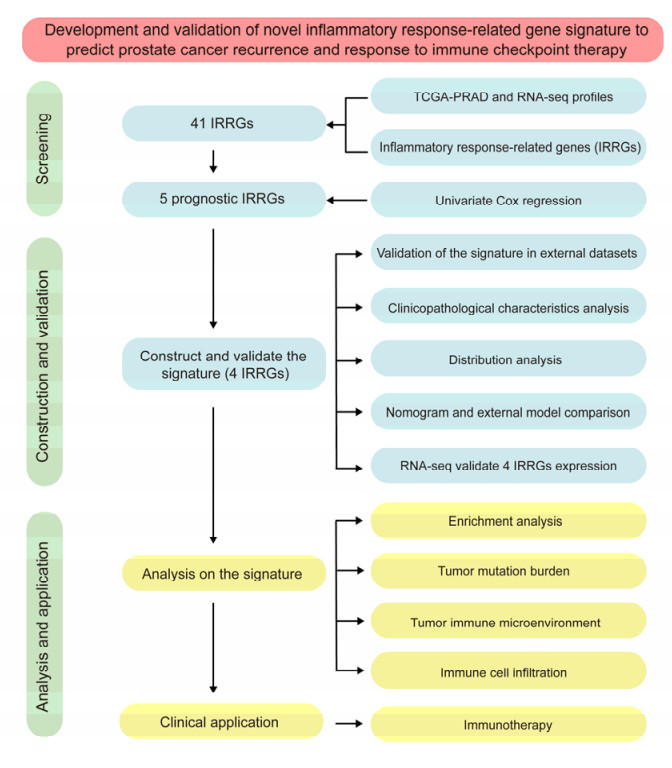
The aim of this study is to construct an inflammatory response-related genes (IRRGs) signature to monitor biochemical recurrence (BCR) and treatment effects in prostate cancer patients (PCa). A gene signature for inflammatory responses was constructed on the basis of the data from the Cancer Genome Atlas (TCGA) database, and validated in external datasets. It was analyzed using receiver operating characteristic curve, BCR-free survival, Cox regression, and nomogram. Distribution analysis and external model comparison were utilized. Then, enrichment analysis, tumor mutation burden, tumor immune microenvironment, and immune cell infiltration signatures were investigated. The role of the signature in immunotherapy was evaluated. The expression patterns of core genes were verified by RNA sequencing. We identified an IRRGs signature in the TCGA-PRAD cohort and verified it well in two other independent external datasets. The signature was a robust and independent prognostic index for predicting the BCR of PCa. The high-risk group of our signature predicted a shortened BCR time and an aggressive disease progression. A nomogram was constructed to predict BCR-free time in clinical practices. Neutrophils and CD8+ T cells were in higher abundance among the low-risk individuals. Immune functions varied significantly between the two groups and immune checkpoint therapy worked better for the low-risk patients. The expression of four IRRGs showed significant differences between PCa and surrounding benign tissues, and were validated in BPH-1 and DU145 cell lines by RNA sequencing. Our signature served as a reliable and promising biomarker for predicting the prognosis and evaluating the efficacy of immunotherapy, facilitating a better outcome for PCa patients.
Citation: Yong Luo, Xiaopeng Liu, Jingbo Lin, Weide Zhong, Qingbiao Chen. Development and validation of novel inflammatory response-related gene signature to predict prostate cancer recurrence and response to immune checkpoint therapy[J]. Mathematical Biosciences and Engineering, 2022, 19(11): 11345-11366. doi: 10.3934/mbe.2022528
[1] | Siyuan Tian, Yinan Hu, Chunmei Yang, Jiahao Yu, Jingyi Liu, Guoyun Xuan, Yansheng Liu, Keshuai Sun, Miao Zhang, Shuoyi Ma, Yulong Shang, Xia Zhou, Ying Han . A novel immune checkpoint-related gene signature for hepatocellular carcinoma to predict clinical outcomes and therapeutic response. Mathematical Biosciences and Engineering, 2022, 19(5): 4719-4736. doi: 10.3934/mbe.2022220 |
[2] | Zehao Niu, Yujian Xu, Yan Li, Youbai Chen, Yan Han . Construction and validation of a novel pyroptosis-related signature to predict prognosis in patients with cutaneous melanoma. Mathematical Biosciences and Engineering, 2022, 19(1): 688-706. doi: 10.3934/mbe.2022031 |
[3] | Yu Sheng, Jing Liu, Miao Zhang, Shuyun Zheng . Unveiling the link between inflammasomes and skin cutaneous melanoma: Insights into expression patterns and immunotherapy response prediction. Mathematical Biosciences and Engineering, 2023, 20(11): 19912-19928. doi: 10.3934/mbe.2023881 |
[4] | Xin Lin, Xingyuan Li, Binqiang Ma, Lihua Hang . Identification of novel immunomodulators in lung squamous cell carcinoma based on transcriptomic data. Mathematical Biosciences and Engineering, 2022, 19(2): 1843-1860. doi: 10.3934/mbe.2022086 |
[5] | Bin Ma, Lianqun Cao, Yongmin Li . A novel 10-gene immune-related lncRNA signature model for the prognosis of colorectal cancer. Mathematical Biosciences and Engineering, 2021, 18(6): 9743-9760. doi: 10.3934/mbe.2021477 |
[6] | Xiaowei Zhang, Jiayu Tan, Xinyu Zhang, Kritika Pandey, Yuqing Zhong, Guitao Wu, Kejun He . Aggrephagy-related gene signature correlates with survival and tumor-associated macrophages in glioma: Insights from single-cell and bulk RNA sequencing. Mathematical Biosciences and Engineering, 2024, 21(2): 2407-2431. doi: 10.3934/mbe.2024106 |
[7] | Avner Friedman, Harsh Vardhan Jain . A partial differential equation model of metastasized prostatic cancer. Mathematical Biosciences and Engineering, 2013, 10(3): 591-608. doi: 10.3934/mbe.2013.10.591 |
[8] | Hao Shen, Xiao-Dong Weng, Du Yang, Lei Wang, Xiu-Heng Liu . Long noncoding RNA MIR22HG is down-regulated in prostate cancer. Mathematical Biosciences and Engineering, 2020, 17(2): 1776-1786. doi: 10.3934/mbe.2020093 |
[9] | Ye Hu, Meiling Wang, Kainan Wang, Jiyue Gao, Jiaci Tong, Zuowei Zhao, Man Li . A potential role for metastasis-associated in colon cancer 1 (MACC1) as a pan-cancer prognostic and immunological biomarker. Mathematical Biosciences and Engineering, 2021, 18(6): 8331-8353. doi: 10.3934/mbe.2021413 |
[10] | Yan He, Nannan Cao, Yanan Tian, Xuelin Wang, Qiaohong Xiao, Xiaojuan Tang, Jiaolong Huang, Tingting Zhu, Chunhui Hu, Ying Zhang, Jie Deng, Han Yu, Peng Duan . Development and validation of two redox-related genes associated with prognosis and immune microenvironment in endometrial carcinoma. Mathematical Biosciences and Engineering, 2023, 20(6): 10339-10357. doi: 10.3934/mbe.2023453 |
