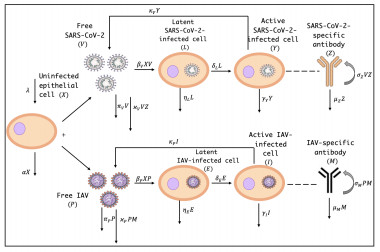
Coronavirus disease 2019 (COVID-19) and influenza are two respiratory infectious diseases of high importance widely studied around the world. COVID-19 is caused by the severe acute respiratory syndrome coronavirus 2 (SARS-CoV-2), while influenza is caused by one of the influenza viruses, A, B, C, and D. Influenza A virus (IAV) can infect a wide range of species. Studies have reported several cases of respiratory virus coinfection in hospitalized patients. IAV mimics the SARS-CoV-2 with respect to the seasonal occurrence, transmission routes, clinical manifestations and related immune responses. The present paper aimed to develop and investigate a mathematical model to study the within-host dynamics of IAV/SARS-CoV-2 coinfection with the eclipse (or latent) phase. The eclipse phase is the period of time that elapses between the viral entry into the target cell and the release of virions produced by that newly infected cell. The role of the immune system in controlling and clearing the coinfection is modeled. The model simulates the interaction between nine compartments, uninfected epithelial cells, latent/active SARS-CoV-2-infected cells, latent/active IAV-infected cells, free SARS-CoV-2 particles, free IAV particles, SARS-CoV-2-specific antibodies and IAV-specific antibodies. The regrowth and death of the uninfected epithelial cells are considered. We study the basic qualitative properties of the model, calculate all equilibria, and prove the global stability of all equilibria. The global stability of equilibria is established using the Lyapunov method. The theoretical findings are demonstrated via numerical simulations. The importance of considering the antibody immunity in the coinfection dynamics model is discussed. It is found that without modeling the antibody immunity, the case of IAV and SARS-CoV-2 coexistence will not occur. Further, we discuss the effect of IAV infection on the dynamics of SARS-CoV-2 single infection and vice versa.
Citation: A. M. Elaiw, Raghad S. Alsulami, A. D. Hobiny. Global dynamics of IAV/SARS-CoV-2 coinfection model with eclipse phase and antibody immunity[J]. Mathematical Biosciences and Engineering, 2023, 20(2): 3873-3917. doi: 10.3934/mbe.2023182
[1] | A. D. Al Agha, A. M. Elaiw . Global dynamics of SARS-CoV-2/malaria model with antibody immune response. Mathematical Biosciences and Engineering, 2022, 19(8): 8380-8410. doi: 10.3934/mbe.2022390 |
[2] | Wenhan Guo, Yixin Xie, Alan E Lopez-Hernandez, Shengjie Sun, Lin Li . Electrostatic features for nucleocapsid proteins of SARS-CoV and SARS-CoV-2. Mathematical Biosciences and Engineering, 2021, 18(3): 2372-2383. doi: 10.3934/mbe.2021120 |
[3] | Tahir Khan, Roman Ullah, Gul Zaman, Jehad Alzabut . A mathematical model for the dynamics of SARS-CoV-2 virus using the Caputo-Fabrizio operator. Mathematical Biosciences and Engineering, 2021, 18(5): 6095-6116. doi: 10.3934/mbe.2021305 |
[4] | Chentong Li, Jinhu Xu, Jiawei Liu, Yicang Zhou . The within-host viral kinetics of SARS-CoV-2. Mathematical Biosciences and Engineering, 2020, 17(4): 2853-2861. doi: 10.3934/mbe.2020159 |
[5] | David Moreno Martos, Benjamin J. Parcell, Raluca Eftimie . Modelling the transmission of infectious diseases inside hospital bays: implications for COVID-19. Mathematical Biosciences and Engineering, 2020, 17(6): 8084-8104. doi: 10.3934/mbe.2020410 |
[6] | Sarafa A. Iyaniwura, Rabiu Musa, Jude D. Kong . A generalized distributed delay model of COVID-19: An endemic model with immunity waning. Mathematical Biosciences and Engineering, 2023, 20(3): 5379-5412. doi: 10.3934/mbe.2023249 |
[7] | Matthew Hayden, Bryce Morrow, Wesley Yang, Jin Wang . Quantifying the role of airborne transmission in the spread of COVID-19. Mathematical Biosciences and Engineering, 2023, 20(1): 587-612. doi: 10.3934/mbe.2023027 |
[8] | Anichur Rahman, Muaz Rahman, Dipanjali Kundu, Md Razaul Karim, Shahab S. Band, Mehdi Sookhak . Study on IoT for SARS-CoV-2 with healthcare: present and future perspective. Mathematical Biosciences and Engineering, 2021, 18(6): 9697-9726. doi: 10.3934/mbe.2021475 |
[9] | Yangyang Yu, Yuan Liu, Shi Zhao, Daihai He . A simple model to estimate the transmissibility of the Beta, Delta, and Omicron variants of SARS-COV-2 in South Africa. Mathematical Biosciences and Engineering, 2022, 19(10): 10361-10373. doi: 10.3934/mbe.2022485 |
[10] | Junyuan Yang, Guoqiang Wang, Shuo Zhang . Impact of household quarantine on SARS-Cov-2 infection in mainland China: A mean-field modelling approach. Mathematical Biosciences and Engineering, 2020, 17(5): 4500-4512. doi: 10.3934/mbe.2020248 |
Coronavirus disease 2019 (COVID-19) and influenza are two respiratory infectious diseases of high importance widely studied around the world. COVID-19 is caused by the severe acute respiratory syndrome coronavirus 2 (SARS-CoV-2), while influenza is caused by one of the influenza viruses, A, B, C, and D. Influenza A virus (IAV) can infect a wide range of species. Studies have reported several cases of respiratory virus coinfection in hospitalized patients. IAV mimics the SARS-CoV-2 with respect to the seasonal occurrence, transmission routes, clinical manifestations and related immune responses. The present paper aimed to develop and investigate a mathematical model to study the within-host dynamics of IAV/SARS-CoV-2 coinfection with the eclipse (or latent) phase. The eclipse phase is the period of time that elapses between the viral entry into the target cell and the release of virions produced by that newly infected cell. The role of the immune system in controlling and clearing the coinfection is modeled. The model simulates the interaction between nine compartments, uninfected epithelial cells, latent/active SARS-CoV-2-infected cells, latent/active IAV-infected cells, free SARS-CoV-2 particles, free IAV particles, SARS-CoV-2-specific antibodies and IAV-specific antibodies. The regrowth and death of the uninfected epithelial cells are considered. We study the basic qualitative properties of the model, calculate all equilibria, and prove the global stability of all equilibria. The global stability of equilibria is established using the Lyapunov method. The theoretical findings are demonstrated via numerical simulations. The importance of considering the antibody immunity in the coinfection dynamics model is discussed. It is found that without modeling the antibody immunity, the case of IAV and SARS-CoV-2 coexistence will not occur. Further, we discuss the effect of IAV infection on the dynamics of SARS-CoV-2 single infection and vice versa.
Severe acute respiratory syndrome coronavirus 2 (SARS-CoV-2) and influenza A virus (IAV) are two respiratory RNA viruses with high pandemic potential. SARS-CoV-2 causes the coronavirus disease 2019 (COVID-19). According to the update provided by the World Health Organization (WHO) on 2 October 2022 [1], over 593 million confirmed cases and over 6.4 million deaths were reported globally. Influenza viruses infect about 20% of the world's population in annual epidemics, resulting in 3-5 million severe illnesses and 290, 000–650, 000 deaths each year [2].
Both SARS-CoV-2 and IAV infect the uninfected epithelial cells of the host respiratory tract [3,4], and have analogous transmission ways. Moreover, they have common clinical manifestations including dyspnea, cough, fever, headache, rhinitis, myalgia and sore throat [5]. Viral shedding usually takes place 5 to 10 days in influenza, whereas it does 2 to 5 weeks in COVID-19 [5]. Acute respiratory distress is less common in influenza than in COVID-19 [5]. Deaths in influenza cases are less than 1%, while in cases of COVID-19 it ranges from 3 to 4% [5]. The precautionary measures implemented by governments to limit the transmission of SARS-CoV-2 can play a role in reducing the transmission of the IAV [6].
Eleven vaccines for COVID-19 were approved by WHO for emergency use. These include Novavax/Nuvaxovid, Bharat Biotech/Covaxin, CanSino/Convidecia, Pfizer/BioNTech/Comirnaty, Moderna/Spikevax, Serum Institute of India COVOVAX (Novavax formulation), Janssen (Johnson & Johnson)/Jcovden, Oxford/AstraZeneca/Vaxzevria, Serum Institute of India Covishield (Oxford/AstraZeneca formulation), Sinopharm (Beijing)/Covilo, and Sinovac/CoronaVac [7]. Currently, there are three types of influenza vaccines used worldwide: live attenuated influenza vaccine, inactivated influenza vaccine and recombinant hemagglutinin vaccine [8].
It was reported in [9] that, 94.2% of individuals with COVID-19 were also coinfected with several other microorganisms, such as fungi, bacteria and viruses. Important viral copathogens include the respiratory syncytial virus (RSV), human enterovirus (HEV), human rhinovirus (HRV), influenza A virus (IAV), influenza B virus (IBV), human metapneumovirus (HMPV), parainfluenza virus (PIV), human immunodeficiency virus (HIV), cytomegalovirus (CMV), dengue virus (DENV), Epstein Barr virus (EBV), hepatitis B virus (HBV) and other coronaviruses (COVs), among which the HRV, HEV and IAV are the most common copathogens [10]. Several coinfection cases of COVID-19 and influenza were reported in [5,9,11,12,13] (see also the review papers [14,15,16,17,18]). Lansbury et al. [14] presented a systematic review and meta-analysis that included 30 studies for evaluating coinfections among patients infected with COVID-19. It was reported that 7% of patients had a bacterial coinfection and 3% of patients had another respiratory virus, with RSV and IAV being among the most common coinfecting viruses. Dao et al. [15] conducted a systematic review and meta-analysis that included 54 publications and found that, 7% of COVID-19 patients are co-infected with influenza viruses. Most influenza co-infections were due to the IAV [15]. A respective study in Wuhan, China showed that the coinfection rate of IAV and SARS-CoV-2 was 49.8% during the outbreak period of COVID-19 [19]. Based on two separate studies presented in [11] and [12], COVID-19-influenza coinfection did not result in worse clinical outcomes [11]. In addition, this condition reduced the mortality rate among COVID-19-influenza coinfected patients. Coinfection with influenza virus in COVID-19 patients might render them less vulnerable to morbidities associated with COVID-19, and therefore, a better prognosis overall [12]. In [18], it is found that, although patients with IAV and SARS-CoV-2 coinfection did not experience longer hospital stays compared with those SARS COV-2 single-infection, they usually presented with a more severe clinical conditions. In an animal study [20], it was found that the disease severity is increased in hamsters with SARS-CoV-2 and IAV coinfection compared with those with SARS-CoV-2 mono-infection.
Viral interference phenomenon can appear in case of infections with multiple competitive respiratory viruses [21,22,23]. One virus may be able to suppress the growth of another virus [21,24]. In [22], it was reported that an H3N2 strain of IAV was inhibited by SARS-CoV-2 coinfection in the hamster model. Oishi et al. [23] used the golden Syrian hamster model, and found that, IAV interferes with SARS-CoV-2 replication in the lung, even more than one week after IAV clearance. Disease progression and outcome in SARS-CoV-2 infection are highly dependent on the host immune response, particularly in the elderly in whom immunosenescence may predispose to increased risk of coinfection [21].
Over the years, mathematical models have demonstrated their ability to provide useful insight to gain a further understanding of the dynamics and mechanisms of the viruses within a host level. These models may assist in the development of viral therapies and vaccines as well as the selection of appropriate therapeutic and vaccine strategies. Moreover, these models are helpful in determining the sufficient number of factors to analyze the experimental results and explain the biological phenomena [3]. Stability analysis of the model's equilibria can help researchers to (i) expect the qualitative features of the model for a given set of values of the model's parameters, (ii) establish the conditions that ensure the persistence or deletion of this infection, and (iii) determine under what conditions the immune system is stimulated against the infection.
Mathematical models of within-host IAV single-infection were developed in several works (see the review papers [25,26,27,28,29]). Baccam et al. [30] presented the following IAV-single-infection with limited target cells and eclipse (or latent) phase:
{˙X=−IAV infectious transmission⏞βPXP,˙E=IAV infectious transmission⏞βPXP−latent transition⏞δEE,˙I=latent transition⏞δEE−natural death⏞γII,˙P=IAV production⏞κPI−natural death⏞πPP, | (1.1) |
where X=X(t), E=E(t), I=I(t) and P=P(t) are the concentrations of uninfected epithelial cells, latent IAV-infected epithelial cells, active IAV-infected epithelial cells and free IAV particles, at time t, respectively. The model was fitted using real data from six patients infected with influenza [30].
Several works were devoted to developing IAV single-infection dynamics models by considering the following:
● Innate immune response: It represents the first line of defense that recognizes the antigens and activate the adaptive immune response. In [30], the effect of interferon (IFN) response was included in the IAV infection model. The dynamics of the IFN are given as:
˙F=ϖFI(t−τ)−μFF, |
where, F is the concentration of IFN, ϖF is the IFN production rate constant, μF is the IFN decay rate constant, and τ is the time lag that occurs between the initiation of an IAV infection and the appearance of IFN. IFN can reduce viral replication in an infected cell, the rate of viral production in the presence of IFN was modeled by replacing δE by δE1+ϵEF. The rate that IAV-infected cells in the eclipse phase begin virus production (κP) may also be altered and was accounted for by replacing this parameter by κP1+ϵPF. The efficiency of these interferon effects is reflected by the parameters ϵE and ϵP [30,31]. Saenz et al. [32] presented an influenza model with interferon response. It is reported that the model with interferon response provided better fitting with real data than that without interferon response.
● Adaptive immune response: Cytotoxic T Lymphocytes (CTLs) and antibodies are the two major components of the adaptive immune response. CTLs destroy the viral-infected cells, while the antibodies neutralize the viruses. An influenza dynamics model with different forms of the CTL response was developed in [33]. It was shown that slight changes in the virus dynamics was observed when different choices of CTL response were implemented. Both CTL and antibody immunities were included into the IAV model in [34].
● Both innate and adaptive immune responses [3,35,36,37,38]. The model presented in [37] predicted that, the level and time of the viral peak are affected by the innate interferon response, while the clearance phase and duration of infection are determined by the CTL response. Handel et al. [38] showed that, both the innate and adaptive immune responses are required to give an appropriate explanation of the real data.
● Drug therapy: There are two approved anti-IAV drugs, adamantane antiviral drugs which block infection by reducing the rate of infection, and neuraminidase inhibitors which block the production of newly formed virions [31]. Beauchemin et al. [39] used model (1.1) to study the effect of the antiviral drug amantadine on IAV infection. Handel et al. [40] presented a mathematical model for within-host influenza infection under the effect of neuraminidase inhibitors drugs. Lee et al. [34] included the effect of a combination of neuraminidase inhibitors and anti-IAV therapies in the IAV model. The IAV model predicts that the drug therapies are more beneficial when they are administered early.
● Regrowth and death of the uninfected epithelial cells. In [34], the first equation of model (1.1) was modified by considering the target cell production and death as:
˙X=epithelial cells production⏞αX(0)−natural death⏞αX−IAV infectious transmission⏞βPXP, | (1.2) |
where X(0) is the initial concentration of the uninfected epithelial cells.
Mathematical analysis of within-host IAV infection model was studied in a few papers [33,41,42].
Model (1.1) was utilized to characterize the dynamics of SARS-CoV-2 within a host in [43,44,45]. Li et al. [46], used Eq (1.2) for the uninfected epithelial cell dynamics in case of SARS-CoV-2 infection. The model with target-cell limited and model with regrowth and death of the uninfected epithelial cells presented, respectively, in [43,46] were extended and modified by including (i) effect of immune response [44,47,48,49,50,51], (ii) effect of different drug therapies [52,53], and (iii) effect of time delay [54].
Stability analysis of within-host SARS-CoV-2 single-infection models was investigated in [49,50,51,55].
Mathematical model of IAV/SARS-CoV-2 coinfection. Recently, mathematical models were developed to characterize within-host co-dynamics of COVID-19 with other diseases, such as: SARS-CoV-2-cancer [56], SARS-CoV-2/HIV coinfection [57], SARS-CoV-2/malaria coinfection [58]. Based on the target cell-limited model (1.1), and the Pinky and Dobrovolny [24] developed a model for the within-host dynamics of two respiratory viruses coinfection (SARS-CoV-2 and IAV).
{˙X=−SARS-CoV-2 infectious transmission⏞βVXV−IAV infectious transmission⏞βPXP,˙L=SARS-CoV-2 infectious transmission⏞βVXV−latent transition⏞δLL,˙E=IAV infectious transmission⏞βPXP−latent transition⏞δEE,˙Y=latent transition⏞δLL−natural death⏞γYY,˙I=latent transition⏞δEE−natural death⏞γII,˙V=SARS-CoV-2 production⏞κVY−natural death⏞πVV,˙P=IAV production⏞κPI−natural death⏞πPP, | (1.3) |
where L=L(t), Y=Y(t) and V=V(t) are the concentrations of latent SARS-CoV-2-infected epithelial cells, active SARS-CoV-2-infected epithelial cells and free SARS-CoV-2 particles, at time t, respectively. Model (1.3) describes the competition between two respiratory viruses, SARS-CoV-2 and IAV. However, the effect of the immune response was not modeled. Further, the regrowth and death of the uninfected epithelial cells were not considered. Furthermore, mathematical analysis of the model was not studied.
The objective of the present work is to formulate a mathematical model for within-host IAV/SARS-CoV-2 coinfection with eclipse phase. The model is a generalization of the model (1.3) by taking into account (i) the regrowth and death of the uninfected epithelial cells, (ii) the death of the latent SARS-CoV-2-infected cells and latent IAV-infected cells, (iii) the effect of SARS-CoV-2-specific antibody and IAV-specific antibody. We study the basic qualitative properties of the model, calculate all equilibria, investigate the global stability of equilibria and demonstrate the theoretical results via numerical simulations. We discuss the importance of including the antibody immunity in the IAV/SARS-CoV-2 co-infection model.
Our proposed model can be helpful to characterize the dynamics of coinfection with SARS-CoV-2 strains (Alpha, Beta, Gamma, Delta, Lambda and Omicron), or coinfection of SARS-CoV-2 (or IAV) and other respiratory viruses. Moreover, the model may help to predict new treatment regimens for viral coinfections.
In this section, we present an IAV/SARS-CoV-2 coinfection dynamics model with a latent phase. The dynamics of IAV/SARS-CoV-2 coinfection is presented in the diagram Figure 1. We denote Z=Z(t) and M=M(t) for the concentrations of SARS-CoV-2-specific antibodies and IAV-specific antibodies, at time t, respectively. The ODEs that describe the coinfection dynamics are:
{˙X=epithelial cells production⏞λ−natural death⏞αX−SARS-CoV-2 infectious transmission⏞βVXV−IAV infectious transmission⏞βPXP,˙L=SARS-CoV-2 infectious transmission⏞βVXV−natural death⏞ηLL−latent transition⏞δLL,˙E=IAV infectious transmission⏞βPXV−natural death⏞ηEE−latent transition⏞δEE,˙Y=latent transition⏞δLL−natural death⏞γYY,˙I=latent transition⏞δEE−natural death⏞γII,˙V=SARS-CoV-2 production⏞κVY−natural death⏞πVV−SARS-CoV-2 neutralization⏞ϰVVZ,˙P=IAV production⏞κPI−natural death⏞πPP−IAV neutralization⏞ϰPPM,˙Z=SARS-CoV-2-specific antibody proliferation⏞σZVZ−natural death⏞μZZ,˙M=IAV-specific antibody proliferation⏞σMPM−natural death⏞μMM. | (2.1) |
where (X,L,E,Y,I,V,P,Z,M)=(X(t),L(t),E(t),Y(t),I(t),V(t),P(t),Z(t),M(t)).
In model (2.1) the regrowth death of the uninfected epithelial cells is considered. Further, the death of the latent SARS-CoV-2-infected and latent IAV-infected cells are included, Furthermore, the effect of SARS-CoV-2-specific and IAV-specific antibodies are modeled. First, we start our mathematical analysis of the system by examining the nonnegativity and boundedness of the system's solutions.
Here, we study the basic qualitative properties of system (2.1).
Lemma 1. The solutions of system (2.1) are nonnegative and bounded.
