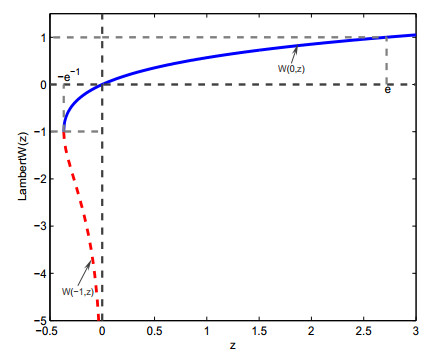
In this paper, we establish an integrated pest management Filippov model with group defense of pests and a constant rate release of natural enemies. First, the dynamics of the subsystems in the Filippov system are analyzed. Second, the dynamics of the sliding mode system and the types of equilibria of the Filippov system are discussed. Then the complex dynamics of the Filippov system are investigated by using numerical analysis when there is a globally asymptotically stable limit cycle and a globally asymptotically stable equilibrium in two subsystems, respectively. Furthermore, we analyze the existence region of a sliding mode and pseudo equilibrium, as well as the complex dynamics of the Filippov system, such as boundary equilibrium bifurcation, the grazing bifurcation, the buckling bifurcation and the crossing bifurcation. These complex sliding bifurcations reveal that the selection of key parameters can control the population density no more than the economic threshold, so as to prevent the outbreak of pests.
Citation: Baolin Kang, Xiang Hou, Bing Liu. Threshold control strategy for a Filippov model with group defense of pests and a constant-rate release of natural enemies[J]. Mathematical Biosciences and Engineering, 2023, 20(7): 12076-12092. doi: 10.3934/mbe.2023537
[1] | Hao Zhou, Xia Wang, Sanyi Tang . Global dynamics of non-smooth Filippov pest-natural enemy system with constant releasing rate. Mathematical Biosciences and Engineering, 2019, 16(6): 7327-7361. doi: 10.3934/mbe.2019366 |
[2] | Yi Yang, Lirong Liu, Changcheng Xiang, Wenjie Qin . Switching dynamics analysis of forest-pest model describing effects of external periodic disturbance. Mathematical Biosciences and Engineering, 2020, 17(4): 4328-4347. doi: 10.3934/mbe.2020239 |
[3] | Aili Wang, Yanni Xiao, Huaiping Zhu . Dynamics of a Filippov epidemic model with limited hospital beds. Mathematical Biosciences and Engineering, 2018, 15(3): 739-764. doi: 10.3934/mbe.2018033 |
[4] | Guodong Li, Wenjie Li, Ying Zhang, Yajuan Guan . Sliding dynamics and bifurcations of a human influenza system under logistic source and broken line control strategy. Mathematical Biosciences and Engineering, 2023, 20(4): 6800-6837. doi: 10.3934/mbe.2023293 |
[5] | Dan Shi, Shuo Ma, Qimin Zhang . Sliding mode dynamics and optimal control for HIV model. Mathematical Biosciences and Engineering, 2023, 20(4): 7273-7297. doi: 10.3934/mbe.2023315 |
[6] | Yuan Tian, Sanyi Tang . Dynamics of a density-dependent predator-prey biological system with nonlinear impulsive control. Mathematical Biosciences and Engineering, 2021, 18(6): 7318-7343. doi: 10.3934/mbe.2021362 |
[7] | Bing Liu, Gang Hu, Baolin Kang, Xin Huang . Analysis of a hybrid pest management model incorporating pest resistance and different control strategies. Mathematical Biosciences and Engineering, 2020, 17(5): 4364-4383. doi: 10.3934/mbe.2020241 |
[8] | Liping Wu, Zhongyi Xiang . A study of integrated pest management models with instantaneous and non-instantaneous impulse effects. Mathematical Biosciences and Engineering, 2024, 21(2): 3063-3094. doi: 10.3934/mbe.2024136 |
[9] | Yansong Pei, Bing Liu, Haokun Qi . Extinction and stationary distribution of stochastic predator-prey model with group defense behavior. Mathematical Biosciences and Engineering, 2022, 19(12): 13062-13078. doi: 10.3934/mbe.2022610 |
[10] | Wenjie Qin, Yue Xia, Yi Yang . An eco-epidemic model for assessing the application of integrated pest management strategies. Mathematical Biosciences and Engineering, 2023, 20(9): 16506-16527. doi: 10.3934/mbe.2023736 |
In this paper, we establish an integrated pest management Filippov model with group defense of pests and a constant rate release of natural enemies. First, the dynamics of the subsystems in the Filippov system are analyzed. Second, the dynamics of the sliding mode system and the types of equilibria of the Filippov system are discussed. Then the complex dynamics of the Filippov system are investigated by using numerical analysis when there is a globally asymptotically stable limit cycle and a globally asymptotically stable equilibrium in two subsystems, respectively. Furthermore, we analyze the existence region of a sliding mode and pseudo equilibrium, as well as the complex dynamics of the Filippov system, such as boundary equilibrium bifurcation, the grazing bifurcation, the buckling bifurcation and the crossing bifurcation. These complex sliding bifurcations reveal that the selection of key parameters can control the population density no more than the economic threshold, so as to prevent the outbreak of pests.
