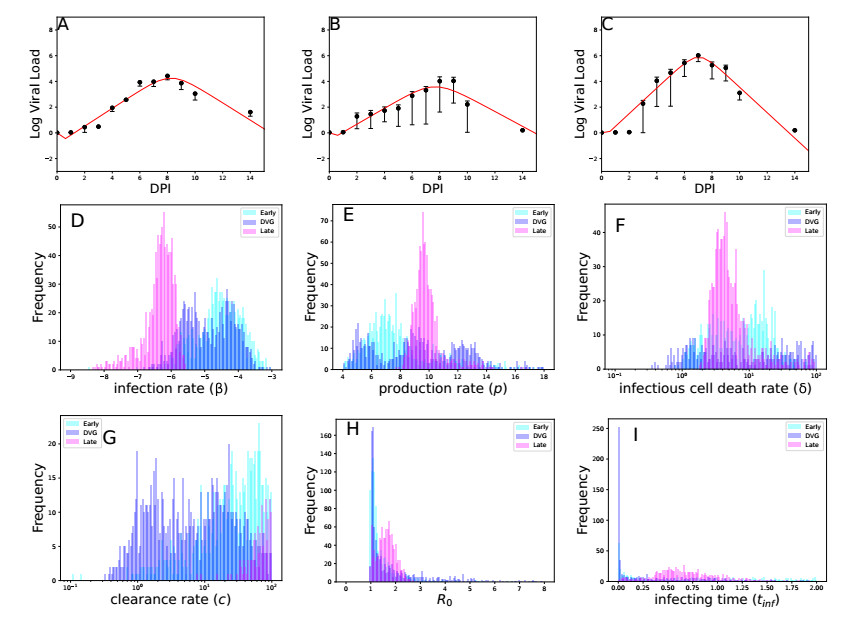
Defective viral genomes (DVGs) are viral genomes that contain only a partial viral RNA and so cannot replicate within cells on their own. If a cell containing DVGs is subsequently infected with a complete viral genome, the DVG can then use the missing proteins expressed by the full genome in order to replicate itself. Since the cell is producing defective genomes, it has less resources to produce fully functional virions and thus release of complete virions is often suppressed. Here, we use data from challenge studies of respiratory syncytial virus (RSV) in healthy adults to quantify the effect of DVGs. We use a mathematical model to fit the data, finding that late onset of DVGs and prolonged DVG detection are associated with lower infection rates and higher clearance rates. This result could have implications for the use of DVGs as a therapeutic.
Citation: Zakarya Noffel, Hana M. Dobrovolny. Quantifying the effect of defective viral genomes in respiratory syncytial virus infections[J]. Mathematical Biosciences and Engineering, 2023, 20(7): 12666-12681. doi: 10.3934/mbe.2023564
[1] | Jianquan Li, Xiaoyu Huo, Yuming Chen . Threshold dynamics of a viral infection model with defectively infected cells. Mathematical Biosciences and Engineering, 2022, 19(7): 6489-6503. doi: 10.3934/mbe.2022305 |
[2] | A. M. Elaiw, Raghad S. Alsulami, A. D. Hobiny . Global dynamics of IAV/SARS-CoV-2 coinfection model with eclipse phase and antibody immunity. Mathematical Biosciences and Engineering, 2023, 20(2): 3873-3917. doi: 10.3934/mbe.2023182 |
[3] | Miguel Ángel Rodríguez-Parra, Cruz Vargas-De-León, Flaviano Godinez-Jaimes, Celia Martinez-Lázaro . Bayesian estimation of parameters in viral dynamics models with antiviral effect of interferons in a cell culture. Mathematical Biosciences and Engineering, 2023, 20(6): 11033-11062. doi: 10.3934/mbe.2023488 |
[4] | Nada Almuallem, Dumitru Trucu, Raluca Eftimie . Oncolytic viral therapies and the delicate balance between virus-macrophage-tumour interactions: A mathematical approach. Mathematical Biosciences and Engineering, 2021, 18(1): 764-799. doi: 10.3934/mbe.2021041 |
[5] | Darrak Moin Quddusi, Sandesh Athni Hiremath, Naim Bajcinca . Mutation prediction in the SARS-CoV-2 genome using attention-based neural machine translation. Mathematical Biosciences and Engineering, 2024, 21(5): 5996-6018. doi: 10.3934/mbe.2024264 |
[6] | Cameron Browne . Immune response in virus model structured by cell infection-age. Mathematical Biosciences and Engineering, 2016, 13(5): 887-909. doi: 10.3934/mbe.2016022 |
[7] | Vivek Sreejithkumar, Kia Ghods, Tharusha Bandara, Maia Martcheva, Necibe Tuncer . Modeling the interplay between albumin-globulin metabolism and HIV infection. Mathematical Biosciences and Engineering, 2023, 20(11): 19527-19552. doi: 10.3934/mbe.2023865 |
[8] | Ting Guo, Zhipeng Qiu . The effects of CTL immune response on HIV infection model with potent therapy, latently infected cells and cell-to-cell viral transmission. Mathematical Biosciences and Engineering, 2019, 16(6): 6822-6841. doi: 10.3934/mbe.2019341 |
[9] | Abdessamad Tridane, Yang Kuang . Modeling the interaction of cytotoxic T lymphocytes and influenza virus infected epithelial cells. Mathematical Biosciences and Engineering, 2010, 7(1): 171-185. doi: 10.3934/mbe.2010.7.171 |
[10] | Tae Jin Lee, Jose A. Vazquez, Arni S. R. Srinivasa Rao . Mathematical modeling of impact of eCD4-Ig molecule in control and management of HIV within a host. Mathematical Biosciences and Engineering, 2021, 18(5): 6887-6906. doi: 10.3934/mbe.2021342 |
Defective viral genomes (DVGs) are viral genomes that contain only a partial viral RNA and so cannot replicate within cells on their own. If a cell containing DVGs is subsequently infected with a complete viral genome, the DVG can then use the missing proteins expressed by the full genome in order to replicate itself. Since the cell is producing defective genomes, it has less resources to produce fully functional virions and thus release of complete virions is often suppressed. Here, we use data from challenge studies of respiratory syncytial virus (RSV) in healthy adults to quantify the effect of DVGs. We use a mathematical model to fit the data, finding that late onset of DVGs and prolonged DVG detection are associated with lower infection rates and higher clearance rates. This result could have implications for the use of DVGs as a therapeutic.
Respiratory syncytial virus (RSV), although the leading cause of hospitalization of infants in developed countries, is still awaiting development of a safe and effective vaccine [1]. Infants typically contract RSV within the first two years of life [2], although there has been a recent suppression of RSV due to COVID-19 mitigation measures [3,4]. This has resulted in earlier and larger epidemics of RSV in many countries this year as COVID mitigation measures have been relaxed [5,6]. RSV can also cause serious illness and death in elderly adults [7]. While the severity of RSV in these two groups is thought to be in part due to a limited immune response [8], other factors might also play a role. A recent study by Felt et al. examined the role of defective viral genomes (DVGs) [9], finding that they might be an indicator of disease severity in RSV.
DVGs, are a feature of a number of viral infections besides RSV [10], including influenza [11,12,13], Sindbis virus [14], vesicular stomatitis virus [15,16], poliovirus [17,18,19], measles [20], SARS-CoV-2 [21], and parvovirus [22]. Defective genomes are thought to have a number of effects on the course of an infection. They interfere with production of fully competent infectious virus when they coinfect cells [11,23]. Interestingly, the within-cell interference can lead to persistence of the infection within the host [23,24,25]. More recently, studies have determined that DVGs also alter the immune response during an infection [9,26,27,28], with evidence suggesting that the presence of DVGs stimulates the innate immune response. Some DVGs are also being investigated as potential vaccines since they have the ability to also stimulate the adaptive immune response [29]. DVGs are also thought to play a role in the evolution of viruses providing increased genetic diversity during infections in a single host [30]. Thus there is a need to develop a better understanding of how the appearance of DVGs alters viral infections.
