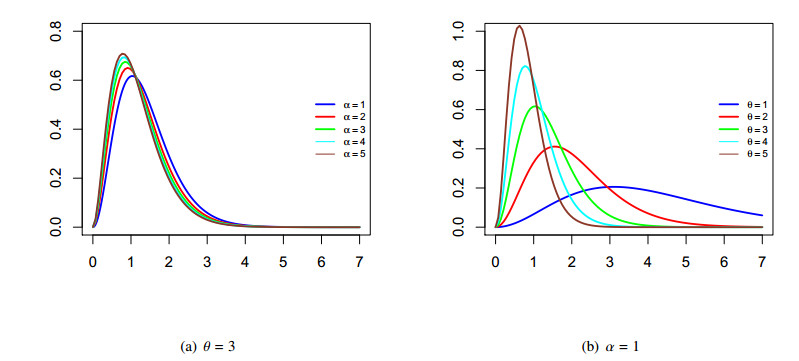
The components of the immune system develop in utero and like a computer, some components are immediately functional (the innate components) but other components must learn the programs and details necessary to function (antigen adaptive components). Like other systems, including military and municipal, the innate and antigen specific components develop into an immune system that helps maintain and surveil the other body processes and systems for aberrations, provide surveillance and protection of the mucoepithelial borders and protection from microbial invasion. Inability, excesses, or errors in these processes cause disease. Aging of the immune system brings immunosenescence, inflammaging, more errors, and decreased surveillance which increases risk for new infections (e.g. COVID-19, influenza), recurrence of latent infections, cancer and autoimmune and inflammatory diseases. With greater understanding of the surveillance, effector and regulatory deficits upon aging, better therapies can be developed.
Citation: Ken S. Rosenthal, Jordan B. Baker. The immune system through the ages[J]. AIMS Allergy and Immunology, 2022, 6(3): 170-187. doi: 10.3934/Allergy.2022013
[1] | M. R. Irshad, S. Aswathy, R. Maya, Amer I. Al-Omari, Ghadah Alomani . A flexible model for bounded data with bathtub shaped hazard rate function and applications. AIMS Mathematics, 2024, 9(9): 24810-24831. doi: 10.3934/math.20241208 |
[2] | Doaa Basalamah, Bader Alruwaili . The weighted Lindley exponential distribution and its related properties. AIMS Mathematics, 2023, 8(10): 24984-24998. doi: 10.3934/math.20231275 |
[3] | Tabassum Naz Sindhu, Zawar Hussain, Naif Alotaibi, Taseer Muhammad . Estimation method of mixture distribution and modeling of COVID-19 pandemic. AIMS Mathematics, 2022, 7(6): 9926-9956. doi: 10.3934/math.2022554 |
[4] | M. G. M. Ghazal, Yusra A. Tashkandy, Oluwafemi Samson Balogun, M. E. Bakr . Exponentiated extended extreme value distribution: Properties, estimation, and applications in applied fields. AIMS Mathematics, 2024, 9(7): 17634-17656. doi: 10.3934/math.2024857 |
[5] | Rashad M. EL-Sagheer, Mohamed S. Eliwa, Khaled M. Alqahtani, Mahmoud El-Morshedy . Bayesian and non-Bayesian inferential approaches under lower-recorded data with application to model COVID-19 data. AIMS Mathematics, 2022, 7(9): 15965-15981. doi: 10.3934/math.2022873 |
[6] | Fiaz Ahmad Bhatti, Azeem Ali, G. G. Hamedani, Mustafa Ç. Korkmaz, Munir Ahmad . The unit generalized log Burr XII distribution: properties and application. AIMS Mathematics, 2021, 6(9): 10222-10252. doi: 10.3934/math.2021592 |
[7] | Ahmed M. Gemeay, Najwan Alsadat, Christophe Chesneau, Mohammed Elgarhy . Power unit inverse Lindley distribution with different measures of uncertainty, estimation and applications. AIMS Mathematics, 2024, 9(8): 20976-21024. doi: 10.3934/math.20241021 |
[8] | Refah Alotaibi, Hassan Okasha, Hoda Rezk, Abdullah M. Almarashi, Mazen Nassar . On a new flexible Lomax distribution: statistical properties and estimation procedures with applications to engineering and medical data. AIMS Mathematics, 2021, 6(12): 13976-13999. doi: 10.3934/math.2021808 |
[9] | Emrah Altun, Mustafa Ç. Korkmaz, M. El-Morshedy, M. S. Eliwa . The extended gamma distribution with regression model and applications. AIMS Mathematics, 2021, 6(3): 2418-2439. doi: 10.3934/math.2021147 |
[10] | Ayed. R. A. Alanzi, Muhammad Imran, M. H. Tahir, Christophe Chesneau, Farrukh Jamal, Saima Shakoor, Waqas Sami . Simulation analysis, properties and applications on a new Burr XII model based on the Bell-X functionalities. AIMS Mathematics, 2023, 8(3): 6970-7004. doi: 10.3934/math.2023352 |
The components of the immune system develop in utero and like a computer, some components are immediately functional (the innate components) but other components must learn the programs and details necessary to function (antigen adaptive components). Like other systems, including military and municipal, the innate and antigen specific components develop into an immune system that helps maintain and surveil the other body processes and systems for aberrations, provide surveillance and protection of the mucoepithelial borders and protection from microbial invasion. Inability, excesses, or errors in these processes cause disease. Aging of the immune system brings immunosenescence, inflammaging, more errors, and decreased surveillance which increases risk for new infections (e.g. COVID-19, influenza), recurrence of latent infections, cancer and autoimmune and inflammatory diseases. With greater understanding of the surveillance, effector and regulatory deficits upon aging, better therapies can be developed.
Recently, some researchers in statistics have been interested in generating new flexible statistical models based on different techniques such as the weight distributions which are commonly used in many fields of life situations such as sciences, ecology, biostatistics, medicine, engineering, pharmacy and environment and so on.
The concept of weighted distribution is suggested by [15] to study how verification methods can affect the form of the distribution of recorded observations. Later, the weighted distributions are unified and formulated by [31] in general terms in connection with modeling statistical data.
Suppose X is a non-negative random variable with a probability density function (pdf) f(x). Let W(x) be a non negative weight function, then the probability density function of the weighted random variable Xw is given by:
fw(x)=W(x)f(x)E(W(x)). | (1.1) |
If the weight function has the form W(x)=xλ, the resulting distribution is known as a size biased distribution of order λ with pdf given by:
fλ(x)=xλf(x)E(Xλ). | (1.2) |
If λ = or 2, the yielded distributions are known as the length biased and area biased distributions, respectively.
For example, the length-biased Suja distribution is proposed by [5] as a generalization of the Suja distribution. The size biased Ishita distribution is offered by [6] as a new modification of the Ishita distribution. The Marshall-Olkin length-biased exponential distribution is suggested by [18]. The length-biased weighted generalized Rayleigh distribution with its properties is proposed by [2]. The weighted Lomax distribution is introduced by [23]. Other types of distributions are also suggested based on other procedures as [8] for the exponentiated new Weibull-Pareto distribution. The Topp-Leone Mukherjee-Islam distribution is offered by [7]. Also, see [27,16,17,26].