The aim of this study is to construct an inflammatory response-related genes (IRRGs) signature to monitor biochemical recurrence (BCR) and treatment effects in prostate cancer patients (PCa). A gene signature for inflammatory responses was constructed on the basis of the data from the Cancer Genome Atlas (TCGA) database, and validated in external datasets. It was analyzed using receiver operating characteristic curve, BCR-free survival, Cox regression, and nomogram. Distribution analysis and external model comparison were utilized. Then, enrichment analysis, tumor mutation burden, tumor immune microenvironment, and immune cell infiltration signatures were investigated. The role of the signature in immunotherapy was evaluated. The expression patterns of core genes were verified by RNA sequencing. We identified an IRRGs signature in the TCGA-PRAD cohort and verified it well in two other independent external datasets. The signature was a robust and independent prognostic index for predicting the BCR of PCa. The high-risk group of our signature predicted a shortened BCR time and an aggressive disease progression. A nomogram was constructed to predict BCR-free time in clinical practices. Neutrophils and CD8+ T cells were in higher abundance among the low-risk individuals. Immune functions varied significantly between the two groups and immune checkpoint therapy worked better for the low-risk patients. The expression of four IRRGs showed significant differences between PCa and surrounding benign tissues, and were validated in BPH-1 and DU145 cell lines by RNA sequencing. Our signature served as a reliable and promising biomarker for predicting the prognosis and evaluating the efficacy of immunotherapy, facilitating a better outcome for PCa patients.
Prostate cancer (PCa), a highly malignant tumor of the urinary tract, is an important public health problem. 2020 saw the addition of 1,414,000 new cases and the death of 375,000 people worldwide [1]. In China, PCa has become the ninth most prevalent malignancy in 2022 [2]. It was estimated that more than 260,000 people in the United States would develop into PCa in 2022 [3]. The current common treatments for PCa include radical prostatectomy (RP), endocrinotherapy, radiotherapy, chemotherapy, and immunotherapy. Nevertheless, more than 30% of PCa patients with post-RP would undergo biochemical recurrence (BCR), which could facilitate the development of castration-resistant prostate cancer (CRPC), eventually progressing into distant metastases or PCa-specific mortality [4]. The existing clinical characteristics were not adequate for clinicians to determine the prognosis of the increasing number of PCa patients. In light of this, it was imperative to develop more accurate methods of predicting prognosis and treatment outcomes.
FDA-approved immune checkpoint therapies (ICTs) have been determined as the routine treatment of locally advanced and other metastatic cancers such as melanoma, lung cancer, bladder cancer [5,6,7]. Targeting immune checkpoints such as programmed cell death ligand 1 (PD-L1), programmed cell death protein 1 (PD-1), and cytotoxic T-lymphocyte-associated protein 4 (CTLA4) to boost anti-tumor immunity was currently one of the most prospective therapeutic regimens in immunotherapy [8]. However, multiple preclinical studies undertaken to harness its benefit for PCa have met with limited success [9,10]. Meanwhile, clinically useful biomarkers to guide treatment selection remained unclear, restricting patients from getting better treatments. ICTs were promising strategies in cancer treatments that were strongly influenced by immune microenvironmental factors [11]. Novel treatment strategies utilizing novel molecular targets, drugs, and combination of immunotherapy exhibited an enormous potential in PCa treatment, especially for CRPC [12,13]. It was well known that when the immune microenvironment was deficient in the immune infiltrates of CD8+ T cell, anti-PD-1 treatment results would not be encouraging, while patients would receive favorable results when such a high infiltration was present [14]. Identifying a reliable biomarker involved with the tumor immune microenvironment (TIME) for predicting the therapeutic sensitivity and effectiveness of ICTs appeared to be imminent.
A growing body of literatures reported that inflammation played a crucial role in the occurrence, development, and metastasis of PCa [15,16]. A significant host defense mechanism, inflammation occurred when body tissues were confronted with harmful stimuli such as irritants, pathogens, or damaged cells [17]. Nevertheless, it has also been revealed that cancer development was linked to chronic inflammation through the activation of a range of inflammatory cytokines and signals. An increased likelihood in PCa risk was paralleled with chronic inflammation, be it local or systemic. Additionally, carcinogenic alteration might induce chronic inflammation in the microenvironment, and had a variety of cancer-promoting effects in PCa, including angiogenesis, proliferation, invasion, and distant metastasis [18]. Hence, a comprehensive insight of inflammatory response-related genes (IRRGs) in PCa could enhance our understanding of the tumor immune status and provide novel and improved immunotherapeutic strategies for patients.
In this research, a unique genomic signature involved in IRRGs that could independently predict the progression and recurrence of PCa patients was determined. Superior to conventional clinical features, the signature was closely correlated with clinicopathological characteristics. Then a nomogram was constructed to apply in clinical practices. Moreover, comprehensive studies of TIME were conducted. The signature played an essential role in predicting response to immunotherapy. In all, this was an innovative approach to survival prediction and clinical treatments of PCa patients.
