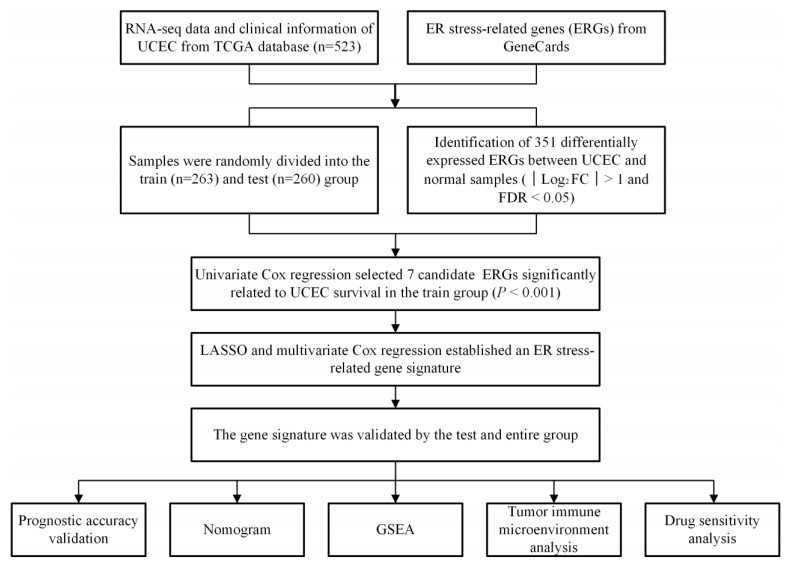
Counterfactual analysis of the impact of Covid-19 can be based on a solution of a macroeconomic model for a scenario without the coronavirus interfering with the macroeconomic system. Two measures of impact are introduced with the aid of a simple theoretical model, and then used in the empirical analysis: (Ⅰ) The difference between the counterfactual without Covid-19 and a baseline model solution. (Ⅱ) The difference between the counterfactual and the actual development of the economy. In order to analyze the impact on GDP we use two model categories. First, empirical final form model equations, which were purpose-built with the aid of a machine learning algorithm. Second, an operational multiple-equation model of the Norwegian macroeconomic system. Empirically, we find a significant impact of Covid-19 on the GDP Mainland Norway in 2020. For some of the estimator/model combinations, the impacts are also significant in the two first quarters of 2021. Using the multiple-equation model, the assessment is extended to the impact of Covid-19 on value added in four Mainland Norway industries, on imports and exports, and on final consumption expenditure and gross capital formation.
Citation: Ragnar Nymoen. Economic Covid-19 effects analysed by macro econometric models—the case of Norway[J]. National Accounting Review, 2023, 5(1): 1-22. doi: 10.3934/NAR.2023001
[1] | Yan He, Nannan Cao, Yanan Tian, Xuelin Wang, Qiaohong Xiao, Xiaojuan Tang, Jiaolong Huang, Tingting Zhu, Chunhui Hu, Ying Zhang, Jie Deng, Han Yu, Peng Duan . Development and validation of two redox-related genes associated with prognosis and immune microenvironment in endometrial carcinoma. Mathematical Biosciences and Engineering, 2023, 20(6): 10339-10357. doi: 10.3934/mbe.2023453 |
[2] | Bin Ma, Lianqun Cao, Yongmin Li . A novel 10-gene immune-related lncRNA signature model for the prognosis of colorectal cancer. Mathematical Biosciences and Engineering, 2021, 18(6): 9743-9760. doi: 10.3934/mbe.2021477 |
[3] | Xiaowei Zhang, Jiayu Tan, Xinyu Zhang, Kritika Pandey, Yuqing Zhong, Guitao Wu, Kejun He . Aggrephagy-related gene signature correlates with survival and tumor-associated macrophages in glioma: Insights from single-cell and bulk RNA sequencing. Mathematical Biosciences and Engineering, 2024, 21(2): 2407-2431. doi: 10.3934/mbe.2024106 |
[4] | Yong Luo, Xiaopeng Liu, Jingbo Lin, Weide Zhong, Qingbiao Chen . Development and validation of novel inflammatory response-related gene signature to predict prostate cancer recurrence and response to immune checkpoint therapy. Mathematical Biosciences and Engineering, 2022, 19(11): 11345-11366. doi: 10.3934/mbe.2022528 |
[5] | Chen Zheng, Zhaobang Tan . A novel identified pyroptosis-related prognostic signature of colorectal cancer. Mathematical Biosciences and Engineering, 2021, 18(6): 8783-8796. doi: 10.3934/mbe.2021433 |
[6] | Dong-feng Li, Aisikeer Tulahong, Md. Nazim Uddin, Huan Zhao, Hua Zhang . Meta-analysis identifying epithelial-derived transcriptomes predicts poor clinical outcome and immune infiltrations in ovarian cancer. Mathematical Biosciences and Engineering, 2021, 18(5): 6527-6551. doi: 10.3934/mbe.2021324 |
[7] | Jiaping Wang . Prognostic score model-based signature genes for predicting the prognosis of metastatic skin cutaneous melanoma. Mathematical Biosciences and Engineering, 2021, 18(5): 5125-5145. doi: 10.3934/mbe.2021261 |
[8] | Jihong Yang, Hao Xu, Congshu Li, Zhenhao Li, Zhe Hu . An explorative study for leveraging transcriptomic data of embryonic stem cells in mining cancer stemness genes, regulators, and networks. Mathematical Biosciences and Engineering, 2022, 19(12): 13949-13966. doi: 10.3934/mbe.2022650 |
[9] | Linlin Tan, Dingzhuo Cheng, Jianbo Wen, Kefeng Huang, Qin Zhang . Identification of prognostic hypoxia-related genes signature on the tumor microenvironment in esophageal cancer. Mathematical Biosciences and Engineering, 2021, 18(6): 7743-7758. doi: 10.3934/mbe.2021384 |
[10] | Han Zhao, Yun Chen, Peijun Shen, Lan Gong . Construction and validation of a novel prognostic signature for uveal melanoma based on five metabolism-related genes. Mathematical Biosciences and Engineering, 2021, 18(6): 8045-8063. doi: 10.3934/mbe.2021399 |
Counterfactual analysis of the impact of Covid-19 can be based on a solution of a macroeconomic model for a scenario without the coronavirus interfering with the macroeconomic system. Two measures of impact are introduced with the aid of a simple theoretical model, and then used in the empirical analysis: (Ⅰ) The difference between the counterfactual without Covid-19 and a baseline model solution. (Ⅱ) The difference between the counterfactual and the actual development of the economy. In order to analyze the impact on GDP we use two model categories. First, empirical final form model equations, which were purpose-built with the aid of a machine learning algorithm. Second, an operational multiple-equation model of the Norwegian macroeconomic system. Empirically, we find a significant impact of Covid-19 on the GDP Mainland Norway in 2020. For some of the estimator/model combinations, the impacts are also significant in the two first quarters of 2021. Using the multiple-equation model, the assessment is extended to the impact of Covid-19 on value added in four Mainland Norway industries, on imports and exports, and on final consumption expenditure and gross capital formation.
