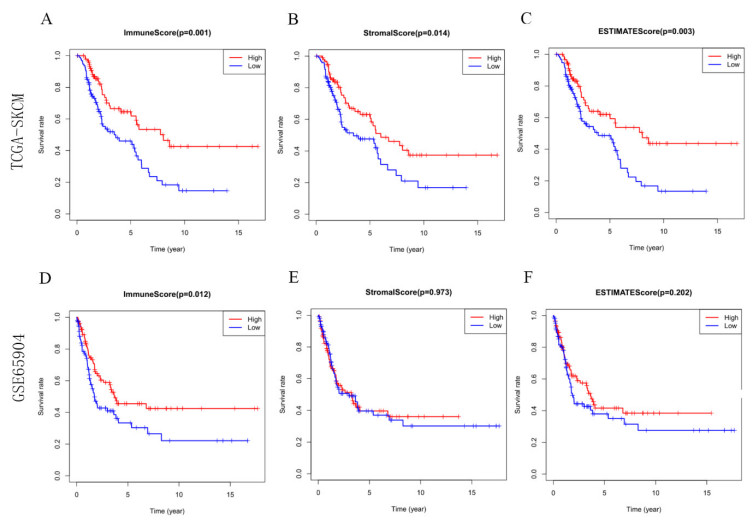
Most of the malignant melanomas are already in the middle and advanced stages when they are diagnosed, which is often accompanied by the metastasis and spread of other organs. Besides, the prognosis of patients is bleak. The characteristics of the local immune microenvironment in metastatic melanoma have important implications for both tumor progression and tumor treatment. In this study, data on patients with metastatic melanoma from the TCGA and GEO datasets were selected for immune, stromal, and estimate scores, and overlapping differentially expressed genes were screened. A nine-IRGs prognostic model (ALOX5AP, ARHGAP15, CCL8, FCER1G, GBP4, HCK, MMP9, RARRES2 and TRIM22) was established by univariate COX regression, LASSO and multivariate COX regression. Receiver operating characteristic curves were used to test the predictive accuracy of the model. Immune infiltration was analyzed by using CIBERSORT and Xcell in high-risk and low-risk groups. The immune infiltration of the high-risk group was significantly lower than that of the low-risk group. Immune checkpoint analysis revealed that the expression of PDCD1, CTLA4, TIGIT, CD274, HAVR2 and LAG3 demonstrated the visible difference in groups with different levels of risk scores. WGCNA analysis found that the yellow-green module contained seven genes from the nine-IRG prognostic model, and the yellow-green module had the highest correlation with risk scores. The results of GO and KEGG suggested that the genes in the yellow-green module were mainly enriched in immune-related biological processes. Finally, the expression characteristics of ALOX5AP, ARHGAP15, CCL8, FCER1G, GBP4, HCK, MMP9, RARRES2 and TRIM22 were analyzed between metastatic melanoma and normal samples. Overall, a prognostic model for metastatic melanoma based on the tumor immune microenvironment characteristics was established, which left plenty of space for further studies. It could function well in helping people to understand characteristics of the immune microenvironment in metastatic melanoma.
Citation: Jing Liu, Xuefang Zhang, Ting Ye, Yongjian Dong, Wenfeng Zhang, Fenglin Wu, Huaben Bo, Hongwei Shao, Rongxin Zhang, Han Shen. Prognostic modeling of patients with metastatic melanoma based on tumor immune microenvironment characteristics[J]. Mathematical Biosciences and Engineering, 2022, 19(2): 1448-1470. doi: 10.3934/mbe.2022067
[1] | Li-Sha Pan, Zacharia Ackbarkha, Jing Zeng, Min-Li Huang, Zhen Yang, Hao Liang . Immune marker signature helps to predict survival in uveal melanoma. Mathematical Biosciences and Engineering, 2021, 18(4): 4055-4070. doi: 10.3934/mbe.2021203 |
[2] | Linqian Guo, Qingrong Meng, Wenqi Lin, Kaiyuan Weng . Identification of immune subtypes of melanoma based on single-cell and bulk RNA sequencing data. Mathematical Biosciences and Engineering, 2023, 20(2): 2920-2936. doi: 10.3934/mbe.2023138 |
[3] | Chen Zheng, Zhaobang Tan . A novel identified pyroptosis-related prognostic signature of colorectal cancer. Mathematical Biosciences and Engineering, 2021, 18(6): 8783-8796. doi: 10.3934/mbe.2021433 |
[4] | Xin Lin, Xingyuan Li, Binqiang Ma, Lihua Hang . Identification of novel immunomodulators in lung squamous cell carcinoma based on transcriptomic data. Mathematical Biosciences and Engineering, 2022, 19(2): 1843-1860. doi: 10.3934/mbe.2022086 |
[5] | Kaiyu Shen, Shuaiyi Ke, Binyu Chen, Tiantian Zhang, Hongtai Wang, Jianhui Lv, Wencang Gao . Identification and validation of biomarkers for epithelial-mesenchymal transition-related cells to estimate the prognosis and immune microenvironment in primary gastric cancer by the integrated analysis of single-cell and bulk RNA sequencing data. Mathematical Biosciences and Engineering, 2023, 20(8): 13798-13823. doi: 10.3934/mbe.2023614 |
[6] | Jiaping Wang . Prognostic score model-based signature genes for predicting the prognosis of metastatic skin cutaneous melanoma. Mathematical Biosciences and Engineering, 2021, 18(5): 5125-5145. doi: 10.3934/mbe.2021261 |
[7] | Xiaowei Zhang, Jiayu Tan, Xinyu Zhang, Kritika Pandey, Yuqing Zhong, Guitao Wu, Kejun He . Aggrephagy-related gene signature correlates with survival and tumor-associated macrophages in glioma: Insights from single-cell and bulk RNA sequencing. Mathematical Biosciences and Engineering, 2024, 21(2): 2407-2431. doi: 10.3934/mbe.2024106 |
[8] | Yuan Yang, Lingshan Zhou, Xi Gou, Guozhi Wu, Ya Zheng, Min Liu, Zhaofeng Chen, Yuping Wang, Rui Ji, Qinghong Guo, Yongning Zhou . Comprehensive analysis to identify DNA damage response-related lncRNA pairs as a prognostic and therapeutic biomarker in gastric cancer. Mathematical Biosciences and Engineering, 2022, 19(1): 595-611. doi: 10.3934/mbe.2022026 |
[9] | Sidan Long, Shuangshuang Ji, Kunmin Xiao, Peng Xue, Shijie Zhu . Prognostic and immunological value of LTB4R in pan-cancer. Mathematical Biosciences and Engineering, 2021, 18(6): 9336-9356. doi: 10.3934/mbe.2021459 |
[10] | Han Zhao, Yun Chen, Peijun Shen, Lan Gong . Construction and validation of a novel prognostic signature for uveal melanoma based on five metabolism-related genes. Mathematical Biosciences and Engineering, 2021, 18(6): 8045-8063. doi: 10.3934/mbe.2021399 |