Since ancient times, the struggle between human beings and pests has never been interrupted, which has brought serious impacts on agricultural production, so pest control has always been a hot issue. Particularly, rice planthoppers, armyworms, aphids, cotton bollworms, meadow moths, corn borers, tea silkworms and locusts have become a common and widespread problem in recent years [1,2], with part of them exhibiting group defense. Group defence is a term used to describe the phenomenon whereby predation is decreased, or even prevented altogether, due to the increased ability of the prey to better defend or disguise themselves when their density is large enough [3,4]. This phenomenon described by Holmes and Bethel involves certain insect populations [5]. For example, large swarms of the locusts can release a lot of toxic substances, which helps them escape natural enemies, and then the locusts can reproduce in large numbers, causing pest population outbreaks, which are extremely destructive to crops. In early 2020, the worst outbreak of desert locusts in 70 years destroyed tens of thousands of hectares of farmland in East Africa, posing a huge threat to global food security [6,7]. Obviously, the ability of the group defense of pests has brought a great challenge to pest control.
When controlling pests, it is necessary to take into account integrated pest management strategies [8,9,10,11,12] in order to reduce the density of a pest population to a tolerable level, which involves choosing appropriate measures such as spraying pesticides and releasing natural enemies. One of the main purposes of an integrated pest management strategy is to take control measures when the pest population density exceeds the economic threshold (ET) [13,14] so as to prevent the pest population density from reaching the economic injury level [14,15]. Therefore, a semi-continuous differential dynamic system, namely, a differential dynamic system with impulsive state feedback control has been proposed [16,17,18,19,20]. This can not only avoid unnecessary environmental pollution and resource waste caused by the abuse of pesticides and release of natural enemies, but it also protects the diversity and stability of the ecosystem. The basic premise of the above model is that the control measures are completed in an instant. However, in the practical application process, once the pest population density exceeds the ET, people will continue to use pesticides for a period of time or continuously release natural enemies to make the pest population density equal to the ET or fall below the ET; otherwise, no control measures will be taken. This control strategy is called the threshold control strategy or the switching strategy, which is characterized by the switched Filippov differential dynamical system [21,22,23,24]. In recent years, piecewise smooth Filippov systems have been widely used in many fields, such as control engineering, biology, medicine, physics and economics [25,26,27,28,29,30,31,32,33,34].
Based on the predator-prey model with group defense proposed by Xiao et al. [35], Hou et al. [36] established and studied a Filippov system to simulate the process of integrated pest management in which pest populations had group defense behaviors and natural enemies were released at a linear rate. The results showed that, although there were no closed orbits in the subsystem, a stable periodic solution may exist for a Filippov system after switching-induced perturbation. The linear release rate of natural enemies in [36] requires us to monitor the density of natural enemies to control pests. However, in the real world, it is difficult to do that. In this paper, we study an integrated pest management Filippov model with group defense and a constant-rate release of natural enemies to simulate the pest control process. Our main purpose of this paper is to study the effects of a threshold control strategy in a Filippov system on the dynamics of subsystems and the pest control.
In the following section, a Filippov pest control model with group defense behavior is proposed. Then, relevant definitions and preliminaries of the Filippov system are given in Section 3. By using Filippov's theory and numerical simulations, the comprehensive dynamics of the Filippov system are analyzed in Section 4. A brief discussion is given in the last section.
Based on the model proposed by Xiao et al. [35], it is assumed that the pest population exhibits group defense behavior against natural enemies, which is described by the following model:
{dx(t)dt=rx(t)[1−x(t)K]−αx(t)y(t)e−βx(t).=F11,dy(t)dt=y(t)[kαx(t)e−βx(t)−d].=F12. | (2.1) |
Once the pest population density exceeds the ET, the integrated pest management strategy is implemented, that is, a constant rate of natural enemies and insecticide spraying is applied at the same time; otherwise no intervention measures will be taken. Therefore, when x(t)>ET, the following model is established.
{dx(t)dt=rx(t)[1−x(t)K]−αx(t)y(t)e−βx(t)−p1x(t).=F21,dy(t)dt=y(t)[kαx(t)e−βx(t)−d]−p2y(t)+τ.=F22, | (2.2) |
where x(t) and y(t) represent the densities of the pest and natural enemy populations at time t, respectively; K>0 is the carrying capacity of the pest population and r>0 represents its intrinsic growth rate; αx(t)e−βx(t)(α>0,β>0) represents the predation rate of natural enemies against pests with group defense behavior, which is a non-monotonic saturating response function; d>0 represents the death rate of natural enemies; k>0 represents the conversion rate of natural enemies preying on pests; p1>0 and p2>0 represent the reduction rates of the densities of pests and natural enemies due to the effects of pesticides, respectively; τ>0 represents the constant release rate of natural enemies, and as time passes, natural enemies are continuously and linearly released. The constant τ represents the degree of control of pests. In other words, the larger the constant, the more significant the reduction in the pest population through the release of natural enemies. Therefore, by studying and adjusting this constant, we can better understand and optimize pest management strategies to improve their effectiveness and sustainability. It is assumed that the effect of pesticides on natural enemies is ignored, so the value of p2 is approximately 0.
