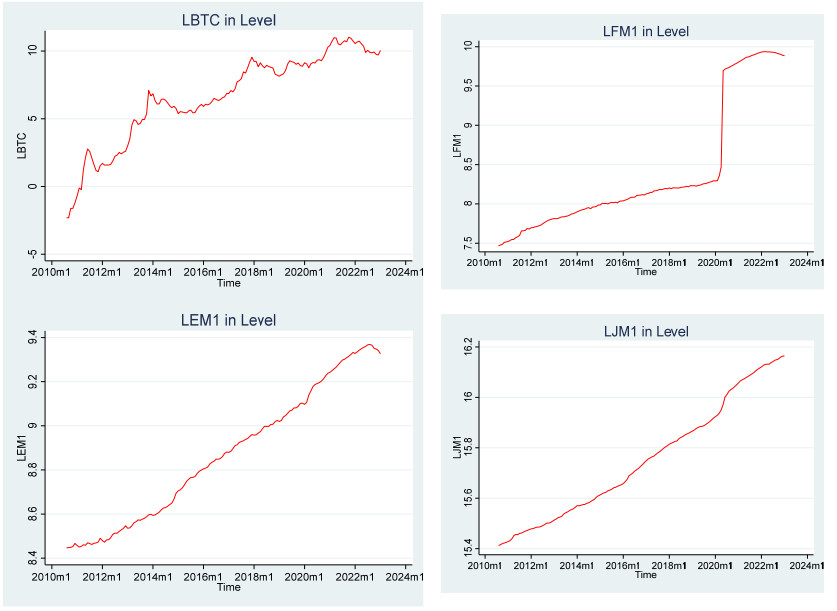
Bitcoin has become quite known after the 2008 economic crisis and the COVID-19 health crisis. For some, these cryptocurrencies constitute rebellion against the existing system as governments encourage uncontrolled expansions in the money supply; for some others, it is a quick source of income. Undeniably, the volume of the crypto money market has grown considerably in recent years, regardless of the reasoning of the people who invest and trade in this field. At this point, one of the most important questions to be investigated is "what variables have caused the tremendous growth in the crypto money quantities in recent years?" This study tests the assumption that changes in cryptocurrencies are affected by changes in national currencies. Thus, the Bitcoin price is the dependent variable, and M1 monetary supply changes in the USA, European Union and Japanese economies are considered independent variables. The variables in this study were tested using the time-varying Granger causality method. The results obtained from this study confirm the philosophy of Bitcoin's emergence and the possibility that it can be a hedge against the inflationary effects of money, especially after the COVID-19 pandemic.
Citation: Nilcan Mert, Mustafa Caner Timur. Bitcoin and money supply relationship: An analysis of selected country economies[J]. Quantitative Finance and Economics, 2023, 7(2): 229-248. doi: 10.3934/QFE.2023012
[1] | Huseyin Tastan, Sercin Sahin . Low-frequency relationship between money growth and inflation in Turkey. Quantitative Finance and Economics, 2020, 4(1): 91-120. doi: 10.3934/QFE.2020005 |
[2] | Patrick Mumbi Chileshe . Banking structure and the bank lending channel of monetary policy transmission: Evidence from panel data methods. Quantitative Finance and Economics, 2018, 2(2): 497-524. doi: 10.3934/QFE.2018.2.497 |
[3] | Bonang N. Seoela . Efficacy of monetary policy in a currency union? Evidence from Southern Africa's Common Monetary Area. Quantitative Finance and Economics, 2022, 6(1): 35-53. doi: 10.3934/QFE.2022002 |
[4] | Richard J. Cebula, Robert Boylan . Uncertainty regarding the effectiveness of Federal Reserve monetary policies over time in the U.S.: an exploratory empirical assessment. Quantitative Finance and Economics, 2019, 3(2): 244-256. doi: 10.3934/QFE.2019.2.244 |
[5] | Mustafa Hassan Mohammad Adam . Nexus among foreign direct investment, financial development, and sustainable economic growth: Empirical aspects from Sudan. Quantitative Finance and Economics, 2022, 6(4): 640-657. doi: 10.3934/QFE.2022028 |
[6] | Abderahman Rejeb, Karim Rejeb, John G. Keogh . Centralized vs. decentralized ledgers in the money supply process: a SWOT analysis. Quantitative Finance and Economics, 2021, 5(1): 40-66. doi: 10.3934/QFE.2021003 |
[7] | Melike E. Bildirici, Mesut M. Badur . The effects of oil prices on confidence and stock return in China, India and Russia. Quantitative Finance and Economics, 2018, 2(4): 884-903. doi: 10.3934/QFE.2018.4.884 |
[8] | Cunyi Yang, Li Chen, Bin Mo . The spillover effect of international monetary policy on China's financial market. Quantitative Finance and Economics, 2023, 7(4): 508-537. doi: 10.3934/QFE.2023026 |
[9] | Tram Thi Xuan Huong, Tran Thi Thanh Nga, Tran Thi Kim Oanh . Liquidity risk and bank performance in Southeast Asian countries: a dynamic panel approach. Quantitative Finance and Economics, 2021, 5(1): 111-133. doi: 10.3934/QFE.2021006 |
[10] | Guillermo Peña . Interest rates affect public expenditure growth. Quantitative Finance and Economics, 2023, 7(4): 622-645. doi: 10.3934/QFE.2023030 |
Bitcoin has become quite known after the 2008 economic crisis and the COVID-19 health crisis. For some, these cryptocurrencies constitute rebellion against the existing system as governments encourage uncontrolled expansions in the money supply; for some others, it is a quick source of income. Undeniably, the volume of the crypto money market has grown considerably in recent years, regardless of the reasoning of the people who invest and trade in this field. At this point, one of the most important questions to be investigated is "what variables have caused the tremendous growth in the crypto money quantities in recent years?" This study tests the assumption that changes in cryptocurrencies are affected by changes in national currencies. Thus, the Bitcoin price is the dependent variable, and M1 monetary supply changes in the USA, European Union and Japanese economies are considered independent variables. The variables in this study were tested using the time-varying Granger causality method. The results obtained from this study confirm the philosophy of Bitcoin's emergence and the possibility that it can be a hedge against the inflationary effects of money, especially after the COVID-19 pandemic.
LBTC: Bitcoin price; LFM1: USA central bank money supply, LEM1: European central bank money supply; LJM1: Japan central bank money supply
The feeling of trust is one of the most important resources for societies to ensure a long-term happy and peaceful coexistence. In this context, the sense of trust ensures continuity in the relations between individuals and institutions; it can be expressed as a conscious choice that includes the fulfillment of commitments, sincerity, truth, honesty and virtue. In addition to social order and individual lives, it forms the basis of economic and democratic development (Gökalp, 2003).
Today, in many societies which have developed their institutional capacities and are expressed as "modern societies", it is easier to establish trust given the supporting elements such as the rule of law and openness, as compared to other underdeveloped and developing countries. It is known that, in developed countries that have successfully established their institutional structure, the division of labor between institutions and their capacity to deal with problems are easier than in underdeveloped countries. In other words, while the solution to a problem in developed countries is generally evaluated within itself, in underdeveloped and developing countries, the solution of a problem is mostly evaluated based on the solution of other problems (Savaş, 2016).
One of the areas where the feeling of trust is most sensitive is undoubtedly the economy. Whether in a developed or underdeveloped and developing society, investors, capitalists and entrepreneurs are expected to flee when the sense of trust is lost. Thus, the countries that have built their institutional infrastructure are able to attract investments to their countries with the help of the legal guarantees they offer in the constitutional context, although they often promise low profitability.
Independent central banks are among the most important institutions necessary for establishing economic confidence. Central banks, which direct the economy using monetary policy tools in line with the economic goals of the countries, have been given great responsibilities. These responsibilities include ensuring price stability, monetary expansion proportional to economic growth, financial stability and controlling exchange rate regimes. Many studies consider the simultaneous independence of central banks and the fulfillment of their duties a very important factor (Acemoglu et al., 2008; Alesina and Summers, 1993; Cukierman et al., 1993; Arnone et al., 2007).
There are various concerns about the possibility of central banks losing independence. These concerns arise mostly due to political reasons, such as the politicians' efforts to increase production and prosperity in the short term to ensure their good performance in elections. The possibility that politicians may utilize the ability of central banks to create money to finance government debt is another such factor. In general terms, the autonomy of central banks is important because the politicians in power are not considered credible and may use their powers for their own interests (De Haan and Eijffinger, 2016).