To the best of our knowledge, the use of the weighted method to extend the generalized Quasi Lindley distribution introduced by [9] is still unexplored in the literature. In this study, the weighted generalized Quasi Lindley distribution is proposed. Indeed, the importance of the suggestion of the WGQLD arises from the fact it is a modification of the well known Quasi Lindley distribution which is considered by many researchers in different life situations.
The layout of this paper is organized as follows. Section 2 concerns with the pdf and cumulative distribution function (cdf) of the WGQLD and its shapes. Moment generating function and moments includes the rth moment, variance, the coefficient of skewness, kurtosis, and variation are presented in Section 3 theoretically and supported by some simulations. The distribution of order statistics, median deviations and harmonic mean are presented in Section 4. The stochastic ordering, reliability, hazard, reversed hazard rate and odds functions are given in Section 5. Bonferroni and Lorenz curves as well as the Gini index are provided in Section 6. In Section 7, different methods of estimation for the distribution parameters are discussed including maximum likelihood, maximum product of spacing's, ordinary least squares, weighted least squares, Cramer-von-Mises, and Anderson-Darling methods. A simulation study is conducted to compare the performance of the proposed estimators in Section 8. Illustrative examples of real data applications are given in Section 9. The paper is concluded with some suggestions for future works in Section 10.
This section introduces the pdf and cdf of the WGQLD along with the shapes of the model. The probability density function of the generalized Quasi Lindley distribution is given by:
fGQLD(x;θ,α)=θ2(θ2x36+αθx2+α2x)(α+1)2e−θx; x≥0, α>−1, θ≥0, | (2.1) |
and the corresponding cdf is:
FGQLD(x;θ,α)=1−(θ3x3+3(2α+1)θ2x2+6(α+1)2(θx+1))6(α+1)2e−θx. | (2.2) |
With reference to Eq 1.1, and in this work without loss of generality we considered the weight function as W(x)=x given that the mean of the GQLD is E(X)=2(2+α)θ(1+α). Hence, the weighted generalized Quasi Lindley distribution can be obtained by substituting fGQLD(x;θ,α), E(X) and W(x) in Eq 1.1 to get:
fWGQLD(x;θ,α)=θ32(α2+3α+2)(θ2x46+αθx3+α2x2)e−θx; x≥0, α>−1, θ≥0. | (2.3) |
Figures 1 and 2 demonstrate the graphs of the pdf of the WGQLD for different values of θ and α. Also, Figures 3 and 4 show various curves of the cdf of WGQLD for selected distribution parameters.
Based on Figures 1 and 2, it can be observed that the WGQLD is a unimodel and positively skewed. Also, it is approximately symmetric as in Figure 2 when θ=1 for some parameters. The curve of the distribution function is more flat for α=1,θ=0.25 and when α=θ=1. than other values.
The corresponding cdf equation of the WGQLD is given by
FWGQLD(x;θ,α)=1−24+6α2[2+xθ(2+xθ)]+6α[6+xθ(6+xθ(3+xθ))]+xθ[24+xθ(12+xθ(4+xθ))]12(1+α)(2+α)e−θx. | (2.4) |
Figure 3 reveals that the cdf plots are approach 1 when θ=3 (a) faster than that of α=1 (b). In comparing Figure 3(b) with Figure 4(b), it can be observed that the cdf plots are more spread with smaller values of θ. However, the same thing can be concluded in comparing Figure 3(a) with Figure 4(a).
Theorem 1. : Let X∼fWGQLD(x,θ,α), then the rth moment of X about the origin is:
E(Xr)=(r2+6(1+α)(2+α)+r(7+6α))Γ(3+r)12θr(2+3α+α2),α>−1,θ>0, r=1,2,3... | (3.1) |
Proof. : The proof is direct by using E(Xr)=∫∞0xrf(x,θ,α)dx as
E(Xr)=∞∫0xrθ32(α2+3α+2)(α2x2+αθx3+θ2x46)e−θxdx=θ32(α2+3α+2)∞∫0(xrα2x2e−θx+xrαθx3e−θx+xrθ2x46e−θx)dx=θ32(α2+3α+2)(∞∫0xrα2x2e−θxdx+∞∫0xrαθx3e−θxdx+∞∫0xrθ2x46e−θxdx)=θ32(α2+3α+2)(∞∫0xr+2α2e−θxdx+∞∫0xr+3αθe−θxdx+∞∫016xr+4θ2e−θxdx)=θ32(α2+3α+2)(α2(r+2)!θr+3+αθ(r+3)!θr+4+θ2(r+3)!6θr+5)=θ32(α2+3α+2)(α2(r+2)!θr+3+α(r+3)(r+2)!θr+3+(r+4)(r+3)(r+2)!6θr+3)=(r+2)!12θr(α2+3α+2)(6α2+6α(r+3)+(r+4)(r+3))=[r2+6(1+α)(2+α)+r(7+6α)]Γ[3+r]12θr(2+3α+α2). |
Based on Eq 7, it is simple to have the first, second, third and fourth moments of the WGQLD, respectively, as
E(X)=6(α+1)(α+2)+6α+82(α2+3α+2)θ=3α(α+4)+10(α+1)(α+2)θ, | (3.2) |
E(X2)=2(2(6α+7)+6(α+1)(α+2)+4)(α2+3α+2)θ2=12(α(α+5)+5)(α+1)(α+2)θ2, | (3.3) |
E(X3)=10(3(6α+7)+6(α+1)(α+2)+9)(α2+3α+2)θ3=60(α(α+6)+7)(α+1)(α+2)θ3, | (3.4) |
E(X4)=60(4(6α+7)+6(α+1)(α+2)+16)(α2+3α+2)θ4=120(3α(α+7)+28)(α+1)(α+2)θ4. | (3.5) |
Therefore, the variance of the WGQLD can be obtained as
V(X)=E(X2)−(E(X))2=3α4+24α3+60α2+60α+20(α+1)2(α+2)2θ2. | (3.6) |
The distribution shape analysis can be performed by studying the coefficient of skewness, coefficient of kurtosis, and coefficient of variation. For the WGQLD, these coefficients, respectively, are given by:
SKWGQLD=2(3α6+36α5+150α4+306α3+330α2+180α+40)(α+1)(α+2)(α+1)(α+2)(3α4+24α3+60α2+60α+20)32, | (3.7) |
KuWGQLD=315α8+240α7+1496α6+4968α5+9776α4+11760α3+8480α2+3360α+560(3α4+24α3+60α2+60α+20)2, | (3.8) |
CVWGQLD=(α+1)(α+2)√3α4+24α3+60α2+60α+20(3α2+12α+10)(α+1)(α+2). | (3.9) |
It is of interest to note here that the coefficient of skewness, coefficient of variation and coefficient of kurtosis are free of θ. Table (1) presents some values of the mean, standard deviation, coefficient of variation, coefficient of skewness and coefficient of kurtosis of WGQLD for various values of the parameters α and θ.
