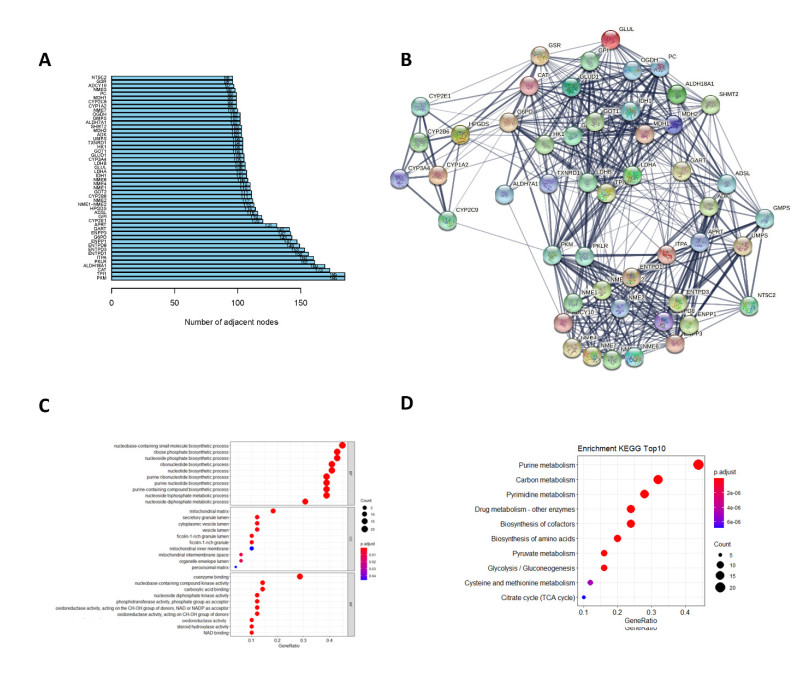
Uveal melanoma (UM) is the most aggressive intraocular tumor worldwide. Accurate prognostic models are urgently needed. The present research aimed to construct and validate a prognostic signature is associated with overall survival (OS) for UM patients based on metabolism-related genes (MRGs).
MRGs were obtained from molecular signature database (MSigDB). The gene expression profiles and patient clinical data were downloaded from The Cancer Genome Atlas (TCGA) database. In the training datasets, MRGs were analyzed through univariate Cox regression analyses and least absolute shrinkage and selection operator (LASSO) Cox analyses to build a prognostic model. The GSE84976 was treated as the validation cohort. In addition, time-dependent receiver operating characteristic (ROC) and Kaplan-Meier survival curve analyses the reliability of the developed model. Then, gene set enrichment analysis (GSEA) was used for gene enrichment analysis. Nomogram that combined the five-gene signature was used to evaluate the predictive OS value of UM patients.
Five MRGs were identified and used to establish the prognostic model for UM patients. The model was successfully validated using the testing cohort. Moreover, ROC analysis demonstrated a strong predictive ability that our prognostic signature had for UM prognosis. Multivariable Cox regression analysis revealed that the risk model was an independent predictor of prognosis. UM patients with a high-risk score showed a higher level of immune checkpoint molecules.
We established a novel metabolism-related signature that could predict survival and might be therapeutic targets for the treatment of UM patients.
Citation: Han Zhao, Yun Chen, Peijun Shen, Lan Gong. Construction and validation of a novel prognostic signature for uveal melanoma based on five metabolism-related genes[J]. Mathematical Biosciences and Engineering, 2021, 18(6): 8045-8063. doi: 10.3934/mbe.2021399
[1] | Wei Niu, Lianping Jiang . A seven-gene prognostic model related to immune checkpoint PD-1 revealing overall survival in patients with lung adenocarcinoma. Mathematical Biosciences and Engineering, 2021, 18(5): 6136-6154. doi: 10.3934/mbe.2021307 |
[2] | Jing Liu, Xuefang Zhang, Ting Ye, Yongjian Dong, Wenfeng Zhang, Fenglin Wu, Huaben Bo, Hongwei Shao, Rongxin Zhang, Han Shen . Prognostic modeling of patients with metastatic melanoma based on tumor immune microenvironment characteristics. Mathematical Biosciences and Engineering, 2022, 19(2): 1448-1470. doi: 10.3934/mbe.2022067 |
[3] | Zehao Niu, Yujian Xu, Yan Li, Youbai Chen, Yan Han . Construction and validation of a novel pyroptosis-related signature to predict prognosis in patients with cutaneous melanoma. Mathematical Biosciences and Engineering, 2022, 19(1): 688-706. doi: 10.3934/mbe.2022031 |
[4] | Kaiyu Shen, Shuaiyi Ke, Binyu Chen, Tiantian Zhang, Hongtai Wang, Jianhui Lv, Wencang Gao . Identification and validation of biomarkers for epithelial-mesenchymal transition-related cells to estimate the prognosis and immune microenvironment in primary gastric cancer by the integrated analysis of single-cell and bulk RNA sequencing data. Mathematical Biosciences and Engineering, 2023, 20(8): 13798-13823. doi: 10.3934/mbe.2023614 |
[5] | Li-Sha Pan, Zacharia Ackbarkha, Jing Zeng, Min-Li Huang, Zhen Yang, Hao Liang . Immune marker signature helps to predict survival in uveal melanoma. Mathematical Biosciences and Engineering, 2021, 18(4): 4055-4070. doi: 10.3934/mbe.2021203 |
[6] | Jiaping Wang . Prognostic score model-based signature genes for predicting the prognosis of metastatic skin cutaneous melanoma. Mathematical Biosciences and Engineering, 2021, 18(5): 5125-5145. doi: 10.3934/mbe.2021261 |
[7] | Xin Yu, Jun Liu, Ruiwen Xie, Mengling Chang, Bichun Xu, Yangqing Zhu, Yuancai Xie, Shengli Yang . Construction of a prognostic model for lung squamous cell carcinoma based on seven N6-methylandenosine-related autophagy genes. Mathematical Biosciences and Engineering, 2021, 18(5): 6709-6723. doi: 10.3934/mbe.2021333 |
[8] | Siyuan Tian, Yinan Hu, Chunmei Yang, Jiahao Yu, Jingyi Liu, Guoyun Xuan, Yansheng Liu, Keshuai Sun, Miao Zhang, Shuoyi Ma, Yulong Shang, Xia Zhou, Ying Han . A novel immune checkpoint-related gene signature for hepatocellular carcinoma to predict clinical outcomes and therapeutic response. Mathematical Biosciences and Engineering, 2022, 19(5): 4719-4736. doi: 10.3934/mbe.2022220 |
[9] | Yun-xiang Li, Shi-ming Wang, Chen-quan Li . Four-lncRNA immune prognostic signature for triple-negative breast cancer. Mathematical Biosciences and Engineering, 2021, 18(4): 3939-3956. doi: 10.3934/mbe.2021197 |
[10] | Yan He, Nannan Cao, Yanan Tian, Xuelin Wang, Qiaohong Xiao, Xiaojuan Tang, Jiaolong Huang, Tingting Zhu, Chunhui Hu, Ying Zhang, Jie Deng, Han Yu, Peng Duan . Development and validation of two redox-related genes associated with prognosis and immune microenvironment in endometrial carcinoma. Mathematical Biosciences and Engineering, 2023, 20(6): 10339-10357. doi: 10.3934/mbe.2023453 |
Uveal melanoma (UM) is the most aggressive intraocular tumor worldwide. Accurate prognostic models are urgently needed. The present research aimed to construct and validate a prognostic signature is associated with overall survival (OS) for UM patients based on metabolism-related genes (MRGs).
