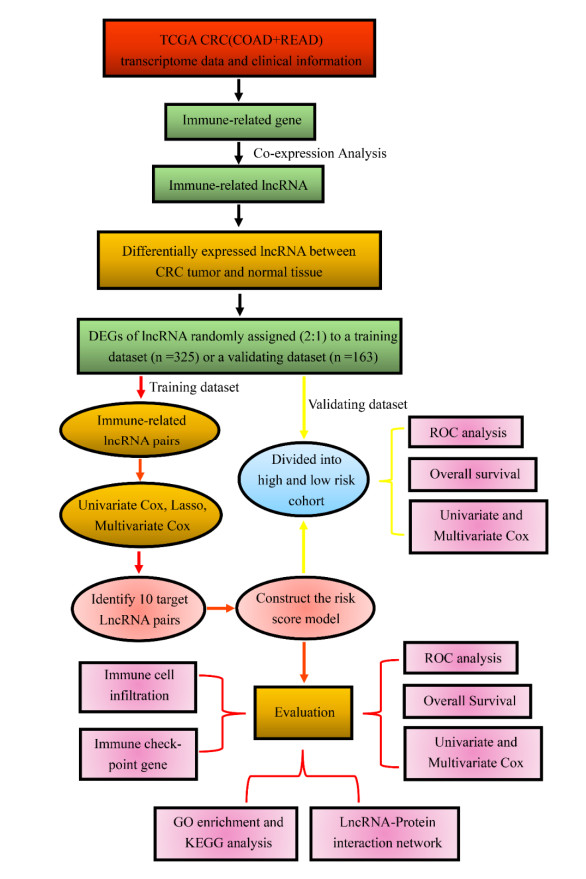
The tumor immune microenvironment of colorectal cancer (CRC) affects tumor development, prognosis and immunotherapy strategies. Recently, immune-related lncRNA were shown to play vital roles in the tumor immune microenvironment. The objective of this study was to identify lncRNAs involved in the immune response, tumorigenesis and progression of CRC and to establish an immune-related lncRNA signature for predicting the prognosis of CRC.
We used data retrieved from the cancer genome atlas (TCGA) dataset to construct a 10-gene immune-related lncRNA pair (IRLP) signature model using a method based on the ranking and comparison of paired gene expression in CRC. The clinical prognosis, immune checkpoints and lncRNA-protein networks were analyzed to evaluate the signature.
The signature was closely associated with overall survival of CRC patients (p < 0.001 in both of the training and validating cohorts) and the 3-year AUC values for the training and validating cohorts were 0.884 and 0.739, respectively. And, there were positive correlations between the signature and age (p = 0.048), clinical stage (p < 0.01), T stage (p < 0.01), N stage (p < 0.001) and M stage (p < 0.01). In addition, the signature model appeared to be highly relevant to some checkpoints, including CD160, TNFSF15, HHLA2, IDO2 and KIR3DL1. Further, molecular functional analysis and lncRNA-protein networks were applied to understand the molecular mechanisms underlying the carcinogenic effect and progression.
The 10-gene IRLP signature model is an independent prognostic factor for CRC patient and can be utilized for the development of immunotherapy.
Citation: Bin Ma, Lianqun Cao, Yongmin Li. A novel 10-gene immune-related lncRNA signature model for the prognosis of colorectal cancer[J]. Mathematical Biosciences and Engineering, 2021, 18(6): 9743-9760. doi: 10.3934/mbe.2021477
[1] | Yuan Yang, Lingshan Zhou, Xi Gou, Guozhi Wu, Ya Zheng, Min Liu, Zhaofeng Chen, Yuping Wang, Rui Ji, Qinghong Guo, Yongning Zhou . Comprehensive analysis to identify DNA damage response-related lncRNA pairs as a prognostic and therapeutic biomarker in gastric cancer. Mathematical Biosciences and Engineering, 2022, 19(1): 595-611. doi: 10.3934/mbe.2022026 |
[2] | Yun-xiang Li, Shi-ming Wang, Chen-quan Li . Four-lncRNA immune prognostic signature for triple-negative breast cancer. Mathematical Biosciences and Engineering, 2021, 18(4): 3939-3956. doi: 10.3934/mbe.2021197 |
[3] | Zehao Niu, Yujian Xu, Yan Li, Youbai Chen, Yan Han . Construction and validation of a novel pyroptosis-related signature to predict prognosis in patients with cutaneous melanoma. Mathematical Biosciences and Engineering, 2022, 19(1): 688-706. doi: 10.3934/mbe.2022031 |
[4] | Chen Zheng, Zhaobang Tan . A novel identified pyroptosis-related prognostic signature of colorectal cancer. Mathematical Biosciences and Engineering, 2021, 18(6): 8783-8796. doi: 10.3934/mbe.2021433 |
[5] | Wei Niu, Lianping Jiang . A seven-gene prognostic model related to immune checkpoint PD-1 revealing overall survival in patients with lung adenocarcinoma. Mathematical Biosciences and Engineering, 2021, 18(5): 6136-6154. doi: 10.3934/mbe.2021307 |
[6] | Changxiang Huan, Jiaxin Gao . A novel cuproptosis-related lncRNA prognostic signature for predicting treatment and immune environment of head and neck squamous cell carcinoma. Mathematical Biosciences and Engineering, 2022, 19(12): 12127-12145. doi: 10.3934/mbe.2022564 |
[7] | Yong Ding, Jian-Hong Liu . The signature lncRNAs associated with the lung adenocarcinoma patients prognosis. Mathematical Biosciences and Engineering, 2020, 17(2): 1593-1603. doi: 10.3934/mbe.2020083 |
[8] | Yan He, Nannan Cao, Yanan Tian, Xuelin Wang, Qiaohong Xiao, Xiaojuan Tang, Jiaolong Huang, Tingting Zhu, Chunhui Hu, Ying Zhang, Jie Deng, Han Yu, Peng Duan . Development and validation of two redox-related genes associated with prognosis and immune microenvironment in endometrial carcinoma. Mathematical Biosciences and Engineering, 2023, 20(6): 10339-10357. doi: 10.3934/mbe.2023453 |
[9] | Han Zhao, Yun Chen, Peijun Shen, Lan Gong . Construction and validation of a novel prognostic signature for uveal melanoma based on five metabolism-related genes. Mathematical Biosciences and Engineering, 2021, 18(6): 8045-8063. doi: 10.3934/mbe.2021399 |
[10] | Jie Chen, Jinggui Chen, Bo Sun, Jianghong Wu, Chunyan Du . Integrative analysis of immune microenvironment-related CeRNA regulatory axis in gastric cancer. Mathematical Biosciences and Engineering, 2020, 17(4): 3953-3971. doi: 10.3934/mbe.2020219 |
The tumor immune microenvironment of colorectal cancer (CRC) affects tumor development, prognosis and immunotherapy strategies. Recently, immune-related lncRNA were shown to play vital roles in the tumor immune microenvironment. The objective of this study was to identify lncRNAs involved in the immune response, tumorigenesis and progression of CRC and to establish an immune-related lncRNA signature for predicting the prognosis of CRC.