In addition to the duty of ensuring price stability, which is generally accepted in the economic literature, new duties of independent central banks, such as ensuring financial stability, have been added after the mortgage crisis that emerged in the USA economy in 2008. During the 2008 mortgage crisis, many financial institutions that had difficulty collecting their receivables went bankrupt. Some institutions were saved at the brink of bankruptcy by the bailout packages prepared by the state with the "too big to fail" approach due to the concerns that it would lead to chain bankruptcies. Liabilities owned by these organizations and described as "toxic assets" were assumed by the USA's Department of the Treasury and, thus, by the US citizens. To ensure that the liabilities of this procedure were not just shared between the USA's Department of the Treasury and residents, but also had global implications, it was crucial that the planned rescue packages were carried out using the United States dollar, which serves as the world's reserve currency.
After the COVID-19 crisis, which emerged approximately 10 years after the 2008 mortgage crisis, central banks increased their asset purchases due to economic concerns. In this period, the determination of central banks to reduce inflation on the global scale decreased and gave way to other economic concerns, such as unemployment and growth, which are considered to be more important. At this point, the line between the central banks and government policies began to blur. Monetary policy adjustments tend to adapt and support expansionary fiscal policies rather than long-term inflation targeting. Central bank autonomy, which was once critical for market confidence in a country's bond market, has now become an absolute asset buyer in the market, irrespective of its long-term goals (Insight Investment, 2020).
This extra liquidity provided to the market by central banks for various reasons causes a real loss in the assets of those who previously owned the assets due to inflationary effects. The excessive growth of the monetary bases controlled by central banks at the global scale degrades the reliability of the national currencies of these countries; in addition, the savings-earnings of the households that are largely unaware of this process are reduced by the central banks and governments.
One of the most important reactions to this economic policy came from the Internet. On November 1, 2008, Satoshi Nakamoto announced the whitepaper of the popular cryptocurrency Bitcoin. On this date, Nakamoto defined Bitcoin, the first currency based on cryptography, as a medium of exchange that is decentralized and does not rely on any central authority or record-holder. With this feature, it possesses a cryptographic proof-based electronic payment system in which two parties can directly transact with each other without the need for a third trusted person, thus replacing the trust element traditionally needed during transactions (Nakamoto, 2008).
As a result of governments' uncontrolled use of policy tools during the 2007–2008 financial crisis, the real economy was separated from the paper economy; this separation has resulted in debts from firms and households that cannot be expected to be repaid (Perez, 2009). The functioning of the monetary system has been discussed extensively by the public since the 2008 mortgage crisis. Thus, some people began to explore solutions to solve reputational difficulties in the fiat money system by turning to non-monetary assets, such as gold and silver, as a means of holding value. Undertaking this task, Bitcoin portrayed itself as an alternative monetary system in reaction to the current monetary system (Weber, 2014). This vantage point suggests that the emergence of Bitcoin after the 2008 mortgage crisis is not a coincidence. This poses a challenge to the Bitcoin credit system's viability, as well as to the notion that debt has always been a vital aspect of money. It also acts as a test environment for a new alternative system (Kostakis and Giotitsas, 2014). Fiat money, which is under the control of central banks, can be produced freely and endlessly, as in the Weimar Republic; however, Bitcoin can be produced in a limited way with a pre-planned and periodically increasing cost. This situation is expected to cause an inflationary effect on the purchasing power of nominal currencies over time, and Bitcoin, which is accepted in some circles and whose supply is limited, is expected to increase in value. In reality, there are two intrinsically worthless currencies, both of which can be used for transactions: Bitcoin and central bank currencies (Schilling and Uhlig, 2019).
The major thesis of this study is that there is a causal relationship between central bank money supplies and cryptocurrency prices. The FED (Federal Reserve System), ECB (European Central Bank) and BOJ (Bank of Japan) central banks, which have significant weight in the global money market, and Bitcoin, which has the largest volume in the crypto money market, were chosen to test this idea. The data were used to examine the link between the M1 money supplies of the three central banks in the sample with high representativeness of the research universe, and the values of the cryptocurrency Bitcoin cover the period 2010: M8–2023: M1.
Although cryptocurrency is a fairly new field, it has attracted many researchers given the popularity it has gained in a short time. The number of theoretical and empirical studies in this field is increasing daily, and their relationships with different variables are being examined. The relationship of Bitcoin, which is considered an investment and savings tool by many people today, has been the focus of many studies, along with other variables. This study was aimed to include relevant studies in this area.
Many studies have examined whether cryptocurrencies can be used as hedging instruments. Bouri et al.(2017a, 2017b), Dyhrberg (2016), Fang et al. (2019), Su et al. (2020), Urquhart and Zhang (2019) and Wu et al. (2019) studied the possible use of cryptocurrency as a hedging tool. Dyhrberg (2016) found that hedges against the FTSE can be made using the Bitcoin Prices Index and the United States dollar. This can be added to the investment basket for portfolio diversification and risk control. According to Bouri et al. (2017a), Bitcoin is a weak hedge tool that should only be used for diversification. Wu et al. (2019) discovered that it has a modest protection function under economic policy uncertainty. However, Su et al. (2020) proved that Bitcoin can be used to lower geopolitical concerns for economies and investors. Bouri et al. (2017b) discovered that it could operate as a buffer against uncertainties. Urquhart and Zhang (2019) discovered that Bitcoin could be used as a hedge against certain currencies. Fang et al. (2019) noted that it can function as a hedging mechanism in particular situations of economic instability. Urquhart and Zhang (2019) discovered that Bitcoin functions as a daily trading hedge, a form of investment and a safe zone against specific currencies.
Many important studies have linked cryptocurrencies with money supply and inflation. Some important studies were conducted by Blau et al. (2021), Chohan (2021), Conlon et al. (2021), Narayan et al. (2019) and Wang et al. (2022). Blau et al. (2021) offered strong evidence: a sudden surge in the price of Bitcoin is linked to a large increase in the inflation rate. This lends credence to the notion that Bitcoin can serve as an inflation hedge. According to Narayan et al. (2019), Indonesia's monetary aggregates and Bitcoin growth rates are connected. In addition, Bitcoin price hikes have been found to reduce the velocity of money and evaluate currency. Chohan (2021) claims that the citizens of a developing country can use Bitcoin to protect themselves from hyperinflationary processes. Conlon et al. (2021) studied the time-series association between cryptocurrency values and future inflation expectations. The findings from the wavelet timescale technique indicate that expected inflation forecasts have a brief positive correlation with both Bitcoin and Ethereum. This also coincides with the early beginnings of the COVID-19 crisis. Outside of this time frame, there is only limited proof that cryptocurrencies act as a hedge against rising inflation expectations. Wang et al. (2022) examined the long- and short-term interactions between Bitcoin prices and money supply, the consumer price index (CPI) and economic policy uncertainty (EPU). The findings show that money supply has an impact on Bitcoin prices, and that money supply, CPI and EPU, all exhibit dynamic inter-shocks. Bitcoin prices are negatively affected by money supply and EPU. Bitcoin values, which are viewed as a type of hedge mechanism, are positively impacted by CPI. Ma et al. (2022) studied the impact of USA monetary policy shocks on Bitcoin prices. The study's findings emphasize how monetary policy crucially affects the price of Bitcoin. Despite arguments to the contrary, monetary policy shocks contribute to a relatively minor percentage of Bitcoin price volatility. Schilling and Uhlig (2019a) emphasized the significance of monetary policy for cryptocurrencies in an economy where fiat and cryptocurrencies coexist and are both seen as worthless.