Mathematical modeling can help us develop an understanding of the role of DVGs in viral infections. There have already been a number of studies that examined models incorporating defective interfering particles (DIPs). DIPs occur when DVGs are packaged and exported out of the cell. DIPs can infect cells, but cannot produce new progeny virions on their own. They can, however, produce new DIPs when in a cell that is infected with both the DIP and a fully competent virus, since the full virus provides the missing components needed for replication, virion formation, and release [31,32]. For example, Liao et al. used a model to determine conditions under which current DIP quantification techniques resulted in correct estimates of the number of DIPs [11]. Shirogane et al. [19] examined how the DIPs competed with wild-type virus in a competitive coinfection model. Other within host models examined the possibility of using defective particles to treat viral infections [33,34,35]. More recent modeling efforts combined intracellular models with within host models in order to understand not only the effect of DIPs at the within host level, but to understand how DVGs might interfere with normal viral replication within the cell [36,37,38].
A limitation of all of these studies is that the models were developed based on assumptions of the effect of DIPs and the models were then used to examine possible outcomes of these assumptions. Unfortunately, many of the known effects of DVGs, such as their effect on the immune response [9,26,27,28,29], were not incorporated into these models, perhaps missing an important process that could alter the interplay between DVGs and fully competent virions. A recent study by Felt et al. [9] could allow for assessment of how DVGs alter the time course of a viral infection — information that can then be incorporated into future modeling efforts in order to produce more accurate mathematical models.
Here, we use mathematical models and the Felt at el. study to examine how DVGs alter the time course of viral load. Without directly incorporating DVGs, we fit a simple mathematical model to experimental data in order to identify differences in viral kinetics parameters under different infection conditions, such as infections with no DVGs and infections where DVGs appear. This type of approach has been used to quantify differences in viral kinetics parameters between wild-type and drug-resistant strains of influenza [39,40], quantify differences in aging animals with RSV [41,42] or SARS-CoV-2 [43], and examine the effect of an antiviral [44]. We find that the appearance of DVGs primarily changes the infection rate and the clearance rate of infections, but only when the DVGs appear late in the infection or are prolonged.
We use a basic model of viral infection that does not include an eclipse phase and assumes that cells produce and release virus immediately upon infection [45],
dTdt=−βTVdIdt=βTV−δIdVdt=pI−cV. | (2.1) |
In this model, T, I, and V are the variables of the model and β, δ, c, and p are the parameters. T represents the number of target cells infected with virus V at a rate β. Once infected, the cells enter the infectious phase I, in which they produce virus at a rate p and die at rate δ. Virus is cleared at rate c. While this model excludes the eclipse phase — where the cell is infected, but not yet producing virus — inclusion of this phase would add an additional parameter that is known to not be identifiable from viral titer data alone [46].
The experimental data used in this study was digitized from Felt et al. [9], which describes a challenge study of RSV infections in healthy adults. In this study, data was collected from nasal swabs taken from healthy adults challenged with RSV. 61 experimentally infected healthy non-smoking adults, aged 18-50 years old, were inoculated experimentally via intranasal drops. Nasal washes and blood samples were taken immediately before inoculation, daily for the first 10 days post infection, and again on days 14 and 28. For all patients, quantitative polymerase chain reaction with reverse transcription methods were used to estimate viral loads. Patients were separated into different groups based on the dynamics of DVGs during the infection and median viral titer curves were used to compare the different groups. One comparison was between patients who had no DVGs detected, those who had DVGs appear early (before 3, 5, or 6 days post infection), and those who had DVGs appear late (after 3, 5, or 6 days post infection). Another comparison was between patients who had no DVGs detected, those who had transient DVG detection (not detected after 6 dpi), and those who had prolonged DVG detection (detected at least once before 6 dpi and detected again after 6 dpi).
Data was extracted from figures using Webplotdigitizer (Webplotdigitizer.com). To fit the data and calculate the parameter estimates for data sets from this study, we used Python's built-in optimizing function scipy.optimize.minimize and minimized the sum of squared residuals (SSR) using the Nelder-Mead algorithm. In addition to estimating the free parameters of the model (β, p, δ, c), we use the estimates to calculate the basic reproductive number, R0=βpcδ, which is the average number of secondary infections caused by a single infected cell, and the infecting time tinf=√2pβ [47], which is the average time between release of virus from one cell and infection of the next cell.
Bootstrapping was used to estimate the distributions of the parameter estimates [48]. We shuffled the residuals at each time point using the numpy shuffle function, which uses a Fisher-Yates shuffle. We then repeated the minimization process to produce another set of parameter estimates. After repeating this process 1000 times, we calculated the 95% confidence interval for each set of parameters.
To compare the parameter distributions, we performed Mann-Whitney U tests using Python's built-in mannwhitneyu function to check if parameter distributions were distinct. The Mann-Whitney test is used since parameter distributions cannot all be assumed to be normal. p values less than 0.05 are considered statistically significant.
In the study by Felt et al. [9], infections are compared using two main features: the time at which DVGs are first detected, and the duration of DVG detection. In the first comparison, participants are divided into three groups: those where DVGs are not detected, those that have DVGs detected early and those that have DVGs detected late. Cutoffs to define early versus late start were set at 3, 5, or 6 days post-infection. The second comparison also consists of three groups: those who do not shed DVGs, those who shed DVGs transiently, and those who shed DVGs for a prolonged period of time. Prolonged DVG was defined as detection of DVGs on two or more days after 6 days post-infection. We perform our analysis using the same groupings.
We show the viral load curves of Figure 5C in the Felt et al. manuscript and our best fit curves along with distributions for the parameter estimates in Figure 1. These viral load curves represent the mean viral load of people with no DVGs detected (A), early DVGs (B), and late DVGs (C) with the early/late cutoff set at 5 days post-infection. The best fit parameter values are given in Table 1. Distributions for the parameters of the viral kinetics model, β, p, c, and δ, as well as distributions for the calculated values R0 and tinf, for all three groups are given in Figure 1 (bottom two rows). p values from the Mann-Whitney test are also presented in Table 1.
Group | β (day)−1⋅(copies/ml)−1 | p (copies/ml)⋅(day)−1 | δ (day)−1 | c (day)−1 | R0 | tinf (day) | SSR |
DVG- | 2.93×10−6 | 1.77×109 | 26.9 | 181 | 1.07 | 0.0200 | 1.82 |
95% CI | (0.569–221)×10−6 | 3.76×104–1.01×1015 | 0.864–401 | 0.614–96.1 | 1.02–6.82 | 1.96×10−5–0.506 | |
Early | 4.38×10−5 | 5.06×106 | 7.62 | 23.1 | 1.26 | 0.0950 | 2.09 |
95% CI | (0.106–42.5)×10−5 | 2.44×104–5.78×1014 | 1.28–2066 | 1.64–472 | 1.01–3.52 | 3.88×10−5–0.442 | |
Late | 2.21×10−8 | 4.95×1012 | 226 | 476 | 1.01 | 0.00428 | 3.26 |
95% CI | (2.10–186)×10−8 | (0.000309–12.6)×1012 | 2.34–408 | 50.6–1350 | 1.02–2.35 | 0.00279–0.0703 | |
p values | |||||||
Early/DVG- | 0.389 | 0.356 | 0.247 | 0.076 | 0.405 | 0.431 | |
Early/Late | 0.0005 | 0.098 | 0.365 | 0.008 | 0.440 | 0.269 | |
DVG-/Late | 0.001 | 0.478 | 0.141 | 5.82×10−5 | 0.436 | 0.475 |
The model is able to fit the viral load curves quite well. The R0 values are fairly low, just over the threshold needed to initiate an infection (R0=1), which is smaller than other estimates found in literature [42,49], although those RSV infections were in animals other than humans. The infecting times range from about 6 minutes to 2 hours, which are more in line with previous estimates [42,49,50]. When comparing parameter estimates between the 3 groups, we note that parameter differences show up primarily in the infection rate, β, and the clearance rate c. When DVGs appear late, the infection rate appears to be lower and the clearance rate appears to be higher, than when DVGs appear early or not at all.