Then, models (2.1) and (2.2) can be rewritten as the following generalized non-smooth Filippov system [22,24]:
dZdt={FM1(Z),Z∈M1,FM2(Z),Z∈M2, | (2.3) |
where Z=(x,y)T,FM1=(F11,F12)T,FM2=(F21,F22)T,M1={Z∈R2+|H(Z)<0},M2={Z∈R2+|H(Z)>0},H(Z)=x(t)−ET and R2+={Z=(x,y)T|x≥0,y≥0}. Note that Σ={(x,y)∈R2+|H(Z)=0} represents the dividing line of M1 and M2.
First, we give the sliding mode of the Filippov system (2.3), which can be defined as
Σs={Z∈Σ|⟨HZ,FM1(Z)⟩⟨HZ,FM2(Z)⟩<0}, |
where ⟨⋅⟩ represents the standard scalar product, H(Z) is a smooth scalar function and the gradient of H(Z) on Σ is HZ=(1,0). Note that a sliding mode is stable for ⟨HZ,FM1(Z)⟩>0 and ⟨HZ,FM2(Z)⟩<0; correspondingly, when ⟨HZ,FM1(Z)⟩<0 and ⟨HZ,FM2(Z)⟩>0, the sliding mode is unstable [24]. Obviously, based on the right-hand expressions of system (2.1) and system (2.2), it can be concluded that there is no type of unstable sliding mode. Therefore, we only discuss stable cases.
A sliding mode may occur if there exist subsets Σs of the manifold Σ such that the vectors FM1(Z) and FM2(Z) are directed toward each other. By Filippov's convex theory [24], if the sliding mode is smooth on a one-dimensional sliding interval Σs, then the sliding mode dynamics can be determined by
˙Z(t)=λFM1(Z)+(1−λ)FM2(Z), |
where 0<λ=⟨HZ,FM2(Z)⟩⟨HZ,FM2(Z)−FM1(Z)⟩<1 and Z=(x,y)T∈Σs.
In a Filippov system, there exist different types of equilibria, which are defined as follows:
Definition 1 [24] (1) Let FMi(E)=0(i=1,2); then, a point E is called a regular equilibrium of system (2.3) if it belongs to Mi, and a virtual equilibrium if it belongs to Mj,j≠i, as denoted by Er,Ev, respectively.
(2) A point E is called a pseudo-equilibrium of system (2.3) if it is an equilibrium of the sliding mode Σs, i.e., λFM1(E)+(1−λ)FM2(E)=0,H(E)=0 and 0<λ<1, as denoted by Ep.
(3) A point E is called a tangent point of system (2.3) if E∈Σs,⟨HZ(E),FM1(E)⟩=0 or ⟨HZ(E),FM2(E)⟩=0, as denoted by Et.
(4) A point E is called a boundary equilibrium of system (2.3) if E∈Σs,FM1(E)=0 or FM2(E)=0, as denoted by Eb.
The global sliding bifurcations of a Filippov system can be divided into the following types:
Definition 2 [24] (1) If the standard limit circle of the Filippov system collides with the discontinuity boundary, it is called grazing bifurcation.
(2) If a stable sliding limit cycle of the Filippov system collides with another boundary point of the system, and then contains the entire sliding mode, it is called the buckling bifurcation.
(3) If the sliding limit cycle becomes a crossing limit cycle in the Filippov system with the parameters changing, it is called crossing bifurcation.
The Lambert W function [37] plays an important role in solving the equilibria of subsystem (2.1). Now, we introduce the following definition:
Definition 3 The multivalued inverse of the function z→zez is called the Lambert W function, satisfying
LambertW(z)exp(LambertW(z))=z. |
It can be calculated that the derivative of the Lambert W function with respect to the variable z is
W′(z)=W(z)z(1+W(z)). |
The inverse function of zez is denoted as W(z)≐W(0,z), as defined on the interval [−e−1,+∝) for z∈[−1,+∝); Similarly, the inverse function of zez is denoted as W(z)≐W(−1,z), as defined on the interval [−e−1,0) for z∈[−∝,−1),as shown in Figure 1. For further details about the background and properties of the Lambert W function, see [37].
When x(t)<ET, the subsystem (2.1) has a trivial equilibrium (0,0) and a boundary equilibrium (K,0). According to the definition of the Lambert W function, if eβd<kα, the equation kαx(t)e−βx(t)−d=0 has two positive roots, that is, the subsystem (2.1) has two positive equilibria E11(x11,y11),E12(x12,y12), where
x11=−W[0,−βdkα]β,y11=krdx11(1−x11K),x12=−W[−1,−βdkα]β,y12=krdx12(1−x12K). |
The stability of the equilibria and the existence of limit cycles in subsystem 2.1 are discussed in detail from [36], which will not be elaborated here.
When x(t)>ET, subsystem (2.2) has a boundary equilibrium, that is, the pest extinction equilibrium (0,τd). It is easy to know that the vertical and horizontal isoclines of subsystem (2.2) are y=r(1−xK)−p1αe−βx and y=τd−kαxe−βx, respectively. Combining the two formulas, we know that the positive equilibrium of subsystem (2.2) satisfies the following equation:
rkKx2+(p1−r)kx+(r−rKx−p1)dαeβx−τ=0. | (4.1) |
Lemma 4.1 If τr−p1<dα<kβ, then Eq (4.1) has at least one positive real solution.
