | 470072 | 2 | 2 | 1 | 43 | 2 | |
17 | 470075 | 10 | 12 | 4 | 22 | 9 | 6 |
18 | 470076 | 12 | 13 | 5 | 18 | 10 | 7 |
Note: "Consolidated Rank" column ranked the drugs as per the customized Borda ranking, and "Refined Recommendation" provided the final recommendation ranks after revisiting Network Based dissimilarities. The grey coloured entries showed the ranks of the cids which were below the rank of 15. Our proposed modification to the Borda ranking incorporated these ranks also. The violet entries signified drugs which had identical network based attribute properties to the queried drug, EMB with cid 14092. |