We used data retrieved from the cancer genome atlas (TCGA) dataset to construct a 10-gene immune-related lncRNA pair (IRLP) signature model using a method based on the ranking and comparison of paired gene expression in CRC. The clinical prognosis, immune checkpoints and lncRNA-protein networks were analyzed to evaluate the signature.
The signature was closely associated with overall survival of CRC patients (p < 0.001 in both of the training and validating cohorts) and the 3-year AUC values for the training and validating cohorts were 0.884 and 0.739, respectively. And, there were positive correlations between the signature and age (p = 0.048), clinical stage (p < 0.01), T stage (p < 0.01), N stage (p < 0.001) and M stage (p < 0.01). In addition, the signature model appeared to be highly relevant to some checkpoints, including CD160, TNFSF15, HHLA2, IDO2 and KIR3DL1. Further, molecular functional analysis and lncRNA-protein networks were applied to understand the molecular mechanisms underlying the carcinogenic effect and progression.
The 10-gene IRLP signature model is an independent prognostic factor for CRC patient and can be utilized for the development of immunotherapy.
Abbreviations: AJCC: American joint committee on cancer; AUC: Area under the curve; BP: Biological process; CC: Cellular component; COAD: Colon adenocarcinoma; CRC: Colorectal cancer; DEirlncRNA: Different expression immune related lncRNA; DEG: Different expression gene; GO: Gene ontology; HR: Hazard ratio; IRLP: Immune-related lncrna pair; KEGG: Kyoto encyclopedia of genes and genomes; LncRNA: Long non-coding RNA; MF: Molecular function; OS: Overall survival; PPI: Protein-protein interaction; READ: Rectum adenocarcinoma; ROC: Receiver operating characteristic; TCGA: The cancer genome atlas; TME: Tumor microenvironment
According to the latest GLOBOCAN 2018 data, colorectal cancer (CRC) is the fourth most commonly diagnosed cancer worldwide (10.2%) and the fourth cause of malignancy related death in the world [1]. Due to a lack of notable symptoms in the early stages, most of patients usually diagnosed at an advanced stage with local progression or distant metastasis. Although there has been substantial progress in the management of CRC, the five years survival down to 50%-70.4% once metastasis occurs [2]. Therefore, there is an urgent need for the development of CRC early diagnosis.
At present, the clinical TNM stage classification is most commonly used to detection of cancer stage and estimate prognosis in CRC patients. But, there still exist the problem that TNM stage system inconsistent with the five years survival in clinical [3,4]. Therefore, more effective and sensitive biomarkers are urgently needed for screening, diagnosis, prognosis in CRC. With the development of genetic testing, gene-based biomarkers have become more and more popular and improved the standard in the diagnosis and treatment of CRC [5].
Long-non coding RNAs (lncRNAs) refer to a class of transcripts with over 200 nucleotides in length and have no or limited coding protein capacity [6]. LncRNAs are involved in diverse biological processes including cell cycle, cell differentiation and myogenesis [7]. Moreover, mounting evidence suggests that the tumor microenvironment (TME) serves pivotal roles in determining tumor behavior. Recently, tumor immunotherapy using the immune system to anti-tumor response and elimination of tumor cells are breakthrough treatments for several malignancies [8]. Therefore, the regulation of immune-related gene expression appears especially important in hosting immune responses to combat cancer cells. Although a majority of the studies thus far have focused on coding genes, researchers have begun to pay more attention to lncRNAs recently [9]. For example, lncRNA MIR17HG can directly bound to PD-L1 to regulate tumor-immune microenvironment and plays an oncogenic role in CRC [10]. And lncRNAs have also been verified as potential biomarkers for many cancers [11].
In this study, we analyzed the data set of lncRNA expression in the cancer genome atlas (TCGA), and a prognostic model of 10 immune-related lncRNA pair (IRLP) associated with the clinical prognosis of CRC was identified according to bioinformatic prediction. The effect of the signature model involved in the regulation of tumor immune microenvironment and checkpoints. In addition, biological function and lncRNA-protein networks of the signature model were explored as well.
The workflow of the analyses carried out in this study is shown in Figure 1 Co-expression module and differential expression analysis were used to identify immune-related lncRNAs from TCGA-COAD and -READ data. The patients were then divided randomly into the training and validating group. Thereafter, prognostic lncRNA pairs were determined using univariate Cox, Lasso, and multivariate Cox regression analysis. From these analyses, 10 IRLPs were used to construct a signature model. The model was then evaluated using ROC analysis, overall survival (OS), univariate and multivariate Cox, immune cell infiltration, immune checkpoints, GO enrichment and KEGG analysis, as well as lncRNA-protein interaction network. Lastly, the validating group was also tested against the signature model.