MRGs were obtained from molecular signature database (MSigDB). The gene expression profiles and patient clinical data were downloaded from The Cancer Genome Atlas (TCGA) database. In the training datasets, MRGs were analyzed through univariate Cox regression analyses and least absolute shrinkage and selection operator (LASSO) Cox analyses to build a prognostic model. The GSE84976 was treated as the validation cohort. In addition, time-dependent receiver operating characteristic (ROC) and Kaplan-Meier survival curve analyses the reliability of the developed model. Then, gene set enrichment analysis (GSEA) was used for gene enrichment analysis. Nomogram that combined the five-gene signature was used to evaluate the predictive OS value of UM patients.
Five MRGs were identified and used to establish the prognostic model for UM patients. The model was successfully validated using the testing cohort. Moreover, ROC analysis demonstrated a strong predictive ability that our prognostic signature had for UM prognosis. Multivariable Cox regression analysis revealed that the risk model was an independent predictor of prognosis. UM patients with a high-risk score showed a higher level of immune checkpoint molecules.
We established a novel metabolism-related signature that could predict survival and might be therapeutic targets for the treatment of UM patients.
Uveal melanoma (UM) is the most aggressive intraocular malignant tumor in adults and has a poor prognosis. The mean age-adjusted incidence is 5.1 per million in the United States [1]. UM is considered a high molecular heterogeneous and immunogenicity disease [2]. The progression of clinical treatment includes surgery, photocoagulation, and radiotherapy, which can achieve great local tumor control [3]. Nevertheless, previous studies have reported that up to 50% of UM patients will have experienced a local tumor recurrence by 10 years, and the survival rate remains poor [4,5]. As UM has the tendency to early metastasis, nearly 50% of UM patients develop tumor metastasis via hematogenous spread primarily to the liver [1]. The metastatic UM patients are usually fatal within 1 year of the onset of symptoms [6]. During the past decades, immune checkpoint blockade (ICB) has been proven efficacious in cutaneous melanoma. Unlike cutaneous melanoma metastases, UM metastases generally respond poorly to ICB, with a response rate of only 0-5% [7]. Despite being treated for their primary intraocular tumor, these patients are at lifetime risk of developing metastasis. Therefore, it is crucial to reveal a new biomarker to assess UM prognosis.
Dysregulated metabolism is one of the well-recognized hallmarks of solid human tumors. It relates to the rapid proliferation and preferential survival of cancer cells [8]. During tumorigenesis and metastasis, aberrantly activated nutrient acquisition metabolism pathways facilitate the reprogramming of cancer cell metabolism to meet the bioenergetic, biosynthetic, and redox demands of malignant cells [9]. Otto Warburg first observed the reprogrammed metabolic pathway in the cancer cell, which was later termed the "Warburg effect" or "aerobic glycolysis" to describe that cancer cells take up glucose and produce lactate regardless of oxygen availability [10]. Previous studies have reported a critical metabolic program within poor prognostic monosomy 3 UM, associated with higher metastasis and poor prognosis [11]. Monosomy 3 UM exhibited a less glycogenetic and more insulin-resistant phenotype, together with reduced glycogen levels, which were associated with the development of metastases and a reduced survival rate [12]. UM has the highest oxidative phosphorylation characteristics among 31 tumor types. A recent study also indicates that high cysteinyl leukotriene receptors, a lipid-signaling molecule, expression correlates with poor survival in UM patients [13]. In addition, some energy metabolism protein levels were significantly different in glycolysis after irradiation [14]. However, the relationship between metabolism-related genes (MRGs) and UM prognosis has not been fully elucidated.
With the development of next-generation sequencing technology, high-dimensional datasets, large-scale gene expression is accessible, which allowing us to detect aberrantly expressed MRGs related to occurrence or metastasis of cancers and patients' survival probability and the response to immunotherapy.