μWGQLD | σWGQLD | SKWGQLD | KuWGQLD | CVWGQLD | |
α | θ=1.25 | ||||
0.1 | 3.889177 | 1.786969 | 0.897106 | 4.205054 | 0.459472 |
0.2 | 3.793939 | 1.782395 | 0.903213 | 4.217285 | 0.469801 |
0.3 | 3.711037 | 1.776240 | 0.910968 | 4.233681 | 0.478637 |
0.4 | 3.638095 | 1.769174 | 0.919440 | 4.252427 | 0.486291 |
0.5 | 3.573333 | 1.761615 | 0.928126 | 4.272400 | 0.492989 |
0.6 | 3.515385 | 1.753829 | 0.936749 | 4.292896 | 0.498901 |
0.7 | 3.463181 | 1.745987 | 0.945154 | 4.313464 | 0.504157 |
0.8 | 3.415873 | 1.738199 | 0.953260 | 4.333820 | 0.508859 |
0.9 | 3.372777 | 1.730537 | 0.961027 | 4.353780 | 0.513090 |
1 | 3.333333 | 1.723046 | 0.968437 | 4.373230 | 0.516914 |
1.1 | 3.297081 | 1.715755 | 0.975492 | 4.392101 | 0.520386 |
1.2 | 3.263636 | 1.708680 | 0.982196 | 4.410356 | 0.523551 |
1.3 | 3.232675 | 1.701828 | 0.988563 | 4.427977 | 0.526446 |
1.4 | 3.203922 | 1.695202 | 0.994608 | 4.444961 | 0.529102 |
1.5 | 3.177143 | 1.688801 | 1.000346 | 4.461313 | 0.531547 |
θ | α=2 | ||||
0.1 | 38.333333 | 20.749833 | 1.025012 | 4.534152 | 0.5413 |
0.2 | 19.166667 | 10.374916 | 1.025012 | 4.534152 | 0.5413 |
0.3 | 12.777778 | 6.916611 | 1.025012 | 4.534152 | 0.5413 |
0.4 | 9.583333 | 5.187458 | 1.025012 | 4.534152 | 0.5413 |
0.5 | 7.666667 | 4.149967 | 1.025012 | 4.534152 | 0.5413 |
0.6 | 6.388889 | 3.458305 | 1.025012 | 4.534152 | 0.5413 |
0.7 | 5.476190 | 2.964262 | 1.025012 | 4.534152 | 0.5413 |
0.8 | 4.791667 | 2.593729 | 1.025012 | 4.534152 | 0.5413 |
0.9 | 4.259259 | 2.305537 | 1.025012 | 4.534152 | 0.5413 |
1 | 3.833333 | 2.074983 | 1.025012 | 4.534152 | 0.5413 |
1.1 | 3.484848 | 1.886348 | 1.025012 | 4.534152 | 0.5413 |
1.2 | 3.194444 | 1.729153 | 1.025012 | 4.534152 | 0.5413 |
1.3 | 2.948718 | 1.596141 | 1.025012 | 4.534152 | 0.5413 |
1.4 | 2.738095 | 1.482131 | 1.025012 | 4.534152 | 0.5413 |
1.5 | 2.555556 | 1.383322 | 1.025012 | 4.534152 | 0.5413 |
It can be noted that the mean and the standard deviation are decreasing when values of α and θ are increasing for fixed values of θ and α, respectively. The results of simulation emphasize that the coefficient of skewness, coefficient of variation and coefficient of kurtosis don't depend on θ and increasing when α values are increasing.
The moment generating function (MGF) of the WGQLD is given in the following theorem.
Theorem 2. : Let X∼fWGQLD(x,θ,α), then the MGF of X about the origin is:
M(t)=θ3(θ+αθ−tα)((2+α)θ−tα)(2+3α+α2)(θ−t)5. | (3.10) |
Proof. : To prove the MGF of the WGQLD, let
E(etx)=∞∫0etxθ32(α2+3α+2)(α2x2+αθx3+θ2x46)e−θxdx=θ32(α2+3α+2)(∞∫0α2x2e−(θ−t)xdx+∞∫0αθx3e−(θ−t)xdx+∞∫0θ2x46e−(θ−t)xdx)=θ32(α2+3α+2)(α22!(θ−t)3+αθ3!(θ−t)4+θ24!6(θ−t)5)=2θ32(α2+3α+2)(α2(θ−t)3+3αθ(θ−t)4+2θ2(θ−t)5)=θ3(α2+3α+2)(θ−t)3(α2+3αθ(θ−t)+2θ2(θ−t)2)=θ3(α2+3α+2)(θ−t)3(t2α2−2tθα2+θ2α2−3tαθ+3αθ2+2θ2(θ−t)2)=θ3(αθ−αt+θ)((α+2)θ−αt)(α2+3α+2)(θ−t)5. |
Let X1,X2,…,Xm be a random sample of size m from a distribution with pdf f(x) and cdf F(x). The pdf of the ith order statistic X(j:m) for j=1,2,…,m are defined by [14] as
f(j:m)(x)=m!(j−1)(m−j)F(x)]j[1−F(x)]m−jf(x). | (4.1) |
Based on Eq (4.1), the pdf of the ith order statistic, X(i:m), from the WGQLD, will be
f(j:m)(x)=θ3(C+1)j−122j−2m−13j−mΓ(m+1)e−θxAm−j(θ2x46+αθx3+α2x2)(α+1)(α+2)Γ(j)Γ(−j+m+1), | (4.2) |
where
A=e−θx(12(α+1)(α+2)+θ4x4+2(3α+2)θ3x3+6(α+1)(α+2)θ2x2+12(α+1)(α+2)θx)(α+1)(α+2), |
and
C=e−θx(−6α2(θx(θx+2)+2)−6α(θx(θx(θx+3)+6)+6)−θx(θx(θx(θx+4)+12)+24)−24)12(α+1)(α+2). |
Based on Eq (18), the pdf of the minimum and maximum order statistics, respectively, are given by
f(1:m)(x)=θ321−2m31−mme−θmx(θ2x46+αθx3+α2x2)[−(−6α2(θx(θx+2)+2)−B)]m−1(α2+3α+2)m, |
where B={6α(θx(θx(θx+3)+6)+6)+θx(θx(θx(θx+4)+12)+24)+24}, and
f(m:m)(x)=θ3me−θx(θ2x46+αθx3+α2x2)(e−θxD12(α+1)(α+2)+1)m−12(α2+3α+2), |
where
D=−6α2(θx(θx+2)+2)−6α(θx(θx(θx+3)+6)+6)−θx(θx(θx(θx+4)+12)+24)−24 |
.
To measure the scatter in the population, the mean deviation about the median denoted by MD(x) can be used, where
MD(x)=∫∞0|x−M|f(x)dx=μ−2∫M0xf(x)dx, | (4.3) |
where M is the population median. The mean deviation about the median for the WGQL distribution is defined as:
MD=(6α2(θM(θM(θM+3)+6)+6)−6(3α(α+4)+10)eθM+6α(θM(θM(θM(θM+4)+12)+24)+24)+θM(θM(θM(θM(θM+5)+20)+60)+120)+120)6(α+1)(α+2)θe−θM. | (4.4) |
Let X∼fWGQLD(x,θ,α), then
E(1X)=(α+1)2θ2(α2+3α+2). |
Therefore, the harmonic mean of the WGQLD is given by
HM(θ,α)=2(α2+3α+2)(α+1)2θ. | (4.5) |
Table 2 presents some values of the harmonic mean of WGQLD for various values of the parameters θ and α.