Firstly, the relevant clinical information and RNA sequencing transcriptome with HTSeq-FPKM format, containing 499 PCa and 52 corresponding adjacent normal samples, were extracted from the Cancer Genome Atlas database (TCGA-PRAD, https://portal.gdc.cancer.gov/projects/TCGA-PRAD). The detail information of samples' IDs was attached to our supplementary file (Table S1). All ensembl gene IDs were transferred to gene symbols using an annotation GTF file for the following analysis. Clinicopathological data included age, grade, AJCC-TNM stage, BCR-free time, and BCR status. After excluding samples without BCR events or recorded BCR time, 427 PCa samples were screened out for the following investigation. Moreover, tumor mutation burden (TMB) data of 475 PCa samples in Mutation Annotation Format was retrieved from the TCGA database and was implemented through the maftools R package [19]. Meanwhile, we downloaded copy number variation (CNV) data, including 497 PCa patients, from the TCGA database, which was analyzed based on Perl scripts and the RCircos R package [20]. The TCGA-PRAD dataset was appointed as the training cohort to build the signature. 200 IRRGs were obtained from hallmark gene sets in the gene set enrichment analysis (GSEA) Molecular Signatures Database (MSigDB, http://www.gsea-msigdb.org/gsea/msigdb/index.jsp). We extracted the GSE21034 cohort (https://www.ncbi.nlm.nih.gov/geo/query/acc.cgi?acc=GSE21034), including genomic profile of 140 PCa patients (Table S2), from the Gene Expression Omnibus (GEO) and the DKFZ cohort (https://www.cbioportal.org/study/summary?id=prostate_dkfz_2018) of 82 PCa samples (Table S3) from the cbioportal website to validate the signature. Additionally, we distinguish the mRNAs and lncRNAs from the RNA sequencing profiles, including 4 of BPH-1 (the benign prostate cell line) and 4 of DU145 (the PCa cell line). The mRNAs were applied to evaluate the differentially expressed genes (DEGs) of our signature. The total RNA was isolated and obtained from above two cell lines using TRIzol and chloroform. On the whole, we removed growth media and added 1.0 mL of TRIzol reagent directly to the culture dish to lyse a total of 3 × 106 cells. Then, we pipette the lysate up and down several times to homogenize and incubate for 5 minutes. 0.2 mL of chloroform was added for lysis and incubating for 2–3 minutes. Finally, we centrifuged the sample for 15 minutes at 12,000 × g at 4 ℃ and then transferred the aqueous phase containing the RNA to a new tube for the following study. The entire process of RNA sequencing was carried out by Sinotech Genomics (China) and the RNA seq data presented in our study has been deposited in GEO (GSE210205).
The limma package was utilized to single out differentially expressed IRRGs with a threshold of P value < 0.05 in the TCGA-PRAD dataset and RNA sequencing profile respectively [21]. Venn diagram was performed to determine the upregulated or downregulated IRRGs between the above-mentioned two datasets. In addition, the position changes of the IRRGs on the human chromosomes were depicted by R package RCircos [20]. Furthermore, the correlation network and protein-protein interaction (PPI) were constructed using the igraph and reshape2 R packages and the STRING website separately.
We determined the IRRGs with prognostic value through Cox regression analysis and developed an IRRGs signature using the least absolute shrinkage and selection operator (Lasso) regression test.
Riskscore=n∑i=1coef(i)×Exp(i) |
PCa patients were further stratified into the high- and low-risk groups, with the median risk score as the threshold. The receiver operating characteristic (ROC) curve was employed to assess the area under the curve (AUC) value and the effectiveness of prediction based on survminer, survival, and timeROC R packages. The independence of the signature was confirmed by univariate and multivariate analysis through survival R package.
Principal component analysis (PCA) and t-distributed stochastic neighbour embedding (t-SNE) were illustrated by ggplot2, scatterplot3d, and Rtsne R packages. The rms R package was adopted to set up a nomogram integrating risk score and diverse clinical variables for predicting BCR-free survival [22]. The calibration curves were depicted to evaluate the accuracy of the nomogram. We also assessed the superiority of our signature compared to other prognostic models constructed by the researchers using concordance index (C-index). Additionally, GO and KEGG enrichment analysis was analyzed by org.Hs.eg.db, enrichplot, ggplot2, and clusterProfiler R packages [23,24,25].
In order to evaluate the immune status in both groups, including estimate score, stromal score, immune score, and corresponding tumor purity, the ESTIMATE algorithm was performed by limma and ggpubr R packages. We further evaluated the immune cell infiltration levels of the core IRRGs based on TIMER database (https://cistrome.shinyapps.io/timer/) [26]. A differential immune cell abundance of hub IRRGs in PCa was determined using the QUANTISEQ, XCELL, CIBERSORT, CIBERSORT-ABS, TIMER, EPIC, and MCPCOUNTER algorithms [27]. The analysis of Wilcoxon test revealed the difference between the high-risk and low-risk groups regarding immune infiltration. The lollipop graph depicted the connection of the risk score with immune cell subtypes through Spearman correlation analysis. The discrepancy of immune landscape, comprising of immune function and immune cell subtypes, between both subgroups was assessed by the single-sample gene set enrichment approach (ssGSEA). Additionally, the tumor immune dysfunction and exclusion (TIDE) was computed by the online tool (http://tide.dfci.harvard.edu/).
A series of above-mentioned R packages were carried out to conduct statistical analyses using R version 4.1.1. Continuous variables were analyzed by Student t-test or Wilcoxon test. Categorical variables, including grade and AJCC-TNM stage, were calculated and classified as 1 (grade 1 or stage Ⅰ), 2 (grade 2 or stage Ⅱ), 3 (grade 3 or stage Ⅲ), and 4 (grade 4 or stage Ⅳ) in Cox regression and nomogram analysis. The P-value < 0.05 indicated the statistical significance.
As shown in the workflow of Figure 1, we first obtained 114 DEGs via investigating the expression patterns of 200 IRRGs in PCa and normal tissues in the TCGA database (Figure 2A), and screened out 8663 DEGs between BPH-1 and DU145 cell lines by RNA sequencing (Figure 2B). A total of 41 intersection genes between TCGA-PRAD and RNA-seq profiles, containing 10 upregulated and 31 downregulated DEGs (Tables S4 and S5), were further displayed in the Venn diagram (Figure 2C). The frequency of CNV indicated that most DEGs appeared as deletion copy numbers (Figure 2D). The CNV alteration sites of DEGs were annotated on chromosomes (Figure 2E). The correlation and PPI network of these DEGs were presented in the Figure 3A, B. As a result, a list of DEGs were identified as the hub genes, including RELA, IL1A, and IL1B, the prognostic values of which were validated in PCa [28,29]. Functional enrichment analyses indicated that these DEGs were mainly involved in regulation of inflammatory response, NF-κB signaling pathway, cytokine production, and PD-L1 expression and PD-1 checkpoint pathway in cancer (Figure 3C, D).
To obtain genes with prognostic significance from IRRGs, we determined five IRRGs associated with prognosis for the next investigation (Figure 4A). Lasso regression is widely used to eliminate multi-collinearity. After feature selection by Lasso regression and backward elimination regression, correlations within five prognostic IRRGs were dramatically reduced. In our risk signature, the coefficient of LPAR1 was 0 so that LPAR1 was excluded from the model. The formula to calculate the signature was as follow: Riskscore = -0.347 × BTG2 - 0.183 × CX3CL1 + 0.646 × KCNJ2 + 1.171 × RELA (Figure 4B, C). We stratified the patients into two groups (ie, high-risk and low-risk). It was found that there was an apparent difference in BCR-free survival between the two groups where PCa patients' mortality gradually increased with rising risk scores (Figure 4D). PCa patients in the high-risk group conferred undesirable BCR-free survival outcomes (Figure 4E). Furthermore, time-dependent ROC analysis illustrated the specificity and sensitivity of our signature in BCR-free survival prediction and the AUC values were 0.686, 0.692, and 0.674 at 1-, 3-, and 5-years respectively (Figure 4F). The results of univariate and multivariate analysis revealed our signature could act as an independent prognostic predictor for PCa patients (Figure 4G, H).