Proof. We have that
˙X∣X=0=λ>0,˙L∣L=0=βVXV≥0 for all X,V≥0,˙E∣E=0=βPXP≥0 for all X,P≥0,˙Y∣Y=0=δLL for all L≥0,˙I∣I=0=δEE for all E≥0,˙V∣V=0=κVY≥0 for all Y≥0,˙P∣P=0=κPI≥0 for all I≥0,˙Z∣Z=0=0,˙M∣M=0=0. |
This guarantees that, (X(t),L(t),E(t),Y(t),I(t),V(t),P(t),Z(t),M(t))∈R9≥0 for all t≥0 when (X(0),L(0),E(0),Y(0),I(0),V(0),P(0),Z(0),M(0))∈R9≥0. Let us define
Ψ=X+L+E+Y+I+γY2κVV+γI2κPP+γYϰV2κVσZZ+γIϰP2κPσMM. |
Then
˙Ψ=λ−αX−ηLL−ηEE−γY2Y−γI2I−γYπV2κVV−γIπP2κPP−γYϰVμZ2κVσZZ−γIϰPμM2κPσMM≤λ−ϕ[X+L+E+Y+I+γY2κVV+γI2κPP+γYϰV2κVσZZ+γIϰP2κPσMM]=λ−ϕΨ, |
where ϕ=min{α,ηL,ηE,γY2,γI2,πV,πP,μZ,μM}. Hence, 0≤Ψ(t)≤Δ1 if Ψ(0)≤Δ1 for t≥0, where Δ1=λϕ. Since X,L,E,Y,I,V,P,Z and M are all nonnegative, then 0≤X(t),L(t),E(t),Y(t),I(t)≤Δ1, 0≤V(t)≤Δ2, 0≤P(t)≤Δ3, 0≤Z(t)≤Δ4, 0≤M(t)≤Δ5 if X(0)+L(0)+E(0)+Y(0)+I(0)+γY2κVV(0)+γI2κPP(0)+γYϰV2κVσZZ(0)+γIϰP2κPσMM(0)≤Δ1, where Δ2=2κVγYΔ1, Δ3=2κPγIΔ1, Δ4=2σZκVϰVγYΔ1 and Δ5=2σMκPϰPγIΔ1. This proves the boundedness of the solutions.
Here, we calculate the system's equilibria and deduce the conditions of their existence. Any equilibrium point Ξ=(X,L,E,Y,I,V,P,Z,M) satisfies:
0=λ−αX−βVXV−βPXP, | (4.1) |
0=βVXV−(ηL+δL)L, | (4.2) |
0=βPXP−(ηE+δE)E, | (4.3) |
0=δLL−γYY, | (4.4) |
0=δEE−γII, | (4.5) |
0=κVY−πVV−ϰVVZ, | (4.6) |
0=κPI−πPP−ϰPPM, | (4.7) |
0=σZVZ−μZZ, | (4.8) |
0=σMPM−μMM. | (4.9) |
Solving Eqs (4.1)–(4.9), we get eight equilibria.
(i) Infection-free equilibrium, Ξ0=(X0,0,0,0,0,0,0,0,0), where X0=λ/α.
(ii) SARS-CoV-2 single-infection equilibrium without antibody immunity Ξ1=(X1,L1,0,Y1,0,V1,0,0,0), where
X1=γYπV(ηL+δL)κVβVδL, L1=αγYπVκVβVδL[X0κVβVδLγYπV(ηL+δL)−1],Y1=απVκVβV[X0κVβVδLγYπV(ηL+δL)−1], V1=αβV[X0κVβVδLγYπV(ηL+δL)−1]. |
Therefore, L1>0,Y1>0 and V1>0 when X0κVβVδLγYπV(ηL+δL)>1. We define the basic SARS-CoV-2 single-infection reproductive ratio as:
ℜ1=X0κVβVδLγYπV(ηL+δL). |
The parameter ℜ1 determines whether or not a SARS-CoV-2 single-infection can be established. Thus, we can write
X1=X0ℜ1, L1=αγYπVκVβVδL(ℜ1−1),Y1=απVκVβV(ℜ1−1), V1=αβV(ℜ1−1). |
It follows that, Ξ1 exists if ℜ1>1.
(iii) IAV single-infection equilibrium without antibody immunity, Ξ2=(X2,0,E2,0,I2,0,P2,0,0), where
X2=γIπP(ηE+δE)κPβPδE, E2=αγIπPκPβPδE[X0κPβPδEγIπP(ηE+δE)−1],I2=απPκPβP[X0κPβPδEγIπP(ηE+δE)−1], P2=αβP[X0κPβPδEγIπP(ηE+δE)−1]. |
Therefore, E2>0,I2>0 and P2>0 when X0κPβPδEγIπP(ηE+δE)>1. We define the basic IAV-infection reproductive ratio as:
ℜ2=X0κPβPδEγIπP(ηE+δE). |
The parameter ℜ2, determines whether or not the IAV single-infection can be established. In terms of ℜ2, we can write:
X2=X0ℜ2, E2=αγIπPκPβPδE(ℜ2−1),I2=απPκPβP(ℜ2−1), P2=αβP(ℜ2−1). |
Therefore, Ξ2 exists if ℜ2>1
(iv) SARS-CoV-2 single-infection equilibrium with stimulated SARS-CoV-2-specific antibody immunity, Ξ3=(X3,L3,0,Y3,0,V3,0,Z3,0), where
X3=λσZβVμZ+ασZ, L3=λβVμZ(ηL+δL)(βVμZ+ασZ), Y3=λβVμZδLγY(ηL+δL)(βVμZ+ασZ),V3=μZσZ, Z3=πVϰV[λβVσZκVδLγYπV(ηL+δL)(βVμZ+ασZ)−1]. |
We note that Ξ3 exists when λβVσZκVδLγYπV(ηL+δL)(βVμZ+ασZ)>1. Let us define the SARS-CoV-2-specific antibody activation ratio in case of SARS-CoV-2 single-infection as:
ℜ3=λβVσZκVδLγYπV(ηL+δL)(βVμZ+ασZ). |
Thus, Z3=πVϰV(ℜ3−1). The parameter ℜ3 determines whether or not the SARS-CoV-2-specific antibody immunity is activated in the absence of IAV infection.
(v) IAV single-infection equilibrium with stimulated of IAV-specific antibody immunity, Ξ4=(X4,0,E4,0,I4,0,P4,0,M4), where
X4=λσMβPμM+ασM, E4=λβPμM(ηE+δE)(βPμM+ασM), I4=λβPμMδEγI(ηE+δE)(βPμM+ασM), P4=μMσM, M4=πPϰP[λβPσMκPδEγIπP(ηE+δE)(βPμM+ασM)−1]. |
We note that Ξ4 exists when λβPσMκPδEγIπP(ηE+δE)(βPμM+ασM)>1. We define the IAV-specific antibody immunity activation ratio for IAV single-infection as:
ℜ4=λβPσMκPδEγIπP(ηE+δE)(βPμM+ασM). |
Thus, M4=πPϰP(ℜ4−1). The parameter ℜ4 determines whether or not the IAV-specific antibody immunity is activated in the absence of SARS-CoV-2 infection.
(vi) IAV/SARS-CoV-2 coinfection equilibrium with only stimulated SARS-CoV-2-specific antibody immunity, Ξ5=(X5,L5,E5,Y5,I5,V5,P5,Z5,0), where
X5=γIπP(ηE+δE)κPβPδE, L5=βVμZγIπP(ηE+δE)κPβPδEσZ(ηL+δL),E5=γIπP(βVμZ+ασZ)κPβPδEσZ[λβPκPδEσZγIπP(ηE+δE)(βVμZ+ασZ)−1], Y5=βVμZγIπPδL(ηE+δE)κPβPδEσZγY(ηL+δL),I5=πP(βVμZ+ασZ)κPβPσZ[λβPκPδEσZγIπP(ηE+δE)(βVμZ+ασZ)−1], V5=μZσZ,P5=βVμZ+ασZβPσZ[λβPκPδEσZγIπP(ηE+δE)(βVμZ+ασZ)−1],Z5=πVϰV[κVβVγIδLπP(ηE+δE)κPβPγYδEπV(ηL+δL)−1]=πVϰV(ℜ1/ℜ2−1). |
We note that Ξ5 exists when,
ℜ1ℜ2>1 and λβPκPδEσZγIπP(ηE+δE)(βVμZ+ασZ)>1. |
Now, we define the SARS-CoV-2 infection reproductive ratio in the presence of IAV infection as:
ℜ5=λβPκPδEσZγIπP(ηE+δE)(βVμZ+ασZ). |
The parameter ℜ5 determines whether or not SARS-CoV-2-infected patients could be coinfected with IAV. Hence,
E5=γIπP(βVμZ+ασZ)κPβPδEσZ(ℜ5−1), I5=πP(βVμZ+ασZ)βPσZκP(ℜ5−1),P5=βVμZ+ασZβPσZ(ℜ5−1). |
and then Ξ5 exists if ℜ1ℜ2>1 and ℜ5>1.
(vii) IAV/SARS-CoV-2 coinfection equilibrium with only stimulated IAV-specific antibody immunity, Ξ6=(X6,L6,E6,Y6,I6,V6,P6,0,M6), where
X6=γYπV(ηL+δL)κVβVδL, L6=γYπV(βPμM+ασM)κVβVδLσM[λβVκVδLσMγYπV(ηL+δL)(βPμM+ασM)−1],E6=γYβPμMπV(ηL+δL)κVβVδLσM(ηE+δE), Y6=πV(βPμM+ασM)κVβVσM[λβVκVδLσMγYπV(ηL+δL)(βPμM+ασM)−1],I6=βPδEμMπVγY(ηL+δL)κVβVδLσMγI(ηE+δE), V6=βPμM+ασMβVσM[λβVκVδLσMγYπV(ηL+δL)(βPμM+ασM)−1],P6=μMσM, M6=πPϰP[κPβPγYδEπV(ηL+δL)κVβVγIδLπP(ηE+δE)−1]=πPϰP(ℜ2/ℜ1−1). |
We note that Ξ6 exists when
ℜ2ℜ1>1 and λβVκVδLσMγYπV(ηL+δL)(βPμM+ασM)>1. |
We define the SARS-CoV-2 infection reproductive ratio in the presence of IAV infection as:
ℜ6=λβVκVδLσMγYπV(ηL+δL)(βPμM+ασM). |
Thus,
L6=γYπV(βPμM+ασM)κVβVδLσM(ℜ6−1), Y6=πV(βPμM+ασM)βVσMκV(ℜ6−1),V6=βPμM+ασMβVσM(ℜ6−1). |
The parameter ℜ6 determines whether or not SARS-CoV-2-infected patients could be coinfected with IAV.
(viii) IAV/SARS-CoV-2 coinfection equilibrium with stimulated both SARS-CoV-2-specific and IAV-specific antibody immunities, Ξ7=(X7,L7,E7,Y7,I7,V7,P7,Z7,M7), where
X7=λσZσMβPμMσZ+βVμZσM+ασZσM, L7=βVλμZσM(ηL+δL)(βPμMσZ+βVμZσM+ασZσM),E7=βPλμMσZ(ηE+δE)(βPμMσZ+βVμZσM+ασZσM),Y7=βVδLλμZσMγY(ηL+δL)(βPμMσZ+βVμZσM+ασZσM),I7=βPδEλμMσZγI(ηE+δE)(βPμMσZ+βVμZσM+ασZσM), V7=μZσZ, P7=μMσM,Z7=πVϰV[λβVκVδLσMσZγYπV(ηL+δL)(βPμMσZ+βVμZσM+ασZσM)−1],M7=πPϰP[λβPκPδEσMσZγIπP(ηE+δE)(βPμMσZ+βVμZσM+ασZσM)−1]. |
It is obvious that Ξ7 exists when
λβVκVδLσMσZγYπV(ηL+δL)(βPμMσZ+βVμZσM+ασZσM)>1, λβPκPδEσMσZγIπP(ηE+δE)(βPμMσZ+βVμZσM+ασZσM)>1. |
Now, we define
ℜ7=λβVκVδLσMσZγYπV(ηL+δL)(βPμMσZ+βVμZσM+ασZσM),ℜ8=λβPκPδEσMσZγIπP(ηE+δE)(βPμMσZ+βVμZσM+ασZσM). |
Here, ℜ7 is the SARS-CoV-2-specific antibody activation ratio in case of IAV/SARS-CoV-2 coinfection, ℜ8 is the IAV-specific antibody activation ratio in case of IAV/SARS-CoV-2 coinfection. Hence, Z7=πVϰV(ℜ7−1) and M7=πPϰP(ℜ8−1). If ℜ7>1 and ℜ8>1, then Ξ7 exists.
In summary, we have eight threshold parameters which determine the existence of the model's equilibria
![]() |
(4.10) |
This section is devoted to studying the global asymptotic stability of all equilibria. We configure Lyapunov functions following the way outlined in [59]. The following arithmetic-mean-geometric-mean inequality will be utilized:
u1+u2+...+unn≥n√(u1)(u2)...(un), ui≥0, i=1,2,...,n. | (5.1) |
Let a function Λj(X,L,E,Y,I,V,P,Z,M) and ˜Ωj be the largest invariant subset of
Ωj={(X,L,E,Y,I,V,P,Z,M):dΛjdt=0}, j=0,1,...,7. |
Define a function
ϝ(υ)=υ−1−lnυ, υ>0. |
Theorem 1. If ℜ1≤1 and ℜ2≤1, then Ξ0 is globally asymptotically stable (G.A.S).
Proof. Define
Λ0=X0ϝ(XX0)+L+E+ηL+δLδLY+ηE+δEδEI+βVX0πVV+βPX0πPP+βVX0ϰVσZπVZ+βPX0ϰPσMπPM. |
We note that, Λ0>0 for all X,L,E,Y,I,V,P,Z,M>0 and Λ0(X0,0,0,0,0,0,0,0,0)=0. We calculate dΛ0dt along the solutions of model (2.1) as:
dΛ0dt=(1−X0X)[λ−αX−βVXV−βPXP]+βVXV−(ηL+δL)L+βPXP−(ηE+δE)E+ηL+δLδL[δLL−γYY]+ηE+δEδE[δEE−γII]+βVX0πV[κVY−πVV−ϰVVZ]+βPX0πP[κPI−πPP−ϰPPM]+βVX0ϰVσZπV[σZVZ−μZZ]+βPX0ϰPσMπP[σMPM−μMM]=(1−X0X)(λ−αX)−γY(ηL+δL)δLY−γI(ηE+δE)δEI+κVβVX0πVY+κPβPX0πPI−βVX0ϰVμZπVσZZ−βPX0ϰPμMπPσMM. |
Using the equilibrium condition, λ=αX0 we get
dΛ0dt=−α(X−X0)2X+γY(ηL+δL)δL(κVβVδLX0γYπV(ηL+δL)−1)Y+γI(ηE+δE)δE(κPβPδEX0γIπP(ηE+δE)−1)I−βVX0ϰVμZπVσZZ−βPX0ϰPμMπPσMM=−α(X−X0)2X+γY(ηL+δL)δL(ℜ1−1)Y+γI(ηE+δE)δE(ℜ2−1)I−βVX0ϰVμZπVσZZ−βPX0ϰPμMπPσMM. |
Since ℜ1≤1 and ℜ2≤1, then dΛ0dt≤0 for all X,Y,I,Z,M>0. In addition dΛ0dt=0, when X=X0 and Y=I=Z=M=0. The solutions of system (2.1) tend to ˜Ω0 [60] which includes elements with Y=I=0. Thus, ˙Y=˙I=0 and from the fourth and fifth equations of system (2.1) we have:
0=˙Y=δLL⟹L(t)=0, for all t,0=˙I=δEE⟹E(t)=0, for all t. |
In addition, from the second and third equations of system (2.1) we have:
0=˙L=βVX0V⟹V(t)=0, for all t,0=˙E=βPX0P⟹P(t)=0, for all t. |
Therefore, ˜Ω0={Ξ0} and applying Lyapunov-LaSalle Asymptotic Stability Theorem (L-LAST) [61,63], we obtain that Ξ0 is G.A.S.
Theorem 2. Suppose that ℜ1>1, ℜ2/ℜ1≤1 and ℜ3≤1, then Ξ1 is G.A.S.
Proof. Let us formulate a Lyapunov function Λ1 as:
Λ1=X1ϝ(XX1)+L1ϝ(LL1)+E+ηL+δLδLY1ϝ(YY1)+ηE+δEδEI+βVX1πVV1ϝ(VV1)+βPX1πPP+βVX1ϰVσZπVZ+βPX1ϰPσMπPM. |
We calculate dΛ1dt as:
dΛ1dt=(1−X1X)[λ−αX−βVXV−βPXP]+(1−L1L)[βVXV−(ηL+δL)L]+βPXP−(ηE+δE)E+ηL+δLδL(1−Y1Y)[δLL−γYY]+ηE+δEδE[δEE−γII]+βVX1πV(1−V1V)[κVY−πVV−ϰVVZ]+βPX1πP[κPI−πPP−ϰPPM]+βVX1ϰVσZπV[σZVZ−μZZ]+βPX1ϰPσMπP[σMPM−μMM]. | (5.2) |
Simplifying Eq (5.2), we get
dΛ1dt=(1−X1X)(λ−αX)−βVXVL1L+(ηL+δL)L1−γY(ηL+δL)δLY−(ηL+δL)LY1Y+γY(ηL+δL)δLY1−γI(ηE+δE)δEI+βVX1κVπVY−βVX1κVπVYV1V+βVX1V1+βVX1ϰVπVV1Z+βPX1κPπPI−βVX1ϰVμZσZπVZ−βPX1ϰPμMσMπPM. |
Using the equilibrium conditions for Ξ1:
λ=αX1+βVX1V1, βVX1V1=(ηL+δL)L1,L1=γYδLY1, V1=κVπVY1, |
we obtain
dΛ1dt=(1−X1X)(αX1−αX)+4βVX1V1−βVX1V1X1X−βVX1V1L1XVLX1V1−βVX1V1Y1LYL1−βVX1V1V1YVY1+γI(ηE+δE)δE(βPX1κPδEγIπP(ηE+δE)−1)I+βVX1ϰVμZσZπV(σZμZV1−1)Z−βPX1ϰPμMσMπPM. | (5.3) |
Then collecting terms of (5.3), we get
dΛ1dt=−α(X−X1)2X+βVX1V1[4−X1X−L1XVLX1V1−Y1LYL1−V1YVY1]+γI(ηE+δE)δE(ℜ2ℜ1−1)I+ϰVX1(ασZ+βVμZ)σZπV(ℜ3−1)Z−βPX1ϰPμMσMπPM. |
Using inequality (5.1), we get
4−X1X−L1XVLX1V1−Y1LYL1−V1YVY1≤0. |
Since ℜ2/ℜ1≤1 and ℜ3≤1, then dΛ1dt≤0 for all X,L,Y,I,V,Z,M>0. Moreover, dΛ1dt=0 when X=X1, L=L1,Y=Y1, V=V1 and I=Z=M=0. The solutions of system (2.1) tend to ˜Ω1 where I=0. Hence, ˙I=0 and the fifth equation of system (2.1) gives
0=˙I=δEE⟹E(t)=0, for all t. |
In addition, from the third equation of system (2.1) we get,
0=˙E=βPX1P⟹P(t)=0, for all t. |
Hence, ˜Ω1={Ξ _{1} } and Ξ1 is G.A.S by using L-LAST [61,62,63].
Theorem 3. Let ℜ2>1, ℜ1/ℜ2≤1 and ℜ4≤1, then Ξ2 is G.A.S.
Proof. Consider
Λ2=X2ϝ(XX2)+L+E2ϝ(EE2)+ηL+δLδLY+ηE+δEδEI2ϝ(II2)+βVX2πVV+βPX2πPP2ϝ(PP2)+βVX2ϰVσZπVZ+βPX2ϰPσMπPM. |
We calculate dΛ2dt as:
dΛ2dt=(1−X2X)[λ−αX−βVXV−βPXP]+βVXV−(ηL+δL)L+(1−E2E)[βPXP−(ηE+δE)E]+ηL+δLδL[δLL−γYY]+ηE+δEδE(1−I2I)[δEE−γII]+βVX2πV[κVY−πVV−ϰVVZ]+βPX2πP(1−P2P)[κPI−πPP−ϰPPM]+βVX2ϰVσZπV[σZVZ−μZZ]+βPX2ϰPσMπP[σMPM−μMM]. | (5.4) |
Then simplifying Eq (5.4), we get
dΛ2dt=(1−X2X)(λ−αX)−βPXPE2E+(ηE+δE)E2−γY(ηL+δL)δLY−γI(ηE+δE)δEI−(ηE+δE)EI2I+γI(ηE+δE)δEI2+κVπVβVX2Y+κPπPβPX2I−κPπPβPX2IP2P+βPX2P2+ϰPπPβPX2P2M−ϰVμZσZπVβVX2Z−ϰPμMσMπPβPX2M. |
Using the equilibrium conditions for Ξ2:
λ=αX2+βPX2P2, βPX2P2=(ηE+δE)E2,E2=γIδEI2, P2=κPπPI2, |
we obtain,
dΛ2dt=(1−X2X)(αX2−αX)+4βPX2P2−βPX2P2X2X−βPX2P2E2XPEX2P2−βPX2P2I2EIE2−βPX2P2P2IPI2+γY(ηL+δL)δL(δLκVβVX2γYπV(ηL+δL)−1)Y+βPX2ϰPμMσMπP(σMμMP2−1)M−βVX2ϰVμZσZπVZ=−α(X−X2)2X+βPX2P2(4−X2X−E2XPEX2P2−I2EIE2−P2IPI2)+γY(ηL+δL)δL(ℜ1ℜ2−1)Y+X2ϰP(ασM+βPμM)πPσM(ℜ4−1)M−βVX2ϰVμZσZπVZ. |
If \Re_{1}/\Re_{2}\leq1 and \Re_{4}\leq1 , then employing inequality (5.1), we get \frac{d\Lambda_{2}}{dt}\leq0 for all X, E, Y, I, P, Z, M > 0 . Further, \frac{d\Lambda_{2}}{dt} = 0 when X = X_{2}, \; E = E_{2} , I = I_{2}, P = P_{2} and Y = Z = M = 0. The solutions of system (2.1) tend to \tilde{\Omega}_{2} which has Y = 0 , and gives \dot{Y} = 0 . The fourth equation of system (2.1) gives
0 = \dot{Y} = \delta_{L}L\Longrightarrow L(t) = 0, \text{ for all }t. |
In addition, from the second equation of system (2.1) gives
0 = \dot{L} = \beta_{V}X_{2}V\Longrightarrow V(t) = 0, \text{ for all }t. |
Therefore, \tilde{\Omega}_{2} = \left \{ \Xi \text{ _{2} }\right \} . Applying L-LAST, we get \Xi_{2} is G.A.S.
