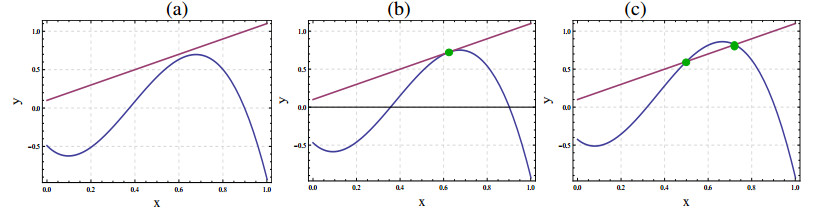
In the present study, the effects of the strong Allee effect on the dynamics of the modified Leslie-Gower predator-prey model, in the presence of nonlinear prey-harvesting, have been investigated. In our findings, it is seen that the behaviors of the described mathematical model are positive and bounded for all future times. The conditions for the local stability and existence for various distinct equilibrium points have been determined. The present research concludes that system dynamics are vulnerable to initial conditions. In addition, the presence of several types of bifurcations (e.g., saddle-node bifurcation, Hopf bifurcation, Bogdanov-Takens bifurcation, homoclinic bifurcation) has been investigated. The first Lyapunov coefficient has been evaluated to study the stability of the limit cycle that results from Hopf bifurcation. The presence of a homoclinic loop has been demonstrated by numerical simulation. Finally, possible phase drawings and parametric figures have been depicted to validate the outcomes.
Citation: Manoj K. Singh, Brajesh K. Singh, Poonam, Carlo Cattani. Under nonlinear prey-harvesting, effect of strong Allee effect on the dynamics of a modified Leslie-Gower predator-prey model[J]. Mathematical Biosciences and Engineering, 2023, 20(6): 9625-9644. doi: 10.3934/mbe.2023422
[1] | Mengyun Xing, Mengxin He, Zhong Li . Dynamics of a modified Leslie-Gower predator-prey model with double Allee effects. Mathematical Biosciences and Engineering, 2024, 21(1): 792-831. doi: 10.3934/mbe.2024034 |
[2] | Hongqiuxue Wu, Zhong Li, Mengxin He . Dynamic analysis of a Leslie-Gower predator-prey model with the fear effect and nonlinear harvesting. Mathematical Biosciences and Engineering, 2023, 20(10): 18592-18629. doi: 10.3934/mbe.2023825 |
[3] | Juan Ye, Yi Wang, Zhan Jin, Chuanjun Dai, Min Zhao . Dynamics of a predator-prey model with strong Allee effect and nonconstant mortality rate. Mathematical Biosciences and Engineering, 2022, 19(4): 3402-3426. doi: 10.3934/mbe.2022157 |
[4] | Fang Liu, Yanfei Du . Spatiotemporal dynamics of a diffusive predator-prey model with delay and Allee effect in predator. Mathematical Biosciences and Engineering, 2023, 20(11): 19372-19400. doi: 10.3934/mbe.2023857 |
[5] | Kawkab Al Amri, Qamar J. A Khan, David Greenhalgh . Combined impact of fear and Allee effect in predator-prey interaction models on their growth. Mathematical Biosciences and Engineering, 2024, 21(10): 7211-7252. doi: 10.3934/mbe.2024319 |
[6] | Rongjie Yu, Hengguo Yu, Chuanjun Dai, Zengling Ma, Qi Wang, Min Zhao . Bifurcation analysis of Leslie-Gower predator-prey system with harvesting and fear effect. Mathematical Biosciences and Engineering, 2023, 20(10): 18267-18300. doi: 10.3934/mbe.2023812 |
[7] | Christian Cortés García . Bifurcations in a discontinuous Leslie-Gower model with harvesting and alternative food for predators and constant prey refuge at low density. Mathematical Biosciences and Engineering, 2022, 19(12): 14029-14055. doi: 10.3934/mbe.2022653 |
[8] | Kunlun Huang, Xintian Jia, Cuiping Li . Analysis of modified Holling-Tanner model with strong Allee effect. Mathematical Biosciences and Engineering, 2023, 20(8): 15524-15543. doi: 10.3934/mbe.2023693 |
[9] | Yun Kang, Sourav Kumar Sasmal, Amiya Ranjan Bhowmick, Joydev Chattopadhyay . Dynamics of a predator-prey system with prey subject to Allee effects and disease. Mathematical Biosciences and Engineering, 2014, 11(4): 877-918. doi: 10.3934/mbe.2014.11.877 |
[10] | Moitri Sen, Malay Banerjee, Yasuhiro Takeuchi . Influence of Allee effect in prey populations on the dynamics of two-prey-one-predator model. Mathematical Biosciences and Engineering, 2018, 15(4): 883-904. doi: 10.3934/mbe.2018040 |
In the present study, the effects of the strong Allee effect on the dynamics of the modified Leslie-Gower predator-prey model, in the presence of nonlinear prey-harvesting, have been investigated. In our findings, it is seen that the behaviors of the described mathematical model are positive and bounded for all future times. The conditions for the local stability and existence for various distinct equilibrium points have been determined. The present research concludes that system dynamics are vulnerable to initial conditions. In addition, the presence of several types of bifurcations (e.g., saddle-node bifurcation, Hopf bifurcation, Bogdanov-Takens bifurcation, homoclinic bifurcation) has been investigated. The first Lyapunov coefficient has been evaluated to study the stability of the limit cycle that results from Hopf bifurcation. The presence of a homoclinic loop has been demonstrated by numerical simulation. Finally, possible phase drawings and parametric figures have been depicted to validate the outcomes.
The future of human civilization depends on sustainable natural resources such as forestry, wildlife, marine life, and many more, as they not only provide food, energy, medicines, and other necessities for the human population but also help to regulate the Earth's biodiversity. The increasing food demand has led to an increase in the exploitation of these resources, which is exerting a negative impact on the environment. As a result, it is critical to devise harvesting tactics that maximize economic rewards while simultaneously considering the ecological-health of the related ecological-system. Predator-prey interactions, which are ubiquitous in nature, have a wide-ranging impact on our biological-system. Lotka [1] and Volterra [2] devised separately the first mathematical model to examine predator-prey interactions, and later it was referred to as the Lotka-Volterra predator-prey model. In the conceptualization of this model, all the components, like growth rate, predator's mortality rate and altering the rate of prey biomass into predator reproduction, are assumed to be linear functions [3], but predator-prey interactions depend on nonlinear components in nature. As a consequence, the model fails to account for specific real-world scenarios, so a number of improvements have been suggested by researchers. Leslie and Gower devised the Leslie-Gower predator-prey (LGPP) model [4], where the growth function of predator species is distinct from the predation function of predator species. In [5], the authors have investigated this model and found that for all ecologically acceptable parameters, there exists a unique, globally asymptotically stable, co-existing equilibrium point. Aziz-Alaoui and Okiye [6] enhanced the realism of this model, and later it was termed the modified Leslie-Gower prey-predator (MLGPP) model.