In addition, to confirm the predictive ability of the signature, we verified it in two independent external datasets. The corresponding results in the GSE21034 cohort were similar to those in the TCGA-PRAD cohort (Figure 5A). Analogously, the results of ROC, survival, and Cox regression analysis were consistent with those of the TCGA-PRAD cohort, indicating that the high-risk patients represented poorer prognosis and the validity and generalizability of our signature (Figure 5B, C, E). Additionally, the DKFZ cohort further validated our signature (Figure 5D) and the random effects model of these three cohorts based on the meta-analysis further confirmed the role of the signature as an independent prognostic factor in PCa patients (Figure 5F).
We have demonstrated the ability of the signature to predict BCR-free survival in PCa, but the connection of the signature to several clinicopathologic characteristics in the course of the disease remained to be elucidated. The results demonstrated that there were significant differences in grade, TNM stage, and survival state between the two subgroups (Figure 6A–D). Further exploring the relationship between the signature and clinical features, we found that as risk score increased, the progression was more like to develop into high-grade or advanced-stage PCa (Figure 6E). Collectively, the signature had a crucial impact on disease progression and was closely related to PCa prognosis.
t-SNE and PCA analyses showed that both subgroups were distributed in opposite directions (Figure 7A, B). Nevertheless, the IRRGs expression and the genome-wide expression profiles didn't display a clear separation (Figure 7C, D). To facilitate the assessment of prognosis in PCa patients, we constructed a nomogram integrating multiple clinical variables to quantitatively predict BCR-free survival (Figure 7E). A higher point represented a higher mortality rate for PCa patients. In addition, there was a steady consistency between nomogram-predicted probability and actual observations regarding the 1-, 2-, and 3-years BCR-free survival (Figure 7F). Furthermore, the C-index was higher than those of any other five published models [30,31,32,33,34] (Figure 7G, H). Therefore, our results indicated that the signature could serve as an effective and reliable indicator for predicting the prognosis of PCa in clinical applications.
We found that the signature was associated with many biological processes of immunity including neutrophil activation, immune response, and neutrophil mediated immunity by GO enrichment analysis (Figure 8A). Additionally, the result of KEGG pathway indicated that our signature was enriched in TNF signaling pathway, which were related to inflammatory response (Figure 8B). Next, we explored gene mutations to further obtain biological perspective into immunological characteristic of two risk groups. The waterfall plot revealed that missense variations were more frequently than other mutation types and higher mutation counts occurred in the high risk group. We then determined top 20 mutated genes in both subgroups (Figure 8C, D).
We used the ESTIMATE algorithm to find that the high-risk group possessed lower levels of infiltrating stromal cells and immune cells (Figure 9A–E). Meanwhile, the four genes that constructed the signature were also robustly related to a series of immune cell subtypes (Figure 10A–D). To further elucidate the impact of our signature on TIME, a thorough examination of immune cell infiltration in PCa was conducted. The heatmap further depicted the differences in the distribution of immune cell subtypes between both risk subgroups (Figure 11A). Multiple algorithms were applied to analyze the infiltration patterns of immune cell subtypes, the results of which indicated that the signature had a close relationship with a range of immune cell subtypes (Figure 11B). The low-risk group exhibited a significantly higher infiltrated abundance of neutrophils, macrophages, regulatory cells (Tregs), B cells, and CD8+ T cells compared to the high-risk group (Figure 11C). The results also revealed that significant differences were observed in promoting inflammation, parainflammation, human leukocyte antigen (HLA), cytolytic activity, and checkpoint between two subgroups (Figure 11C).
We examined the expression of ICT-related genes in two subgroups to understand the impact of our signature on immunotherapy. The expression of immune checkpoints such as PD-1, CTLA-4, and PD-L1 was higher in the low-risk group (Figure 12A). Moreover, analysis of HLAs gene expression disclosed that the low-risk group exhibited higher expression levels (Figure 12B). Next, The TIDE algorithm was adopted to determine the likelihood of immunotherapy response, and the results indicated that low-risk patients were suitable for ICTs (Figure 12C). PCa patients in the low-risk group might make a sensitive treatment response in ICTs applications and they should receive more attention from clinicians.
We performed RNA sequencing on BPH-1 (the benign prostate cell line) and DU145 (the PCa cell line). The results indicated higher expression of BTG2, CX3CL1 and KCNJ2 in BPH-1 and higher expression of RELA in DU145 (Figure 13A). The mRNA expression in normal samples and tumor tissues from TCGA-PRAD also showed the same results as RNA sequencing (Figure 13B). The increased expression of BTG2 predicted a favorable survival outcome in PCa patients with post-RP [35]. The overexpression of CX3CL1 enhanced spinal metastasis via activating the Src/FAK pathway in PCa [36]. Interference with KCNJ2 played a vital role in the proliferation and invasion in multiple malignancies [37,38]. Additionally, RELA was an independent predictor of BCR in PCa [28].
The currently available treatments could not meet the increasing number of PCa patients who needed better outcomes. About 34% of patients with localized disease at initial diagnosis eventually suffered BCR at ten years after RP without neoadjuvant or adjuvant therapy [39]. The median survival time from BCR to inoperable locally advanced or metastatic stage and from metastasis to death was approximately 8 and 5 years, separately [40]. However, the most clinically used TMN staging system primarily relied on anatomical information regardless of any biological information, which was out of step with the needs for clinical application. It was of crucial significance to predict the patients' survival for guiding the treatments, necessitating further insights to ascertain a biomarker that could accurately predict PCa patients' prognosis.