Theorem 4. Let \Re_{3} > 1 and \Re_{5}\leq1 , then \Xi_{3} is G.A.S.
Proof. Define
\begin{array}{l} \Lambda_{3} = X_{3}\digamma \left( \frac{X}{X_{3}}\right) +L_{3} \digamma \left( \frac{L}{L_{3}}\right) +E+\frac{\eta_{L}+\delta_{L}} {\delta_{L}}Y_{3}\digamma \left( \frac{Y}{Y_{3}}\right) +\frac{\eta _{E}+\delta_{E}}{\delta_{E}}I\\ +\frac{\gamma_{Y}\left( \eta_{L}+\delta_{L}\right) }{\kappa_{V}\delta _{L}}V_{3}\digamma \left( \frac{V}{V_{3}}\right) +\frac{\gamma_{I}\left( \eta_{E}+\delta_{E}\right) }{\kappa_{P}\delta_{E}}P+\frac{\gamma_{Y} \varkappa_{V}\left( \eta_{L}+\delta_{L}\right) }{\kappa_{V}\delta_{L} \sigma_{Z}}Z_{3}\digamma \left( \frac{Z}{Z_{3}}\right) +\frac{\gamma _{I}\varkappa_{P}\left( \eta_{E}+\delta_{E}\right) }{\kappa_{P}\delta _{E}\sigma_{M}}M. \end{array} |
We calculate \frac{d\Lambda_{3}}{dt} as:
\begin{array}{l} \frac{d\Lambda_{3}}{dt} = \left( 1-\frac{X_{3}}{X}\right) [\lambda-\alpha X-\beta_{V}XV-\beta_{P}XP]+\left( 1-\frac{L_{3}}{L}\right) [\beta _{V}XV-\left( \eta_{L}+\delta_{L}\right) L]+\beta_{P}XP \\ -\left( \eta_{E}+\delta_{E}\right) E+\frac{\eta_{L}+\delta_{L}} {\delta_{L}}\left( 1-\frac{Y_{3}}{Y}\right) \left[ \delta_{L}L-\gamma _{Y}Y\right] +\frac{\eta_{E}+\delta_{E}}{\delta_{E}}\left[ \delta _{E}E-\gamma_{I}I\right] \\ +\frac{\gamma_{Y}\left( \eta_{L}+\delta_{L}\right) }{\kappa_{V}\delta _{L}}\left( 1-\frac{V_{3}}{V}\right) \left[ \kappa_{V}Y-\pi_{V} V-\varkappa_{V}VZ\right] +\frac{\gamma_{I}\left( \eta_{E}+\delta_{E}\right) }{\kappa_{P}\delta_{E}}\left[ \kappa_{P}I-\pi_{P}P-\varkappa_{P}PM\right] \\ +\frac{\gamma_{Y}\varkappa_{V}\left( \eta_{L}+\delta_{L}\right) } {\kappa_{V}\delta_{L}\sigma_{Z}}\left( 1-\frac{Z_{3}}{Z}\right) \left[ \sigma_{Z}VZ-\mu_{Z}Z\right] +\frac{\gamma_{I}\varkappa_{P}\left( \eta _{E}+\delta_{E}\right) }{\kappa_{P}\delta_{E}\sigma_{M}}\left[ \sigma _{M}PM-\mu_{M}M\right] . \end{array} | (5.5) |
Then simplifying Eq (5.5), we get
\begin{align*} \frac{d\Lambda_{3}}{dt} & = \left( 1-\frac{X_{3}}{X}\right) (\lambda-\alpha X)+\beta_{V}X_{3}V+\beta_{P}X_{3}P-\beta_{V}XV\frac{L_{3}}{L}+\left( \eta _{L}+\delta_{L}\right) L_{3}-\left( \eta_{L}+\delta_{L}\right) L\frac {Y_{3}}{Y}\\ & +\frac{\gamma_{Y}\left( \eta_{L}+\delta_{L}\right) }{\delta_{L}} Y_{3}-\frac{\pi_{V}\gamma_{Y}\left( \eta_{L}+\delta_{L}\right) }{\kappa _{V}\delta_{L}}V-\frac{\gamma_{Y}\left( \eta_{L}+\delta_{L}\right) YV_{3} }{\delta_{L}V}+\frac{\pi_{V}\gamma_{Y}\left( \eta_{L}+\delta_{L}\right) }{\kappa_{V}\delta_{L}}V_{3}\\ & +\frac{\varkappa_{V}\gamma_{Y}\left( \eta_{L}+\delta_{L}\right) } {\kappa_{V}\delta_{L}}ZV_{3}-\frac{\pi_{P}\gamma_{I}\left( \eta_{E} +\delta_{E}\right) }{\kappa_{P}\delta_{E}}P-\frac{\varkappa_{V}\gamma_{Y} \mu_{Z}\left( \eta_{L}+\delta_{L}\right) }{\sigma_{Z}\kappa_{V}\delta_{L} }Z-\frac{\varkappa_{V}\gamma_{Y}\left( \eta_{L}+\delta_{L}\right) } {\kappa_{V}\delta_{L}}Z_{3}V\\ & +\frac{\varkappa_{V}\gamma_{Y}\mu_{Z}\left( \eta_{L}+\delta_{L}\right) }{\sigma_{Z}\kappa_{V}\delta_{L}}Z_{3}-\frac{\varkappa_{P}\gamma_{I}\mu _{M}\left( \eta_{E}+\delta_{E}\right) }{\sigma_{M}\kappa_{P}\delta_{E}}M. \end{align*} |
Using the equilibrium conditions for \Xi_{3} :
\begin{align*} \lambda & = \alpha X_{3}+\beta_{V}X_{3}V_{3}{, \ \ \ }\beta_{V}X_{3} V_{3} = \left( \eta_{L}+\delta_{L}\right) L_{3}, \\ L_{3} & = \frac{\gamma_{Y}}{\delta_{L}}Y_{3}, {\ \ \ }\kappa_{V}Y_{3} = \pi_{V}V_{3}+\varkappa_{V}V_{3}Z_{3}, { \ }V_{3} = \frac{\mu_{Z}} {\sigma_{Z}}, \end{align*} |
we obtain,
\begin{align*} \frac{d\Lambda_{3}}{dt} & = \left( 1-\frac{X_{3}}{X}\right) \left( \alpha X_{3}-\alpha X\right) +4\beta_{V}X_{3}V_{3}-\beta_{V}X_{3}V_{3}\frac{X_{3} }{X}-\beta_{V}X_{3}V_{3}\frac{L_{3}XV}{LX_{3}V_{3}}\\ & -\beta_{V}X_{3}V_{3}\frac{Y_{3}L}{YL_{3}}-\beta_{V}X_{3}V_{3}\frac{V_{3} Y}{VY_{3}}+\frac{\pi_{P}\gamma_{I}\left( \eta_{E}+\delta_{E}\right) } {\kappa_{P}\delta_{E}}\left( \frac{\beta_{P}X_{3}\kappa_{P}\delta_{E}} {\pi_{P}\gamma_{I}\left( \eta_{E}+\delta_{E}\right) }-1\right) P\\ & -\frac{\varkappa_{P}\gamma_{I}\mu_{M}\left( \eta_{E}+\delta_{E}\right) }{\sigma_{M}\kappa_{P}\delta_{E}}M\\ & = -\frac{\alpha(X-X_{3})^{2}}{X}+\beta_{V}X_{3}V_{3}\left( 4-\frac{X_{3} }{X}-\frac{L_{3}XV}{LX_{3}V_{3}}-\frac{Y_{3}L}{YL_{3}}-\frac{V_{3}Y}{VY_{3} }\right) \\ & +\frac{\pi_{P}\gamma_{I}\left( \eta_{E}+\delta_{E}\right) }{\kappa _{P}\delta_{E}}\left( \Re_{5}-1\right) P-\frac{\varkappa_{P}\gamma_{I} \mu_{M}\left( \eta_{E}+\delta_{E}\right) }{\sigma_{M}\kappa_{P}\delta_{E}}M. \end{align*} |
Using inequality (5.1) and \Re_{5}\leq1, we get \frac{d\Lambda_{3} }{dt}\leq0 for all X, L, Y, V, P, M > 0 . Further, \frac{d\Lambda_{3}}{dt} = 0 when X = X_{3} , L = L_{3} , Y = Y_{3}, \; V = V_{3} and P = M = 0. Further, the trajectories of system (2.1) tend to \tilde{\Omega}_{3} which has elements with V = V_{3} and P = 0 . Then \dot{V} = 0 and \dot{P} = 0. The sixth and seventh equations of system (2.1), provide
\begin{align*} 0 & = \dot{V} = \kappa_{V}Y_{3}-\pi_{V}V_{3}-\varkappa_{V}V_{3}Z\Longrightarrow Z(t) = Z_{3}, \text{ for all }t\text{, }\\ 0 & = \dot{P} = \kappa_{P}I\Longrightarrow I(t) = 0, \text{ for all }t. \end{align*} |
In addition, from the fifth equation of system (2.1) gives
0 = \dot{I} = \delta_{E}E\Longrightarrow E(t) = 0, \text{ for all }t. |
Consequently, \tilde{\Omega}_{3} = \left \{ \Xi \text{ _{3} }\right \} . Applying L-LAST, we find that \Xi_{3} is G.A.S.
Theorem 5. If \Re_{4} > 1 and \Re_{6}\leq1 , then \Xi_{4} is G.A.S.
Proof. Define a function \Lambda_{4} as:
\begin{align*} \Lambda_{4} & = X_{4}\digamma \left( \frac{X}{X_{4}}\right) +L+E_{4} \digamma \left( \frac{E}{E_{4}}\right) +\frac{\eta_{L}+\delta_{L}}{\delta _{L}}Y+\frac{\eta_{E}+\delta_{E}}{\delta_{E}}I_{4}\digamma \left( \frac {I}{I_{4}}\right) +\frac{\gamma_{Y}\left( \eta_{L}+\delta_{L}\right) }{\kappa_{V}\delta_{L}}V\\ & +\frac{\gamma_{I}\left( \eta_{E}+\delta_{E}\right) }{\kappa_{P}\delta _{E}}P_{4}\digamma \left( \frac{P}{P_{4}}\right) +\frac{\gamma_{Y} \varkappa_{V}\left( \eta_{L}+\delta_{L}\right) }{\kappa_{V}\delta_{L} \sigma_{Z}}Z+\frac{\gamma_{I}\varkappa_{P}\left( \eta_{E}+\delta_{E}\right) }{\kappa_{P}\delta_{E}\sigma_{M}}M_{4}\digamma \left( \frac{M}{M_{4}}\right) . \end{align*} |
Calculating \frac{d\Lambda_{4}}{dt} as:
\begin{align} \frac{d\Lambda_{4}}{dt} & = \left( 1-\frac{X_{4}}{X}\right) [\lambda-\alpha X-\beta_{V}XV-\beta_{P}XP]+\beta_{V}XV-\left( \eta_{L}+\delta_{L}\right) L \\ & +\left( 1-\frac{E_{4}}{E}\right) \left[ \beta_{P}XP-\left( \eta _{E}+\delta_{E}\right) E\right] +\frac{\eta_{L}+\delta_{L}}{\delta_{L} }\left[ \delta_{L}L-\gamma_{Y}Y\right] \\ & +\frac{\eta_{E}+\delta_{E}}{\delta_{E}}\left( 1-\frac{I_{4}}{I}\right) \left[ \delta_{E}E-\gamma_{I}I\right] +\frac{\gamma_{Y}\left( \eta _{L}+\delta_{L}\right) }{\kappa_{V}\delta_{L}}\left[ \kappa_{V}Y-\pi _{V}V-\varkappa_{V}VZ\right] \\ & +\frac{\gamma_{I}\left( \eta_{E}+\delta_{E}\right) }{\kappa_{P}\delta _{E}}\left( 1-\frac{P_{4}}{P}\right) \left[ \kappa_{P}I-\pi_{P} P-\varkappa_{P}PM\right] +\frac{\gamma_{Y}\varkappa_{V}\left( \eta _{L}+\delta_{L}\right) }{\kappa_{V}\delta_{L}\sigma_{Z}}\left[ \sigma _{Z}VZ-\mu_{Z}Z\right] \\ & +\frac{\gamma_{I}\varkappa_{P}\left( \eta_{E}+\delta_{E}\right) } {\kappa_{P}\delta_{E}\sigma_{M}}\left( 1-\frac{M_{4}}{M}\right) \left[ \sigma_{M}PM-\mu_{M}M\right] . \end{align} | (5.6) |
Equation (5.6) can be written as:
\begin{align*} \frac{d\Lambda_{4}}{dt} & = \left( 1-\frac{X_{4}}{X}\right) (\lambda-\alpha X)+\beta_{V}X_{4}V+\beta_{P}X_{4}P-\beta_{P}XP\frac{E_{4}}{E}+\left( \eta _{E}+\delta_{E}\right) E_{4}-\left( \eta_{E}+\delta_{E}\right) E\frac {I_{4}}{I}\\ & +\frac{\gamma_{I}\left( \eta_{E}+\delta_{E}\right) }{\delta_{E}} I_{4}-\frac{\pi_{V}\gamma_{Y}\left( \eta_{L}+\delta_{L}\right) }{\kappa _{V}\delta_{L}}V-\frac{\pi_{P}\gamma_{I}\left( \eta_{E}+\delta_{E}\right) }{\kappa_{P}\delta_{E}}P-\frac{\gamma_{I}\left( \eta_{E}+\delta_{E}\right) P_{4}}{\delta_{E}P}I\\ & +\frac{\pi_{P}\gamma_{I}\left( \eta_{E}+\delta_{E}\right) }{\kappa _{P}\delta_{E}}P_{4}+\frac{\varkappa_{P}\gamma_{I}\left( \eta_{E}+\delta _{E}\right) }{\kappa_{P}\delta_{E}}MP_{4}-\frac{\varkappa_{V}\gamma_{Y} \mu_{Z}\left( \eta_{L}+\delta_{L}\right) }{\kappa_{V}\delta_{L}\sigma_{Z} }Z-\frac{\varkappa_{P}\gamma_{I}\mu_{M}\left( \eta_{E}+\delta_{E}\right) }{\kappa_{P}\delta_{E}\sigma_{M}}M\\ & -\frac{\varkappa_{P}\gamma_{I}\left( \eta_{E}+\delta_{E}\right) } {\kappa_{P}\delta_{E}}M_{4}P+\frac{\varkappa_{P}\gamma_{I}\mu_{M}\left( \eta_{E}+\delta_{E}\right) }{\sigma_{M}\kappa_{P}\delta_{E}}M_{4}. \end{align*} |
Using the equilibrium conditions for \Xi_{4} :
\begin{align*} \lambda & = \alpha X_{4}+\beta_{P}X_{4}P_{4}, { \ \ \ }\beta_{P}X_{4} P_{4} = \left( \eta_{E}+\delta_{E}\right) E_{4}, \\ \kappa_{P}I_{4} & = \pi_{P}P_{4}+\varkappa_{P}P_{4}M_{4}, { \ \ } E_{4} = \frac{\gamma_{I}}{\delta_{E}}I_{4}, { \ \ }P_{4} = \frac{\mu_{M} }{\sigma_{M}}, \end{align*} |
we obtain,
\begin{align*} \frac{d\Lambda_{4}}{dt} & = \left( 1-\frac{X_{4}}{X}\right) \left( \alpha X_{4}-\alpha X\right) +4\beta_{P}X_{4}P_{4}-\beta_{P}X_{4}P_{4}\frac{X_{4} }{X}-\beta_{P}X_{4}P_{4}\frac{E_{4}XP}{EX_{4}P_{4}}\\ & -\beta_{P}X_{4}P_{4}\frac{I_{4}E}{IE_{4}}-\beta_{P}X_{4}P_{4}\frac{P_{4} I}{PI_{4}}-\frac{\varkappa_{V}\gamma_{Y}\mu_{Z}\left( \eta_{L}+\delta _{L}\right) }{\kappa_{V}\delta_{L}\sigma_{Z}}Z\\ &+\frac{\pi_{V}\gamma_{Y}\left( \eta_{L}+\delta_{L}\right) }{\kappa_{V}\delta_{L}}\left( \frac{\beta _{V}X_{4}\kappa_{V}\delta_{L}}{\pi_{V}\gamma_{Y}\left( \eta_{L}+\delta _{L}\right) }-1\right) V\\ & = -\frac{\alpha(X-X_{4})^{2}}{X}+\beta_{P}X_{4}P_{4}\left( 4-\frac{X_{4} }{X}-\frac{E_{4}XP}{EX_{4}P_{4}}-\frac{I_{4}E}{IE_{4}}-\frac{P_{4}I}{PI_{4} }\right) \\ & +\frac{\pi_{V}\gamma_{Y}\left( \eta_{L}+\delta_{L}\right) }{\kappa _{V}\delta_{L}}\left( \Re_{6}-1\right) V-\frac{\varkappa_{V}\gamma_{Y} \mu_{Z}\left( \eta_{L}+\delta_{L}\right) }{\kappa_{V}\delta_{L}\sigma_{Z}}Z. \end{align*} |
Since \Re_{6}\leq1 , then employing inequality (5.1), we get \frac{d\Lambda_{4}}{dt}\leq0 for all X, E, I, V, P, Z > 0 . Further, \frac{d\Lambda_{4}}{dt} = 0 when X = X_{4} , E = E_{4}, \; I = I_{4}, \; P = P_{4} and V = Z = 0. The solutions of system (2.1) tend to \tilde{\Omega}_{4} which contains elements with P = P_{4} and V = 0 , then \dot{V} = \dot{P} = 0 . The sixth and seventh equations of system (2.1) imply
\begin{align*} 0 & = \dot{V} = \kappa_{V}Y\Longrightarrow Y(t) = 0, \text{ for all }t\text{, }\\ 0 & = \dot{P} = \kappa_{P}I_{4}-\pi_{P}P_{4}-\varkappa_{P}P_{4}M\Longrightarrow M(t) = M_{4}, \text{ for all }t\text{.} \end{align*} |
In addition, since Y = 0 , then \dot{Y} = 0 . The fourth equation of system (2.1) gives
0 = \dot{Y} = \delta_{L}L\Longrightarrow L(t) = 0, \text{ for all }t\text{.} |
Therefore, \tilde{\Omega}_{4} = \left \{ \Xi_{4}\right \}. Applying L-LAST, we get \Xi_{4} is G.A.S.
Theorem 6. If \Re_{5} > 1 , \Re_{1}/\Re_{2} > 1 and \Re_{8}\leq1 , then \Xi_{5} is G.A.S.