Since the cut-off of 5 days for early vs. late is arbitrary, two other cut-off times were tested. An earlier cut-off of 3 days is shown in Figure 2. In this case, the mean viral load in the early DVG group stayed below the threshold of detection, so we show only the viral time courses and best fit curves for no DVGs (A) and late DVGs (B). The best fit parameter values along with 95% confidence intervals are given in Table 2. Distributions for the parameters of the viral kinetics model, β, p, c, and δ, as well as distributions for the calculated values R0 and tinf, for all three groups are given in Figure 2 (bottom two rows). p values from the Mann-Whitney test are also presented in Table 2.
Group | β (day)−1⋅(copies/ml)−1 | p (copies/ml)⋅(day)−1 | δ (day)−1 | c (day)−1 | R0 | tinf (day) | SSR |
DVG- | 3.10×10−5 | 3.66×107 | 2.41 | 251 | 1.88 | 0.0420 | 0.755 |
95% CI | (0.0948–6.86)×10−5 | 2.56×106–1.53×1016 | 1.53–558 | 16.5–3880 | 1.00–2.48 | 9.41×10−6–0.117 | |
Late | 3.27×10−7 | 5.48×109 | 9.01 | 151 | 1.32 | 0.0334 | 0.821 |
95% CI | (0.453–12.3)×10−6 | (0.262–1280)×109 | 3.47–159 | 31.2–365 | 1.03–1.86 | 0.00653–0.0838 | |
p values | |||||||
DVG-/Late | 0.015 | 0.483 | 0.228 | 0.521 | 0.167 | 0.281 |
The mean viral titer curve for the early DVG group remained below threshold for all time points, making it impossible to fit the model to this data. However, there were three patients in the early DVG group with the earliest cut-off of 3 days. This means that the early DVG patients were shedding very low amounts throughout the infection. For the remaining two groups, we see slightly higher basic reproduction numbers, although they are still fairly close to the threshold of 1. The infecting times are 45 minutes to 1 hour, which are similar to previous estimates [42,49,50]. We can only compare the no DVG and late DVG groups in this case, and we note that the only parameter that is statistically significantly different between the two groups is the infection rate.
A later cut-off date of 6 days was also tested. This is shown in Figure 3. The best fit parameter values along with 95% confidence intervals are given in Table 3. Distributions of the parameter estimates of the viral kinetics model for all three groups are given in Figure 3 (bottom two rows). p values from the Mann-Whitney test are also presented in Table 3.
Group | β (day)−1⋅(copies/ml)−1 | p (copies/ml)⋅(day)−1 | δ (day)−1 | c (day)−1 | R0 | tinf (day) | SSR |
DVG- | 2.20×10−5 | 1.82×107 | 3.25 | 78.0 | 1.58 | 0.0707 | 1.47 |
95% CI | (0.0667–24.8)×10−5 | (0.00348–100)×107 | 0.771–1840 | 0.542–92.3 | 1.02–6.57 | 8.26×10−6–0.521 | |
Early | 5.00×10−6 | 2.19×109 | 31.7 | 326 | 1.06 | 0.0135 | 2.06 |
95% CI | (0.508–180)×10−6 | (0.000128–572)×109 | 1.58–1760 | 2.70–869 | 1.01–2.77 | 4.10×10−6–0.296 | |
Late | 1.68×10−8 | 1.31×1013 | 69.0 | 3060 | 1.04 | 0.00301 | 2.26 |
95% CI | (0.864–91.3)×10−8 | 9.15×108–3.92×1013 | 2.67–476 | 57.7–2080 | 1.01–2.24 | 0.00257–0.0562 | |
p values | |||||||
Early/DVG- | 0.441 | 0.488 | 0.514 | 0.005 | 0.426 | 0.476 | |
Early/Late | 0.005 | 0.238 | 0.365 | 0.024 | 0.468 | 0.446 | |
DVG-/Late | 7.44×10−5 | 0.216 | 0.197 | 2.17×10−5 | 0.503 | 0.478 |
As with the two previous assessments of the effect of time of appearance of DVGs, we see a statistically significant difference in the infection rates of early or no DVGs and late DVGs. We also see a statistically significant difference in the clearance rate between early or no DVGs and late DVGs. In this case, there is also a statistically significant difference between the clearance rates of early and no DVGs. So even when the cut-off day marking the difference between early and late DVGs is shifted, there is a consistent difference in infection rates between early or no DVGs and late DVGs. The differences in clearance rate appear to be more dependent on the chosen cut-off point.
We show the viral load curves of Figure 6C in the Felt et al. manuscript and our best fit curves along with distributions for the parameter estimates in Figure 4. These viral load curves represent the mean viral load of people shedding no DVGs (A), transient DVGs (B), and prolonged DVGs (C). The best fit parameter values are given in Table 4. Distributions for the parameters of the viral kinetics model, β, p, c, and δ as well as distributions for the calculated values R0 and tinf, for all three groups are given in Figure 4 (bottom two rows). p values from the Mann-Whitney test are presented in Table 4.
Group | β (day)−1⋅(copies/ml)−1 | p (copies/ml)⋅(day)−1 | δ (day)−1 | c (day)−1 | R0 | tinf (day) | SSR |
DVG- | 2.55×10−7 | 1.89×1012 | 387 | 1240 | 1.01 | 0.00200 | 2.21 |
95% CI | (0.377–2400)×10−7 | 4.25×104–1.47×1017 | 1.61–486 | 0.853–3910 | 1.00–3.98 | 2.76×10−6–0.455 | |
Transient | 1.01×10−5 | 1.78×108 | 11.8 | 131 | 1.16 | 0.0334 | 1.53 |
95% CI | (0.0654–20.2)×10−5 | 5.50×104–1.08×1016 | 1.17–1817 | 0.961–139 | 1.01–4.82 | 7.22×10−6–0.461 | |
Prolonged | 1.02×10−8 | 2.18×1013 | 61.1 | 3460 | 1.05 | 0.00300 | 2.83 |
95% CI | (0.496–24.9)×10−8 | 5.35×108–7.91×1014 | 18.1–2600 | 3.56–1330 | 1.01–1.96 | 0.00106–0.0908 | |
p values | |||||||
Transient/DVG- | 0.371 | 0.411 | 0.510 | 0.129 | 0.469 | 0.443 | |
Transient/Prolonged | 1.30×10−5 | 0.206 | 0.249 | 0.001 | 0.493 | 0.519 | |
DVG-/Prolonged | 2.37×10−5 | 0.428 | 0.227 | 0.034 | 0.343 | 0.382 |
The model, again, fits the viral load curves well. R0 values are again just over the threshold for infection. The infecting times range from 3 to 48 minutes. The distribution for β estimates for prolonged DVGs is clearly lower than the β distributions for no DVGs or transient DVGs. This is reflected in the statistical tests, which show p values less than 0.05 for no DVG/prolonged DVGs and transient DVGs/prolonged DVGs comparisons of β estimates. We also see similar statistically significant differences in the estimates for the clearance rate c when comparing the prolonged DVGs to the other two groups.
In this manuscript, we fit a mathematical model of viral infections to data examining the appearance of DVGs in RSV infections. We found that the infection rate and the clearance rate are the parameters most affected by the presence of DVGs. Specifically, the infection rate is lower when DVGs appear later and are prolonged during the infection, while the clearance rate is higher in these two cases. In the case of the clearance rate, a possible mechanism is fairly straightforward. When DVGs occur late and are prolonged, they are essentially displacing fully competent virions, which then decrease more quickly than in infections that do not have DVGs or where DVGs are not present later in the infection. The link between later and longer DVGs and lower infection rate is less clear. Defective particles are known to interfere directly with intracellular replication of fully competent virus [51], but they can also interfere with infection dynamics by stimulating the immune response [52]. Since DVGs are known to stimulate the innate immune response in particular [9,26,27,28], this could be an indirect mechanism that reduces the infection rate since the innate immune response typically rises early and rapidly during infections [53]. It is also possible that a lower infection rate leads to a later appearance of DVGs. Since replication is slower in the case of low infection rates, replication of DVGs would presumably also be slower causing them to be detected later in the infection.