With the advances of high-throughput methodologies like microarrays and RNA sequencing, gene expression profiling has become an effective and practical tool for the discovery of molecular biomarkers associated with the prognosis of malignancies [41]. In our research, we have constructed an IRRGs signature including four IRRGs in the TCGA-PRAD cohort. As PCa patients were separated into the high- and low-risk groups by the intermediate risk score, the high-risk patients were obviously at poorer clinical prognosis. We determined the signature was an independent prognostic predictor for PCa patients. Subsequently, the results were well verified in two independent external datasets and the statistical power of our study was improved by a meta-analysis. Additionally, we found that the signature exhibited excellent prediction accuracy compared to five published models. Clinicopathological factors were clinically used to diagnose and differentiate PCa. However, the outcomes of some patients with similar clinical characteristics were totally different. Hence, we established a nomogram to explore the effect of the signature on patients with the same clinical features. What excited us was that our signature could still accurately distinguish the different prognoses of patients with the same clinical characteristics. The nomogram could help clinicians better formulate treatment strategies based on the patient's condition and help personalize treatments. Finally, we analyzed the differential expression between BPH-1 and DU145 cell lines through RNA sequencing, and the expression of four core IRRGs constituting the signature differed significantly in cancer and adjacent non-tumor tissues from TCGA, finding that BTG2, CX3CL1, and KCNJ2 were lowly expressed in cancer, while the expression of RELA was exactly the opposite. Collectively, the potential of our signature to predict survival of PCa patients independently and correctly at various clinical stages was comprehensively and robustly elucidated, making it possible for patients to receive better treatments.
The chronic inflammation promoted the development of PCa and contributed to prostate carcinogenesis, the microenvironment of which was composed of immune cells, stromal cells, and tumor cells [18]. The complex and diverse interactions between tumor cells and immune cells influenced tumor development and response to therapeutics [14,42]. The results revealed that our signature was enriched in neutrophil activation, immune response, and neutrophil mediated immunity. Additionally, immune function analysis further identified our signature was related to inflammation-promoting, parainflammation, and other immune processes or cell subtypes. Therefore, to further explore the effects of immune cells on the immunotherapy of PCa, a variety of algorithms were applied to explore the differences in immune cells between two subgroups. CD8+ T cells as major effector immune cells played a vital role in antitumor effects and were critical for tumorigenesis and progression [43,44].
CD8+ T cell was considered to act as an anti-tumor factor in most conditions [45,46], the cut down of which might promote BCR in PCa [47]. High abundance of CD8+ T cells in the TIME was associated with improved survival or prolonged BCR in PCa patients [47,48]. Additionally, the activation of CD8+ T cell could induce prostate inflammation [49]. Here, a close relationship between the prognosis of PCa and immune cells was revealed. This was paralleled with our result that the high-risk patients conferred a worse prognosis along with lower infiltrated levels of CD8+ T cells. Our signature not only stratified patients with different prognosis, but also effectively analyzed the status of immune infiltration cells in different PCa patients, facilitating the choice of ICTs for clinicians.
With the introduction of ICTs into the treatment of PCa, ICTs had dramatically changed the treatment landscape of metastatic CRPC patients and the scenario of immunotherapy was promising [50]. Detecting patients who could benefit most from ICTs and the combination of other treatment modalities were essential, given the relatively limited success and low overall response rates [8,51]. Some studies had found that anti-PD-1 therapy was broadly taken into clinical practice in PCa [52,53]. The expression of PD-L1 was related to the infiltrated level of CD8+ T cell and aggressive clinical progression or BCR whether in localized PCa or metastatic disease [54,55,56]. PD-L1 was not only an an independent prognostic predictor for PCa patients but also achieved higher treatment response to ICTs [57,58]. Increased immune checkpoint gene suppressed the anti-tumor immune response of T cells by increasing the expression of PD-1, CTLA4, and PD-L1 reporters. With the help of the TIDE, as a predictor of ICTs efficacy, the low-risk patients would be likely to escape from tumor immune surveillance. Our study showed that patients in the low-risk group had higher expression of PD-1, CTLA4, and PD-L1, suggesting that the low-risk patients might have a favorable response to anti-PD-1/CTLA4/PD-L1 therapy. Considering that HLA-related genes played an essential role in regulating the immune response, we compared the expression of HLA-related genes between two risk groups. The immune score and expression levels of HLA-related genes in the low-risk group were significantly higher than those in the high-risk group, while the tumor purity exhibited the opposite trend, likely explaining why the low-risk group patients were more suitable for immunotherapy. Collectively, our signature offered innovative insights into improving the clinical reaction of PCa patients to ICTs, which helped patients get more suitable and accurate treatments.
However, there were still some deficiencies in our study. Firstly, more prostate cell line quantities and types were further needed to verify our conclusions in the future. Besides, immunohistochemical staining of clinical samples from our hospital would verify the expression levels of four hub genes in PCa on the other hand.
In summary, our signature was an effective and promising IRRGs prognostic biomarker for PCa patients. The widespread regulatory mechanism of the signature in the immune landscape and immunotherapy of PCa was comprehensively elucidated. We provided a novel insight into survival prediction and immunotherapy in PCa.
This study was supported by Guangdong Medical Science and Technology Research Fund Project (B2022332, B2020127) granted to Qingbiao Chen, Foshan Science and Technology Innovation Project (2020001005794) granted to Jingbo Lin, National Natural Science Foundation of China (82072813, 81571427) and Guangzhou Municipal Science and Technology Project (201803040001) granted to Weide Zhong.
The authors declare no competing interests.