Most of the malignant melanomas are already in the middle and advanced stages when they are diagnosed, which is often accompanied by the metastasis and spread of other organs. Besides, the prognosis of patients is bleak. The characteristics of the local immune microenvironment in metastatic melanoma have important implications for both tumor progression and tumor treatment. In this study, data on patients with metastatic melanoma from the TCGA and GEO datasets were selected for immune, stromal, and estimate scores, and overlapping differentially expressed genes were screened. A nine-IRGs prognostic model (ALOX5AP, ARHGAP15, CCL8, FCER1G, GBP4, HCK, MMP9, RARRES2 and TRIM22) was established by univariate COX regression, LASSO and multivariate COX regression. Receiver operating characteristic curves were used to test the predictive accuracy of the model. Immune infiltration was analyzed by using CIBERSORT and Xcell in high-risk and low-risk groups. The immune infiltration of the high-risk group was significantly lower than that of the low-risk group. Immune checkpoint analysis revealed that the expression of PDCD1, CTLA4, TIGIT, CD274, HAVR2 and LAG3 demonstrated the visible difference in groups with different levels of risk scores. WGCNA analysis found that the yellow-green module contained seven genes from the nine-IRG prognostic model, and the yellow-green module had the highest correlation with risk scores. The results of GO and KEGG suggested that the genes in the yellow-green module were mainly enriched in immune-related biological processes. Finally, the expression characteristics of ALOX5AP, ARHGAP15, CCL8, FCER1G, GBP4, HCK, MMP9, RARRES2 and TRIM22 were analyzed between metastatic melanoma and normal samples. Overall, a prognostic model for metastatic melanoma based on the tumor immune microenvironment characteristics was established, which left plenty of space for further studies. It could function well in helping people to understand characteristics of the immune microenvironment in metastatic melanoma.
Melanoma is a highly lethal skin malignancy with low morbidity, easily early metastasis [1]. There are approximately 200,000 new cases diagnosed each year worldwide, and the mortality rate is showing a rapid increase [2]. For melanoma in the early stage, complete resection of the primary tumor can achieve a good prognosis and be cured [3]. However, for melanoma in the late stage, especially stage IV melanoma, the risk of recurrence after surgery is high and the prognosis is poor [4]. Stage I and II involve localized disease, stage III is characterized by metastasis to the local lymphnodes, and stage IV represents distant metastasis [5]. In recent years, the management of patients with metastatic melanoma has evolved considerably through targeted therapies and immunotherapy [6]. These findings underscore the importance of discovering new prognostic biomarkers and immunotherapy for patients with stage III or IV melanoma who have developed metastases.
Immunotherapy has become a new therapeutic approach to fight cancer by activating the immune system, which is widely used in various tumors, especially melanoma, lung cancer and renal cell carcinoma [7]. Significant progress has been made in prolonging patient survival and improving the patients' living quality. The research of immunotherapy focuses on the recruitment of tumor-infiltrating T cells. T cells are important lymphocytes in tumor immunity and play a leading role in tumor immunity by not only playing an immunosurveillance role in the body but also correcting the body's tumor immune deficiency and directly killing tumor cells [8,9]. As a malignant tumor with a high mutation rate, melanoma with high immunogenicity can produce a large number of tumor antigens to be recognized by the immune system [10]. Immune checkpoint inhibitors are a kind of immunomodulator, which has completely changed the treatment strategy of tumors and become the standard therapy for a variety of tumors, including SKCM [11]. For example, CTLA-4 monoclonal antibody is a protein expressed on the surface of T cells. After binding to CD80/CD86 of antigen-presenting cells, it can negatively regulate the immune response of T cells and block CTLA-4 to enhance the anti-tumor effect [12]. Effective immunotherapy largely depends on the immune status of the tumor microenvironment [13]. What's more, immunotherapy would be more effective if the patients contain a high density of tumor-infiltrating lymphocytes. The tumor microenvironment is the cellular environment where the tumor is located, which composed of immune cells, mesenchymal cells, endothelial cells, inflammatory mediators and extracellular matrix molecules [14]. The cells and molecules of the tumor microenvironment are in a dynamic process that reflects the evolutionary nature of cancer and jointly promotes immune escape, tumor growth, and metastasis [15]. Immune and stromal cells are two major non-tumor components that are considered to be of great value in the diagnosis and prognosis of tumors [16,17]. There is growing evidence that the tumor microenvironment is strongly correlated with the survival of patients with metastatic melanoma treated with immunotherapy [18]. Therefore, understanding the composition and function of the tumor microenvironment and screening for immune-related biological targets are essential to effectively control the progression of metastatic melanoma and improve the overall survival of patients with metastatic melanoma.
In this study, samples with metastatic melanoma were obtained from the TCGA and GSE65904 databases, and all samples were analyzed for immune, stromal and estimate scores by using the ESTIMATE algorithm. Taking differentially expressed genes (DEGs) as the object, the nine-IRGs prognostic model in metastatic melanoma was determined by univariate Cox regression, LASSO and multivariate Cox regression. This model could effectively distinguish the high and low risks of patients with metastatic melanoma, with patients in the low-risk group showing longer survival and more immune cell infiltration. Further analysis of the expression characteristics of the nine genes in the prognostic model in normal samples and in metastatic melanoma revealed that our study provided a potential model and biomarker for further immune-related work and personalized drugs for the treatment of patients with metastatic melanoma.
The RNA-seq FPKM data of skin melanoma patients, containing corresponding clinical data such as age, TNM, staging, gender, survival-time and status were downloaded from the TCGA database (https://tcga-data.nci.nih.gov/tcga/). 189 patients with metastatic melanoma in Stage III and Stage IV were screened for subsequent analysis. The gene expression data of GSE65904 were downloaded from the GEO database (https://www.ncbi.nlm.nih.gov/geo/) and 812 normal skin samples were obtained from the Genotype-Tissue Expression (GTEx) database(https://commonfund.nih.gov/GTEx/).
