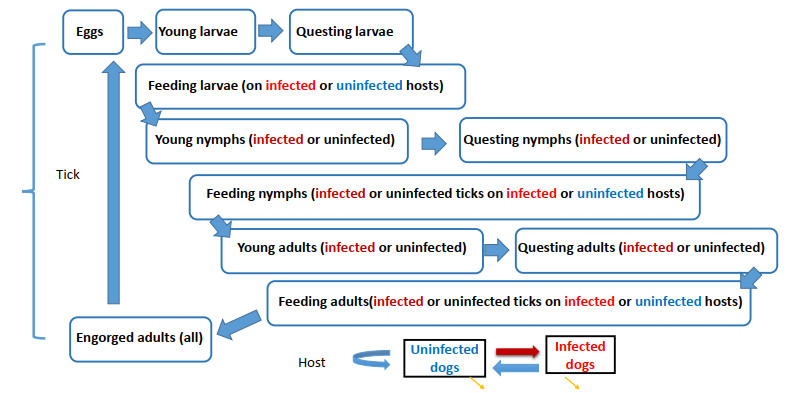
Human immunodeficiency virus (HIV) infection is a major public health concern with 1.2 million people living with HIV in the United States. The role of nutrition in general, and albumin/globulin in particular in HIV progression has long been recognized. However, no mathematical models exist to describe the interplay between HIV and albumin/globulin. In this paper, we present a family of models of HIV and the two protein components albumin and globulin. We use albumin, globulin, viral load and target cell data from simian immunodeficiency virus (SIV)-infected monkeys to perform model selection on the family of models. We discover that the simplest model accurately and uniquely describes the data. The selection of the simplest model leads to the observation that albumin and globulin do not impact the infection rate of target cells by the virus and the clearance of the infected target cells by the immune system. Moreover, the recruitment of target cells and immune cells are modeled independently of globulin in the selected model. Mathematical analysis of the selected model reveals that the model has an infection-free equilibrium and a unique infected equilibrium when the immunological reproduction number is above one. The infection-free equilibrium is locally stable when the immunological reproduction number is below one, and unstable when the immunological reproduction number is greater than one. The infection equilibrium is locally stable whenever it exists. To determine the parameters of the best fitted model we perform structural and practical identifiability analysis. The structural identifiability analysis reveals that the model is identifiable when the immune cell infection rate is fixed at a value obtained from the literature. Practical identifiability reveals that only seven of the sixteen parameters are practically identifiable with the given data. Practical identifiability of parameters performed with synthetic data sampled a lot more frequently reveals that only two parameters are practically unidentifiable. We conclude that experiments that will improve the quality of the data can help improve the parameter estimates and lead to better understanding of the interplay of HIV and albumin-globulin metabolism.
Citation: Vivek Sreejithkumar, Kia Ghods, Tharusha Bandara, Maia Martcheva, Necibe Tuncer. Modeling the interplay between albumin-globulin metabolism and HIV infection[J]. Mathematical Biosciences and Engineering, 2023, 20(11): 19527-19552. doi: 10.3934/mbe.2023865
[1] | Yijun Lou, Li Liu, Daozhou Gao . Modeling co-infection of Ixodes tick-borne pathogens. Mathematical Biosciences and Engineering, 2017, 14(5&6): 1301-1316. doi: 10.3934/mbe.2017067 |
[2] | Holly Gaff . Preliminary analysis of an agent-based model for a tick-borne disease. Mathematical Biosciences and Engineering, 2011, 8(2): 463-473. doi: 10.3934/mbe.2011.8.463 |
[3] | Luis Almeida, Michel Duprez, Yannick Privat, Nicolas Vauchelet . Mosquito population control strategies for fighting against arboviruses. Mathematical Biosciences and Engineering, 2019, 16(6): 6274-6297. doi: 10.3934/mbe.2019313 |
[4] | Mlyashimbi Helikumi, Moatlhodi Kgosimore, Dmitry Kuznetsov, Steady Mushayabasa . Dynamical and optimal control analysis of a seasonal Trypanosoma brucei rhodesiense model. Mathematical Biosciences and Engineering, 2020, 17(3): 2530-2556. doi: 10.3934/mbe.2020139 |
[5] | Meng Zhang, Xiaojing Wang, Jingan Cui . Sliding mode of compulsory treatment in infectious disease controlling. Mathematical Biosciences and Engineering, 2019, 16(4): 2549-2561. doi: 10.3934/mbe.2019128 |
[6] | Wandi Ding . Optimal control on hybrid ODE Systems with application to a tick disease model. Mathematical Biosciences and Engineering, 2007, 4(4): 633-659. doi: 10.3934/mbe.2007.4.633 |
[7] | Yunfeng Liu, Guowei Sun, Lin Wang, Zhiming Guo . Establishing Wolbachia in the wild mosquito population: The effects of wind and critical patch size. Mathematical Biosciences and Engineering, 2019, 16(5): 4399-4414. doi: 10.3934/mbe.2019219 |
[8] | Marco Tosato, Xue Zhang, Jianhong Wu . A patchy model for tick population dynamics with patch-specific developmental delays. Mathematical Biosciences and Engineering, 2022, 19(5): 5329-5360. doi: 10.3934/mbe.2022250 |
[9] | Rinaldo M. Colombo, Mauro Garavello . Optimizing vaccination strategies in an age structured SIR model. Mathematical Biosciences and Engineering, 2020, 17(2): 1074-1089. doi: 10.3934/mbe.2020057 |
[10] | Bevina D. Handari, Dipo Aldila, Bunga O. Dewi, Hanna Rosuliyana, Sarbaz H. A. Khosnaw . Analysis of yellow fever prevention strategy from the perspective of mathematical model and cost-effectiveness analysis. Mathematical Biosciences and Engineering, 2022, 19(2): 1786-1824. doi: 10.3934/mbe.2022084 |
Human immunodeficiency virus (HIV) infection is a major public health concern with 1.2 million people living with HIV in the United States. The role of nutrition in general, and albumin/globulin in particular in HIV progression has long been recognized. However, no mathematical models exist to describe the interplay between HIV and albumin/globulin. In this paper, we present a family of models of HIV and the two protein components albumin and globulin. We use albumin, globulin, viral load and target cell data from simian immunodeficiency virus (SIV)-infected monkeys to perform model selection on the family of models. We discover that the simplest model accurately and uniquely describes the data. The selection of the simplest model leads to the observation that albumin and globulin do not impact the infection rate of target cells by the virus and the clearance of the infected target cells by the immune system. Moreover, the recruitment of target cells and immune cells are modeled independently of globulin in the selected model. Mathematical analysis of the selected model reveals that the model has an infection-free equilibrium and a unique infected equilibrium when the immunological reproduction number is above one. The infection-free equilibrium is locally stable when the immunological reproduction number is below one, and unstable when the immunological reproduction number is greater than one. The infection equilibrium is locally stable whenever it exists. To determine the parameters of the best fitted model we perform structural and practical identifiability analysis. The structural identifiability analysis reveals that the model is identifiable when the immune cell infection rate is fixed at a value obtained from the literature. Practical identifiability reveals that only seven of the sixteen parameters are practically identifiable with the given data. Practical identifiability of parameters performed with synthetic data sampled a lot more frequently reveals that only two parameters are practically unidentifiable. We conclude that experiments that will improve the quality of the data can help improve the parameter estimates and lead to better understanding of the interplay of HIV and albumin-globulin metabolism.