The COAD-FPKM and READ-FPKM RNA-seq data and their corresponding clinical data were retrieved from TCGA database (https://portal.gdc.cancer.gov). This dataset was composed of 488 CRC tissues and 42 paired non-cancerous normal tissues. In addition, a list of immune-related genes was downloaded from the ImmPort database (https://www.immport.org/). Data containing complete OS was used to determine the correlation between immune-related lncRNAs and the corresponding prognosis of CRC.
We identified a list of immune-related genes from the ImmPort database and used the list to calculate the correlation between immune-related genes and lncRNAs from the TCGA database. LncRNAs with a correlation coefficient > 0.7 and p < 0.001 were selected for further analysis. Differential lncRNA expression between CRC and non-cancerous normal tissues was determined using the 'limma' package, and lncRNAs with |log2FC| > 1 and P value < 0.05 were considered as statistically significant. The patients were then divided randomly into the training (n = 325) and validation group (n = 163) in the ratio of 2:1. A pairwise comparison was performed between each lncRNA expression value within each sample of TCGA to obtain a score for IRLP signature. In each lncRNA pair, when the first gene exhibited a higher expression relative to the other one, the score was set to 1, otherwise was 0. Samples with a ratio of 0 and 1 less than 20% were excluded. We further carried out univariate (p < 0.01), LASSO and multivariate Cox regression analyses to select genes that were significantly associated with survival and included them in the IRLP signature. Receiver operating characteristic (ROC) curve analysis on the training dataset was used to determine the threshold risk score to divide the patients into high-risk and low-risk groups. The formula can be expressed as follows: RiskScore = ∑Ni = 1Expi*Wi, where exp is the expression value of every DEirlncRNA pair, and W is the multivariate cox regression analysis coefficient of each DEirlncRNA pair in the signature.
The pairwise comparison analysis was performed in validation group (n = 163), and then the 'limma' package was used to screened out the identified 10 immune-related lncRNA pairs from the validating group. Furthermore, multivariate Cox regression analysis and the riskscore formula were performed to work out the risk score of each patient in validating group. And the ROC, OS, univariate and multivariate cox regression analysis were performed as well.
ROC curve analysis was used to evaluate the performance of the IRLPs risk model (short for risk model) in predicting 1-, 2- and 3-years OS in the training cohort and validating cohort. Univariate and multivariate Cox proportional hazards regression analysis were employed to verify if the risk model was independent of age, gender, American Joint Committee on Cancer (AJCC) stage, T stage, N stage, and M stage as a prognostic factor. Afterwards, calibration curves and a nomogram representing the clinical parameters and the risk model were established to evaluate the risk model.
CIBERSORT is an algorithm that employs linear support vector regression to characterize the cell composition of tissues based on their gene expression profiles [12]. We used CIBERSORT (https://cibersortx.stanford.edu/) to determine the degree of infiltration of the tumor microenvironment by 22 tumor-infiltrating immune cells. The results of this analysis were used to establish the relationship between the risk model and the number of tumor-infiltrating immune cells. We further characterized the tumor microenvironment through differential gene expression analysis of immune checkpoints between the high-and low-risk groups.
Results of co-expression analysis identified 21 immune-related genes that correlated with 10 IRLPs. Gene ontology (GO) terms and Kyoto Encyclopedia of Genes and Genomes (KEGG) pathways enrichment analysis for the 21 immune-related genes was carried using DAVID Bioinformatics Resources (https://david.ncifcrf.gov/). Enrichment terms with count > 2 and p < 0.05 were selected for further analysis. The biological functional enrichments were visualized using the ggplot2 Package in R. Furthermore, we constructed and downloaded the protein–protein interaction (PPI) network of the 21 immune-related genes using the STRING database (https://string-db.org/) and along with the risk model were visualized using Cytoscape software (version 3.6.1). Ten hub genes in the network were chosen based on the MCC algorithm using cytoHubba app in Cytoscape.
Statistical analysis was carried out using R software (version 3.6.3). The R packages used in this study include: 'Limma' (version 3.46.0), 'pheatmap' (1.0.12 version), 'Survival' (3.2-7 version), 'survminer' (0.4.8 version), 'glmnet' (version 4.0-2), 'survivalROC' (version 1.0.3), 'ggpubr' (0.4.0 version), 'ComplexHeatmap' (2.6.2 version) and 'ggplot2' (version 3.3.2). Pearson correlation was used to determine the linear association between two groups. P < 0.05 was considered to be significant. Statistical significance was defined as follows: *P < 0.05, **P < 0.01, ***P < 0.001.
In this study, the TCGA CRC transcriptome data was used as an exploratory dataset (Figure 1). We used the immune-related gene list identified from ImmPort to select 1340 immune-related genes from the transcriptome data for further study. Co-expression analysis was used to select 302 immune-related lncRNAs with a Pearson correlation coefficient over 0.7. A total of 118 differentially expressed lncRNAs, including 102 up-regulated and 16 down-regulated lncRNAs, were identified from the normal and tumor tissues using edgeR. The heatmap and volcano plot of these differentially expressed lncRNAs are shown in Figures 2A, B.
After the data was randomly divided into two groups, a pairwise comparison analysis was performed in the training group. A total of 4073 lncRNA pairs were obtained based on these differentially expressed lncRNAs. Univariate Cox regression analysis and Lasso Cox regression were used to select 13 IRLPs with prognostic potential in the training group (Figures 3A, B). Finally, multivariate Cox proportional hazards modeling was employed to select 10 IRLPs that were used to construct the risk model (Figures 3C, D). We also calculated the risk score for each patient in the TCGA dataset based on the risk model. ROC curve analysis was performed to determine the threshold value (2.199) of the model, that could categorize the patients into a high- or low-risk groups (Figure 3E).