Stromal, immune and estimate scores for patients with metastatic melanoma were calculated by the ESTIMATE package of R software (Version: 3.6.3) in the GSE65904 and TCGA-SKCM cohorts. Survival analysis of stromal, immune and estimate scores was performed by Kaplan-Meyer analysis by using survival package. Statistical significance was tested by a log-rank test with the significance threshold for p-value set at 0.05.
Patients with metastatic melanoma from the TCGA-SKCM and GSE65904 cohorts were divided into high and low score groups based on median immune score. Differentially expressed genes were analyzed using the limma package of R software in the GSE65904 cohort. Differential expression analysis of the count matrix of the samples was performed in the TCGA-SKCM cohort using the DESeq2 package. Genes with |log fold change| > 1 and adjusted p-values < 0.05 were considered to be significantly differentially expressed genes (DEGs). Venn diagram (https://bioinfogp.cnb.csic.es/tools/venny/index.html) was used to identify overlapping DEGs. The ClusterProfiler package was used for gene ontology (GO) enrichment analysis and Kyoto Encyclopedia of Genes and Genomes (KEGG) pathway analysis for the overlapping DEGs. GO enrichment analysis focused on describing biological processes (BP), cellular components (CC) and molecular functions (MF) associated with DEGs. KEGG pathway analysis revealed biological pathways associated with DEGs.
In the TCGA database, clinical data and clinical related information were downloaded, and samples with Over Survival (OS) data were used for further survival analysis. To get survival and immune-related genes (IRGs), univariate Cox regression analysis of DEGs was performed by the "survivff" function in the survival package of R software (P < 0.05). The least absolute shrinkage and selection operator (LASSO) regression was used for dimensionality reduction analysis of survival-associated genes, and survival and glmnet packages in LASSO were used to select prognostic genes by imposing a penalty proportional to the contraction of the regression coefficients. Then, multivariate Cox regression analysis was performed using the R software survminer package to screen candidate prognostic IRGs. Finally, the regression coefficients obtained from COX regression multivariate analysis were multiplied by the expression level of each marker gene to construct the optimal prognostic model.
Patients with metastatic melanoma in the training cohort and the GSE65904 test cohort were divided into high-risk and low-risk groups based on the median value of the TCGA-SKCM training cohort risk score. Kaplan-Meier curves and log-rank tests were applied to compare the survival differences between the different risk groups. The receiver operating characteristic (ROC) curves were constructed via the R software survivalROC package and used to assess the predictive power of the risk score model. Forest plots were constructed via the R software ggplot2 package and used to show hazard ratios for selected prognostic genes.
To verify whether the prediction of the risk score model was independent of the traditional clinical characteristics of patients with metastatic melanoma, we performed univariate and multivariate Cox regression analyses on risk score, TNM, age, sex and stage. A nomogram integrating the prognostic signatures was constructed by the R software rms package for predicting OS at 1, 3 and 5 years in patients with metastatic melanoma, and calibration curves were plotted to validate the accuracy of the prediction model.
To explore the biological pathways involved between the high-risk and low-risk groups of patients, the "KEGG" and "GO" gene sets were acquired from Molecular Signatures Database (MSigDB), which were analyzed by GSEA via the GSEA software (Version: 4.0.3). Gene expression profile files were used as input phenotypic data. Genomic alignments were analyzed 1000 times [19]. P-values < 0.05 were set as statistical significance.
We used the CIBERSORT method, a generalized deconvolution algorithm based on a gene expression matrix to quantify large tissue cell fractions, to estimate the proportions of 22 immune cell phenotypes in high- and low-risk cohorts [20]. Xcell is a method for inferring the proportion of 64 immune and stromal cell types in samples using gene expression signatures and determines the enrichment score of each cell type by ssGSEA [21]. In addition, ssGSEA is a method that performs ordinal normalization based on the gene expression values of samples and then calculates an enrichment score using an empirical cumulative distribution function to assess the level of immune infiltration of the sample [22]. Wilcoxon rank-sum test was used to compare the differential abundance of immune cells in high- and low-risk cohorts. To ensure the accuracy of the algorithm, the threshold was set as P value < 0.05.
To investigate the expression of immune checkpoint inhibitors between high-risk and low-risk score groups, we analyzed the differences between high-risk and low-risk groups based on gene expression data of PDCD1, CTLA4, TIGIT, CD274, HAVR2 and LAG3 in the TCGA-SKCM cohort. Wilcoxon rank-sum test was used to determine the significance of the difference of results and P < 0.05 was considered to be significant. Box plot was plotted by the boxplot package of R software to indicate the differential expression of immune checkpoint inhibitors between high-risk and low-risk groups.
The TCGA-SKCM cohort included 189 metastatic melanoma samples with detailed overall survival information, which was suitable for the construction of WGCNA. Thus, the module signature genes and the survival data of the samples can be connected together. WGCNA analysis was performed on the top 25% of genes with the highest variance in the tumor samples by using the WGCNA package of R software. The sample hierarchical clustering method was used to detect and exclude anomalous samples before selecting the appropriate soft threshold power to implement a standard scale-free network. In the next stage, the gene dendrogram and module identification were completed with dynamic tree cutting by the construction of adjacency and topological overlap matrices (TOM) and the calculation of the corresponding phase dissimilarity, and the minimum module size was 40. Then the clustering of the module characteristic genes realized the merging of highly similar modules. Thus, the correlation between the module characteristic genes and the clinical phenotype of the sample can be calculated. The module with the highest correlation with risk scores was selected for further analysis.
The FPKM data of the TCGA-SKCM cohort was transformed into transcripts per kilobase million (TPM) values. The batch effect between different data sets was eliminated by the "sva" R package. The Log-Rank test and Kaplan-Meier method were used for survival analysis. The distributions in two sets of any continuous variable were compared using the Wilcoxon rank-sum test. Univariate and multivariate Cox regression analyses were performed using the "survival" R package. The ROC curves were generated using the "survivalROC" R package and the corresponding area under the curve was obtained. Differences were considered statistically significant at P < 0.05. All statistical analyses were performed by using R software (Version 3.6.3).