Several species of ticks specialize as parasites of domestic dogs, including both temperate and tropical Rhipicephalus species [1]. In the U.S., Rhipicephalus sanguineus carries multiple pathogens of veterinary importance as well as Rickettsia rickettsii, the species that causes Rocky Mountain Spotted fever (RMSF) in humans [2,3,4,5,6].
Rocky Mountain Spotted fever is caused by one of several Rickettsia species which, together, comprise multiple pathogens, have worldwide distribution, and are carried by several vector species [7,8,9]. The resulting diseases range from mild to, in the case of RMSF, fatal [10,11].
Rhipicephalus sanguineus maturation times and death rates are dependent on temperature and humidity [12,13]. Thus it is not surprising that climate has been offered as an explanation of the expansion of ticks and tick-borne disease northwards [14,15]. Both temperate and tropical lineages of Rh. sanguineus have been identified in the U.S. [16,17,18,19]. Additionally, the incidence of RMSF has increased in the the U.S. [20,21,22,23,24].
In general, vector borne pathogens cause a large burden of disease and mortality worldwide, and are principally controlled by vector suppression, either alone or as part of a holistic approach that may include wall treatments, dog collars or other interventions [25,26,27]. As vector control carries a cost as well as benefits, it is possible to approach the questions of how much and when to apply interventions via the use of optimal control methods [28,29]. This approach requires a system of differential equations describing the life cycle of Rh. sanguineus and disease transmission of Ri. rickettsii. Such a model was developed for populations of ticks and dogs in a community in Sonora, Mexico, and is used as the basis for the control problem solved for that model [30,31]. The population/transmission model takes into account the multiple stages in tick development, including temperature and humidity dependence [12,32]. It incorporates insecticidal wall treatment and the resulting death rate for questing ticks.
In the Sonora intervention, a small number of houses were treated with insecticidal wall treatments [31]. The model created for this intervention indicated that it was likely that sufficient wall coverage would remove the need for the primary intervention in that situation, which was treated dog collars [30]. Wall treatments in general have a short half-life, requiring re-application. Without re-application it is possible to compare limitations of treatment using models, as was done in the case of malaria. In this study we omit decay of the treatment and instead use an optimal control approach to ask how long and at what level of effectiveness the treatment must remain in place to suppress the vector population.
The numerical approach includes a system of ordinary differential equations describing the life cycle of Rh. sanguineus, dog population growth and transmission of Ri. rickettsii between these, shown in equations (2.1-refhumidity). The control problem is framed by a system of ordinary differential equations for the adjoint variables, in equations (2.44-2.69). Results of numerical simulations are shown in Figures 2-4.
The process based model for Rh. sanguineus life cycle and disease transmission, on which optimal control is based, is taken from Álvarez-Hernandez et al, parameterized to reflect local conditions in a town in Sonora, Mexico [30]. The default death rate due to treatment is set at 0.7 to reflect 70% coverage of walls. That model includes temperature dependent maturation rates for non-questing stages as well as a temperature and humidity dependent death rate for questing nymphs. A condensed version of the compartment model is illustrated in Figure 1. Like other hard-bodied ticks, Rh. sanguineus passes through maturation stages (larva, nymph, adult). These stages conclude with relatively short questing (searching for a host) and feeding intervals that provide all the needed food and water for the tick to complete its lifecycle, after which it ovipositions (if female) and dies [32]. During the relatively long intervals between feeding on a host and the next questing event, these ticks sequester in cracks in walls, floors, and other peri-domestic areas, making them difficult to observe. Parameters are therefore adjusted to match data for questing and feeding ticks.
During the feeding stages disease transmission can take place from an infected tick to an uninfected dog, or from an infected dog to an uninfected tick. The disease transmission model is based on the Ross-Macdonald model for mosquito borne disease, with the additional feature that transmission may occur during any of the three feeding stages of the vector. There is evidence that vertical transmission may also occur, as it does in closely related diseases [33,34,35]. Little, however, is known about the rates involved or whether it is indeed a characteristic of this particular vector/disease pair, so this aspect of transmission is omitted from the model.
Eggs, E
dEdt=bA5−me(metemp)E−deE, | (2.1) |
Young, hardening larvae, L1
dL1dt=me(metemp)E−dULL1−m1L1, | (2.2) |
Questing larvae, L2
dL2dt=m1L1−dULL2−m2L2−wdWTL2, | (2.3) |
Larvae feeding on uninfected host, LU
dLUdt=m2L2FdQd−d3LU−m3LU; | (2.4) |
Larvae feeding on infected host, LI
dLIdt=m2L2FfQf−d3dLI−m3fdLI, | (2.5) |
Uninfected engorged maturing larvae/young nymphs, NU1
dNU1dt=m3dLU+(1−pL)(m3fLI)−dLNU1−mL(m3temp)NU1, | (2.6) |
Infected engorged maturing larvae/young nymphs, NI1
dNI1dt=pL(m3fLI)−dLNI1−mL(m3temp)NI1, | (2.7) |
Questing uninfected nymphs, NU2
dNU2dt=mL(m3temp)NU1−dUNNU2−mn2NU2−wdWTNU2, | (2.8) |
Questing infected nymphs, NI2
dNI2dt=mL(m3temp)NI1−dUNNI2−mn2NI2−wdWTNI2, | (2.9) |
Uninfected nymphs feeding on uninfected hosts, FNUU