The Kaplan-Meier survival curves were used to compare the OS between the high- and low-risk groups in both the training (Figure 4A) and validating cohorts (Figure 4B). The OS of the low-risk group was significantly higher than that of the high-risk group in both cohorts. As shown in Figures 4C, D, the AUC values for the training cohort (0.842, 0.869 and 0.884 for the 1-, 2- and 3-year OS, respectively) and validating cohort (0.738, 0.690 and 0.739) showed that the risk model was able to discriminate between the high- and low-risk groups. In addition, the risk curves and scatterplots illustrated that the patients with higher risk scores had a higher risk of mortality in the training (Figures 4E, G) and validating cohorts (Figures 4G, H).
Univariate and multivariate Cox analyses were used to investigate if the 10-gene IRLPs risk model was independent of age, gender, AJCC stage, T stage, N stage, and M stage as a prognostic factor. The results showed that the risk model of univariate Cox analysis was 1.023 (Hazard Ratio (HR): 1.017-1.029, p < 0.001) and the multivariate Cox was 1.028 (HR: 1.019-1.037, p < 0.001) in the training cohort (Figures 5A, C), while the risk model of univariate and multivariate Cox analysis was 1.485 (HR: 1.210-1.822, p < 0.001) and 1.815 (HR: 1.380-2.387, p < 0.001) in the validating cohort, respectively (Figure 5B, D). On the other hand, the AUC values for the training cohort (0.842) and validating cohort (0.738) showed that the model had good discriminative ability when compared with clinical features (Figures E, F). As a result, the 10-gene IRLPs risk model can serve as an independent prognosis factor in CRC.
The correlation between the risk model and various clinical parameters was then assessed. The results showed that there were positive correlations between the risk score and age, clinical stage, T stage, N stage and M stage, while there was no relation between the risk score and patient gender (Figures 6A-F). A heatmap showing various clinical parameters differentially distributed in different cohorts is depicted in Figure 6G.
To further explore the ability of the risk model to predict the infiltration of immune cells in CRC, CIBERSORT was used to analyze the relationship between the risk score and 22 immune cells. Only Macrophages M0 infiltration was correlated with the risk model. High levels of Macrophages M0 were detected in the low-risk group (Figure 7A).
Immune checkpoints are molecules that modulate signaling pathways in the regulation of immune response and have an important role in the field of anti-cancer immunotherapy. According to the results shown in Figure 7, the expression levels of CD160, TNFSF15, HHLA2 and IDO2 were significantly higher in the low-risk group, while the levels of KIR3DL1 and PD-1 were higher in the high-risk group, although the latter had no significant difference (Figure 7B).
The GO annotation system includes three major branches: cellular component (CC), biological process (BP) and molecular function (MF). GO and KEGG enrichment analysis were performed to predict the function of the 21 immune-related genes that correlated with the 10 IRLPs. The first three of these enrichments were as follows (Figure 8A-C): Neuronal cell body, plasma membrane and receptor complex were enriched for CC; while for BP, they were signal transduction by protein phosphorylation, activin receptor signaling pathway and signal transduction; and for MF, they include growth factor binding, receptor signaling protein serine/threonine kinase activity and transmembrane receptor protein serine/threonine kinase activity. Further analysis of KEGG pathways included 31 pathways, containing Chronic myeloid leukemia, ErbB signaling pathway and Pathways in cancer (Figure 8D).
Using the 21 immune-related genes downloaded from the STRING database and the 10 IRLPs, we established a lncRNA-protein network, which consisted of 38 nodes and 102 edges (Figure 8E). Ten hub genes, which include three lncRNAs (AL157786.1, LINC01355, AF117829.1) and seven protein coding gene (PIK3CA, SOS1, CBLB, CBL, CREB1, ACVR2A and TGFBR1) were identified from the lncRNA-protein network using the MCC algorithm (Figure 8F).
CRC is one of the leading causes of cancer-related mortality in the world. Advances in CRC research has allowed the characterization of CRC into different molecular subtypes presenting with distinct clinical features [13]. On the other hand, LncRNAs have been shown to modulate cancer progression by influencing the molecular characteristics of tumors [14,15]. LncRNAs regulate various immune-related genes [16,17,18], and have recently been associated with drug resistance and immune escape in human cancers [19]. It is therefore important to establish an immune-related lncRNA signature for CRC that can be used to develop novel strategies for CRC prevention and treatment.
We built the signature model using a method based on the ranking and pairing comparison of relative gene expression. And we used data retrieved from TCGA to construct a 10-gene IRLPs signature for the prognosis of CRC. Using the model, we divided the patients into two groups with different immune microenvironment for evaluating clinical features, immune cell infiltration, immune check-point, GO enrichment, KEGG analysis and the interaction network between lncRNAs and immune-related protein coding genes.
We performed LASSO, univariate and multivariate Cox regression to select immune-related lncRNA pairs for establishing the IRLPs signature model. The model was composed of 10 immune-related lncRNA pairs containing 17 unique immune-related lncRNAs, which were overexpressed in cancer tissues compared to normal tissues. Each of the IRLPs was an independent prognostic factor for CRC patients. The model was able to categorize the CRC patients into low-risk and high-risk groups in the training and the validating cohorts and acted as an independent prognostic factor for CRC. Furthermore, ROC analysis revealed that the AUCs of the model (0.842 and 0.738 in training and validating cohorts, respectively) were higher than those of other clinical features. Although, there have been a series of methods for immune related lncRNA signature establishment, but the prognostic power of each study was different (Table 1) [20,21,22,23]. Firstly, the AUC value of our model was higher than that of models proposed in other studies. Secondly, we used a method based on the ranking and pairing comparison of relative gene expression, and this approach for constructing the model eliminates the need for data scaling and normalization when using different platforms [24,25]. Thirdly, our model contains relatively fewer lncRNAs, while maintaining a high AUC value. Therefore, considering clinical application value, the 10-gene IRLP risk signature is a reliable prognostic model with potential clinical significance.