[1] |
H. Sung, J. Ferlay, R. L. Siegel, M. Laversanne, I. Soerjomataram, A. Jemal, et al., Global cancer statistics 2020: GLOBOCAN estimates of incidence and mortality worldwide for 36 cancers in 185 countries, CA Cancer J. Clin., 71 (2021), 209–249. https://doi.org/10.3322/caac.21660 doi: 10.3322/caac.21660
![]() |
[2] |
C. Xia, X. Dong, H. Li, M. Cao, D. Sun, S. He, et al., Cancer statistics in China and United States, 2022: Profiles, trends, and determinants, Chin. Med. J. Peking, 135 (2022), 584–590. https://doi.org/10.1097/CM9.0000000000002108 doi: 10.1097/CM9.0000000000002108
![]() |
[3] |
R. L. Siegel, K. D. Miller, H. E. Fuchs, A. Jemal, Cancer statistics, 2022, CA Cancer J. Clin., 72 (2022), 7–33. https://doi.org/10.3322/caac.21708 doi: 10.3322/caac.21708
![]() |
[4] |
S. R. Hawken, D. E. Spratt, J. Qi, S. M. Linsell, M. L. Cher, K. R. Ghani, et al., Utilization of salvage radiation therapy for biochemical recurrence after radical prostatectomy, Int. J. Radiat. Oncol. Biol. Phys., 104 (2019), 1030–1034. https://doi.org/10.1016/j.ijrobp.2019.01.006 doi: 10.1016/j.ijrobp.2019.01.006
![]() |
[5] |
J. D. Wolchok, H. Kluger, M. K. Callahan, M. A. Postow, N. A. Rizvi, A. M. Lesokhin, et al., Nivolumab plus ipilimumab in advanced melanoma, N. Engl. J. Med., 369 (2013), 122–133. https://doi.org/10.1056/NEJMoa1302369 doi: 10.1056/NEJMoa1302369
![]() |
[6] |
P. M. Ellis, E. T. Vella, Y. C. Ung, Immune checkpoint inhibitors for patients with advanced non-small-cell lung cancer: A systematic review, Clin. Lung Cancer, 18 (2017), 444–459. https://doi.org/10.1016/j.cllc.2017.02.001 doi: 10.1016/j.cllc.2017.02.001
![]() |
[7] |
M. Rouanne, M. Roumiguié, N. Houédé, A. Masson-Lecomte, P. Colin, G. Pignot, et al., Development of immunotherapy in bladder cancer: Present and future on targeting PD(L)1 and CTLA-4 pathways, World J. Urol., 36 (2018), 1727–1740. https://doi.org/10.1007/s00345-018-2332-5 doi: 10.1007/s00345-018-2332-5
![]() |
[8] |
S. Venkatachalam, T. R. McFarland, N. Agarwal, U. Swami, Immune checkpoint inhibitors in prostate cancer, Cancers, 13 (2021), 2187. https://doi.org/10.3390/cancers13092187 doi: 10.3390/cancers13092187
![]() |
[9] |
F. Massari, C. Ciccarese, A. Caliò, E. Munari, L. Cima, A. B. Porcaro, et al., Magnitude of PD-1, PD-L1 and T lymphocyte expression on tissue from castration-resistant prostate adenocarcinoma: An exploratory analysis, Targeted Oncol, 11 (2016), 345–351. https://doi.org/10.1007/s11523-015-0396-3 doi: 10.1007/s11523-015-0396-3
![]() |
[10] |
W. Baas, S. Gershburg, D. Dynda, K. Delfino, K. Robinson, D. Nie, et al., Immune characterization of the programmed death receptor pathway in high risk prostate cancer, Clin. Genitourin. Cancer, 15 (2017), 577–581. https://doi.org/10.1016/j.clgc.2017.04.002 doi: 10.1016/j.clgc.2017.04.002
![]() |
[11] |
R. M. Zemek, W. L. Chin, A. K. Nowak, M. J. Millward, R. A. Lake, W. J. Lesterhuis, Sensitizing the tumor microenvironment to immune checkpoint therapy, Front. Immunol., 11 (2020), 223. https://doi.org/10.3389/fimmu.2020.00223 doi: 10.3389/fimmu.2020.00223
![]() |
[12] |
K. D. Runcie, M. C. Dallos, Prostate cancer immunotherapy-finally in from the cold? Curr. Oncol. Rep., 23 (2021), 88. https://doi.org/10.1007/s11912-021-01084-0 doi: 10.1007/s11912-021-01084-0
![]() |
[13] |
H. Cha, J. H. Lee, S. Ponnazhagan, Revisiting immunotherapy: A focus on prostate cancer, Cancer Res., 80 (2020), 1615–1623. https://doi.org/10.1158/0008-5472.CAN-19-2948 doi: 10.1158/0008-5472.CAN-19-2948
![]() |
[14] |
M. Binnewies, E. W. Roberts, K. Kersten, V. Chan, D. F. Fearon, M. Merad, et al., Understanding the tumor immune microenvironment (TIME) for effective therapy, Nat. Med., 24 (2018), 541–550. https://doi.org/10.1038/s41591-018-0014-x doi: 10.1038/s41591-018-0014-x
![]() |
[15] |
K. S. Sfanos, S. Yegnasubramanian, W. G. Nelson, A. M. De Marzo, The inflammatory microenvironment and microbiome in prostate cancer development, Nat. Rev. Urol., 15 (2018), 11–24. https://doi.org/10.1038/nrurol.2017.167 doi: 10.1038/nrurol.2017.167
![]() |
[16] |
M. Archer, N. Dogra, N. Kyprianou, Inflammation as a driver of prostate cancer metastasis and therapeutic resistance, Cancers, 12 (2020), 2984. https://doi.org/10.3390/cancers12102984 doi: 10.3390/cancers12102984
![]() |
[17] |
L. Ferrero-Miliani, O. H. Nielsen, P. S. Andersen, S. E. Girardin, Chronic inflammation: Importance of NOD2 and NALP3 in interleukin-1beta generation, Clin. Exp. Immunol., 147 (2007), 227–235. https://doi.org/10.1111/j.1365-2249.2006.03261.x doi: 10.1111/j.1365-2249.2006.03261.x
![]() |
[18] |
A. K. Tewari, J. A. Stockert, S. S. Yadav, K. K. Yadav, I. Khan, Inflammation and prostate cancer, Adv. Exp. Med. Biol., 1095 (2018), 41–65. https://doi.org/10.1007/978-3-319-95693-0_3 doi: 10.1007/978-3-319-95693-0_3
![]() |
[19] |
A. Mayakonda, D. Lin, Y. Assenov, C. Plass, H. P. Koeffler, Maftools: Efficient and comprehensive analysis of somatic variants in cancer, Genome Res., 28 (2018), 1747–1756. https://doi.org/10.1101/gr.239244.118 doi: 10.1101/gr.239244.118
![]() |
[20] |
H. Zhang, P. Meltzer, S. Davis, RCircos: An R package for circos 2D track plots, BMC Bioinf., 14 (2013), 244. https://doi.org/10.1186/1471-2105-14-244 doi: 10.1186/1471-2105-14-244
![]() |
[21] |