α | HM(5,α) | α | HM(5,α) | α | HM(5,α) | θ | HM(θ,2) | θ | HM(θ,2) | θ | HM(θ,2) |
1 | 0.6000 | 16 | 0.4235 | 31 | 0.4125 | 1 | 2.6666 | 16 | 0.1666 | 31 | 0.0860 |
2 | 0.5333 | 17 | 0.4222 | 32 | 0.4121 | 2 | 1.3333 | 17 | 0.1568 | 32 | 0.0833 |
3 | 0.5000 | 18 | 0.4210 | 33 | 0.4117 | 3 | 0.8888 | 18 | 0.1481 | 33 | 0.0808 |
4 | 0.4800 | 19 | 0.4200 | 34 | 0.4114 | 4 | 0.6666 | 19 | 0.1403 | 34 | 0.0784 |
5 | 0.4666 | 20 | 0.4190 | 35 | 0.4111 | 5 | 0.5333 | 20 | 0.1333 | 35 | 0.0761 |
6 | 0.4571 | 21 | 0.4181 | 36 | 0.4108 | 6 | 0.4444 | 21 | 0.1269 | 36 | 0.0740 |
7 | 0.4500 | 22 | 0.4173 | 37 | 0.4105 | 7 | 0.3809 | 22 | 0.1212 | 37 | 0.0720 |
8 | 0.4444 | 23 | 0.4166 | 38 | 0.4102 | 8 | 0.3333 | 23 | 0.1159 | 38 | 0.0701 |
9 | 0.4400 | 24 | 0.4160 | 39 | 0.4100 | 9 | 0.2962 | 24 | 0.1111 | 39 | 0.0683 |
10 | 0.4363 | 25 | 0.4153 | 40 | 0.4097 | 10 | 0.2666 | 25 | 0.1066 | 40 | 0.0666 |
11 | 0.4333 | 26 | 0.4148 | 41 | 0.4095 | 11 | 0.2424 | 26 | 0.1025 | 41 | 0.0650 |
12 | 0.4307 | 27 | 0.4142 | 42 | 0.4093 | 12 | 0.2222 | 27 | 0.0987 | 42 | 0.0634 |
13 | 0.4285 | 28 | 0.4137 | 43 | 0.4090 | 13 | 0.2051 | 28 | 0.0952 | 43 | 0.0620 |
14 | 0.4266 | 29 | 0.4133 | 44 | 0.4088 | 14 | 0.1904 | 29 | 0.0919 | 44 | 0.0606 |
15 | 0.4250 | 30 | 0.4129 | 45 | 0.4086 | 15 | 0.1777 | 30 | 0.0888 | 45 | 0.0592 |
Based on Table 2, we can conclude that: the harmonic mean values are decreasing in α when θ=5 and are decreasing in θ when α=2. Also, in general the harmonic mean values when θ<α are larger than the case of θ>α.
The stochastic ordering is an important tool in finance and reliability theory to evaluate the comparative behaviour of the models or systems. Let the random variables X and Y having the probability density functions, cumulative distribution functions and survival functions f(x),f(y),F(x),F(y),¯F(x)=1−F(x), and ¯F(y)=1−F(y), respectively, then X≤Y in:
1. Mean residual life order denoted by X≤MRLOY, if mX(x)≤mY(x) for all x,
2. Hazard rate order denoted by X≤HROY, if ¯FX(x)/¯FY(x) is decreasing in x≥0,
3. Stochastic order denoted by X≤SOY, if ¯FX(x)≤≤¯FY(x) for all x,
4. Likelihood ratio order denoted by X≤LROY, if fx(x)fY(x) is decreasing in x≥0.
All these stochastic orders defined above are related to each other and [29] showed the following relation is hold.
X≤LROY⇒X≤HROY⇒X≤MRLOY.⇓X≤SoY. |
Theorem 3. : Let the random variables X and Y be independent follow the pdf fX(x,θ,α) and fY(x,μ,ω), respectively. If θ>μ and α>ω, then X≤LROY,X≤HROY,X≤MRLOY and X≤SOY.
Proof: Let X∼fX(x,θ,α), Y∼fY(x,μ,ω), then
fX(x,θ,α)fY(x,μ,ω)=θ3(θ2x46+αθx3+α2x2)e−θx2(α2+3α+2)μ3(μ2x46+ωμx3+ω2x2)e−μx2(ω2+3ω+2), |
and
log(fX(x,θ,α)fY(x,μ,ω))=log(θ3(θ2x46+αθx3+α2x2)e−θx2(α2+3α+2)μ3(μ2x46+ωμx3+ω2x2)e−μx2(ω2+3ω+2)),=log(θ3(ω2+3ω+2)μ3(α2+3α+2)(θ2x46+αθx3+α2x2μ2x46+ωμx3+ω2x2)exp((μ−θ)x)),=log(θ3(ω2+3ω+2)μ3(α2+3α+2))+log(θ2x46+αθx3+α2x2μ2x46+ωμx3+ω2x2)+(μ−θ)x. |
Taking the derivative of the last equation with respect to x yields
ddxlog(fX(x,θ,α)fY(x,μ,ω))=2θ2x+6αθθ2x2+6αθx+6α2−2μ2x+6μωμ2x2+6μωx+6ω2+μ−θ. |
Hence, if θ>μ, α>ω, then ddxlog(fX(x,θ,α)fY(x,μ,ω))<0. Therefore, X≤LROY, X≤HROY, X≤MRLOY and X≤SOY.
The corresponding reliability and hazard functions of the WGQLD distribution are given, respectively by:
RWGQLD(x;θ, α)=1−FWGQLD(x;θ, α)=(24+6α2[2+xθ(2+xθ)]+6α[6+xθ(6+xθ(3+xθ))]+xθ[24+xθ(12+xθ(4+xθ))])12(1+α)(2+α)e−θx, | (5.1) |
and
HWGQLD(x;θ,α)=fWGQLD(x;θ,α)1−FWGQLD(x;θ,α)=θ3(θ2x46+αθx3+α2x2)24+6α2[2+xθ(2+xθ)]+6α[6+xθ(6+xθ(3+xθ))]+xθ[24+xθ(12+xθ(4+xθ))]. | (5.2) |
Figure 5, reveals that the reliability of the WGQLD are decreasing while the hazard rate function is increasing for α=1,2,…,5. The reversed hazard rate and odds functions for the WGQLD distribution, respectively, are defined as
RHWGQLD(x;θ,α)=fWGQLD(x;θ,α)FWGQLD(x;θ,α)=θ3x2(θ2x2+6αθx+6α2)12(α+1)(α+2)eθx−12(α+1)(α+2)−θx(θx(θx(θx+6α+4)+6(α+1)(α+2))+12(α+1)(α+2)), |
and
OWGQLD(x;θ,α)=FWGQLD(x;θ,α)1−FWGQLD(x;θ,α)=12(α+1)(α+2)eθxθ4x+4θ3x3+12θ2x2+24θx+6αθ3x3+18αθ2x2+36αθx+36α+6α2θ2x2+12α2θx+12α2+24−1. |
As it is shown in Figure 6, the reversed hazard function is decreasing taking inverse J shape while the odds functions is increasing with J shape.