study | database | methods | lncRNA signature | AUC (3 years) | |
Feng et al | TCGA-COAD, READ | DElncRNAs, Cox regression analysis | 8-immune related lncRNA | 0.751 | |
Meng et al | TCGA-COAD, READ | univariate analysis, Lasso and Cox regression | 16-immune-related lncRNA pair (30 lncRNAs) | 0.875 | |
Yan et al | TCGA-COAD, GEO (colon cancer) | CIBERSORT algorithm, Cox regression analysis | four-immune related lncRNA | 0.659 | |
Mei et al | TCGA-COAD | univariate and multivariate Cox regression | 14-immune related lncRNA | 0.776 | |
this study | TCGA-COAD, READ | DElncRNAs, ranking and pairing comparison, univariate Cox, Lasso and multivariate Cox regression | 10-immune-related lncRNA pair (17 lncRNAs) | 0.884 |
The CIBERSORT algorithm has high specificity and sensitivity [12], and is able to evaluate the gene expression data of 22 different types of immune cells. A recent study showed that the infiltration of tumors by M0 macrophages was associated with favorable prognosis [26], a finding that is consistent with the results from our study. And macrophages are indispensable in immunotherapy of tumor. It can remove anti-PD1 antibodies from T cells to weaken their response, when the antibodies target the PD1-PDL1 axis [27]. Cancer immunotherapy is a novel anti-cancer approach that targets immune checkpoint receptors such as PD1/PDL1 [28]. Therefore, we compared the expression of immune checkpoints between the low- and high-risk groups. The analysis showed that there was higher expression of inhibitory immune checkpoints (PD1 (no statistical significance) and KIR3DL1), and low expression of stimulatory checkpoints (CD160, HHLA2, IDO2 and TNFSF15), in the high-risk patients. The upregulation of KIR3DL1 causes a reduction in CD4+ T cell count and is associated with rapid disease progression [29]. Reduced CD160 expression impairs the function of NK cells, causes immune escape of cancer and is associated with poor outcomes in patients with hepatocellular carcinoma [30]. HHLA2 can promote tumor differentiation and increase the levels of CD8+ infiltrating lymphocytes. Downregulation of HHLA2 contributes to the immunosuppressive microenvironment and progression of ovarian cancer [31]. IDO2 acts as a stimulatory immune checkpoint to enhance the efficacy of dendritic cell-based cancer immunotherapy [32]. Finally, TNFSF15 is a member of the tumor necrosis factor family, and can influence the proliferation, activation and differentiation of immune cells [33]. Down regulation of TNFSF15 is associated with poor prognosis in various cancers [34,35,36]. Thus, the risk model might be a promising reference function for cancer immunotherapy.
KEGG enrichment analysis was carried out to predict the biological pathways involved in the regulation of CRC by the 10-gene IRLP signature. The results indicated that the gene signature was enriched in pathways such as Chronic myeloid leukemia, ErbB signaling pathway, Pathways in cancer, Insulin signaling pathway and Signaling pathways regulating pluripotency of stem cells. These pathways play key roles in the progression of CRC. For example, CARF increases the expression of MAPK8 and JUN by binding directly to their promoters, which in turn activates the ErbB signaling pathway that maintains the stemness of CRC [37]. PIK3CA and BRAF are members of the Insulin signaling pathway, and are the most common biomarkers for the genetic testing of CRC. Mutations in PIK3CA and/or BRAF in CRC are associated with poor outcome [38], and PIK3CA mutation is associated with poor prognosis among patients with curatively resected CRC [39].
To better understand the correlation between lncRNA and immune-related genes, a lncRNA-protein network was established. From this network, 10 hub genes were identified including 7 protein coding genes: PIK3CA, SOS1, CBLB, CBL, CREB1, ACVR2A and TGFBR1. These genes have been associated with CRC [39,40,41,42,43,44,45]. For example, SOS1 is a key activator and feedback node of KRAS, which is the most frequently mutated driver of CRC [46]. Downregulation of SOS1 expression can lead to decreased survival of cancer cells harboring a KRAS mutation [47]. CBL have been reported to promote the progression of colon cancer by forming a complex with MUC1 and CIN85, and increased level of CBL in the early stage of colon cancer contributes to a poor prognosis [42]. ACVR2A acts a protective factor and a decrease in its expression is crucial for cancer progression and distant metastasis and may serve as a prognostic biomarker for the patients with colon cancer [44].
Here remain several limitations. On the one hand, our study was limited by its retrospective nature. Further prospective clinical researches are needed to validate these results. On the other hand, we only performed enrichment analysis and made assumptions regarding the function of 17 immune related lncRNAs without additional research for further understanding of the underlying mechanism.
In conclusion, we constructed a 10-gene prognostic IRLPs signature for CRC patients based on the immune-related genes in the TCGA database. The signature was associated with immune checkpoints that play key roles in immune regulation and immunotherapy of CRC Molecular functional and lncRNA-protein network analysis were used to understand the molecular mechanisms underlying the carcinogenic effect and progression. Further prospective clinical researches and additional vitro experiments are needed to be done in the future study for making the results more convincing.
This study was supported by the Natural Guiding Plan foundation of Liaoning Province, grant number [2019-ZD-0584]; Natural Grant Program foundation of Liaoning Province, grant number [2020-ZLLH-44]; National Science Foundation of China, grant number [81902383]; Revitalizing Liaoning Talents Program, grant number [XLYC1907004]; Cultivation Program of National Science Foundation of Liaoning Cancer Hospital, grant number [2021-ZLLH-03].
All authors declare no conflicts of interest in this paper.