Proof. Define
\begin{align*} \Lambda_{5} & = X_{5}\digamma \left( \frac{X}{X_{5}}\right) +L_{5} \digamma \left( \frac{L}{L_{5}}\right) +E_{5}\digamma \left( \frac{E}{E_{5} }\right) +\frac{\eta_{L}+\delta_{L}}{\delta_{L}}Y_{5}\digamma \left( \frac {Y}{Y_{5}}\right) \\ & +\frac{\eta_{E}+\delta_{E}}{\delta_{E}}I_{5}\digamma \left( \frac{I}{I_{5} }\right) +\frac{\gamma_{Y}\left( \eta_{L}+\delta_{L}\right) }{\kappa _{V}\delta_{L}}V_{5}\digamma \left( \frac{V}{V_{5}}\right) +\frac{\gamma _{I}\left( \eta_{E}+\delta_{E}\right) }{\kappa_{P}\delta_{E}}P_{5} \digamma \left( \frac{P}{P_{5}}\right) \\ & +\frac{\gamma_{Y}\varkappa_{V}\left( \eta_{L}+\delta_{L}\right) } {\kappa_{V}\delta_{L}\sigma_{Z}}Z_{5}\digamma \left( \frac{Z}{Z_{5}}\right) +\frac{\gamma_{I}\varkappa_{P}\left( \eta_{E}+\delta_{E}\right) }{\kappa _{P}\delta_{E}\sigma_{M}}M. \end{align*} |
Calculating \frac{d\Lambda_{5}}{dt} as:
\begin{array}{l} \frac{d\Lambda_{5}}{dt} = \left( 1-\frac{X_{5}}{X}\right) [\lambda-\alpha X-\beta_{V}XV-\beta_{P}XP]+\left( 1-\frac{L_{5}}{L}\right) \left[ \beta _{V}XV-\left( \eta_{L}+\delta_{L}\right) L\right] \\ +\left( 1-\frac{E_{5}}{E}\right) \left[ \beta_{P}XP-\left( \eta _{E}+\delta_{E}\right) E\right] +\frac{\eta_{L}+\delta_{L}}{\delta_{L} }\left( 1-\frac{Y_{5}}{Y}\right) \left[ \delta_{L}L-\gamma_{Y}Y\right] \\ +\frac{\eta_{E}+\delta_{E}}{\delta_{E}}\left( 1-\frac{I_{5}}{I}\right) \left[ \delta_{E}E-\gamma_{I}I\right] +\frac{\gamma_{Y}\left( \eta _{L}+\delta_{L}\right) }{\kappa_{V}\delta_{L}}\left( 1-\frac{V_{5}} {V}\right) \left[ \kappa_{V}Y-\pi_{V}V-\varkappa_{V}VZ\right] \\ +\frac{\gamma_{I}\left( \eta_{E}+\delta_{E}\right) }{\kappa_{P}\delta _{E}}\left( 1-\frac{P_{5}}{P}\right) \left[ \kappa_{P}I-\pi_{P} P-\varkappa_{P}PM\right] +\frac{\gamma_{Y}\varkappa_{V}\left( \eta _{L}+\delta_{L}\right) }{\kappa_{V}\delta_{L}\sigma_{Z}}\left( 1-\frac {Z_{5}}{Z}\right) \left[ \sigma_{Z}VZ-\mu_{Z}Z\right] \\ +\frac{\gamma_{I}\varkappa_{P}\left( \eta_{E}+\delta_{E}\right) } {\kappa_{P}\delta_{E}\sigma_{M}}\left[ \sigma_{M}PM-\mu_{M}M\right] . \end{array} | (5.7) |
Equation (5.7) can be simplified as:
\begin{align*} \frac{d\Lambda_{5}}{dt} & = \left( 1-\frac{X_{5}}{X}\right) (\lambda-\alpha X)+\beta_{V}X_{5}V+\beta_{P}X_{5}P-\beta_{V}XV\frac{L_{5}}{L}+\left( \eta _{L}+\delta_{L}\right) L_{5}-\beta_{P}XP\frac{E_{5}}{E}\\ & +\left( \eta_{E}+\delta_{E}\right) E_{5}-\left( \eta_{L}+\delta _{L}\right) L\frac{Y_{5}}{Y}+\frac{\gamma_{Y}\left( \eta_{L}+\delta _{L}\right) }{\delta_{L}}Y_{5}-\left( \eta_{E}+\delta_{E}\right) E\frac{I_{5}}{I}+\frac{\gamma_{I}\left( \eta_{E}+\delta_{E}\right) } {\delta_{E}}I_{5}\\ & -\frac{\pi_{V}\gamma_{Y}\left( \eta_{L}+\delta_{L}\right) }{\kappa _{V}\delta_{L}}V-\frac{\gamma_{Y}\left( \eta_{L}+\delta_{L}\right) V_{5} }{\delta_{L}V}Y+\frac{\pi_{V}\gamma_{Y}\left( \eta_{L}+\delta_{L}\right) }{\kappa_{V}\delta_{L}}V_{5}+\frac{\varkappa_{V}\gamma_{Y}\left( \eta _{L}+\delta_{L}\right) }{\kappa_{V}\delta_{L}}V_{5}Z\\ & -\frac{\pi_{P}\gamma_{I}\left( \eta_{E}+\delta_{E}\right) }{\kappa _{P}\delta_{E}}P-\frac{\gamma_{I}\left( \eta_{E}+\delta_{E}\right) P_{5} }{\delta_{E}P}I+\frac{\pi_{P}\gamma_{I}\left( \eta_{E}+\delta_{E}\right) }{\kappa_{P}\delta_{E}}P_{5}+\frac{\varkappa_{P}\gamma_{I}\left( \eta _{E}+\delta_{E}\right) }{\kappa_{P}\delta_{E}}MP_{5}\\ & -\frac{\varkappa_{V}\gamma_{Y}\mu_{Z}\left( \eta_{L}+\delta_{L}\right) }{\kappa_{V}\delta_{L}\sigma_{Z}}Z-\frac{\varkappa_{V}\gamma_{Y}\left( \eta_{L}+\delta_{L}\right) }{\kappa_{V}\delta_{L}}VZ_{5}+\frac{\varkappa _{V}\gamma_{Y}\mu_{Z}\left( \eta_{L}+\delta_{L}\right) }{\kappa_{V} \delta_{L}\sigma_{Z}}Z_{5}\\ & -\frac{\varkappa_{P}\gamma_{I}\mu_{M}\left( \eta_{E}+\delta_{E}\right) }{\kappa_{P}\delta_{E}\sigma_{M}}M. \end{align*} |
Using the equilibrium conditions for \Xi_{5} :
\begin{align*} \lambda & = \alpha X_{5}+\beta_{V}X_{5}V_{5}+\beta_{P}X_{5}P_{5} , \ \ \ \ \beta_{V}X_{5}V_{5} = \left( \eta_{L}+\delta_{L}\right) L_{5}, \\ \beta_{P}X_{5}P_{5} & = \left( \eta_{E}+\delta_{E}\right) E_{5} , \ \ \ \ \kappa_{V}Y_{5} = \pi_{V}V_{5}+\varkappa_{V}V_{5}Z_{5}, \\ \kappa_{P}I_{5} & = \pi_{P}P_{5}, \ \ \ \ V_{5} = \frac{\mu_{Z}}{\sigma_{Z}}, \\ L_{5} & = \frac{\gamma_{Y}}{\delta_{L}}Y_{5}, \ \ \ \ E_{5} = \frac{\gamma_{I} }{\delta_{E}}I_{5}, \end{align*} |
we obtain,
\begin{align*} \frac{d\Lambda_{5}}{dt} & = \left( 1-\frac{X_{5}}{X}\right) \left( \alpha X_{5}-\alpha X\right) +4\beta_{V}X_{5}V_{5}+4\beta_{P}X_{5}P_{5}-\beta _{V}X_{5}V_{5}\frac{X_{5}}{X}-\beta_{P}X_{5}P_{5}\frac{X_{5}}{X}\\ & -\beta_{V}X_{5}V_{5}\frac{L_{5}XV}{LX_{5}V_{5}}-\beta_{P}X_{5}P_{5} \frac{E_{5}XP}{EX_{5}P_{5}}-\beta_{V}X_{5}V_{5}\frac{Y_{5}L}{YL_{5}}-\beta _{P}X_{5}P_{5}\frac{I_{5}E}{IE_{5}}\\ & -\beta_{V}X_{5}V_{5}\frac{V_{5}Y}{VY_{5}}-\beta_{P}X_{5}P_{5}\frac{P_{5} I}{PI_{5}}+\frac{\varkappa_{P}\gamma_{I}\mu_{M}\left( \eta_{E}+\delta _{E}\right) }{\kappa_{P}\delta_{E}\sigma_{M}}\left( \frac{\sigma_{M}} {\mu_{M}}P_{5}-1\right) M\\ & = -\frac{\alpha(X-X_{5})^{2}}{X}+\beta_{V}X_{5}V_{5}\left( 4-\frac{X_{5} }{X}-\frac{L_{5}XV}{LX_{5}V_{5}}-\frac{Y_{5}L}{YL_{5}}-\frac{V_{5}Y}{VY_{5} }\right) \\ & +\beta_{P}X_{5}P_{5}\left( 4-\frac{X_{5}}{X}-\frac{E_{5}XP}{EX_{5}P_{5} }-\frac{I_{5}E}{IE_{5}}-\frac{P_{5}I}{PI_{5}}\right) \\ & +\frac{\varkappa_{P}\gamma_{I}\left( \eta_{E}+\delta_{E}\right) \left( \alpha \sigma_{Z}\sigma_{M}+\beta_{V}\mu_{Z}\sigma_{M}+\beta_{P}\mu_{M} \sigma_{Z}\right) }{\kappa_{P}\delta_{E}\beta_{P}\sigma_{Z}\sigma_{M}}\left( \Re_{8}-1\right) M. \end{align*} |
Since \Re_{8}\leq1 , then employing inequality (5.1), we get \frac{d\Lambda_{5}}{dt}\leq0 for all X, L, E, Y, I, V, P, M > 0 . Moreover, we have \frac{d\Lambda_{5}}{dt} = 0, when X = X_{5}, \; L = L_{5}, \; E = E_{5} , Y = Y_{5}, I = I_{5}, \; V = V_{5}, \; P = P_{5} and M = 0. The trajectories of system (2.1) converge to \tilde{\Omega}_{5} which comprises elements with Y = Y_{5} and V = V_{5}, then \dot{V} = 0 . The sixth equation of system (2.1) implies that
0 = \dot{V} = \kappa_{V}Y_{5}-\pi_{V}V_{5}-\varkappa_{V}V_{5}Z\Longrightarrow Z(t) = Z_{5}, \text{ for all }t. |
Consequently, \tilde{\Omega}_{5} = \left \{ \Xi \text{ _{5} }\right \}. and by applying L-LAST, we get \Xi_{5} is G.A.S.
Theorem 7. Let \Re_{6} > 1 , \Re_{7}\leq1 and \Re_{2}/\Re_{1} > 1 , then \Xi_{6} is G.A.S.
Proof. Consider a function \Lambda_{6} as:
\begin{align*} \Lambda_{6} & = X_{6}\digamma \left( \frac{X}{X_{6}}\right) +L_{6} \digamma \left( \frac{L}{L_{6}}\right) +E_{6}\digamma \left( \frac{E}{E_{6} }\right) +\frac{\eta_{L}+\delta_{L}}{\delta_{L}}Y_{6}\digamma \left( \frac {Y}{Y_{6}}\right) \\ & +\frac{\eta_{E}+\delta_{E}}{\delta_{E}}I_{6}\digamma \left( \frac{I}{I_{6} }\right) +\frac{\gamma_{Y}\left( \eta_{L}+\delta_{L}\right) }{\kappa _{V}\delta_{L}}V_{6}\digamma \left( \frac{V}{V_{6}}\right) +\frac{\gamma _{I}\left( \eta_{E}+\delta_{E}\right) }{\kappa_{P}\delta_{E}}P_{6} \digamma \left( \frac{P}{P_{6}}\right) \\ & +\frac{\gamma_{Y}\varkappa_{V}\left( \eta_{L}+\delta_{L}\right) } {\kappa_{V}\delta_{L}\sigma_{Z}}Z+\frac{\gamma_{I}\varkappa_{P}\left( \eta_{E}+\delta_{E}\right) }{\kappa_{P}\delta_{E}\sigma_{M}}M_{6} \digamma \left( \frac{M}{M_{6}}\right) . \end{align*} |
Calculating \frac{d\Lambda_{6}}{dt} as:
\begin{align} \frac{d\Lambda_{6}}{dt} & = \left( 1-\frac{X_{6}}{X}\right) [\lambda-\alpha X-\beta_{V}XV-\beta_{P}XP]+\left( 1-\frac{L_{6}}{L}\right) \left[ \beta _{V}XV-\left( \eta_{L}+\delta_{L}\right) L\right] \\ & +\left( 1-\frac{E_{6}}{E}\right) \left[ \beta_{P}XP-\left( \eta _{E}+\delta_{E}\right) E\right] +\frac{\eta_{L}+\delta_{L}}{\delta_{L} }\left( 1-\frac{Y_{6}}{Y}\right) \left[ \delta_{L}L-\gamma_{Y}Y\right] \\ & +\frac{\eta_{E}+\delta_{E}}{\delta_{E}}\left( 1-\frac{I_{6}}{I}\right) \left[ \delta_{E}E-\gamma_{I}I\right] +\frac{\gamma_{Y}\left( \eta _{L}+\delta_{L}\right) }{\kappa_{V}\delta_{L}}\left( 1-\frac{V_{6}} {V}\right) \left[ \kappa_{V}Y-\pi_{V}V-\varkappa_{V}VZ\right] \\ & +\frac{\gamma_{I}\left( \eta_{E}+\delta_{E}\right) }{\kappa_{P}\delta _{E}}\left( 1-\frac{P_{6}}{P}\right) \left[ \kappa_{P}I-\pi_{P} P-\varkappa_{P}PM\right] +\frac{\gamma_{Y}\varkappa_{V}\left( \eta _{L}+\delta_{L}\right) }{\kappa_{V}\delta_{L}\sigma_{Z}}\left[ \sigma _{Z}VZ-\mu_{Z}Z\right] \\ & +\frac{\gamma_{I}\varkappa_{P}\left( \eta_{E}+\delta_{E}\right) } {\kappa_{P}\delta_{E}\sigma_{M}}\left( 1-\frac{M_{6}}{M}\right) \left[ \sigma_{M}PM-\mu_{M}M\right] . \end{align} | (5.8) |
We collect the terms of Eq (5.8) as:
\begin{array}{l} \frac{d\Lambda_{6}}{dt} = \left( 1-\frac{X_{6}}{X}\right) (\lambda-\alpha X)+\beta_{V}X_{6}V+\beta_{P}X_{6}P-\beta_{V}XV\frac{L_{6}}{L}+\left( \eta _{L}+\delta_{L}\right) L_{6}-\beta_{P}XP\frac{E_{6}}{E}\\ +\left( \eta_{E}+\delta_{E}\right) E_{6}-\left( \eta_{L}+\delta _{L}\right) L\frac{Y_{6}}{Y}+\frac{\gamma_{Y}\left( \eta_{L}+\delta _{L}\right) }{\delta_{L}}Y_{6}-\left( \eta_{E}+\delta_{E}\right) E\frac{I_{6}}{I}+\frac{\gamma_{I}\left( \eta_{E}+\delta_{E}\right) } {\delta_{E}}I_{6}\\ -\frac{\pi_{V}\gamma_{Y}\left( \eta_{L}+\delta_{L}\right) }{\kappa _{V}\delta_{L}}V-\frac{\gamma_{Y}\left( \eta_{L}+\delta_{L}\right) V_{6} }{\delta_{L}V}Y+\frac{\pi_{V}\gamma_{Y}\left( \eta_{L}+\delta_{L}\right) }{\kappa_{V}\delta_{L}}V_{6}+\frac{\varkappa_{V}\gamma_{Y}\left( \eta _{L}+\delta_{L}\right) }{\kappa_{V}\delta_{L}}V_{6}Z\\ -\frac{\pi_{P}\gamma_{I}\left( \eta_{E}+\delta_{E}\right) }{\kappa _{P}\delta_{E}}P-\frac{\gamma_{I}\left( \eta_{E}+\delta_{E}\right) P_{6} }{\delta_{E}P}I+\frac{\pi_{P}\gamma_{I}\left( \eta_{E}+\delta_{E}\right) }{\kappa_{P}\delta_{E}}P_{6}+\frac{\varkappa_{P}\gamma_{I}\left( \eta _{E}+\delta_{E}\right) }{\kappa_{P}\delta_{E}}MP_{6}\\ -\frac{\varkappa_{V}\gamma_{Y}\mu_{Z}\left( \eta_{L}+\delta_{L}\right) }{\kappa_{V}\delta_{L}\sigma_{Z}}Z-\frac{\varkappa_{P}\gamma_{I}\mu_{M}\left( \eta_{E}+\delta_{E}\right) }{\kappa_{P}\delta_{E}\sigma_{M}}M-\frac {\varkappa_{P}\gamma_{I}\left( \eta_{E}+\delta_{E}\right) }{\kappa_{P} \delta_{E}}PM_{6}+\frac{\varkappa_{P}\gamma_{I}\mu_{M}\left( \eta_{E} +\delta_{E}\right) }{\kappa_{P}\delta_{E}\sigma_{M}}M_{6}. \end{array} |
Using the equilibrium conditions for \Xi_{6} :
\begin{align*} \lambda & = \alpha X_{6}+\beta_{V}X_{6}V_{6}+\beta_{P}X_{6}P_{6}, { \ \ \ }\beta_{V}X_{6}V_{6} = \left( \eta_{L}+\delta_{L}\right) L_{6}, { \ \ }\beta_{P}X_{6}P_{6} = \left( \eta_{E}+\delta_{E}\right) E_{6}, \\ & \left. L_{6} = \frac{\gamma_{Y}}{\delta_{L}}Y_{6}, { \ \ }E_{6} = \frac{\gamma_{I}}{\delta_{E}}I_{6}, { \ \ }Y_{6} = \frac{\pi_{V}} {\kappa_{V}}V_{6}, \right. \\ & \left. I_{6} = \frac{\pi_{P}}{\kappa_{P}}P_{6}+\frac{\varkappa_{P}} {\kappa_{P}}P_{6}M_{6}, { \ \ }P_{6} = \frac{\mu_{M}}{\sigma_{M}}, \right. \end{align*} |
we obtain,
\begin{align*} \frac{d\Lambda_{6}}{dt} & = \left( 1-\frac{X_{6}}{X}\right) \left( \alpha X_{6}-\alpha X\right) +4\beta_{V}X_{6}V_{6}+4\beta_{P}X_{6}P_{6}-\beta _{V}X_{6}V_{6}\frac{X_{6}}{X}-\beta_{P}X_{6}P_{6}\frac{X_{6}}{X}\\ & -\beta_{V}X_{6}V_{6}\frac{L_{6}XV}{LX_{6}V_{6}}-\beta_{P}X_{6}P_{6} \frac{E_{6}XP}{EX_{6}P_{6}}-\beta_{V}X_{6}V_{6}\frac{Y_{6}L}{YL_{6}}-\beta _{P}X_{6}P_{6}\frac{I_{6}E}{IE_{6}}\\ & -\beta_{V}X_{6}V_{6}\frac{V_{6}Y}{VY_{6}}-\beta_{P}X_{6}P_{6}\frac{P_{6} I}{PI_{6}}+\frac{\varkappa_{V}\gamma_{Y}\mu_{Z}\left( \eta_{L}+\delta _{L}\right) }{\kappa_{V}\delta_{L}\sigma_{Z}}\left( \frac{\sigma_{Z}} {\mu_{Z}}V_{6}-1\right) Z\\ & = -\frac{\alpha(X-X_{6})^{2}}{X}+\beta_{V}X_{6}V_{6}\left( 4-\frac{X_{6} }{X}-\frac{L_{6}XV}{LX_{6}V_{6}}-\frac{Y_{6}L}{YL_{6}}-\frac{V_{6}Y}{VY_{6} }\right) \\ & +\beta_{P}X_{6}P_{6}\left( 4-\frac{X_{6}}{X}-\frac{E_{6}XP}{EX_{6}P_{6} }-\frac{I_{6}E}{IE_{6}}-\frac{P_{6}I}{PI_{6}}\right) \\ & +\frac{\varkappa_{V}\gamma_{Y}\left( \eta_{L}+\delta_{L}\right) \left( \alpha \sigma_{Z}\sigma_{M}+\beta_{V}\mu_{Z}\sigma_{M}+\beta_{P}\mu_{M} \sigma_{Z}\right) }{\kappa_{V}\delta_{L}\sigma_{M}\beta_{V}\sigma_{Z}}\left( \Re_{7}-1\right) Z. \end{align*} |
Since \Re_{7}\leq1 , then employing inequality (5.1), we get \frac{d\Lambda_{6}}{dt}\leq0 for all X, L, E, Y, I, V, P, Z > 0 . Moreover, \frac{d\Lambda_{6}}{dt} = 0 when X = X_{6} , L = L_{6}, \; E = E_{6}, \; Y = Y_{6}, I = I_{6}, \; V = V_{6}, \; P = P_{6} and Z = 0. The solutions of system (2.1) tend to \tilde{\Omega}_{6} which contains elements with P = P_{6} then, \dot{P} = 0 . The seven equation of system (2.1) implies that
0 = \dot{P} = \kappa_{P}I_{6}-\pi_{P}P_{6}-\varkappa_{P}P_{6}M\Longrightarrow M(t) = M_{6}, \text{ for all }t. |
Consequently, \tilde{\Omega}_{6} = \left \{ \Xi_{6}\right \} . Using L-LAST we deduce that \Xi_{6} is G.A.S.