Assume that the random variable X is a non-negative with continuous and twice differentiable cumulative distribution function F(x). The Bonferroni curve of the random variable X is defined as
BC=1pμ(∫∞0xf(x)dx−∫∞qxf(x)dx)=1pμ(μ−∫∞qxf(x)dx), | (6.1) |
where q=F−1(p) and p∈(0,1]. The Lorenz curve is defined as
LC=1μ(∫∞0xf(x)dx−∫∞qxf(x)dx)=1μ(μ−∫∞qxf(x)dx). | (6.2) |
The Gini index is given by
GI=1−1μ∫∞0(1−F(x))2dx=1μ∫∞0F(x)(1−F(x))dx. | (6.3) |
Now, for the WGQL distribution, the Bonferroni curve, Lorenz curve and Gini index are given, respectively, as
BC=(12eqθ(10+3α(4+α))−120−6α2(6+qθ[6+qθ(3+qθ)])−6α(24+qθ(24+qθ[12+qθ(4+qθ)]))−qθ(120+qθ[60+qθ(20+qθ(5+qθ))]))12p(10+3α(4+α))e−qθ, | (6.4) |
LC=(−120+12eqθ(10+3α(4+α))−6α2(6+qθ(6+qθ(3+qθ)))−6α(24+qθ(24+qθ(12+qθ(4+qθ))))−qθ(120+qθ(60+qθ(20+qθ(5+qθ)))))12(10+3α(4+α))e−qθ, | (6.5) |
GI=5(63+α[189+4α(49+3α(7+α))])64(1+α)(2+α)[10+3α(4+α)]. | (6.6) |
Table 3 presents some values of the Gini Index for WGQLD for different values of α
α | GI(α) | α | GI(α) | α | GI(α) |
1 | 0.2833333 | 16 | 0.3115732 | 31 | 0.3122193 |
2 | 0.2956578 | 17 | 0.3116666 | 32 | 0.3122354 |
3 | 0.3014769 | 18 | 0.3117464 | 33 | 0.3122502 |
4 | 0.3047121 | 19 | 0.3118154 | 34 | 0.3122637 |
5 | 0.3067016 | 20 | 0.3118753 | 35 | 0.3122762 |
6 | 0.3080137 | 21 | 0.3119276 | 36 | 0.3122877 |
7 | 0.3089251 | 22 | 0.3119736 | 37 | 0.3122984 |
8 | 0.3095841 | 23 | 0.3120143 | 38 | 0.3123082 |
9 | 0.3100762 | 24 | 0.3120505 | 39 | 0.3123174 |
10 | 0.3104533 | 25 | 0.3120828 | 40 | 0.3123259 |
11 | 0.3107487 | 26 | 0.3121117 | 41 | 0.3123339 |
12 | 0.3109844 | 27 | 0.3121377 | 42 | 0.3123413 |
13 | 0.3111755 | 28 | 0.3121611 | 43 | 0.3123482 |
14 | 0.3113326 | 29 | 0.3121824 | 44 | 0.3123547 |
15 | 0.3114633 | 30 | 0.3122017 | 45 | 0.3123607 |
Table 3 explains that the Gini index values for the WGQL distribution are increasing as α values are increasing, and on the average its value is about 0.31.
In this section, we consider six methods of estimation for estimating the unknowns parameters α and θ of the WGQLD distribution. These methods include the (1) maximum likelihood (ML) method, (2) method of maximum product of spacings, (3) ordinary least square method, (4) weight least square method, (5) method of Cramer-Von-Mises, and (6) method of Anderson-Darling.
First of all, we investigate the ML estimates (MLEs) of θ and α. Let X1,X2…,Xn be a random sample of size n selected from the WGQLD. The likelihood function is given by:
L(x;θ, α)=n∏i=1f(xi, θ, α)=(θ32(α2+3α+2))nn∏i=1 (α2x2i+αθx3i+θ2x4i6)e−θxi, | (7.1) |
and the log-likelihood function Ξ=lnL (x;θ, α) is:
Ξ=nln(θ32(α2+3α+2))+n∑i=1ln(α2x2i+αθx3i+θ2x4i6)−θn∑i=1xi. | (7.2) |
The derivatives of Ξ with respect to θ and α are:
dΞdθ=3nθ+n∑i=12xi(xiθ+3α)x2iθ2+6αxiθ+6α2−n∑i=1xi, | (7.3) |
dΞdα=−n(2α+3)α2+3α+2+n∑i=112α+6θxi6α2+6θxiα+θ2x2i. | (7.4) |
Since there is no closed form for these equations, then the MLEs ˆθ and ˆα of θ and α, respectively, can be solved simultaneously using a numerical method as Newton Raphson method.
The maximum product of spacing (MPS) method is proposed by [11,12] as an alternative to the maximum likelihood method. The MPS method requires a maximization of the geometric mean of the spacings in the data with respect to the parameters. Consider a random sample of size n, X1,X2,…,Xn from WGQLD distribution, then uniform spacings is given as:
Υi(θ,α)=F(xi:n|θ,α)−F(xi−1:n|θ,α),i=1,…,n, |
where F(x0:n|θ,α)=0 and F(xn+1:n|θ,α)=1. Clearly ∑n+1i=1Υi(θ,α)=1.
The MPSs, ˆαMPS and ˆθMPS, are the values of α and θ, which maximize the geometric mean of the spacing:
Z(θ,α|x)=[n+1∏i=1Υi(θ,α)]1n+1. | (7.5) |
The natural logarithm of (7.5) is:
H(θ,α|x)=1n+1n+1∑i=1logΥi(θ,α). |
The MPSs estimators ˆαMPS and ˆθMPS of the parameters α and θ, respectively, can also be obtained by solving the nonlinear equations:
∂∂θH(θ,α)=1n+1n+1∑i=11Υi(θ,α)[Δ1(xi:n|θ,α)−Δ1(xi−1:n|θ,α)]=0, |
∂∂αH(θ,α)=1n+1n+1∑i=11Υi(θ,α)[Δ2(xi:n|θ,α)−Δ2(xi−1:n|θ,α)]=0, |
where
Δ1(xi:n|θ,α)=∂∂θF(xi:n|θ,α)=θ3x3i:ne−θxi:n(6α2+(2θxi:n+8)α+3θxi:n)12(α+1)2(α+2)2, | (7.6) |
Δ2(xi:n|θ,α)=∂∂αF(xi:n|θ,α)=x3i:nθ2(x2i:nθ2+6αxi:nθ+6α2)e−xi:nθ12(α+1)(α+2), | (7.7) |
which can be obtained numerically.
The least square methods are introduced by [28] to estimate the parameters of beta distribution. Let Xi:n be the ith order statistic of the random sample X1,X2,…,Xn with distribution function F(x), then a main result in probability theory indicates that F(Xi:n)∼Beta(i,n−i+1). Moreover, we have
E[F(Xi:n)]=in+1 and Var[F(Xi:n)]=i(n−i+1)(n+1)2(n+2).