[1] |
F. Bray, J. Ferlay, I. Soerjomataram, R. Siegel, L. Torre, A. Jemal, Global cancer statistics 2018: GLOBOCAN estimates of incidence and mortality worldwide for 36 cancers in 185 countries, CA Cancer J. Clin., 68 (2018), 394-424. doi: 10.3322/caac.21492
![]() |
[2] | A. Jemal, E. M. Ward, C. J. Johnson, K. A. Cronin, J. Ma, B. Ryerson, et al., Annual report to the nation on the status of cancer, 1975-2014, featuring survival, J. Natl. Cancer Inst., 109 (2017). |
[3] |
L. Bertero, F. Massa, J. Metovic, R. Zanetti, I. Castellano, U. Ricardi, et al., Eighth Edition of the UICC Classification of Malignant Tumours: an overview of the changes in the pathological TNM classification criteria-What has changed and why?, Virchows Arch., 472 (2018), 519-531. doi: 10.1007/s00428-017-2276-y
![]() |
[4] |
S. Perakis, J. Thomas, M. Pichler, Non-coding RNAs enabling prognostic stratification and prediction of therapeutic response in colorectal cancer patients, Adv. Exp. Med. Biol., 937 (2016), 183-204. doi: 10.1007/978-3-319-42059-2_10
![]() |
[5] |
Y. Liu, R. Jing, J. Xu, K. Liu, J. Xue, Z. Wen, et al., Comparative analysis of oncogenes identified by microarray and RNA-sequencing as biomarkers for clinical prognosis, Biomark Med., 9 (2015), 1067-1078. doi: 10.2217/bmm.15.97
![]() |
[6] |
R. Spizzo, M. I. Almeida, A. Colombatti, G. A. Calin, Long non-coding RNAs and cancer: a new frontier of translational research?, Oncogene, 31(2012), 4577-4587. doi: 10.1038/onc.2011.621
![]() |
[7] |
D. Dimartino, A. Colantoni, M. Ballarino, J. Martone, D. Mariani, J. Danner, et al., The long non-coding RNA lnc-31 interacts with rock1 mRNA and mediates its YB-1-dependent translation, Cell Rep., 23 (2018), 733-740. doi: 10.1016/j.celrep.2018.03.101
![]() |
[8] |
S. H. E. Kaufmann, Immunology's Coming of Age, Front Immunol., 10 (2019), 684. doi: 10.3389/fimmu.2019.00684
![]() |
[9] |
Y. Chen, A. Satpathy, H. Chang, Gene regulation in the immune system by long noncoding RNAs, Nat. Immunol., 18 (2017), 962-972. doi: 10.1038/ni.3771
![]() |
[10] |
J. Xu, Q. Meng, X. Li, H. Yang, J. Xu, N. Gao, et al., Long noncoding RNA mir17HG promotes colorectal cancer progression via miR-17-5p, Cancer Res., 79 (2019), 4882-4895. doi: 10.1158/0008-5472.CAN-18-3880
![]() |
[11] |
S. Djebali, C. Davis, A. Merkel, A. Dobin, T. Lassmann, A. Mortazavi, et al., Landscape of transcription in human cells, Nature, 489 (2012), 101-108. doi: 10.1038/nature11233
![]() |
[12] |
A. M. Newman, C. L. Liu, M. R. Green, A. J. Gentles, W. Feng, Y. Xu, et al., Robust enumeration of cell subsets from tissue expression profiles, Nat. Methods, 12 (2015), 453-457. doi: 10.1038/nmeth.3337
![]() |
[13] | R. Dienstmann, L. Vermeulen, J. Guinney, S. Kopetz, S. Tejpar, J. Tabernero, Consensus molecular subtypes and the evolution of precision medicine in colorectal cancer. Nat. Rev. Cancer, 17 (2017), 268. |
[14] |
X. Wang, Q. Jin, X. Wang, W. Chen, Z. Cai, LncRNA ZFAS1 promotes proliferation and migration and inhibits apoptosis in nasopharyngeal carcinoma via the PI3K/AKT pathway in vitro, Cancer Biomark., 26 (2019), 171-182.15. doi: 10.3233/CBM-182080
![]() |
[15] |
X. Jia, P. Niu, C. Xie, H. Liu, Long noncoding RNA PXN-AS1-L promotes the malignancy of nasopharyngeal carcinoma cells via upregulation of SAPCD2, Cancer Med., 8 (2019), 4278-4291. doi: 10.1002/cam4.2227
![]() |
[16] |
H. Kambara, F. Niazi, L. Kostadinova, D. K. Moonka, C. T. Siegel, A. B. Post, et al., Negative regulation of the interferon response by an interferon-induced long non-coding RNA, Nucleic. Acids Res., 42 (2014), 10668-10680. doi: 10.1093/nar/gku713
![]() |
[17] |
P. Wang, Y. Xue, Y. Han, L. Lin, C. Wu, S. Xu, et al., The STAT3-binding long noncoding RNA lnc-DC controls human dendritic cell differentiation, Science, 344 (2014), 310-313. doi: 10.1126/science.1251456
![]() |
[18] |
G. Hu, Q. Tang, Su. Sharma, F. Yu, T. M. Escobar, S. A. Muljo, et al., Expression and regulation of intergenic long noncoding RNAs during T cell development and differentiation, Nat. Immunol., 14 (2013), 1190-1198. doi: 10.1038/ni.2712
![]() |
[19] |
Q. Chen, C. Wei, Z. X. Wang, M. Sun, Long non-coding RNAs in anti-cancer drug resistance, Oncotarget, 8 (2017), 1925-1936. doi: 10.18632/oncotarget.12461
![]() |
[20] | M. Mu, Y. Tang, Z. Yang, Y. Qiu, X. Li, W. Mo, et al., Effect of different expression of immune-related lncRNA on colon adenocarcinoma and its relation to prognosis, Biomed. Res. Int., 2020 (2020), 6942740. |
[21] |