Studies have been conducted to determine the relationships between cryptocurrencies and other financial instruments. Haşlak (2018) evaluated the causality between Bitcoin and the USD, EUR GBP and JPY, which are accepted as stable currencies, as well as gold and oil. The findings show that Bitcoin has no association with currencies or commodity prices over the long term, but that there is a short-term reciprocal causal relationship with commodity prices. Zhu et al. (2017) analyzed monthly data for the period of 2011–2016 using a vector error correction model. In the model, the Custom Price Index, US Dollar Index, Dow Jones Industrial Average, Federal Funds Rate and gold price were used as economic variables influencing Bitcoin prices. The results suggest that all of these variables affect Bitcoin prices, but their respective influences differ. The relationship between Bitcoin and the numerous currencies, commodities, securities and altcoins was explored by Pirgaip et al. (2019). According to their findings, Bitcoin has no long-term association with any asset. Fang et al. (2019) investigated whether uncertainty in global economic policy affects the long-term volatility of Bitcoin, global stocks, commodities and bonds. They discovered that uncertainty in global economic policy has a favorable effect on Bitcoin-stock correlation and a negative and substantial effect on Bitcoin-bond correlation. Warsito (2020) used the variables, gold, dollar index and composite stock price index in the Indonesia Stock Exchange variables and Bitcoin and Ethereum cryptocurrencies. Warsito (2020) determined that the volatility of Bitcoin and Ethereum is not affected by other variables, but by their own historical prices. Schilling and Uhlig (2019b) have created a model that shows that Bitcoin can compete as a viable alternative to traditional fiat currencies based on transfer costs, taxes and exchange rates. Benigno et al. (2022) developed a model in which there are two national fiat monetary systems and one global currency (cryptocurrency). If the global currency is used in both countries, the national nominal interest rates should be equal. Deviation from the interest rate parity introduces the risk of approaching the zero lower bound or abandoning the national currency.
The aim of this study is to model the dynamics between Bitcoin prices and the money supply of the three major economies. To this end, Bayesian-VAR, Toda-Yamamoto (1995) causality tests were carried out in the first stage of the empirical analysis. Then, the time-varying causality test was modeled with the thought that the causality relationship may change over time due to the occurrence of some structural changes. The remainder of the paper is arranged in follows: The section titled "Data and methodology" describes the econometric approach and data. This section also includes the empirical analysis. The discussion of empirical findings is detailed in the section titled "Findings". The section under "Conclusion" contains the results and comments.
This study econometrically investigates the effect of money supply increases in the USA, the eurozone (19 countries) and Japan on Bitcoin prices. These three economies were evaluated within the scope of the study due to the reliability and accessibility of the data in terms of transparency and the volumes of the economies. Due to the monthly data set available for Bitcoin prices, the sample period ran from August 2010 to January 2023, with a total of 150 monthly observations. The data set for Bitcoin prices was obtained from the historical data page published by Yahoo Finance; the narrow money series for the USA, Japan and eurozone (19 countries) economies were obtained from the monthly economic indicators of the Organization for Economic Co-operation and Development data bank. LBTC, LFM1, LEM1 and LJM1 represent a series of Bitcoin prices, the USA central bank money supply, the European central bank money supply and Japan's central bank money supply, respectively.
Although the LBTC series has shown a downward trend in some periods, we see that, in Figure 1, it has an upward trend in general. It is noteworthy that the eurozone and Japanese money supply series show a similar upward trend. The graph of the Fed's money supply series shows that there was an increase of less than 0.5 basis points between 2010 and 2020, a dramatic increase was experienced in 2020 and the increasing trend continued until 2022.
We carried out the Leybourne (1995, ADFmax) test based on the maximum Augmented Dickey-Fuller ((Dickey and Fuller, 1981), ADF) t-statistic and nonlinear STAR type Kepatanios, Shin, & Snell (2003, KSS) tests, in order to investigate whether the time series used in the study have a random walk process. Kepatanios et al. (2003) proposed a test procedure to detect no stationarity for exponential smooth transition autoregressive processes in nonlinear series.
yt=βyt−1+γyt−1Θ(θ;yt−d)+εt,t=1,…,T | (1) |
In Equation (1), we have that εtiid(0,σ2); β and γ are prediction parameters; the exponential pass function is defined as Θ(θ;yt−d)=1−exp(−θy2t−d). In addition, Equation (1) is assumed to be θ≥0, and the delay parameter is d≥1. Under this assumption, Equation (1) can be expressed as follows:
Δyt=φyt−1+γyt−1[1−exp(−θy2t−d)]+εt | (2) |
In Equation (2), φ=β−1. If θ is positive, it effectively determines the average rate of return. Equation (3) is written under constraints of φ=0 and d=1.
Δyt=γyt−1[1−exp(−θy2t−1)]+εt | (3) |
The null hypothesis expressing the unit root is defined as H0:θ=0, and the alternative hypothesis expressing a nonlinear return to the mean is defined as H1:θ>0. Emphasizing that it is not possible to test the null hypothesis directly, Kapetanios et al. (2003) proposed a new auxiliary regression equation by calculating the first-order Taylor series and considering that the error terms of the equation may have autocorrelation.
Δyt=∑pj=1ρjΔyt−j+δy3t−1+εt | (4) |
For Equation (4), the t-statistic to test the H0:δ=0 hypothesis against the H1:δ<0 hypothesis is defined as tNL=ˆδs.e.(ˆδ) (Kapetanios et al., 2003). Noting that the tNL statistic is not a normally distributed asymptotic standard, Kapetanios et al. (2003) calculated new critical values for three cases in their study.
In their work on the concept of time change using the Granger causality test, Shi et al. (2018) and Shi et al. (2020) emphasized that evaluating the persistence of causal links throughout time is possible. Tests based on the lag-augmented VAR (LA-VAR) approach developed by Dolado and Lütkepohl (1996) and Yamada and Toda (1998) allow for non-stationary variables. In addition, although Shi et al.(2018, 2020) developed a new method, they considered the other two methods suggested by Thoma (1994), Swanson (1998) and Balcılar et al. (2010) (Kendirkiran, 2021). They developed three different algorithms using recursive estimation methods: the forward expanding (FE) window, the rolling (RO) window and the recursive evolving (RE) window.
The unconstrained VAR(p) model can be expressed as the following multivariate matrices:
yt=Πxt+εt,t=1,…,T | (5) |
In Equation (5), yt=(y1t,y2t)' is the endogenous variable vector; xt=(1,y't−1,y't−2,…,y't−p)' is the explanatory variable matrix; Π2×(2p+1)=[Φ0,Φ1,…,Φp] represents the coefficient vector. ˆΠ is the least squares estimator; ˆΩ=T−1∑Tt=1ˆεtˆε't is the error term variance; X'=[x1,x2,…,xT] shows the observed explanatory variables. The Wald test statistic calculated to test the null hypothesis that y2t is not the Granger cause of y1t is as follows:
W=[Rvec(ˆΠ)<]'[R(ˆΩ⊗(X'X))R']−1[Rvec(ˆΠ)] | (6) |
In Equation (6), R, represents the selection matrix p×2(2p+1), and the 2(2p+1)×1 dimensional coefficient vector of ˆΠ is represented by →(ˆΠ). Under the null hypothesis stating that there is no causality, each R row chooses one of the p coefficients over the lagged values of y2t, in Equation (5), to set it to zero. Under the assumption of non-causality and conditional homoscedasticity, the Wald test statistic exhibits an asymptotic χ2p distribution (Shi et al., 2018).
To reveal changes in causal relationships over time, Thoma (1994) proposed the FE window Wald test. According to Thoma's (1994) FE window Granger causality method, the starting point is fixed at f1 for all subsamples, where f is the fractional observation of interest, and f0 is the necessary smallest window size to predict the model. The fractional f0 indicates the minimum window size; observation f, which expresses the causality period of interest, progresses from f0 to 1 (Shi et al., 2018).
The regression array's regression window (fw) size is maintained constant using the Swanson rolling technique and, accordingly, keeps a fixed distance from the starting point f2 as the corresponding observation (f, and hence the endpoint of regression f2) is rounded from f0 to 1. According to Swanson's (1985) procedure, a remarkable change in causality is observed when an element of the Wald statistical progression exceeds the associated critical value. The onset date of an alteration in causality is defined as the initial observation that exceeds the critical value corresponding to the test statistical value.
Drawing on the work of Phillips et al.(2015a, 2015b), a supplemental test based on the supremum norm (sup) of an iteratively developed set of Wald statistics is proposed.
Accordingly, for each relevant fractional observation (f∈[f0,1]), the Wald statistics are obtained for a set of backward-expanding samples. The endpoint of the sample strings is provisionally steady at the final observation under the f=f2 run, and they blossom forward from that point. According to this procedure, the starting points of the sample series utilized in the regressions vary between f1=(f2−f0) and the first observation. The Wald statistic procured for each subsample regression is defined in Equation (7) to be represented by Wf2(f1).