Multiple biological resources are being overused because of the increasing demand for food and other supplies. However, protecting the environment as a whole is a topic of concern on a worldwide scale. In light of these two opposing realities, it is necessary for the commercial use of renewable biological resources, such as fisheries and forestry, to be managed scientifically and to search for a sustainable development strategy. In 1976, Clark [7] made the first attempt to develop a mathematical model to examine the challenges and strategies of harvesting renewable natural resources. In mathematical ecology, harvesting is classified into three forms: constant harvesting, linear harvesting and nonlinear harvesting; see [8,9]. The nonlinear harvesting is one of the most feasible options [9,10]. Hu and Cao [11] examined a predator-prey system in which non-linear harvesting regulates the proliferation of predator species. They obtained certain parametric conditions for the existence of several bifurcations: namely, Hopf bifurcation, transcritical bifurcation, saddle-node bifurcation and Bogdanov-Takens bifurcation. The qualitative behavior of a ratio-dependent Holling-Tanner predator-prey system with prey harvesting of nonlinear type was examined by Singh and Bhadauria [12], where the system's behavior in the neighborhood of its origin was studied and found to be non-hyperbolic. Abid et al. [13] examined the dynamics of a harvested MLGPP model. Al-Momen and Naji [14] investigated the impact of the fear effect on the dynamics of a harvested MLGPP model.
W. C. Allee [15] suggested an inspiring biological phenomenon termed the Allee effect (mechanism that results in a positive association between an aspect of the individual's fitness and the density/quantity of conspecifics) or negative competition effect [16]. It is a widespread phenomenon in many animals that can be caused by a variety of reasons [17]. One of the key reasons is that it is difficult to find partners within the same species in the case of low population density. The Allee effect enhances the likelihood of local/global extinction of a predator-prey system [18]. In recent decades, many mathematicians/ecologists have employed the Allee effect to improve the realism of mathematical models. AlSharawi et al. [19] provided experimental evidence of the Allee effect in a range of natural species. Based on how much the per capita growth rate is slowed down for the low population densities, the Allee effect is classified in two classes: strong Allee effect (in case the per capita growth rate is negative at the limit of low density) and weak Allee effect (in case the per capita growth rate is positive at zero density) [20,21]. In the literature, a lot of research demonstrated that the Allee effect makes a predator-prey system more dynamic and fascinating, providing new insights into the connections between the two species [22,23]. When the proliferation of prey species is subjected to the double Allee effect, Singh et al. [24] examined how this affects the stability and bifurcations of the MLGPP model. Feng and Kang [25] reported a comprehensive qualitative study of the MLGPP model that assumes the Allee effect influences the proliferation of both species. In [26], a comprehensive study of a modified Volterra predator-prey model in which the prey population is growing under the influence of the double Allee effect has been provided. One of the most interesting results they found was that the Allee effect can change the number of limit cycles. A Holling-Tanner predator-prey system was investigated by Arancibia-Ibarra et al. [27], where prey species experience a strong Allee effect. Recently, Zhu et al. [28] improved the MLGPP model by incorporating the Allee effect and analyzed its qualitative behaviors.
In the present study, the MLGPP model is made more realistic by incorporating nonlinear prey harvesting and a strong Allee-effect within the prey species. The remaining part of the paper is laid out as shown below. The described model is developed in Section 2. Additionally, the solutions to the model are analyzed for their positivity as well as their boundedness. Section 3 deals with the emergence of system equilibrium points and their local stability. In Section 4, two local bifurcations (saddle node and Hopf bifurcations), the nature of Hopf-bifurcating periodic solutions and a global bifurcations (Bogdanov-Takens bifurcation) are examined. The analytical findings are verified numerically in Section 5. In Section 6, the combined impact of nonlinear harvesting and the Allee effect on the dynamics of the MLGPP system is reported. Finally, the results obtained with biological conclusions are discussed in Section 7.
Gupta and Chandra [8] proposed the following continuous-time two species MLGPP model, where prey growth is governed by nonlinear harvesting:
{dudt=ru(1−uK)−a1uvn+u−qEum1E+m2u,dvdt=sv(1−a2vn+u), | (2.1) |
where, at time t, u=u(t) and v=v(t) denote the densities of prey and predator, respectively. All parameter values (r,s,a1,sa2,n,K,E and q) are positive and have the following ecological meanings: r and s are the maximum specific growth rates of prey and predator, a1 is the encounter rate of predators, n is the degree to which the ecosystem protects prey and predator, K is the carrying capacity for the prey species, sa2 is the maximum value of the per capita decreasing rate of the predator, E represents harvesting effort, and q represents the catchability coefficient. Further, m1 and m2 are suitable constants. Here, the authors assumed that the degree of the environment's protection is equal for both species. Consider that the growth of the first species of system (2.1) is subjected to a strong Allee effect. This consideration leads to the following form:
{dudt=ru(1−uK)(u−M)−a1uvn+u−qEum1E+m2u,dvdt=sv(1−a2vn+u), | (2.2) |
where (u−M) stands for Allee effect, and −K≤M<K is its threshold value. This variable determines how much the Allee effect affects the system. Since the strong Allee effect is of interest in this study, so we have 0<M≤K. Consider the following transformations to make the analysis easier.
x=uK,y=a2vK,τ=rt. |
The system (2.2) transforms into the following non-dimensionalized system:
{dxdτ=xK((1−x)(x−m)−βya+x−hc+x),dydτ=γy(1−ya+x), | (2.3) |
where a=nK,m=MK,β=a1a2rK,h=qErm2K2,γ=sr,c=m1Em2K.
Consider dt=Kdτ and γ=Kρ to eliminate the parameter K from the system (2.3), and we get
{dxdt=((x−m)(1−x)−βya+x−hc+x)x,dydt=(1−ya+x)ρy. | (2.4) |
Some results are provided to confirm that the proposed system is well posed.
Lemma 2.1. (a) Let (x(t),y(t)) be a solution behavior of model (2.4) with initial conditions (I-Cs) x(0)>0,y(0)>0. Then, x(t)>0,y(t)>0,∀t≥0.
(b) Each solution of the model Eq (2.4) with the I-Cs starting in the positive quadrant is bounded for every t≥0.
Proof. (a) The prey and predator behavior for the model Eq (2.4) can be evaluated as follows:
x(t)/x(0)=exp(∫t0((1−x(z))(x(z)−m)−βy(z)a+x(z)−hc+x(z))dz), | (2.5) |
and
y(t)/y(0)=exp[∫t0ρ(1−y(z)a+x(z))dz]. | (2.6) |
From (2.5) one can see that for all t≥0 the solution x(t) will be non-negative whenever I-C x(0) is non-negative. The similar finding for predator species, y(t), holds true from (2.6), so the set R2+ = {(x,y):x,y≥0} is an invariant set.
(b) Suppose (x(t),y(t)) is a non-negative solution behavior of the model Eq (2.4), among them the behavior of prey species is as follows:
x(t)=x(0)exp[∫t0((1−x(z))(x(z)−m)−βy(z)a+x(z)−hc+x(z))dz]. | (2.7) |
For the sake of convenience, take F(x(z),y(z))=((1−x(z))(x(z)−m)−βy(z)a+x(z)−hc+x(z)).
Now, we have the two cases that are explained below:
Case (i) When x(0)≤1. Our goal is to show x(t)≤1,∀t≥1.