M. E. Ritchie, B. Phipson, D. Wu, Y. Hu, C. W. Law, W. Shi, et al., Limma powers differential expression analyses for RNA-sequencing and microarray studies, Nucleic Acids Res., 43 (2015), e47. https://doi.org/10.1093/nar/gkv007 doi: 10.1093/nar/gkv007
![]() |
[22] |
Y. Luo, G. Zhang, Identification of a necroptosis-related prognostic index and associated regulatory axis in kidney renal clear cell carcinoma, Int. J. Gen. Med., 15 (2022), 5407–5423. https://doi.org/10.2147/IJGM.S367173 doi: 10.2147/IJGM.S367173
![]() |
[23] | Gene Ontology Consortium, Gene Ontology Consortium: Going forward, Nucleic Acids Res., 43 (2015), D1049–D1056. https://doi.org/10.1093/nar/gku1179 |
[24] |
G. Yu, L. Wang, Y. Han, Q. He, ClusterProfiler: An R package for comparing biological themes among gene clusters, Omics J. Integr. Biol., 16 (2012), 284–287. https://doi.org/10.1089/omi.2011.0118 doi: 10.1089/omi.2011.0118
![]() |
[25] |
M. Kanehisa, S. Goto, KEGG: Kyoto encyclopedia of genes and genomes, Nucleic Acids Res., 28 (2000), 27–30. https://doi.org/10.1093/nar/28.1.27 doi: 10.1093/nar/28.1.27
![]() |
[26] |
T. Li, J. Fan, B. Wang, N. Traugh, Q. Chen, J. S. Liu, et al., TIMER: A web server for comprehensive analysis of tumor-infiltrating immune cells, Cancer Res., 77 (2017), e108–e110. https://doi.org/10.1158/0008-5472.CAN-17-0307 doi: 10.1158/0008-5472.CAN-17-0307
![]() |
[27] |
Y. Luo, Q. Chen, J. Lin, Identification and validation of a tumor mutation burden-related signature combined with immune microenvironment infiltration in adrenocortical carcinoma, Math. Biosci. Eng., 19 (2022), 7055–7075. https://doi.org/10.3934/mbe.2022333 doi: 10.3934/mbe.2022333
![]() |
[28] |
A. Grosset, V. Ouellet, C. Caron, G. Fragoso, V. Barrès, N. Delvoye, et al., Validation of the prognostic value of NF-κB P65 in prostate cancer: A retrospective study using a large multi-institutional cohort of the Canadian prostate cancer biomarker network, PLoS Med., 16 (2019), e1002847. https://doi.org/10.1371/journal.pmed.1002847 doi: 10.1371/journal.pmed.1002847
![]() |
[29] |
H. Xu, Q. Ding, H. Jiang, Genetic Polymorphism of interleukin-1A (IL-1A), IL-1B, and IL-1 receptor antagonist (IL-1RN) and prostate cancer risk, Asian Pac. J. Cancer Prev., 15 (2014), 8741–8747. https://doi.org/10.7314/apjcp.2014.15.20.8741 doi: 10.7314/apjcp.2014.15.20.8741
![]() |
[30] |
J. P. Corradi, C. W. Cumarasamy, I. Staff, J. Tortora, A. Salner, T. McLaughlin, et al., Identification of a five gene signature to predict time to biochemical recurrence after radical prostatectomy, Prostate, 81 (2021), 694–702. https://doi.org/10.1002/pros.24150 doi: 10.1002/pros.24150
![]() |
[31] |
S. Ge, X. Hua, J. Chen, H. Xiao, L. Zhang, J. Zhou, et al., Identification of a costimulatory molecule-related signature for predicting prognostic risk in prostate cancer, Front. Genet., 12 (2021), 666300. https://doi.org/10.3389/fgene.2021.666300 doi: 10.3389/fgene.2021.666300
![]() |
[32] |
H. Liu, L. Gao, T. Xie, J. Li, T. Zhai, Y. Xu, Identification and validation of a prognostic signature for prostate cancer based on ferroptosis-related genes, Front. Oncol., 11 (2021), 623313. https://doi.org/10.3389/fonc.2021.623313 doi: 10.3389/fonc.2021.623313
![]() |
[33] |
Z. Sun, Y. Mao, X. Zhang, S. Lu, H. Wang, C. Zhang, et al., Identification of ARHGEF38, NETO2, GOLM1, and SAPCD2 associated with prostate cancer progression by bioinformatic analysis and experimental validation, Front. Cell Dev. Biol., 9 (2021), 718638. https://doi.org/10.3389/fcell.2021.718638 doi: 10.3389/fcell.2021.718638
![]() |
[34] |
H. Zhao, Y. Zeng, Z. Han, Y. Zhuo, Y. Liang, C. T. Hon, et al., Novel immune-related signature for risk stratification and prognosis in prostatic adenocarcinoma, Cancer Sci., 112 (2021), 4365–4376. https://doi.org/10.1111/cas.15062 doi: 10.1111/cas.15062
![]() |
[35] |
S. E. Jalava, A. Urbanucci, L. Latonen, K. K. Waltering, B. Sahu, O. A. Jänne, et al., Androgen-regulated miR-32 targets BTG2 and is overexpressed in castration-resistant prostate cancer, Oncogene, 31 (2012), 4460–4471. https://doi.org/10.1038/onc.2011.624 doi: 10.1038/onc.2011.624
![]() |
[36] |
P. Liu, Y. Liang, L. Jiang, H. Wang, S. Wang, J. Dong, CX3CL1/fractalkine enhances prostate cancer spinal metastasis by activating the Src/FAK pathway, Int. J. Oncol., 53 (2018), 1544–1556. https://doi.org/10.3892/ijo.2018.4487 doi: 10.3892/ijo.2018.4487
![]() |
[37] |
S. Chen, M. Huang, X. Hu, Interference with KCNJ2 inhibits proliferation, migration and EMT progression of apillary thyroid carcinoma cells by upregulating GNG2 expression, Mol. Med. Rep., 24 (2021), 1–10. https://doi.org/10.3892/mmr.2021.12261 doi: 10.3892/mmr.2021.12261
![]() |
[38] |
C. Ji, Y. Wang, D. Xiang, Q. Liu, Z. Zhou, F. Qian, et al., Kir2.1 interaction with Stk38 promotes invasion and metastasis of human gastric cancer by enhancing MEKK2-MEK1/2-ERK1/2 signaling, Cancer Res., 78 (2018), 3041–3053. https://doi.org/10.1158/0008-5472.CAN-17-3776 doi: 10.1158/0008-5472.CAN-17-3776
![]() |
[39] |
L. Liesenfeld, M. Kron, J. E. Gschwend, K. Herkommer, Prognostic factors for biochemical recurrence more than 10 years after radical prostatectomy, J. Urol., 197 (2017), 143–148. https://doi.org/10.1016/j.juro.2016.07.004 doi: 10.1016/j.juro.2016.07.004
![]() |
[40] |