SWf(f0)(f|1,f2)∈Λ0,f2=f{Wf2(f1)} | (7) |
Λ0={(f1,f2):0<f0+f1≤f2≤1,ve0≤f1≤1−f0} |
In this procedure, which is called the RE procedure, f0∈(0,1) is assumed for the minimum sample size. In contrast to the RO window approach, the RE procedure permits a discrepancy in the window widths fw=f2−f1≥f0 utilized in the models.
In the FE window procedure, f1=0 is fixed and arranges f=f2, whereas, in the RO window procedure, the fixed window width is defined as fw=f2−f1≥f0, and window initialization is defined as f1=f2−f0. For all three procedures, f can be used for real-time tracing in the current observation, because they are based on historical information. The goal is to find the largest Wald statistic. To this end, the procedure allows regression to seek the optimum starting point for each observation. This flexibility means restarting the sub-observation to accommodate any change in structure or the causal side that may occur inside the observation (Shi et al., 2018).
fe and ff indicate the start and end points in causality and are estimated as the test statistics of the first observation that surpasses or falls under the critical value. In the case of a single pass, the dating principles are given according to the following transition times:
Forward:ˆfe=inff∈[f0,1]{f:Wf(0)>cv}∧ˆff=inff∈[ˆfe,1]{f:Wf(0)<cv} |
Rolling:ˆfe=inff∈[f0,1]{f:Wf(f−f0)>cv}∧ˆff=inff∈[ˆfe,1]{f:Wf(f−f0)<cv} |
RecursiveEvolving:ˆfe=inff∈[f0,1]{f:SWf(f0)>scv}∧ˆff=inff∈[ˆfe,1]{f:SWf(f0)<scv} |
where cv and scv respectively show the critical values of the Wf and SWf test statistics. The start and end dates are estimated similarly if there is more than one key during the sampling period. The procedure looks for the start and end dates of episode i, with i≥2 in the sample intervals [̂ˆfi−1f, 1] and [̂ˆfie, 1], respectively (Shi et al., 2018).
The empirical part of the study consisted of examining the dynamics between Bitcoin and the money supply of the three major economies. Table 1 summarizes the descriptive statistics of the series. We see that the maximum value of BTC is USD 61309.6, and the minimum value is USD 0.1. While all series are skewed to the right, it can be said that FM1, EM1 and JM1 series are kurtic compared to the normal curve, that is, their variability is high. Finally, it is observed that no series exhibited a normal distribution.
BTC | FM1 | EM1 | JM1 | |
Mean | 8759.647 | 6555.911 | 7432.246 | 7124373 |
Median | 951.1000 | 3337.150 | 7094.844 | 6781044 |
Maximum | 61309.60 | 20699.60 | 11714.43 | 10480547 |
Minimum | 0.100000 | 1748.700 | 4663.060 | 4939771 |
Std. Dev. | 14460.71 | 6790.338 | 2247.773 | 1686738 |
Skewness | 2.038437 | 1.356002 | 0.467187 | 0.551846 |
Kurtosis | 6.304107 | 2.930469 | 1.959786 | 2.046827 |
Jarque-Bera | 172.1127 | 45.99874 | 12.21939 | 13.29171 |
Probability | 0.000000 | 0.000000 | 0.002221 | 0.001299 |
Observations | 150 | 150 | 150 | 150 |
First, we applied logarithmic transformations to the series, and then investigated the correlative relationship between the Bitcoin price and the money supply series.
It has been observed that there is a positive, strong and statistically significant correlation between the Bitcoin price series and the money supply series in Table 2. We examined interactions between money supply series and Bitcoin prices with Bayesian-VAR, Toda-Yamamoto (1995) and time-varying Granger causality models. An important consideration in determining the VAR model is to choose the optimal length of lags. From a Bayesian point of view, the most convenient way to solve this problem is to use Bayesian model comparison. For this, we first selected a reasonable set of possible lags, 1, …, pmax, and then we applied a Bayesian VAR(p) model for each p lag. Finally, we compared the fitted models using their log-marginal probabilities (Litterman, 1980, 1986). We used FPE, AIC, HQIC, and SBIC information criteria to determine the appropriate maximum length in the VAR model.
Correlation | Probability | |
LFM1 | 0.7633 | 0.0000 |
LEM1 | 0.9131 | 0.0000 |
LJM1 | 0.9087 | 0.0000 |
In Table 3, the FPE and AIC suggested the maximum lag length of 4, and the HQIC and SBIC suggested a value of 3. Here, we set pmax = 5 and estimated five possible VAR models. We compare the models in Table 4.
FPE | AIC | HQIC | SBIC | |
1 | 1.6e-07 | −4.27582 | −4.20686 | 4.10613 |
2 | 2.0e-13 | −17.8646 | −17.6577 | 17.3555 |
3 | 1.1e-13 | −18.5142 | −18.1694* | 17.6657* |
4 | 9.7e-14* | −18.619* | −18.1362 | 17.4311 |
5 | 1.0e-13 | −18.5716 | −17.951 | 17.0443 |
6 | 1.1e-13 | −18.4832 | −17.7246 | 16.6165 |
7 | 1.2e-13 | −18.447 | −17.5505 | −16.241 |
8 | 1.2e-13 | −18.4068 | −17.3724 | 15.8614 |
9 | 1.4e-13 | −18.2919 | −17.1196 | 15.4071 |
10 | 1.4e-13 | −18.2801 | −16.9699 | 15.0559 |
11 | 1.7e-13 | −18.152 | −16.7038 | 14.5883 |
12 | 1.7e-13 | −18.1668 | −16.5807 | 14.2638 |
*: optimal lag. An exogenous dummy variable has been added as of March 2020 to show the effect of the COVID-19 outbreak. FPE: Final Prediction Error, AIC: Akaike Information Criterion, HQIC: Hannan-Quin Information Criterion, SBIC: Schwarz Information Criterion. |
Models | Log (ML) | P(M) | P(M|y) |
1 | 1232.9545 | 0.1000 | 0.7540 |
2 | 1231.8244 | 0.1000 | 0.2460 |
3 | 1221.1161 | 0.1000 | 0.0000 |
4 | 1212.1161 | 0.1000 | 0.0000 |
5 | 1198.3390 | 0.1000 | 0.0000 |
As can be seen in the second column of Table 4, all five models were assumed to be equally probable a priori. The third column shows the final model probabilities, and the model with the highest probability is considered the optimum model. The 1 lag model has the highest posterior probability of 0.754; therefore, we chose the lag number to be 1. We applied the Minnesota prior specification (with a fixed autoregressive (AR) covariance) to estimate the Bayesian VAR model. This method is optimal when time series are used at model levels. It also performs well as a tool to obtain the dynamic information about the relationships between variables (in the short and medium term) by using the levels of time series that we can capture by analyzing impulse-response (IRFs) and prediction error variance decomposition functions (FEVDs). Since the error covariance is considered fixed in the Minnesota equation, it reduces the number of parameters to be simulated and speeds up the calculations (Litterman, 1980, 1986). In Figure 2, the IRF curves show the effects of shock in LFM1, LEM1 and LJM1 series on LBTC.
In Figure 2, we present the one standard deviation shock response of LBTC from the money supply series for the Bayesian-VAR model over a period of 13 years (150 steps). According to the impulse-response graphs, the shocks that will occur in the three money supply series do not have statistically significant effects on Bitcoin prices.
To measure the contribution of the impulse variables to the estimation error in the response variables in terms of variability, we obtained the estimation error variance decompositions (FEVD) function plots. FEVDs depend on the causal ordering of response variables (StataCorp, 2021). In Figure 3, over the long run, Bitcoin prices contribute about 0.70 of the forecast error of Bitcoin prices, while LEM1 contributes 0.22 and LJM1 and LFM1 contribute about 0.04.
Table 5 summarizes the ADFmax and KSS unit root test results. According to both unit root tests, when the series is considered at levels, the null hypothesis stating that there is no unit root is not rejected, as it is rejected when they are in the first difference. Both unit root tests support the view that LBTC, LFM1, LEM1 and LJM1 can best be described as I(1).