dFNUUdt=mn2NU2GdQd−dfnFNUU−mfnFNUU, | (2.10) |
Uninfected nymphs feeding on infected hosts, FNUI
dFNUIdt=mn2NU2GfQf−dfnFNUI−mfnFNUI, | (2.11) |
Infected nymphs feeding on uninfected hosts, FNIU
dFNIUdt=mn2NI2GdQd−dfnFNIU−mfnFNIU, | (2.12) |
Infected nymphs feeding on Infected hosts, FNII
dFNIIdt=mn2NI2GfQf−dfnFNII−mfnFNII, | (2.13) |
Uninfected engorged maturing nymphs/young adults, AU1
dAU1dt=mfn(FNUU)+mfn(1−pN)∗(FNUI)−fNDAU1−(mfntemp)AU1, | (2.14) |
Infected engorged maturing nymphs/young adults, AI1
dAI1dt=mfn(FNII)+mfnFNII+mfn(pN)(FNUI)−fNDAI1−(mfntemp)AI1, | (2.15) |
Questing uninfected adult, AU2
dAU2dt=(mfntemp)AU1−dUAAU2−mA2AU2−wdWTAU2, | (2.16) |
Questing infected adult, AI2
dAI2dt=(mfntemp)AI1−dUAAI2−mA2AI2−wdWTAI2, | (2.17) |
Uninfected adults feeding on uninfected hosts, FAUU
dFAUUdt=mA2AU2Hd∗Qd−dA3FAUU−mA3FAU, | (2.18) |
Uninfected adults feeding on infected hosts, FAUI
dFAUIdt=mA2AU2HfQf−dA3FAUI−mA3FAUI, | (2.19) |
Infected adults feeding on uninfected hosts, FAIU
dFAIUdt=mA2AI2HdQd−dA3FAIU−mA3FAIU, | (2.20) |
Infected adults feeding on infected hosts, FAII
dFAIIdt=mA2AI2HfQf−dA3FAII−mA3FAII, | (2.21) |
Engorged adults, A4
dA4dt=mA3∗(FAUU+FAUI+FAIU+FAII)−(mfntemp)A4, | (2.22) |
Gestating adults, A5
dA5dt=(mfntemp)A4−dA5∗A5, | (2.23) |
Uninfected hosts (dogs), U
dUdt=bH(U+I)(1−(U+I)/KH)−dHU−JH, | (2.24) |
Infected hosts (dogs), I
dIdt=JH−dHII, | (2.25) |
All nymphs and adults feeding on uninfected hosts, TU
TU=FNUU+FNIU+FAUU+FAIU, | (2.26) |
All nymphs and adults feeding on infected hosts
TI=FNUI+FNII+FAUI+FAII | (2.27) |
Percent available space per uninfected host weighted by probability (qL) of larvae finding any host, Fd
Fd=max(qL(CU−TU)/(CU+ϵ),0), | (2.28) |
Percent available space per infected host weighted by probability (qL) of larvae finding any host, Ff
Ff=max(qL(CI−TI)/(CI+ϵ),0), | (2.29) |
Percent available space per uninfected host weighted by probability (qN) of nymph finding any host, Gd
Gd=max(qN(CU−TU)/(CU+ϵ),0), | (2.30) |
Percent available space per infected host weighted by probability (qN) of nymph finding any host, Gf
Gf=max(qN(CI−TI)/(CI+ϵ),0), | (2.31) |
Percent available space per uninfected host weighted by probability (qA) of adult finding any host, Hd
Hd=max(qA(CU−TU)/(CU+ϵ),0), | (2.32) |
Percent available space per infected host weighted by probability (qA) of adult finding any host, Hf
Hf=max(qA(CI−TI)/(CI+ϵ),0), | (2.33) |
Total number of hosts of all types, S
S=U+I, | (2.34) |
Fraction of hosts that are uninfected, Qd
Qd=U/(S+P3d), | (2.35) |
Fraction of hosts that are infected, Qf
Qf=I/(S+P3f), | (2.36) |
Transmission term for host infection, J
J=pUI(FNIU+FAIU)U, | (2.37) |
Temperature approximation for study area, T
T=24.84+−8.501∗cos(t∗0.01721)+−1.668∗sin(t∗0.01721)+ | (2.38) |
−0.08626∗cos(2∗t∗0.01721)+1.192∗sin(2∗t∗0.01677), | (2.39) |
Percent humidity approximation for study area, H
H=62.93+9.866∗cos(t∗0.01721)+−10.86∗sin(t∗0.01721)+ | (2.40) |
3.166∗cos(2∗t∗0.01721)+0.6116∗sin(2∗t∗0.01721), | (2.41) |
In this section we will obtain the existence, uniqueness, nonnegativity, and boundedness of solutions to our model in a single theorem.
Theorem 2.1. For nonnegative initial conditions, the model (2.1-2.25) has a unique solution which exists for all time and is nonnegative in each component.
Proof: Local existence and uniqueness is standard via arguments in [36]. A supersolution argument establishes that the solutions are bounded on their interval of existence [37]. A subsolution argument proves that the solutions are bounded below by zero.
We wish to minimize the tick population during the questing life stages, L2,NU2,NI2,AU2 and AI2, while also minimizing the death rate caused by the wall treatment intervention, represented by the coefficient w in equations (2.3, 2.8, 2.9, 2.16, 2.17).
J(w)=minw(t)∫T0(L22(t)+NU22(t)+NI22(t)+AU22(t)+AI22(t)+Kw2(t))dt | (2.42) |
over the set of admissible controls
V={w measurable |0≤w(t)≤1,∀t∈[0,T]}. | (2.43) |
We use quadratic terms in the cost function, J(w), as is typical for epidemiology control problems, because linear control does not offer closed-form solutions for the optimal control [38,39,40,41]. Often a linear-quadratic cost function is used as well. These cost functions represent the nonlinear increase in the effect of each quantity in J(w). The cost of increased infective ticks is probably closer to quadratic than linear because at low levels ticks would prefer the dog host, while at high levels they might prefer humans as space on dogs becomes saturated. Similarly, the effects of the wall treatment probably represent a nonlinear function to the system because after the more willing participants have treated their walls it becomes increasingly expensive to convince the holdouts.
The quadratic term is multiplied by a coefficient, K, which allows for the relative importance of the term to be varied. The final time T determines the size of the interval of existence for the optimal control.
Theorem 2.2. Given the objective functional (2.42), subject to the system given by Eqs. (2.1-2.25) with nonnegative initial conditions, and the admissible control set (2.43) then there exists an optimal control w∗(t) such that
minw∈VJ(w)=J(w∗). |
Proof: In order to apply the theory of Fleming and Rishel, [42], we must show that the following conditions are met:
1. The class of all initial conditions with a control fnction w(t) in the admissible control set along with each state equation being satisfied is not empty.
2. The admissible control set V is closed and convex.
3. Each right hand side of the state system is continuous, is bounded above by a sum of the bounded control and the state, and can be written as a linear function of the control function w(t) with coefficients depending on time and the state.