Theorem 8. If \Re_{7} > 1 and \Re_{8} > 1 , then \Xi_{7} is G.A.S.
Proof. Define a function \Lambda_{7} as:
\begin{align*} \Lambda_{7} & = X_{7}\digamma \left( \frac{X}{X_{7}}\right) +L_{7} \digamma \left( \frac{L}{L_{7}}\right) +E_{7}\digamma \left( \frac{E}{E_{7} }\right) +\frac{\eta_{L}+\delta_{L}}{\delta_{L}}Y_{7}\digamma \left( \frac {Y}{Y_{7}}\right) \\ & +\frac{\eta_{E}+\delta_{E}}{\delta_{E}}I_{7}\digamma \left( \frac{I}{I_{7} }\right) +\frac{\gamma_{Y}\left( \eta_{L}+\delta_{L}\right) }{\kappa _{V}\delta_{L}}V_{7}\digamma \left( \frac{V}{V_{7}}\right) +\frac{\gamma _{I}\left( \eta_{E}+\delta_{E}\right) }{\kappa_{P}\delta_{E}}P_{7} \digamma \left( \frac{P}{P_{7}}\right) \\ & +\frac{\gamma_{Y}\varkappa_{V}\left( \eta_{L}+\delta_{L}\right) } {\kappa_{V}\delta_{L}\sigma_{Z}}Z_{7}\digamma \left( \frac{Z}{Z_{7}}\right) +\frac{\gamma_{I}\varkappa_{P}\left( \eta_{E}+\delta_{E}\right) }{\kappa _{P}\delta_{E}\sigma_{M}}M_{7}\digamma \left( \frac{M}{M_{7}}\right) . \end{align*} |
Calculating \frac{d\Lambda_{7}}{dt} as:
\begin{array}{l} \frac{d\Lambda_{7}}{dt} = \left( 1-\frac{X_{7}}{X}\right) [\lambda-\alpha X-\beta_{V}XV-\beta_{P}XP]+\left( 1-\frac{L_{7}}{L}\right) \left[ \beta _{V}XV-\left( \eta_{L}+\delta_{L}\right) L\right] \\ +\left( 1-\frac{E_{7}}{E}\right) \left[ \beta_{P}XP-\left( \eta _{E}+\delta_{E}\right) E\right] +\frac{\eta_{L}+\delta_{L}}{\delta_{L} }\left( 1-\frac{Y_{7}}{Y}\right) \left[ \delta_{L}L-\gamma_{Y}Y\right] \\ +\frac{\eta_{E}+\delta_{E}}{\delta_{E}}\left( 1-\frac{I_{7}}{I}\right) \left[ \delta_{E}E-\gamma_{I}I\right] +\frac{\gamma_{Y}\left( \eta _{L}+\delta_{L}\right) }{\kappa_{V}\delta_{L}}\left( 1-\frac{V_{7}} {V}\right) \left[ \kappa_{V}Y-\pi_{V}V-\varkappa_{V}VZ\right] \\ +\frac{\gamma_{I}\left( \eta_{E}+\delta_{E}\right) }{\kappa_{P}\delta _{E}}\left( 1-\frac{P_{7}}{P}\right) \left[ \kappa_{P}I-\pi_{P} P-\varkappa_{P}PM\right] +\frac{\gamma_{Y}\varkappa_{V}\left( \eta _{L}+\delta_{L}\right) }{\kappa_{V}\delta_{L}\sigma_{Z}}\left( 1-\frac {Z_{7}}{Z}\right) \left[ \sigma_{Z}VZ-\mu_{Z}Z\right] \\ +\frac{\gamma_{I}\varkappa_{P}\left( \eta_{E}+\delta_{E}\right) } {\kappa_{P}\delta_{E}\sigma_{M}}\left( 1-\frac{M_{7}}{M}\right) \left[ \sigma_{M}PM-\mu_{M}M\right] . \end{array} | (5.9) |
We collect the terms of Eq (5.9) as:
\begin{array}{l} \frac{d\Lambda_{7}}{dt} = \left( 1-\frac{X_{7}}{X}\right) (\lambda-\alpha X)+\beta_{V}X_{7}V+\beta_{P}X_{7}P-\beta_{V}XV\frac{L_{7}}{L}+\left( \eta _{L}+\delta_{L}\right) L_{7}-\beta_{P}XP\frac{E_{7}}{E}\\ +\left( \eta_{E}+\delta_{E}\right) E_{7}-\left( \eta_{L}+\delta _{L}\right) L\frac{Y_{7}}{Y}+\frac{\gamma_{Y}\left( \eta_{L}+\delta _{L}\right) }{\delta_{L}}Y_{7}-\left( \eta_{E}+\delta_{E}\right) E\frac{I_{7}}{I}+\frac{\gamma_{I}\left( \eta_{E}+\delta_{E}\right) } {\delta_{E}}I_{7}\\ -\frac{\pi_{V}\gamma_{Y}\left( \eta_{L}+\delta_{L}\right) }{\kappa _{V}\delta_{L}}V-\frac{\gamma_{Y}\left( \eta_{L}+\delta_{L}\right) V_{7} }{\delta_{L}V}Y+\frac{\pi_{V}\gamma_{Y}\left( \eta_{L}+\delta_{L}\right) }{\kappa_{V}\delta_{L}}V_{7}+\frac{\varkappa_{V}\gamma_{Y}\left( \eta _{L}+\delta_{L}\right) }{\kappa_{V}\delta_{L}}V_{7}Z\\ -\frac{\pi_{P}\gamma_{I}\left( \eta_{E}+\delta_{E}\right) }{\kappa _{P}\delta_{E}}P-\frac{\gamma_{I}\left( \eta_{E}+\delta_{E}\right) P_{7} }{\delta_{E}P}I+\frac{\pi_{P}\gamma_{I}\left( \eta_{E}+\delta_{E}\right) }{\kappa_{P}\delta_{E}}P_{7}+\frac{\varkappa_{P}\gamma_{I}\left( \eta _{E}+\delta_{E}\right) }{\kappa_{P}\delta_{E}}MP_{7}\\ -\frac{\varkappa_{V}\gamma_{Y}\mu_{Z}\left( \eta_{L}+\delta_{L}\right) }{\kappa_{V}\delta_{L}\sigma_{Z}}Z-\frac{\varkappa_{V}\gamma_{Y}\left( \eta_{L}+\delta_{L}\right) }{\kappa_{V}\delta_{L}}VZ_{7}+\frac{\varkappa _{V}\gamma_{Y}\mu_{Z}\left( \eta_{L}+\delta_{L}\right) }{\kappa_{V} \delta_{L}\sigma_{Z}}Z_{7}-\frac{\varkappa_{P}\gamma_{I}\mu_{M}\left( \eta_{E}+\delta_{E}\right) }{\kappa_{P}\delta_{E}\sigma_{M}}M\\ -\frac{\varkappa_{P}\gamma_{I}\left( \eta_{E}+\delta_{E}\right) } {\kappa_{P}\delta_{E}}PM_{7}+\frac{\varkappa_{P}\gamma_{I}\mu_{M}\left( \eta_{E}+\delta_{E}\right) }{\kappa_{P}\delta_{E}\sigma_{M}}M_{7}. \end{array} |
Using the equilibrium conditions for \Xi_{7} :
\begin{align*} \lambda & = \alpha X_{7}+\beta_{V}X_{7}V_{7}+\beta_{P}X_{7}P_{7}, { \ \ \ \ \ }\beta_{V}X_{7}V_{7} = \left( \eta_{L}+\delta_{L}\right) L_{7, { \ \ \ }}\\ & \beta_{P}X_{7}P_{7} = \left( \eta_{E}+\delta_{E}\right) E_{7}, { \ \ \ }L_{7} = \frac{\gamma_{Y}}{\delta_{L}}Y_{7}, { \ \ \ }E_{7} = \frac{\gamma_{I}}{\delta_{E}}I_{7}, \\ & Y_{7} = \frac{\pi_{V}}{\kappa_{V}}V_{7}+\frac{\varkappa_{V}}{\kappa_{V}} V_{7}Z_{7}, { \ \ \ }I_{7} = \frac{\pi_{P}}{\kappa_{P}}P_{7}+\frac {\varkappa_{P}}{\kappa_{P}}P_{7}M_{7}, \\ & V_{7} = \frac{\mu_{Z}}{\sigma_{Z}}, { \ \ \ }P_{7} = \frac{\mu_{M}} {\sigma_{M}}, \end{align*} |
we obtain,
\begin{align*} \frac{d\Lambda_{7}}{dt} & = \left( 1-\frac{X_{7}}{X}\right) \left( \alpha X_{7}-\alpha X\right) +4\beta_{V}X_{7}V_{7}+4\beta_{P}X_{7}P_{7}-\beta _{V}X_{7}V_{7}\frac{X_{7}}{X}-\beta_{P}X_{7}P_{7}\frac{X_{7}}{X}\\ & -\beta_{V}X_{7}V_{7}\frac{L_{7}XV}{LX_{7}V_{7}}-\beta_{P}X_{7}P_{7} \frac{E_{7}XP}{EX_{7}P_{7}}-\beta_{V}X_{7}V_{7}\frac{Y_{7}L}{YL_{7}}-\beta _{P}X_{7}P_{7}\frac{I_{7}E}{IE_{7}}\\ & -\beta_{V}X_{7}V_{7}\frac{V_{7}Y}{VY_{7}}-\beta_{P}X_{7}P_{7}\frac{P_{7} I}{PI_{7}}\\ & = -\frac{\alpha(X-X_{7})^{2}}{X}+\beta_{V}X_{7}V_{7}\left( 4-\frac{X_{7} }{X}-\frac{L_{7}XV}{LX_{7}V_{7}}-\frac{Y_{7}L}{YL_{7}}-\frac{V_{7}Y}{VY_{7} }\right) \\ & +\beta_{P}X_{7}P_{7}\left( 4-\frac{X_{7}}{X}-\frac{E_{7}XP}{EX_{7}P_{7} }-\frac{I_{7}E}{IE_{7}}-\frac{P_{7}I}{PI_{7}}\right) . \end{align*} |
Using inequality (5.1), we get \frac{d\Lambda_{7}}{dt}\leq0 for all X, L, E, Y, I, V, P > 0 , where \frac{d\Lambda_{7}}{dt} = 0 when X = X_{7} , L = L_{7}, \; E = E_{7}, \; Y = Y_{7}, \; I = I_{7}, \; V = V_{7} and P = P_{7}. The solutions of system (2.1) tend to \tilde{\Omega}_{7} which includes element with V = V_{7} and P = P_{7} which gives \dot{V} = \dot{P} = 0 and from the sixth and seventh equations of system (2.1) we get
\begin{align*} 0 & = \dot{V} = \kappa_{V}Y_{7}-\pi_{V}V_{7}-\varkappa_{V}V_{7}Z\Longrightarrow Z(t) = Z_{7}, \text{ for all }t, \\ 0 & = \dot{P} = \kappa_{P}I_{7}-\pi_{P}P_{7}-\varkappa_{P}P_{7}M\Longrightarrow M(t) = M_{7}, \text{ for all }t. \end{align*} |
Therefore, \tilde{\Omega}_{7} = \left \{ \Xi_{7}\right \} and by employing L-LAST, we get \Xi_{7} is G.A.S. Based on the above findings, we summarize the existence and global stability conditions for all equilibrium points in Table 1.
Equilibrium point | \text{Existence conditions} | Global stability \text{ conditions} |
\Xi_{0}=(X_{0}, 0, 0, 0, 0, 0, 0, 0, 0) | None | \Re_{1}\leq1 and \Re_{2}\leq 1 |
\Xi_{1}=(X_{1}, L_{1}, 0, Y_{1}, 0, V_{1}, 0, 0, 0) | \Re_{1} > 1 | \Re_{1} > 1 , \Re_{2}/\Re_{1}\leq1 and \Re_{3}\leq1 |
\Xi_{2}=(X_{2}, 0, E_{2}, 0, I_{2}, 0, P_{2}, 0, 0) | \Re_{2} > 1 | \Re_{2} > 1 , \Re_{1}/\Re_{2}\leq1 and \Re_{4}\leq1 |
\Xi_{3}=(X_{3}, L_{3}, 0, Y_{3}, 0, V_{3}, 0, Z_{3}, 0) | \Re_{3} > 1 | \Re_{3} > 1 and \Re_{5}\leq1 |
\Xi_{4}=(X_{4}, 0, E_{4}, 0, I_{4}, 0, P_{4}, 0, M_{4}) | \Re_{4} > 1 | \Re_{4} > 1 and \Re_{6}\leq1 |
\Xi_{5}=(X_{5}, L_{5}, E_{5}, Y_{5}, I_{5}, V_{5}, P_{5}, Z_{5}, 0) | \Re_{5} > 1 and \Re_{1}/\Re_{2} > 1 | \Re_{5} > 1 , \Re_{8}\leq1 and \Re _{1}/\Re_{2} > 1 |
\Xi_{6}=(X_{6}, L_{6}, E_{6}, Y_{6}, I_{6}, V_{6}, P_{6}, 0, M_{6}) | \Re_{6} > 1 and \Re_{2}/\Re_{1} > 1 | \Re_{6} > 1 , \Re_{7}\leq1 and \Re_{2}/\Re_{1} > 1 |
\Xi_{7}=(X_{7}, L_{7}, E_{7}, Y_{7}, I_{7}, V_{7}, P_{7}, Z_{7}, M_{7}) | \Re _{7} > 1 and \Re_{8} > 1 | \Re_{7} > 1 and \Re_{8} > 1 |
We noted that system (2.1) has eight equilibria for which the coexistence case of IAV and SARS-CoV-2 can only be occurred if at least one type of the specific antibody immunities is active. Now, we discuss the importance of considering the antibody immune response in the IAV/SARS-CoV-2 dynamics model. If the antibody immune response is neglected then system (2.1) becomes:
\begin{equation} \left \{ \begin{array} [c]{l} \dot{X} = \lambda-\alpha X-\beta_{V}XV-\beta_{P}XP, \\ \dot{L} = \beta_{V}XV-(\eta_{L}+\delta_{L})L, \\ \dot{E} = \beta_{P}XP-(\eta_{E}+\delta_{E})E, \\ \dot{Y} = \delta_{L}L-\gamma_{Y}Y, \\ \dot{I} = \delta_{E}E-\gamma_{I}I, \\ \dot{V} = \kappa_{V}Y-\pi_{V}V, \\ \dot{P} = \kappa_{P}I-\pi_{P}P. \end{array} \right. \end{equation} | (6.1) |
We can see that system (6.1) describes the competition between IAV and SARS-CoV-2 on one source of target cells, epithelial cells. The model admits only three equilibria:
(i) Infection-free equilibrium, \tilde{\Xi}_{0} = (\tilde{X} _{0}, 0, 0, 0, 0, 0, 0) , where both IAV and SARS-CoV-2 are cleared,
(ii) SARS-CoV-2 single-infection equilibrium \tilde{\Xi}_{1} = (\tilde{X}_{1}, \tilde{L}_{1}, 0, \tilde{Y}_{1}, 0, \tilde{V}_{1}, 0) , where the IAV is blocked,
(iii) IAV single-infection equilibrium, \tilde{\Xi}_{2} = (\tilde {X}_{2}, 0, \tilde{E}_{2}, 0, \tilde{I}_{2}, 0, \tilde{P}_{2}) , where the SARS-CoV-2 is blocked, where \tilde{X}_{i} = X_{i} , i = 0, 1, 2 , \tilde{L}_{1} = L_{1} , \tilde{Y}_{1} = Y_{1} , \tilde{V}_{1} = V_{1} , \tilde{E}_{2} = E_{2} , \tilde {I}_{2} = I_{2}, and \tilde{P}_{2} = P_{2} .
We note that the case of IAV and SARS-CoV-2 coexistence does not appear. In the recent studies presented in [5,9,11,12], it was recorded that some COVID-19 patients were detected to be coinfected with IAV. Therefore, neglecting the immune response may not describe the coinfection dynamics accurately. This supports the idea of including the immune response into the IAV/SARS-CoV-2 coinfection model, where the case of IAV and SARS-CoV-2 coexistence is observed.
The global stability of the system's equilibria will be illustrated numerically. We use the values of the parameters presented in Table 2. In addition, we make a comparison between single-infection and coinfection.
Parameter | Value | Parameter | Value | Parameter | Value | Parameter | Value |
\lambda | 0.5 | \gamma_{I} | 0.2 | \varkappa_{V} | 0.05 | \mu _{M} | 0.04 |
\alpha | 0.05 | \kappa_{V} | 0.2 | \varkappa_{P} | 0.04 | \eta_{L} | 0.05 |
\beta_{V} | Varied | \kappa_{P} | 0.4 | \sigma_{Z} | Varied | \eta_{E} | 0.06 |
\beta_{P} | Varied | \pi_{V} | 0.2 | \sigma_{M} | Varied | \delta _{L} | 0.05 |
\gamma_{Y} | 0.11 | \pi_{P} | 0.1 | \mu_{Z} | 0.05 | \delta_{E} | 0.06 |
Now, we solve system (2.1) with three different initial conditions (states) as:
\begin{align*} \text{C1} & :\left( X(0), L\left( 0\right) , E\left( 0\right) , Y(0), I(0), V(0), P(0), Z(0), M(0)\right) = \left( 8, 0.5, 1, 1, 0.5, 1, 0.5, 1, 4\right) , \\ \text{C2} & :\left( X(0), L\left( 0\right) , E\left( 0\right) , Y(0), I(0), V(0), P(0), Z(0), M(0)\right) = \left( 7, 1, 1.5, 1.5, 0.7, 1.5, 0.8, 2, 6\right) , \\ \text{C3} & :\left( X(0), L\left( 0\right) , E\left( 0\right) , Y(0), I(0), V(0), P(0), Z(0), M(0)\right) = \left( 6, 1.5, 2, 2, 1, 2, 1.4, 3, 8\right) . \end{align*} |
Selecting the values of \beta_{V} , \beta_{P} , \sigma_{Z} and \sigma_{M} leads to the following situations:
Situation 1 (Stability of \Xi_{0} ): \beta_{V} = 0.001, \; \beta_{P} = 0.001 , \sigma_{Z} = 0.01 and \sigma_{M} = 0.02 . For these values of parameters, we have \Re_{1} = 0.0455 < 1 and \Re_{2} = 0.1 < 1 . Figure 2 shows that the trajectories tend to the equilibrium \Xi_{0} = (10, 0, 0, 0, 0, 0, 0, 0, 0) for all initials C1-C3. This demonstrates that, \Xi_{0} is G.A.S based on Theorem 1. In this situation, both SARS-CoV-2 and IAV will be removed.
Situation 2 (Stability of \Xi_{1} ): \beta_{V} = 0.05, \; \beta_{P} = 0.001, \sigma_{Z} = 0.002 and \sigma_{M} = 0.02 . With such selection we obtain \Re_{1} = 2.2727 > 1 , \Re _{3} = 0.0874 < 1 and hence \Re_{2}/\Re_{1} = 0.044 < 1 . The equilibrium point \Xi_{1} exists with \Xi_{1} = (4.4, 2.8, 0, 1.27, 0, 1.27, 0, 0, 0) . It is clear from Figure 3 that, the trajectories tend to \Xi_{1} for all initials C1-C3. Thus, the numerical results agree with Theorem 2. This case simulates a SARS-CoV-2 single-infection without antibody immunity.
Situation 3 (Stability of \Xi_{2} ): \beta_{V} = 0.005, \; \beta_{P} = 0.03 , \sigma_{Z} = 0.01 and \sigma_{M} = 0.001 . This gives \Re_{2} = 3 > 1 , \Re_{4} = 0.12 < 1 and then \Re_{1}/\Re_{2} = 0.0758 < 1 . The numerical results show that, \Xi_{2} = \left(3.33, 0, 2.78, 0, 0.83, 0, 3.33, 0, 0\right) exists. We can observe from Figure 4 that, the trajectories converge to \Xi_{2} regardless of the initial states. This result supports the result of Theorem 3. This situation represents an IAV single-infection without antibody immunity.
Situation 4 (Stability of \Xi_{3} ): \beta_{V} = 0.09, \; \beta_{P} = 0.002 , \sigma_{Z} = 0.05 and \sigma_{M} = 0.05 . This yields \Re_{3} = 1.461 > 1 and \Re_{5} = 0.0714 < 1 . Figure 5 shows that the trajectories tend to \Xi_{3} = (3.57, 3.21, 0, 1.46, 0, 1, 0, 1.84, 0) regardless of the initial states. Therefore, \Xi_{3} is G.A.S and this supports Theorem 4. Hence, a SARS-CoV-2 single-infection with stimulated SARS-CoV-2-specific antibody is attained.