C. R. Pound, A. W. Partin, M. A. Eisenberger, D. W. Chan, J. D. Pearson, P. C. Walsh, Natural history of progression after PSA elevation following radical prostatectomy, JAMA, 281 (1999), 1591–1597. https://doi.org/10.1001/jama.281.17.1591 doi: 10.1001/jama.281.17.1591
![]() |
[41] |
S. Shen, R. Zhang, Y. Jiang, Y. Li, L. Lin, Z. Liu, et al., Comprehensive analyses of m6A regulators and interactive coding and non-coding RNAs across 32 cancer types, Mol. Cancer, 20 (2021), 67. https://doi.org/10.1186/s12943-021-01362-2 doi: 10.1186/s12943-021-01362-2
![]() |
[42] |
T. Wu, Y. Dai, Tumor microenvironment and therapeutic response, Cancer Lett., 387 (2017), 61–68. https://doi.org/10.1016/j.canlet.2016.01.043 doi: 10.1016/j.canlet.2016.01.043
![]() |
[43] |
M. St Paul, P. S. Ohashi, The roles of CD8(+) T cell subsets in antitumor immunity, Trends Cell Biol., 30 (2020), 695–704. https://doi.org/10.1016/j.tcb.2020.06.003 doi: 10.1016/j.tcb.2020.06.003
![]() |
[44] |
M. Philip, A. Schietinger, CD8(+) T cell differentiation and dysfunction in cancer, Nat. Rev. Immunol., 22 (2022), 209–223. https://doi.org/10.1038/s41577-021-00574-3 doi: 10.1038/s41577-021-00574-3
![]() |
[45] |
J. Qiao, Z. Liu, C. Dong, Y. Luan, A. Zhang, C. Moore, et al., Targeting tumors with IL-10 prevents dendritic cell-mediated CD8(+) T cell apoptosis, Cancer Cell, 35 (2019), 901–915. https://doi.org/10.1016/j.ccell.2019.05.005 doi: 10.1016/j.ccell.2019.05.005
![]() |
[46] |
G. M. Lynn, C. Sedlik, F. Baharom, Y. Zhu, R. A. Ramirez-Valdez, V. L. Coble, et al., Peptide-TLR-7/8a conjugate vaccines chemically programmed for nanoparticle self-assembly enhance CD8 T-cell immunity to tumor antigens, Nat. Biotechnol., 38 (2020), 320–332. https://doi.org/10.1038/s41587-019-0390-x doi: 10.1038/s41587-019-0390-x
![]() |
[47] |
S. Wang, W. Su, C. Zhong, T. Yang, W. Chen, G. Chen, et al., An eight-circRNA assessment model for predicting biochemical recurrence in prostate cancer, Front. Cell Dev. Biol., 8 (2020), 599494. https://doi.org/10.3389/fcell.2020.599494 doi: 10.3389/fcell.2020.599494
![]() |
[48] |
Y. Yanai, T. Kosaka, S. Mikami, H. Hongo, Y. Yasumizu, T. Takeda, et al., CD8-positive T cells and CD204-positive M2-like macrophages predict postoperative prognosis of very high-risk prostate cancer, Sci. Rep., 11 (2021), 22495. https://doi.org/10.1038/s41598-021-01900-4 doi: 10.1038/s41598-021-01900-4
![]() |
[49] |
J. R. Lees, B. Charbonneau, J. D. Hayball, K. Diener, M. Brown, R. Matusik, et al., T-cell recognition of a prostate specific antigen is not sufficient to induce prostate tissue destruction, Prostate, 66 (2006), 578–590. https://doi.org/10.1002/pros.20307 doi: 10.1002/pros.20307
![]() |
[50] |
V. R. de Porras, J. C. Pardo, L. Notario, O. Etxaniz, A. Font, Immune checkpoint inhibitors: A promising treatment option for metastatic castration-resistant prostate cancer, Int. J. Mol. Sci., 22 (2021), 4712. https://doi.org/10.3390/ijms22094712 doi: 10.3390/ijms22094712
![]() |
[51] |
M. Bilusic, R. A. Madan, J. L. Gulley, Immunotherapy of prostate cancer: Facts and hopes, Clin. Cancer Res., 23 (2017), 6764–6770. https://doi.org/10.1158/1078-0432.CCR-17-0019 doi: 10.1158/1078-0432.CCR-17-0019
![]() |
[52] |
E. S. Antonarakis, J. M. Piulats, M. Gross-Goupil, J. Goh, K. Ojamaa, C. J. Hoimes, et al., Pembrolizumab for treatment-refractory metastatic castration-resistant prostate cancer: Multicohort, open-label phase Ⅱ KEYNOTE-199 study, J. Clin. Oncol., 38 (2020), 395–405. https://doi.org/10.1200/JCO.19.01638 doi: 10.1200/JCO.19.01638
![]() |
[53] |
A. R. Hansen, C. Massard, P. A. Ott, N. B. Haas, J. S. Lopez, S. Ejadi, et al., Pembrolizumab for advanced prostate adenocarcinoma: Findings of the KEYNOTE-028 study, Ann. Oncol., 29 (2018), 1807–1813. https://doi.org/10.1093/annonc/mdy232 doi: 10.1093/annonc/mdy232
![]() |
[54] |
R. Mo, Z. Han, Y. Liang, J. Ye, S. Wu, S. X. Lin, et al., Expression of PD-L1 in tumor-associated nerves correlates with reduced CD8(+) tumor-associated lymphocytes and poor prognosis in prostate cancer, Int. J. Cancer, 144 (2019), 3099–3110. https://doi.org/10.1002/ijc.32061 doi: 10.1002/ijc.32061
![]() |
[55] |
F. Petitprez, N. Fossati, Y. Vano, M. Freschi, E. Becht, R. Lucianò, et al., PD-L1 expression and CD8(+) T-cell infiltrate are associated with clinical progression in patients with node-positive prostate cancer, Eur. Urol. Focus, 5 (2019), 192–196. https://doi.org/10.1016/j.euf.2017.05.013 doi: 10.1016/j.euf.2017.05.013
![]() |
[56] |
C. Vicier, P. Ravi, L. Kwak, L. Werner, Y. Huang, C. Evan, et al., Association between CD8 and PD-L1 expression and outcomes after radical prostatectomy for localized prostate cancer, Prostate, 81 (2021), 50–57. https://doi.org/10.1002/pros.24079 doi: 10.1002/pros.24079
![]() |
[57] |
H. Gevensleben, D. Dietrich, C. Golletz, S. Steiner, M. Jung, T. Thiesler, et al., The immune checkpoint regulator PD-L1 is highly expressed in aggressive primary prostate cancer, Clin. Cancer Res., 22 (2016), 1969–1977. https://doi.org/10.1158/1078-0432.CCR-15-2042 doi: 10.1158/1078-0432.CCR-15-2042
![]() |
[58] |
B. T. Rekoske, B. M. Olson, D. G. McNeel, Antitumor vaccination of prostate cancer patients elicits PD-1/PD-L1 regulated antigen-specific immune responses, Oncoimmunology, 5 (2016), e1165377. https://doi.org/10.1080/2162402X.2016.1165377 doi: 10.1080/2162402X.2016.1165377
![]() |
![]() |
![]() |