Proof. Equation (4.1) can be transformed into
(r−p1−rkx)(dαeβx−kx)=τ, |
and then it is further transformed into Ax+B=1eβx−Cx, where A=−rdταk<0, B=d(r−p1)τα and C=αkd.
We first discuss the existence of a positive real solution of equation eβx−Cx=0. Let g(x)=eβx−Cx; therefore, the derivative of g(x) with respect to x is g′(x)=βeβx−C. From the condition dα<kβ, one can derive the equation g′(x)=0 to have the unique positive real root x=1βlnCβ. Thus we conclude that g′(x)<0 on the interval [0,1βlnCβ), which implies that g(x) is decreasing on [0,1βlnCβ), and that g′(x)>0 on the interval [1βlnCβ,+∞), which implies that g(x) is increasing on [1βlnCβ,+∞). Furthermore, the minimum value of the function g(x) is g(1βlnCβ)=Cβ(1−lnCβ). Thus, the existence of the positive real solution of the equation g(x)=0 can be divided into three cases:
(A1) if 1−lnCβ>0, that is Cβ=αkβd<e, then the equation has no positive roots;
(A2) if 1−lnCβ=0, that is αkβd=e, then the equation has one positive root;
(A3) if 1−lnCβ<0, that is αkβd>e, then the equation has two positive roots.
For convenience, denote h(x)=Ax+B and f(x)=1g(x), whereby the y-intercept of h(x) is B and the y-intercept of the function f(x) is 1. Now, we discuss the intersection of h(x) and f(x) in different cases. For case (A1), f(x) is a continuous function on the interval [0,+∞), and the x axis is the asymptote in the first quadrant. According to τr−p1<dα, we know that B>1. Since A<0, h(x) and f(x) must intersect (see Figure 2(a)). For case (A2), the f(x) has one discontinuity point. For the sake of convenience, we establish that x=α is the vertical asymptote. When 0≤x<α, f′(x)>0, and when x→α−, f(x)→+∞. Thus, if A<0 and B>1, that is, τr−p1<dα<kβ, then h(x) and f(x) must also intersect (see Figure 2(b)). For case A3, we can discuss it in a similar manner as case A2, and we will not elaborate on it here. This completes the proof.
Remark 4.1: As the intercept of function h(x) with the x-axis increases, the number of intersection points between h(x) and f(x) will increase. As shown in Figure 2, it can be seen that there can be, at most, three intersection points.
According to the above analysis, the existence of positive equilibria of subsystem (2.2) has been proved. When the subsystem (2.2) has only one positive equilibrium, we can analyze the stability of this equilibrium via the vector field of subsystem (2.2). As shown in Figure 3, the isolines x′(t)=0 and y′(t)=0 divide the phase plane into four regions: G1, G2, G3 and G4. In the region G1, x′(t)<0 and y′(t)>0; this means that the trajectories move upward to the left and are tangent to y′(t)=0 into region G2. In the region G2, x′(t)<0 and y′(t)<0; this means that the trajectories go down to the left and are tangent to x′(t)=0 into region G3. In the region G3, x′(t)>0 and y′(t)<0; this means that the trajectories move to the right and are tangent to y′(t)=0 into region G4. In the region G4, x′(t)>0 and y′(t)>0; this means that the trajectories move to the upper right and are tangent to x′(t)=0 into region G1 again. According to the direction of the track, as shown in Figure 3, it can be inferred that the trajectories tend to the equilibrium. Therefore, the equilibrium is an asymptotically stable focus.
According to the definition of the sliding mode in the Filippov system, we have
Σs={Z=(x,y)T∈Σ|F11>0,F21<0},={(x,y)T∈Σ|reβET(1−ETK)α−p1eβETα≤y≤reβET(1−ETK)α}. |
For convenience, we denote yc1.=reβET(1−ETK)α−p1eβETα,yc2.=reβET(1−ETK)α. Therefore, the sliding mode of system (2.3) can be denoted as Σs={(x,y)T∈Σ|yc1≤y≤yc2}. From the Filippov convex theory, the dynamics of the sliding mode system are determined by the following equation:
˙y=y(kαETe−βET−d)+r(1−ETK)−αye−βETp1τ.=ψ(y). |
Let ψ(y)=0; we get yQ1=rτ(K−ET)eβETαK(τ−p1kET)+p1dKeβET. If yQ1∈(yc1,yc2), that is
yc1<yQ1=rτ(K−ET)eβETαK(τ−p1kET)+p1dKeβET<yc2, |
then Filippov system (2.3) has a unique pseudo-equilibrium Ep(ET,yQ1). Differentiate ψ(y) with respect to y; then, we have
ψ′(y)=p1αkET−(ατ+p1deβET)p1eβET. |
Therefore, if ψ′(y)<0, that is, if the condition
p1αkET<ατ+p1deβET |
holds, then the pseudo-equilibrium Ep(ET,yQ1) is locally asymptotically stable.