Using the expectations and variances, we obtain two variants of the least squares methods.
For the WGQLD distribution parameters estimation, the ordinary least square estimators ˆθOLS and ˆαOLS of the parameters θ and α, respectively can be obtained by minimizing the function:
Ω(θ,α|x)=n∑i=1[F(xi:n|θ,α)−in+1]2=n∑i=1[1−(24+6α2[2+xi:nθ(2+xi:nθ)]+6α[6+xi:nθ(6+xi:nθ(3+xi:nθ))]+xθ[24+xi:nθ(12+xi:nθ(4+xi:nθ))])12(1+α)(2+α)e−θxi:n−in+1]2, |
with respect to θ and α. Alternatively, these estimates can also be obtained by solving the following nonlinear equations:
n∑i=1[F(xi:n|θ,α)−in+1]Δ1(xi:n|θ,α)=0, |
n∑i=1[F(xi:n|θ,α)−in+1]Δ2(xi:n|θ,α)=0, |
where Δ1(xi:n|θ,α) and Δ2(xi:n|θ,α) are defined as in 7.6 and 7.7, respectively.
For the WGQLD distribution, the weighted least square estimators of θ and α say, ˆθWLS and ˆαWLS, respectively can be obtained by minimizing the function:
W(θ,α|x)=n∑i=1(n+1)2(n+2)i(n−i+1)[F(xi:n|θ,α)−in+1]2=n∑i=1(n+1)2(n+2)i(n−i+1)[1−(24+6α2[2+xi:nθ(2+xi:nθ)]+6α[6+xi:nθ(6+xi:nθ(3+xi:nθ))]+xθ[24+xi:nθ(12+xi:nθ(4+xi:nθ))])12(1+α)(2+α)e−θxi:n−in+1]2, |
with respect to θ and α. Equivalently, these estimators are the solution of the following nonlinear equations:
n∑i=1(n+1)2(n+2)i(n−i+1)[F(xi:n|θ,α)−in+1]Δ1(xi:n|θ,α)=0, |
n∑i=1(n+1)2(n+2)i(n−i+1)[F(xi:n|θ,α)−in+1]Δ2(xi:n|θ,α)=0, |
where Δ1(xi:n|θ,α) and Δ2(xi:n|θ,α) are specified as in 7.6 and 7.7, respectively.
Here, we use two popular methods based on the minimization of test statistics between the theoretical and empirical cumulative distribution functions. The methods are Cramer-von-Mises method and the method of Anderson-Darling (for more details see [13] and [24]).
The Cramer-von-Mises estimators (CVEs) ˆθ and ˆα of θ and α respectively, are obtained by minimizing the following function:
CV(θ,α)=112n+n∑i=1(n+1)2(n+2)i(n−i+1)[F(x(i:n);θ,α)−2i−12n]2=112n+n∑i=1(n+1)2(n+2)i(n−i+1)[1−(24+6α2[2+xi:nθ(2+xi:nθ)]+6α[6+xi:nθ(6+xi:nθ(3+xi:nθ))]+xθ[24+xi:nθ(12+xi:nθ(4+xi:nθ))])12(1+α)(2+α)e−θxi:n−2i−12n]2, |
with respect to θ and α. Equivalently, these estimators are the solution of the following nonlinear equations:
n∑i=1(n+1)2(n+2)i(n−i+1)[F(xi:n|θ,α)−2i−12n]Δ1(xi:n|θ,α)=0, |
n∑i=1(n+1)2(n+2)i(n−i+1)[F(xi:n|θ,α)−2i−12n]Δ2(xi:n|θ,α)=0, |
where Δ1(xi:n|θ,α) and Δ2(xi:n|θ,α) are given in 7.6 and 7.7, respectively.
The Anderson-Darling (AD) estimates of the WGQLD distribution parameters θ and α denoted by ˆθAD and ˆαAD can be obtained by minimizing the following function
A(α, θ)=−n−1nn∑i=1(2i−1){log F(xi:n|α, θ)+log¯F(xn−i+1:n|α, θ)}, |
with respect to θ and α, or by solving the following two equations
∂A(α,λ)∂λ=n∑i=1(2i−1){Δ1(xi:n|α,λ)F(xi:n|α,λ)−Δ1(xn−i+1:n|α,λ)¯F(xn−i+1:n|α,λ)}=0, |
and
∂A(α,λ)∂α=n∑i=1(2i−1){Δ2(xi:n|α,λ)F(xi:n|α,λ)−Δ2(xn−i+1:n|α,λ)¯F(xn−i+1:n|α,λ)}=0, |
where Δ1(xi:n|θ,α) and Δ2(xi:n|θ,α) are specified in 7.6 and 7.7, respectively.
This section compares the performances of the proposed estimators of the WGQLD parameters α and θ. This comparison is carried out by taking random samples of different sizes (n=20, 40, 60, 80,100 and 200) with various pairs of parameters values (θ,α) = (0.25, 1), (0.5, 1.5), (0.75, 2), (1, 1), (0.3, 2), (0.2, 3), (0.8, 1), (1, 3). The estimators are compared in terms of there mean square errors (MSE) and the estimated (Es) values of the parameters. The results are summarized in the Tables 4–7.