According to the ESTIMATE algorithm, for the TCGA-SKCM cohort, the stromal score ranged between -1836.9 and 1854.648, the immune score between -1002.3 and 3833.031 and the estimate score between -2696.16 and 5144.515, and for the GSE65904 cohort, the stromal score ranged between -1111.36 and 2282.686, the immune score between -570.15 and 3381.81 and estimate score between -1343.02 and 4989.343. To identify potential correlations between overall survival and immune, stromal and estimate scores, patients with metastatic melanoma based on the median score were divided into high and low score groups in the TCGA-SKCM and GSE65904 cohorts. Kaplan-Meier survival curve showed that the overall survival of the high immune, stromal and estimate score group was longer than that in the low score group (immune score, p = 0.001; stromal score, p = 0.014; estimate score, p = 0.003) in the TCGA-SKCM cohort, which was statistically significant (Figure 1A–C). Similarly, in the GSE65904 cohort, the overall survival of the high immune score group was also longer than that of the low score group (p = 0.012), while there was no significant difference in OS between patients with high and low stromal and estimate scores (stromal score, p = 0.973; estimate score, p = 0.202) (Figure 1D–F). The above results indicated that the good prognosis of patients with metastatic melanoma may be related to the remodeling of immune components.
To reveal the relationship between patients with metastatic melanoma and the tumor microenvironment, we performed the differential analysis of gene expression profiles of samples from the TCGA-SKCM and GSE65904 cohorts based on the immune scores. In the TCGA-SKCM cohort, 3716 differentially expressed genes were identified using the DESeq2 package in R software, of which 744 genes were down-regulated and 2942 genes were up-regulated (Figure 2A). In the GSE65904 cohort, 347 differentially expressed genes were identified by the limma package, of which 9 genes were down-regulated and 338 genes were up-regulated (Figure 2B). Venn analysis of the differential genes identified in the TCGA-SKCM and GSE65904 cohorts revealed 272 overlapping genes (Figure 2C), of which one gene was down-regulated and 271 genes were up-regulated. We performed functional enrichment analysis, including GO: BP, CC, MF and KEGG pathway analysis on the obtained 272 overlapping genes. GO function was mainly enriched in response to T cell activation, leukocyte cell-cell adhesion, leukocyte proliferation, and regulation of lymphocyte activation (Figure 2D), while the KEGG pathway was mainly enriched in several signaling pathways in immune diseases, such as Hematopoietic cell lineage, Allograft rejection, Graft-versus-host disease, and Type I diabetes mellitus (Figure 2E).
A univariate Cox proportional hazard regression model was used to analyze 272 overlapping differential genes and 177 genes related to survival were identified. In addition, to prevent overfitting of the model, we obtained a gene signature for 17 prognostic genes by employing the least absolute shrinkage selection operator (LASSO) regression method (Figure 3A, B). We further used multivariate COX regression analysis to establish a prognostic risk regression model containing nine genes ALOX5AP, ARHGAP15, CCL8, FCER1G, GBP4, HCK, MMP9, RARRES2 and TRIM22(Figure 3C). Risk scores for each sample were calculated based on the regression coefficients and expression levels of the nine genes. Risk score = –(0.00069*expression level of ALOX5AP) – (0.00056*expression level of ARHGAP15) – (0.00096*expression level of CCL8) – (0.00039*expression level of FCER1G) – (0.00077* expression level of GBP4) + (0.007688*expression level of HCK) – (0.00033*expression level of MMP9) – (0.00057*expression level of RARRES2) – (0.00178 * expression level of TRIM22). Patients were divided into high-risk and low-risk groups according to the median risk score. We then generated risk curves and scatter plots of risk scores and survival status for each sample in the TCGA-SKCM cohort and found that as risk scores increased, the number of deaths also increased (Figure 3D–E). Kaplan-Meier analysis showed that patients in the high-risk group had lower OS than those in the low-risk group (P < 0.05) (Figure 3F). Additionally, the area under the curve (AUC) at 1, 3 and 5 years OS was 0.712, 0.813 and 0.814, respectively (Figure 3G). Our results suggested that the nine-IRGs prognostic model for survival prediction had acceptable sensitivity and specificity.
To determine the stability of the prognostic model, the performance of the nine-IRGs prognostic model was evaluated in the GSE65904 cohort. Based on the nine-IRGs prognostic model, the risk score and survival status of each sample in the GSE65904 validation cohort were shown in the figure (Figure 4A, B). The receiver operating characteristic (ROC) curves were used to predict survival rates at 1, 3 and 5 years and the area under the curve was 0.586, 0.640 and 0.590, respectively (Figure 4C). Kaplan-Meier survival curves showed that the survival rate of high-risk patients is significantly shorter than that of low-risk patients (Figure 4D). Consistent with the results of the training cohort, in the GSE65904 validation cohort, patients with metastatic melanoma who were assigned to the high-risk group according to the prognostic model had significantly worse overall survival (OS) than those assigned to the low-risk group.
To further explore the independent predictive ability of the prognostic model, traditional clinical variables such as age, gender, tumor stage, TNM stage, and risk score were again analyzed by univariate and multivariate Cox regression analysis to evaluate the independent predictive ability of the model (T0 refers to no evidence of primary tumor; T1 refers to tumor thickness ≤0.75 mm; T2 refers to tumor thickness > 0.75 mm, < 1.5 mm; T3 refers to tumor thickness > 0.15 mm, < 4 mm; T4 refers to tumor thickness > 4 mm. N0 refers to no local lymph node metastasis; N1 refers to local lymph node metastasis, whose largest lymph node ≤3 cm; N2 refers to local lymph node metastasis, whose largest lymph node. M0 refers to no distant metastasis; M1 refers to distant metastasis). In the TCGA-SKCM cohort, age, TN stage, and risk score models were statistically significant in the univariate analysis (Figure 5A). Multivariate analysis further showed that T N stage and risk score were independent parameters associated with survival (Figure 5B).In addition, to extend the availability of the nine-IRGs prognostic model and clinical applications, a nomogram was made for clinical variables and risk scores that could be used to visually predict the 1-year, 2-year and 3-year survival times of patients (Figure 5C), and calibration curves were plotted to validate the accuracy of the prediction model (Figure 5D–F). The predicted values matched well with the actual values, indicating that our model could be used to predict the prognosis of patients with metastatic melanoma. Notably, the findings suggested that the risk score remained independent in predictive ability and could be used as an independent factor in the prognosis of patients with metastatic melanoma.