According to a global cancer report, uterine corpus cancer ranks as the sixth most common cancer in women, with approximately 417,000 new cases (2.2% of all sites) and 97,000 deaths (1.0% of all sites) in 2020 worldwide [1]. Among all American female cancer survivors, uterine corpus cancer is the second most prevalent after breast cancer [2]. More than 90% of uterine corpus cancer originated from the endometrium, namely, uterine corpus endometrial cancer (UCEC) [2]. Due to early detection and improved treatment, the 5-year overall survival rate of uterine corpus cancer is about 81% [2], but the mortality rate of UCEC remains the highest among women with low socioeconomic status [3]. Considering the growing morbidity and large number of surviving UCEC patients, it is imperative to explore biomarkers to predict prognosis and guide treatment.
The endoplasmic reticulum (ER) is an organelle involved in multiple cellular processes, including protein synthesis, folding and transport, lipid biosynthesis and calcium balance [4]. However, in the tumor microenvironment, many harsh factors can disrupt the protein-handling capacity of ER and cause excessive accumulation of misfolded/unfolded protein, such as nutrient starvation, oxidative stress, intracellular calcium imbalance and high metabolic demand, thereby leading to a state of "ER stress" [5]. Subsequently, unfolded protein response (UPR) is activated in an attempt to restore ER homeostasis, ultimately causing cellular reprogramming and adaptation, autophagy or even cell death [6]. A body of studies has indicated that sustained and robust ER stress responses contribute to cancer cell survival, metastasis, angiogenesis and therapy resistance [7,8,9]. In addition, ER stress responses can play an immunoregulatory role [10]. ER stress-related genes (ERGs), a series of genes involved in the ER stress signaling, have been reported to correlate with various tumors' development, such as breast, glioma, liver and prostate cancer [11,12,13,14]. However, the roles and therapeutic value of ERGs in UCEC have not been extensively investigated. It was reported that the UPR target glucose-regulated protein 78 took part in the endometrial cancer cell growth, invasion and chemotherapy resistance and was correlated with advanced stage and poor prognosis [15,16]. Therefore, ERGs may be valuable prognostic factors and potential therapeutic avenues for UCEC.
As a patient's prognosis exerts a great influence on personal and clinical decisions, accurate prognostic models have gained great attention in recent years [17,18]. The present study aimed to construct a risk model based on ERGs to forecast clinical outcomes and provide guidance for UCEC treatment. By bioinformatic analysis, we constructed a prognostic gene signature containing 4 ERGs, which exhibited excellent prognostic capacity. In addition, based on the model, we analyzed the immune infiltration and drug sensitivity in order to improve the prognosis of UCEC patients.
RNA sequencing of transcriptome profiling data (HTSeq-FPKM) and corresponding clinical information for UCEC were obtained from TCGA database (https://portal.gdc.cancer.gov/repository), containing 554 malignancy samples and 32 normal samples. We obtained the clinical data of 548 UCEC cases, including survival time, survival status, age, gender, grade and stage. After eliminating the samples with survival time < 30 days and unknown survival time or status, 523 UCEC patients were ultimately retained in our study. What's more, 1371 ERGs were extracted from GeneCards (https://www.genecards.org/) with relevance score > 5. The "limma" R package and Wilcoxon test were used to identify differentially expressed ERGs (DEERGs) between UCEC tumor and normal samples, with the significance thresholds set as │Log2 fold change (FC)│ > 1 and false discovery rate (FDR) < 0.05 [19]. Finally, 351 DEERGs were obtained for further analysis (Table S1). To identify the potential biological functions of hub DEERGs, we did Gene Ontology (GO) term and Kyoto Encyclopedia of Genes and Genomes (KEGG) pathway enrichment analysis by "GOplot, " "enrichplot" and "ggplot2" R packages (https://bioconductor.org/packages/release/bioc/html/enrichplot.html) [20,21]. GO terms contain biological process (BP), cellular components (CC) and molecular functions (MF) analysis. The results were visualized with "clusterProfiler" and "org.Hs.eg.db" R packages (https://www.bioconductor.org/packages/release/data/annotation/html/org.Hs.eg.db.html) [22].
351 DEERGs' transcriptome data and 523 UCEC clinical data were integrated by the "limma" R package, and they were then randomly assigned into test and training groups, with 260 and 263 samples, respectively. The training group was used to establish the risk signature, while the test group and entire group were used to verify its effectiveness. First, by univariate Cox regression, we obtained 7 candidate prognostic ERGs in the training group with the significant P value < 0.001. Next, these ERGs were subjected to least absolute shrinkage and selection operator (LASSO) regression to exclude overfitting genes and select more robust biomarkers [23,24]. As a result, 4 ERGs were selected and identified as the prognostic biomarkers of UCEC (P < 0.05). Finally, multivariate Cox regression was performed to establish a risk score system. The risk score was calculated as follows: risk score = Coefi × Expi, where the Coef represents the coefficient calculated by multivariate Cox regression, and the Exp represents the expression level of 4 hub ERGs.
The test group was further classified into high risk and low risk subgroups based on the median risk score of the training group. There were totally 256 samples of the high-risk group and 267 samples of the low-risk group in our study. Overall survival (OS) of UCEC patients between the high and low risk groups was compared by Kaplan-Meier survival analysis and log-rank test using "survival" and "survminer" R packages (https://CRAN.R-project.org/package=survival, https://rpkgs.datanovia.com/survminer/index.html). In addition to OS analysis, we grouped all patients according to their clinical characteristics (age ≤ 60 or > 60, grade I-II or III, stage I-II or III-IV) and compared their survival between high and low risk groups, respectively. We also analyzed the association of the 4 hub ERGs with OS.
Receiver Operating Characteristic (ROC) curves were plotted to indicate the value of the constructed risk model in predicting survival at 1, 3 and 5 years using "timeROC" R package [25]. The predictive efficacy of the risk model and clinical variables (age, grade and stage) was compared using the area under the ROC curve (AUC). To determine whether the risk signature was an independent prognostic predictor, we employed univariate and multivariate regression analyses with the risk score and clinical factors. Principal component analysis (PCA) and t-distributed stochastic neighbor embedding (t-SNE), linear and non-linear dimensionality reduction methods, respectively, were performed by "Rtsne" (https://github.com/jkrijthe/Rtsne) and "ggplot2" R packages to detect the transcriptomic heterogeneity, thereby further confirming the feasibility of the risk model [21,26].
Nomograms are popular prognostic tools which can integrate multiple clinical and biological prognostic factors to generate an individualized probability of a certain clinical event [27]. Nomograms based on the risk, age, grade and stage were developed to predict the 1-, 3- and 5-year survival probabilities of UCEC patients using "survival, " "rms" and "regplot" R packages (https://hbiostat.org/R/rms, https://cran.r-project.org/web/packages/regplot/index.html). The calibration plot demonstrated the consistency between the nomogram predicted OS and observed OS at 1, 3 and 5 years. The concordance index (C-index) curve was plotted to estimate the predictive consistence among risk score, grade and stage using "dplyr, " "survival, " "rms" and "pec" R packages (https://dplyr.tidyverse.org) [28].