In the present study, we performed a comprehensive analysis in multiple databases and explored the effect of MRGs on the survival of UM patients. Notably, the least absolute shrinkage and selection operator (LASSO) Cox regression algorithm was used to analyze high-dimensional data. Then, a five-gene prognostic model was constructed to generate a prognostics risk score, and patients were stratified based on risk score, which was further validated in the gene expression omnibus (GEO) databases. In addition, Cox regression analysis was used to relationship between the predictive value and clinical information of UM patients. The nomogram was built for predicting the overall survival (OS) of UM. Moreover, GSEA and immune infiltration analysis were used to identify the role of a signature in the tumor microenvironment. The high-risk group patients have higher expression of immune checkpoint molecules. In summary, this study provided a reliable five-genes signature based on MRGs that could function as an independent prognostic marker for UM.
The gene expression profiles and the corresponding clinical data of 80 UM patients were downloaded from The University of California Santa Cruz (UCSC) Xena browser (https://xenabrowser.net/) with cohort name: TCGA-UVM. Then, the gene expression profiles of the TCGA-UVM dataset (FPKM value) were transformed into transcripts per kilobase million (TPM) value. Ensemble IDs converted to gene symbols using the "org.Hs.eg.db" and "clusterProfiler" R packages. Besides, the gene expression profiles and survival data of GSE84976 (platform GPL10558, Illumina HumanHT-12 V4.0 expression beadchip Illumina, Inc., San Diego, CA, United States) were downloaded from GEO (https://www.ncbi.nlm.nih.gov/geo/) databases, which was chosen for this study as the validation group. Strawberry Perl (version 5.32.0; http://strawberryperl.com/) was used to extract the gene expression data from the TCGA-UVM and GSE84976 datasets and construct a data matrix for further analysis. The MRGs were downloaded from the HALLMARK gene sets in the Molecular Signature Database (MSigDB) (http://www.gsea-msigdb.org/gsea/msigdb/). Finally, a total of 944 MRGs were identified for our study.
The STRING (search tool for the retrieval of interacting genes/proteins) database (https://string-db.org/) contains known and predicted PPI [15]. After constructing the PPI, the core genes of the network need to be identified. We obtained the top 50 hub genes with the largest numbers of adjacent nodes for subsequent analysis using R software.
GO and KEGG enrichment analysis was performed utilizing hub genes. The KEGG results were analyzed and visualized using the "ggplot2" and "clusterProfiler" R packages. The GO biological process (BP), cellular component (CC), and molecular function (MF) results were visualized using the "cnetplots" R package. We used p-value < 0.05 and FDR adjusted p-value < 0.05 as the GO and KEGG enrichment analysis threshold.
The univariate Cox analysis was performed to screen out potential prognostics MRGs (p < 0.001) on the training cohort. Only genes that showed significantly associated with OS of patients with UM were considered for the subsequent analysis. Then, the LASSO Cox regression was used to screen the narrow candidate genes and avoid overfitting by using "glmnet" and "survival" R packages [16]. The penalty parameter lambda was detected by using 10-fold cross-validation [17]. The minimum lambda was defined as the optimal value, and we obtain a list of prognostic signatures with correlation coefficients. Next, the multivariate Cox regression analysis was used to identify the independent prognostic MRGs, and a prognostic model was constructed. The risk score of each patient was calculated as the following equation:
Riskscore(patients)=∑ni=1Expressiongenei×Coefficientgenei |
Here, "n" represents the number of prognostic genes; "I" the serial number of each gene. UM patients were divided into high-risk and low-risk groups with the median risk score as a cutoff value according to the risk score formula. The Kaplan-Meier (K-M) survival curve was used to compare the prognostic gene signature and OS based on the two groups through the "survival" R package. Besides, the time-dependent receiver operating characteristic (ROC) curve was constructed by the "timeROC" R package, and the area under the curve (AUC) was calculated to measure the sensitivity and specificity of the multi-gene prognostic model.
To ensure the stability and repeatability of the multi-gene prognostic model, we calculated the risk score of each patient in GSE84976 cohorts, and patients were stratified into the high-risk and low-risk groups with the median risk score. The K-M curve and log-rank test were performed to evaluate the differences in survival rate between the two groups. The ROC curve and AUC analysis were implemented to show the predictive ability of prognostic signatures in the validation cohorts. The 1-, 3-, and 5-year survival rates of patients in the validation cohort were evaluated.
GSEA is a computational method determining whether an a priori defined set of genes shows statistically significant, concordant differences between two biological states. The GSEA analysis was performed to identify differences in the set of genes expression between the high-risk and low-risk groups. In this study, the KEGG gene sets (v7.4) and HALLMARK gene sets (v7.4) were downloaded from the MSigDB. According to the GSEA user guide, NOM p-val < 0.05 and FDR q-val < 0.25 were considered as statistically significant. The top 5 pathways were selected and visualized.
In order to predict the 1-, 3-, and 5-year survival probability of UM patients, the nomogram was constructed based on the results of multivariate Cox regression analysis. Moreover, the calibration curve for 1-, 3-, and 5-year survival rates was used to access the prognostic accuracy of the nomogram. The nomogram and calibration curve were constructed by using "rms" R package.
All analyses were performed with R software (version 4.0.4, 64-bit; https://www.r-project.org/) and its appropriate packages. In addition to noting before, "ggplot2", "ggpubr", "limma", "tidyverse", "dplyr" and "plyr" R packages were also used for data analysis and graph plotting. The Perl programming language (version 5.34.0, https://www.perl.org/) was used to process data. The K-M curve and log-rank test were used to evaluate the differences in OS between the high-risk and low-risk groups. The Wilcoxon rank-sum test was used to verify the differences in expression of immune-related genes between the high-risk and low-risk groups. The p < 0.05 was considered statistically significant.
We first downloaded the KEGG metabolism-related genes from the MSigDB. A total of 944 MRGs were obtained from this gene set. Then, we used the STRING website to perform a PPI network analysis between MRGs (Figure 1A). After identifying the core genes of the network and the number of adjacent nodes for each protein, we obtained the top 50 core genes (Figure 1B). To understand the potential biological functions of these core genes, GO and KEGG analyses were conducted. The GO analysis showed that MRGs were closely involved in a variety of terms, including "nucleobase-containing small-molecule biosynthetic process" in the BP category, "mitochondrial matrix" in the CC category, and "coenzyme binding" in the MF category (Figure 1C). In the KEGG pathway enrichment analysis, MRGs are involved in material synthesis and material metabolism, including the "purine metabolism", "carbon metabolism", "pyrimidine metabolism" and et al. (Figure 1D).