Suppose that our claim is not correct. In this case, two positive reals, say, t1 and t2, exist (t2>t1) with the property that x(t1)=1, x(t)>1,∀t∈(t1,t2). This yields
x(t)=x(0).e∫t0F(x(z),y(z))dz,∀t∈(t1,t2), | (2.8) |
Equation (2.8) leads to
x(t)=x(0).e∫t10F(x(z),y(z))dz.e∫tt1F(x(z),y(z))dz<x(t1). |
As F(x(t),y(t))<0,∀t∈(t1,t2), we have a contradiction, and our claim is accepted.
Case (ii) When x(0)>1. Clearly, F(x(t),y(t))<0 whenever x(t)≥1, so, as long as x(t)≥1,
x(t)=x(0).e∫t0F(x(z),y(z))dz<x(0). |
From cases (ⅰ) and (ⅱ), we get
x(t)≤max{1,x(0)}=N2,∀t≥0. | (2.9) |
Inequality (2.9) with the predator equation in (2.4) leads to
dydt≤ρy(1−ya+N2). | (2.10) |
The inequality (2.10) implies
y(t)≤max{y(0),a+N2},∀t≥0. |
Hence the result.
This portion deals with the number of feasible equilibria admitted by model (2.4). The nullclines of model (2.4) are given by
x((x−m)(1−x)−βya+x−hc+x)=0,y(1−ya+x)=0. | (3.1) |
Thus, the model (2.4) always admits the trivial equilibrium point E0=(0,0) and the prey free equilibrium point E5=(0,a). In addition to the above, two types of equilibrium points are evaluated as follows:
(i) Predator Free Equilibrium Points: Ei=(xi,0), where xi can be evaluated by the cubic equation
x3−T(c,m)x2−L(c,m)x+mc+h=0, | (3.2) |
where T(c,m)=1−c+m, and L(c,m)=c(1+m)−m. Descartes' sign rule assures that Eq (3.2) always has a negative root if any of the conditions i)T>0,L≥0,ii)T>0,L≤0 and iii)T≤0,L>0 holds true.
Let us assume this negative root to be x1=−α,α>0. To obtain the other two roots of the Eq (3.2), we divide it by (x+α), and consequently, the following quadratic equation is obtained.
x2−x(T(c,m)+α)+α(T(c,m)+α)−L(c,m)=0. | (3.3) |
(ii) Interior Equilibrium Points: E∗i=(x∗i,a+x∗i), where x∗i is the root of the cubic equation
x∗3−T(c,m)x∗2−R(c,m,β)x∗+(m+β)c+h=0, | (3.4) |
where T(c,m)=1−c+m, and R(c,m,β)=(1+m)c−m−β. Descartes' sign rule assures that Eq (3.4) always admits a negative root if any of the conditions i) T>0,R≥0,ii) T>0,R≤0 and iii) T≤0,R>0 holds true. Let us assume the negative root to be x∗1=−ξ,ξ>0, and the remaining two roots are obtained by finding the roots of the following quadratic equation, obtained by dividing Eq (3.4) by x∗+ξ:
x∗2−x∗(ξ+T(c,m))+ξ(ξ+T(c,m))−R(c,m,β)=0. | (3.5) |
Take Δ1=(α+T(c,m))2−4(α(α+T(c,m))−L(c,m)), Δ2=(ξ+T(c,m))2−4(ξ(ξ+T(c,m))−R(c,m,β)), α∗=−T(c,m)+2√T2(c,m)+3L(c,m)3, and ξ∗=−T(c,m)+2√T2(c,m)+3R(c,m,β)3, provided L(c,m)≥0 and R(c,m,β)≥0.
As a summary of the preceding discussion, we get the following.
Lemma 3.1. If any of the conditions i) T>0,L≥0, ii) T>0,L≤0 and iii) T≤0,L>0 holds true, then the model Eq (2.4) has
(a) no predator-free point of equilibrium whenever α>α∗,
(b) a unique predator-free point equilibrium E2(x2,0) whenever α=α∗, where x2=1−c+m+α2, and
(c) two distinct predator-free point equilibrium points E3(x3,0) and E4(x4,0) whenever 0<α<α∗, where x3=α+T(c,m)+√Δ12, and x4=α+T(c,m)−√Δ12.
Lemma 3.2. If any of the conditions i) T>0,R≥0, ii) T>0,R≤0 and iii) T≤0,R>0 holds true, then the model (2.4) has
(a) no interior point of equilibrium whenever ξ>ξ∗,
(b) a unique interior equilibrium point E∗2(x∗2,a+x∗2) if ξ=ξ∗, where x∗2=1−c+m+α∗2,
(c) two distinct interior equilibrium points E∗3(x∗3,a+x∗3) and E∗4(x∗4,a+x∗4) if 0<ξ<ξ∗, where x∗3=α∗+T(c,m)+√Δ22 and x∗4=α∗+T(c,m)−√Δ22.
Using the conditions of Lemma 3.2, Figure 1 depicts the numbers of interior equilibrium points.
This portion deals with the linearization approach to examine local stability of equilibria for model (2.4).
Theorem 3.1. Always, the trivial point E0 is a saddle.
Proof. The Jacobian matrix of model (2.4) at E0(0,0) is
JE0=[−1c(mc+h)00ρ], |
whose eigenvalues are λ1=−1c(mc+h)<0 and λ2=ρ>0. We get the result from the fact that these eigenvalues are of opposite signs.
Theorem 3.2. Always, the point E3 is a saddle, whereas E4 is unstable.
Proof. The Jacobian matrix for model (2.4) at an axial feasible point E(x,0) of equilibrium is given by
JE(x,0)=[(1−2x+m+h(c+x)2)x−βx(a+x)0ρ], |
whose eigenvalues are λ1=x(1−2x+m+h(c+x)2) and λ2=ρ.
Interpreting x(1−2x+m+h(c+x)2) at x3=1−c+m+α+√Δ12, the eigenvalue λ1=−x3√Δ1(c+x3)(α+x3)<0. Since the eigenvalues of the matrix JE(x3,0) are of opposite signs, the point E3 is always a saddle point.
Interpreting x(1−2x+m+h(c+x)2) at x4=1−c+m+α−√Δ12, the eigenvalue λ1=x4√Δ1(c+x4)(α+x4)>0. Since the eigenvalues of the matrix JE(x3,0) are of positive signs, the point E4 is always an unstable point.
Theorem 3.3. The equilibrium point E5=(0,a) is always asymptotically stable.
Proof. The Jacobian matrix for model (2.4) at E5=(0,a) is given by
JE5=[−(m+β+hc)0ρ−ρ], |
and eigenvalues of this are λ1=−(m+β+hc)<0 and λ2=−ρ<0. Since both eigenvalues are of negative sign, the point E5 is always stable.
Theorem 3.4. The point E∗4 is a saddle point always, while point E∗3 is
(i) unstable if x∗3(1−2x∗3+m+βa+x∗3+h(c+x∗3)2)>ρ,
(ii) stable if x∗3(1−2x∗3+m+βa+x∗3+h(c+x∗3)2)<ρ.
Proof. The Jacobian matrix for model (2.4) at E=(x,y) is
JE=[x(1−2x+m+βa+x+h(c+x)2)−βxa+xρ−ρ]. |
The determinant and trace of the above matrix are evaluated as
detJE=ρx(2x−(1+m+h(c+x)2)), and trJE=x(1−2x+m+β(a+x)+h(c+x)2)−ρ.