Situation 5 (Stability of \Xi_{4} ): \beta_{V} = 0.01, \; \beta_{P} = 0.1 , \sigma_{Z} = 0.01 and \sigma _{M} = 0.02 . The values of \Re_{4} and \Re_{6} are computed as \Re _{4} = 2 > 1 and \Re_{6} = 0.0909 < 1 . Thus, \Xi_{4} exists with \Xi _{4} = (2, 0, 3.33, 0, 1, 0, 2, 0, 2.5) . In Figure 6 we see that the trajectories tend to \Xi_{4} regardless of the initial states. It follows that \Xi_{4} is G.A.S according to Theorem 5. Hence, an IAV single-infection with activated IAV-specific antibody is achieved.
Situation 6 (Stability of \Xi_{5} ): \beta_{V} = 0.09, \; \beta_{P} = 0.02 , \sigma_{Z} = 0.095 and \sigma_{M} = 0.009 . Then, we calculate \Re_{5} = 1.027 > 1 , \Re_{8} = 0.5369 < 1 and \Re_{1}/\Re_{2} = 2.0455 > 1 The numerical results drawn in Figure 7 show that \Xi_{5} = (5, 2.37, 0.11, 1.08, 0.03, 0.53, 0.13, 4.18, 0) exists and it is G.A.S and this is consistent with Theorem 6. As a result, a coinfection with SARS-CoV-2 and IAV is attained where only SARS-CoV-2-specific antibody is stimulated.
Situation 7 (Stability of \Xi_{6} ): \beta_{V} = 0.09, \; \beta_{P} = 0.09 , \sigma_{Z} = 0.03 and \sigma _{M} = 0.03 . We compute \Re_{6} = 1.2032 > 1 , \Re_{7} = 0.6392 < 1 and \Re_{2} /\Re_{1} = 2.2 > 1 . We find that, the equilibrium \Xi_{6} = \left(2.44, 0.84, 2.44, 0.38, 0.73, 0.38, 1.33, 0, 3\right) exists. Further, the numerical solutions outlined in Figure 8 show that, \Xi_{6} is G.A.S and this boosts the result of Theorem 7. In this situation, a coinfection with SARS-CoV-2 and IAV are attained where only IAV-specific antibody is activated.
Situation 8 (Stability of \Xi_{7} ): \beta_{V} = 0.09, \; \beta_{P} = 0.09 , \sigma_{Z} = 0.5 and \sigma _{M} = 0.5 . This selection yields \Re_{7} = 3.0898 > 1 and \Re_{8} = 6.7976 > 1 . Figure 9 shows that \Xi_{7} = (7.55, 0.68, 0.45, 0.31, 0.14, 0.1, 0.08, 8.36, 14.49) exists and it is G.A.S based on Theorem 8. In this situation, a coinfection with SARS-CoV-2 and IAV is established regardless of the initial states. In this case, both SARS-CoV-2-specific antibodies and IAV-specific antibodies are working against the coinfection.
For more confirmation, we investigate the local stability of the system's equilibria. Calculating the Jacobian matrix J = J(X, L, E, Y, I, V, P, Z, M) of system (2.1) as:
J = \left( \begin{array} [c]{ccccccccc} J\_{11} & 0 & 0 & 0 & 0 & -\beta\_{V}X & -\beta\_{P}X & 0 & 0\\ \beta\_{V}V & J_{22} & 0 & 0 & 0 & \beta\_{V}X & 0 & 0 & 0\\ \beta\_{P}P & 0 & J_{33} & 0 & 0 & 0 & \beta\_{P}X & 0 & 0\\ 0 & \delta\_{L} & 0 & -\gamma\_{Y} & 0 & 0 & 0 & 0 & 0\\ 0 & 0 & \delta\_{E} & 0 & -\gamma\_{I} & 0 & 0 & 0 & 0\\ 0 & 0 & 0 & \kappa\_{V} & 0 & J_{66} & 0 & -\varkappa\_{V}V & 0\\ 0 & 0 & 0 & 0 & \kappa\_{P} & 0 & J_{77} & 0 & -\varkappa\_{P}P\\ 0 & 0 & 0 & 0 & 0 & \sigma\_{Z}Z & 0 & J_{88} & 0\\ 0 & 0 & 0 & 0 & 0 & 0 & \sigma\_{M}M & 0 & J_{99} \end{array} \right) |
where J_{11} = -\left(\alpha+\beta_{V}V+\beta_{P}P\right), \ \ J_{22} = -\left(\eta_{L}+\delta_{L}\right), \ \ J_{33} = -\left(\eta_{E}+\delta_{E}\right), \ \ J_{66} = -\left(\pi_{V}+\varkappa_{V}Z\right), J_{77} = -\left(\pi_{P}+\varkappa_{P}M\right), \ \ J_{88} = \sigma_{Z}V-\mu_{Z}, \ \ J_{99} = \sigma_{M}P-\mu_{M}.
At each equilibrium, we compute the eigenvalues \lambda_{j}, \; j = 1, 2, ..., 9 of J. If \operatorname{Re}(\lambda_{j}) < 0, \; i = 1, 2, ..., 9 , then the equilibrium point is locally stable. We select the parameters \beta_{V} , \beta_{P} , \sigma_{Z} and \sigma_{M} as given in situations 1-8, then we compute all nonnegative equilibria and the accompanying eigenvalues. Table 3 outlined the nonnegative equilibria, the real parts of the eigenvalues and whether or not the equilibrium point is stable.
Situatio | The equilibria | Re(λj), j = 1, 2, …, 9 | Stability |
1 | \Xi 0 = (10, 0, 0, 0, 0, 0, 0, 0, 0) | (−0.18, −0.18, −0.18, −0.15, −0.08, −0.07, −0.05, −0.05, −0.04) | stable |
2 | \Xi 0 = (10, 0, 0, 0, 0, 0, 0, 0, 0) | (−0.22, −0.22, −0.18, −0.18, −0.07, −0.05, −0.05, −0.04, 0.04) | unstable |
\Xi 1 = (4.4, 2.8, 01.27, 0, 1.27, 0, 0, 0) | (−0.22, −0.22, −0.17, −0.17, −0.08, −0.04, −0.04, −0.05, −0.04) | stable | |
3 | \Xi 0 = (10, 0, 0, 0, 0, 0, 0, 0, 0) | (−0.24, −0.24, −0.18, −0.18, 0.06, −0.05, −0.05, −0.05, −0.04) | unstable |
\Xi 2 = (3.33, 0, 2.78, 0, 0.83, 0, 3.33, 0, 0) | (−0.24, −0.24, −0.17, −0.17, −0.07, −0.05, −0.05, −0.05, −0.04) | stable | |
4 | \Xi 0 = (10, 0, 0, 0, 0, 0, 0, 0, 0) | (−0.24, −0.24, −0.18, −0.18, 0.08, −0.05, −0.05, −0.05, −0.04) | unstable |
\Xi 1 = (2.44, 3.78, 0, 1.27, 0, 1.27, 0, 0, 0) | (−0.25, −0.25, −0.17, −0.17, −0.08, −0.06, −0.06, −0.04, 0.04) | unstable | |
\Xi 3 = (3.57, 3.21, 0, 1.46, 0, 1, 0, 1.84, 0) | (−0.27, −0.27, −0.17, −0.17, −0.07, −0.04, −0.04, −0.04, −0.03) | stable | |
5 | \Xi 0 = (10, 0, 0, 0, 0, 0, 0, 0, 0) | (−0.29, −0.29, −0.19, −0.19, 0.15, −0.05, −0.05, −0.04, −0.03) | unstable |
\Xi 2 = (1, 0, 3.75, 0, 1.13, 0, 4.5, 0, 0) | (−0.47, −0.29, −0.18, −0.15, −0.08, −0.08, −0.08, −0.05, 0.05) | unstable | |
\Xi 4 = (2, 0, 3.33, 0, 1, 0, 2, 0, 2.5) | (−0.31, −0.31, −0.17, −0.17, −0.06, −0.06, −0.07, −0.05, −0.03) | stable | |
6 | \Xi 0 = (10, 0, 0, 0, 0, 0, 0, 0, 0) | (−0.24, −0.24, −0.23, −0.23, 0.08, −0.05, −0.05, −0.04, 0.03) | unstable |
\Xi 1 = (2.44, 3.78, 0, 1.72, 0, 1.72, 0, 0, 0) | (−0.25, −0.25, −0.2, −0.2, 0.11, −0.06, −0.06, −0.04, −0.03) | unstable | |
\Xi 2 = (5, 0, 2.08, 0, 0.63, 0, 2.5, 0, 0) | (−0.22, −0.22, −0.22, −0.22, −0.05, −0.04, −0.04, 0.04, −0.02) | unstable | |
\Xi 3 = (5.14, 2.43, 0, 1.11, 0, 0.53, 0, 4.4, 0) | (−0.32, −0.32, −0.21, −0.21, −0.02, −0.02, −0.04, −0.04, 0.001) | unstable | |
\Xi 5 = (5, 2.37, 0.11, 1.08, 0.03, 0.53, 0.13, 4.18, 0) | (−0.31, −0.31, −0.21, −0.21, −0.02, −0.02, −0.04, −0.03, −0.001) | stable | |
7 | \Xi 0 = (10, 0, 0, 0, 0, 0, 0, 0, 0) | (−0.28, −0.28, −0.24, −0.24, 0.14, 0.07, −0.05, −0.05, −0.04) | unstable |
\Xi 1 = (2.44, 3.78, 0, 1.72, 0, 1.72, 0, 0, 0) | (−0.25, −0.25, −0.23, −0.23, −0.06, −0.06, −0.04, 0.04, 0.002) | unstable | |
\Xi 2 = (1.11, 0, 3.71, 0, 1.11, 0, 4.44, 0, 0) | (−0.4, −0.31, −0.19, −0.19, 0.09, −0.08, −0.08, −0.05, −0.03) | unstable | |
\Xi 3 = (2.5, 3.75, 0, 1.7, 0, 1.67, 0, 0.09, 0) | (−0.25, −0.25, −0.23, −0.23, −0.06, −0.06, 0.04, −0.04, −0.001) | unstable | |
\Xi 4 = (2.94, 0, 2.94, 0, 0.88, 0, 1.33, 0, 4.12) | (−0.32, −0.32, −0.21, −0.21, −0.04, −0.04, −0.05, −0.04, 0.008) | unstable | |
\Xi 6 = (2.44, 0.84, 2.44, 0.38, 0.73, 0.38, 1.33, 0, 3) | (−0.3, −0.3, −0.21, −0.21, −0.05, −0.05, −0.04, −0.02, −0.02) | stable | |
8 | \Xi 0 = (10, 0, 0, 0, 0, 0, 0, 0, 0) | (−0.28, −0.28, −0.24, −0.24, 0.14, 0.08, −0.05, −0.05, −0.04) | unstable |
\Xi 1 = (2.44, 3.78, 0, 1.72, 0, 1.72, 0, 0, 0) | (0.81, −0.25, −0.25, −0.23, −0.23, −0.06, −0.06, −0.04, 0.04) | unstable | |
\Xi 2 = (1.11, 0, 3.7, 0, 1.11, 0, 4.44, 0, 0) | (2.18, −0.4, −0.31, −0.19, −0.19, −0.08, −0.08, −0.05, −0.03) | unstable | |
\Xi 3 = (8.47, 0.76, 0, 0.35, 0, 0.1, 0, 9.87, 0) | (−0.63, −0.27, −0.27, −0.26, 0.13, −0.05, −0.01, −0.01, −0.04) | unstable | |
\Xi 4 = (8.74, 0, 0.52, 0, 0.16, 0, 0.08, 0, 17.17) | (−0.67, −0.41, −0.24, −0.24, 0.07, −0.01, −0.01, −0.05, −0.05) | unstable | |
\Xi 6 = (2.44, 9.27, 0.15, 1.64, 0.04, 1.64, 0.08, 0, 3) | (0.77, −0.27, −0.27, −0.25, −0.25, −0.06, −0.06, −0.006, −0.006) | unstable | |
\Xi 7 = (7.55, 0.68, 0.45, 0.31, 0.14, 0.1, 0.08, 8.36, 14.49) | (−0.54, −0.49, −0.49, −0.27, −0.02, −0.02, −0.05, −0.01, −0.01) | stable |
In this subsection, we present a comparison between the single-infection and coinfection.
Here, we compare the solutions of model (2.1) and the following SARS-CoV-2 single-infection model:
\begin{equation} \left \{ \begin{array} [c]{l} \dot{X} = \lambda-\alpha X-\beta_{V}XV, \\ \dot{L} = \beta_{V}XV-\left( \eta_{L}+\delta_{L}\right) L, \\ \dot{Y} = \delta_{L}L-\gamma_{Y}Y, \\ \dot{V} = \kappa_{V}Y-\pi_{V}V-\varkappa_{V}VZ, \\ \dot{Z} = \sigma_{Z}VZ-\mu_{Z}Z. \end{array} \right. \end{equation} | (7.1) |
We fix parameters \beta_{V} = 0.09 , \beta_{P} = 0.05 , \sigma_{Z} = 0.5 , and \sigma_{M} = 0.9 and select the initial state as:
\text{C4}:\left( X(0), L\left( 0\right) , E\left( 0\right) , Y(0), I(0), V(0), P(0), Z(0), M(0)\right) = \left( 7.5, 0.3, 5, 0.5, 0.4, 0.05, 0.04, 9, 9.5\right) . |
From Figure 10 we observe that when the SARS-CoV-2 single-infected individual is coinfected with IAV, then the concentrations of uninfected epithelial cells, latent SARS-CoV-2-infected cells, active SARS-CoV-2-infected cells and SARS-CoV-2-specific antibodies are reduced. However, the concentration of free SARS-CoV-2 particles tends to be the same value in both SARS-CoV-2 single-infection and IAV/SARS-CoV-2 coinfection. This result agrees with the observation of Ding et al. [11] which said that "IAV/SARS-CoV-2 coinfection did not result in worse clinical outcomes in comparison with SARS-CoV-2 single-infection".
To examine the impact of SARS-CoV-2 infection on IAV single-infection, we compare the solutions of model (2.1) and the following IAV single-infection model:
\begin{equation} \left \{ \begin{array} [c]{l} \dot{X} = \lambda-\alpha X-\beta_{P}XP, \\ \dot{E} = \beta_{P}XV-\left( \eta_{E}+\delta_{E}\right) E, \\ \dot{I} = \delta_{E}E-\gamma_{I}I, \\ \dot{P} = \kappa_{P}I-\pi_{P}P-\varkappa_{P}PM, \\ \dot{M} = \sigma_{M}PM-\mu_{M}M. \end{array} \right. \end{equation} | (7.2) |
We fix parameters \beta_{V} = \; 0.09 , \beta_{P} = 0.08, \; \sigma_{Z} = 0.07 and \sigma_{M} = 0.05 and consider the following initial condition:
\text{C5}:(X(0), L\left( 0\right) , E\left( 0\right) , Y(0), I(0), V(0), P(0), Z(0), M(0)) = \left( 4, 1, 5, 0.6, 0.5, 0.2, 0.05, 3, 8\right) . |
It can be observed from Figure 11 that, when the IAV single-infected individual is coinfected with SARS-CoV-2 then the concentrations of uninfected epithelial cells, latent IAV-infected cells, active IAV-infected cells and IAV-specific antibodies are decreased. However, the concentration of free IAV particles tend to the same value in both IAV single-infection and IAV/SARS-CoV-2 coinfection.
IAV and SARS-CoV-2 coinfection cases were reported in some works (see [5,9,11,12]). Therefore, it is important to understand the within-host dynamics of this coinfection. In this paper, we develop and examine a within-host IAV/SARS-CoV-2 coinfection model. The model considered the interactions between uninfected epithelial cells, latent SARS-CoV-2-infected cells, latent IAV-infected cells, active SARS-CoV-2-infected cells, active IAV-infected cells, free SARS-CoV-2 particles, free IAV particles, SARS-CoV-2-specific antibodies and IAV-specific antibodies. We examined the nonnegativity and boundedness of the solutions. We found that the system has eight equilibria and we proved the following:
(I) The infection-free equilibrium \Xi_{0} always exists. It is G.A.S when \Re_{1}\leq1 and \Re_{2}\leq1 . In this case, the patient is recovered from both IAV and SARS-CoV-2.
(II) The SARS-CoV-2 single-infection equilibrium without antibody immunity \Xi_{1} exists if \Re_{1} > 1 . It is G.A.S when \Re_{1} > 1 , \Re_{2} /\Re_{1}\leq1 and \Re_{3}\leq1 . This case leads to the situation of the patient only infected by SARS-CoV-2 with an inactive immune response. As we will see below that if both SARS-CoV-2-specific antibody and IAV-specific antibody immunities are not activated against the two viruses, then according to the competition between the two viruses, SARS-CoV-2 may be able to block the IAV.
(III)- The IAV single-infection equilibrium without antibody immunity \Xi _{2} exists if \Re_{2} > 1 . It is G.A.S when \Re_{2} > 1 , \Re_{1}/\Re _{2}\leq1 and \Re_{4}\leq1 . This case leads to the situation of the patient only infected by IAV with an unstimulated immune response. Then, IAV may be able to block the SARS-CoV-2.
(IV) The SARS-CoV-2 single-infection equilibrium with stimulated SARS-CoV-2-specific antibody immunity \Xi_{3} exists if \Re_{3} > 1 . It is G.A.S when \Re_{3} > 1 and \Re_{5}\leq1 . This point represents the situation of SARS-CoV-2 single-infection patient with active SARS-CoV-2-specific antibody immunity.
(V) The IAV single-infection equilibrium with stimulated IAV-specific antibody immunity \Xi_{4} exists if \Re_{4} > 1 . It is G.A.S when \Re_{4} > 1 and \Re_{6}\leq1 . This point represents the case of IAV single-infection patient with active IAV-specific antibody immunity.
(VI) The IAV/SARS-CoV-2 coinfection equilibrium with only stimulated SARS-CoV-2-specific antibody immunity \Xi_{5} exists if \Re_{5} > 1 and \Re_{1}/\Re_{2} > 1 . It is G.A.S when \Re_{5} > 1 , \Re_{8}\leq1 and \Re _{1}/\Re_{2} > 1 . Here, the IAV/SARS-CoV-2 coinfection occurs with only stimulated SARS-CoV-2-specific antibody immunity.
(VII) The IAV/SARS-CoV-2 coinfection equilibrium with only stimulated IAV-specific antibody immunity \Xi_{6} exists if \Re_{6} > 1 and \Re _{2}/\Re_{1} > 1 . It is G.A.S when \Re_{6} > 1 , \Re_{7}\leq1 and \Re_{2} /\Re_{1} > 1 . It means that the IAV/SARS-CoV-2 coinfection occurs with only stimulated IAV-specific antibody immunity.
(VIII) The IAV/SARS-CoV-2 coinfection equilibrium with stimulated both SARS-CoV-2-specific antibodies and IAV-specific antibody immunities \Xi_{7} exists and it is G.A.S if \Re_{7} > 1 and \Re_{8} > 1 . It means that, the IAV/SARS-CoV-2 coinfection occurs with both SARS-CoV-2-specific antibodies and IAV-specific antibody immunities are activated.
The global stability of equilibria was established using the Lyapunov method. We performed numerical simulations and demonstrated that they are in good agreement with the theoretical results. We discussed the influence of IAV infection on SARS-CoV-2 single-infection dynamics and vice versa. We found that the concentration of free IAV or SARS-CoV-2 particles cells tends to be the same value in both single-infection and coinfection. This agrees with the work of Ding et al. [11] which reported that IAV/SARS-CoV-2 coinfection did not result in worse clinical outcomes. In addition, the spread of seasonal influenza can increase the likelihood of coinfection in patients with COVID-19 [9].
The model developed in this work can be improved by (i) utilizing real data to find a good estimation of the parameters' values, (ii) studying the effect of time delays that occur during infection or production of IAV and SARS-CoV-2 particles, (iii) considering viral mutations [64,65], (iv) considering the effect of treatments on the progression of both viruses, and (v) including the influence of CTLs in killing SARS-CoV-2-infected and IAV-infected cells. Memory is an important characteristic of viral infections and immune response. It will be important to address the effect of memory on the dynamics of IAV/SARS-CoV-2 coinfection by formulation of the model via fractional differential equations [66,67,68].