4. The integrand of the objective functional (2.42) is convex on V and is bounded below.
Since all conditions are satisfied in this case, it follows that there exists an optimal control w∗(t) such that
minw∈VJ(w)=J(w∗). |
Theorem 2.3. Given the optimal controls w∗ and solutions of the corresponding state system, there exist adjoint variables λ1,λ2,…,λ25 satisfying the following:
dλ1dt=λ1(me(metemp)+de)−λ2me(metemp) | (2.44) |
dλ2dt=λ2(dw+m1)−λ3m1 | (2.45) |
dλ3dt=−1+λ3(dw+m2+wdWT)−λ4m2FdQd−λ5m2FfQf | (2.46) |
dλ4dt=λ4(d3+m3d)−λ6m3d | (2.47) |
dλ5dt=λ5(d3+m3f)−λ6(1−pL)m3f+λ7pLm3f | (2.48) |
dλ6dt=λ6(dL+mL(m3temp))−λ8mL(m3temp) | (2.49) |
dλ7dt=λ7(dL+mL(m3temp))−λ9mL(m3temp) | (2.50) |
dλ8dt=−1+λ8(dUN+mn2+wdWT)−λ10mn2GdQd−λ11mn2GfQf | (2.51) |
dλ9dt=−1+λ9(dUN+mn2+wdWT)−λ12mn2GdQd−λ13mn2GfQf | (2.52) |
dλ10dt=λ10(dfn+mfn)−λ14mfn−λ4m2L2∂Fd∂FNUUQd−λ10mn2NU2∂Gd∂FNUUQd−λ12mn2NI2∂Gd∂FNUUQd−λ18mA2AU2∂Hd∂FNUUQd−λ20mA2AI2∂Hd∂FNUUQd | (2.53) |
dλ11dt=λ11(dfn+mfn)−λ14mfn(1−pN)−λ15mfnpN−λ5m2L2∂Ff∂FNUIQf−λ11mn2NU2∂Gf∂FNUIQf−λ13mn2NI2∂Gf∂FNUIQf−λ19mA2AU2∂Hf∂FNUIQf−λ21mA2AI2∂Hf∂FNUIQf | (2.54) |
dλ12dt=λ12(dfn+mfn)−λ25pUIU−λ4m2L2∂Fd∂FNIUQd−λ10mn2NU2∂Gd∂FNIUQd−λ12mn2NI2∂Gd∂FNIUQd−λ18mA2AU2∂Hd∂FNIUQd−λ20mA2AI2∂Hd∂FNIUQd | (2.55) |
dλ13dt=λ13(dfn+mfn)−λ15mfn−λ5m2L2∂Ff∂FNIIQf−λ11mn2NU2∂Gf∂FNIIQf−λ13mn2NI2∂Gf∂FNIIQf−λ19mA2AU2∂Hf∂FNIIQf−λ21mA2AI2∂Hf∂FNIIQf | (2.56) |
λ14dt=λ14(fND+(mfntemp)+dWT)+λ16(mfntemp) | (2.57) |
λ15dt=λ15(fND+(mfntemp))+λ17(mfntemp) | (2.58) |
λ16dt=−1+λ16(dUA+mA2+wdWT)−λ18mA2HdQd−λ19mA2HfQf | (2.59) |
λ17dt=−1+λ17(dUA+mA2+wdWT)−λ20mA2HdQd−λ21mA2HfQf | (2.60) |
dλ18dt=λ18(dA3+mA3)−λ22mA3−λ4m2L2∂Fd∂FAUUQd−λ10mn2NU2∂Gd∂FAUUQd−λ12mn2NI2∂Gd∂FAUUQd−λ18mA2AU2∂Hd∂FAUUQd−λ20mA2AI2∂Hd∂FAUUQd | (2.61) |
dλ19dt=λ19(dA3+mA3)−λ22mA3−λ5m2L2∂Ff∂FAUIQf−λ11mn2NU2∂Gf∂FAUIQf−λ13mn2NI2∂Gf∂FAUUIQf−λ19mA2AU2∂Hf∂FAUIQf−λ21mA2AI2∂Hf∂FAUIQf | (2.62) |
dλ20dt=λ20(dA3+mA3)−λ22mA3−λ25pUIU−λ4m2L2∂Fd∂FAIUQd−λ10mn2NU2∂Gd∂FAIUQd−λ12mn2NI2∂Gd∂FAIUQd−λ18mA2AU2∂Hd∂FAIUQd−λ20mA2AI2∂Hd∂FAIUQd | (2.63) |
dλ21dt=λ21(dA3+mA3)−λ22mA3−λ5m2L2∂Ff∂FAIIQf−λ11mn2NU2∂Gf∂FAIIQf−λ13mn2NI2∂Gf∂FAIUIQf−λ19mA2AU2∂Hf∂FAIIQf−λ21mA2AI2∂Hf∂FAIIQf | (2.64) |
dλ22dt=λ22(mfntemp)−λ23(mfntemp) | (2.65) |
dλ23dt=−λ1b+λ23(dA5) | (2.66) |
dλ24dt=−λ4m2L2(∂Fd∂UQd+Fd∂Qd∂U)−λ5m2L2Ff∂Qf∂U−λ10mn2NU2(∂Gd∂UQd+Gd∂Qd∂U)−λ11mn2NU2Gf∂Qf∂U−λ12mn2NI2(∂Gd∂UQd+Gd∂Qd∂U)−λ13mn2NI2Gf∂Qf∂U−λ18mA2AU2(∂Hd∂UQd+Hd∂Qd∂U)−λ19mA2AU2Hf∂Qf∂U−λ20mA2AI2(∂Hd∂UQd+Hd∂Qd∂U)−λ21mA2AI2Hf∂Qf∂U−λ24(bH(1−2(U+I)KH)−dH)−λ25pUI(FNIU+FAIU) | (2.67) |
dλ25dt=−λ4m2L2Fd∂Qd∂I−λ5m2L2(∂Ff∂IQf+Ff∂Qf∂I)−λ10mn2NU2Gd∂Qd∂I−λ11mn2NU2(∂Gf∂IQf+Gf∂Qf∂I)−λ12mn2NI2Gd∂Qd∂I−λ13mn2NI2(∂Gf∂IQf+Gf∂Qf∂I)−λ18mA2AU2Hd∂Qd∂I−λ19mA2AU2(∂Hf∂IQf+Hf∂Qf∂I)−λ20mA2AI2Hd∂Qd∂I−λ21mA2AI2(∂Hf∂IQf+Hf∂Qf∂I)−λ24bH(1−2(U+I)KH)−λ25dHI | (2.68) |
where λ1(T)=λ2(T)=⋯=λ25(T)=0. Furthermore, the analytic representation of the optimal control w∗ is given by
w∗(t)=min(max(0,(λ3L2+λ8NU2+λ9NI2+λ16AU2+λ17AI2)dWT2K),1) | (2.69) |
Note that
∂Fd∂U={qL(C(TU+ϵ)(CU+ϵ)2)if CU−TU>00otherwise |
and
∂Fd∂FNUU=∂Fd∂FNIU=∂Fd∂FAUU=∂Fd∂FAIU={−qLCU+ϵif CU−TU>00otherwise |
and
∂Ff∂I={qL(C(TI+ϵ)(CI+ϵ)2)if CI−TI>00otherwise |
and
∂Ff∂FNUI=∂Ff∂FNII=∂Ff∂FAUI=∂Fd∂FAII={−qLCI+ϵif CI−TI>00otherwise |
with similar expressions for Gd,Gf,Hd,Hf.