Gene Set Enrichment Analysis (GSEA) Version 4.2.1 is freely available software that analyzes genes with common biological characteristics, such as function, chromosomal location or regulation [29]. By estimating enrichment score (ES), assessing significance, adjusting for multiple hypothesis testing and ultimately calculating an FDR q value, it can identify the distribution of gene sets in phenotypic distinction on the gene expression level. We conducted GESA to distinguish significantly enriched KEGG and GO pathways between high and low risk groups. If the ES > 0, the functional pathway was significantly enriched in the high-risk group; otherwise, it was active in the low-risk group. We took the top 10 KEGG pathways with the largest absolute value of normalized enrichment score (NES) and plotted a multi GSEA enrichment map via the "plyr, " "ggplot2, " "grid" and "gridExtra" R packages (https://github.com/pmur002/gridgraphics) [30].
To analyze the tumor microenvironment of all UCEC samples, we calculated the stromal score, immune score and ESTIMATE score to evaluate the tumor purity using the "estimate" R package [31]. The higher the tumor microenvironment score is, the lower the tumor purity. In addition, we visualized and compared 22 tumor-infiltrating immune cells (TIICs) between high and low risk groups among all UCEC samples via the CIBERSORT algorithm [32]. By "limma, " "survival" and "survminer" R packages, we merged survival data and CIBERSORT results of all UCEC patients, and we then compared the survival probability of different TIICs between high and low risk groups. Survival curves were plotted for TIICs with P < 0.05. To further indicate the differences of immune status between high and low risk groups, single-sample gene set enrichment analysis (ssGSEA) was performed to compare the infiltrating scores of 13 immune functions and 16 immune cells via the "GSVA, " "limma" and "GSEABase" R packages (https://bioconductor.org/packages/GSEABase/) [19,33].
The R package "pRRophetic" was used to predict the drug sensitivity by estimating the half-maximal inhibitory concentration (IC50), thereby filtering out potential anti-UCEC drugs [34]. The drug sensitivity differences were compared between high and low risk groups with the significance P value < 0.001. The correlations between the risk score and drug sensitivity were evaluated by Spearman's correlation analysis. Connectivity Map (CMap) (https://clue.io) is a database which collects gene expression profiles of human cells exposed to various small molecules. It connects genes, drugs and diseases by comparing drug-specific gene expression profiles with a disease-specific gene signature via nonparametric rank-based Kolmogorov-Smirnov test [35]. We uploaded the query files of the differentially expressed genes between high and low risk groups in an effort to identify candidate drugs for UCEC.
Strawberry Perl (Version 5.32.1 for Windows 64-bit) was used to prepare the data. All statistical analyses were performed using R software (version 4.2.1 for Windows 64-bit). The "limma" R package and Wilcoxon test were used to identify differently expressed genes between tumor and normal tissues. Wilcoxon test was also employed to compare 22 TIICs' proportions between two risk groups. Spearman's correlation analysis was applied to evaluate the association between risk score and drug sensitivity. P < 0.05 was considered statistically significant unless otherwise specified.
The flow diagram is exhibited in Figure 1. 351 DEERGs were identified among 554 UCEC samples and 35 normal samples with│Log2 FC│ > 1 and FDR < 0.05. Of them, 202 were upregulated with log2FC > 1, and 149 were downregulated with log2FC < -1 (Figure 2A). The expression of the top 50 DEERGs is shown in the heat map (Figure 2B). The GO term enrichment analysis showed that the 351 DEERGs mainly took part in the biological processes of cellular divalent inorganic cation homeostasis, calcium ion homeostasis and transport and divalent metal ion transport, whose cellular component was mainly endoplasmic reticulum lumen, and the molecular functions were mainly passive transmembrane transporter activity and channel activity (Figure 2C). KEGG pathway analysis showed these DEERGs were significantly associated with the pathways of neurodegeneration and multiple disease (Figure 2D).
The 351 DEERGs were subjected to univariate Cox regression analysis in the training group, and 7 of them were shown to be significantly associated with OS of UCEC patients with P < 0.001 (Figure 3A). Then, the 7 genes were introduced into LASSO Cox regression analysis to identify the most predictive gene. Consequently, 4 genes were obtained to build the prognostic model: namely, ATPase secretory pathway Ca2+ transporting 2 (ATP2C2), cold inducible RNA binding protein (CIRBP), cysteine rich with EGF like domains 2 (CRELD2) and dopamine receptor D2 (DRD2) (Figure 3B, C). Multivariate Cox regression analysis of the 4 genes was performed to create the risk signature (Table 1). Ultimately, the risk score = (0.7845*expression value of DRD2) + (-0.6562*expression value of CIRBP) + (-0.4710 *expression value of CRELD2) + (-0.4241*expression value of ATP2C2). Among the 4 genes, DRD2 was a risk factor for UCEC patients' prognosis with hazard ratio (HR) > 1, while the remaining 3 genes were protective factors. We further explored the association between the expression levels of the 4 genes with survival in all UCEC cases. The survival curves illustrated that high expression of DRD2 was correlated with poor OS, but high expression of CIRBP, CRELD2 and ATP2C2 contributed to favorable OS (Figure 3D–G), which was consistent with the multivariate Cox regression analysis.
ID | Coefficient | HR | HR.95L | HR.95H | P value |
DRD2 | 0.7845 | 2.1912 | 1.2044 | 3.9864 | 0.0102 |
CIRBP | -0.6562 | 0.5188 | 0.3163 | 0.8512 | 0.0094 |
CRELD2 | -0.4710 | 0.6244 | 0.4004 | 0.9736 | 0.0377 |
ATP2C2 | -0.4241 | 0.6544 | 0.4418 | 0.9691 | 0.0343 |
HR: hazard ratio, HR.95L: lower 95% confidence interval of HR, HR.95H: higher 95% confidence interval of HR. |
All the UCEC cases were classified into high and low risk groups according to the median risk score of the training group; thereby, there were 131 and 132 cases of high and low risk, respectively, in the training group and 125 and 135 cases of high and low risk, respectively, in the test group. The high-risk group showed reduced OS whether in the entire, test or training group (Figure 3H–J). The risk score distribution, survival status of each UCEC patient and expression heatmap of 4 hub genes in the entire, test and training groups are also shown in Figure 3K–M respectively. The expression heatmap displayed the upregulation of DRD2 and the downregulation of ATP2C2, CIRBP and CRELD2 in the high-risk group in both the test and training sets, which totally coincided with the above mentioning that DRD2 may be a risk factor.