We obtained the gene expression data and corresponding clinical information from the TCGA-UVM cohort. The univariate Cox proportional hazard regression analysis was performed to identify the association of MRGs with OS in UM patients. Our results showed that 15 MRGs were significantly associated with the OS of UM patients (Figure 2A). Among the survival-related genes, overexpression of 13 genes (OGHD, MDH1, TPI1, MDH2, HK1, NME3, NME1, ITPA, TXNRD1, GOT1, SHMT2, NT5C2, and GLUD1) was found to be significantly related to worse survival outcomes. In comparison, overexpression of 2 genes (PC and ENPP1) showed the prognostic value of indicating better OS. Then, we performed a LASSO regression analysis of these genes, and regression coefficients were calculated (Figure 2B). the LASSO analysis indicated that the model achieves the best performance when it includes 10 genes (Figure 2C). Finally, the multivariate Cox regression analysis was performed to construct the prognostic model, and five MRGs were identified as the independent prognostic factors (Figure 2D). The prognostic risk score was calculated as follow: Risk score = (expression level of MDH2 * 2.761058) + (expression level of NME1 * 1.384644) + (expression level of NT5C2 * 1.056515) + (expression of level PC * -1.443098) + (expression level of ENPP1 * -0.732329) (Table 1).
Gene symbol | Description | Ensembl ID | Coefficient |
MDH2 | Malate dehydrogenase 2 | ENSG00000146701 | 2.761058 |
NME1 | NME/NM23 nucleoside diphosphate kinase 1 | ENSG00000239672 | 1.384644 |
NT5C2 | Cytosolic 5′ nucleotidase II | ENSG00000076685 | 1.056515 |
PC | Pyruvate carboxylase | ENSG00000173599 | -1.443098 |
ENPP1 | Ectonucleotide pyrophosphatase/phosphodiesterase 1 | ENSG00000197594 | -0.732329 |
The five-genes prognostic signature model was established based on multivariate Cox regression analysis. According to the prognostic model, the risk score of each patient was calculated. The patient in TCGA and GEO cohorts were divided into high-risk and low-risk groups based on the median risk score value. To identify the survival difference between these two groups, we conduct a K-M curve analysis. Our results reveal significant differences between the high-risk and low-risk groups. Patients in the high-risk group show markedly poorer OS than those in the low-risk group (Figure 3A). Also, an unfavorable OS outcome was seen in the high-risk group of the testing group (Figure 3B). Subsequently, the accuracy of the OS estimate derived from the prognostic model was performed through a time-dependent ROC curve. The ROC curve showed a gradually increasing accuracy of predicting the 1-year, 3-year, and 5-year survival rates. The AUC of the ROC curve was 0.861 at 1-year, 0.767 at 3-year, and 0.909 at 5-year in the training cohort (Figure 3C). The AUC of the ROC curve was 0.807 at 3-year and 0.861 at 5-year in the testing cohort (Figure 3D).
Furthermore, the distribution of survival status of the five-genes signature of patients both in training and testing cohort were plotted in Figure 4A, B. The histogram showed that a higher proportion of survival patients in the low-risk group than the high-risk group. We also analyzed the interaction between the MRGs identified as the prognostic model (Figure 4C, D). Then, the risk score, survival status, and gene expression were presented in Figure 4E and F. As showed in the figure, the survival time was longer in the low-risk group than in the high-risk group. In addition, the heatmap showed that NT5C2, MDH2, and NME1 were highly expressed in the high-risk group, PC and ENPP1 were highly expressed in the low-risk group.
The K-M survival analysis was applied to determine the relationship between OS rate in different subgroups of patients according to the risk score level and clinical characters, such as different stages, age, grade, gender, and TMN status. The results indicated that five-gene signature can significantly distinguish the prognosis of patients with the following characteristics: female, male, age ≤ 65, age > 65, stage I-II, stage III-IV, T1-2, T3-4, M0, M1, N0, and N1, respectively (Figure 5A-L). Subsequently, the univariate and multivariate Cox regression analysis also indicated that the novel prognostic model could work as an independent prognostic factor related to the OS rate of UM patients (Figure 5M, N).
Immunotherapy is a novel treatment for advanced tumors, activating the host's natural immune defense system. However, the limitation of immunotherapy is that it can only benefit a minority part of patients. So, a more reliable biomarker to predict patient response to immunotherapy. Thus, we analyzed the expression level of the immune-related genes between high-risk and low-risk groups. A heatmap was then drawn to visualize the expression of these genes of patients in TCGA and GEO databases. As shown in Figure 6A, B, the expression level of EDNRB, MICA, and CCL28, which negatively regulated the trafficking and infiltration of immune cells into cancer, were significantly higher in high-risk groups than in low-risk groups group (p < 0.01). Next, we investigated the expression of ICB molecules in the high-risk and low-risk groups. Our results showed that programmed cell death-1 (PD-1) was positively correlated with a risk score, was upregulated in the high-risk group (Figure 6C, D). In addition, the expression level of critical immune checkpoints (CD272, CD27, and IDO1) in the high-risk group was significantly higher than that in the low-risk group (Figures 6G-J). We also observed that immunosuppressive cytokines were also upregulated in the high-risk group (Figures 6K, L). Altogether, these results indicated that the patient with high-risk scores was found to tend to develop an immunosuppressive microenvironment through the upregulation of immunosuppressive cytokines and immune checkpoint-related genes.