Proof: Suppose w∗(t) is the optimal control and that E,Ł1,…,U,I is the corresponding solution to the system (2.1-2.25). We use standard work in Pontryagin et al. [32] to obtain the result. To find the analytic representation of the optimal control w∗(t), begin by forming the Lagrangian. Since the control is bounded, the Lagrangian is
L=H−W1(t)(w(t)−0)−W2(t)(1−w(t)) |
where H is the Hamiltonian given by
H=L2+NU2+NI2+AU2+AI2+Kw2+25∑i=1λi(rhsi) |
and Wi(t)≥0 are penalty multipliers such that
W1(t)(w(t)−0)=0W2(t)(1−w(t))=0}at w∗(t) |
To find the analytic representation for w∗(t), we analyze the necessary conditions for optimality ∂L∂w=0.
∂L∂w=∂H∂w−W1+W2=0 |
or
2Kw+λ3(−dWTL2)+λ8(−dWTNU2)+λ9(−dWTNI2)+λ16(dWTAU2+λ17(−dWTAI2)−W1+W2=0. |
By standard optimality techniques for the characterization for the optimal control w∗(t), we find that
w∗(t)=min(max(0,(λ3L2+λ8NU2+λ9NI2+λ16AU2+λ17AI2)dWT2K),1) |
At the optimum w∗, the model differential equations move forward in time from an initial condition, while the adjoint differential equations move backward in time from a final condition. In some cases, it is possible to use Matlab's bvp4c to solve ODE systems with a variety of different types of boundary conditions like this one [43]. However, there are often convergence problems with this approach. For this paper, we followed the algorithm developed by Hackbusch [44] and recommended by Lenhart and Workman [45] to solve our optimality system.
Numerical Scheme:
1. Initialize the adjoint variables, λ01=λ02=⋯=λ025=0, and the control w0=0.5.
2. Use the current adjoint variables λj−11,λj−12,…λj−125 and control wj−1 to solve the state equations for the state variables Ej,Lj1,…,Ij.
3. Use the current state variables Ej,Lj1,…,Ij.. to solve the adjoint equations for the adjoint variables λj1,λj2,…λj25.
4. Update the control wj using the control characterizations.
5. Repeat steps 2–4 until convergence.
The algorithm was implemented in Matlab [43], using ode45 to solve the ODEs and interp1 to pass the solutions from step to step.
Recall that the objective function is
J(w)=minw(t)∫T0(L22(t)+NU22(t)+NI22(t)+AU22(t)+AI22(t)+Kw2(t))dt |
where we are simultaneously minimizing the tick populations at the questing stages and the cost of the wall-treatment with the term Kw2.
Initial conditions were found by running the original model with an initial number of tick eggs of E0=1,000,000, an initial number of uninfected dogs of U=1248 and one infected dog I=1. This simulation was run until T=1000 days and these steady state population values were used as initial conditions for all simulations in this paper.
The model with no treatment results in a seasonally fluctuating steady state, with ticks always present and abundant, seen in Figure 2a. Ri. rickettsii prevalence in ticks and dogs reaches steady state will little seasonal fluctuation, seen in Figure 2b. In the absence of control, we note that the percentage of infected dogs and ticks remains essentially stable, indicating persistence of the disease in the absence of interventions, seen Figure 2b. With the relatively expensive control at K = 100, tick abundance and disease prevalence decline steadily, as in Figure 2c and Figure 2d, while the optimal control is allowed to decline starting at approximately t = 100, seen in Figure 2d.
Using the same initial conditions as Example 1, we consider simulations with dWT=0.7, or 70% of houses treated. Under the assumption that the treatment is more expensive, we set the penalty affecting the cost of treatment in the objective function J to be K=1,10, and K=100 and run the optimal control algorithm for two years, T=730 days seen in Figure 3a, 3c and 3e. Control was discontinued at T=730, and the subsequent two years tracked in Figure 3b, 3d and 3f for each of the controls respectively. We were unable to obtain convergence of the algorithm for K=1000.
The risk of human infection with RMSF depends on the likelihood of contact with an infected tick, which in turn depends on the abundance of infected ticks, not just pathogen prevalence in the tick population. The risk of an individual exposure to RMSF depends on the population of infected questing nymphs and adults. Three scenarios are computed, with the optimal control calculated for days 1-730, followed by the rebound of tick populations for the following 730 days. Results are shown in Figure 3 for K = 1, 10, and 100.
As the cost of treatment increases from K = 1 to K = 100, the optimal control goes from always on at full strength with K = 1 as seen in Figure 3a, to always on for an initial period, then declining to a lower level, as seen in Figure 3c and 3e. In all three cases, Ri. rickettsii prevalence is greatly reduced at the 2-year point, seen in Figure 3a, 3c and 3e. When treatment is removed completely the disease prevalence increases, seen in Figure 3b, 3d and 3f. The treatment is 100% effective for much of the time interval, although it's efficacy drops later in the time interval. We note that for K=1, the treatment is 100% effective for the whole time interval. In the presence of control, we note that the percentage of infected dogs and ticks decreases dramatically over time, supporting the theory that the wall treatment is an effective approach to reducing the the number of RMSF infections in both ticks and dogs.
The death rate of questing ticks due to the wall treatment, dWT, can be varied to reflect maximum coverage of houses in the community. Setting K=1 and varying dWT gives an example of the effect of coverage levels in Figure 4. The death rate of questing ticks at full strength of treatment may vary due to coverage levels or efficacy of the product chosen. The default death rate of 70% of questing ticks per day (at full control (w = 1), Figure 4c and 4d) was increased to 100% per day (Figure 4a and 4b) and decreased to 35% (Figure 4e and 4f). Although the control patterns look similar (Figure 4b, 4d, 4f) the resulting decline in pathogen prevalence is more pronounced as the death rate rises (Figure 4a, 4c and 4e).
Acaricidal wall treatments have the potential to drastically reduce tick populations as seen in Figure 2. It is clear from both Figures 3 and 4 that an optimal wall treatment must remain at full strength for a considerable period, followed by declining efficacy. Even the shortest duration of high intensity, shown in Figure 3e, is a year in duration.
Figure 3 shows the suppression of disease risk for three choices of optimal control (K = 1, 10,100 respectively). As K increases, the duration of 100% treatment decreases with subsequent decline in treatment strength for K = 10,100, seen in Figure 3c and 3e. This decline is a normal feature of insecticidal treatments [46,47,48]. For K = 1 treatment is at full strength until day 730 and then is abruptly discontinued. Recall that we assume 70% coverage of surfaces for all three examples. The duration of full strength treatment is 730,600, and 450 days respectively for K = 1, 10,100. In Figures 3b, 3d, 3f the rebound of infected tick populations is shown. For all three examples there is an immediate drop in infected tick populations from over 9000 nymphs and 4500 adults on day 1 to less than 10% of this number by day 9, representing a 90% reduction of disease risk. Suppression of infected tick populations persists for 1152, 1123, and 1094 days, for K = 1, 10,100 respectively (1095 days = 1 year). Taken together, these examples show that if treatment remains at full strength for 450 days or more, with declining partial strength for the shorter treatments, disease risk is reduced by 90% for 1094 days or more. These examples show both the effectiveness of wall treatment and the importance of treatments with effectiveness that persists for a relatively long time. By contrast, note that applications of liquid deltamethrin, must be reapplied every 8 weeks, so a single treatment does not persist that long [30,31].