If x(t)<ET, subsystem (2.1) may have two internal equilibria E11(x11,y11) and E12(x12,y12), where x11<x12. We have the following results about Filippov system (2.3).
(1) If ET<x11, E11(x11,y11) and E12(x12,y12) are virtual equilibria, denoted by Ev11 and Ev12, respectively.
(2) If x11<ET<x12, E11(x11,y11) is a regular equilibrium and E12(x12,y12) is a virtual equilibrium, denoted by Er11 and Ev12, respectively.
(3) If ET>x12, E11(x11,y11) and E12(x12,y12) are regular equilibria, denoted by Er11 and Er12, respectively.
If x(t)>ET, assume that subsystem (2.2) have three positive equilibria, E21(x21,y21), E22(x22,y22) and E23(x23,y23), where x21<x22<x23. We have the following results about Filippov system (2.3).
(1) If ET<x21, E21(x21,y21), E22(x22,y22) and E23(x23,y23) are regular equilibria, denoted by Er21, Er22 and Er23, respectively.
(2) If x21<ET<x22, E21(x21,y21) is a virtual equilibrium, and E22(x22,y22) and E23(x23,y23) are regular equilibria, denoted by Ev21, Er22 and Er23, respectively.
(3) If x22<ET<x23, E21(x21,y21) and E22(x22,y22) are virtual equilibria, and E23(x23,y23) is a regular equilibrium, denoted by Ev21, Ev22 and Er23, respectively.
(4) If ET>x23, E21(x21,y21), E22(x22,y22) and E23(x23,y23) are virtual equilibria, denoted by Ev21, Ev22 and Ev23, respectively.
The tangent point of Σs satisfies the following equations:
{r(1−xK)−αye−βx=0,x=ET,or{r(1−xK)−αye−βx−p1=0,x=ET, |
so, the tangent points of the Filippov system (2.3) are Et1(ET,yc2) and Et2(ET,yc1).
The boundary equilibrium of Σs satisfies the following equations:
{r(1−xK)−αye−βx=0,kαxe−βx−d=0,x=ETor{r(1−xK)−αye−βx−p1=0,y(kαxe−βx−d)+τ=0,x=ET. |
If d=kαETe−βET, Filippov system (2.3) has a boundary equilibrium Eb1(ET,yc2); if y=τd−kαETe−βET, Filippov system (2.3) has a boundary equilibrium Eb2(ET,yc1).
Select the parameters as those in Figure 4, where subsystem (2.1) has a globally asymptotically stable limit cycle (see Figure 4(a)), and subsystem (2.2) has a global asymptotic stability focus (see Figure 4(b)). When the ET is small, Filippov system (2.3) has a virtual equilibrium Ev21 and regular equilibrium Er21, which yields a globally asymptotically stable focus (see Figure 5(a)). When ET increases to 7.1, the equilibrium E21, the tangent point Et2 and the pseudo-equilibrium Ep collide at the boundary equilibrium Eb2 and all orbits approach Eb2 (see Figure 5(b)). As the ET continues to increase, both equilibrium points E11 and E21 become virtual equilibria; in this case, there is a pseudo-equilibrium Ep, which is globally asymptotically stable (see Figure 5(c)). When ET=8.7, the equilibrium E11, tangent point Et1 and pseudo-equilibrium Ep collide at the boundary equilibrium Eb1 all orbits will approach Eb1 (see Figure 5(d)). When ET increases to 9.5, the unstable equilibrium Er11 and Ev21 coexist and a stable sliding limit cycle intersecting with the sliding mode Σs appears in Filippov system (2.3) (see Figure 5(e)). With the increase of ET, the sliding limit cycle will occur in the order of grazing bifurcation, buckle bifurcation and crossing bifurcation (see Figure 5(e)–(h)). When the ET is sufficiently large, a globally asymptotically stable standard limit cycle is completely located in the region M1, and all orbits approach the stable standard limit cycle (2.3) (see Figure 5(i)).
From the analysis of the sliding mode Σs and pseudo-equilibrium Ep, Filippov system (2.3) has rich and complex dynamic properties. The following numerical simulations method is used to discuss the effects of killing rate p1 on the sliding mode Σs and pseudo-equilibrium Ep. It can be seen in Figure 6 that, when the killing rate p1=0.25, with the gradual increase of ET, the sliding mode will gradually increase and the pseudo- equilibrium Ep always exists. This shows that the density of the pest population is controlled to ET, which avoids the large-scale outbreak of the pest population. The pests and natural enemies will always be in a relatively stable coexistence state, which is beneficial to the stability of the ecological environment (see Figure 6(a)). When the killing rate p1=0.06, which is relatively small, with the gradual increase of ET, the pseudo-equilibrium changes from existence to non-existence, which may lead to the outbreak of the pest population (see Figure 6(b)).
In what follows, ET is used as the bifurcation parameter to analyze the boundary equilibrium bifurcation in Filippov system (2.3). The boundary focus or boundary node bifurcation phenomenon occurs at ET=x21 and ET=x11, respectively. At this time, the tangent point Et, the pseudo- equilibrium Ep and the equilibrium E11 (or E21) all collide together at the boundary equilibrium Eb.