Y. Wang, J. Liu, F. Ren, Y. Chu, B. Cui, Identification and validation of a four-long non-coding RNA signature associated with immune infiltration and prognosis in colon cancer, Front. Genet., 12 (2021), 671128. doi: 10.3389/fgene.2021.671128
![]() |
[22] |
F. Qin, H. Xu, G. Wei, Y. Ji, J. Yu, C. Hu, et al., A prognostic model based on the immune-related lncRNAs in colorectal cancer, Front. Genet., 12 (2021), 658736. doi: 10.3389/fgene.2021.658736
![]() |
[23] |
M. Sun, T. Zhang, Y. Wang, W. Huang, L. Xia, A novel signature constructed by immune-related lncRNA predicts the immune landscape of colorectal cancer, Front. Genet., 12 (2021), 695130. doi: 10.3389/fgene.2021.695130
![]() |
[24] |
J. A. Eddy, J. Sung, D. Geman, N. D. Price, Relative expression analysis for molecular cancer diagnosis and prognosis, Technol. Cancer Res. Treat., 9 (2010), 149-159. doi: 10.1177/153303461000900204
![]() |
[25] |
J. Luo, P. Liu, L. Wang, Y. Huang, Y. Wang, W. Geng, et al., Establishment of an immune-related gene pair model to predict colon adenocarcinoma prognosis, BMC Cancer, 20 (2020), 1071. doi: 10.1186/s12885-020-07532-7
![]() |
[26] |
X. Mo, X. Huang, Y. Feng, C. Wei, H. Liu, H. Ru, et al., Immune infiltration and immune gene signature predict the response to fluoropyrimidine-based chemotherapy in colorectal cancer patients, Oncoimmunology, 9 (2020), 1832347. doi: 10.1080/2162402X.2020.1832347
![]() |
[27] | S. P. Arlauckas, C. S. Garris, R. H. Kohler, M. Kitaoka, M. F. Cuccarese, K. S. Yang, et al., In vivo imaging reveals a tumor-associated macrophage-mediated resistance pathway in anti-PD-1 therapy, Sci. Transl. Med., 9 (2017), |
[28] |
A. Furukawa, M. Meguro, R. Yamazaki, H. Watanabe, A. Takahashi, K. Kuroki, et al., Evaluation of the reactivity and receptor competition of HLA-G isoforms toward available antibodies: implications of structural characteristics of hla-g isoforms, Int. J. Mol. Sci., 20 (2019), 5947. doi: 10.3390/ijms20235947
![]() |
[29] |
Y. Jiang, O. Chen, C. Cui, B. Zhao, X. Han, Z. Zhang, et al., KIR3DS1/L1 and HLA-Bw4-80I are associated with HIV disease progression among HIV typical progressors and long-term nonprogressors, BMC Infect. Dis., 13 (2013), 405. doi: 10.1186/1471-2334-13-405
![]() |
[30] |
H. Sun, J. Xu, Q. Huang, M. Huang, K. Li, K. Qu, et al., Correction: reduced CD160 expression contributes to impaired NK-cell function and poor clinical outcomes in patients with HCC, Cancer Res., 79 (2019), 1714. doi: 10.1158/0008-5472.CAN-19-0630
![]() |
[31] |
Xu G, Shi Y, Ling X, Wang D, Liu Y, Lu H, et al., HHLA2 predicts better survival and exhibits inhibited proliferation in epithelial ovarian cancer, Cancer Cell Int., 21 (2021), 252. doi: 10.1186/s12935-021-01930-y
![]() |
[32] |
Y. Liu, P. Xu, H. Liu, C. Fang, H. Guo, X. Chen, et al., Silencing IDO2 in dendritic cells: A novel strategy to strengthen cancer immunotherapy in a murine lung cancer model, Int. J. Oncol., 57 (2020), 587-597. doi: 10.3892/ijo.2020.5073
![]() |
[33] |
J. Bayry, Immunology: TL1A in the inflammatory network in autoimmune diseases, Nat. Rev. Rheumatol., 6 (2010), 67-68. doi: 10.1038/nrrheum.2009.263
![]() |
[34] |
W. Hou, D. Medynski, S. Wu, X. Lin, L. Y. Li, VEGI-192, a new isoform of TNFSF15, specifically eliminates tumor vascular endothelial cells and suppresses tumor growth, Clin. Cancer Res., 11 (2005), 5595-5602. doi: 10.1158/1078-0432.CCR-05-0384
![]() |
[35] |
C. Cavallini, O. Lovato, A. Bertolaso, E. Zoratti, G. Malpeli, E. Mimiola, et al., Expression and function of the TL1A/DR3 axis in chronic lymphocytic leukemia, Oncotarget, 6 (2015), 32061-32074. doi: 10.18632/oncotarget.5201
![]() |
[36] |
N. Zhang, P. Wu, D. Shayiremu, L. Wu, H. Shan, L. Ye, et al., Suppression of renal cell carcinoma growth in vivo by forced expression of vascular endothelial growth inhibitor, Int. J. Oncol., 42 (2013), 1664-1673. doi: 10.3892/ijo.2013.1877
![]() |
[37] |
W. Dong, Z. Cao, Y. Pang, T. Feng, H. Tian, CARF, as an oncogene, promotes colorectal cancer stemness by activating erbb signaling pathway, Onco. Targets Ther., 12 (2019), 9041-9051. doi: 10.2147/OTT.S225733
![]() |
[38] |
L. Barault, N. Veyrie, V. Jooste, D. Lecorre, C. Chapusot, J. Ferraz, et al., Mutations in the RAS-MAPK, PI(3)K (phosphatidylinositol-3-OH kinase) signaling network correlate with poor survival in a population-based series of colon cancers, Int. J. Cancer, 122 (2008), 2255-2259. doi: 10.1002/ijc.23388
![]() |
[39] |
Ogino S, Nosho K, Kirkner GJ, Shima K, Irahara N, Kure S, et al., PIK3CA mutation is associated with poor prognosis among patients with curatively resected colon cancer, J Clin. Oncol, 27 (2009), 1477-1484. doi: 10.1200/JCO.2008.18.6544