While our study could not quantify any differences in the viral time courses of early and no DVGs, the original study by Felt et al. noted that there was a marked difference in the clinical severity of the infection in these two cases. Specifically, they found that the appearance of DVGs early in the infection reduced the severity of the illness. Other recent studies have examined the correlation between viral load and clinical severity, with mixed results. Some studies have found no link between single viral load measures and severity [54,55] while others noted a correlation [56,57,58,59]. More detailed challenge studies note that there appears to be a time delay between rise of viral load and rise of symptoms [60,61], suggesting that there might be some intermediary steps linking viral load and symptoms.
There has been interest in using DVGs to potentially treat viral infections [51,52]. This treatment strategy has been tested in a number of animal models [62,63,64,65,66,67,68,69]. Our results indicate that the timing of treatment initiation could be an important factor since the timing of the appearance of DVGs is associated with different changes in viral kinetics parameters. Duration of production of the DVGs could also be an important factor in the efficacy of treatment since prolonged persistence of DVGs was associated with a lower infection rate and faster clearance of virus. While the idea is that a single injection of DVGs is sufficient because the DVGs should continue to replicate and will persist, this might not always happen since there were some patients in the Felt et al. study who had transient DVGs [9]. Transient DVGs showed no statistically significant difference in infection rate or clearance rate from no DVG patients in this study, suggesting that DVGs need to persist for some period of time in order to affect the time course of the infection.
This study used a very simple model of viral infection that neglects many details of the infection. Our understanding of how DVGs alter the time course of infections would be helped by fitting models that incorporate DVGs [11,33,34,36,37,38] to clinical data. In order to properly parameterize such a model, more data needs to be collected. In addition to viral time courses, we also need the time course of DVGs. A model that explicitly incorporates DVGs could provide insight into how late appearance of DVGs leads to lower infection rates, higher viral loads and more rapid clearance. The explicit inclusion of DVGs will also allow for estimation of parameters related to DVGs such as the DVG production rate, DVG infection rate, and DVG clearance rate.
Despite the simplicity of the model, we might not have found a global minimum and there was still difficulty in identifying all the parameters. It is known that for this simple model δ and c are not both uniquely identifiable from viral titer data alone [46]. We note that some of the confidence intervals are fairly broad, so there is room for improvement on the identifiability of the parameters. In order to improve parameter estimates measurement of other quantities will be needed. For example, measuring both infectious virus and RNA copies can narrow the confidence intervals around parameters estimates [70], while measurement of infected cells in addition to viral measurements can help separate the effects of δ and c [71].
In spite of these limitations, our application of a mathematical model to challenge infections of RSV found a robust link between the appearance and duration of DVGs and the infection rate. Further studies to refine both the model and the parameter estimates will lead to a better understanding of causal link between the appearance of DVGs and the infection rate.
The authors declare there is no conflict of interest.
[1] |
A. Chatterjee, K. Mavunda, L. R. Krilov, Current state of respiratory syncytial virus disease and management, Infect. Dis. Ther., 10 (2021), 5–16. https://doi.org/10.1007/s40121-020-00387-2 doi: 10.1007/s40121-020-00387-2
![]() |
[2] |
D. M. Bowser, K. R. Rowlands, D. Hariharan, R. M. Gervasio, L. Buckley, Y. Halasa-Rappel, et al., Cost of respiratory syncytial virus infections in us infants: Systematic literature review and analysis, J. Infect. Dis., 226 (2022), S225–S235. https://doi.org/10.1093/infdis/jiac172 doi: 10.1093/infdis/jiac172
![]() |
[3] | K. Wagatsuma, I. S. Koolhof, Y. Shobugawa, R. Saito, Decreased human respiratory syncytial virus activity during the COVID-19 pandemic in japan: An ecological time-series analysis, BMC Infect. Dis., 21 (2021), 734. https://doi.org/0.1186/s12879-021-06461-5 |
[4] |
D. Danino, S. Ben-Shimol, B. A. Van der Beek, N. Givon-Lavi, Y. S. Avni, D. Greenberg, et al., Decline in pneumococcal disease in young children during the coronavirus disease 2019 (COVID-19) pandemic in Israel associated with suppression of seasonal respiratory viruses, despite persistent pneumococcal carriage: A prospective cohort study, Clin. Infect. Dis., 75 (2022), E1154–E1164. https://doi.org/10.1093/cid/ciab1014 doi: 10.1093/cid/ciab1014
![]() |
[5] |
I. Kuitunen, M. Artama, M. Haapanen, M. Renko, Respiratory virus circulation in children after relaxation of COVID-19 restrictions in fall 2021-A nationwide register study in Finland, J. Med. Virol., 94 (2022), 4528–4532. https://doi.org/10.1002/jmv.27857 doi: 10.1002/jmv.27857
![]() |
[6] |
P. Hodjat, P. A. Christensen, S. Subedi, D. W. Bernard, R. J. Olsen, S. W. Long, The reemergence of seasonal respiratory viruses in Houston, Texas, after relaxing COVID-19 restrictions, Microbiol. Spectrum, 9 (2021), e00430–21. https://doi.org/10.1128/Spectrum.00430-21 doi: 10.1128/Spectrum.00430-21
![]() |
[7] |
E. E. Walsh, D. R. Peterson, A. R. Falsey, Viral shedding and immune responses to respiratory syncytial virus infection in older adults, J. Infect. Dis., 207 (2013), 1424–1432. https://doi.org/10.1093/infdis/jit038 doi: 10.1093/infdis/jit038
![]() |
[8] |
R. C. Welliver, The immune response to respiratory syncytial virus infection: Friend or foe?, Clin. Rev. Allergy Immunol., 24 (2008), 163–173. https://doi.org/10.1007/s12016-007-8033-2 doi: 10.1007/s12016-007-8033-2