We performed a univariate Cox regression algorithm on the entire group to identify potential prognostic factors, including age, grade, stage and risk score. As shown in Figure 4A, they were all potential risk factors for unfavorable clinical outcome of UCEC (P < 0.05). To explore the prognostic independence of risk score, we applied multivariate Cox regression analysis. It turned out that risk signature was still an independent survival predictor (P < 0.05, HR > 1) (Figure 4B). We further classified UCEC patients according to their clinical characteristics to evaluate the prognostic value of the risk signature. Patients with low risk score showed better OS (all P < 0.05), and the trends exhibited high consistence between patients with age ≤ 60 and > 60, grade I-II and III and stage I-II and III-IV (Figure 4C–E), which reflected the solid prognostic feasibility of the risk score.
Time dependent ROC curves further elucidated that the AUC values of risk score for predicting 1, 3, and 5-year survival in the training group were 0.807, 0.793 and 0.769, respectively; and those in the test group were 0.695, 0.652 and 0.664, respectively, indicating that the ER stress related risk model processed moderate prognostic accuracy in UCEC (Figure 4F–H). Compared with age, grade and stage, the AUC of risk score was the highest and reached 0.736, showing the better prognostic sensitivity and specificity of risk score compared to these clinical factors (Figure 4I). Also, the C-indexes among risk score, grade and stage were satisfactory, about 0.7, which also reflected the accuracy of the risk model (Figure 4J). To predict the 1, 3, and 5-year survival probability of each specific UCEC patient, we constructed a nomogram with clinical variables and risk score (Figure 4K). The higher the total points were, the poorer the prognosis. The calibration curve showed the nomogram predicted 1, 3, 5-year OS highly matched the observed OS (Figure 4L). PCA and t-SNE plots indicated that the gene expression patterns between high and low risk groups were clearly separated after dimensionality reduction (Supplementary Figure 1). All of the above confirmed that the ERG-related risk model was a powerful and reliable outcome predictor of UCEC.
To investigate the functions of differentially expressed genes between high and low-risk groups, we performed GSEA based on gene expression level. The larger the│NES│and the smaller the FDR q value are, the higher the indicated reliability of the enrichment analysis results. In the high-risk group, KEGG enrichment analysis showed highly expressed genes were mainly enriched in the extracellular matrix (ECM) receptor interaction (NES = 2.014, FDR = 0.127), axon guidance (NES = 1.935, FDR = 0.150) and dilated cardiomyopathy pathway (NES = 1.921, FDR = 0.113) (Figure 5A). In the low-risk group, they were enriched in alpha linolenic acid metabolism (NES = -2.169, FDR = 0.005), fatty acid metabolism (NES = -2.141, FDR = 0.004) and ether lipid metabolism (NES = -2.136, FDR = 0.003) (Figure 5B). Table 2 elucidates the top 2 enriched GO terms in BP, MF and CC of differentially expressed genes between high and low risk groups. Highly expressed genes of the high-risk group were enriched in nerve development and voltage gated sodium channel activity, while those of the low-risk group were mainly enriched in cilium movement (Table 2).
Group | Name | Size | ES | NES | FDR |
High-risk group | GOBP-nerve development | 81 | 0.588 | 2.232 | 0.099 |
GOBP-positive regulation of synapse assembly | 62 | 0.652 | 2.202 | 0.077 | |
GOMF-voltage gated sodium channel activity | 25 | 0.715 | 2.147 | 0.047 | |
GOMF-GABA receptor activity | 22 | 0.733 | 2.111 | 0.032 | |
GOCC-voltage gated channel complex | 17 | 0.791 | 2.188 | 0.047 | |
GOCC-presynaptic membrane | 142 | 0.541 | 2.097 | 0.034 | |
Low-risk group | GOBP-cilium movement | 179 | -0.711 | -2.308 | 0.002 |
GOBP-sperm motility | 119 | -0.645 | -2.259 | 0.005 | |
GOMF-oxidoreductase activity acting on a sulfur group of donors | 58 | -0.617 | -2.129 | 0.016 | |
GOMF-nucleoside diphosphate kinase activity | 18 | -0.759 | -2.088 | 0.022 | |
GOCC-motile cilium | 223 | -0.678 | -2.339 | 0.003 | |
GOCC-9plus2 motile cilium | 146 | -0.658 | -2.310 | 0.003 | |
GO: gene ontology, BP: biological process, MF: molecular function, CC: cellular component, Size: the number of genes enriched, ES: enrichment score, NES: normalized enrichment score, FDR: false discovery rate. |
In order to estimate the immune statuses of the two risk groups, we calculated tumor immune microenvironment scores. As shown in Supplementary Figure 2, there was no significant difference between the two groups in stromal score, immune score and ESTIMATE score, indicating the similar tumor purities between different risk groups. We further compared 22 TIICs via CIBERSORT. The results showed the fractions of CD8+ T cells, regulatory T cells (Tregs) and resting dendritic cells in the low-risk group were significantly higher than those of the high-risk group (P < 0.05) (Figure 6A). Conversely, the M1 macrophages and activated dendritic cells (aDCs) showed higher infiltration in the high-risk group (P < 0.05) (Figure 6A). Immune function analysis showed type I interferon (IFN) response, major histocompatibility complex (MHC) class I, antigen presenting cell (APC) co-inhibition and parainflammation were significantly upregulated in the high-risk group (P < 0.05), while type II IFN response and T cell co-stimulation were more active in the low-risk group (P < 0.05) (Figure 6B). We further conducted ssGSEA for immunocytes and immune functions, and the results showed high consistence with those of CIBERSORT. The aDCs were more abundant in high-risk cases (P < 0.001), while immature dendritic cells (iDCs), neutrophils and T helper cells were significantly enriched in the low-risk group (P < 0.05) (Figure 6C). The immune function analysis results of ssGSEA totally accorded with those of CIBERSORT (Figure 6D). We further investigated the relationship between 22 TIICs and survival. The Kaplan-Meier survival curves showed that UCEC patients with low infiltration of plasma cells and aDCs had more favorable OS (P < 0.05) (Figure 6E, I). Highly enriched Tregs, CD8+ T cells and activated neutral killer cells were related with favorable OS (P < 0.05) (Figure 6F–H), which might be potential immunotherapy targets.
To further explore the instructional significance of the risk model for clinical treatment, we analyzed the differences in drug sensitivity between the two risk groups by estimating the IC50. As shown in Figure 7, the IC50 of the drugs, such as cisplatin, cetuximab, bortezomib and navitoclax, were negatively correlated with risk score (P < 0.001). Patients with high-risk scores were more sensitive to these drugs, indicating they might be effective anti-UCEC drugs. We further analyzed the small molecular drugs that were sensitive to differentially expressed genes between high and low risk groups via CMap. Table 3 exhibits the top 10 potential sensitive drugs.