LBTC | LFM1 | LEM1 | LJM1 | |||
Level | ADFmax | Constant | 1.065 (9) | −0.061 (0) | −2.194 (12) | 1.032 (1) |
Constant & Trend | −1.436 (9) | −1.600 (0) | −1.317 (12) | −1.340 (1) | ||
KSS | Constant | −1.056 (9) | 0.227 (0) | −3.273 (12)** | 0.485 (6) | |
Constant & Trend | −3.339 (9)** | −1.609 (0) | −2.305 (12) | −1.602 (1) | ||
First Differences | ADFmax | Constant | −9.017 (0)*** | −10.528 (0)*** | −3.179 (4)*** | −5.793 (0)*** |
Constant & Trend | −9.161 (0)*** | −10.541 (0)*** | −2.907 (4)* | −5.963 (0)*** | ||
KSS | Constant | −3.926 (7)*** | −12.482 (0)*** | −3.266 (1)** | −4.549 (4)*** | |
Constant & Trend | −3.847 (7)*** | −12.488 (0)*** | −3.309 (1)* | −4.758 (4)*** | ||
The optimal lags determined according to the Akaike information criterion are in parentheses. ***, ** and * represent 0.01, 0.05 and 0.10 significance levels, respectively. The critical values defined by Otero and Smith (2012) were used for the ADFmax test. The Otero and Smith (2017) critical values were used for the KSS test. |
After no statistically significant interaction was found as a result of Bayesian-VAR analysis, we carried out the Toda-Yamamoto (1995) causality test to investigate the short-term dynamics between the series. For the Toda-Yamamoto (1995) test, the optimum lag length (k) was accepted as 1, similar to the Bayesian-VAR system. Considering that all series are I(1), the system equation was estimated with k + dmax = 2. The calculated Wald statistics for the coefficients obtained from the seemingly unrelated regression model are reported in Table 6. When we examine the Wald test results in Table 6, we can see that there is no causality relationship from LFM1 (p > 0.05) and LEM1 to LBTC (p > 0.05) in the short run. On the other hand, according to the model result whereby causality was investigated from LJM1 to LBTC, we found a statistically significant causality in the short term at a significance level of 0.05.
Direction of causality | Wald Test | df | Prob. |
LFM1GC?→LBTC | 1.3504 | 2 | 0.5090 |
LEM1GC?→LBTC | 1.8757 | 2 | 0.3915 |
LJM1GC?→LBTC | 7.7732 | 2 | 0.0205 |
Regarding structural changes, the results of the robust full-sample time-varying Granger causality analysis are given in Table 7. With reference to Table 7, the null hypothesis, which states that there is no causality toward LBTC from the LFM1 is rejected according to the Wald tests with the FE, RO and RE procedures. On the other hand, the Granger causality relationship from the LEM1 and LJM1 series to LBTC is rejected according to the FE window; It is accepted in the 95th and 99th percentile according to the RE and RO procedures.
Direction of causality | Max_Wald FE | Max_Wald RO | Max_Wald RE |
LFM1GC?→LBTC | 13.482 (7.202) [9.517] |
31.936 (7.794) [9.603] |
35.625 (8.420) [9.903] |
LEM1GC?→LBTC | 4.666 (8.181) [9.235] |
9.307 (9.063) [11.167] |
11.964 (9.555) [12.049] |
LJM1GC?→LBTC | 9.353 (7.687) [9.847] |
20.138 (8.372) [9.677] |
20.138 (8.767) [9.915] |
The 95th and 99th percentiles of the bootstrap statistics distribution are shown in parenthesis and brackets, respectively. The minimum window size was set at 30 observations (20% of the number of available observations was used). The number of lags was set at p=1, and the number of lags in the lag-augmented part was set at d=1. The Wald statistics were calculated using a heteroscedastic-robust specification of the variance-covariance matrix. |
In order to explore how causal relationships change over time, we can graphically examine a set of Wald statistics taken from the three procedures. Figures 4–6 show the time-varying Granger causality test results. While the red line denotes the maximum range of Wald statistics, the long and short intermittent lines in the figures show the critical values obtained from the bootstrap method at the 5% and 10% significance levels, respectively. When the test statistic series exceed the critical values, the null hypothesis stating that there is no causality is rejected and it is decided that there is causality.
The findings of the FE, RO and RE Wald tests, which show the period or periods in which there is an interaction between the price of Bitcoin and the Fed's money supply, are presented in Figure 4. According to the FE window, while there was no causality from LFM1 to LBTC until 2020, we detected a strong causality after mid-2020. According to the RO window WALD test, no causality could be detected from the Fed's money supply to Bitcoin prices except for certain periods. Excluded periods include mid-2016 and between 2018M4 and 2019M4. RE window Wald test results are similar to RO test in terms of the period when causality began. On the other hand, it is consistent with the FE test as of the period when causality continues. At the end of 2015, the Fed policymakers slowed down the interest rate increase, and this process continued until the end of 2016, causing the money to shift to different yield instruments, such as BTC. The excess liquidity that emerged as a result of the aid and subsidies made after the COVID-19 epidemic shifted to investment instruments such as the stock market and cryptocurrencies.
Figure 5 shows the test statistical sequences and their corresponding 10% and 5% critical values for forward, rolling and recursive expanding procedures for causality from the European Central Bank money supply to Bitcoin prices. All three test procedures produced similar results, with LEM1 not exhibiting a Granger cause of LBTC. However, according to the RE procedure, it can be said that there is weak causality for the first and last months of 2018 and the first half of 2019.
Finally, Figure 6 presents the Granger causality change points identified by the three algorithms between the central bank of Japan's money supply and Bitcoin prices. For the FE procedure, there is not Granger causality between LJM1 and LBTC. On the other hand, for the RO and RE procedures, there are three similar periods of causality running from LJM1 to LBTC. Period Ⅰ started in March 2013 and ended in mid-2013. With the election of the new government in Japan in September 2012, the scale of expansionary fiscal and monetary policy was further increased. In this way, the desired growth rates were achieved in the first quarter of 2013. Due to the tax increases implemented after the success achieved, the economy again entered into a depression trend (Eğilmez, 2014). Period Ⅱ started in the mid-2019 and continued until the end of that year. Finally, Period Ⅲ indicates strong causality in January 2023. Japan continued to pursue its ultra-expansive economic policies in 2023, which it has pursued for many years. In Japan, where there are negative real interest rates, inflationary effects started to be seen after many years due to the increase in raw material and energy prices.
The nominal value and popularity of Bitcoin, which is claimed to have emerged as a reaction to the bailout packages of governments and national currencies after the 2008 crisis, have increased since the first day. In recent years, crypto money, which is one of the best return tools for investors aiming to protect themselves from inflationary effects and gain returns, has also found a place in the academic literature. Many studies in this field have investigated how the value of cryptocurrencies, especially Bitcoin, interacts with other economic indicators. To contribute to the literature, the effects of national money supplies of selected countries on Bitcoin prices were investigated in this study.
The findings from the Bayesian-VAR and traditional Granger causality test show that there is no interaction between money supply and Bitcoin prices for the USA and eurozone, but there is such interaction for Japan. Sub-sample causality test results between the indicators revealed a causal relationship from money supply to Bitcoin prices for the USA and Japan. According to the findings from the USA money supply, the existence of Granger causality toward Bitcoin prices was determined during certain periods, namely, mid-2016, 2018M4–2019M4 and after mid-2020. The abundant money supply resulting from the financial support packages after the COVID-19 health crisis can be shown as the reason that the causal link was not broken in 2020 and later. The results of the sub-sample causality test, which was investigated from the eurozone money supply to Bitcoin prices, showed weak causality for the first and last months of 2018 and the first half of 2019. The time-varying Granger causality relationship was determined from the Japanese economy money supply series to Bitcoin prices from March 2013 to mid-2013, mid-to-end 2019 and January 2023.
As a result, when the results of analysis were evaluated as a whole in the context of the analyzed data and countries, a nonlinear causality relationship was determined between the money supply and Bitcoin prices. This causal relationship has emerged stronger for the USA, especially after the COVID-19 crisis, owing to the rapid increase in the amount of money put into circulation. These findings confirm the philosophy of Bitcoin's emergence and the possibility that it can be a hedge against the inflationary effects of money, especially after the COVID-19 pandemic.
All authors declare no conflict of interest that could affect the publication of this paper.