From Section 4.2, Filippov system (2.3) exhibits the boundary focus bifurcation phenomenon at the two endpoints of the sliding mode when ET=7.1 and ET=8.7, respectively (see Figure 5(b), (d)). Selecting the parameters in Figure 7, when ET=6.1 and ET=8.7, Filippov system (2.3) exhibits the boundary node bifurcation phenomenon at the two endpoints of the sliding mode (see Figure 7(b), (d)).
When ET=30, an unstable regular equilibrium Er11 and a virtual Ev21 of Filippov system (2.3) coexist and all orbits tend to be a stable standard limit cycle in M1 (see Figure 8(a)). With the decrease of ET, when ET=28.8, the standard limit cycle of Filippov system (2.3) collides with the upper tangent point Et1, that is, the grazing bifurcation phenomenon occurs (see Figure 8(b)). When the parameter ET continues to decrease to 28, the sliding mode Σs becomes part of the limit cycle (see Figure 8(c)). Specifically, when ET decreases to 25.7, all orbits of system (2.3) tend to a stable sliding limit cycle, which is located in M1 and contains the whole sliding mode Σs (Figure 8(d)). In this case, the pest population density is controlled within the reasonable range of the ET, which is beneficial for pest control.
When ET=23, the sliding limit cycle of system (2.3) is located in M1 and M2, which contains part of the sliding mode and the upper tangent point Et1 (Figure 8(e)). When ET decreases to 22.2, the sliding limit cycle is located in M1 and M2, passes through a tangent point Et1 and contains the entire sliding mode Σs of Filippov system (2.3) (see Figure 8(f)). When the parameter ET continues to decrease to 20, Filippov system (2.3) still has an unstable regular equilibrium Er11 and a virtual equilibrium Ev21, and it exhibits a stable crossing limit cycle containing the whole sliding mode (see Figure 8(g)). Obviously, Figures 8(c)–(e) depicts the process of buckling bifurcation, and Figures 8(e)–(g) depicts the process of crossing bifurcation.
In conclusion, with ET decreasing from 30 to 20, the sliding limit cycle of system (2.3) changes gradually from being completely located in M1 to containing part or the whole section of Σs, and to being partially located in M2, and this process progresses from the grazing bifurcation to the complete buckling bifurcation phenomenon, and then to the complete crossing bifurcation phenomenon. It can be seen from the numerical simulations that, when ET is small, the complex sliding bifurcation phenomenon occurs in system (2.3), which makes the density of the pest population sometimes exceed ET, brings uncertainty to pest control and is not conducive to the monitoring and control of pests (see Figure 8(e)–(g)).
In this paper, an integrated pest management Filippov model with group defense and a constant-rate release of natural enemies is established, and the complex dynamics of Filippov system (2.3) are studied. Combined with the conclusions of this paper, the establishment of x21>ET can be avoided by controlling the dose of sprayed pesticides or the release rate of natural enemies. Otherwise, Er21 becomes a globally asymptotically stable equilibrium. In this case, the density of the pest population exceeds ET, which may lead to pest population outbreak and is not conducive to pest control (see Figures 5(a) and 7(a)). With the gradual increase of pest population group defense ability β, x21 also gradually increases (see Figure 9). This suggests that the stronger the group defense ability of the pest population, the more difficult it is to control pests. If a regular equilibrium that is globally asymptotically stable in both subsystems and a virtual equilibrium coexist, then the regular equilibrium will become the globally asymptotically stable equilibrium in Filippov system (2.3) after the switching disturbance. If a regular equilibrium that is unstable in both subsystems and a virtual equilibrium coexist, then Filippov system (2.3) may appear in a globally asymptotically stable limit cycle. When two virtual equilibria coexist, Filippov system (2.3) has a globally asymptotically stable pseudo-equilibrium (see Figures 5(c) and 7(c)). When the equilibrium reaches the dividing line x(t)=ET, the tangent point Et, the pseudo-equilibrium Ep and the regular equilibrium collide at the boundary equilibrium Eb. This collision results in a bifurcation at the boundary, which can take the form of either a focus or a node, depending on whether the equilibrium is itself a focus or a node (see Figures 5(b), (d) and 7(b), (d)). With the change of the ET, the limit cycle will generate complex global sliding bifurcation phenomena such as grazing bifurcation, buckling bifurcation, and crossing bifurcation. If the grazing bifurcation occurs naturally for the Filippov system as the limit cycle collides with the upper tangent point Et1 and the standard limit cycle is completely located in M1, then this situation can control the density of the pest population within a reasonable range that does not exceed ET, which is conducive to integrated pest management. On the contrary, if there is buckling bifurcation, crossing bifurcation and standard limit cycles located in M2, then these situations can cause the density of the pest population to exceed the ET, which is not conducive to pest control (see Figure 8). In this paper, we assume that if x>ET, we adopt a continuous threshold control strategy and the killing rate of pests is linear owing to the effects of pesticides. In fact, pesticides are sprayed periodically and have certain residual and delay effects on the pests. In this case, it becomes more difficult to analyze the dynamics of the system with the threshold control strategy. We will leave it for future work.
This work was supported by the National Natural Science Foundation of China (12171004).