Parameters | MLEs | MPS | OLS | WLS | CVEs | AD | ||||||
Es | MSE | Es | MSE | Es | MSE | Es | MSE | Es | MSE | Es | MSE | |
α=0.25 | 0.426 | 0.3075 | 0.377 | 0.2919 | 0.486 | 0.4311 | 0.472 | 0.3890 | 0.456 | 0.3717 | 0.463 | 0.3680 |
θ=1 | 1.010 | 0.0118 | 1.026 | 0.0129 | 1.006 | 0.0134 | 1.005 | 0.0127 | 1.008 | 0.0127 | 1.005 | 0.0121 |
α=0.5 | 0.781 | 1.0029 | 0.718 | 1.0197 | 0.835 | 1.1394 | 0.822 | 1.0732 | 0.804 | 1.0553 | 0.811 | 1.0250 |
θ=1.5 | 1.523 | 0.0288 | 1.550 | 0.0325 | 1.516 | 0.0335 | 1.515 | 0.0319 | 1.521 | 0.0321 | 1.515 | 0.0304 |
α=0.75 | 1.133 | 1.8082 | 1.075 | 1.9053 | 1.196 | 2.0488 | 1.177 | 1.9076 | 1.157 | 1.8866 | 1.167 | 1.8744 |
θ=2 | 2.031 | 0.0562 | 2.068 | 0.0626 | 2.018 | 0.0639 | 2.017 | 0.0604 | 2.025 | 0.0607 | 2.017 | 0.0583 |
α=1 | 1.485 | 2.5509 | 1.429 | 2.6727 | 1.552 | 2.7457 | 1.530 | 2.6005 | 1.511 | 2.5964 | 1.514 | 2.528 |
θ=1 | 1.016 | 0.0140 | 1.036 | 0.0159 | 1.012 | 0.0165 | 1.010 | 0.0156 | 1.015 | 0.0156 | 1.010 | 0.0148 |
α=0.3 | 0.496 | 0.4830 | 0.441 | 0.4607 | 0.546 | 0.5756 | 0.534 | 0.5275 | 0.518 | 0.5111 | 0.527 | 0.5156 |
θ=2 | 2.021 | 0.0487 | 2.053 | 0.0538 | 2.010 | 0.0551 | 2.008 | 0.0527 | 2.015 | 0.0528 | 2.009 | 0.0509 |
α=0.2 | 0.412 | 0.3789 | 0.368 | 0.3773 | 0.464 | 0.5029 | 0.455 | 0.4562 | 0.440 | 0.4340 | 0.445 | 0.4371 |
θ=3 | 3.046 | 0.1080 | 3.094 | 0.1213 | 3.034 | 0.1251 | 3.033 | 0.1202 | 3.044 | 0.1202 | 3.033 | 0.1134 |
α=0.8 | 1.202 | 2.0044 | 1.148 | 2.1251 | 1.266 | 2.2173 | 1.248 | 2.0845 | 1.228 | 2.0673 | 1.237 | 2.0439 |
θ=1 | 1.014 | 0.0141 | 1.034 | 0.0161 | 1.009 | 0.0166 | 1.009 | 0.0157 | 1.013 | 0.0157 | 1.009 | 0.0151 |
α=1 | 1.480 | 2.6725 | 1.429 | 2.8627 | 1.546 | 2.9849 | 1.519 | 2.8085 | 1.502 | 2.7973 | 1.511 | 2.7333 |
θ=3 | 3.043 | 0.1308 | 3.103 | 0.1497 | 3.027 | 0.154 | 3.023 | 0.1462 | 3.036 | 0.1464 | 3.024 | 0.1392 |
Parameters | MLEs | MPS | OLS | WLS | CVEs | AD | ||||||
Es | MSE | Es | MSE | Es | MSE | Es | MSE | Es | MSE | Es | MSE | |
α=0.25 | 0.315 | 0.0771 | 0.283 | 0.0674 | 0.335 | 0.0949 | 0.331 | 0.0878 | 0.323 | 0.0849 | 0.329 | 0.0868 |
θ=1 | 1.001 | 0.0047 | 1.010 | 0.0049 | 0.999 | 0.0053 | 0.999 | 0.0050 | 1.001 | 0.0050 | 0.999 | 0.0049 |
α=0.5 | 0.576 | 0.1816 | 0.534 | 0.1741 | 0.604 | 0.2142 | 0.596 | 0.1981 | 0.586 | 0.1943 | 0.595 | 0.1965 |
θ=1.5 | 1.505 | 0.0113 | 1.520 | 0.0120 | 1.504 | 0.0130 | 1.503 | 0.0123 | 1.506 | 0.0123 | 1.503 | 0.0121 |
α=0.75 | 0.875 | 0.4170 | 0.829 | 0.4199 | 0.902 | 0.4771 | 0.894 | 0.4451 | 0.884 | 0.4409 | 0.892 | 0.4370 |
θ=2 | 2.012 | 0.0217 | 2.033 | 0.0231 | 2.007 | 0.0256 | 2.007 | 0.0238 | 2.011 | 0.0239 | 2.007 | 0.0234 |
α=1 | 1.145 | 0.5971 | 1.101 | 0.6059 | 1.174 | 0.6490 | 1.163 | 0.6149 | 1.153 | 0.6132 | 1.162 | 0.6064 |
θ=1 | 1.004 | 0.0051 | 1.014 | 0.0054 | 1.002 | 0.0058 | 1.002 | 0.0055 | 1.004 | 0.0055 | 1.002 | 0.0054 |
α=0.3 | 0.363 | 0.0849 | 0.328 | 0.0754 | 0.389 | 0.1047 | 0.383 | 0.0972 | 0.375 | 0.0941 | 0.381 | 0.0957 |
θ=2 | 2.007 | 0.0180 | 2.025 | 0.0192 | 2.003 | 0.0211 | 2.003 | 0.0198 | 2.007 | 0.0198 | 2.003 | 0.0195 |
α=0.2 | 0.281 | 0.0654 | 0.252 | 0.0560 | 0.306 | 0.0862 | 0.300 | 0.0780 | 0.293 | 0.0748 | 0.298 | 0.0777 |
θ=3 | 3.015 | 0.0415 | 3.040 | 0.0441 | 3.010 | 0.0479 | 3.010 | 0.0452 | 3.015 | 0.0453 | 3.009 | 0.0444 |
α=0.8 | 0.935 | 0.4789 | 0.891 | 0.4883 | 0.958 | 0.5383 | 0.953 | 0.5067 | 0.943 | 0.5026 | 0.950 | 0.5011 |
θ=1 | 1.005 | 0.0054 | 1.016 | 0.0058 | 1.003 | 0.0062 | 1.003 | 0.0059 | 1.005 | 0.0059 | 1.003 | 0.0058 |
α=1 | 1.157 | 0.7315 | 1.115 | 0.7467 | 1.187 | 0.8219 | 1.174 | 0.7597 | 1.164 | 0.7562 | 1.175 | 0.7566 |
θ=3 | 3.010 | 0.0528 | 3.042 | 0.0561 | 3.004 | 0.0615 | 3.003 | 0.0577 | 3.009 | 0.0578 | 3.003 | 0.0570 |
Parameters | MLEs | MPS | OLS | WLS | CVEs | AD | ||||||
Es | MSE | Es | MSE | Es | MSE | Es | MSE | Es | MSE | Es | MSE | |
α=0.25 | 0.287 | 0.0343 | 0.265 | 0.0312 | 0.3042 | 0.0433 | 0.298 | 0.0393 | 0.294 | 0.0383 | 0.299 | 0.0395 |
θ=1 | 1.003 | 0.0023 | 1.008 | 0.0024 | 1.003 | 0.0026 | 1.002 | 0.0025 | 1.003 | 0.0025 | 1.002 | 0.0024 |
α=0.5 | 0.540 | 0.0819 | 0.511 | 0.0797 | 0.551 | 0.0967 | 0.547 | 0.0892 | 0.542 | 0.0882 | 0.548 | 0.0892 |
θ=1.5 | 1.504 | 0.0057 | 1.513 | 0.0059 | 1.503 | 0.0066 | 1.503 | 0.0062 | 1.504 | 0.0062 | 1.503 | 0.0061 |
α=0.75 | 0.797 | 0.1301 | 0.768 | 0.1295 | 0.811 | 0.1509 | 0.806 | 0.1397 | 0.801 | 0.1387 | 0.807 | 0.1392 |
θ=2 | 2.006 | 0.0099 | 2.018 | 0.0104 | 2.003 | 0.0116 | 2.003 | 0.0107 | 2.005 | 0.0107 | 2.003 | 0.0106 |
α=1 | 1.096 | 0.2412 | 1.069 | 0.2411 | 1.107 | 0.2800 | 1.104 | 0.2593 | 1.098 | 0.2578 | 1.104 | 0.2577 |
θ=1 | 1.005 | 0.0028 | 1.011 | 0.0029 | 1.003 | 0.0033 | 1.004 | 0.0030 | 1.005 | 0.0031 | 1.003 | 0.0030 |