![]() |
[9] |
S. A. Felt, Y. Sun, A. Jozwik, A. Paras, M. S. Habibi, D. Nickle, et al., Detection of respiratory syncytial virus defective genomes in nasal secretions is associated with distinct clinical outcomes, Nat. Microbiol., 6 (2021), 672–681. https://doi.org/10.1038/s41564-021-00882-3 doi: 10.1038/s41564-021-00882-3
![]() |
[10] | M. Treuhaft, M. Beem, Defective interfering particles of respiratory syncytial virus, J. Bacteriol., 91 (1966), 1282–1288. |
[11] |
L. E. Liao, S. Iwami, C. A. A. Beauchemin, (in)validating experimentally derived knowledge about influenza A defective interfering particles, J. R. Soc. Interface, 13 (2016), 20160412. https://doi.org/10.1098/rsif.2016.0412 doi: 10.1098/rsif.2016.0412
![]() |
[12] | N. Kaverin, I. Rudneva, V. Kolodkina, Y. Smirnov, Autocomplementation of influenza-virus defective interfering particles –- cells at high multiplicity infected with defective interfering particles produce defective virions, Acta Virol., 26 (1982), 512–516. |
[13] |
R. Penn, J. S. Tregoning, K. E. Flight, L. Baillon, R. Frise, D. H. Goldhill, et al., Levels of influenza A virus defective viral genomes determine pathogenesis in the BALB/c mouse model, J. Virol., 96 (2022). https://doi.org/10.1128/jvi.01178-22 doi: 10.1128/jvi.01178-22
![]() |
[14] |
T. Shenk, V. Stollar, Defective interfering particles of sindbis virus.2. homologous interference, Virology, 55 (1973), 530–534. https://doi.org/10.1016/0042-6822(73)90197-9 doi: 10.1016/0042-6822(73)90197-9
![]() |
[15] |
C. Kang, R. Allen, Host function dependent induction of defective interfering particles of vesicular stomatitis virus, J. Virol., 25 (1978), 202–206. https://doi.org/10.1128/JVI.25.1.202-206.1978 doi: 10.1128/JVI.25.1.202-206.1978
![]() |
[16] |
J. Keene, M. Rosenberg, R. Lazzarini, Characterization of 3' terminus of RNA isolated from vesicular stomatitis virus and from its defective interfering particles, Proc. Natl. Acad. Sci. U.S.A., 74 (1977), 1353–1357. https://doi.org/10.1073/pnas.74.4.1353 doi: 10.1073/pnas.74.4.1353
![]() |
[17] |
C. Cole, D. Smoler, E. Wimmer, D. Baltimore, Defective interfering particles of poliovirus.2. isolation and physical properties, J. Virol., 7 (1971), 478. https://doi.org/10.1128/JVI.7.4.478-485.1971 doi: 10.1128/JVI.7.4.478-485.1971
![]() |
[18] |
C. Cole, D. Baltimore, Defective interfering particles of poliovirus.2. nature of defect, J. Mol. Biol., 76 (1973), 325–343. https://doi.org/10.1016/0022-2836(73)90508-1 doi: 10.1016/0022-2836(73)90508-1
![]() |
[19] |
Y. Shirogane, Elsa Rousseau, Jakub Voznica, Yinghong Xiao, Weiheng Su, Adam Catching, Z. J. Whitfield, I. M. Rouzine, Simone Bianco, Raul Andino, Experimental and mathematical insights on the interactions between poliovirus and a defective interfering genome, Plos Path., 17 (2021), e1009277. https://doi.org/10.1371/journal.ppat.1009277 doi: 10.1371/journal.ppat.1009277
![]() |
[20] |
W. Hall, S. Martin, Defective interfering particles produced during replication of measles-virus, Med. Microbiol. Immunol., 160 (1974), 155–164. https://doi.org/10.1007/BF02121722 doi: 10.1007/BF02121722
![]() |
[21] |
S. Girgis, Z. K. Xu, S. Oikonomopoulos, A. D. Fedorova, E. P. Tchesnokov, C. J. Gordon, et al., Evolution of naturally arising SARS-CoV-2 defective interfering particles, Comm. Biol., 5 (2022), 1140. https://doi.org/10.1038/s42003-022-04058-5 doi: 10.1038/s42003-022-04058-5
![]() |
[22] |
S. Rhode, Defective interfering particles of parvovirus H-1, J. Virol., 27 (1978), 347–356. https://doi.org/10.1128/JVI.27.2.347-356.1978 doi: 10.1128/JVI.27.2.347-356.1978
![]() |
[23] |
C. Bangham, T. Kirkwood, Defective interfering particles — effects in modulating virus growth and persistance, Virology, 179 (1990), 821–826. https://doi.org/10.1016/0042-6822(90)90150-P doi: 10.1016/0042-6822(90)90150-P
![]() |
[24] |
C. M. Ziegler, J. W. Botten, Defective interfering particles of negative-strand rna viruses, Trends in Microbiol., 28 (2020), 554–565. https://doi.org/10.1016/j.tim.2020.02.006 doi: 10.1016/j.tim.2020.02.006
![]() |
[25] |
M. Valdovinos, B. Gomez, Establishment of respiratory syncytial virus persistence in cell lines: Association with defective interfering particles, Intervirol., 46 (2003), 90–198. https://doi.org/10.1159/000071461 doi: 10.1159/000071461
![]() |
[26] |
Y. Sun, D. Jain, C. J. Koziol-White, E. Genoyer, M. Gilbert, K. Tapia, et al., Immunostimulatory defective viral genomes from respiratory syncytial virus promote a strong innate antiviral response during infection in mice and humans, Plos Path., 11 (2015), e1005122. https://doi.org/10.1371/journal.ppat.1005122 doi: 10.1371/journal.ppat.1005122
![]() |
[27] |
C. Wang, C. V. Forst, T.-W. Chou, A. Geber, M. Wang, W. Hamou, et al., Cell-to-cell variation in defective virus expression and effects on host responses during influenza virus infection, MBIO, 11 (2020), e02880–19. https://doi.org/10.1128/mBio.02880-19 doi: 10.1128/mBio.02880-19
![]() |
[28] |
X. Mercado-Lopez, C. R. Cotter, W. keun Kim, Y. Sun, L. Munoz, K. Tapia, et al., Highly immunostimulatory RNA derived from a Sendai virus defective viral genome, Vaccine, 31 (2013), 5713–5721. https://doi.org/10.1016/j.vaccine.2013.09.040 doi: 10.1016/j.vaccine.2013.09.040
![]() |
[29] |
Y. Xiao, P. V. Lidsky, Y. Shirogane, R. Aviner, C.-T. Wu, W. Y. Li, et al., A defective viral genome strategy elicits broad protective immunity against respiratory viruses, Cell, 184 (2021), 6037. https://doi.org/10.1016/j.cell.2021.11.023 doi: 10.1016/j.cell.2021.11.023
![]() |
[30] |
C. Bangham, T. Kirkwood, Defective interfering particles and virus evolution, Trends Microbiol., 1 (1993), 260–264. https://doi.org/10.1016/0966-842X(93)90048-V doi: 10.1016/0966-842X(93)90048-V
![]() |
[31] |
V. V. Rezelj, L. I. Levi, M. Vignuzzi, The defective component of viral populations, Curr. Opin. Virol., 33 (2018), 74–80. https://doi.org/10.1016/j.coviro.2018.07.014 doi: 10.1016/j.coviro.2018.07.014
![]() |
[32] |
T. Bhat, A. Cao, J. Yin, Virus-like particles: Measures and biological functions, Viruses, 14 (2022), 383. https://doi.org/10.3390/v14020383 doi: 10.3390/v14020383