![]() |
[40] |
M. H. Hofmann, M. Gmachl, J. Ramharter, F. Savarese, D. Gerlach, J. R. Marszalek, et al., BI-3406, a Potent and Selective SOS1-KRAS Interaction Inhibitor, Is Effective in KRAS-Driven Cancers through Combined MEK Inhibition, Cancer Discov., 11 (2021), 142-157. doi: 10.1158/2159-8290.CD-20-0142
![]() |
[41] |
N. Ishaque, M. L. Abba, C. Hauser, N. Patil, N. Paramasivam, D. Huebschmann, et al., Whole genome sequencing puts forward hypotheses on metastasis evolution and therapy in colorectal cancer, Nat. Commun., 9 (2018), 4782. doi: 10.1038/s41467-018-07041-z
![]() |
[42] |
S. Cascio, O. J. Finn, Complex of MUC1, CIN85 and Cbl in colon cancer progression and metastasis, Cancers (Basel), 7 (2015), 342-352. doi: 10.3390/cancers7010342
![]() |
[43] |
W. Dai, Y. Xu, S. Mo, Q. Li, J. Yu, R. Wang, et al., GLUT3 induced by AMPK/CREB1 axis is key for withstanding energy stress and augments the efficacy of current colorectal cancer therapies, Signal Transduct Tar. Ther., 5 (2020), 177. doi: 10.1038/s41392-020-00220-9
![]() |
[44] |
C. Zhuo, D. Hu, J. Li, H. Yu, X. Lin, Y. Chen, et al., Downregulation of activin a receptor type 2a is associated with metastatic potential and poor prognosis of colon cancer, J. Cancer, 9 (2018), 3626-3633. doi: 10.7150/jca.26790
![]() |
[45] | R. Zhou, Y. Huang, B. Cheng, Y. Wang, B. Xiong, TGFBR1*6A is a potential modifier of migration and invasion in colorectal cancer cells, Oncol. Lett., 15 (2018), 3971-3976. |
[46] |
T. S. Freedman, H. Sondermann, G. D. Friedland, T. Kortemme, D. Bar-Sagi, S. Marqusee, et al., A Ras-induced conformational switch in the Ras activator Son of sevenless, Proc. Natl. Acad. Sci. USA, 103 (2006), 16692-16697. doi: 10.1073/pnas.0608127103
![]() |
[47] |
H. Jeng, L. J. Taylor, D. Bar-Sagi, Sos-mediated cross-activation of wild-type Ras by oncogenic Ras is essential for tumorigenesis, Nat. Commun., 3 (2012), 1168. doi: 10.1038/ncomms2173
![]() |
1. | Ivan Izonin, Nataliya Shakhovska, Special issue: informatics & data-driven medicine-2021, 2022, 19, 1551-0018, 9769, 10.3934/mbe.2022454 | |
2. | Xiaojie Liang, Zhaoxiang Cheng, Xinhao Chen, Jun Li, Prognosis analysis of necroptosis-related genes in colorectal cancer based on bioinformatic analysis, 2022, 13, 1664-8021, 10.3389/fgene.2022.955424 | |
3. | Jia He, Wenhan Wu, A glimpse of research cores and frontiers on the relationship between long noncoding RNAs (lncRNAs) and colorectal cancer (CRC) using the VOSviewer tool, 2023, 58, 0036-5521, 254, 10.1080/00365521.2022.2124537 | |
4. | Abdullah Al-Danakh, Mohammed Safi, Yuli Jian, Linlin Yang, Xinqing Zhu, Qiwei Chen, Kangkang Yang, Shujing Wang, Jianjun Zhang, Deyong Yang, Aging-related biomarker discovery in the era of immune checkpoint inhibitors for cancer patients, 2024, 15, 1664-3224, 10.3389/fimmu.2024.1348189 | |
5. | Agnieszka Kula, Dominika Koszewska, Anna Kot, Miriam Dawidowicz, Sylwia Mielcarska, Dariusz Waniczek, Elżbieta Świętochowska, The Importance of HHLA2 in Solid Tumors—A Review of the Literature, 2024, 13, 2073-4409, 794, 10.3390/cells13100794 |
study | database | methods | lncRNA signature | AUC (3 years) | |
Feng et al | TCGA-COAD, READ | DElncRNAs, Cox regression analysis | 8-immune related lncRNA | 0.751 | |
Meng et al | TCGA-COAD, READ | univariate analysis, Lasso and Cox regression | 16-immune-related lncRNA pair (30 lncRNAs) | 0.875 | |
Yan et al | TCGA-COAD, GEO (colon cancer) | CIBERSORT algorithm, Cox regression analysis | four-immune related lncRNA | 0.659 | |
Mei et al | TCGA-COAD | univariate and multivariate Cox regression | 14-immune related lncRNA | 0.776 | |
this study | TCGA-COAD, READ | DElncRNAs, ranking and pairing comparison, univariate Cox, Lasso and multivariate Cox regression | 10-immune-related lncRNA pair (17 lncRNAs) | 0.884 |
study | database | methods | lncRNA signature | AUC (3 years) | |
Feng et al | TCGA-COAD, READ | DElncRNAs, Cox regression analysis | 8-immune related lncRNA | 0.751 | |
Meng et al | TCGA-COAD, READ | univariate analysis, Lasso and Cox regression | 16-immune-related lncRNA pair (30 lncRNAs) | 0.875 | |
Yan et al | TCGA-COAD, GEO (colon cancer) | CIBERSORT algorithm, Cox regression analysis | four-immune related lncRNA | 0.659 | |
Mei et al | TCGA-COAD | univariate and multivariate Cox regression | 14-immune related lncRNA | 0.776 | |
this study | TCGA-COAD, READ | DElncRNAs, ranking and pairing comparison, univariate Cox, Lasso and multivariate Cox regression | 10-immune-related lncRNA pair (17 lncRNAs) | 0.884 |