Drug name | MOA | Raw-CS | -log10(FDR) | Norm-CS |
Leflunomide | Dihydroorotate dehydrogenase inhibitor, PDGFR inhibitor | -0.5523 | 15.6536 | -1.8867 |
Pentoxifylline | Phosphodiesterase inhibitor | -0.5303 | 15.6536 | -1.8117 |
Methiopril | ACE inhibitor | -0.5109 | 15.6536 | -1.7452 |
Pterostilbene | Cyclooxygenase inhibitor, PPAR receptor agonist | -0.5109 | 15.6536 | -1.7453 |
Tetramethylsilane | Internal standard for NMR spectroscopy | -0.5057 | 15.6536 | -1.7275 |
Fostamatinib | Syk inhibitor | -0.5007 | 15.6536 | -1.7105 |
Ebelactone-b | Lipase inhibitor | -0.5001 | 15.6536 | -1.7085 |
Tipifarnib-P2 | Farnesyltransferase inhibitor | -0.4968 | 15.6536 | -1.6971 |
Medroxyprogesterone-acetate | Progesterone receptor agonist | -0.4951 | 15.6536 | -1.6914 |
CO-102862 | Sodium channel inhibitor | -0.495 | 15.6536 | -1.6912 |
MOA: mechanism of action, CS: connectivity score, FDR: false discovery rate, Norm-CS: normalized connectivity score, PDGFR: platelet-derived growth factor receptor, ACE: angiotensin converting enzyme, PPAR: peroxisome proliferator activated receptor, NMR: nuclear magnetic resonance. |
Due to a growing and ageing population, uterus cancer is predicted to account for 5% of all female cancers in the UK in 2035 with 11,576 cases, a 24.17% increase over 2014 [36]. Given the large number of surviving UCEC patients, survivorship and follow-up to improve their prognosis are crucial [37]. However, there has not been much advancement in UCEC biomarkers or treatment in the last few decades, particularly for advanced and recurrent cases [38]. The treatment of UCEC is multimodal, including accurate surgical staging, proper systemic adjuvant therapies and molecular targeted therapy. Tyrosine kinase inhibitors (TKIs) and immunotherapy have emerged as new treatment avenues for UCEC [38]. ER plays an essential role in calcium homeostasis, lipid biosynthesis and the synthesis and folding of nearly a third of the protein. ER stress occurs when its ability to fold proteins or dispose of unfolded proteins is compromised, subsequently influencing the cellular function and fate [39]. Numerous studies have proposed that dysregulated UPR secondary to ER stress is involved in tumorigenesis and progression [40,41]. However, ER stress in UCEC has been rarely investigated.
The present study identified DEERGs between UCEC and normal samples and constructed a 4-ERG risk model to predict prognosis and instruct treatment via LASSO regression analysis. Nowadays, gene signatures have been an effective tool to forecast prognosis and assist clinical decision making. ER stress related prognostic risk models have been constructed in various cancers, such as esophageal cancer [42], diffuse glioma [43,44] and hepatocellular cancer [45], but with few reports in UCEC. The risk signature constructed in our present study was validated to possess considerable prognostic accuracy and feasibility. In addition, we constructed a nomogram integrating the risk score and clinical variables, which could effectively predict the 1, 3 and 5-year survival probability of UCEC patients. In addition, we explored the difference of immune infiltration between different risk groups, thereby providing reference for immunotherapy in UCEC. We analyzed the drug sensitivity and screened the sensitive drugs for UCEC patients with different risk scores. Our established signature may serve as a novel biomarker for personalized treatment and ideal follow-up for UCEC patients.
In our study, 4 DEERGs were identified by LASSO Cox regression analysis: namely, ATP2C2, CIRBP, CRELD2 and DRD2. High expression of DRD2 was associated with poor outcome, while the other three were just the opposite (Figure 3D–G). Combined with the results of multivariate Cox regression, DRD2 is a risk factor for UCEC patients' survival. The controversial roles of the 4 genes in various types of cancers have been extensively investigated. For example, in the aspect of etiology of tumors, DRD2 polymorphisms were reported to correlate with reward-motivated behaviors, such as smoking and obesity [46], which may be adverse exposure factors for cancers. Gemignani et al. reported DRD2 polymorphisms could modulate the risk of colorectal cancer [47]. Tan et al. found that higher expression of DRD2 correlated with better survival and could inhibit the tumorigenesis in breast cancer [48], which seems inconsistent with the role in UCEC. Nevertheless, it was reported that DRD2 inhibitor might be a potential therapeutic target in neuroendocrine tumor, prostate cancer and glioblastoma [49,50]. Similarly, as shown in our study, DRD2 may be an adverse factor for the prognosis of UCEC. ATP2C2 is involved in calcium ion homeostasis and was reported to be related to the development and unfavorable OS of breast cancer [51]. CIRBP was reported to negatively correlate with the prognosis of pancreatic ductal adenocarcinoma patients. Inhibiting CIRBP could hinder the tumor progression and enhance treatment sensitivity [52]. CRELD2 was also involved in the progression of breast cancer and could serve as a potential therapeutic target [53]. However, in our multivariate Cox regression, the HRs of ATP2C2, CIRBP and CRELD2 were all below 1, and their high expressions were correlated with better survival, indicating that they may be protective factors for UCEC. Further studies are still needed to investigate their exact roles in UCEC.
Individualized immunotherapy according to molecular classification has been an emerging therapeutic direction for UCEC [54]. The U. S. Food and Drug Administration has approved a series of immunotherapy options, such as pembrolizumab and dostarlimab, to be used in advanced or recurrent UCEC patients with mismatch repair deficient [54,55]. These drugs work by blocking the inhibition of programmed death 1 on T cells. A number of immune-related gene signatures have been established in UCEC with the attempt to shed light on immunotherapy [56,57,58]. In our study, we analyzed the difference of 22 TIICs between different risk groups. CD8+ T cells and Tregs were more abundant in the low-risk group, which may be related to better OS of the low-risk group, but previous studies reported controversial results [59,60]. Conversely, aDCs were active in the high-risk group and associated with unfavorable OS. Therefore, a higher TIIC infiltration status may indicate a better prognosis. Strikingly, the TIICs can also predict the response to immunotherapy. UCEC subtypes with high abundance of TIICs may have active anti-tumor response and respond better to immunotherapy [61]. Thus, our risk signature may indirectly predict the response to immunotherapy, but how ER stress influences the anti-tumor immune response in UCEC remains to be elucidated [4].
By R packages and CMap, we investigated potential sensitive anti-UCEC drugs. Notably, among the drugs sensitive to the high-risk group, we mainly screened out several kinds of drugs: TKIs, such as dasatinib, foretinib, and saracatinib; monoclonal antibodies, such as cetuximab; and cell cycle blockers, such as RO-3306 and TAE684 (Figure 7). TKIs are important components in the combination therapy of UCEC. Wang et al. used sunitinib, a multitargeted TKI, prior to radiotherapy and found its radiosensitization activity in endometrial cancer cell lines [62]. A phase 3 clinical trial found that lenvatinib, also a multitargeted TKI, plus pembrolizumab contributed to better prognosis than chemotherapy in advanced UCEC patients [63]. Therefore, our model can relatively accurately predict the sensitive drugs and have implications for treatment. Appropriate treatment should be based on correct clinical stages, pathological grades and molecular classification. More clinical trials and practice are warranted to verify the synergistic effect of combination therapy strategies in UCEC.