![]() |
[33] |
K. A. Stauffer, G. A. Rempala, J. Yin, Multiple-hit inhibition of infection by defective interfering particles, J. Gen. Virol., 90 (2009), 888–899. https://doi.org/10.1099/vir.0.005249-0 doi: 10.1099/vir.0.005249-0
![]() |
[34] |
T. Mapder, S. Clifford, J. Aaskov, K. Burrage, A population of bang-bang switches of defective interfering particles makes within-host dynamics of dengue virus controllable, PLOS Comp. Biol., 15 (2009), e1006668. https://doi.org/10.1371/journal.pcbi.1006668 doi: 10.1371/journal.pcbi.1006668
![]() |
[35] |
F. Fatehi, R. J. Bingham, Pierre-Philippe Dechant, P. G. Stockley, R. Twarock, Therapeutic interfering particles exploiting viral replication and assembly mechanisms show promising performance: a modelling study, Sci. Rep., 11 (2021), 23847. https://doi.org/10.1038/s41598-021-03168-0 doi: 10.1038/s41598-021-03168-0
![]() |
[36] |
V. Sharov, V. V. Rezelj, V. V. Galatenko, A. Titievsky, J. Panov, K. Chumakov, et al., Intra- and inter-cellular modeling of dynamic interaction between zika virus and its naturally occurring defective viral genomes, J. Virol., 95 (2021), e00977. https://doi.org/10.1128/JVI.00977–21 doi: 10.1128/JVI.00977–21
![]() |
[37] |
D. Ruediger, S. Y. Kupke, T. Laske, P. Zmora, U. Reichl, Multiscale modeling of influenza A virus replication in cell cultures predicts infection dynamics for highly different infection conditions, Plos Comp. Biol., 15 (2019), e1006819. https://doi.org/10.1371/journal.pcbi.1006819 doi: 10.1371/journal.pcbi.1006819
![]() |
[38] |
D. Ruediger, L. Pelz, M. D. Hein, S. Y. Kupke, U. Reichl, Multiscale model of defective interfering particle replication for influenza A virus infection in animal cell culture, Plos Comp. Biol., 17 (2021), e1009357. https://doi.org/10.1371/journal.pcbi.1009357 doi: 10.1371/journal.pcbi.1009357
![]() |
[39] |
L. T. Pinilla, B. P. Holder, Y. Abed, G. Boivin, C. A. A. Beauchemin, The H275Y neuraminidase mutation of the pandemic A/H1N1 influenza virus lengthens the eclipse phase and reduces viral output of infected cells, potentially compromising fitness in ferrets, J. Virol., 86 (2012), 10651–10660. https://doi.org/10.1128/JVI.0724411 doi: 10.1128/JVI.0724411
![]() |
[40] |
E. Paradis, L. Pinilla, B. Holder, Y. Abed, G. Boivin, C. Beauchemin, Impact of the H275Y and I223V mutations in the neuraminidase of the 2009 pandemic influenza virus in vitro and evaluating experimental reproducibility, PLoS One, 10 (2015), e0126115. https://doi.org/10.1371/journal.pone.0126115 doi: 10.1371/journal.pone.0126115
![]() |
[41] |
D. Wethington, O. Harder, K. Uppulury, W. C. Stewart, P. Chen, T. King, et al., Mathematical modelling identifies the role of adaptive immunity as a key controller of respiratory syncytial virus in cotton rats, J. Roy. Soc. Interface, 16 (2019), 20190389. https://doi.org/10.1098/rsif.2019.0389 doi: 10.1098/rsif.2019.0389
![]() |
[42] |
S. Khan, H. M. Dobrovolny, A study of the effects of age on the dynamics of RSV in animal models, Virus Res., 304 (2021), 198524. doilinkhttps://doi.org/10.1016/j.virusres.2021.198524 doi: 10.1016/j.virusres.2021.198524
![]() |
[43] |
T. Rodriguez, H. M. Dobrovolny, Estimation of viral kinetics model parameters in young and aged SARS-CoV-2 infected macaques, R. Soc. Open Sci., 8 (2021), 202345. https://doi.org/10.1098/rsos.202345 doi: 10.1098/rsos.202345
![]() |
[44] |
H. M. Dobrovolny, Quantifying the effect of remdesivir in rhesus macaques infected with SARS-CoV-2, Virology, 550 (2020), 61–69. https://doi.org/10.1016/j.virol.2020.07.015 doi: 10.1016/j.virol.2020.07.015
![]() |
[45] |
P. Baccam, C. Beauchemin, C. A. Macken, F. G. Hayden, A. S. Perelson, Kinetics of influenza A virus infection in humans, J. Virol., 80 (2006), 7590–7599. https://doi.org/10.1128/JVI.01623-05 doi: 10.1128/JVI.01623-05
![]() |
[46] |
A. M. Smith, F. R. Adler, A. S. Perelson, An accurate two-phase approximate solution to an acute viral infection model, J. Math. Biol., 60 (2010), 711–726. https://doi.org/10.1007/s00285-009-0281-8 doi: 10.1007/s00285-009-0281-8
![]() |
[47] |
B. P. Holder, C. A. Beauchemin, Exploring the effect of biological delays in kinetic models of influenza within a host or cell culture, BMC Public Health, 11 (2011), S10. https://doi.org/doi:10.1186/1471-2458-11-S1-S10 doi: 10.1186/1471-2458-11-S1-S10
![]() |
[48] | B. Efron, R. Tibshirani, Bootstrap methods for standard errors, confidence intervals, and other measures of statistical accuracy, Stat. Sci., 1 (1986), 54–75. |
[49] |
G. González-Parra, H. M. Dobrovolny, Modeling of fusion inhibitor treatment of RSV in African green monkeys, J. Theor. Biol., 456 (2018), 62–73. https://doi.org/10.1016/j.jtbi.2018.07.029 doi: 10.1016/j.jtbi.2018.07.029
![]() |
[50] |
G. González-Parra, F. De Ridder, D. Huntjens, D. Roymans, G. Ispas, H. M. Dobrovolny, A comparison of RSV and influenza in vitro kinetic parameters reveals differences in infecting time, Plos One, 13 (2018), e0192645. https://doi.org/10.1371/journal.pone.0192645 doi: 10.1371/journal.pone.0192645
![]() |
[51] |
L. Pelz, D. Rudiger, T. Dogra, F. G. Alnaji, Y. Genzel, C. B. Brooke, et al., Semi-continuous propagation of influenza A virus and its defective interfering particles: Analyzing the dynamic competition to select candidates for antiviral therapy, J. Virol., 95 (2021), e01174–21. https://doi.org/10.1128/JVI.01174-21 doi: 10.1128/JVI.01174-21
![]() |
[52] |
N. J. Dimmock, A. J. Easton, Cloned defective interfering influenza RNA and a possible pan-specific treatment of respiratory virus diseases, Viruses, 7 (2015), 3768–3788. https://doi.org/10.3390/v7072796 doi: 10.3390/v7072796
![]() |
[53] |
H. M. Dobrovolny, M. B. Reddy, M. A. Kamal, C. R. Rayner, C. A. Beauchemin, Assessing mathematical models of influenza infections using features of the immune response, PLoS One, 8 (2013), e57088. https://doi.org/10.1371/journal.pone.0057088 doi: 10.1371/journal.pone.0057088
![]() |
[54] |