Computing x(2x−(1+m+h(c+x)2)) at x∗4=1−c+m+α∗−√Δ22 yields
x∗4(2x∗4−(1+m+h(c+x∗4)2))=x∗4√Δ22(c+x∗4)(−1−m+c−3α∗+√Δ2) =−x∗4√Δ2(c+x∗4)(α∗+x∗4),
which indicates that the determinant detJE∗4 is negative, so the point E∗4 is always a saddle point.
Now, interpreting x(2x−(1+m+h(c+x)2)) at x∗3=1−c+m+α∗+√Δ22 yields
x∗3(2x∗3−(1+m+h(c+x∗3)2))=x∗3√Δ22(c+x∗3)(1+m−c+3α∗+√Δ2) =x∗3√Δ2(c+x∗3)(α∗+x∗3),
which shows that the determinant detJE∗3 is positive, and the Routh-Hurwitz criterion confirms the conclusion.
Theorem 3.5. The equilibrium point E∗2 of the model Eq (2.4) is
(i) a stable saddle node whenever βx∗2a+x∗2<ρ and an unstable saddle node whenever βx∗2a+x∗2>ρ,
(ii) a cusp of codimension 2 whenever βx∗2a+x∗2=ρ and aβ(a+x∗2)2−2x∗22(c+x∗2)≠0.
Proof. (i) Use the transformation ˇx=x−x∗2,ˇy=y−y∗2 in the model (2.4) to shift the equilibrium point E∗2 to the origin (0,0), and the Taylor series expansion at (0,0) reduces the corresponding model equation to
{dˇxdt=βx∗2a+x∗2ˇx−βx∗2a+x∗2ˇy+a20ˇx2+a11ˇxˇy+o|(ˇx,ˇy)3|,dˇydt=ρˇx−ρˇy−ρa+x∗2ˇx2+2ρa+x∗2ˇxˇy−ρa+x∗2ˇy2+o|ˇx,ˇy)3|, | (3.6) |
where a20=aβ(a+x∗2)2−x∗22c+x∗2,a11=−aβ(a+x∗2)2.
Evidently, if βx∗2a+x∗2≠ρ, then the trace trJE∗2≠0, but the determinant detJE∗2=0, which confirms that the equilibrium point E∗2 is a saddle node. Additionally, trJE∗2<0 whenever βx∗2a+x∗2<ρ, so E∗2 is a stable saddle node. trJE∗2>0 whenever βx∗2a+x∗2>ρ, so the point E∗2 is an unstable saddle node.
(ii) Let us consider βx∗2a+x∗2=ρ, (3.6) can be expressed as
{dˇxdt=ρ(ˇx−ˇy)+a20ˇx2+a11ˇxˇy+o|(ˇx,ˇy)3|,dˇydt=ρ(ˇx−ˇy)−ρa+x∗2ˇx2+2ρa+x∗2ˇxˇy−ρa+x∗2ˇy2+o|(ˇx,ˇy)3|. | (3.7) |
Let us consider τ=ρt, the system (3.7) becomes
{dˇxdτ=ˇx−ˇy+b20ˇx2+b11ˇxˇy+o|(ˇx,ˇy)3|,dˇydτ=ˇx−ˇy−1a+x∗2ˇx2+2a+x∗2ˇxˇy−1a+x∗2ˇy2+o|(ˇx,ˇy)3|, | (3.8) |
where b20=a20ρ,b11=a11ρ.
The system (3.8) under the transformation ˜x=ˇx,˜y=ˇx−ˇy reduces to
{d˜xdτ=˜y+¯b20˜x2−b11˜x˜y+o|(˜x,˜y)3|,d˜ydτ=¯b20˜x2−b11˜x˜y+1a+x∗2˜y2+o|(˜x,˜y)3|, | (3.9) |
where ¯b20=b20+b11. Further, under the transformation x1=˜x,x2=˜y−1a+x∗2˜x˜y, the system (3.9) reduces to
{dx1dτ=x2+¯b20x21+¯b11x1x2+o|(x1,x2)3|,dx2dτ=¯b20x21−b11x1x2+o|(x1,x2)3|, | (3.10) |
where ¯b11=(1a+x∗2−b11). Finally, with the transformation y1=x1−12¯b11x21,y2=x2+¯b20x21+o|(x1,x2)3|, (3.10) reduces to
{dy1dτ=y2,dy2dτ=¯b20y21+(2¯b20−b11)y1y2+o|(y1,y2)3|. | (3.11) |
As 2¯b20−b11=1ρ(aβ(a+x∗2)2−2x∗22(c+x∗2))≠0, and ¯b20=−x∗22ρ(c+x∗2)≠0, E∗2 is a cusp of codimension 2 in the xy plane.
This section deals with the existence of various types of bifurcations for the model (2.4): saddle node bifurcation, Hopf bifurcations, Bogdanov-Takens bifurcation and homoclinic bifurcation.
It is proven in Lemma 3.2 that there is a unique degenerate positive equilibrium E∗2=(x∗2,y∗2) that develops as a result of the annihilation of the two internal equilibrium points E∗3=(x∗3,y∗3) and E∗4=(x∗4,y∗4). Thus, there is a chance of occurrence of a saddle-node bifurcation at E∗2=(x∗2,y∗2). Satomayor's criterion, which is described in the following theorem, is utilized to ensure the occurrence of saddle-node bifurcation.
Theorem 4.1. The model (2.4) exhibits a saddle-node bifurcation in the vicinity of the point E∗2(x∗2,y∗2) w.r.t. the parameter m whenever β(1−c+m+α∗)(2a+1−c+m+α∗)≠ρ.
Proof. The Jacobian matrix of the model (2.4) at E∗2, is
JE∗2=[β(1−c+m+α∗)(2a+1−c+m+α∗)−β(1−c+m+α∗)(2a+1−c+m+α∗)ρ−ρ]. |
The determinant detJE∗2=0, and trace trJE∗2=β(1−c+m+α∗)(2a+1−c+m+α∗)−ρ. So, we can conclude that whenever β(1−c+m+α∗)(2a+1−c+m+α∗)≠ρ, one eigenvalue of JE∗2 is zero, and the other is non-zero. Let V and U represent eigenvectors of matrices JE∗2 and JTE∗2, respectively, associated with zero-eigenvalue of the matrices. A simple computation implies
V=[11],U=[−ρ(2a+1−c+m+α∗)β(1−c+m+α∗)1]. |
Consider,
g(x,y,m)=((1−x)(x−m)−βya+x−hc+xa+x−y). | (4.1) |
Now,
gm(E∗2,m[sn])=[−1−c+m+α∗20],D2g(E,m[sn])(V,V)=(−2−16h(1+c+m+α∗)30). |
Thus,
UT.gm(E∗2,m[sn])=ρ(1+c−m−α∗)(2a+1−c+m+α∗)β(1−c+m+α∗)≠0. |
and
UT.D2g(E∗2,m[sn])(V,V)=ρ(2a+1−c+m+α∗)β(1−c+m+α∗)(2+16h1+c+m+α∗)≠0. |
The two preceding transversality conditions for saddle-node bifurcation indicate the occurrence of saddle-node bifurcation for the model (2.4) at E∗2.
Theorem 4.2. The model (2.4) exhibits a saddle-node bifurcation in the vicinity of the point E2(x2,0) w.r.t. the parameter m.