To identify potential signaling pathways and biological processes associated with the nine-IRGs prognostic model, gene set enrichment analysis (GSEA) was used between high-low and low-risk groups in the TCGA-SKCM cohort. The GO results showed that the low-risk group was mainly enriched in immune receptor activity (NES = 2.229, FDR < 0.002), cytokine receptor activity (NES = 2.202, FDR < 0.002), regulation of lymphocyte-mediated immunity (NES = 2.196, FDR < 0.002) and regulation of leukocyte-mediated immunity (NES = 2.186, FDR < 0.002) (Figure 6A). KEGG results showed that the low-risk group was mainly enriched in cytokine-cytokine receptor interaction (NES = 2.184, FDR < 0.001), natural killer cell-mediated cytotoxicity (NES = 2.034, FDR < 0.002), antigen processing and presentation (NES = 2.01, FDR < 0.002) and chemokine signaling pathway (NES = 1.960, FDR < 0.004) (Figure 6B). These results indicated that the nine-IRGs prognostic model could be potentially valuable for inferring tumor microenvironment in patients with metastatic melanoma.
To further elucidate the relationship between the prognostic model and tumor-infiltrating immune cells, we performed a series of analyses of cell types on the tumor microenvironment in the TCGA-SKCM cohort. First, 189 patients were divided into high-risk and low-risk groups based on the median risk score. The CIBERSORT algorithm was used to quantify the proportion of immune cells, and the difference of 22 immune cell types in the high-risk and low-risk groups was studied. The violin plot showed that plasma cells, CD8+ T cells, memory-activated CD4 T cells and M1 macrophages had higher infiltration levels in the low-risk group (Figure 7A). In addition, to verify the accuracy of the results, we quantified the level of tumor immune infiltration from patients with metastatic melanoma by Xcell. Visual analysis revealed that the infiltration level of plasma cells, CD8+ T cells, memory-activated CD4+ T cells and M1 macrophages in the low-risk group were significantly higher than that in the high-risk group (Figure 7B). Therefore, we concluded that metastatic melanoma patients with different risk score subtypes significantly affected the tumor microenvironment.
Since immune checkpoint inhibitors (ICIs) were used to treat malignant melanoma in clinical practice, we studied whether the risk model was related to ICIs-related biomarkers based on the TCGA and GSE65904 datasets and found that the high-risk score group was negatively correlated with the high expression of CTLA4 (p < 0.001), HAVR2 (p < 0.001), LAG3 (p < 0.001), TIGIT (p < 0.001), CD274 (p < 0.001) and PDCD1 (p < 0.001) (Figure 8). Therefore, patients in the low-risk group could have a better response to treatment with ICIs.
WGCNA is a systems biology method for analyzing the expression patterns of multiple genes in different samples, which can form clusters or modules containing genes with the same expression pattern [23]. If certain genes are located in one module, they may have the same biological function and the association between the module and the sample characteristics (such as clinical characteristics) can be studied. Three outlier samples were excluded from the TCGA-SKCM cohort so that a total of 186 samples with survival data were included in the WGCNA (Figure 9A). In this study, we chose the power of β = 5 (scale-free R2 = 0.9) as a soft threshold to implement the scale-free network. As a result, six gene co-expression modules were identified after excluding gray modules using merged dynamic tree cut (Figure 9B). The heat map plotted the TOM of the 4709 selected genes in the analysis, which indicated that each module was validated independently of the other. Subsequently, we found that the yellow-green module, containing ALOX5AP, FCER1G, GBP4, HCK, MMP9, RARRES2 and TRIM22 in the nine-IRGs prognostic model, had the highest correlation with risk scores. (R2 = -0.3, P = 3e-05) (Figure 9C). We plotted the scatter plot of GS vs MM and risk scores in the yellow-green module (Cor = 0.44, P = 1.8e-37), elucidating that ALOX5AP(GS = 0.33, MM = 0.72), FCER1G(GS = 0.23, MM = 0.24)GBP4(GS = 0.30, MM = 0.64), HCK(GS = 0.07, MM = 0.89), MMP9(GS = 0.17, MM = 0.22), RARRES2(GS = 0.28, MM = 0.39) and TRIM22(GS = 0.34, MM = 0.80) seven genes were the central genes in the yellow-green module (Figure D).
In gene networks that conform to a scale-free distribution, genes with similar expression patterns can be co-regulated, functionally related, or pathway sharing. To further explore the potential biological functions of ALOX5AP, FCER1G, GBP4, HCK, MMP9, RARRES2 and TRIM22 in metastatic melanoma, we performed GO and KEGG pathway analysis of genes associated with the yellow-green module using the "GO" and "KEGG" packages of R software. GO analysis showed that genes were mainly enriched in response to interferon-gamma, antigen processing and presentation, cellular response to interferon-gamma and adaptive immune response based on somatic recombination of immune receptors built from immunoglobulin superfamily domains (Figure 10A). KEGG pathway analysis was mainly associated with immunological diseases such as allograft rejection, autoimmune thyroid disease and viral myocarditis (Figure 10 B).
To explore the expression characteristics of the nine-IRGs between normal samples and metastatic melanoma, the Wilcoxon rank-sum test was used to analyze the expression differences of the nine-IRGs between normal samples and metastatic melanoma. The results showed that ALOX5AP (p < 0.001), ARHGAP15 (p < 0.001), CCL8 (p < 0.001), FCER1G (p < 0.001), GBP4 (p < 0.001), HCK (p < 0.001) and MMP9 (p < 0.001) were highly expressed in metastatic melanoma while RARRES2 (p = 0.001) and TRIM22 (p < 0.001) were highly expressed in normal samples, and all were differentially significant (Figure 11).
Melanoma is a highly malignant tumor with grant tendency of invasive metastasis and poor prognosis. Besides as a highly immunogenic tumor, immunotherapy has outstanding advantages in improving the efficacy of melanoma [24]. Surgical resection is valuable for certain patients with stage III and IV melanoma, but immunotherapy will expand the role of surgery in stage III and IV cancers to include removal of residual tumor and molecular detection of refractory metastatic cancer cells after exposure to systemic therapy [25]. In recent years, more and more evidence has shown that biomarkers based on tumor immunity can be used for the diagnosis, prognosis and treatment of tumor patients [26]. Therefore, it is meaningful and necessary to identify accurate biomarkers to construct prognostic characteristics to better predict patients with refractory diseases and poor survival (Figure 12).