[1] | Acemoglu D, Johnson S, Querubin P (2008) When does policy reform work? The case of central bank independence. Working Paper 14033. National Bureau of Economic Research. https://doi.org/10.3386/w14033 |
[2] |
Alesina A, Summers LH (1993) Central bank independence and macroeconomic performance: some comparative evidence. J Money Credit Bank 25: 151–162. https://doi.org/10.2307/2077833 doi: 10.2307/2077833
![]() |
[3] | Arnone M, Laurens B, Segalotto JF, et al. (2007) Central bank autonomy: lessons from global trends. https://doi.org/10.2139/ssrn.986816 |
[4] |
Balcilar M, Ozdemir ZA, Arslanturk Y (2010) Economic growth and energy consumption causal nexus viewed through a bootstrap rolling window. Energy Econ 32: 1398–1410. https://doi.org/10.1016/j.eneco.2010.05.015 doi: 10.1016/j.eneco.2010.05.015
![]() |
[5] | Bank of Japan (BOJ) M1 and Components. Available from: https://www.stat-search.boj.or.jp/ssi/cgi-bin/famecgi2?cgi = $nme_a000_en & lstSelection = MD02. |
[6] |
Benigno P, Schilling LM, Uhlig H (2022) Cryptocurrencies, currency competition, and the impossible trinity. J Int Econ 136: 103601. https://doi.org/10.1016/j.jinteco.2022.103601 doi: 10.1016/j.jinteco.2022.103601
![]() |
[7] |
Blau BM, Griffith TG, Whitby RJ (2021) Inflation and Bitcoin: A descriptive time-series analysis. Econ Lett 203: 109848. https://doi.org/10.1016/j.econlet.2021.109848 doi: 10.1016/j.econlet.2021.109848
![]() |
[8] |
Bouri E, Molnár P, Azzi G, et al. (2017a) On the hedge and safe haven properties of Bitcoin: Is it really more than a diversifier? Financ Res Lett 20: 192–198. https://doi.org/10.1016/j.frl.2016.09.025 doi: 10.1016/j.frl.2016.09.025
![]() |
[9] |
Bouri E, Gupta R, Tiwari AK, et al. (2017b) Does Bitcoin hedge global uncertainty? Evidence from wavelet-based quantile-in-quantile regressions. Financ Res Lett 23: 87–95. https://doi.org/10.1016/j.frl.2017.02.009 doi: 10.1016/j.frl.2017.02.009
![]() |
[10] | Chohan UW (2021) Cryptocurrencies and Hyperinflation. Critical Blockchain Research Initiative (CBRI) Working Papers. https://dx.doi.org/10.2139/ssrn.3320702 |
[11] |
Conlon T, Corbet S, Mcgee RJ (2021) Inflation and cryptocurrencies revisited: A time-scale analysis. Econ Lett 206: 109996. https://doi.org/10.1016/j.econlet.2021.109996 doi: 10.1016/j.econlet.2021.109996
![]() |
[12] | Cukierman A, Kalaitzidakis P, Summers LH, et al. (1993) Central bank independence, growth, investment, and real rates, In: Carnegie-Rochester Conference Series on Public Policy, North-Holland, 39: 95–140. https://doi.org/10.1016/0167-2231(93)90005-H |
[13] | De Haan J, Eijffinger SC (2016) The Politics of Central Bank Independence. De Nederlandsche Bank Working Paper No. 539. Available at SSRN. http://doi.org/10.2139/ssrn.2888836 |
[14] |
Dickey DA, Fuller WA (1981) Likelihood ratio statistics for autoregressive time series with a unit root. Econometrica: J Econometric Society, 1057–1072. https://doi.org/10.2307/1912517 doi: 10.2307/1912517
![]() |
[15] |
Dolado JJ, Lütkepohl H (1996) Making Wald Tests Work for Cointegrated VAR Systems. Econometric Rev 15: 369–386. https://doi.org/10.1080/07474939608800362 doi: 10.1080/07474939608800362
![]() |
[16] |
Dyhrberg AH (2016) Hedging capabilities of bitcoin. Is it the virtual gold? Financ Res Lett 16: 139–144. https://doi.org/10.1016/j.frl.2015.10.025 doi: 10.1016/j.frl.2015.10.025
![]() |
[17] | Eğilmez M (2014) Japonya Slumpflasyona Girdi. Kendime Yazılar. Available from: https://www.mahfiegilmez.com/2014/11/japonya-slumpflasyona-girdi.html?m = 1. |
[18] | European Central Bank (ECB) M1 and Components Available from: https://www.ecb.europa.eu/stats/money_credit_banking/monetary_aggregates/html/index.en.html. |
[19] |
Fang L, Bouri E, Gupta R, et al. (2019) Does global economic uncertainty matter for the volatility and hedging effectiveness of Bitcoin? Int Rev Financ Anal 61: 29–36. https://doi.org/10.1016/j.irfa.2018.12.010 doi: 10.1016/j.irfa.2018.12.010
![]() |
[20] | Federal Reserve (FED) M1 and Components. Available from: https://fred.stlouisfed.org/series/M1SL. |
[21] | Gökalp N (2003) Ekonomide güven faktörü. Yönetim ve Ekonomi Dergisi 10: 163–174. |
[22] | Greco TH (2009) The End of Money and the Future of Civilization. Vermont: Chelsea Green. ISBN: 978-1-60358-078-6. |
[23] | Haşlak Ş (2018) Analysis of Bitcoin market volatility. Published master's thesis. Çankaya University, Institute of Social Sciences, Ankara. |
[24] | Insight Investment (2020) Investment Reports: Covid-19 is Likely to End the Inflation Lockdown. |
[25] |
Kapetanios G, Shin Y, Snell A (2003) Testing for a unit root in the nonlinear STAR framework. J Econometrics 112: 359–379. https://doi.org/10.1016/S0304-4076(02)00202-6 doi: 10.1016/S0304-4076(02)00202-6
![]() |
[26] | Kendirkiran G (2021) The relationship between economic growth and foreign trade in Turkey: Time-varying causality approaches. Master's thesis, Ankara Hacı Bayram Veli University, The Institute of Graduate Studies. |
[27] |
Kostakis V, Giotitsas C (2014) The (A) political economy of bitcoin. Triple C: Communication, Capitalism Critique 12: 431–440. https://doi.org/10.31269/triplec.v12i2.606 doi: 10.31269/triplec.v12i2.606
![]() |
[28] |
Leybourne SJ (1995) Testing for unit roots using forward and reverse Dickey-Fuller regressions. Oxford B Econ Stat 57: 559–571. https://doi.org/10.1111/j.1468-0084.1995.tb00040.x doi: 10.1111/j.1468-0084.1995.tb00040.x
![]() |
[29] | Litterman RB (1980) Bayesian procedure for forecasting with vector autoregressions. Massachusetts Institute of Technology. https://doi.org/10.21034/wp.115 |
[30] |
Litterman RB (1986) Forecasting with Bayesian vector autoregressions—five years of experience. J Bus Econ Stat 4: 25–38. https://doi.org/10.1080/07350015.1986.10509491 doi: 10.1080/07350015.1986.10509491
![]() |
[31] |
Ma C, Tian Y, Hsiao S, et al. (2022) Monetary policy shocks and Bitcoin prices. Res Int Bus Financ 62: 101711. https://doi.org/10.1016/j.ribaf.2022.101711 doi: 10.1016/j.ribaf.2022.101711
![]() |
[32] | Nakamoto S (2008) Bitcoin: A peer-to-peer electronic cash system. Available from: https://bitcoin.org/bitcoin. |
[33] |
Narayan PK, Narayan S, Rahman RE, et al. (2019) Bitcoin price growth and Indonesia's monetary system. Emerg Mark Rev 38: 364–376. https://doi.org/10.1016/j.ememar.2018.11.005 doi: 10.1016/j.ememar.2018.11.005
![]() |