When applying an intervention to an entire community, coverage will always be imperfect. Some households will refuse treatment and some parts of a house may be inaccessible or inappropriate for treatment.
The model expresses coverage levels in a parameter, dWT, which is varied in Figure 4. The tradeoff between the duration of maximum treatment, illustrated in the right hand panel, and rate of reduction of disease prevalence, in the left hand panel, is clear. Interventions that are long lasting are seen to compensate somewhat for lack of coverage. For example, Figures 4e and 4f show a scenario in which disease prevalence in dogs is reduced from 90% to 20% in the course of a year of maximal treatment of 35% of walls. By comparison, with 100% coverage, shown in Figures 4a and 4b, the reduction in disease prevalence is better and the control is allowed to decline at about 700 days.
Studies of the efficacy of insecticidal wall treatments describe the decline in efficacy in terms of a half life, similar to linear toxicokinetics of an organism [46,47,48]. Interestingly, the optimal control patterns seen in Figures 3 and 4 show a similar decline after 100% of control is discontinued. The rate of decline of the optimal control seems to be slower than many of the observed rates in the literature, however.
Most insecticidal or acaricidal wall treatments lose efficacy within months of application [46,47,48]. The cost of repeated application may therefore add substantially to the intervention, on top of the cost of the product used. If a product is inconvenient to apply or must be applied repeatedly, this may have an effect on coverage levels as well, as households may decline to participate in the intervention.
The model on which this optimal control problem is built was based on an intervention in Sonora, Mexico in which two treatments were compared [31]. One was application of a liquid acaricide containing 5% deltamethrin, (Bayer K-Othrine WG250, pyrethroid), to the yards and homes of selected houses in the community. Trained personnel were required as well as oversight by licensed pest experts, and reapplied every eight weeks for eight months. Deltamethrin has been used on many species, with emerging resistance in ticks [49].
The other application used in Sonora was a paint developed by Inesfly Corporation that has been used for a range of vector control applications (Safecolor, Codequim, RSCO-USP-39-2016, carbamate) [30]. This product contains a slow release formula of 1% Propoxur. It was used to control Triatoma sp., the vector of Chagas disease [50,51,52,53,54]. It has proven useful against mosquito malaria and dengue vectors [55,56,57,58,59]. It has been used on nets to control Tsetse fly [60] and sand fly [61]. However, propoxur itself is not approved for indoor use in the United States. The developer of the slow release formula claims that the insecticidal effect persists for 2 years on interior walls. Because of the long residual effect, it is possible that this product could satisfy the treatment protocol produced by an optimal control problem if shown to be safe for indoor use. If used only on exterior walls, the coverage level would never be above 50%.
These two interventions are an excellent example of the tradeoffs required in cost versus efficacy. The deltamethrin treatment is straightforward and the product is readily available, but there is considerable cost for reapplication every eight weeks by professionals. The propoxur paint has longevity, but the product is likely to be more expensive and, in some locations such as the U.S., coverage might be limited to exterior walls.
The control problem solved here was approached with the assumption that only questing ticks are susceptible to the acaricide applied to walls. The truth is more complicated, as Rh. sanguineus also sequesters in cracks in the walls (and other locations) when not questing. Whether the acaricide penetrates the cracks, what percent of ticks are not on walls, and other physical and biological uncertainties might change the answer produced here.
The model developed here is based on a community intervention and the tick data that arose from it [30], which was not a controlled experiment. The parameters of the model, in particular, would benefit from a more contained and controlled experiment, perhaps using dogs in kennels that have been colonized by Rh. sanguineus.
The paint formulation of propoxur seems to be a promising intervention for RMSF. Whether it can be shown safe for indoor use would affect the coverage that is possible. In addition there is yet no study of its duration of effectiveness, especially outdoors. Knowing this would allow the model to give a more dependable prediction of the results of any intervention.
The numerical experiments in this study demonstrate the value of long lasting acaricidal wall treatments against the tick Rh. sanguineus, vector of Ri. rickettsii, the vector of RMSF. Risk of RMSF declines by 90% with 70% wall coverage and at least 450 of full strength efficacy. This risk reduction persists well beyond the window of effectiveness for the wall treatment. This study highlights the need for further trials of wall treatment interventions against RMSF both in laboratory settings and in the field.
The authors declare they have not used Artificial Intelligence (AI) tools in the creation of this article.
The model development was funded in part by the National Science Foundation (award 2019609).
All authors declare no conflicts of interest in this paper.
Parameter | Description | Value | Units |
b | oviposition rate | 62.56 | eggs per tick per day |
de | daily death rate of eggs | .015 | percent per day |
d3 | daily death rate of feeding larvae | =.2 | percent per day |
dfn | daily death rate of feeding nymphs | =.01 | percent per day |
dA3 | daily death rate of feeding adults | .01 | percent per day |
dA5 | daily death rate of gestating adults | .0351 | percent per day |
m1 | young larvae to questing larvae maturation rate | .069 | percent per day |
m2 | questing larvae to feeding larvae maturation | .1 | percent per day |
m3 | larvae feeding on host maturation rate | .232 | percent per day |
mn2 | questing nymph maturation rate | .1 | percent per day |
mfn | feeding nymph maturation rate | .142 | percent per day |