However, it must be noted that there are several gaps in our present study. The first is that our data was completely sourced from the TCGA database, so the sample size might be too small to find statistical significance. In addition, the feasibility of the prognostic signature was short of further validation by external databases as well as molecular experiments. What's more, the crosstalk between ER stress and tumor immune microenvironment needs more in-depth molecular mechanism exploration.
In conclusion, although further certification is required, our present study proposed a novel ER stress-related risk model, which has been proven not only to have considerable prognostic capacity but also to offer exciting reference for UCEC immunotherapy and drug treatment, thus improving the prognosis of UCEC patients. In the future, we will further investigate its prognostic value and the underlying mechanism by clinical samples and in vitro molecular experiments.
Thanks to the TCGA project for providing the original data.
The authors declare there is no conflict of interest.
[1] | Bårdsen G, Eitrheim Ø, Jansen ES, et al. (2005) The Econometrics of Macroeconomic Modelling. Oxford University Press, Oxford. |
[2] |
Bårdsen G, Fisher PG (1999) Economic Theory and Econometric Dynamics in Modelling Wages and Prices in the United Kingdom. Empir Econ 24: 483–507. https://doi.org/10.1007/s001810050068 doi: 10.1007/s001810050068
![]() |
[3] | Bårdsen G, Fisher PG, Nymoen R (1998) Business Cycles: Real Facts or Fallacies? In: Strøm, S. (ed.), Econometrics and Economic Theory in the 20th Century: The Ragnar Frisch Centennial Symposium, Cambridge: Cambridge University Press, 499–527. https://doi.org/10.1017/CCOL521633230.016 |
[4] | Bårdsen G, Jansen ES, Nymoen R (2003) Econometric Inflation Targeting. Econom J 6: 429–460. |
[5] |
Bårdsen G, Klovland JT (2000) Shaken or Stirred? Financial Deregulation and the Monetary Transmission Mechanism in Norway. Scand J Econ 102: 563–583. https://doi.org/10.1111/1467-9442.00215 doi: 10.1111/1467-9442.00215
![]() |
[6] |
Bårdsen G, Nymoen R (2003) Testing Steady-State Implications for the NAIRU. Rev Econ Stat 85: 1070–1075. https://doi.org/10.2139/ssrn.223428 doi: 10.2139/ssrn.223428
![]() |
[7] | Bårdsen G, Nymoen R (2009) Macroeconometric Modelling for Policy. In: Mills, T., Patterson, K. (eds.), Palgrave Handbook of Econometrics, London: Palgrave Mac-Millan, 851–916. |
[8] | Bårdsen G, Nymoen R (2022) Documentation of Norwegian Aggregate Model, NAM. Available from: Https://normetrics.no/nam/. |
[9] | Bjertnæs GHM, von Brasch T, Cappelen Å, et al. (2021) COVID-19, tapt verdiskaping og finanspolitikkens rolle. Utredning for Koronakommisjonen. RAPPORTER/REPORTS 2021/13, Statistisk sentralbyrå, Statistics Norway, Oslo-Kongsvinger. |
[10] | Blytt JP, Bougroug A, Sletten P (2022) Økonomisk utvikling gjennom Covid19. En oppdatert sammenligning av Norge, Sverige og Danmark. RAPPORTER/REPORTS 2022/14, Statistisk sentralbyrå, Statistics Norway, Oslo-Kongsvinger. |
[11] |
Castle L, Doornik JJ, Hendry D (2012) Model Selection When There are Multiple Breaks. J Econom 169: 239–247. https://doi.org/10.1016/j.jeconom.2012.01.026 doi: 10.1016/j.jeconom.2012.01.026
![]() |
[12] | Clements MP, Hendry DF (1998) Forecasting Economic Time Series. Cambridge University Press, Cambridge. |
[13] | Doornik JA (2009) Autometrics. In: Castle, J., Shephard, N (eds.), The Methodology and Practice of Econometrics, Oxford: Oxford University Press. 88–121. |
[14] | Doornik JA, Hendry DF (2018a) Empirical Econometric Modelling PcGive 15. Volume 1. Timberlake Consultants, London. |
[15] | Doornik JA, Hendry DF (2018b) Modelling Dynamic Systems PcGive 15. Volume 2. Timberlake Consultants, London. |
[16] |
Engle RF (1982) Autoregressive Conditional Heteroscedasticity with Estimates of the Variance of United Kingdom Inflation. Econometrica, 50: 987–1007. https://doi.org/10.2307/1912773 doi: 10.2307/1912773
![]() |
[17] |
Engle RF, Yoo BS (1987) Forecasting and Testing in Co-integrated Systems. J Econom 35: 143–159. https://doi.org/10.1016/0304-4076(87)90085-6 doi: 10.1016/0304-4076(87)90085-6
![]() |
[18] | Epprect C, Guegan D, Veiga A (2013) Comparing variable selection techniques for linear regression: LASSO and Autometrics. Tech. rep., Centre d'Economie de la Sorbonne. |
[19] | Granger CWJ (1966) The Typical Spectral Shape of an Economic Variable. Econometrrica 34: 150–161. |
[20] | Granger CWJ (1992) Fellow's Opinion: Evaluating Economic Theory. Journal of Econometrics 51: 3–5. |
[21] | Granger CWJ, Newbold P (1986) Forecasting Economic Time Series. Academic Press, San Diego. |
[22] | Harvey AC (1981) The Econometric Analysis of Time Series. Philip Allan, Oxford. |
[23] |
Hendry DF (1995) Econometrics and Business Cycle Empirics. Econ J 105: 1622–1636. https://doi.org/10.2307/2235123 doi: 10.2307/2235123
![]() |
[24] | Hendry DF, Doornik JA (2014) Empirical Model Discovery and Theory Evaluation. Automatic Selection Methods in Econometrics. Arne Ryde Memorial Lectures. MIT Press, Cambridge, Mass. |
[25] |
Hendry DF, Johansen S, Santos C (2008) Automatic Selection of Indicators in a Fully Saturated Regression. Comput Stat, 23: 317–335 and Erratum 337–339. https://doi.org/10.1007/s00180-007-0054-z doi: 10.1007/s00180-007-0054-z
![]() |
[26] | Hendry DF, Pagan AR, Sargan JD (1984) Dynamic Specification. In: Griliches, Z.M.D.I. (ed.), Handbook og Econometrics, vol. Ⅱ, chap. 18. North-Holland. |