All authors declare no conflicts of interest regarding the publication of this paper.
[1] |
W. Peng, N. L. Ma, D. Zhang, Q. Zhou, X. Yue, S. C. Khoo, et al., A review of historical and recent locust outbreaks: Links to global warming, food security and mitigation strategies, Environ. Res., 191 (2020), 110046. https://doi.org/10.1016/j.envres.2020.110046 doi: 10.1016/j.envres.2020.110046
![]() |
[2] |
X. Gao, G. Li, X. Wang, S. Wang, F. Li, Y. Wang, et al., The locust plagues of the Ming and Qing dynasties in the Xiang-E-Gan region, China, Nat. Hazards, 107 (2021), 1149–1165. https://doi.org/10.1007/s11069-021-04622-y doi: 10.1007/s11069-021-04622-y
![]() |
[3] |
H. I. Freedman, G. S. Wolkowicz, Predator-prey systems with group defence: the paradox of enrichment revisited, Bull. Math. Biol., 48 (1986), 493–508. https://doi.org/10.1007/BF02462320 doi: 10.1007/BF02462320
![]() |
[4] |
H. I. Freedman, S. Ruan, Hopf bifurcation in three-species food chain models with group defense, Math. Biosci., 111 (1992), 73–87. https://doi.org/10.1016/0025-5564(92)90079-C doi: 10.1016/0025-5564(92)90079-C
![]() |
[5] | J. C. Holmes, W. M. Bethel, Modification of intermediate host behaviour by parasites, Zool. J. Linn. Soc., 51 (1972), 123–149. |
[6] |
A. A. Salih, M. Baraibar, K. K. Mwangi, G. Artan, Climate change and locust outbreak in East Africa, Nat. Clim. Change, 10 (2020), 584–585. https://doi.org/10.1038/s41558-020-0835-8 doi: 10.1038/s41558-020-0835-8
![]() |
[7] |
C. N. Meynard, M. Lecoq, M. P. Chapuis, C. Piou, On the relative role of climate change and management in the current desert locust outbreak in East Africa, Global. Change. Biol., 26 (2020), 3753–3755. https://doi.org/10.1111/gcb.15137 doi: 10.1111/gcb.15137
![]() |
[8] | M. L. Flint, Integrated Pest Management for Walnuts, 2nd edition, University of California, Oakland, 1987. |
[9] |
J. C. V. Lenteren, J. Woets, Biological and integrated pest control in greenhouses, Ann. Rev. Entomol, 33 (1988), 239–250. https://doi.org/10.1146/annurev.en.33.010188.001323 doi: 10.1146/annurev.en.33.010188.001323
![]() |
[10] |
S. Y. Tang, Y. Tian, R. A. Cheke, Dynamic complexity of a predator-prey model for IPM with nonlinear impulsive control incorporating a regulatory factor for predator releases, Math. Model. Anal., 24 (2019), 134–154. https://doi.org/10.3846/mma.2019.010 doi: 10.3846/mma.2019.010
![]() |
[11] |
T. T. Yu, Y. Tian, H. J. Guo, X. Song, Dynamical analysis of an integrated pest management predator Cprey model with weak Allee effect, J. Biol. Dyn., 13 (2019), 218–244. https://doi.org/10.1080/17513758.2019.1589000 doi: 10.1080/17513758.2019.1589000
![]() |
[12] |
B. P. Baker, T. A. Green, A. J. Loker, Biological control and integrated pest management in organic and conventional systems, Biol. Control, 140 (2020), 104095. https://doi.org/10.1016/j.biocontrol.2019.104095 doi: 10.1016/j.biocontrol.2019.104095
![]() |
[13] | J. C. Headley, Defining the Economic Threshold, in Pest Control Strategies for the Future, National Academy of Sciences, (1972), 100–108. |
[14] | L. G. Higley, D. J. Boethel, Handbook of Soybean Insect Pests, Entomological Society of America, 1994. |
[15] |
L. P. Pedigo, S. H. Hutchins, L. G. Higley, Economic injury levels in theory and practice, Ann. Rev. Entomol., 31 (1986), 341–368. https://doi.org/10.1146/annurev.en.31.010186.002013 doi: 10.1146/annurev.en.31.010186.002013
![]() |
[16] |
Y. Tian, K. Sun, L. S. Chen, Geometric approach to the stability analysis of the periodic solution in a semi-continuous dynamic system, Int. J. Biomath., 7 (2014), 1450018. https://doi.org/10.1142/S1793524514500181 doi: 10.1142/S1793524514500181
![]() |
[17] | S. Y. Tang, W. H. Pang, R. A. Cheke, J. Wu, Global dynamics of a state-dependent feedback control system, Adv. Differ. Equ-Ny, 1 (2015). |
[18] |
B. Liu, Y. Tian, B. L. Kang, Dynamics on a Holling II predator Cprey model with state-dependent impulsive control, Int. J. Biomath., 5 (2012), 1260006. https://doi.org/10.1142/S1793524512600066 doi: 10.1142/S1793524512600066
![]() |
[19] |