[34] |
Otero J, Smith J (2012) Response surface models for the Leybourne unit root tests and lag order dependence. Comput Stat 27: 473–486. https://doi.org/10.1007/s00180-011-0268-y doi: 10.1007/s00180-011-0268-y
![]() |
[35] |
Otero J, Smith J (2017) Response surface models for OLS and GLS detrending-based unit-root tests in nonlinear ESTAR models. Stata J 17: 704–722. https://doi.org/10.1177/1536867X1701700310 doi: 10.1177/1536867X1701700310
![]() |
[36] |
Perez C (2009) The double bubble at the turn of the century: technological roots and structural implications. Camb J Econ 33: 779–805. https://dx.doi.org/10.1093/cje/bep028 doi: 10.1093/cje/bep028
![]() |
[37] |
Phillips PC, Shi S, Yu J (2015a) Testing for multiple bubbles: historical episodes of exuberance and collapse in the S & P 500. Int Econ Rev 56: 1043–1078. https://doi.org/10.1111/iere.12132 doi: 10.1111/iere.12132
![]() |
[38] |
Phillips PC, Shi S, Yu J (2015b) Testing for multiple bubbles: limit theory of real-time detectors Int Econ Rev 56: 1079–1134. https://doi.org/10.1111/iere.12131 doi: 10.1111/iere.12131
![]() |
[39] | Pirgaip B, Dinçergök B, Haşlak Ş (2019) Bitcoin Market Price Analysis and an Empirical Comparison with Main Currencies, Commodities, Securities and Altcoins, In: Hacioglu, U. (eds) Blockchain Economics and Financial Market Innovation, Contributions to Economics. https://doi.org/10.1007/978-3-030-25275-5_8 |
[40] | Savaş VF (2016) Politik İktisat (8. baskı). Beta Yayınları, İstanbul. |
[41] |
Schilling L, Uhlig H (2019a) Some simple bitcoin economics. J Monetary Econ 106: 16–26. https://doi.org/10.1016/j.jmoneco.2019.07.002 doi: 10.1016/j.jmoneco.2019.07.002
![]() |
[42] | Schilling LM, Uhlig H (2019b) Currency substitution under transaction costs, In: AEA Papers and Proceedings, 109: 83–87. https://doi.org/10.1257/pandp.20191017 |
[43] |
Shi S, Hurn S, Phillips PC (2020) Causal change detection in possibly integrated systems: Revisiting the money–income relationship. J Financ Econometrics 18: 158–180. https://doi.org/10.1093/jjfinec/nbz004 doi: 10.1093/jjfinec/nbz004
![]() |
[44] |
Shi S, Phillips PC, Hurn S (2018) Change detection and the causal impact of the yield curve. J Time Series Anal 39: 966–987. https://doi.org/10.1111/jtsa.12427 doi: 10.1111/jtsa.12427
![]() |
[45] | StataCorp (2021) Stata: Release 17. Statistical Software. College Station, TX: StataCorp LLC. |
[46] |
Su CW, Qin M, Tao R, et al. (2020) Can Bitcoin hedge the risks of geopolitical events? Technol Forecast Social Change 159: 120182. https://doi.org/10.1016/j.techfore.2020.120182 doi: 10.1016/j.techfore.2020.120182
![]() |
[47] |
Swanson NR (1998) Money and output viewed through a rolling window. J Monetary Econ 41: 455–474. https://doi.org/10.1016/S0304-3932(98)00005-1 doi: 10.1016/S0304-3932(98)00005-1
![]() |
[48] |
Thoma MA (1994) Subsample Instability and Asymmetries in Money-Income Causality. J Econometrics 64: 279–306. https://doi.org/10.1016/0304-4076(94)90066-3 doi: 10.1016/0304-4076(94)90066-3
![]() |
[49] |
Urquhart A, Zhang H (2019) Is Bitcoin a hedge or safe haven for currencies? An intraday analysis. Int Rev Financ Anal 63: 49–57. https://doi.org/10.1016/j.irfa.2019.02.009 doi: 10.1016/j.irfa.2019.02.009
![]() |
[50] |
Wang L, Sarker PK, Bouri E (2022) Short-and Long-Term Interactions between Bitcoin and Economic Variables: Evidence from the US. Comput Econ, 1–26. https://doi.org/10.1007/s10614-022-10247-5 doi: 10.1007/s10614-022-10247-5
![]() |
[51] |
Warsito OLD (2020) Analisis Volatilitas Cryptocurrency, Emas, Dollar, Dan Indeks Harga Saham (Ihsg). Int J Social Sci Bus 4: 40–46. https://doi.org/10.23887/ijssb.v4i1.23887 doi: 10.23887/ijssb.v4i1.23887
![]() |
[52] | Weber B (2014) Can Bitcoin compete with money? J Peer Prod 4. |
[53] |
Wu S, Tong M, Yang Z, et al. (2019) Does gold or Bitcoin hedge economic policy uncertainty? Financ Res Lett 31: 171–178. https://doi.org/10.1016/j.frl.2019.04.001 doi: 10.1016/j.frl.2019.04.001
![]() |
[54] | Yahoo Finance. Historical Bitcoin Prices. Available From: https://finance.yahoo.com/quote/BTC-USD?p = BTC-USD & .tsrc = fin-srch. |
[55] |
Toda HY, Yamamoto T (1995) Statistical inference in vector autoregressions with possibly integrated processes. J Econometrics 66: 225–250. https://doi.org/10.1016/0304-4076(94)01616-8 doi: 10.1016/0304-4076(94)01616-8
![]() |
[56] |
Yamada H, Toda HY (1998) Inference in possibly integrated vector autoregressive models: Some finite sample evidence. J Econometrics 86: 55–95. https://doi.org/10.1016/S0304-4076(97)00109-7 doi: 10.1016/S0304-4076(97)00109-7
![]() |
[57] |
Zhu Y, Dickinson D, Li J (2017) Analysis on the influence factors of Bitcoin's price based on VEC model. Financ Innov 3: 1–13. https://doi.org/10.1186/s40854-017-0054-0 doi: 10.1186/s40854-016-0051-8
![]() |
1. | Miloš Grujić, Željko Vojinović, Investing in blockchain technologies and digital assets: Accounting perspectives, 2024, 0350-2120, 35, 10.5937/AnEkSub2300032G | |
2. | Zhenghui Li, Hanzi Chen, Siting Lu, Pierre Failler, How does digital payment affect international trade? Research based on the social network analysis method, 2024, 32, 2688-1594, 1406, 10.3934/era.2024065 | |
3. | Tuğba Baş, Orhan Özaydın, Yahya Can Dura, Does Bitcoin Affect Term Deposits? Evidence from MINT Countries, 2023, 17, 1864-6042, 10.1515/econ-2022-0056 |
BTC | FM1 | EM1 | JM1 | |
Mean | 8759.647 | 6555.911 | 7432.246 | 7124373 |
Median | 951.1000 | 3337.150 | 7094.844 | 6781044 |
Maximum | 61309.60 | 20699.60 | 11714.43 | 10480547 |
Minimum | 0.100000 | 1748.700 | 4663.060 | 4939771 |
Std. Dev. | 14460.71 | 6790.338 | 2247.773 | 1686738 |
Skewness | 2.038437 | 1.356002 | 0.467187 | 0.551846 |
Kurtosis | 6.304107 | 2.930469 | 1.959786 | 2.046827 |
Jarque-Bera | 172.1127 | 45.99874 | 12.21939 | 13.29171 |
Probability | 0.000000 | 0.000000 | 0.002221 | 0.001299 |
Observations | 150 | 150 | 150 | 150 |
Correlation | Probability | |
LFM1 | 0.7633 | 0.0000 |
LEM1 | 0.9131 | 0.0000 |
LJM1 | 0.9087 | 0.0000 |
FPE | AIC | HQIC | SBIC | |
1 | 1.6e-07 | −4.27582 | −4.20686 | 4.10613 |
2 | 2.0e-13 | −17.8646 | −17.6577 | 17.3555 |
3 | 1.1e-13 | −18.5142 | −18.1694* | 17.6657* |
4 | 9.7e-14* | −18.619* | −18.1362 | 17.4311 |
5 | 1.0e-13 | −18.5716 | −17.951 | 17.0443 |
6 | 1.1e-13 | −18.4832 | −17.7246 | 16.6165 |
7 | 1.2e-13 | −18.447 | −17.5505 | −16.241 |