X. I. Yan, Y. H. Li, Y. J. Tang, Z. P. Xie, H. C. Gao, X. M. Yang, et al., Clinical characteristics and viral load of respiratory syncytial virus and human metapneumovirus in children hospitaled for acute lower respiratory tract infection, J. Med. Virol., 89 (2017), 589–597. https://doi.org/10.1002/jmv.24687 doi: 10.1002/jmv.24687
![]() |
[55] |
R. A. S. Watanabe, J. S. Cruz, L. K. Luna, V. R. G. Alves, D. D. Conte, L. Lyra, et al., Respiratory syncytial virus: viral load, viral decay, and disease progression in children with bronchiolitis, Brazil. J. Microbiol., 53 (2022), 1241–1247. https://doi.org/10.1007/s42770-022-00742-0 doi: 10.1007/s42770-022-00742-0
![]() |
[56] |
L. Zhou, Q. Y. Xiao, Y. Zhao, A. L. Huang, L. Ren, E. M. Liu, The impact of viral dynamics on the clinical severity of infants with respiratory syncytial virus bronchiolitis, J. Med. Virol., 87 (2015), 1276–1284. https://doi.org/10.1002/jmv.24111 doi: 10.1002/jmv.24111
![]() |
[57] |
Y. Espinosa, C. Martin, A. A. Torres, M. J. Farfan, J. P. Torres, V. Avadhanula, et al., Genomic loads and genotypes of respiratory syncytial virus: Viral factors during lower respiratory tract infection in chilean hospitalized infants, Intl. J. Mol. Sci., 18 (2017), 654. https://doi.org/10.3390/ijms18030654 doi: 10.3390/ijms18030654
![]() |
[58] |
L. Vos, R. Bruyndonckx, N. P. A. Zuithoff, P. Little, J. J. Oosterheert, B. D. L. Broekhuizen, et al., Lower respiratory tract infection in the community: Associations between viral aetiology and illness course, Clin. Microbiol. Infect., 27 (2021), 96–104. https://doi.org/10.1016/j.cmi.2020.03.023 doi: 10.1016/j.cmi.2020.03.023
![]() |
[59] |
E. Uusitupa, M. Waris, T. Heikkinen, Association of viral load with disease severity in outpatient children with respiratory syncytial virus infection, J. Infect. Dis., 222 (2020), 298–304. https://doi.org/10.1093/infdis/jiaa076 doi: 10.1093/infdis/jiaa076
![]() |
[60] |
J. P. DeVincenzo, T. Wilkinson, A. Vaishnaw, J. Cehelsky, R. Meyers, S. Nochur, et al., Viral load drives disease in humans experimentally infected with respiratory syncytial virus, Am. J. Resp. Crit. Care Med., 182 (2010), 1305–1314. https://doi.org/10.1164/rccm.201002-0221OC doi: 10.1164/rccm.201002-0221OC
![]() |
[61] |
B. Bagga, C. W. Woods, T. H. Veldman, A. Gilbert, A. Mann, G. Balaratnam, et al., Comparing influenza and RSV viral disease dynamics in experimentally infected adults predicts clinical effectiveness of RSV antivirals, Antivir. Ther., 18 (2013), 785–791. https://doi.org/10.3851/IMP2629 doi: 10.3851/IMP2629
![]() |
[62] |
P. D. Scott, B. Meng, A. C. Marriott, A. J. Easton, N. J. Dimmock, Defective interfering virus protects elderly mice from influenza, Virol. J., 8 (2011), 212. https://doi.org/10.1186/1743-422X-8-212 doi: 10.1186/1743-422X-8-212
![]() |
[63] |
S. R. Welch, J. R. Spengler, J. R. Harmon, J. D. Coleman-McCray, F. E. Scholte, S. C. Genzer, et al., Defective interfering viral particle treatment reduces clinical signs and protects hamsters from lethal nipah virus disease, MBIO, 13 (2022), e03294. https://doi.org/10.1128/mbio.03294-21 doi: 10.1128/mbio.03294-21
![]() |
[64] |
D. Morgan, L. McLain, N. Dimmock, Apical budding of a recombinant influenza A virus expressing a hemagglutinin protein with a basolateral localization signal, Virus Res., 29 (1993), 179–193. https://doi.org/10.1016/0168-1702(93)90058-U doi: 10.1016/0168-1702(93)90058-U
![]() |
[65] |
A. J. Easton, P. D. Scott, N. L. Edworthy, B. Meng, A. C. Marriott, N. J. Dimmock, A novel broad-spectrum treatment for respiratory virus infections: Influenza-based defective interfering virus provides protection against pneumovirus infection in vivo, Vaccine, 29 (2011), 2777–2784. https://doi.org/10.1016/j.vaccine.2011.01.102 doi: 10.1016/j.vaccine.2011.01.102
![]() |
[66] |
A. Mann, A. Marriott, S. Balasingam, R. Lambkin, J. Oxford, N. Dimmock, Interfering vaccine (defective interfering influenza A virus) protects ferrets from influenza, and allows them to develop solid immunity to reinfection, Vaccine, 24 (2006), 4290–4296. https://doi.org/10.1016/j.vaccine.2006.03.004 doi: 10.1016/j.vaccine.2006.03.004
![]() |
[67] |
M. D. Hein, H. Kollmus, P. Marichal-Gallardo, S. Puttker, D. Benndorf, Y. Genzel, et al., OP7, a novel influenza A virus defective interfering particle: Production, purification, and animal experiments demonstrating antiviral potential, Appl. Microbiol. Biotech., 105 (2021), 129–146. https://doi.org/10.1007/s00253-020-11029-5 doi: 10.1007/s00253-020-11029-5
![]() |
[68] | M.-H. Lin, D. S. Li, B. Tang, L. Li, A. Suhrbier, D. Harrich, Defective interfering particles with broad-acting antiviral activity for dengue, zika, yellow fever, respiratory syncytial and SARS-CoV-2 virus infection, Microbiol. Spectrum, (November 2022). https://doi.org/10.1128/spectrum.03949-22 |
[69] |
S. Chaturvedi, G. Vasen, M. Pablo, X. Y. Chen, N. Beutler, A. Kumar, et al., Identification of a therapeutic interfering particle-a single-dose SARS-CoV-2 antiviral intervention with a high barrier to resistance, Cell, 184 (2021), 6022. https://doi.org/10.1016/j.cell.2021.11.004 doi: 10.1016/j.cell.2021.11.004
![]() |
[70] |
S. M. Petrie, J. Butler, I. G. Barr, J. McVernon, A. C. Hurt, J. M. McCaw, Quantifying relative within-host replication fitness in influenza virus competition experiments, J. Theor. Biol., 382 (2015), 259–271. https://doi.org/10.1016/j.jtbi.2015.07.003 doi: 10.1016/j.jtbi.2015.07.003
![]() |
[71] |
H. Miao, X. Xia, A. S. Perelson, H. Wu, On identifiability of nonlinear ODE models and applications in viral dynamics, SIAM Rev., 53 (2011), 3–39. https://doi.org/10.1137/090757009 doi: 10.1137/090757009
![]() |
1. | Ishaan Gadiyar, Hana M. Dobrovolny, Different routes of infection of H5N1 lead to changes in infecting time, 2024, 367, 00255564, 109129, 10.1016/j.mbs.2023.109129 | |
2. | Gilberto González-Parra, Hana M. Dobrovolny, Editorial: Mathematical foundations in biological modelling and simulation, 2024, 21, 1551-0018, 7084, 10.3934/mbe.2024311 | |
3. | Arjan Suri, Sahaj Satani, Hana M. Dobrovolny, Analyzing Differences in Viral Dynamics Between Vaccinated and Unvaccinated RSV Patients, 2025, 6, 2673-3986, 16, 10.3390/epidemiologia6020016 |