It is proven in Theorem 3.4 that the point E∗3 is unstable whenever x∗3(1−2x∗3+m+βa+x∗3+h(c+x∗3)2)>ρ, and it is stable whenever x∗3(1−2x∗3+m+βa+x∗3+h(c+x∗3)2)<ρ. It is now fascinating to look into the property of the point when x∗3(1−2x∗3+m+β(a+x∗3)+h(c+x∗3)2)−ρ=0. For this parametric condition, tr(JE∗3)=0, whereas det(JE∗3)>0, which leads to the appearance of the Hopf bifurcation at E∗3.
Theorem 4.3. The model (2.4) experiences a Hopf bifurcation around the point E∗3 w.r.t. the parameter ρ in the presence of the parametric condition x∗3(1−2x∗3+m+β(a+x∗3)+h(c+x∗3)2)−ρ=0.
Proof. For ρ=x∗3(1−2x∗3+m+β(a+x∗3)+h(c+x∗3)2), we get detJE∗3>0, trJE∗3=x∗3(1−2x∗3+m+β(a+x∗3)+h(c+x∗3)2)−ρ, and ddρ(trJE∗3)|ρ=ρ[hf]=−1.
This confirms that the transversality conditions of Hopf bifurcation hold true, and the model (2.4) undergoes a Hopf bifurcation around E∗3 w.r.t. the parameter ρ.
The above theorem ensures the existence of a limit cycle. Next, the first Lyapunov coefficient is computed for model (2.4) at E∗3 to examine the stability of the limit cycle.
Take x=ˉx−x∗3, y=ˉy−y∗3 to shift the equilibrium E∗3 to the origin (0,0), and the model (2.4) reduces to
{dˉxdt=a10ˉx+a01ˉy+a20ˉx2+a11ˉxˉy+a02ˉy2+a30ˉx3+a21ˉx2ˉy+a12ˉxˉy2+a03ˉy3+f1(ˉx,ˉy),dˉydt=b10ˉx+b01ˉy+b20ˉx2+b11ˉxˉy+b02ˉy2+b30ˉx3+b21ˉx2ˉy+b12ˉxˉy2+b03ˉy3+f2(ˉx,ˉy), | (4.2) |
where, a10=x∗3−2x∗32+mx∗3+βx∗3y∗3(a+x∗3)2+hx∗3(c+x∗3)2, a01=−βx∗3(a+x∗3), a20=(1−x∗3)+βy∗3(a+x∗3)2+h(c+x∗3)2−(x∗3−m)−x∗3−βx∗3y∗3(a+x∗3)3−hx∗3(c+x∗3)3, a11=−βa(a+x∗3)2, a02=0, a30=βx∗3y∗3(a+x∗3)4+hx∗3(c+x∗3)4−h(c+x∗3)3−βy∗3(a+x∗3)3−1, a21=βa(a+x∗3)3, a12=0, a03=0, b10=ρy∗32(a+x∗3)2, b01=−ρy∗3(a+x∗3), b20=−ρy∗32(a+x∗3)3, b11=2ρy∗3(a+x∗3)2, b02=−ρ(a+x∗3), b30=ρy∗32(a+x∗3)4, b21=−2ρy∗3(a+x∗3)3, b12=ρ(a+x∗3)2, b03=0, f1(ˉx,ˉy)=∑∞i+j=4aijˉxˉy, f2(ˉx,ˉy)=∑∞i+j=4bijˉxˉy.
As a result, the first Lyapunov number σ at the origin is evaluated as in Perko [29], as follows:
σ=−3π2a01Δ3/2[a10b10(a112+a11b02+a02b11)+a10a01(b112+a20b11+a11b02)+b102(a11a02+2a02b02)−2a10b10(b022−a20a02)−2a10a01(a202−b20b02)−a012(2a20b20+b11b20)+(a01b10−2a102)(b11b02−a11a20)]−(a102+a01b10)[3(b10b03−a01a30)+2a10(a21+b12)+(b10a12−a01b21)], where Δ=ρx∗3(2x∗3−(1+m+h(c+x∗3)2)).
An unstable limit cycle appears around E∗3 whenever σ>0; otherwise, a stable limit cycle appears around E∗3.
The Jacobian matrix of model (2.4) at E∗2=(x∗2,y∗2) is
JE∗2=[βx∗2(a+x∗2)−βx∗2(a+x∗2)ρ−ρ], |
det(JE∗2)=0, whereas the trace tr(JE∗2)=0, under the parametric condition βx∗2(a+x∗2)=ρ. As a result of this, the non-zero Jacobian matrix JE∗2 has a zero-eigenvalue of multiplicity two, which means that the Bogdanov - Takens (BT) bifurcation may occur in the model (2.4). As the parameters m,ρ play vital roles in the dynamics of the model equation, it is worthwhile to regard them as bifurcation parameters. The BT point in the parameter space is associated with the point at which the saddle-node bifurcation curve and Hopf-bifurcation curve cross. To ensure the appearance of the BT bifurcation, the non-degeneracy criteria are shown by using the technique introduced in [30].
Theorem 4.4. Bogdanov-Takens bifurcation occurs in the model Eq (2.4) w.r.t. bifurcation parameters m,ρ around the instantaneous point E∗2 whenever ρ=βx∗2(a+x∗2) and aρx∗2(a+x∗2)−2x∗2(α∗+x∗2)(c+x∗2)≠0.
Proof. Suppose m,ρ vary in a small neighborhood of BT point (m∗,ρ∗) and let (m,ρ)=(m∗+λ1,ρ∗+λ2) be a neighboring point of the BT point, where λ1,λ2 are very small, and the model (2.4) reduces to
{dxdt=x((1−x)(x−m∗)−βya+x−hc+x)−x(1−x)λ1=g1(x,y,λ1),dydt=ρ∗y(1−ya+x)+λ2y(1−ya+x)=g2(x,y,λ2). | (4.3) |
Under u1=x−x∗2,u2=y−y∗2 the BT point is shifted to (0,0). As a result, the system (4.3) reduces to
{du1dt=g1(x∗2,y∗2,λ1)+(p+λ1(2x∗2−1))u1+qu2+a112u21+a12u1u2+a222u22,du2dt=g2(x∗2,y∗2,λ2)+(r+λ2)u1+(s−λ2)u2+b112u21+b12u1u2+b222u22, | (4.4) |
where p,q,r and s are the Jacobian coefficients calculated at the equilibrium point E∗2, and the coefficients aij and bij are determined by
a11=[∂2g1∂x2](x∗2,y∗2,m∗,ρ∗)=2(aβ(a+x∗2)2−x∗2−hx∗2(c+x∗2)3)+2λ1,
a12=[∂2g1∂x∂y](x∗2,y∗2,m∗,ρ∗)=−aβ(a+x∗2)2,
a22=[∂2g1∂y2](x∗2,y∗2,m∗,ρ∗)=0,
b11=[∂2g2∂x2](x∗2,y∗2,m∗,ρ∗)=−2ρ(a+x∗2)−2λ2(a+x∗2),
b12=[∂2g2∂x∂y](x∗2,y∗2,m∗,ρ∗)=2ρ(a+x∗2)+2λ2(a+x∗2),
b22=[∂2g2∂y2](x∗2,y∗2,m∗,ρ∗)=−2ρ(a+x∗2)−2λ2(a+x∗2).