[27] |
Jarque CM, Bera AK (1980) Efficient Tests for Normality, Homoscedasticity and Serial Independence of Regression Residuals. Econ Lett 6: 255–259. https://doi.org/10.1016/0165-1765(80)90024-5 doi: 10.1016/0165-1765(80)90024-5
![]() |
[28] | Johansen S (2004) Cointegration: An overview. Manuscript, Unversity of Copenhagen, Department of Applied Mathematics and Stastiics. |
[29] | Johansen S, Nielsen B (2009) Analysis of the Indicator Saturation Estimator as a Robust Regression Estimator. In: Castle, J.L., Shephard, N. (eds.), The Methodology and Practise of Econometrics, Oxford: Oxford University Press. |
[30] |
Kolsrud D, Nymoen R (2014) Macroeconomic Stability or Cycles? The Role of the Wage-Price Spiral. Aust Econ Pap 53: 41–68. https://doi.org/10.1111/1467-8454.12020. doi: 10.1111/1467-8454.12020
![]() |
[31] |
Muhammadullah S, Urooj A, Kahn F, et al. (2022) Comparison of Weighted Lag Adaptive LASSO with Autometrics for Covariate Selection and Forecasting Using Time-Series Data. Complexity, 1–10. https://doi.org/10.1155/2022/2649205 doi: 10.1155/2022/2649205
![]() |
[32] |
Nymoen R (1989a) Modelling Wages in the Small Open Economy: An Error-Correction Model of Norwegian Manufacturing Wages. Oxf Bull Econ Stat 51: 239–258. https://doi.org/10.1111/j.1468-0084.1989.mp51003002.x doi: 10.1111/j.1468-0084.1989.mp51003002.x
![]() |
[33] |
Nymoen R (1989b) Wages and the Length of the Working Day. A Empirical Test Based on Norwegian Quarterly Manufacturing Data. Scand J Econ 91: 599–612. https://doi.org/10.2307/3440087 doi: 10.2307/3440087
![]() |
[34] |
Nymoen R (1991) A Small Linear Model of Wage- and Price-Inflation in the Norwegian Economy. J Appl Econ 6: 255–269. https://doi.org/10.1002/jae.3950060304 doi: 10.1002/jae.3950060304
![]() |
[35] |
Nymoen R (2017) Between Institutions and Global Forces: Norwegian Wage Formation Since Industrialisation. Econometrics 5: 1–54. https://doi.org/10.3390/econometrics5010006. doi: 10.3390/econometrics5010006
![]() |
[36] | Nymoen R (2019) Dynamic Econometrics for Empirical Macroeconomic Modelling. World Scientific, Boston. |
[37] | Nymoen R (2021) The role of wage formation in empirical macroeconometric models., Oxford Research Encyclopedia of Economics and Finance. Oxford University Press, 1–29. Https://doi.org/10.1093/acrefore/9780190625979.013.641 |
[38] |
Padhan R, Prabheesh HP (2021) The Economics of COVID-19 pandemic: A Survey. Econ Anal Policy 70: 220–237. https://doi.org/10.1016/j.eap.2021.02.012 doi: 10.1016/j.eap.2021.02.012
![]() |
[39] |
Pesaran MH, Smith RP (2016) Counterfactual analysis in macroeconometrics: An empirical investigation into the effects of quantitative easing. Res Econ 70: 262–280. https://doi.org/10.1016/j.rie.2016.01.004 doi: 10.1016/j.rie.2016.01.004
![]() |
[40] | Rio-Chanona RM, Mealy P, Pichler A, et al. (2020) Supply and demand shocks in the COVID-19 pandemic: an industry and occupation perspective. Oxford Review of Economic Policy 36: S94–S137. |
[41] |
Rungcharoenkitkul P (2021) Macroeconomic effects of Covid-19: a mid-term review. Pac Econ Rev 20: 439–458. https://doi.org/10.1111/1468-0106.12372 doi: 10.1111/1468-0106.12372
![]() |
[42] | Spanos A (2021) Methodology of Macroeconometrics. Oxford Research Encyclopedias. Https://doi.org/10.1093/acrefore/9780190625979.013.175. |
[43] |
Susskind D, Vines D (2020) The economics of the COVID-19 pandemic: an assessment. Oxford Rev Econ Policy 36: S1–S13. https://doi.org/10.1093/oxrep/graa036 doi: 10.1093/oxrep/graa036
![]() |
[44] |
Vines D, Wills S (2020) The Rebuilding of Macroeconomic Theory Project Part Ⅱ:Multiple Equilbria, Toy Models and Policy Models in a New Macroeconomic Paradigm. Oxford Rev Econ Policy 36: 427–497. Https://doi.org/10.1093/oxrep/graa066. doi: 10.1093/oxrep/graa066
![]() |
[45] | Visco I (2005) From Theory to Practice in Macroeconomic Models: Post Keynesian Eclecticism. BNL Q Rev LVIII(233-234): 67–90. |
[46] | von Brasch T, Cappelen Å, Holden S, et al. (2022) COVID-19, tapt verdiskaping og finanspolitikkens rolle. Utredning for Koronakommisjonen. RAPPORTER/REPORTS 2022/15, Statistisk sentralbyrå, Statistics Norway, Oslo-Kongsvinger. |
[47] | Wallis KF (1977) Multiple time series analysis and the final form of econometric models. Econometrica 45: 1481–97. |
1. | Shanshan Lin, Changqiang Wei, Yiyun Wei, Jiangtao Fan, Construction and verification of an endoplasmic reticulum stress-related prognostic model for endometrial cancer based on WGCNA and machine learning algorithms, 2024, 14, 2234-943X, 10.3389/fonc.2024.1362891 | |
2. | Xunqi Zhang, Yanxiao Han, Chenliu Fan, Yang Jiang, Wen Jiang, Chengyun Zheng, Epigallocatechin gallate induces apoptosis in multiple myeloma cells through endoplasmic reticulum stress induction and cytoskeletal disruption, 2024, 141, 15675769, 112950, 10.1016/j.intimp.2024.112950 | |
3. | Daniel A. Lujan, Joey L. Ochoa, Ellen J. Beswick, Tamara A. Howard, Helen J. Hathaway, Nora I. Perrone-Bizzozero, Rebecca S. Hartley, Cold-Inducible RNA Binding Protein Impedes Breast Tumor Growth in the PyMT Murine Model for Breast Cancer, 2024, 12, 2227-9059, 340, 10.3390/biomedicines12020340 | |
4. | Shohei Hinaga, Mahmoud Kandeel, Kentaro Oh-hashi, Molecular characterization of the ER stress-inducible factor CRELD2, 2024, 82, 1085-9195, 1463, 10.1007/s12013-024-01300-1 | |