The innate immune response is one of the major antiviral responses to explain host-pathogen interaction. Also, it is a trigger to induce adaptive immunity which is the major focus of our proposed model. Model (2.1) can be extended to include the effect of IFN response as:
\begin{align*} \dot{X} & = \lambda-\alpha X-\beta_{V}XV-\beta_{P}XP, \\ \dot{L} & = \beta_{V}XV-\eta_{L}L-\frac{\delta_{L}L}{1+\epsilon_{L}F}, \\ \dot{E} & = \beta_{P}XP-\eta_{E}E-\frac{\delta_{E}E}{1+\epsilon_{E}F}, \\ \dot{Y} & = \frac{\delta_{L}L}{1+\epsilon_{L}F}-\gamma_{Y}Y, \\ \dot{I} & = \frac{\delta_{E}E}{1+\epsilon_{E}F}-\gamma_{I}I, \\ \dot{V} & = \frac{\kappa_{V}Y}{1+\epsilon_{V}F}-\pi_{V}V-\varkappa_{V}VZ, \\ \dot{P} & = \frac{\kappa_{P}I}{1+\epsilon_{P}F}-\pi_{P}P-\varkappa_{P}PM, \\ \dot{Z} & = \sigma_{Z}VZ-\mu_{Z}Z, \\ \dot{M} & = \sigma_{M}PM-\mu_{M}M, \\ \dot{F} & = \varpi_{F}(Y(t-\tau)+I(t-\tau))-\mu_{F}F. \end{align*} |
where \epsilon_{L} , \epsilon_{E} , \epsilon_{V} and \epsilon_{P} are the efficiencies of the IFN effects. These research points need further investigations so we leave them to future works.
This research work was funded by Institutional Fund Projects under grant no. (IFPIP:69-130-1443). The authors gratefully acknowledge technical and financial support provided by the Ministry of Education and King Abdulaziz University, DSR, Jeddah, Saudi Arabia.
[1] | World Health Organization (WHO), Coronavirus disease (COVID-19), weekly epidemiological update (2 October 2022), 2022. Available from: https://www.who.int/publications/m/item/weekly-epidemiological-update-on-covid-19—5-october-2022. |
[2] |
A. D. Iuliano, K. M. Roguski, H. H. Chang, D. J. Muscatello, R. Palekar, S. Tempia, et al., Estimates of global seasonal influenza-associated respiratory mortality: A modelling study, Lancet, 391 (2018), 1285–1300. https://doi.org/10.1016/S0140-6736(17)33293-2 doi: 10.1016/S0140-6736(17)33293-2
![]() |
[3] |
B. Hancioglu, D. Swigon, G. Clermont, A dynamical model of human immune response to influenza A virus infection, J. Theor. Biol., 246 (2007), 70–86. https://doi.org/10.1016/j.jtbi.2006.12.015 doi: 10.1016/j.jtbi.2006.12.015
![]() |
[4] |
Z. Varga, A. J. Flammer, P. Steiger, M. Haberecker, R. Andermatt, A. S. Zinkernagel, et al., Endothelial cell infection and endotheliitis in COVID-19, Lancet, 395 (2020), 1417–1418. https://doi.org/10.1016/S0140-6736(20)30937-5 doi: 10.1016/S0140-6736(20)30937-5
![]() |
[5] |
R. Ozaras, Influenza and COVID-19 coinfection: Report of six cases and review of the literature, J. Med. Virol, 92 (2020), 2657–2665. https://doi.org/10.1002/jmv.26125 doi: 10.1002/jmv.26125
![]() |
[6] | World Health Organization (WHO), Influenza Update No. 428, (19 September 2022), 2022. Available from: https://www.who.int/publications/m/item/influenza-update-n-428. |
[7] | World Health Organization (WHO), Coronavirus disease (COVID-19), Vaccine tracker, 2022. Available from: https://covid19.trackvaccines.org/agency/who/. |
[8] |
R. F. Nuwarda, A. A. Alharbi, V. Kayser, An overview of influenza viruses and vaccines, Vaccines, 9 (2021), 1032. https://doi.org/10.3390/vaccines9091032 doi: 10.3390/vaccines9091032
![]() |
[9] |
X. Zhu, Y. Gea, T. Wua, K. Zhaoa, Y. Chena, B. Wu, et al., Co-infection with respiratory pathogens among COVID-2019 cases, Virus Research, 285 (2020), 198005. https://doi.org/10.1016/j.virusres.2020.198005 doi: 10.1016/j.virusres.2020.198005
![]() |
[10] |
P. S. Aghbash, N. Eslami, M. Shirvaliloo, H. B. Baghi, Viral coinfections in COVID-19, J. Med. Virol., 93 (2021), 5310–5322. https://doi.org/10.1002/jmv.27102 doi: 10.1002/jmv.27102
![]() |
[11] |
Q. Ding, P. Lu, Y. Fan, Y. Xia, M. Liu, The clinical characteristics of pneumonia patients coinfected with 2019 novel coronavirus and influenza virus in Wuhan, China, J. Med. Virol., 92 (2020), 1549–1555. https://doi.org/10.1002/jmv.25781 doi: 10.1002/jmv.25781
![]() |
[12] | G. Wang, M. Xie, J. Ma, J. Guan, Y. Song, Y. Wen, et al., Is co-infection with influenza virus a protective factor of COVID-19?, 2020. |
[13] | M. Wang, Q. Wu, W. Xu, B. Qiao, J. Wang, H. Zheng, et al., Clinical diagnosis of 8274 samples with 2019-novel coronavirus in Wuhan, MedRxiv, (2020). https://doi.org/10.1101/2020.02.12.20022327 |
[14] |
L. Lansbury, B. Lim, V. Baskaran, W. S. Lim, Co-infections in people with COVID-19: A systematic review and meta-analysis, J. Infect., 81 (2020), 266–275. https://doi.org/10.1016/j.jinf.2020.05.046 doi: 10.1016/j.jinf.2020.05.046
![]() |
[15] |
T. L. Dao, P. Colson, M. Million, P. Gautret, Co-infection of SARS-CoV-2 and influenza viruses: A systematic review and meta-analysis, J. Clin. Virol., 1 (2021), 100036. https://doi.org/10.1016/j.jcvp.2021.100036 doi: 10.1016/j.jcvp.2021.100036
![]() |
[16] |
H. Ghaznavi, M. Shirvaliloo, S. Sargazi, Z. Mohammadghasemipour, Z. Shams, Z. Hesari, et al., SARS-CoV-2 and influenza viruses: Strategies to cope with coinfection and bioinformatics perspective, CBI, 46 (2022), 1009–1020. https://doi.org/10.1002/cbin.11800 doi: 10.1002/cbin.11800
![]() |
[17] |
H. Khorramdelazada, M. H. Kazemib, A. Najafib, M. Keykhaeee, R. Z. Emameh, R. Falak, Immunopathological similarities between COVID-19 and influenza: Investigating the consequences of co-infection, Microb. Pathog., 152 (2021), 104554. https://doi.org/10.1016/j.micpath.2020.104554 doi: 10.1016/j.micpath.2020.104554
![]() |
[18] | X. Xiang, Z. Wang, L. Ye, X. He, X. Wei, Y. Ma, et al., Co-infection of SARS-COV-2 and influenza A virus: A case series and fast review, Curr. Med. Sci.41 (2021), 51–57. https://doi.org/10.1007/s11596-021-2317-2 |
[19] |
H. Yue, M. Zhang, L. Xing, K. Wang, X. Rao, H. Liu, et al., The epidemiology and clinical characteristics of co-infection of SARS-CoV-2 and influenza viruses in patients during COVID-19 outbreak, J. Med. Virol., 92 (2020), 2870–2873. https://doi.org/10.1002/jmv.26163 doi: 10.1002/jmv.26163
![]() |
[20] |
A. J. Zhang, A. C. Lee, J. F. Chan, F. Liu, C. Li, Y. Chen, H. Chu, et al., Coinfection by severe acute respiratory syndrome coronavirus 2 and influenza A (H1N1) pdm09 virus enhances the severity of pneumonia in golden Syrian hamsters, Clin. Infect. Dis., 72 (2021), e978–e992. https://doi.org/10.1093/cid/ciaa1747 doi: 10.1093/cid/ciaa1747
![]() |
[21] |
M. D. Nowak, E. M. Sordillo, M. R. Gitman, A. E. P. Mondolfi, Coinfection in SARS-CoV-2 infected patients: Where are influenza virus and rhinovirus/enterovirus?, J. Med. Virol., 92 (2020), 1699. https://doi.org/10.1002/jmv.25953 doi: 10.1002/jmv.25953
![]() |
[22] |
P. J. Halfmann, N. Nakajima, Y. Sato, K. Takahashi, M. Accola, S. Chiba, et al., SARS-CoV-2 interference of influenza virus replication in Syrian hamsters, JID, 225 (2022), 282–286. https://doi.org/10.1093/infdis/jiab587 doi: 10.1093/infdis/jiab587
![]() |
[23] |
K. Oishi, S. Horiuchi, J. M. Minkoff, B. R. tenOever, The host response to influenza A virus interferes with SARS-CoV-2 replication during coinfection, J. Virol., 96 (2022), e0076522. https://doi.org/10.1128/jvi.00765-22 doi: 10.1128/jvi.00765-22
![]() |
[24] |
L. Pinky, H. M. Dobrovolny, SARS-CoV-2 coinfections: Could influenza and the common cold be beneficial?, J. Med. Virol., 92 (2020), 2623–2630. https://doi.org/10.1002/jmv.26098 doi: 10.1002/jmv.26098
![]() |
[25] |
A. M. Smith, R. M. Ribeiro, Modeling the viral dynamics of influenza A virus infection, Crit. Rev. Immunol., 30 (2010), 291–298. https://doi.org/10.1615/critrevimmunol.v30.i3.60 doi: 10.1615/critrevimmunol.v30.i3.60
![]() |
[26] |
C. A. Beauchemin, A. Handel, A review of mathematical models of influenza A infections within a host or cell culture: Lessons learned and challenges ahead, BMC public health, 11 (2011), 1–15. https://doi.org/10.1186/1471-2458-11-S1-S7 doi: 10.1186/1471-2458-11-S1-S7
![]() |
[27] |
L. Canini, A. S. Perelson, Viral kinetic modeling: state of the art, J. Pharmacokinet, 41 (2014), 431–443. https://doi.org/10.1007/s10928-014-9363-3 doi: 10.1007/s10928-014-9363-3
![]() |
[28] |
A. Handel, L. E. Liao, C. A. Beauchemin, Progress and trends in mathematical modelling of influenza A virus infections, Curr. Opin. Syst. Biol., 12 (2018), 30–36. https://doi.org/10.1016/j.coisb.2018.08.009 doi: 10.1016/j.coisb.2018.08.009
![]() |
[29] |
A. Boianelli, V. K. Nguyen, T. Ebensen, K. Schulze, E. Wilk, N. Sharma, et al., Modeling influenza virus infection: a roadmap for influenza research, Viruses, 7 (2015), 5274–5304. https://doi.org/10.3390/v7102875 doi: 10.3390/v7102875
![]() |
[30] |
P. Baccam, C. Beauchemin, C. A. Macken, F. G. Hayden, A. S. Perelson, Kinetics of influenza A virus infection in humans, J. Virol., 80 (2006), 7590–7599. https://doi.org/10.1128/JVI.01623-05 doi: 10.1128/JVI.01623-05
![]() |
[31] |
A. M. Smith, A. S. Perelson, Influenza A virus infection kinetics: quantitative data and models, WIREs Systems Biology and Medicine, 3 (2011), 429–445. https://doi.org/10.1002/wsbm.129 doi: 10.1002/wsbm.129
![]() |
[32] | R. A. Saenz, M. Quinlivan, D. Elton, S. MacRae, A. S. Blunden, J. A. Mumford, et al., Dynamics of influenza virus infection and pathology, J. Virol. 84 (2010), 3974–3983. https://doi.org/10.1128/JVI.02078-09 |
[33] |
A. Tridane, Y. Kuang, Modeling the interaction of cytotoxic T lymphocytes and influenza virus infected epithelial cells, AIMS, 7 (2010), 171–185. https://doi.org/10.3934/mbe.2010.7.171 doi: 10.3934/mbe.2010.7.171
![]() |
[34] |
H. Y. Lee, D. J. Topham, S. Y. Park, J. Hollenbaugh, J. Treanor, T. R. Mosmann, et al., Simulation and prediction of the adaptive immune response to influenza A virus infection, J. Virol., 83 (2009), 7151–7165. https://doi.org/10.1128/JVI.00098-09 doi: 10.1128/JVI.00098-09
![]() |
[35] | E. A. Hernandez-Vargas, E. Wilk, L. Canini, F. R. Toapanta, S. C. Binder, A. Uvarovskii, et al., Effects of aging on influenza virus infection dynamics, J. Virol. 88 (2014), 4123–4131. http://dx.doi.org/10.1128/JVI.03644-13 |
[36] |
K. Li, J. M. McCaw, P. Cao, Modelling within-host macrophage dynamics in influenza virus infection, J. Theor. Biol., 508 (2021), 110492. https://doi.org/10.1016/j.jtbi.2020.110492 doi: 10.1016/j.jtbi.2020.110492
![]() |
[37] |
D. B. Chang, C. S. Young, Simple scaling laws for influenza A rise time, duration, and severity, J. Theor. Biol., 246 (2007), 621–635. https://doi.org/10.1016/j.jtbi.2007.02.004 doi: 10.1016/j.jtbi.2007.02.004
![]() |
[38] |
A. Handel, I. M. Longini Jr, R. Antia, Towards a quantitative understanding of the within-host dynamics of influenza A infections, J. R. Soc. Interface, 7 (2010), 35–47. https://doi.org/10.1098/rsif.2009.0067 doi: 10.1098/rsif.2009.0067
![]() |
[39] |
C. A. Beauchemin, J. J.McSharry, G. L.Drusano, J. T.Nguyen, G. T.Went, R. M.Ribeiro, et al., Modeling amantadine treatment of influenza A virus in vitro, J. Theor. Biol., 254 (2008), 439–451. https://doi.org/10.1016/j.jtbi.2008.05.031 doi: 10.1016/j.jtbi.2008.05.031
![]() |
[40] |
A. Handel, I. M. Longini Jr, R. Antia, Neuraminidase inhibitor resistance in influenza: Assessing the danger of its generation and spread, PLoS Comput. Biol., 3 (2007), e240. https://doi.org/10.1371/journal.pcbi.0030240 doi: 10.1371/journal.pcbi.0030240
![]() |
[41] |
B. Emerenini, R. Williams, R. N. G. R. Grimaldo, K. Wurscher, R. Ijioma, Mathematical modeling and analysis of influenza in-host infection dynamics, Lett. Biomath., 8 (2021), 229–253. https://doi.org/10.30707/LiB8.1.1647878866.124006 doi: 10.30707/LiB8.1.1647878866.124006
![]() |
[42] | M. Barik, C. Swarup, T. Singh, S. Habbi, S. Chauhan, Dynamical analysis, optimal control and spatial pattern in an influenza model with adaptive immunity in two stratified population, AIMS Math., 7(4) (2022), 4898–4935. http://dx.doi.org/10.3934/math.2022273 |
[43] |
E. A. Hernandez-Vargas, J. X. Velasco-Hernandez, In-host mathematical modelling of COVID-19 in humans, Annu. Rev. Control, 50 (2020), 448–456. https://doi.org/10.1016/j.arcontrol.2020.09.006 doi: 10.1016/j.arcontrol.2020.09.006
![]() |
[44] |
R. Ke, C. Zitzmann, D. D. Ho, R. M. Ribeiro, A. S. Perelson, In vivo kinetics of SARS-CoV-2 infection and its relationship with a person's infectiousness, PNAS, 118 (2021), e2111477118. https://doi.org/10.1073/pnas.2111477118 doi: 10.1073/pnas.2111477118
![]() |
[45] |
S. Wang, Y. Pan, Q. Wang, H. Miao, A. N. Brown, L. Rong, Modeling the viral dynamics of SARS-CoV-2 infection, Math. Biosci., 328(2020), 108438. https://doi.org/10.1016/j.mbs.2020.108438 doi: 10.1016/j.mbs.2020.108438
![]() |
[46] |
C. Li, J. Xu, J. Liu, Y. Zhou, The within-host viral kinetics of SARS-CoV-2, Math. Biosci. Engi., 17 (2020), 2853–2861. https://doi.org/10.1101/2020.02.29.965418 doi: 10.1101/2020.02.29.965418
![]() |
[47] |
M. Sadria, A. T. Layton, Modeling within-host SARS-CoV-2 infection dynamics and potential treatments, Viruses, 13 (2021), 1141. https://doi.org/10.3390/v13061141 doi: 10.3390/v13061141
![]() |
[48] |
S. Q. Du, W. Yuan, Mathematical modeling of interaction between innate and adaptive immune responses in COVID-19 and implications for viral pathogenesis, J. Med. Virol., 92 (2020), 1615–1628. https://doi.org/10.1002/jmv.25866 doi: 10.1002/jmv.25866
![]() |
[49] |
K. Hattaf, N. Yousfi, Dynamics of SARS-CoV-2 infection model with two modes of transmission and immune response, Math. Biosci. Engi., 17 (2020), 5326–5340. https://doi.org/10.3934/mbe.2020288 doi: 10.3934/mbe.2020288
![]() |
[50] | J. Mondal, P. Samui, A. N. Chatterjee, Dynamical demeanour of SARS-CoV-2 virus undergoing immune response mechanism in COVID-19 pandemic, Eur. Phys. J. Spec. Top., (2022). https://doi.org/10.1140/epjs/s11734-022-00437-5. |
[51] |
A. E. S. Almoceraa, G. Quiroz, E. A. Hernandez-Vargas, Stability analysis in COVID-19 within-host model with immune response, Commun. Nonlinear Sci. Numer. Simulat., 95 (2021), 105584. https://doi.org/10.1016/j.cnsns.2020.105584 doi: 10.1016/j.cnsns.2020.105584
![]() |
[52] |
A. Gonçalves, J. Bertrand, R. Ke, E. Comets, X. Lamballerie, D. Malvy, et al., Timing of antiviral treatment initiation is critical to reduce SARS-CoV-2 viral load, J. PSP, 9 (2020), 509–514. https://doi.org/10.1002/psp4.12543 doi: 10.1002/psp4.12543
![]() |
[53] |