To understand the level of pathway enrichment between the high-risk and low-risk groups, the GSEA software was used to perform pathway enrichment analysis according to NES, nominal p-value, FDR q-value, and FWER p-value. As shown in Figure 7A, we found that all markedly enriched gene set of HALLMARK collection was seen in the high-risk group, such as angiogenesis, IL-6-JAK-STAT3 signaling, inflammatory response, oxidative phosphorylation, and UV response. The other related pathway annotated by the HALLMARK was shown in Supplementary Table S1. Moreover, all gene sets enriched in the KEGG collection were also enriched in the high-risk group (Figure 7B). These pathways mostly correlated with apoptosis, cell cycle, glutathione metabolism, mTOR signaling pathway, and p53 signaling pathway. The other related pathway annotated by the KEGG was shown in Supplementary Table S2, 3.
Nomogram was constructed with the five prognostic genes to predict the OS rate of patients with UM. In the present study, a nomogram associated with the 1-year, 3-year, and 5-year survival rates was established (Figure 8A). Moreover, the calibration curve of the nomogram indicated an optimal agreement between the nomogram-predicted and observed OS rate (Figure 8B). The same results were seen in the validation cohort (Supplementary Figure S1).
UM is one of the highly aggressive tumors and remarkably variable in patient's survival rate. The advancement of high-throughput technologies and bioinformatic methodology enables us to identify the crucial role of gene signatures based on specific associations in predicting the prognostic outcome of UM patients. Moreover, numerous HALLMARK gene sets related to tumor prognosis have been defined, such as autophagy, hypoxia, angiogenesis, and metabolism. In addition, the novel gene signature may also provide a more reliable and more effective biomarker in early detection and treatment.
Recently, the dysregulated metabolism of cancer has become a hotpot in cancer research, which might serve as a biomarker and a novel therapeutic strategy. Moreover, it has been reported to play a vital role in tumorigenesis, development, progression, and therapeutic resistance of different types of cancer. However, there is little known about the role of MRGs in the pathogenesis and progression of UM.
This study constructed a prognostic signature based on the MRGs in UM patients from the TCGA and GEO databases. Using the univariate Cox regression analysis, we found 15 MRGs were significantly correlated with OS rate in UM patients. After conducting the LASSO regression and multivariate Cox regression analysis between the patient's prognosis and gene expression, we obtained a novel prognostic signature, which consisted of five MRGs (NT5C2, MDH2, NME1, PC, and ENPP1). Then, we divided the UM cohort into the high-risk and low-risk groups according to the median risk score. The K-M survival curve analysis showed that the prognosis of patients in the high-risk and low-risk groups was significantly different. The OS rate in the low-risk group was markedly higher than that of their counterpart. Moreover, the time-dependent ROC curve, which aimed to evaluate the accuracy of the OS estimate, demonstrated that the AUC for 1-year, 3-year, and 5-year was 0.861, 0.767, and 0.909, respectively. Thus, the nomogram was constructed to represent the relations between MRGs expression level and prognosis. The calibration of the nomogram indicated that it has a good agreement between the predicted and observed survival rates. In addition, we performed a K-M analysis of the correlation of risk score with other clinical factors, such as gender, age, Stage, and TMN status. We also evaluated the prognostic value of this signature in the validation cohort, which indicated that the five-genes prognostic signature could be used as an independent prognosis indicator in both databases.
Five MRGs (NT5C2, PC, MDH2, ENPP1, and NME1) were selected as the prognostic signature in this study. Some of the genes in our signature have previously been shown to be involved in melanoma. However, several genes' role in UM remains unclear. NT5C2, cytosolic 5' nucleotidase II, plays an essential role in cellular nucleotide homeostasis. Recently studies revealed that NT5C2 is associated with cancer cell survival and chemotherapy resistance, including relapsed acute lymphoblastic leukemia and glioblastoma [18,19]. Pyruvate carboxylase (PC), a mitochondrial enzyme during the tricarboxylic acid cycle, plays a crucial role in catalyzes the ATP-dependent carboxylation of pyruvate to oxaloacetate [20]. Overexpression of PC is related to cancer cell survival and proliferation in non-small-cell lung cancer [21]. The PC inhibitor phenylacetic acid could suppress vemurafenib-treated melanoma cells growth, sensitizing melanoma cells to vemurafenib treatment [22,23]. Malate dehydrogenase 2 (MDH2), a member of malate dehydrogenase, is a crucial enzyme involved in the citric acid cycle in mitochondria [24]. Zhuang et al. reported that MDH2 could promote the proliferation, migration, and invasion but inhibited the apoptosis of the endometrial cancer cell line by suppressing PTEN [25]. The previous study also indicated that MDH2 is considered a candidate therapeutic target for cancer metabolism and tumor growth [26]. Ectonucleotide pyrophosphatase/phosphodiesterase 1 (ENPP1) is a type II transmembrane glycoprotein involved in insulin resistance and bone metabolism [27]. Abnormal expression of ENPP1 can increase human lung cancer malignancy via epithelial-mesenchymal transition [28]. In addition, the expression level of ENPP1 from patients with triple-negative breast cancer was significantly associated with recurrence-free survival and overall survival [29]. NME1 is a metastasis suppressor gene that inhibits tumor cells' metastatic activity in different types of cancer. NME1 was associated with a statistically significant risk of breast cancer relapse and tumor metastasis [30]. NME1 was also able to suppress metastasis in a mouse model of UV-induced melanoma [31]. However, Wang et al. reported that NME1 could promote the expansion of melanoma cells, thus contributing to the melanoma growth and lung colonizing activities, which indicated NME1 could act as a driver of melanoma growth distinct from its function as a metastasis suppressor [32].