Group | β (day)−1⋅(copies/ml)−1 | p (copies/ml)⋅(day)−1 | δ (day)−1 | c (day)−1 | R0 | tinf (day) | SSR |
DVG- | 2.93×10−6 | 1.77×109 | 26.9 | 181 | 1.07 | 0.0200 | 1.82 |
95% CI | (0.569–221)×10−6 | 3.76×104–1.01×1015 | 0.864–401 | 0.614–96.1 | 1.02–6.82 | 1.96×10−5–0.506 | |
Early | 4.38×10−5 | 5.06×106 | 7.62 | 23.1 | 1.26 | 0.0950 | 2.09 |
95% CI | (0.106–42.5)×10−5 | 2.44×104–5.78×1014 | 1.28–2066 | 1.64–472 | 1.01–3.52 | 3.88×10−5–0.442 | |
Late | 2.21×10−8 | 4.95×1012 | 226 | 476 | 1.01 | 0.00428 | 3.26 |
95% CI | (2.10–186)×10−8 | (0.000309–12.6)×1012 | 2.34–408 | 50.6–1350 | 1.02–2.35 | 0.00279–0.0703 | |
p values | |||||||
Early/DVG- | 0.389 | 0.356 | 0.247 | 0.076 | 0.405 | 0.431 | |
Early/Late | 0.0005 | 0.098 | 0.365 | 0.008 | 0.440 | 0.269 | |
DVG-/Late | 0.001 | 0.478 | 0.141 | 5.82×10−5 | 0.436 | 0.475 |
Group | β (day)−1⋅(copies/ml)−1 | p (copies/ml)⋅(day)−1 | δ (day)−1 | c (day)−1 | R0 | tinf (day) | SSR |
DVG- | 3.10×10−5 | 3.66×107 | 2.41 | 251 | 1.88 | 0.0420 | 0.755 |
95% CI | (0.0948–6.86)×10−5 | 2.56×106–1.53×1016 | 1.53–558 | 16.5–3880 | 1.00–2.48 | 9.41×10−6–0.117 | |
Late | 3.27×10−7 | 5.48×109 | 9.01 | 151 | 1.32 | 0.0334 | 0.821 |
95% CI | (0.453–12.3)×10−6 | (0.262–1280)×109 | 3.47–159 | 31.2–365 | 1.03–1.86 | 0.00653–0.0838 | |
p values | |||||||
DVG-/Late | 0.015 | 0.483 | 0.228 | 0.521 | 0.167 | 0.281 |
Group | β (day)−1⋅(copies/ml)−1 | p (copies/ml)⋅(day)−1 | δ (day)−1 | c (day)−1 | R0 | tinf (day) | SSR |
DVG- | 2.20×10−5 | 1.82×107 | 3.25 | 78.0 | 1.58 | 0.0707 | 1.47 |
95% CI | (0.0667–24.8)×10−5 | (0.00348–100)×107 | 0.771–1840 | 0.542–92.3 | 1.02–6.57 | 8.26×10−6–0.521 | |
Early | 5.00×10−6 | 2.19×109 | 31.7 | 326 | 1.06 | 0.0135 | 2.06 |
95% CI | (0.508–180)×10−6 | (0.000128–572)×109 | 1.58–1760 | 2.70–869 | 1.01–2.77 | 4.10×10−6–0.296 | |
Late | 1.68×10−8 | 1.31×1013 | 69.0 | 3060 | 1.04 | 0.00301 | 2.26 |
95% CI | (0.864–91.3)×10−8 | 9.15×108–3.92×1013 | 2.67–476 | 57.7–2080 | 1.01–2.24 | 0.00257–0.0562 | |
p values | |||||||
Early/DVG- | 0.441 | 0.488 | 0.514 | 0.005 | 0.426 | 0.476 | |
Early/Late | 0.005 | 0.238 | 0.365 | 0.024 | 0.468 | 0.446 | |
DVG-/Late | 7.44×10−5 | 0.216 | 0.197 | 2.17×10−5 | 0.503 | 0.478 |
Group | β (day)−1⋅(copies/ml)−1 | p (copies/ml)⋅(day)−1 | δ (day)−1 | c (day)−1 | R0 | tinf (day) | SSR |
DVG- | 2.55×10−7 | 1.89×1012 | 387 | 1240 | 1.01 | 0.00200 | 2.21 |
95% CI | (0.377–2400)×10−7 | 4.25×104–1.47×1017 | 1.61–486 | 0.853–3910 | 1.00–3.98 | 2.76×10−6–0.455 | |
Transient | 1.01×10−5 | 1.78×108 | 11.8 | 131 | 1.16 | 0.0334 | 1.53 |
95% CI | (0.0654–20.2)×10−5 | 5.50×104–1.08×1016 | 1.17–1817 | 0.961–139 | 1.01–4.82 | 7.22×10−6–0.461 | |
Prolonged | 1.02×10−8 | 2.18×1013 | 61.1 | 3460 | 1.05 | 0.00300 | 2.83 |
95% CI | (0.496–24.9)×10−8 | 5.35×108–7.91×1014 | 18.1–2600 | 3.56–1330 | 1.01–1.96 | 0.00106–0.0908 | |
p values | |||||||
Transient/DVG- | 0.371 | 0.411 | 0.510 | 0.129 | 0.469 | 0.443 | |
Transient/Prolonged | 1.30×10−5 | 0.206 | 0.249 | 0.001 | 0.493 | 0.519 | |
DVG-/Prolonged | 2.37×10−5 | 0.428 | 0.227 | 0.034 | 0.343 | 0.382 |
Group | β (day)−1⋅(copies/ml)−1 | p (copies/ml)⋅(day)−1 | δ (day)−1 | c (day)−1 | R0 | tinf (day) | SSR |
DVG- | 2.93×10−6 | 1.77×109 | 26.9 | 181 | 1.07 | 0.0200 | 1.82 |
95% CI | (0.569–221)×10−6 | 3.76×104–1.01×1015 | 0.864–401 | 0.614–96.1 | 1.02–6.82 | 1.96×10−5–0.506 | |
Early | 4.38×10−5 | 5.06×106 | 7.62 | 23.1 | 1.26 | 0.0950 | 2.09 |
95% CI | (0.106–42.5)×10−5 | 2.44×104–5.78×1014 | 1.28–2066 | 1.64–472 | 1.01–3.52 | 3.88×10−5–0.442 | |
Late | 2.21×10−8 | 4.95×1012 | 226 | 476 | 1.01 | 0.00428 | 3.26 |
95% CI | (2.10–186)×10−8 | (0.000309–12.6)×1012 | 2.34–408 | 50.6–1350 | 1.02–2.35 | 0.00279–0.0703 | |
p values | |||||||
Early/DVG- | 0.389 | 0.356 | 0.247 | 0.076 | 0.405 | 0.431 | |
Early/Late | 0.0005 | 0.098 | 0.365 | 0.008 | 0.440 | 0.269 | |
DVG-/Late | 0.001 | 0.478 | 0.141 | 5.82×10−5 | 0.436 | 0.475 |
Group | β (day)−1⋅(copies/ml)−1 | p (copies/ml)⋅(day)−1 | δ (day)−1 | c (day)−1 | R0 | tinf (day) | SSR |
DVG- | 3.10×10−5 | 3.66×107 | 2.41 | 251 | 1.88 | 0.0420 | 0.755 |
95% CI | (0.0948–6.86)×10−5 | 2.56×106–1.53×1016 | 1.53–558 | 16.5–3880 | 1.00–2.48 | 9.41×10−6–0.117 | |
Late | 3.27×10−7 | 5.48×109 | 9.01 | 151 | 1.32 | 0.0334 | 0.821 |
95% CI | (0.453–12.3)×10−6 | (0.262–1280)×109 | 3.47–159 | 31.2–365 | 1.03–1.86 | 0.00653–0.0838 | |
p values | |||||||
DVG-/Late | 0.015 | 0.483 | 0.228 | 0.521 | 0.167 | 0.281 |
Group | β (day)−1⋅(copies/ml)−1 | p (copies/ml)⋅(day)−1 | δ (day)−1 | c (day)−1 | R0 | tinf (day) | SSR |
DVG- | 2.20×10−5 | 1.82×107 | 3.25 | 78.0 | 1.58 | 0.0707 | 1.47 |
95% CI | (0.0667–24.8)×10−5 | (0.00348–100)×107 | 0.771–1840 | 0.542–92.3 | 1.02–6.57 | 8.26×10−6–0.521 | |
Early | 5.00×10−6 | 2.19×109 | 31.7 | 326 | 1.06 | 0.0135 | 2.06 |
95% CI | (0.508–180)×10−6 | (0.000128–572)×109 | 1.58–1760 | 2.70–869 | 1.01–2.77 | 4.10×10−6–0.296 | |
Late | 1.68×10−8 | 1.31×1013 | 69.0 | 3060 | 1.04 | 0.00301 | 2.26 |
95% CI | (0.864–91.3)×10−8 | 9.15×108–3.92×1013 | 2.67–476 | 57.7–2080 | 1.01–2.24 | 0.00257–0.0562 | |
p values | |||||||
Early/DVG- | 0.441 | 0.488 | 0.514 | 0.005 | 0.426 | 0.476 | |
Early/Late | 0.005 | 0.238 | 0.365 | 0.024 | 0.468 | 0.446 | |
DVG-/Late | 7.44×10−5 | 0.216 | 0.197 | 2.17×10−5 | 0.503 | 0.478 |
Group | β (day)−1⋅(copies/ml)−1 | p (copies/ml)⋅(day)−1 | δ (day)−1 | c (day)−1 | R0 | tinf (day) | SSR |
DVG- | 2.55×10−7 | 1.89×1012 | 387 | 1240 | 1.01 | 0.00200 | 2.21 |
95% CI | (0.377–2400)×10−7 | 4.25×104–1.47×1017 | 1.61–486 | 0.853–3910 | 1.00–3.98 | 2.76×10−6–0.455 | |
Transient | 1.01×10−5 | 1.78×108 | 11.8 | 131 | 1.16 | 0.0334 | 1.53 |
95% CI | (0.0654–20.2)×10−5 | 5.50×104–1.08×1016 | 1.17–1817 | 0.961–139 | 1.01–4.82 | 7.22×10−6–0.461 | |
Prolonged | 1.02×10−8 | 2.18×1013 | 61.1 | 3460 | 1.05 | 0.00300 | 2.83 |
95% CI | (0.496–24.9)×10−8 | 5.35×108–7.91×1014 | 18.1–2600 | 3.56–1330 | 1.01–1.96 | 0.00106–0.0908 | |
p values | |||||||
Transient/DVG- | 0.371 | 0.411 | 0.510 | 0.129 | 0.469 | 0.443 | |
Transient/Prolonged | 1.30×10−5 | 0.206 | 0.249 | 0.001 | 0.493 | 0.519 | |
DVG-/Prolonged | 2.37×10−5 | 0.428 | 0.227 | 0.034 | 0.343 | 0.382 |