mA2 | questing adult maturation rate | .1 | percent per day |
mA3 | feeding adult maturation rate | .1058 | percent per day |
C | per host carrying capacity | 50 | maximum feeding nymphs and adults per host |
bH | birth rate of host | .0135 | per dog per day |
KH | carrying capacity of hosts | 2000 | number of dogs |
dH | death rate of uninfected host | 0.0002739726 | percent per day |
dHI | death rate of infected hosts | 0.005479 | percent per day |
pUI | probability of host infection by one feeding tick | .0001 | per infective tick per day |
pL | percent of feeding larvae infected | .1 | percent per day |
pN | percent of feeding nymphs infected | .1 | percent per day |
ϵ | numerical feature | .01 | no units |
[1] | National Institute of Health, HIV and Nutrition and Food Safety, Accessed: 2023-01-12. Available from: https://hivinfo.nih.gov/understanding-hiv/fact-sheets/hiv-and-nutrition-and-food-safety. |
[2] | N. S. Scrimshaw, J. P. SanGiovanni, Synergism of nutrition, infection and immunity: An overview, Am. J. Clin. Nutr., 66 (1997), 464S–477S. |
[3] |
E. Colecraft, HIV/AIDS: nutritional implications and impact on human development, Proc. Nutr. Soc., 67 (1997), 109–113. https://doi.org/10.1017/S0029665108006095 doi: 10.1017/S0029665108006095
![]() |
[4] |
W. Baisel, Nutrition and immune function: Overview, J. Nutr., 126 (1996), 2611S–2615S. https://doi.org/10.1093/jn/126.suppl_10.2611S doi: 10.1093/jn/126.suppl_10.2611S
![]() |
[5] |
S. V. Thuppal, S. Jun, A. Cowan, R. L. Bailey, The nutritional status of HIV-infected US adults, Curr. Dev. Nutr., 1 (2017), 1–6. https://doi.org/10.3945/cdn.117.001636 doi: 10.3945/cdn.117.001636
![]() |
[6] | S. H. Mehta, J. Astemborski, T. R. Sterling, D. L. Thomas, D. Vlahov, Serum albumin as a prognostic indicator for HIV disease progression, AIDS Res. Hum. Retroviruses, 22 (2006), 14–21. https://doi.org/10.1089/aid.2006.22.14 |
[7] | M. E. Linares, J. F. Bencomo, L. E. Perez, O. Torrez, O. Barrera, Influence of HIV/AIDS infection on some biochemical indicators of the nutritional status, Biomedica, 22 (2002), 116–122. |
[8] |
S. H. Mehta, J. Astemborski, L. E. Perez, T. R. Sterling, D. L. Thomas, Serum albumin as a prognostic indicator for HIV disease progression, AIDS Res. Hum. Retroviruses, 22 (2006), 14–21. https://doi.org/10.1089/aid.2006.22.14 doi: 10.1089/aid.2006.22.14
![]() |
[9] |
L. Lang, Z. Zhu, Z. Xu, S. Zhu, P. Meng, H. Wang, et al., The association between the albumin and viral negative conversion rate in patients infected with novel coronavirus disease 2019 (COVID-19), Infect. Drug Resist., 15 (2022), 1687–1694. https://doi.org/10.2147/IDR.S353091 doi: 10.2147/IDR.S353091
![]() |
[10] |
N. Schenker, L. G. Borrud, V. L. Burt, L. R. Curtin, K. M. Flegal, J. Hughes, et al., Multiple imputation of missing dual-energy X-ray absorptiometry data in the National Health and Nutrition Examination Survey, Stat. Med., 30 (2011), 260–276. https://doi.org/10.1002/sim.4080 doi: 10.1002/sim.4080
![]() |
[11] |
S. P. Caudill, Use of pooled samples from the national health and nutrition examination survey, Stat. Med., 31 (2012), 3269–3277. https://doi.org/10.1002/sim.5341 doi: 10.1002/sim.5341
![]() |
[12] |
M. T. L. Vasconcellos, P. L. N. Silva, L. A. Anjos, Sample design for the nutrition, physical activity and health survey (PNAFS), Niterói, Rio de Janeiro, Brazil, Estadistica, 65 (2013), 83–98. https://doi.org/10.1007/978-3-319-43851-1 doi: 10.1007/978-3-319-43851-1
![]() |
[13] |
J. Ma, W. Chan, C. L. Tsai, M. Xiong, B. C. Tilley, Analysis of transtheoretical model of health behavioral changes in a nutrition intervention study—a continuous time Markov chain model with Bayesian approach, Stat. Med., 34 (2015), 3577–3589. https://doi.org/10.1002/sim.6571 doi: 10.1002/sim.6571
![]() |
[14] | J. A. Novotny, M. H. Green, R. C. Boston, Mathematical Modeling in Nutrition and the Health Sciences, in Advances in Experimental Medicine and Biology, Kluwer Academic/Plenum Publishers, New York, 2003. |
[15] | B. Juillet, J. Salomon, D. Tomé, H. Fouillet, Development and calibration of a modeling tool for the analysis of clinical data in human nutrition, in ESAIM: Proceedings, EDP Sciences, 14 (2005), 124–155. https://doi.org/10.1051/proc: 2005011 |
[16] |
D. A. Drew, A mathematical model for nutrient metabolic chemistry, Appl. Math., 9 (2018), 647–671. https://doi.org/10.4236/am.2018.96045 doi: 10.4236/am.2018.96045
![]() |
[17] |
M. Chudtong, A. De Gaetano, A mathematical model of food intake, Math. Biosci. Eng., 18 (2021), 1238–1279. https://doi.org/10.3934/mbe.2021067 doi: 10.3934/mbe.2021067
![]() |
[18] | N. H. Shah, F. A. Thakkar, B. M. Yeolekar, Optimal control model for poor nutrition in life cycle, Dyn. Contin. Discrete Impuls. Syst. Ser. B Appl. Algorithms, 24 (2017), 387–400. |
[19] |
D. Baleanu, A. Jajarmi, E. Bonyah, M. Hajipour, New aspects of poor nutrition in the life cycle within the fractional calculus, Adv. Differ. Equations, 230 (2018), 14. https://doi.org/10.1186/s13662-018-1684-x doi: 10.1186/s13662-018-1684-x
![]() |
[20] | Mathematical Nutrition Models, Accessed: 2023-01-11. Available from: https://www.nutritionmodels.com. |
[21] | S. Coburn, D. W. Townsend, Mathematical Modeling in Experimental Nutrition: Vitamins, Proteins, Methods, Academic Press, San Diego, 1996. |
[22] | M. Nowak, R. May, Virus Dynamics: Mathematical Principles of Immunology and Virology, Oxford University Press, New York, USA, 2000. |
[23] |
R. de Boer, A. Perelson, Target cell limited and immune control models of HIV infection: A comparison, J. Theor. Biol., 190 (1998), 201–214. https://doi.org/10.1006/jtbi.1997.0548 doi: 10.1006/jtbi.1997.0548
![]() |
[24] |
S. Baral, R. Antia, N. M. Dixit, A dynamical motif comprising the interactions between antigens and CD8 T cells may underlie the outcomes of viral infections, PNAS, 116 (2019), 17393–17398. https://doi.org/10.1073/pnas.1902178116 doi: 10.1073/pnas.1902178116
![]() |
[25] | SelfDecode, Albumin/Globulin Ratio: High and Low Ratios + Normal Range, Accessed: 2021-08-04. Available from: https://labs.selfdecode.com/blog/albumin-globulin-ratio/\#Normal_AlbuminGlobulin_Ratio. |
[26] | J. W. Haefner, Modeling Biological Systems: Principles and Applications, 2nd edition, Springer, New York, 2005. |
[27] | K. P. Burnham, D. R. Anderson, Model Selection and Multi Model Inference: A Practical Information-Theoretic Approach, Springer, 2002. |