To establish a suitable and simple protocol to observe the immune status of patients with metastatic melanoma and to suggest clinical outcomes, we used the ESTIMATE algorithm to calculate the stromal, immune and estimate scores of samples in the TCGA-SKCM and GSE65904 cohorts. Survival analysis showed that the survival time of patients in the low immune score group in the TCGA-SKCM cohort and GSE65904 cohort was significantly shorter than that in the high immune score group. Therefore, we suggest that the good prognosis of patients with metastatic melanoma may be related to remodeling of the immune component. The samples were divided into high and low scoring groups according to the median immune score for differential analysis, and 3716 and 347 differential genes were screened in the TCGA-SKCM and the GSE65904 cohorts, respectively. Venn diagram showed that there were 272 overlapping differential genes in the TCGA-SKCM and the GSE65904 cohorts. GO and KEGG analysis identified 247 overlapping differential genes were significantly associated with immune responses (such as T cell activation, Leukocyte adhesion and leukocyte proliferation). We constructed a nine-IRGs prognostic model (ALOX5AP, ARHGAP15, CCL8, FCER1G, GBP4, HCK, MMP9, RARRES2 and TRIM22) by univariate COX regression, LASSO and multivariate COX regression for 272 differential genes in the TCGA-SKCM cohort and demonstrated its validity in the GSE65904 cohort. Based on a nine-IRGs prognostic model, 189 patients were divided into high-risk and low-risk groups based on the median value of the risk score and we found that patients with high-risk group values had a poor prognosis and shorter survival time with increasing high-risk risk values. In addition, univariate COX and multivariate COX regression analyses of risk scores and other clinical factors found that risk scores could be used as independent prognostic factors. A nomograph consisting of risk scores and other clinical factors was used to predict survival. Our study suggested that the nine-IRGs can be used as prognostic markers and indicators for patients with metastatic melanoma.
To explore the relationship between high and low-risk groups and tumor-infiltrating immune cells, we used two common used methods, CIBERSORT and Xcell, to assess the difference in immune infiltration between high and low-risk groups. Due to the complexity and shortcomings of these algorithms, they are rarely compared with each other. CIBERSORT results indicated that plasma cells, T cells CD8, T cells CD4 memory activated and macrophages M1 had higher infiltration levels in the low-risk group. Xcell analysis showed the same results. Therefore, the longer survival time in the low-risk group may be related to the different immune infiltration characteristics of the patients
Interestingly, we found that the risk score correlated not only with immune cell infiltration, but also with immune checkpoint inhibitors. Immune checkpoint inhibitors (ICIs) have become an important therapeutic option for many patients with malignant melanoma over the past few years, aiming to restore and promote the function of effector T cells to specifically recognize and kill tumor cells and systemically enhance the systemic anti-tumor immune response [27]. It is a better treatment option for patients with high risk of recurrence after surgical resection or for those with advanced disease (unresectable or with metastases) [28]. Although immune checkpoint inhibitors (ICIs) have been written into the clinical application guidelines for various recurrent malignant tumors and advanced cancers, they are only effective for a long time in a part of the population, and drug resistance or immune-related adverse events (irAEs) may occur in another part of the population [3]. Currently, there are two main classes of immune checkpoint inhibitors: antibodies targeting cytotoxic T-lymphocyte antigen-4 (CTLA-4) and antibodies targeting programmed cell death protein-1 (PD-1) and its ligand programmed death receptor ligand-1 (PD-L1) [29]. Ipilimumab, the first ICIs approved for marketing by the FDA, is an antibody targeting CTLA-4 and is primarily used for the treatment of melanoma [30]. The high expression of PD-L1 protein in tumor cells or tumor immune microenvironment is considered to be a biomarker for predicting the treatment of melanoma by PD-1 inhibitors, and has been approved by the FDA as a predictive indicator for selecting patients with potential for ICI therapy [31,32]. Our study found the expression of CTLA4, HAVR2, LAG3, TIGIT, CD274 and PDCD1 is higher in the low-risk group, so it is possible that the low-risk group had a better response to immune checkpoint inhibitor therapy, which is consistent with the poor prognosis of patients in the high-risk group.
To further understand the relationship between prognostic models and genes, the top 25% of the genes with variance ranking were selected for WGCNA analysis. The results showed that the yellow-green module had the highest correlation with the risk score model and had a positive correlation with survival time, so the genes in the yellow-green module may be good prognostic genes. We also found that ALOX5AP, FCER1G, GBP4, HCK, MMP9, RARRES2 and TRIM22 of the nine-IRGs prognostic model were located in the yellow-green module. Next, we further analyzed the differences in the expression of genes used to construct the risk model in normal samples and metastatic melanoma and found that ALOX5AP, ARHGAP15, CCL8, FCER1G, GBP4, HCK, and MMP9 are highly expressed in metastatic melanoma, while RARRES2 and TRIM22 highly expressed in normal tissues. GO and KEGG analysis revealed that the genes of the yellow-green module were mainly enriched in immune-related functions and pathways such as response to interferon-gamma, antigen processing and presentation, cellular response to interferon-gamma and adaptive immune response based on somatic recombination of immune receptors built from immunoglobulin superfamily domains. Therefore, the high expression of the genes (ALOX5AP, ARHGAP15, CCL8, FCER1G, GBP4, HCK, and MMP9) used to construct the model in tumors may be due to the fact that the body activated the expression of these genes to resist the tumor after tumorigenesis, thus enhancing the local anti-tumor immune response through the expression of these genes, resulting in a good prognosis for patients with high expression of these genes.
Despite the remarkable results of this study, there were still shortcomings. Although our study provided new insights into the immune microenvironment of metastatic melanoma and related therapeutic targets, it had its limitations because it was retrospective. Therefore, further prospective studies were needed to confirm our findings.
A nine-IRGs prognostic model was identified based on the tumor microenvironment, which can effectively predict survival outcomes in patients with metastatic melanoma, and the model was validated in both internal and external datasets. This model could also predict the infiltration of immune cells in metastatic melanoma. In conclusion, our research provided a potential model and biomarker for further immune-related work and personalized medicine for the treatment of metastatic melanoma.
This study was supported by the National Natural Science Foundation of China (grant No. 31300737), the Natural Science Foundation of Guangdong Province (grant Nos. 2018A030313114, 2018A030313860 and 2020A1515010889) and the Research Development Fund of Dongguan People's Hospital (grant No. 2021-5-02).
The authors declare that there is no conflict of interests.