The clinical trial indicated that immunotherapy has high efficacy in tumor treatment and can improve the quality of life in patients with advanced melanoma. However, the main disadvantage of immunotherapy is that only a minority of patients respond to it. Previous studies reported that immune checkpoint molecules could affect the metabolic communication of T cells. For instance, PD-1–PD-L1 axis might impair the metabolism pathway, such as aerobic glycolysis and glutaminolysis, in T cells through the PI3K–AKT–mTOR pathway [33,34]. In addition, to impair the metabolic profile of tumor-infiltrating lymphocytes, immune checkpoint molecules can also directly support T cell activation via reprogramming of metabolism. For example, CD28 signaling can enhance aerobic glycolysis and stimulate mitochondrial fusion of T cells [35]. In this study, we identified the association between risk score and immune checkpoint molecules. The high-risk group patients have a higher expression level of immune checkpoint molecules than those in the low-risk group.
Furthermore, we found different gene expression levels, which encode chemokines between the high-risk and low-risk groups. We found that the high-risk group patients have a high expression of six chemokines (CXCL9, CXCL10, CXCL11, CCR5, CCL20, CXCR3) low-risk group. Chemokines promote the migration, localization, and progression of several cancers. It has been reported that CCL20 is a poor prognostic factor for cutaneous melanoma [36]. CXCL10 is secreted by the various immune cell, is associated with melanoma invasion, proliferation, and metastasis [37]. It has also been observed that anti-PD-1 therapy is not effective without CXCL9, CXCL10, CXCL11/CXCR3 axis [38]. For anti-CTLA4 cancer immunotherapy, the expression levels of this axis were significantly increased in melanoma patients with good clinical responses [39]. CCR5 acts as a modulator of the immune response of interleukin-2 signaling therapy, contribute to the harmful inflammatory response [40]. Taken together, the outcome of our study revealed that the five-genes prognostic signature might be a potential predictive indicator in accessing the response of UM patients to immunotherapy.
However, there are some limitations to our study. First, the size of TCGA-UVM and GSE84976 cohorts is relatively small, which may not be consistent with the clinical population. Secondly, due to the limited clinical characteristic of patients in the GSE84976 cohort, some clinical subgroups analysis could not be performed. Finally, long-term prospective clinical research is needed to validate the robustness of the prognostic signature.
In summary, we constructed a novel five-gene signature prognostic risk model based on MRGs in the TCGA database that was an independent prognostic factor for UM patients and validated by an independent cohort from the GEO database. Our study may provide guidance for targeted therapy and potential biomarkers in the future.
This study was supported by the National Natural Science Foundation of China (Grant nos.82070924) and the Open Sharing Fund for the Large-scale Instruments and Equipment of Central South University.
The authors declare no competing interests.
[1] | A. D. Singh, M. E. Turell, A. K. Topham, Uveal melanoma: trends in incidence, treatment, and survival, Ophthalmology, 118 (2011), 1881-1885. |
[2] |
M. H. Abdel-Rahman, K. M. Sample, R. Pilarski, T. Qalsh, T. Grosel, D. Kinnamon, et al., Whole exome sequencing identifies candidate genes associated with hereditary predisposition to uveal melanoma, Ophthalmology, 127 (2020), 668-678. doi: 10.1016/j.ophtha.2019.11.009
![]() |
[3] |
M. W. Wilson, J. L. Hungerford, Comparison of episcleral plaque and proton beam radiation therapy for the treatment of choroidal melanoma, Ophthalmology, 106 (1999), 1579-1587. doi: 10.1016/S0161-6420(99)90456-6
![]() |
[4] |
B. Damato, S. E. Coupland, An audit of conjunctival melanoma treatment in liverpool, Eye, 23 (2009), 801-809. doi: 10.1038/eye.2008.154
![]() |
[5] | S. Tuomaala, S. Eskelin, A. Tarkkanen, T. Kivela, Population-based assessment of clinical characteristics predicting outcome of conjunctival melanoma in whites, Invest. Ophth. Vis. Sci., 43 (2002), 3399-3408. |
[6] |
P. Rietschel, K. S. Panageas, C. Hanlon, A. Patel, D. H. Abramson, P. B. Chapman, Variates of survival in metastatic uveal melanoma, J. Clin. Oncol., 23 (2005), 8076-8080. doi: 10.1200/JCO.2005.02.6534
![]() |
[7] |
M. V. Heppt, T. Steeb, J. G. Schlager, S. Rosumeck, C. Dressler, T. Ruzicka, et al., Immune checkpoint blockade for unresectable or metastatic uveal melanoma: A systematic review, Cancer Treat. Rev., 60 (2017), 44-52. doi: 10.1016/j.ctrv.2017.08.009
![]() |
[8] |
M. G. Vander Heiden, R. J. DeBerardinis, Understanding the intersections between metabolism and cancer biology, Cell, 168 (2017), 657-669. doi: 10.1016/j.cell.2016.12.039
![]() |