[28] | Healthline, Total Protein Test, Accessed: 2021-07-18. Available from: https://www.healthline.com/health/total-protein#results. |
[29] | Y. S. Sarro, A. Tounkara, E. Tangara, O. Guindo, H. L. White, E. Chamot, et al., Serum protein electrophoresis: any role in monitoring for antiretroviral therapy, Afr. Health Sci., 10 (2010), 138–143. |
[30] |
A. Ronit, S. Sharma, J. V. Baker, R. Mngqibisa, T. Delory, L. Caldeira, et al., Serum albumin as a prognostic marker for serious non-AIDS endpoints in the strategic timing of antiretroviral treatment (START) study, J. Infect. Dis., 217 (2017), 405–412. https://doi.org/10.1093/infdis/jix350 doi: 10.1093/infdis/jix350
![]() |
[31] |
J. M. Conway, A. S. Perelson, Post-treatment control of HIV infection, PNAS, 12 (2015), 5467–5472. https://doi.org/10.1073/pnas.1419162112 doi: 10.1073/pnas.1419162112
![]() |
[32] | S. Duggal, T. D. Chugh, A. K. Duggal, HIV and malnutrition: effects on immune system, Clin. Dev. Immunol., 2012 (2012). https://doi.org/10.1155/2012/784740 |
[33] | N. K. Vaidya, R. M. Ribeiro, A. S. Perelson, A. Kumar, Modeling the effects of morphine on simian immunodeficiency virus dynamics, PLOS Comput. Biol., 12 (2016), https://doi.org/10.1371/journal.pcbi.1005127 |
[34] | S. M. Graham, S. Holte, J. T. Kimata, M. H. Wener, J. Overbaugh, A decrease in Albumin in early SIV infection is related to viral pathogenicity, AIDS Res. Hum. Retroviruses, 25 (2009), 433–440. |
[35] |
J. Sun, H. Li, J. Liu, J. Zhao, D. Yuan, J. Guo, et al., The DTI changes and peripheral blood test results corroborate the early brain damage of SIV-infected rhesus, Radiol. Infect. Dis., 6 (2019), 8–14. https://doi.org/10.1016/j.jrid.2019.01.001 doi: 10.1016/j.jrid.2019.01.001
![]() |
[36] |
L. Lavreys, J. M. Baeten, V. Chohan, R. S. McClelland, W. M. Hassan, B. A. Richardson, et al., Higher set point plasma viral load and more-severe acute HIV type 1 (HIV-1) illness predict mortality among high-risk HIV-1-infected african women, Clin. Infect. Dis., 42 (2006), 1333–1339. https://doi.org/10.1086/503258 doi: 10.1086/503258
![]() |
[37] |
J. M. Conway, A. S. Perelson, Post-treatment control of hiv infection, Proc. Natl. Acad. Sci., 112 (2015), 5467–5472. https://doi.org/10.1073/pnas.1419162112 doi: 10.1073/pnas.1419162112
![]() |
[38] |
H. Miao, X. Xia, A. S. Perelson, H. Wu, On identifiability of nonlinear ode models and applications in viral dynamics, SIAM Rev., 53 (2011), 3–39. https://doi.org/10.1137/090757009 doi: 10.1137/090757009
![]() |
[39] |
G. Bellu, M. Saccomani, S. Audoly, L. D'Angio, Daisy: a new software tool to test global identifiability of biological and physiological systems, Comput. Methods Programs Biomed., 88 (2007), 52–61. https://doi.org/10.1016/j.cmpb.2007.07.002 doi: 10.1016/j.cmpb.2007.07.002
![]() |
[40] |
N. Tuncer, C. Mohanakumar, S. Swanson, M. Martcheva, Efficacy of control measures in the control of Ebola, Liberia, 2014–2015, J. Biol. Dyn., 12 (2018), 913–937. https://doi.org/10.1080/17513758.2018.1535095 doi: 10.1080/17513758.2018.1535095
![]() |
[41] |
N. Tuncer, M. Martcheva, Determining reliable parameter estimates for within-host and within-vector models of zika virus, J. Biol. Dyn., 15 (2021), 430–454. https://doi.org/10.1080/17513758.2021.1970261 doi: 10.1080/17513758.2021.1970261
![]() |
Parameter | Description | Value | Units |
b | oviposition rate | 62.56 | eggs per tick per day |
de | daily death rate of eggs | .015 | percent per day |
d3 | daily death rate of feeding larvae | =.2 | percent per day |
dfn | daily death rate of feeding nymphs | =.01 | percent per day |
dA3 | daily death rate of feeding adults | .01 | percent per day |
dA5 | daily death rate of gestating adults | .0351 | percent per day |
m1 | young larvae to questing larvae maturation rate | .069 | percent per day |
m2 | questing larvae to feeding larvae maturation | .1 | percent per day |
m3 | larvae feeding on host maturation rate | .232 | percent per day |
mn2 | questing nymph maturation rate | .1 | percent per day |
mfn | feeding nymph maturation rate | .142 | percent per day |
mA2 | questing adult maturation rate | .1 | percent per day |
mA3 | feeding adult maturation rate | .1058 | percent per day |
C | per host carrying capacity | 50 | maximum feeding nymphs and adults per host |
bH | birth rate of host | .0135 | per dog per day |
KH | carrying capacity of hosts | 2000 | number of dogs |
dH | death rate of uninfected host | 0.0002739726 | percent per day |
dHI | death rate of infected hosts | 0.005479 | percent per day |
pUI | probability of host infection by one feeding tick | .0001 | per infective tick per day |
pL | percent of feeding larvae infected | .1 | percent per day |
pN | percent of feeding nymphs infected | .1 | percent per day |
ϵ | numerical feature | .01 | no units |
Parameter | Description | Value | Units |
b | oviposition rate | 62.56 | eggs per tick per day |
de | daily death rate of eggs | .015 | percent per day |
d3 | daily death rate of feeding larvae | =.2 | percent per day |
dfn | daily death rate of feeding nymphs | =.01 | percent per day |
dA3 | daily death rate of feeding adults | .01 | percent per day |
dA5 | daily death rate of gestating adults | .0351 | percent per day |
m1 | young larvae to questing larvae maturation rate | .069 | percent per day |
m2 | questing larvae to feeding larvae maturation | .1 | percent per day |
m3 | larvae feeding on host maturation rate | .232 | percent per day |
mn2 | questing nymph maturation rate | .1 | percent per day |
mfn | feeding nymph maturation rate | .142 | percent per day |
mA2 | questing adult maturation rate | .1 | percent per day |
mA3 | feeding adult maturation rate | .1058 | percent per day |
C | per host carrying capacity | 50 | maximum feeding nymphs and adults per host |
bH | birth rate of host | .0135 | per dog per day |
KH | carrying capacity of hosts | 2000 | number of dogs |
dH | death rate of uninfected host | 0.0002739726 | percent per day |
dHI | death rate of infected hosts | 0.005479 | percent per day |
pUI | probability of host infection by one feeding tick | .0001 | per infective tick per day |
pL | percent of feeding larvae infected | .1 | percent per day |
pN | percent of feeding nymphs infected | .1 | percent per day |
ϵ | numerical feature | .01 | no units |