Making the affine transformations v1=u1 and v2=pu1+qu2, the system (4.4) reduces to
{dv1dt=ξ00(λ)+ξ10(λ)v1+ξ01(λ)v2+12ξ20(λ)v21+ξ11(λ)v1v2+12ξ02(λ)v22+p1(v1,v2),dv2dt=η00(λ)+η10(λ)v1+η01(λ)v2+12η20(λ)v21+η11(λ)v1v2+12η02(λ)v22+p2(v1,v2), | (4.5) |
where,
ξ00(λ)=g1(x∗2,y∗2,λ1), ξ10(λ)=λ1(2x∗2−1),ξ01(λ)=1,ξ20(λ)=a11−2pqa12+p2q2a22,
ξ11(λ)=a12q−pq2a22,ξ02(λ)=a22q2,η00(λ)=pg1(x∗2,y∗2,λ1)+qg2(x∗2,y∗2,λ2),
η10(λ)=pλ1(2x∗2−1),η01(λ)=−λ2,
η20(λ)=(pa11+qb11−2pq(pa12+qb12)+p2q2(pa22+qb22)),
η11(λ)=(pa12+qb12q−pq2(pa22+qb22)),η02(λ)=(pa22+qb22)q2.
In order to ensure the existence of Bogodanov-Takens bifurcation, we examine the following non-degeneracy conditions [30]:
i) [pqrs]=θ2×2,
ii) ξ20(0)+η11(0)≠0,
iii) η20(0)≠0.
The first restriction is obvious. Using the values of aij,bij,p and q, we get
ξ20(0)+η11(0)=aρx∗2(a+x∗2)−2x∗2(α∗+x∗2)(c+x∗2).
η20=−2ρx∗2(α∗+x∗2)a+x∗2≠0.
Hence, the proof is complete.
This section deals with the numerical simulations to validate the presented analytical findings. For computations and plotting phase portrait diagrams, we utilized the MATHEMATICA 10.0 software.
1)
{dxdt=x((1−x)(x−0.17)−0.07995y0.10+x−0.100.5+x),dydt=ρy(1−y0.10+x). | (5.1) |
From an ecological point of view, ρ<1. The model (5.1) exhibits six feasible equilibrium points: E0=(0,0), E3=(0.902701,0)E4=(0.351066,0), E5=(0,0.1),E∗3=(0.665439,0.765439) and E∗4=(0.583736,0.683736). The natures of the equilibrium points are shown in Table 1.
Equilibrium | Value of ρ | Nature | Figure(s) |
[0.5ex] E0 | 0<ρ<1 | Saddle | 2 |
E3 | 0<ρ<1 | Saddle | 2 |
E4 | 0<ρ<1 | unstable | 2 |
E5 | 0<ρ<1 | stable | 2 |
0<ρ<0.0114431 | unstable | 2 a | |
ρ=0.0114431 | unstable limit cycle encloses | ||
it (σ=936.161π>0) | 2 b | ||
E∗3 | 0.0114431<ρ<0.018011 | stable | 2 c |
ρ=0.018011 | stable and enclosed by | ||
unstable homoclinic loop | 2 d | ||
ρ>0.018011 | stable but homoclinic loop | ||
will disappear | 2 e | ||
E∗4 | 0<ρ<1 | Saddle | 2 |
2)
{dxdt=x((1−x)(x−0.174772)−0.07995y0.10+x−0.100.5+x),dydt=ρy(1−y0.10+x). | (5.2) |
There are four axial equilibrium points, E0=(0,0),E3=(0.901899,0),E4=(0.356186,0) and E5(0,0.1) in the model (5.2), along with a unique interior point E∗2(0.626768,0.726768). Quantitatively, the points of axial equilibria are identical with the axial equilibria of the model (5.1). Unique interior equilibrium point E∗2 occurs due to collision of the interior points E∗3 and E∗4 of model (5.1). The nature of E∗2 is reported in Table 2.
Equilibrium | Value of ρ | Nature | Figure |
[0.5ex] | ρ<βx∗2a+x∗2, | Unstable Saddle node | 3 b |
E∗2=(0.626768,0.726768) | ρ=βx∗2a+x∗2 | Cusp | 3 c |
ρ>βx∗2a+x∗2 | Stable Saddle node | 3 d |
3)
{dxdt=x((1−x)(x−m)−0.07995y0.10+x−0.100.5+x),dydt=0.5y(1−y0.10+x). | (5.3) |
The model Eq (5.3) consists of only axial equilibrium points, and their number depends upon the parameter m. Table 3 records the numbers of axial equilibrium points and their natures.
Value of m | Number of equilibria | Name | Nature | Figure |
[0.5ex] 0<m<0.430034 | 4 | E0=(0,0) | Saddle | 4 (a) |
E3=(0.9,0) | Saddle | |||
E4=(0.4,0) | Unstable | |||
E5=(0,0.1) | Stable | |||
m=0.430034 | 3 | E0=(0,0) | Saddle | 4 (c) |
E2=(0.75,0) | Unstable saddle node | |||
E5=(0,0.1) | Stable | |||
0.430034<m<1 | 2 | E0=(0,0) | Saddle | 4 (d) |
E1=(0,0.1) | Globally Stable |
4)
{dxdt=x((1−x)(x−m)−0.0799y0.08+x−0.080.35+x),dydt=ρy(1−y0.08+x). | (5.4) |
The saddle-node bifurcation curve for the model (5.4) is m=0.193603. The hopf-bifurcation curve for the model Eq (5.4) is ρ=x∗3(1−2x∗3+m+0.07990.08+x∗3+0.08(0.35+x∗3)2). The intersection point of these two curves (BT point) is (m∗,ρ∗)=(0.193603,0.070995). These two curves divide the feasible region into three distinct regions: Region Ⅰ, Region Ⅱ and Region Ⅲ. The behaviors of these regions are reported in Table 4 and depicted in Figure 5.
Region | Nature of the equilibria |
Region Ⅰ | Absence of interior points |
Region Ⅱ | Two interior points (first is saddle, while the other is unstable) |
Region Ⅲ | Two interior points (first is saddle, while the other is stable) |
This work deals with a MLGPP model with two important phenomena, the Allee effect and nonlinear harvesting. These two phenomena have strong impacts on the dynamics of a predator-prey system. Also, the phenomenon of the Allee effect is natural as it occurs frequently in species. It has been found that at low population density, it may enhance the risk of extinction [21]. On the other hand, the harvesting provides a scientific study of the exploitation of renewable resources. Thus, a predator-prey model that includes the Allee effect and non-linear harvesting in prey species is more realistic. To the best of the authors' knowledge, this is the first effort to study any predator-prey model experiencing both the Allee effect and non-linear prey harvesting.
The model (2.4) with no Allee effect undergoes a number of bifurcations, known as saddle-node bifurcation, Hopf bifurcation, transcritical bifurcation and Bogdanov-Takens bifurcation [8]. Meanwhile, the considered model equation with Allee effect experiences all aforesaid bifurcations except the transcritical one, but this model provides more general parametric conditions for appearance of these bifurcations. The dynamics of the populations of the model (2.4) in the hm− plane have been plotted in Figure 6, which provides the maximum harvesting rate for a threshold value of Allee effect.