[1] |
X. Li, Z. Li, X. Li, B. Liu, Z. Liu, Mechanisms of Tanshinone Ⅱ a inhibits malignant melanoma development through blocking autophagy signal transduction in A375 cell, BMC Cancer, 17 (2017), 357. doi: 10.1186/s12885-017-3329-y. doi: 10.1186/s12885-017-3329-y
![]() |
[2] | Y. Si, A. Lin, W. Ding, H. Meng, P. Luo, J. Zhang, CARD11 alteration as a candidate biomarker of skin cutaneous melanoma treated with immune checkpoint blockade, Am. J. Transl. Res., 13 (2021). |
[3] |
C. Trojaniello, J. J. Luke, P. A. Ascierto, Therapeutic advancements across clinical stages in melanoma, with a focus on targeted immunotherapy, Front. Oncol., 11 (2021), 670726. doi: 10.3389/fonc.2021.670726l. doi: 10.3389/fonc.2021.670726l
![]() |
[4] |
L. Tonella, V. Pala, R. Ponti, M. Rubatto, G. Gallo, L. Mastorino, et al., Prognostic and predictive biomarkers in stage Ⅲ melanoma: current insights and clinical implications, Int. J. Mol. Sci., 22 (2021). doi: 10.3390/ijms22094561. doi: 10.3390/ijms22094561
![]() |
[5] |
A. Villani, M. Scalvenzi, G. Fabbrocini, J. Ocampo-Candiani, S. S. Ocampo-Garza, Looking into a better future: novel therapies for metastatic melanoma, Dermatol. Ther. (Heidelb), 11 (2021), 751-767. doi: 10.1007/s13555-021-00525-9. doi: 10.1007/s13555-021-00525-9
![]() |
[6] |
F. Valenti, I. Falcone, S. Ungania, F. Desiderio, P. Giacomini, C. Bazzichetto, et al., Precision medicine and melanoma: multi-omics approaches to monitoring the immunotherapy response, Int. J. Mol. Sci., 22 (2021). doi: 10.3390/ijms22083837. doi: 10.3390/ijms22083837
![]() |
[7] |
S. Upadhrasta, L. Zheng, Strategies in developing immunotherapy for pancreatic cancer: recognizing and correcting multiple immune "Defects" in the tumor microenvironment, J. Clin. Med., 8 (2019). doi: 10.3390/jcm8091472. doi: 10.3390/jcm8091472
![]() |
[8] |
E. N. Scott, A. M. Gocher, C. J. Workman, D. A. A. Vignali, Regulatory T cells: Barriers of immune infiltration into the tumor microenvironment, Front. Immunol., 12 (2021), 702726. doi: 10.3389/fimmu.2021.702726. doi: 10.3389/fimmu.2021.702726
![]() |
[9] |
A. Pasetto, Y. C. Lu, Single-cell TCR and transcriptome analysis: An indispensable tool for studying T-cell biology and cancer immunotherapy, Front. Immunol., 12 (2021), 689091. doi: 10.3389/fimmu.2021.689091. doi: 10.3389/fimmu.2021.689091
![]() |
[10] |
P. F. Ferrucci, L. Pala, F. Conforti, E. Cocorocchio, Talimogene laherparepvec (T-VEC): An intralesional cancer immunotherapy for advanced melanoma, Cancers (Basel), 13 (2021). doi: 10.3390/cancers13061383. doi: 10.3390/cancers13061383
![]() |
[11] |
L. Peng, Z. Chen, Y. Chen, X. Wang, N. Tang, MIR155HG is a prognostic biomarker and associated with immune infiltration and immune checkpoint molecules expression in multiple cancers, Cancer Med., 8 (2019), 7161-7173. doi: 10.1002/cam4.2583. doi: 10.1002/cam4.2583
![]() |
[12] |
T. Adam, T. M. Becker, W. Chua, V. Bray, T. L. Roberts, The multiple potential biomarkers for predicting immunotherapy response-finding the needle in the haystack, Cancers (Basel), 13 (2021). doi: 10.3390/cancers13020277. doi: 10.3390/cancers13020277
![]() |
[13] |
B. Hu, Q. Wei, X. Li, M. Ju, L. Wang, C. Zhou, et al., Development of an IFNgamma response-related signature for predicting the survival of cutaneous melanoma, Cancer Med., 9 (2020), 8186-8201. doi: 10.1002/cam4.3438. doi: 10.1002/cam4.3438
![]() |
[14] |
H. Ying, A. Lin, J. Liang, J. Zhang, P. Luo, Association between FSIP2 mutation and an improved efficacy of immune checkpoint inhibitors in patients with skin cutaneous melanoma, Front. Mol. Biosci., 8 (2021), 629330. doi: 10.3389/fmolb.2021.629330. doi: 10.3389/fmolb.2021.629330
![]() |
[15] |
F. Gómez-Valenzuela, E. Escobar, R. Pérez-Tomás, V. P. Montecinos, The inflammatory profile of the tumor microenvironment, orchestrated by cyclooxygenase-2, promotes epithelial-mesenchymal transition, Front. Oncol., 11 (2021). doi: 10.3389/fonc.2021.686792. doi: 10.3389/fonc.2021.686792
![]() |
[16] |
M. Kakarla, S. ChallaSivaKanaka, S. W. Hayward, O. E. Franco, Race as a Contributor to Stromal Modulation of Tumor Progression, Cancers (Basel), 13 (2021). doi: 10.3390/cancers13112656. doi: 10.3390/cancers13112656
![]() |
[17] |
X. Huang, F. Zhang, D. He, X. Ji, J. Gao, W. Liu, et al., Immune-Related Gene SERPINE1 Is a Novel Biomarker for Diffuse Lower-Grade Gliomas via Large-Scale Analysis, Front. Oncol., 11 (2021), 646060. doi: 10.3389/fonc.2021.646060. doi: 10.3389/fonc.2021.646060
![]() |
[18] |
V. Romano, I. Belviso, A. Venuta, M. R. Ruocco, S. Masone, F. Aliotta, et al., Influence of tumor microenvironment and fibroblast population plasticity on melanoma growth, therapy resistance and immunoescape, Int. J. Mol. Sci., 22 (2021). doi: 10.3390/ijms22105283. doi: 10.3390/ijms22105283
![]() |
[19] |
J. Zhang, J. Zhang, C. Yuan, Y. Luo, Y. Li, P. Dai, et al., Establishment of the prognostic index of lung squamous cell carcinoma based on immunogenomic landscape analysis, Cancer Cell Int., 20 (2020), 330. doi: 10.1186/s12935-020-01429-y. doi: 10.1186/s12935-020-01429-y
![]() |
[20] |