Y. Tian, S. Y. Tang, Dynamics of a density-dependent predator-prey biological system with nonlinear impulsive control, Math. Biosci. Eng., 18 (2021), 7318–7343. https://doi.org/10.3934/mbe.2021362 doi: 10.3934/mbe.2021362
![]() |
[20] |
I. Ullah Khan, S. Ullah, E. Bonyah, B. A. Alwan, A. Alshehri, A state-dependent impulsive nonlinear system with ratio-dependent action threshold for investigating the pest-natural enemy model, Complexity, 2022 (2022), 7903450. https://doi.org/10.1155/2022/7903450 doi: 10.1155/2022/7903450
![]() |
[21] | V. I. Utkin, Sliding Modes and Their Applications in Variable Structure Systems, Mir Publishers, Moscow, 1994. |
[22] | A. F. Filippov, Differential Equations with Discontinuous Righthand Sides, Kluwer Academic Publishers, Dordrecht, 1988. |
[23] | V. I. Utkin, Sliding Modes in Control and Optimization, Springer-Verlag, Berlin, 1992. |
[24] |
Y. A. Kuznetsov, S. Rinaldi, A. Gragnani, One parameter bifurcations in planar Filippov systems, Int. J. Bifurc. Chaos, 13 (2003), 2157–2188. https://doi.org/10.1142/S0218127403007874 doi: 10.1142/S0218127403007874
![]() |
[25] |
W. J. Qin, S. Y. Tang, C. C. Xiang, Y. Yang, Effects of limited medical resource on a Filippov infectious disease model induced by selection pressure, Appl. Math. Comput., 283 (2016), 339–354. https://doi.org/10.1016/j.amc.2016.02.042 doi: 10.1016/j.amc.2016.02.042
![]() |
[26] | D. Y. Wu, H. Y. Zhao, Y. Yuan, Complex dynamics of a diffusive predator-prey model with strong Allee effect and threshold harvesting, J. Math. Anal. Appl., 469 (2019), 982–1014. |
[27] |
Y. N. Xiao, X. X. Xu, S. Y. Tang, Sliding mode control of outbreaks of emerging infectious diseases, Bull. Math. Biol., 74 (2012), 2403–2422. https://doi.org/10.1007/s11538-012-9758-5 doi: 10.1007/s11538-012-9758-5
![]() |
[28] |
W. J. Qin, X. W. Tan, X. T. Shi, J. Chen, X. Liu, Dynamics and bifurcation analysis of a Filippov predator-prey ecosystem in a seasonally fluctuating environment, Int. J. Bifurc. Chaos, 29 (2019), 1950020. https://doi.org/10.1142/S0218127419500202 doi: 10.1142/S0218127419500202
![]() |
[29] |
J. Bhattacharyya, P. T. Piiroinen, S. Banerjee, Dynamics of a Filippov predator-prey system with stage-specific intermittent harvesting, Nonlinear Dyn., 105 (2021), 1019–1043. https://doi.org/10.1007/s11071-021-06549-2 doi: 10.1007/s11071-021-06549-2
![]() |
[30] |
K. Enberg, Benefits of threshold strategies and age-selective harvesting in a fluctuating fish stock of Norwegian spring spawning herring Clupea harengus, Mar. Ecol. Prog. Ser., 298 (2005), 277–286. https://doi.org/10.3354/meps298277 doi: 10.3354/meps298277
![]() |
[31] | V. I. Utkin, J. Guldner, J. X. Shi, Sliding Model Control in Electromechanical Systems, Taylor Francis Group, London, 2009. |
[32] |
C. Dong, C. Xiang, W. Qin, Y. Yang, Global dynamics for a Filippov system with media effects, Math. Biosci. Eng., 19 (2022), 2835–2852. https://doi.org/10.3934/mbe.2022130 doi: 10.3934/mbe.2022130
![]() |
[33] |
C. Erazo, M. E. Homer, P. T. Piiroinen, M. Bernardo, Dynamic cell mapping algorithm for computing basins of attraction in planar Filippov systems, Int. J. Bifurc. Chaos, 27 (2017), 1730041. https://doi.org/10.1142/S0218127417300415 doi: 10.1142/S0218127417300415
![]() |
[34] | J. Deng, S. Tang, H. Shu, Joint impacts of media, vaccination and treatment on an epidemic Filippov model with application to COVID-19, J. Theor. Biol., 523 (2021), 110698. |
[35] | D. M. Xiao, S. R. Ruan, Codimension two bifurcations in a predator Cprey system with group defense, Int. J. Bifurc. Chaos, 11 (2001), 2123–2131. |
[36] |
X. Hou, B. Liu, Y. Wang, Z. Zhao, Complex dynamics in a Filippov pest control model with group defense, Int. J. Biomath., 15 (2022), 2250053. https://doi.org/10.1142/S179352452250053X doi: 10.1142/S179352452250053X
![]() |
[37] | R. M. Corless, G. H. Gonnet, D. E. G. Hare, D. J. Jeffrey, D. E. Knuth, On the LambertW function, Adv. Comput. Math., 5 (1996), 329–359. |
1. | Soliman A. A. Hamdallah, Dynamics analysis of Filippov pest control model with two economic thresholds, 2024, 1468-9367, 1, 10.1080/14689367.2024.2430690 |