In the present work, the dynamics of a harvested MLGPP model have been studied in the case of a strong Allee effect. It has been assumed that the growth of the prey species is subjected to non-linear harvesting. The analysis was simplified by the study of the equivalent system (2.4). It has been found that the model (2.4) has at most six equilibrium points, in which the trivial equilibrium point and a predator-free equilibrium are always saddle points, whereas another predator-free equilibrium point is unstable. The prey-free equilibrium point is always asymptotically stable. Ecologically speaking, predator species are always active in the ecosystem. If two interior points of equilibrium exist, then one of them is always a saddle, whereas the other's behavior depends on parametric conditions. It has been proven that this point is enclosed by unstable limit cycles in the presence of certain parametric conditions. Thus, the system exhibits bistability and oscillatory coexistence for both the populations for a given parametric domain. The first Lyapunov number has been calculated for studying the stability of limit cycles.
The equivalent model (2.4) can have 0 to 2 interior equilibrium points as the bifurcation parameter m crosses a certain threshold value. The appearance of saddle-node bifurcation has been demonstrated via Sotomayor's theorem. Ecologically speaking, if m is less than the maximum of the threshold value of m, then both species will coexist; and above that, extinction is faced by the prey species. Moreover, the model (2.4) undergoes Bogdanov-Taken bifurcation near the degenerate equilibrium point. The parameters m and ρ are used as bifurcation parameters, and the system is reduced to standard form. In terms of ecology, a small disturbance in the bifurcation parameters can lead to coexistence, oscillation, or even extinction. Finally, we have discussed the combined influence of the non-linear harvesting and the Allee effect, which may be useful to protect the system at low population density of prey species.
The second author is thankful to Banasthali Vidyapith for giving an opportunity and motivation to write this research paper.
The authors declare there is no conflict of interest.
[1] | A. J. Lotka, Elements of physical biology, Williams and Wilkins, Baltimore MD, 1925. |
[2] |
V. Volterra, Fluctuations in the abundance of species considered Mathematically, Nature, 118 (1926), 558–560. http://dx.doi.org/10.1038/118558a0 doi: 10.1038/118558a0
![]() |
[3] |
C. S. Holling, Some characteristics of simple types of predation and parasitism, Canadian Entom., 91 (1959), 385–398. https://doi.org/10.4039/Ent91385-7 doi: 10.4039/Ent91385-7
![]() |
[4] |
P. H. Leslie, J. C. Gower, The properties of a stochastic model for the predator-prey type of interaction between two species, Biometrika, 47 (1960), 219–234. https://doi.org/10.1093/biomet/45.3-4.316 doi: 10.1093/biomet/45.3-4.316
![]() |
[5] |
S. B. Hsu, T. W. Huang, Global stability for a class of predator-prey systems, SIAM J. Appl. Math., 55 (1995), 763–783. https://doi.org/10.1137/S00361399932532 doi: 10.1137/S00361399932532
![]() |
[6] |
M. A. Aziz-Alaoui, M. Daher Okiye, Boundedness and global stability for a predator-prey model with modified Leslie-Gower and Holling-type Ⅱ schemes, Appl. Math. Lett., 16 (2003), 1069–1075. https://doi.org/10.1016/S0893-9659(03)90096-6 doi: 10.1016/S0893-9659(03)90096-6
![]() |
[7] |
C. W. Clark, A delayed-recruitment model of population dynamics, with an application to baleen whale populations, J. Math. Biol., 3 (1976), 381–391. https://doi.org/10.1007/BF00275067 doi: 10.1007/BF00275067
![]() |
[8] |
R. P. Gupta, P. Chandra, Bifurcation analysis of modified Leslie-Gower predator-prey model with Michaelis-Menten type prey harvesting., J. Math. Anal. Appl., 398 (2013), 278–295. https://doi.org/10.1016/j.jmaa.2012.08.057 doi: 10.1016/j.jmaa.2012.08.057
![]() |
[9] |
M. K. Singh, B. S. Bhadauria, B. K. Singh, Qualitative analysis of a Leslie-Gower predator-prey system with nonlinear harvesting in predator, Int. J. Eng. Math., 2741891 (2016). https://doi.org/10.1155/2016/2741891 doi: 10.1155/2016/2741891
![]() |
[10] |
M. Li, B. Chen, H. Ye, A bioeconomic differential algebraic predator–prey model with nonlinear prey harvesting, Appl. Math. Model., 42 (2017), 17–28. https://doi.org/10.1016/j.apm.2016.09.029 doi: 10.1016/j.apm.2016.09.029
![]() |
[11] |
D. Hu, H. Cao, Stability and bifurcation analysis in a predator-prey system with Michaelis-Menten type predator harvesting, Nonlinear Anal. Real World Appl., 33 (2017), 58–82. https://doi.org/10.1016/j.nonrwa.2016.05.010 doi: 10.1016/j.nonrwa.2016.05.010
![]() |
[12] | M. K. Singh, B. S. Bhadauria, The impact of nonlinear harvesting on a ratio-dependent Holling-Tanner predator-prey system and optimum harvesting, Appl. Appl. Math., 15 (2020), 117–148. https://digitalcommons.pvamu.edu/aam/vol15/iss1/8 |
[13] |
W. Abid, R. Yafia, M. A. Aziz-Alaoui, A. Aghriche, Dynamics analysis and optimality in selective harvesting predator-prey model with modified leslie-gower and Holling-Type Ⅱ, Nonauton. Dyn. Syst., 6 (2019), 1–17. https://doi.org/10.1515/msds-2019-0001 doi: 10.1515/msds-2019-0001
![]() |
[14] |
S. Al-Momen, R. K. Naji, The dynamics of modified leslie-gower predator-prey model under the influence of nonlinear harvesting and fear effect, Iraqi J. Sci., 63 (2022), 259–282. https://doi.org/10.24996/ijs.2022.63.1.27 doi: 10.24996/ijs.2022.63.1.27
![]() |
[15] | W. C. Allee, Co-operation among animals, Am. J. Sociol., 37 (1931), 386–398. https://www.jstor.org/stable/2766608 |
[16] | M. Begon, M. Mortimer, Population ecology: an unified study of animals and plants, 2nd edition Sinauer, Sunderland, Massachusetts, USA, 1986. |
[17] |