8 | 1.2e-13 | −18.4068 | −17.3724 | 15.8614 |
9 | 1.4e-13 | −18.2919 | −17.1196 | 15.4071 |
10 | 1.4e-13 | −18.2801 | −16.9699 | 15.0559 |
11 | 1.7e-13 | −18.152 | −16.7038 | 14.5883 |
12 | 1.7e-13 | −18.1668 | −16.5807 | 14.2638 |
*: optimal lag. An exogenous dummy variable has been added as of March 2020 to show the effect of the COVID-19 outbreak. FPE: Final Prediction Error, AIC: Akaike Information Criterion, HQIC: Hannan-Quin Information Criterion, SBIC: Schwarz Information Criterion. |
Models | Log (ML) | P(M) | P(M|y) |
1 | 1232.9545 | 0.1000 | 0.7540 |
2 | 1231.8244 | 0.1000 | 0.2460 |
3 | 1221.1161 | 0.1000 | 0.0000 |
4 | 1212.1161 | 0.1000 | 0.0000 |
5 | 1198.3390 | 0.1000 | 0.0000 |
LBTC | LFM1 | LEM1 | LJM1 | |||
Level | ADFmax | Constant | 1.065 (9) | −0.061 (0) | −2.194 (12) | 1.032 (1) |
Constant & Trend | −1.436 (9) | −1.600 (0) | −1.317 (12) | −1.340 (1) | ||
KSS | Constant | −1.056 (9) | 0.227 (0) | −3.273 (12)** | 0.485 (6) | |
Constant & Trend | −3.339 (9)** | −1.609 (0) | −2.305 (12) | −1.602 (1) | ||
First Differences | ADFmax | Constant | −9.017 (0)*** | −10.528 (0)*** | −3.179 (4)*** | −5.793 (0)*** |
Constant & Trend | −9.161 (0)*** | −10.541 (0)*** | −2.907 (4)* | −5.963 (0)*** | ||
KSS | Constant | −3.926 (7)*** | −12.482 (0)*** | −3.266 (1)** | −4.549 (4)*** | |
Constant & Trend | −3.847 (7)*** | −12.488 (0)*** | −3.309 (1)* | −4.758 (4)*** | ||
The optimal lags determined according to the Akaike information criterion are in parentheses. ***, ** and * represent 0.01, 0.05 and 0.10 significance levels, respectively. The critical values defined by Otero and Smith (2012) were used for the ADFmax test. The Otero and Smith (2017) critical values were used for the KSS test. |
Direction of causality | Wald Test | df | Prob. |
LFM1GC?→LBTC | 1.3504 | 2 | 0.5090 |
LEM1GC?→LBTC | 1.8757 | 2 | 0.3915 |
LJM1GC?→LBTC | 7.7732 | 2 | 0.0205 |
Direction of causality | Max_Wald FE | Max_Wald RO | Max_Wald RE |
LFM1GC?→LBTC | 13.482 (7.202) [9.517] |
31.936 (7.794) [9.603] |
35.625 (8.420) [9.903] |
LEM1GC?→LBTC | 4.666 (8.181) [9.235] |
9.307 (9.063) [11.167] |
11.964 (9.555) [12.049] |
LJM1GC?→LBTC | 9.353 (7.687) [9.847] |
20.138 (8.372) [9.677] |
20.138 (8.767) [9.915] |
The 95th and 99th percentiles of the bootstrap statistics distribution are shown in parenthesis and brackets, respectively. The minimum window size was set at 30 observations (20% of the number of available observations was used). The number of lags was set at p=1, and the number of lags in the lag-augmented part was set at d=1. The Wald statistics were calculated using a heteroscedastic-robust specification of the variance-covariance matrix. |
BTC | FM1 | EM1 | JM1 | |
Mean | 8759.647 | 6555.911 | 7432.246 | 7124373 |
Median | 951.1000 | 3337.150 | 7094.844 | 6781044 |
Maximum | 61309.60 | 20699.60 | 11714.43 | 10480547 |
Minimum | 0.100000 | 1748.700 | 4663.060 | 4939771 |
Std. Dev. | 14460.71 | 6790.338 | 2247.773 | 1686738 |
Skewness | 2.038437 | 1.356002 | 0.467187 | 0.551846 |
Kurtosis | 6.304107 | 2.930469 | 1.959786 | 2.046827 |
Jarque-Bera | 172.1127 | 45.99874 | 12.21939 | 13.29171 |
Probability | 0.000000 | 0.000000 | 0.002221 | 0.001299 |
Observations | 150 | 150 | 150 | 150 |
Correlation | Probability | |
LFM1 | 0.7633 | 0.0000 |
LEM1 | 0.9131 | 0.0000 |
LJM1 | 0.9087 | 0.0000 |
FPE | AIC | HQIC | SBIC | |
1 | 1.6e-07 | −4.27582 | −4.20686 | 4.10613 |
2 | 2.0e-13 | −17.8646 | −17.6577 | 17.3555 |
3 | 1.1e-13 | −18.5142 | −18.1694* | 17.6657* |
4 | 9.7e-14* | −18.619* | −18.1362 | 17.4311 |
5 | 1.0e-13 | −18.5716 | −17.951 | 17.0443 |
6 | 1.1e-13 | −18.4832 | −17.7246 | 16.6165 |
7 | 1.2e-13 | −18.447 | −17.5505 | −16.241 |
8 | 1.2e-13 | −18.4068 | −17.3724 | 15.8614 |
9 | 1.4e-13 | −18.2919 | −17.1196 | 15.4071 |
10 | 1.4e-13 | −18.2801 | −16.9699 | 15.0559 |
11 | 1.7e-13 | −18.152 | −16.7038 | 14.5883 |
12 | 1.7e-13 | −18.1668 | −16.5807 | 14.2638 |
*: optimal lag. An exogenous dummy variable has been added as of March 2020 to show the effect of the COVID-19 outbreak. FPE: Final Prediction Error, AIC: Akaike Information Criterion, HQIC: Hannan-Quin Information Criterion, SBIC: Schwarz Information Criterion. |
Models | Log (ML) | P(M) | P(M|y) |
1 | 1232.9545 | 0.1000 | 0.7540 |
2 | 1231.8244 | 0.1000 | 0.2460 |
3 | 1221.1161 | 0.1000 | 0.0000 |
4 | 1212.1161 | 0.1000 | 0.0000 |
5 | 1198.3390 | 0.1000 | 0.0000 |
LBTC | LFM1 | LEM1 | LJM1 | |||
Level | ADFmax | Constant | 1.065 (9) | −0.061 (0) | −2.194 (12) | 1.032 (1) |
Constant & Trend | −1.436 (9) | −1.600 (0) | −1.317 (12) | −1.340 (1) | ||
KSS | Constant | −1.056 (9) | 0.227 (0) | −3.273 (12)** | 0.485 (6) | |
Constant & Trend | −3.339 (9)** | −1.609 (0) | −2.305 (12) | −1.602 (1) | ||
First Differences | ADFmax | Constant | −9.017 (0)*** | −10.528 (0)*** | −3.179 (4)*** | −5.793 (0)*** |
Constant & Trend | −9.161 (0)*** | −10.541 (0)*** | −2.907 (4)* | −5.963 (0)*** | ||
KSS | Constant | −3.926 (7)*** | −12.482 (0)*** | −3.266 (1)** | −4.549 (4)*** | |
Constant & Trend | −3.847 (7)*** | −12.488 (0)*** | −3.309 (1)* | −4.758 (4)*** | ||
The optimal lags determined according to the Akaike information criterion are in parentheses. ***, ** and * represent 0.01, 0.05 and 0.10 significance levels, respectively. The critical values defined by Otero and Smith (2012) were used for the ADFmax test. The Otero and Smith (2017) critical values were used for the KSS test. |
Direction of causality | Wald Test | df | Prob. |
LFM1GC?→LBTC | 1.3504 | 2 | 0.5090 |
LEM1GC?→LBTC | 1.8757 | 2 | 0.3915 |
LJM1GC?→LBTC | 7.7732 | 2 | 0.0205 |
Direction of causality | Max_Wald FE | Max_Wald RO | Max_Wald RE |
LFM1GC?→LBTC | 13.482 (7.202) [9.517] |
31.936 (7.794) [9.603] |
35.625 (8.420) [9.903] |
LEM1GC?→LBTC | 4.666 (8.181) [9.235] |
9.307 (9.063) [11.167] |
11.964 (9.555) [12.049] |
LJM1GC?→LBTC | 9.353 (7.687) [9.847] |
20.138 (8.372) [9.677] |
20.138 (8.767) [9.915] |
The 95th and 99th percentiles of the bootstrap statistics distribution are shown in parenthesis and brackets, respectively. The minimum window size was set at 30 observations (20% of the number of available observations was used). The number of lags was set at p=1, and the number of lags in the lag-augmented part was set at d=1. The Wald statistics were calculated using a heteroscedastic-robust specification of the variance-covariance matrix. |