5. | Yingping Zhu, Wei Xu, Yuanfang He, Wenjuan Yang, Siyue Song, Chengping Wen, Therapeutic implications of endoplasmic reticulum stress gene CCL3 in cervical squamous cell carcinoma, 2025, 41, 1573-6822, 10.1007/s10565-024-09949-3 |
ID | Coefficient | HR | HR.95L | HR.95H | P value |
DRD2 | 0.7845 | 2.1912 | 1.2044 | 3.9864 | 0.0102 |
CIRBP | -0.6562 | 0.5188 | 0.3163 | 0.8512 | 0.0094 |
CRELD2 | -0.4710 | 0.6244 | 0.4004 | 0.9736 | 0.0377 |
ATP2C2 | -0.4241 | 0.6544 | 0.4418 | 0.9691 | 0.0343 |
HR: hazard ratio, HR.95L: lower 95% confidence interval of HR, HR.95H: higher 95% confidence interval of HR. |
Group | Name | Size | ES | NES | FDR |
High-risk group | GOBP-nerve development | 81 | 0.588 | 2.232 | 0.099 |
GOBP-positive regulation of synapse assembly | 62 | 0.652 | 2.202 | 0.077 | |
GOMF-voltage gated sodium channel activity | 25 | 0.715 | 2.147 | 0.047 | |
GOMF-GABA receptor activity | 22 | 0.733 | 2.111 | 0.032 | |
GOCC-voltage gated channel complex | 17 | 0.791 | 2.188 | 0.047 | |
GOCC-presynaptic membrane | 142 | 0.541 | 2.097 | 0.034 | |
Low-risk group | GOBP-cilium movement | 179 | -0.711 | -2.308 | 0.002 |
GOBP-sperm motility | 119 | -0.645 | -2.259 | 0.005 | |
GOMF-oxidoreductase activity acting on a sulfur group of donors | 58 | -0.617 | -2.129 | 0.016 | |
GOMF-nucleoside diphosphate kinase activity | 18 | -0.759 | -2.088 | 0.022 | |
GOCC-motile cilium | 223 | -0.678 | -2.339 | 0.003 | |
GOCC-9plus2 motile cilium | 146 | -0.658 | -2.310 | 0.003 | |
GO: gene ontology, BP: biological process, MF: molecular function, CC: cellular component, Size: the number of genes enriched, ES: enrichment score, NES: normalized enrichment score, FDR: false discovery rate. |
Drug name | MOA | Raw-CS | -log10(FDR) | Norm-CS |
Leflunomide | Dihydroorotate dehydrogenase inhibitor, PDGFR inhibitor | -0.5523 | 15.6536 | -1.8867 |
Pentoxifylline | Phosphodiesterase inhibitor | -0.5303 | 15.6536 | -1.8117 |
Methiopril | ACE inhibitor | -0.5109 | 15.6536 | -1.7452 |
Pterostilbene | Cyclooxygenase inhibitor, PPAR receptor agonist | -0.5109 | 15.6536 | -1.7453 |
Tetramethylsilane | Internal standard for NMR spectroscopy | -0.5057 | 15.6536 | -1.7275 |
Fostamatinib | Syk inhibitor | -0.5007 | 15.6536 | -1.7105 |
Ebelactone-b | Lipase inhibitor | -0.5001 | 15.6536 | -1.7085 |
Tipifarnib-P2 | Farnesyltransferase inhibitor | -0.4968 | 15.6536 | -1.6971 |
Medroxyprogesterone-acetate | Progesterone receptor agonist | -0.4951 | 15.6536 | -1.6914 |
CO-102862 | Sodium channel inhibitor | -0.495 | 15.6536 | -1.6912 |
MOA: mechanism of action, CS: connectivity score, FDR: false discovery rate, Norm-CS: normalized connectivity score, PDGFR: platelet-derived growth factor receptor, ACE: angiotensin converting enzyme, PPAR: peroxisome proliferator activated receptor, NMR: nuclear magnetic resonance. |
ID | Coefficient | HR | HR.95L | HR.95H | P value |
DRD2 | 0.7845 | 2.1912 | 1.2044 | 3.9864 | 0.0102 |
CIRBP | -0.6562 | 0.5188 | 0.3163 | 0.8512 | 0.0094 |
CRELD2 | -0.4710 | 0.6244 | 0.4004 | 0.9736 | 0.0377 |
ATP2C2 | -0.4241 | 0.6544 | 0.4418 | 0.9691 | 0.0343 |
HR: hazard ratio, HR.95L: lower 95% confidence interval of HR, HR.95H: higher 95% confidence interval of HR. |
Group | Name | Size | ES | NES | FDR |
High-risk group | GOBP-nerve development | 81 | 0.588 | 2.232 | 0.099 |
GOBP-positive regulation of synapse assembly | 62 | 0.652 | 2.202 | 0.077 | |
GOMF-voltage gated sodium channel activity | 25 | 0.715 | 2.147 | 0.047 | |
GOMF-GABA receptor activity | 22 | 0.733 | 2.111 | 0.032 | |
GOCC-voltage gated channel complex | 17 | 0.791 | 2.188 | 0.047 | |
GOCC-presynaptic membrane | 142 | 0.541 | 2.097 | 0.034 | |
Low-risk group | GOBP-cilium movement | 179 | -0.711 | -2.308 | 0.002 |
GOBP-sperm motility | 119 | -0.645 | -2.259 | 0.005 | |
GOMF-oxidoreductase activity acting on a sulfur group of donors | 58 | -0.617 | -2.129 | 0.016 | |
GOMF-nucleoside diphosphate kinase activity | 18 | -0.759 | -2.088 | 0.022 | |
GOCC-motile cilium | 223 | -0.678 | -2.339 | 0.003 | |
GOCC-9plus2 motile cilium | 146 | -0.658 | -2.310 | 0.003 | |
GO: gene ontology, BP: biological process, MF: molecular function, CC: cellular component, Size: the number of genes enriched, ES: enrichment score, NES: normalized enrichment score, FDR: false discovery rate. |
Drug name | MOA | Raw-CS | -log10(FDR) | Norm-CS |
Leflunomide | Dihydroorotate dehydrogenase inhibitor, PDGFR inhibitor | -0.5523 | 15.6536 | -1.8867 |
Pentoxifylline | Phosphodiesterase inhibitor | -0.5303 | 15.6536 | -1.8117 |
Methiopril | ACE inhibitor | -0.5109 | 15.6536 | -1.7452 |
Pterostilbene | Cyclooxygenase inhibitor, PPAR receptor agonist | -0.5109 | 15.6536 | -1.7453 |
Tetramethylsilane | Internal standard for NMR spectroscopy | -0.5057 | 15.6536 | -1.7275 |
Fostamatinib | Syk inhibitor | -0.5007 | 15.6536 | -1.7105 |
Ebelactone-b | Lipase inhibitor | -0.5001 | 15.6536 | -1.7085 |
Tipifarnib-P2 | Farnesyltransferase inhibitor | -0.4968 | 15.6536 | -1.6971 |
Medroxyprogesterone-acetate | Progesterone receptor agonist | -0.4951 | 15.6536 | -1.6914 |
CO-102862 | Sodium channel inhibitor | -0.495 | 15.6536 | -1.6912 |
MOA: mechanism of action, CS: connectivity score, FDR: false discovery rate, Norm-CS: normalized connectivity score, PDGFR: platelet-derived growth factor receptor, ACE: angiotensin converting enzyme, PPAR: peroxisome proliferator activated receptor, NMR: nuclear magnetic resonance. |