P. A. Stephens, W. J. Sutherland, R. P. Freckleton, What is the Allee effect, Oikos, 87 (1999), 185–190. https://doi.org/10.2307/3547011 doi: 10.2307/3547011
![]() |
[18] |
J. Zu, Global qualitative analysis of a predator–prey system with Allee effect on the prey species, Math. Comput. Simul., 94 (2013), 33–54. https://doi.org/10.1016/j.matcom.2013.05.009 doi: 10.1016/j.matcom.2013.05.009
![]() |
[19] |
Z. AlSharawi, S. Pal, N. Pal, J. Chattopadhyay, A discrete-time model with non-monotonic functional response and strong Allee effect in prey, J. Difference Equ. Appl., 26 (2020), 404–431. https://doi.org/10.1080/10236198.2020.1739276 doi: 10.1080/10236198.2020.1739276
![]() |
[20] | F. Courchamp, L. Berec, J. Gascoigne, Allee effects in ecology and conservation, Oxford University Press, Oxford, 2008. https://doi.org/10.1093/acprof: oso/9780198570301.001.0001 |
[21] |
B. Dennis, Population growth, critical density, and the chance of extinction, Nat. Res. Model, 3 (1989), 481–538. https://doi.org/10.1111/j.1939-7445.1989.tb00119.x doi: 10.1111/j.1939-7445.1989.tb00119.x
![]() |
[22] |
M. Sen, M. Banerjee, A. Morozov, Bifurcation analysis of a ratio-dependent prey-predator model with the Allee effect, Ecol. Complex., 11 (2012), 12–27. https://doi.org/10.1016/j.ecocom.2012.01.002 doi: 10.1016/j.ecocom.2012.01.002
![]() |
[23] |
J. Wang, J. Shi, J. Wei, Predator-prey system with strong Allee effect in prey, J. Math. Biol., 62 (2011), 291–331. https://doi.org/10.1007/s00285-010-0332-1 doi: 10.1007/s00285-010-0332-1
![]() |
[24] |
M. K. Singh, B. S. Bhadauria, B. K. Singh, Bifurcation analysis of modified Leslie-Gower predator-prey model with double Allee effect, Ain Shams Eng. J., 9 (2018), 1263–1277. https://doi.org/10.1016/j.asej.2016.07.007 doi: 10.1016/j.asej.2016.07.007
![]() |
[25] |
P. Feng, Y. Kang, Dynamics of a modified Leslie-Gower model with double Allee effects, Nonlinear Dyn., 80 (2015), 1051–1062. https://doi.org/10.1007/s11071-015-1927-2 doi: 10.1007/s11071-015-1927-2
![]() |
[26] |
E. González-Olivares, B. González-Yañez, J. Mena-Lorca, A. Rojas-Palma, J. D. Flores, Consequences of double Allee effect on the number of limit cycles in a predator–prey model, Comput. Math. Appl., 62 (2011), 3449–3463. https://doi.org/10.1016/j.camwa.2011.08.061 doi: 10.1016/j.camwa.2011.08.061
![]() |
[27] |
C. Arancibia-Ibarra, J. D. Flores, G. Pettet, P. Van Heijster, A Holling-Tanner predator-prey model with strong Allee effect, Int. J. Bifurc. Chaos, 29 (2019), 1930032. https://doi.org/10.1142/S0218127419300325 doi: 10.1142/S0218127419300325
![]() |
[28] |
Z. Zhu, Y. Chen, Z. Li, F. Chen, Stability and bifurcation in a leslie–gower predator–prey model with Allee effect, Int. J. Bifurc. Chaos, 32 (2022), 2250040. https://doi.org/10.1142/S0218127422500407 doi: 10.1142/S0218127422500407
![]() |
[29] | L. Perko, Differential Equations and Dynamical Systems, 3nd edition, Springer-Verlag, New York, 2001. |
[30] | Y. A. Kuznetsov, Elements of applied bifurcation theory, Springer Science and Business Media, New York, 2004. |
1. | Swagata Dutta, Gourav Mandal, Lakshmi Narayan Guin, Santabrata Chakravarty, Identifying numerical bifurcation structures of codimensions 1 and 2 in interacting species system, 2024, 47, 0170-4214, 14639, 10.1002/mma.10295 | |
2. | Mengyun Xing, Mengxin He, Zhong Li, Dynamics of a modified Leslie-Gower predator-prey model with double Allee effects, 2023, 21, 1551-0018, 792, 10.3934/mbe.2024034 |
Equilibrium | Value of ρ | Nature | Figure(s) |
[0.5ex] E0 | 0<ρ<1 | Saddle | 2 |
E3 | 0<ρ<1 | Saddle | 2 |
E4 | 0<ρ<1 | unstable | 2 |
E5 | 0<ρ<1 | stable | 2 |
0<ρ<0.0114431 | unstable | 2 a | |
ρ=0.0114431 | unstable limit cycle encloses | ||
it (σ=936.161π>0) | 2 b | ||
E∗3 | 0.0114431<ρ<0.018011 | stable | 2 c |
ρ=0.018011 | stable and enclosed by | ||
unstable homoclinic loop | 2 d | ||
ρ>0.018011 | stable but homoclinic loop | ||
will disappear | 2 e | ||
E∗4 | 0<ρ<1 | Saddle | 2 |
Value of m | Number of equilibria | Name | Nature | Figure |
[0.5ex] 0<m<0.430034 | 4 | E0=(0,0) | Saddle | 4 (a) |
E3=(0.9,0) | Saddle | |||
E4=(0.4,0) | Unstable | |||
E5=(0,0.1) | Stable | |||
m=0.430034 | 3 | E0=(0,0) | Saddle | 4 (c) |
E2=(0.75,0) | Unstable saddle node | |||
E5=(0,0.1) | Stable | |||
0.430034<m<1 | 2 | E0=(0,0) | Saddle | 4 (d) |
E1=(0,0.1) | Globally Stable |
Region | Nature of the equilibria |
Region Ⅰ | Absence of interior points |
Region Ⅱ | Two interior points (first is saddle, while the other is unstable) |
Region Ⅲ | Two interior points (first is saddle, while the other is stable) |
Equilibrium | Value of ρ | Nature | Figure(s) |
[0.5ex] E0 | 0<ρ<1 | Saddle | 2 |
E3 | 0<ρ<1 | Saddle | 2 |
E4 | 0<ρ<1 | unstable | 2 |
E5 | 0<ρ<1 | stable | 2 |
0<ρ<0.0114431 | unstable | 2 a | |
ρ=0.0114431 | unstable limit cycle encloses | ||
it (σ=936.161π>0) | 2 b | ||
E∗3 | 0.0114431<ρ<0.018011 | stable | 2 c |
ρ=0.018011 | stable and enclosed by | ||
unstable homoclinic loop | 2 d | ||
ρ>0.018011 | stable but homoclinic loop | ||
will disappear | 2 e | ||
E∗4 | 0<ρ<1 | Saddle | 2 |
Equilibrium | Value of ρ | Nature | Figure |
[0.5ex] | ρ<βx∗2a+x∗2, | Unstable Saddle node | 3 b |
E∗2=(0.626768,0.726768) | ρ=βx∗2a+x∗2 | Cusp | 3 c |
ρ>βx∗2a+x∗2 | Stable Saddle node | 3 d |
Value of m | Number of equilibria | Name | Nature | Figure |
[0.5ex] 0<m<0.430034 | 4 | E0=(0,0) | Saddle | 4 (a) |
E3=(0.9,0) | Saddle | |||
E4=(0.4,0) | Unstable | |||
E5=(0,0.1) | Stable | |||
m=0.430034 | 3 | E0=(0,0) | Saddle | 4 (c) |
E2=(0.75,0) | Unstable saddle node | |||
E5=(0,0.1) | Stable | |||
0.430034<m<1 | 2 | E0=(0,0) | Saddle | 4 (d) |
E1=(0,0.1) | Globally Stable |
Region | Nature of the equilibria |
Region Ⅰ | Absence of interior points |
Region Ⅱ | Two interior points (first is saddle, while the other is unstable) |
Region Ⅲ | Two interior points (first is saddle, while the other is stable) |