P. Abuin, A. Anderson, A. Ferramosca, E. A. Hernandez-Vargas, A. H. Gonzalez, Characterization of SARS-CoV-2 dynamics in the host, Annu. Rev. Control, 50 (2020), 457–468. https://doi.org/10.1016/j.arcontrol.2020.09.008 doi: 10.1016/j.arcontrol.2020.09.008
![]() |
[54] |
A. M. Elaiw, A. J. Alsaedi, A. D. Al Agha, A. D. Hobiny, Global stability of a humoral immunity COVID-19 model with logistic growth and delays, Mathematics, 10 (2022), 1857. https://doi.org/10.3390/math10111857 doi: 10.3390/math10111857
![]() |
[55] |
B. J. Nath, K. Dehingia, V. N. Mishra, Y.-M. Chu, H. K. Sarmah, Mathematical analysis of a within-host model of SARS-CoV-2, Adv. Differ. Equ., 2021 (2021), 113. https://doi.org/10.1186/s13662-021-03276-1 doi: 10.1186/s13662-021-03276-1
![]() |
[56] |
A. M. Elaiw, A. D. Hobiny, A. D. Al Agha, Global dynamics of SARS-CoV-2/cancer model with immune responses, Applied Mathematics and Computation, 408 (2021), 126364. https://doi.org/10.1016/j.amc.2021.126364 doi: 10.1016/j.amc.2021.126364
![]() |
[57] | A. D. Al Agha, A. M. Elaiw, S. A. Azoz, E. Ramadan, Stability analysis of within-host SARS-CoV-2/HIV coinfection model, Math. Methods Appl. Sci., (2022), 1–20, https://doi.org/10.1002/mma.8457. |
[58] |
A.D. Al Agha, A.M. Elaiw, Global dynamics of SARS-CoV-2/malaria model with antibody immune response, Math. Biosci. Engi., 19 (2022), 8380–8410. http://dx.doi.org/10.3934/mbe.2022390 doi: 10.3934/mbe.2022390
![]() |
[59] |
A. Korobeinikov, Global properties of basic virus dynamics models, Bull. Math. Biol., 66 (2004), 879–883. https://doi.org/10.1016/j.bulm.2004.02.001 doi: 10.1016/j.bulm.2004.02.001
![]() |
[60] | J. K. Hale, S. V. Lunel, Introduction to functional differential equations, Springer-Verlag, New York, (1993). http://dx.doi.org/10.1007/978-1-4612-4342-7 |
[61] | E. A. Barbashin, Introduction to the theory of stability, Wolters-Noordhoff, Groningen, 1970. https://doi.org/10.1007/978-1-4612-4046-4 |
[62] | J. P. LaSalle, The Stability of Dynamical Systems, SIAM, Philadelphia, 1976. https://doi.org/10.1137/1021079 |
[63] | A. M. Lyapunov, The general problem of the stability of motion, Taylor Francis, Ltd., London, 1992. https://doi.org/10.1080/00207179208934253 |
[64] |
N. Bellomo, D. Burini, N. Outada, Multiscale models of Covid-19 with mutations and variants, Netw. Heterog. Media., 17 (2022), 293–310. https://doi.org/10.3934/nhm.2022008. doi: 10.3934/nhm.2022008
![]() |
[65] |
N. Bellomo, D. Burini, N. Outada, Pandemics of mutating virus and society: a multi-scale active particles approach, Philos. Trans. Math. Phys. Eng. Sci., 380 (2022), 1–14. https://doi.org/10.1098/rsta.2021.0161. doi: 10.1098/rsta.2021.0161
![]() |
[66] |
K. Hattaf, A new generalized definition of fractional derivative with non-singular kernel, Computation, 8 (2020), 49. https://doi.org/10.3390/computation8020049 doi: 10.3390/computation8020049
![]() |
[67] | K. Hattaf, On the stability and numerical scheme of fractional differential equations with application to biology, Computation, 10(6) (2022), 97. https://doi.org/10.3390/computation10060097 |
[68] | A. N. Chatterjee, F. Al Basir, M.A. Almuqrin, J. Mondal, I. Khan, SARS-CoV-2 infection with lytic and nonlytic immune responses: a fractional order optimal control theoretical study, Results Phys., 26, (2021), 104260. https://doi.org/10.1016/j.rinp.2021.104260 |
1. | Ahmed M. Elaiw, Raghad S. Alsulami, Aatef D. Hobiny, Dynamic Behaviors of a COVID-19 and Influenza Co-Infection Model with Time Delays and Humoral Immunity, 2023, 12, 2075-1680, 151, 10.3390/axioms12020151 | |
2. | Jocelyne Piret, Guy Boivin, The impact of trained immunity in respiratory viral infections, 2024, 34, 1052-9276, 10.1002/rmv.2510 |
Equilibrium point | \text{Existence conditions} | Global stability \text{ conditions} |
\Xi_{0}=(X_{0}, 0, 0, 0, 0, 0, 0, 0, 0) | None | \Re_{1}\leq1 and \Re_{2}\leq 1 |
\Xi_{1}=(X_{1}, L_{1}, 0, Y_{1}, 0, V_{1}, 0, 0, 0) | \Re_{1} > 1 | \Re_{1} > 1 , \Re_{2}/\Re_{1}\leq1 and \Re_{3}\leq1 |
\Xi_{2}=(X_{2}, 0, E_{2}, 0, I_{2}, 0, P_{2}, 0, 0) | \Re_{2} > 1 | \Re_{2} > 1 , \Re_{1}/\Re_{2}\leq1 and \Re_{4}\leq1 |
\Xi_{3}=(X_{3}, L_{3}, 0, Y_{3}, 0, V_{3}, 0, Z_{3}, 0) | \Re_{3} > 1 | \Re_{3} > 1 and \Re_{5}\leq1 |
\Xi_{4}=(X_{4}, 0, E_{4}, 0, I_{4}, 0, P_{4}, 0, M_{4}) | \Re_{4} > 1 | \Re_{4} > 1 and \Re_{6}\leq1 |
\Xi_{5}=(X_{5}, L_{5}, E_{5}, Y_{5}, I_{5}, V_{5}, P_{5}, Z_{5}, 0) | \Re_{5} > 1 and \Re_{1}/\Re_{2} > 1 | \Re_{5} > 1 , \Re_{8}\leq1 and \Re _{1}/\Re_{2} > 1 |
\Xi_{6}=(X_{6}, L_{6}, E_{6}, Y_{6}, I_{6}, V_{6}, P_{6}, 0, M_{6}) | \Re_{6} > 1 and \Re_{2}/\Re_{1} > 1 | \Re_{6} > 1 , \Re_{7}\leq1 and \Re_{2}/\Re_{1} > 1 |
\Xi_{7}=(X_{7}, L_{7}, E_{7}, Y_{7}, I_{7}, V_{7}, P_{7}, Z_{7}, M_{7}) | \Re _{7} > 1 and \Re_{8} > 1 | \Re_{7} > 1 and \Re_{8} > 1 |
Parameter | Value | Parameter | Value | Parameter | Value | Parameter | Value |
\lambda | 0.5 | \gamma_{I} | 0.2 | \varkappa_{V} | 0.05 | \mu _{M} | 0.04 |
\alpha | 0.05 | \kappa_{V} | 0.2 | \varkappa_{P} | 0.04 | \eta_{L} | 0.05 |
\beta_{V} | Varied | \kappa_{P} | 0.4 | \sigma_{Z} | Varied | \eta_{E} | 0.06 |
\beta_{P} | Varied | \pi_{V} | 0.2 | \sigma_{M} | Varied | \delta _{L} | 0.05 |
\gamma_{Y} | 0.11 | \pi_{P} | 0.1 | \mu_{Z} | 0.05 | \delta_{E} | 0.06 |
Situatio | The equilibria | Re(λj), j = 1, 2, …, 9 | Stability |
1 | \Xi 0 = (10, 0, 0, 0, 0, 0, 0, 0, 0) | (−0.18, −0.18, −0.18, −0.15, −0.08, −0.07, −0.05, −0.05, −0.04) | stable |
2 | \Xi 0 = (10, 0, 0, 0, 0, 0, 0, 0, 0) | (−0.22, −0.22, −0.18, −0.18, −0.07, −0.05, −0.05, −0.04, 0.04) | unstable |
\Xi 1 = (4.4, 2.8, 01.27, 0, 1.27, 0, 0, 0) | (−0.22, −0.22, −0.17, −0.17, −0.08, −0.04, −0.04, −0.05, −0.04) | stable | |
3 | \Xi 0 = (10, 0, 0, 0, 0, 0, 0, 0, 0) | (−0.24, −0.24, −0.18, −0.18, 0.06, −0.05, −0.05, −0.05, −0.04) | unstable |
\Xi 2 = (3.33, 0, 2.78, 0, 0.83, 0, 3.33, 0, 0) | (−0.24, −0.24, −0.17, −0.17, −0.07, −0.05, −0.05, −0.05, −0.04) | stable | |
4 | \Xi 0 = (10, 0, 0, 0, 0, 0, 0, 0, 0) | (−0.24, −0.24, −0.18, −0.18, 0.08, −0.05, −0.05, −0.05, −0.04) | unstable |
\Xi 1 = (2.44, 3.78, 0, 1.27, 0, 1.27, 0, 0, 0) | (−0.25, −0.25, −0.17, −0.17, −0.08, −0.06, −0.06, −0.04, 0.04) | unstable | |
\Xi 3 = (3.57, 3.21, 0, 1.46, 0, 1, 0, 1.84, 0) | (−0.27, −0.27, −0.17, −0.17, −0.07, −0.04, −0.04, −0.04, −0.03) | stable | |
5 | \Xi 0 = (10, 0, 0, 0, 0, 0, 0, 0, 0) | (−0.29, −0.29, −0.19, −0.19, 0.15, −0.05, −0.05, −0.04, −0.03) | unstable |
\Xi 2 = (1, 0, 3.75, 0, 1.13, 0, 4.5, 0, 0) | (−0.47, −0.29, −0.18, −0.15, −0.08, −0.08, −0.08, −0.05, 0.05) | unstable | |
\Xi 4 = (2, 0, 3.33, 0, 1, 0, 2, 0, 2.5) | (−0.31, −0.31, −0.17, −0.17, −0.06, −0.06, −0.07, −0.05, −0.03) | stable | |
6 | \Xi 0 = (10, 0, 0, 0, 0, 0, 0, 0, 0) | (−0.24, −0.24, −0.23, −0.23, 0.08, −0.05, −0.05, −0.04, 0.03) | unstable |
\Xi 1 = (2.44, 3.78, 0, 1.72, 0, 1.72, 0, 0, 0) | (−0.25, −0.25, −0.2, −0.2, 0.11, −0.06, −0.06, −0.04, −0.03) | unstable | |
\Xi 2 = (5, 0, 2.08, 0, 0.63, 0, 2.5, 0, 0) | (−0.22, −0.22, −0.22, −0.22, −0.05, −0.04, −0.04, 0.04, −0.02) | unstable | |
\Xi 3 = (5.14, 2.43, 0, 1.11, 0, 0.53, 0, 4.4, 0) | (−0.32, −0.32, −0.21, −0.21, −0.02, −0.02, −0.04, −0.04, 0.001) | unstable | |
\Xi 5 = (5, 2.37, 0.11, 1.08, 0.03, 0.53, 0.13, 4.18, 0) | (−0.31, −0.31, −0.21, −0.21, −0.02, −0.02, −0.04, −0.03, −0.001) | stable | |
7 | \Xi 0 = (10, 0, 0, 0, 0, 0, 0, 0, 0) | (−0.28, −0.28, −0.24, −0.24, 0.14, 0.07, −0.05, −0.05, −0.04) | unstable |
\Xi 1 = (2.44, 3.78, 0, 1.72, 0, 1.72, 0, 0, 0) | (−0.25, −0.25, −0.23, −0.23, −0.06, −0.06, −0.04, 0.04, 0.002) | unstable | |
\Xi 2 = (1.11, 0, 3.71, 0, 1.11, 0, 4.44, 0, 0) | (−0.4, −0.31, −0.19, −0.19, 0.09, −0.08, −0.08, −0.05, −0.03) | unstable | |
\Xi 3 = (2.5, 3.75, 0, 1.7, 0, 1.67, 0, 0.09, 0) | (−0.25, −0.25, −0.23, −0.23, −0.06, −0.06, 0.04, −0.04, −0.001) | unstable | |
\Xi 4 = (2.94, 0, 2.94, 0, 0.88, 0, 1.33, 0, 4.12) | (−0.32, −0.32, −0.21, −0.21, −0.04, −0.04, −0.05, −0.04, 0.008) | unstable | |
\Xi 6 = (2.44, 0.84, 2.44, 0.38, 0.73, 0.38, 1.33, 0, 3) | (−0.3, −0.3, −0.21, −0.21, −0.05, −0.05, −0.04, −0.02, −0.02) | stable | |
8 | \Xi 0 = (10, 0, 0, 0, 0, 0, 0, 0, 0) | (−0.28, −0.28, −0.24, −0.24, 0.14, 0.08, −0.05, −0.05, −0.04) | unstable |
\Xi 1 = (2.44, 3.78, 0, 1.72, 0, 1.72, 0, 0, 0) | (0.81, −0.25, −0.25, −0.23, −0.23, −0.06, −0.06, −0.04, 0.04) | unstable | |
\Xi 2 = (1.11, 0, 3.7, 0, 1.11, 0, 4.44, 0, 0) | (2.18, −0.4, −0.31, −0.19, −0.19, −0.08, −0.08, −0.05, −0.03) | unstable | |
\Xi 3 = (8.47, 0.76, 0, 0.35, 0, 0.1, 0, 9.87, 0) | (−0.63, −0.27, −0.27, −0.26, 0.13, −0.05, −0.01, −0.01, −0.04) | unstable | |
\Xi 4 = (8.74, 0, 0.52, 0, 0.16, 0, 0.08, 0, 17.17) | (−0.67, −0.41, −0.24, −0.24, 0.07, −0.01, −0.01, −0.05, −0.05) | unstable | |
\Xi 6 = (2.44, 9.27, 0.15, 1.64, 0.04, 1.64, 0.08, 0, 3) | (0.77, −0.27, −0.27, −0.25, −0.25, −0.06, −0.06, −0.006, −0.006) | unstable | |
\Xi 7 = (7.55, 0.68, 0.45, 0.31, 0.14, 0.1, 0.08, 8.36, 14.49) | (−0.54, −0.49, −0.49, −0.27, −0.02, −0.02, −0.05, −0.01, −0.01) | stable |
Equilibrium point | \text{Existence conditions} | Global stability \text{ conditions} |
\Xi_{0}=(X_{0}, 0, 0, 0, 0, 0, 0, 0, 0) | None | \Re_{1}\leq1 and \Re_{2}\leq 1 |
\Xi_{1}=(X_{1}, L_{1}, 0, Y_{1}, 0, V_{1}, 0, 0, 0) | \Re_{1} > 1 | \Re_{1} > 1 , \Re_{2}/\Re_{1}\leq1 and \Re_{3}\leq1 |
\Xi_{2}=(X_{2}, 0, E_{2}, 0, I_{2}, 0, P_{2}, 0, 0) | \Re_{2} > 1 | \Re_{2} > 1 , \Re_{1}/\Re_{2}\leq1 and \Re_{4}\leq1 |
\Xi_{3}=(X_{3}, L_{3}, 0, Y_{3}, 0, V_{3}, 0, Z_{3}, 0) | \Re_{3} > 1 | \Re_{3} > 1 and \Re_{5}\leq1 |
\Xi_{4}=(X_{4}, 0, E_{4}, 0, I_{4}, 0, P_{4}, 0, M_{4}) | \Re_{4} > 1 | \Re_{4} > 1 and \Re_{6}\leq1 |
\Xi_{5}=(X_{5}, L_{5}, E_{5}, Y_{5}, I_{5}, V_{5}, P_{5}, Z_{5}, 0) | \Re_{5} > 1 and \Re_{1}/\Re_{2} > 1 | \Re_{5} > 1 , \Re_{8}\leq1 and \Re _{1}/\Re_{2} > 1 |
\Xi_{6}=(X_{6}, L_{6}, E_{6}, Y_{6}, I_{6}, V_{6}, P_{6}, 0, M_{6}) | \Re_{6} > 1 and \Re_{2}/\Re_{1} > 1 | \Re_{6} > 1 , \Re_{7}\leq1 and \Re_{2}/\Re_{1} > 1 |
\Xi_{7}=(X_{7}, L_{7}, E_{7}, Y_{7}, I_{7}, V_{7}, P_{7}, Z_{7}, M_{7}) | \Re _{7} > 1 and \Re_{8} > 1 | \Re_{7} > 1 and \Re_{8} > 1 |
Parameter | Value | Parameter | Value | Parameter | Value | Parameter | Value |
\lambda | 0.5 | \gamma_{I} | 0.2 | \varkappa_{V} | 0.05 | \mu _{M} | 0.04 |
\alpha | 0.05 | \kappa_{V} | 0.2 | \varkappa_{P} | 0.04 | \eta_{L} | 0.05 |
\beta_{V} | Varied | \kappa_{P} | 0.4 | \sigma_{Z} | Varied | \eta_{E} | 0.06 |
\beta_{P} | Varied | \pi_{V} | 0.2 | \sigma_{M} | Varied | \delta _{L} | 0.05 |
\gamma_{Y} | 0.11 | \pi_{P} | 0.1 | \mu_{Z} | 0.05 | \delta_{E} | 0.06 |
Situatio | The equilibria | Re(λj), j = 1, 2, …, 9 | Stability |
1 | \Xi 0 = (10, 0, 0, 0, 0, 0, 0, 0, 0) | (−0.18, −0.18, −0.18, −0.15, −0.08, −0.07, −0.05, −0.05, −0.04) | stable |
2 | \Xi 0 = (10, 0, 0, 0, 0, 0, 0, 0, 0) | (−0.22, −0.22, −0.18, −0.18, −0.07, −0.05, −0.05, −0.04, 0.04) | unstable |
\Xi 1 = (4.4, 2.8, 01.27, 0, 1.27, 0, 0, 0) | (−0.22, −0.22, −0.17, −0.17, −0.08, −0.04, −0.04, −0.05, −0.04) | stable | |
3 | \Xi 0 = (10, 0, 0, 0, 0, 0, 0, 0, 0) | (−0.24, −0.24, −0.18, −0.18, 0.06, −0.05, −0.05, −0.05, −0.04) | unstable |
\Xi 2 = (3.33, 0, 2.78, 0, 0.83, 0, 3.33, 0, 0) | (−0.24, −0.24, −0.17, −0.17, −0.07, −0.05, −0.05, −0.05, −0.04) | stable | |
4 | \Xi 0 = (10, 0, 0, 0, 0, 0, 0, 0, 0) | (−0.24, −0.24, −0.18, −0.18, 0.08, −0.05, −0.05, −0.05, −0.04) | unstable |
\Xi 1 = (2.44, 3.78, 0, 1.27, 0, 1.27, 0, 0, 0) | (−0.25, −0.25, −0.17, −0.17, −0.08, −0.06, −0.06, −0.04, 0.04) | unstable | |
\Xi 3 = (3.57, 3.21, 0, 1.46, 0, 1, 0, 1.84, 0) | (−0.27, −0.27, −0.17, −0.17, −0.07, −0.04, −0.04, −0.04, −0.03) | stable | |
5 | \Xi 0 = (10, 0, 0, 0, 0, 0, 0, 0, 0) | (−0.29, −0.29, −0.19, −0.19, 0.15, −0.05, −0.05, −0.04, −0.03) | unstable |
\Xi 2 = (1, 0, 3.75, 0, 1.13, 0, 4.5, 0, 0) | (−0.47, −0.29, −0.18, −0.15, −0.08, −0.08, −0.08, −0.05, 0.05) | unstable | |
\Xi 4 = (2, 0, 3.33, 0, 1, 0, 2, 0, 2.5) | (−0.31, −0.31, −0.17, −0.17, −0.06, −0.06, −0.07, −0.05, −0.03) | stable | |
6 | \Xi 0 = (10, 0, 0, 0, 0, 0, 0, 0, 0) | (−0.24, −0.24, −0.23, −0.23, 0.08, −0.05, −0.05, −0.04, 0.03) | unstable |
\Xi 1 = (2.44, 3.78, 0, 1.72, 0, 1.72, 0, 0, 0) | (−0.25, −0.25, −0.2, −0.2, 0.11, −0.06, −0.06, −0.04, −0.03) | unstable | |
\Xi 2 = (5, 0, 2.08, 0, 0.63, 0, 2.5, 0, 0) | (−0.22, −0.22, −0.22, −0.22, −0.05, −0.04, −0.04, 0.04, −0.02) | unstable | |
\Xi 3 = (5.14, 2.43, 0, 1.11, 0, 0.53, 0, 4.4, 0) | (−0.32, −0.32, −0.21, −0.21, −0.02, −0.02, −0.04, −0.04, 0.001) | unstable | |
\Xi 5 = (5, 2.37, 0.11, 1.08, 0.03, 0.53, 0.13, 4.18, 0) | (−0.31, −0.31, −0.21, −0.21, −0.02, −0.02, −0.04, −0.03, −0.001) | stable | |
7 | \Xi 0 = (10, 0, 0, 0, 0, 0, 0, 0, 0) | (−0.28, −0.28, −0.24, −0.24, 0.14, 0.07, −0.05, −0.05, −0.04) | unstable |
\Xi 1 = (2.44, 3.78, 0, 1.72, 0, 1.72, 0, 0, 0) | (−0.25, −0.25, −0.23, −0.23, −0.06, −0.06, −0.04, 0.04, 0.002) | unstable | |
\Xi 2 = (1.11, 0, 3.71, 0, 1.11, 0, 4.44, 0, 0) | (−0.4, −0.31, −0.19, −0.19, 0.09, −0.08, −0.08, −0.05, −0.03) | unstable | |
\Xi 3 = (2.5, 3.75, 0, 1.7, 0, 1.67, 0, 0.09, 0) | (−0.25, −0.25, −0.23, −0.23, −0.06, −0.06, 0.04, −0.04, −0.001) | unstable | |
\Xi 4 = (2.94, 0, 2.94, 0, 0.88, 0, 1.33, 0, 4.12) | (−0.32, −0.32, −0.21, −0.21, −0.04, −0.04, −0.05, −0.04, 0.008) | unstable | |
\Xi 6 = (2.44, 0.84, 2.44, 0.38, 0.73, 0.38, 1.33, 0, 3) | (−0.3, −0.3, −0.21, −0.21, −0.05, −0.05, −0.04, −0.02, −0.02) | stable | |
8 | \Xi 0 = (10, 0, 0, 0, 0, 0, 0, 0, 0) | (−0.28, −0.28, −0.24, −0.24, 0.14, 0.08, −0.05, −0.05, −0.04) | unstable |
\Xi 1 = (2.44, 3.78, 0, 1.72, 0, 1.72, 0, 0, 0) | (0.81, −0.25, −0.25, −0.23, −0.23, −0.06, −0.06, −0.04, 0.04) | unstable | |
\Xi 2 = (1.11, 0, 3.7, 0, 1.11, 0, 4.44, 0, 0) | (2.18, −0.4, −0.31, −0.19, −0.19, −0.08, −0.08, −0.05, −0.03) | unstable | |
\Xi 3 = (8.47, 0.76, 0, 0.35, 0, 0.1, 0, 9.87, 0) | (−0.63, −0.27, −0.27, −0.26, 0.13, −0.05, −0.01, −0.01, −0.04) | unstable | |
\Xi 4 = (8.74, 0, 0.52, 0, 0.16, 0, 0.08, 0, 17.17) | (−0.67, −0.41, −0.24, −0.24, 0.07, −0.01, −0.01, −0.05, −0.05) | unstable | |
\Xi 6 = (2.44, 9.27, 0.15, 1.64, 0.04, 1.64, 0.08, 0, 3) | (0.77, −0.27, −0.27, −0.25, −0.25, −0.06, −0.06, −0.006, −0.006) | unstable | |
\Xi 7 = (7.55, 0.68, 0.45, 0.31, 0.14, 0.1, 0.08, 8.36, 14.49) | (−0.54, −0.49, −0.49, −0.27, −0.02, −0.02, −0.05, −0.01, −0.01) | stable |