[9] | S. Katada, A. Imhof, P. Sassone-Corsi, Connecting threads: epigenetics and metabolism, Cell, 148 (2012), 24-28. |
[10] |
O. Warburg, On the origin of cancer cells, Sci., 123 (1956), 309-314. doi: 10.1126/science.123.3191.309
![]() |
[11] |
C. Chattopadhyay, J. Oba, J. Roszik, J. R. Marszalek, K. Chen, Y. Qi, et al., Elevated endogenous SDHA drives pathological metabolism in highly metastatic uveal melanoma, Invest. Ophth. Vis. Sci., 60 (2019), 4187-4195. doi: 10.1167/iovs.19-28082
![]() |
[12] | S. Vardanyan, A. Brosig, H. Merz, M. Ranjbar, V. Kakkassery, S. Grisanti, et al., Metastasis of uveal melanoma with monosomy-3 is associated with a less glycogenetic gene expression profile and the dysregulation of glycogen storage, Cancers, 12 (2020), 2101. |
[13] | K. Slater, A. B. Heeran, S. Garcia-Mulero, H. Kalirai, R. Sanz-Pamplona, A. Rahman, et al., High cysteinyl leukotriene receptor 1 expression correlates with poor survival of uveal melanoma patients and cognate antagonist drugs modulate the growth, cancer secretome, and metabolism of uveal melanoma cells, Cancers, 12 (2020), 2950. |
[14] | L. B. Yan, K. Shi, Z. T. Bing, Y. L. Sun, Y. Shen, Proteomic analysis of energy metabolism and signal transduction in irradiated melanoma cells, Int. J. Ophthalmol., 6 (2013), 286-294. |
[15] | D. Szklarczyk, A. L. Gable, D. Lyon, A. Junge, S. Wyder, J. Huerta-Cepas, et al., String v11: protein-protein association networks with increased coverage, supporting functional discovery in genome-wide experimental datasets, Nucleic Acids Res., 47 (2019), D607-d613. |
[16] |
R. Tibshirani, The lasso method for variable selection in the Cox model, Stat. Med., 16 (1997), 385-395. doi: 10.1002/(SICI)1097-0258(19970228)16:4<385::AID-SIM380>3.0.CO;2-3
![]() |
[17] | J. Friedman, T. Hastie, R. Tibshirani, Regularization paths for generalized linear models via coordinate descent, J. Stat. Softw., 33 (2010), 1-22. |
[18] |
C. L. Dieck, A. Ferrando, Genetics and mechanisms of NT5C2-driven chemotherapy resistance in relapsed ALL, Blood, 133 (2019), 2263-2268. doi: 10.1182/blood-2019-01-852392
![]() |
[19] |
F. Cividini, E. Cros-Perrial, R. Pesi, C. Machon, S. Allegrini, M. Camici, et al., Cell proliferation and drug sensitivity of human glioblastoma cells are altered by the stable modulation of cytosolic 5'-nucleotidase Ⅱ, Int. J. Biochem. Cell Biol., 65 (2015), 222-229. doi: 10.1016/j.biocel.2015.06.011
![]() |
[20] |
A. D. Lietzan, M. Maurice, Functionally diverse biotin-dependent enzymes with oxaloacetate decarboxylase activity, Arch. Biochem. Biophys., 544 (2014), 75-86. doi: 10.1016/j.abb.2013.10.014
![]() |
[21] |
K. Sellers, M. P. Fox, M. Bousamra, S. P. Slone, R. M. Higashi, D. M. Miller, et al., Pyruvate carboxylase is critical for non-small-cell lung cancer proliferation, J. Clin. Invest., 125 (2015), 687-698. doi: 10.1172/JCI72873
![]() |
[22] | T. Delgado-Goñ i, T. C. Galobart, S. Wantuch, D. Normantaite, M. O. Leach, S. R. Whittaker, et al, Increased inflammatory lipid metabolism and anaplerotic mitochondrial activation follow acquired resistance to vemurafenib in BRAF-mutant melanoma cells, Brit. J. Cancer, 122 (2020), 72-81. |
[23] |
T. Delgado-Goni, M. F. Miniotis, S. Wantuch, H. G. Parkes, R. Marais, P. Workman, et al. The BRAF inhibitor vemurafenib activates mitochondrial metabolism and inhibits hyperpolarized pyruvate-lactate exchange in BRAF-mutant human melanoma cells, Mol. Cancer Ther., 15 (2016), 2987-2999. doi: 10.1158/1535-7163.MCT-16-0068
![]() |
[24] | P. Minárik, N. Tomásková, M. Kollárová, M. Antalik, Malate dehydrogenases-structure and function, Gen. Physiol. Biophys., 21 (2002), 257-265. |
[25] |
Y. Zhuang, J. Xiang, W. Bao, Y. Sun, L. Wang, M. Tan, et al., MDH2 stimulated by estrogen-GPR30 pathway down-regulated pten expression promoting the proliferation and invasion of cells in endometrial cancer, Transl. Oncol., 10 (2017), 203-210. doi: 10.1016/j.tranon.2017.01.009
![]() |
[26] |
R. Naik, H. S. Ban, K. Jang, I. Kim, X. Xu, D. Harmalkar, et al., Methyl 3-(3-(4-(2, 4, 4-Trimethylpentan-2-yl)phenoxy)-propanamido)benzoate as a novel and dual malate dehydrogenase (MDH) 1/2 inhibitor targeting cancer metabolism, J Med. Chem., 60 (2017), 8631-8646. doi: 10.1021/acs.jmedchem.7b01231
![]() |
[27] |
K. Kato, H. Nishimasu, S. Okudaira, E. Mihara, R. Ishitani, J. Takagi, et al., Crystal structure of Enpp1, an extracellular glycoprotein involved in bone mineralization and insulin signaling, P. Natl. Acad. Sci. USA, 109 (2012), 16876-16881. doi: 10.1073/pnas.1208017109
![]() |
[28] | M. Hu, W. Guo, Y. Liao, D. Xu, B. Sun, H. Song, et al., Dysregulated ENPP1 increases the malignancy of human lung cancer by inducing epithelial-mesenchymal transition phenotypes and stem cell features, Am. J. Cancer Res., 9 (2019), 134-144. |
[29] |