B. Hu, Q. Wei, C. Zhou, M. Ju, L. Wang, L. Chen, et al., Analysis of immune subtypes based on immunogenomic profiling identifies prognostic signature for cutaneous melanoma, Int. Immunopharmacol., 89 (2020). doi: 10.1016/j.intimp.2020.107162. doi: 10.1016/j.intimp.2020.107162
![]() |
[21] |
W. Y. Cai, Z. N. Dong, X. T. Fu, L. Y. Lin, L. Wang, G. D. Ye, et al., Identification of a tumor microenvironment-relevant gene set-based prognostic signature and related therapy targets in gastric cancer, Theranostics, 10 (2020), 8633-8647. doi: 10.7150/thno.47938. doi: 10.7150/thno.47938
![]() |
[22] |
Y. Mei, M. M. Chen, H. Liang, L. Ma, A four-gene signature predicts survival and anti-CTLA4 immunotherapeutic responses based on immune classification of melanoma, Commun. Biol., 4 (2021), 383. doi: 10.1038/s42003-021-01911-x. doi: 10.1038/s42003-021-01911-x
![]() |
[23] |
Z. Yang, X. Liang, Y. Fu, Y. Liu, L. Zheng, F. Liu, et al., Identification of AUNIP as a candidate diagnostic and prognostic biomarker for oral squamous cell carcinoma, EBioMedicine, 47 (2019), 44-57. doi: 10.1016/j.ebiom.2019.08.013. doi: 10.1016/j.ebiom.2019.08.013
![]() |
[24] |
I. Falcone, F. Conciatori, C. Bazzichetto, G. Ferretti, F. Cognetti, L. Ciuffreda, et al., Tumor microenvironment: Implications in melanoma resistance to targeted therapy and immunotherapy, Cancers (Basel), 12 (2020). doi: 10.3390/cancers12102870. doi: 10.3390/cancers12102870
![]() |
[25] |
C. M. Balch, Revolutionary Advances in Immunotherapy for Melanoma Are Coming into the Surgical Arena: Are We Ready?, Ann. Surg. Oncol., 25 (2018), 1803-1806. doi: 10.1245/s10434-018-6516-4. doi: 10.1245/s10434-018-6516-4
![]() |
[26] |
L. B. Song, J. C. Luan, Q. J. Zhang, L. Chen, H. Y. Wang, X. C. Cao, et al., The identification and validation of a robust immune-associated gene signature in cutaneous melanoma, J. Immunol. Res., 2021 (2021), 6686284. doi: 10.1155/2021/6686284. doi: 10.1155/2021/6686284
![]() |
[27] |
V. Petrova, I. Arkhypov, R. Weber, C. Groth, P. Altevogt, J. Utikal, et al., Modern aspects of immunotherapy with checkpoint inhibitors in melanoma, Int. J. Mol. Sci., 21 (2020). doi: 10.3390/ijms21072367. doi: 10.3390/ijms21072367
![]() |
[28] |
C. Ceci, M. G. Atzori, P. M. Lacal, G. Graziani, Targeting tumor-associated macrophages to increase the efficacy of immune checkpoint inhibitors: A glimpse into novel therapeutic approaches for metastatic melanoma, Cancers (Basel), 12 (2020). doi: 10.3390/cancers12113401. doi: 10.3390/cancers12113401
![]() |
[29] |
N. M. Ratnam, S. C. Frederico, J. A. Gonzalez, M. R. Gilbert, Clinical correlates for immune checkpoint therapy: significance for CNS malignancies, Neurooncol. Adv., 3 (2021), 161. doi: 10.1093/noajnl/vdaa161. doi: 10.1093/noajnl/vdaa161
![]() |
[30] |
T. U. Marron, A. E. Ryan, S. M. Reddy, S. Kaczanowska, R. H. Younis, D. Thakkar, et al., Considerations for treatment duration in responders to immune checkpoint inhibitors, J. Immunother. Cancer, 9 (2021). doi: 10.1136/jitc-2020-001901. doi: 10.1136/jitc-2020-001901
![]() |
[31] |
J. Han, X. Xu, Z. Liu, Z. Li, Y. Wu, D. Zuo, Recent advances of molecular mechanisms of regulating PD-L1 expression in melanoma, Int. Immunopharmacol., 88 (2020), 106971. doi: 10.1016/j.intimp.2020.106971. doi: 10.1016/j.intimp.2020.106971
![]() |
[32] |
S. Bagchi, R. Yuan, E. G. Engleman, Immune checkpoint inhibitors for the treatment of cancer: clinical impact and mechanisms of response and resistance, Annu. Rev. Pathol., 16 (2021), 223-249. doi: 10.1146/annurev-pathol-042020-042741. doi: 10.1146/annurev-pathol-042020-042741
![]() |
1. | Bo Ren, Jie Ren, Minzhi Gu, Xiaohong Liu, Lei You, Yupei Zhao, Construction of a novel model based on PVT1-MYC duet-related genes for predicting survival and characterization of the tumor microenvironment in pancreatic cancer, 2024, 15, 1664-3224, 10.3389/fimmu.2024.1435593 | |
2. | Shuang Xia, Jian-Di Li, Shi-Bai Yan, Zhi-Guang Huang, Zhi-Su Liu, Shu-Wen Jing, Da-Zhi Li, Chang Song, Yi Chen, Li-Ting Wang, Yu-Hong Zhou, Rong Huang, Nan Shi, Song-Yao Lan, Gang Chen, Xiao-Hui Fan, Clinicopathological value of hematopoietic cell kinase overexpression in laryngeal squamous cell carcinoma tissues, 2023, 247, 03440338, 154534, 10.1016/j.prp.2023.154534 | |
3. | Ping Song, Hui Deng, Yushu Liu, Mengxian Zhang, Integrated bioinformatics analysis and experimental validation reveal the relationship between ALOX5AP and the prognosis and immune microenvironment in glioma, 2024, 17, 1755-8794, 10.1186/s12920-024-01991-8 | |
4. | Gabriele Roccuzzo, Eleonora Bongiovanni, Luca Tonella, Valentina Pala, Sara Marchisio, Alessia Ricci, Rebecca Senetta, Luca Bertero, Simone Ribero, Enrico Berrino, Caterina Marchiò, Anna Sapino, Pietro Quaglino, Paola Cassoni, Emerging prognostic biomarkers in advanced cutaneous melanoma: a literature update, 2024, 24, 1473-7159, 49, 10.1080/14737159.2024.2314574 | |
5. | Marco Antonio Gómez-Guzmán, Everardo Inzunza-González, Kenia Palomino-Vizcaino, José Jaime Esqueda-Elizondo, Enrique Efren García-Guerrero, Oscar Roberto López-Bonilla, Ulises Jesús Tamayo-Perez, Laura Jiménez-Beristáin, Analysis of immunotherapeutic control of the TH1/TH2 imbalance in a 4D melanoma model applying the invariant compact set localization method, 2024, 107, 11100168, 838, 10.1016/j.aej.2024.09.023 |