M. Kawaguchi, X. Han, T. Hisada, S. Nishikawa, K. Kano, N. Ieda, et al., Development of an ENPP1 fluorescence probe for inhibitor screening, cellular imaging, and prognostic assessment of malignant breast cancer, J. Med. Chem., 62 (2019), 9254-9269. doi: 10.1021/acs.jmedchem.9b01213
![]() |
[30] |
S. Qu, J. Long, Q. Cai, X. O. Shu, H. Cai, Y. T. Gao, et al., Genetic polymorphisms of metastasis suppressor gene NME1 and breast cancer survival, Clin. Cancer Res., 14 (2008), 4787-4793. doi: 10.1158/1078-0432.CCR-08-0083
![]() |
[31] |
N. Pamidimukkala, G. S. Puts, M. K. Leonard, D. Snyder, S. Dabernat, E. C. De Fabo, et al., Nme1 and Nme2 genes exert metastasis-suppressor activities in a genetically engineered mouse model of UV-induced melanoma, Brit. J. Cancer, 124 (2021), 161-165. doi: 10.1038/s41416-020-01096-w
![]() |
[32] |
Y. Wang, M. K. Leonard, D. E. Snyder, M. L. Fisher, R. L. Eckert, D. M. Kaetzel, NME1 Drives Expansion of Melanoma Cells with Enhanced Tumor Growth and Metastatic Properties, Mol. Cancer Res., 17 (2019), 1665-1674. doi: 10.1158/1541-7786.MCR-18-0019
![]() |
[33] |
A. J. Freemerman, A. R. Johnson, G. N. Sacks, J. J. Milner, E. L. Kirk, M. A. Troester, et al., Metabolic reprogramming of macrophages: glucose transporter 1 (GLUT1)-mediated glucose metabolism drives a proinflammatory phenotype, J. Biol. Chem., 289 (2014), 7884-7896. doi: 10.1074/jbc.M113.522037
![]() |
[34] |
T. Cascone, J. A. McKenzie, R. M. Mbofung, S. Punt, Z. Wang, C. Xu, et al., Increased tumor glycolysis characterizes immune resistance to adoptive T cell therapy, Cell Metab., 27 (2018), 977-987. doi: 10.1016/j.cmet.2018.02.024
![]() |
[35] |
S. R. Jacobs, C. E. Herman, N. J. MacIver, J. A. Wofford, H. L. Wieman, J. J. Hammen, et al., Glucose uptake is limiting in T cell activation and requires CD28-mediated Akt-dependent and independent pathways, J. Immunol., 180 (2008), 4476-4486. doi: 10.4049/jimmunol.180.7.4476
![]() |
[36] | R. Samaniego, A. Gutiérrez-González, A. Gutiérrez-Seijo, S. Sánchez-Gregorio, J. García-Giménez, E. Mercader, et al., CCL20 expression by tumor-associated macrophages predicts progression of human primary cutaneous melanoma, Cancer Immunol. Res., 6 (2018), 267-275. |
[37] | H. Bagheri, M. H. Pourhanifeh, M. Derakhshan, M. Mahjoubin-Tehran, F. Ghasemi, S. Mousavi, et al, CXCL-10: a new candidate for melanoma therapy?, Cell. Oncol., 43 (2020), 353-365. |
[38] |
Z. S. Chheda, R. K. Sharma, V. R. Jala, A. D. Luster, B. Haribabu, Chemoattractant receptors BLT1 and CXCR3 regulate antitumor immunity by facilitating CD8+ t cell migration into tumors, J. Immunol., 197 (2016), 2016-2026. doi: 10.4049/jimmunol.1502376
![]() |
[39] |
R. R. Ji, S. D. Chasalow, L. Wang, O. Hamid, H. Schmidt, J. Cogswell, et al., An immune-active tumor microenvironment favors clinical response to ipilimumab, Cancer Immunol. Immun., 61 (2012), 1019-1031. doi: 10.1007/s00262-011-1172-6
![]() |
[40] |
D. Bedognetti, T. L. Spivey, Y. Zhao, L. Uccellini, S. Tomei, M. E. Dudley, et al., CXCR3/CCR5 pathways in metastatic melanoma patients treated with adoptive therapy and interleukin-2, Brit. J. Cancer, 109 (2013), 2412-2423. doi: 10.1038/bjc.2013.557
![]() |
![]() |
![]() |
1. | Ke Shi, Xinxin Li, Jingfa Zhang, Xiaodong Sun, Development and Validation of a Novel Metabolic Signature-Based Prognostic Model for Uveal Melanoma, 2022, 11, 2164-2591, 9, 10.1167/tvst.11.5.9 | |
2. | Aurélie Fuentes-Rodriguez, Andrew Mitchell, Sylvain L. Guérin, Solange Landreville, Recent Advances in Molecular and Genetic Research on Uveal Melanoma, 2024, 13, 2073-4409, 1023, 10.3390/cells13121023 | |
3. | Xiaoyan Li, Jing Kang, Jing Yue, Dawei Xu, Chunhua Liao, Huina Zhang, Jin Zhao, Qiongwen Liu, Jinke Jiao, Lin Wang, Guoyin Li, Identification and validation of immunogenic cell death-related score in uveal melanoma to improve prediction of prognosis and response to immunotherapy, 2023, 15, 1945-4589, 3442, 10.18632/aging.204680 | |
4. | Xiaoyu Guo, Xin Yu, Yuying Zhang, Huijuan Luo, Rong Huang, Yuyang Zeng, Chaoye Duan, Changzheng Chen, A Novel Glycolysis-Related Signature for Predicting the Prognosis and Immune Infiltration of Uveal Melanoma, 2023, 0030-3747, 692, 10.1159/000529818 |
Gene symbol | Description | Ensembl ID | Coefficient |
MDH2 | Malate dehydrogenase 2 | ENSG00000146701 | 2.761058 |
NME1 | NME/NM23 nucleoside diphosphate kinase 1 | ENSG00000239672 | 1.384644 |
NT5C2 | Cytosolic 5′ nucleotidase II | ENSG00000076685 | 1.056515 |
PC | Pyruvate carboxylase | ENSG00000173599 | -1.443098 |
ENPP1 | Ectonucleotide pyrophosphatase/phosphodiesterase 1 | ENSG00000197594 | -0.732329 |
Gene symbol | Description | Ensembl ID | Coefficient |
MDH2 | Malate dehydrogenase 2 | ENSG00000146701 | 2.761058 |
NME1 | NME/NM23 nucleoside diphosphate kinase 1 | ENSG00000239672 | 1.384644 |
NT5C2 | Cytosolic 5′ nucleotidase II | ENSG00000076685 | 1.056515 |
PC | Pyruvate carboxylase | ENSG00000173599 | -1.443098 |
ENPP1 | Ectonucleotide pyrophosphatase/phosphodiesterase 1 | ENSG00000197594 | -0.732329 |