α=0.3 | 0.326 | 0.0369 | 0.302 | 0.0344 | 0.336 | 0.0442 | 0.333 | 0.0412 | 0.329 | 0.0404 | 0.334 | 0.0411 |
θ=2 | 2.002 | 0.0092 | 2.012 | 0.0094 | 1.999 | 0.0102 | 1.999 | 0.0096 | 2.001 | 0.0096 | 1.999 | 0.0096 |
α=0.2 | 0.240 | 0.0262 | 0.220 | 0.0228 | 0.252 | 0.0320 | 0.248 | 0.0297 | 0.244 | 0.0288 | 0.249 | 0.0296 |
θ=3 | 3.009 | 0.0194 | 3.024 | 0.0201 | 3.005 | 0.0220 | 3.005 | 0.0207 | 3.008 | 0.0207 | 3.005 | 0.0205 |
α=0.8 | 0.846 | 0.1625 | 0.818 | 0.1621 | 0.854 | 0.1858 | 0.852 | 0.1748 | 0.847 | 0.1739 | 0.852 | 0.1725 |
θ=1 | 1.000 | 0.0027 | 1.007 | 0.0028 | 0.999 | 0.0032 | 0.999 | 0.0030 | 1.000 | 0.0030 | 0.999 | 0.0029 |
α=1 | 1.071 | 0.2538 | 1.044 | 0.2564 | 1.087 | 0.2868 | 1.080 | 0.2664 | 1.074 | 0.2651 | 1.081 | 0.2669 |
θ=3 | 3.006 | 0.0248 | 3.025 | 0.0258 | 3.003 | 0.0298 | 3.002 | 0.0274 | 3.006 | 0.0274 | 3.002 | 0.0273 |
Parameters | MLEs | MPS | OLS | WLS | CVEs | AD | ||||||
Es | MSE | Es | MSE | Es | MSE | Es | MSE | Es | MSE | Es | MSE | |
α=0.25 | 0.263 | 0.0162 | 0.249 | 0.0154 | 0.271 | 0.0204 | 0.268 | 0.0185 | 0.266 | 0.0183 | 0.269 | 0.0185 |
θ=1 | 1.000 | 0.0011 | 1.003 | 0.0011 | 1.000 | 0.0013 | 1.000 | 0.0012 | 1.001 | 0.0012 | 1.000 | 0.0012 |
α=0.5 | 0.512 | 0.0364 | 0.495 | 0.0362 | 0.519 | 0.0430 | 0.517 | 0.0397 | 0.514 | 0.0395 | 0.517 | 0.0397 |
θ=1.5 | 1.501 | 0.0028 | 1.506 | 0.0028 | 1.500 | 0.0032 | 1.500 | 0.0030 | 1.501 | 0.0030 | 1.500 | 0.0030 |
α=0.75 | 0.762 | 0.0563 | 0.745 | 0.0564 | 0.770 | 0.0637 | 0.767 | 0.0594 | 0.764 | 0.0592 | 0.767 | 0.0592 |
θ=2 | 2.000 | 0.0048 | 2.007 | 0.0049 | 1.999 | 0.0054 | 1.999 | 0.00511 | 2.000 | 0.0051 | 1.998 | 0.0050 |
α=1 | 1.026 | 0.0976 | 1.011 | 0.0979 | 1.034 | 0.1101 | 1.031 | 0.1031 | 1.028 | 0.1028 | 1.031 | 0.1033 |
θ=1 | 1.000 | 0.0013 | 1.004 | 0.0013 | 0.999 | 0.0015 | 1.000 | 0.0014 | 1.000 | 0.0014 | 1.000 | 0.0014 |
α=0.3 | 0.307 | 0.0191 | 0.291 | 0.0186 | 0.315 | 0.0229 | 0.313 | 0.0212 | 0.310 | 0.0210 | 0.313 | 0.0212 |
θ=2 | 2.000 | 0.0046 | 2.007 | 0.0047 | 1.999 | 0.0051 | 1.999 | 0.0048 | 2.000 | 0.0048 | 1.999 | 0.0048 |
α=0.2 | 0.215 | 0.0125 | 0.202 | 0.0114 | 0.222 | 0.0149 | 0.220 | 0.0138 | 0.217 | 0.0136 | 0.220 | 0.0138 |
θ=3 | 2.999 | 0.0100 | 3.008 | 0.0102 | 2.998 | 0.0113 | 2.998 | 0.0107 | 2.999 | 0.0107 | 2.998 | 0.0106 |
α=0.8 | 0.837 | 0.0749 | 0.821 | 0.0744 | 0.842 | 0.0846 | 0.841 | 0.0793 | 0.838 | 0.0790 | 0.842 | 0.0792 |
θ=1 | 1.002 | 0.0013 | 1.006 | 0.0014 | 1.001 | 0.0015 | 1.002 | 0.0014 | 1.002 | 0.0014 | 1.002 | 0.0014 |
α=1 | 1.030 | 0.0935 | 1.015 | 0.0935 | 1.040 | 0.1044 | 1.036 | 0.0978 | 1.033 | 0.0975 | 1.036 | 0.0976 |
θ=3 | 3.003 | 0.0116 | 3.014 | 0.0119 | 3.002 | 0.0133 | 3.002 | 0.0125 | 3.004 | 0.0125 | 3.001 | 0.0124 |
Based on Tables 4–7 it is clear that:
● The MSEs values are decreasing as the sample sizes values are increasing for all cases considered in this section. As an example, for α=1,θ=3, with n=50 based on the AD, the MSEs are 0.7566 and 0.0570 compared to 0.2669 and 0.0273 for n=100, respectively.
● The bias values of the suggested estimators are decreasing as the sample sizes are increasing, and approaches zero for all cases for large n. For illustration, for α=0.3,θ=2, with n=100 the Es values are 0.302 and 2.012 using the MPS as compared to 0.291 and 2.007 for n=200, respectively.
● It can be observed that for most of the cases, the MLEs method has the smallest values of the MSEs among all methods of estimation.
In this section, we use data sets to illustrate the usefulness of the WGQL model, where four different data sets are used related to the environment, engineering and two medical data sets are considered. We compare the WGQL distribution to some well known distributions of two parameters as the generalized Quasi Lindley distribution, Quasi Lindley distribution, two-parameter Sujatha distribution, and the Pareto distribution.
The first data set is taken from [22] represents the 100 annual maximum precipitation (inches) for one rain gauge in Fort Collins, Colorado, from 1900 through 1999. The data are given below:
Data Set 1: 239,232,434, 85,302,174,170,121,193,168,148,116,132,132,144,183,223, 96,298, 97,116,146, 84,230,138,170,117,115,132,125,156,124,189,193, 71,176,105, 93,354, 60,151,160,219,142,117, 87,223,215,108,354,213,306,169,184, 71, 98, 96,218,176,121,161,321,102,269, 98,271, 95,212,151,136,240,162, 71,110,285,215,103,443,185,199,115,134,297,187,203,146, 94,129,162,112,348, 95,249,103,181,152,135,463,183,241 5.
The second data set from [25] consists of 100 observations on breaking stress of carbon fibers (in Gba). The data are as follows:
Data Set 2: 3.7, 2.74, 2.73, 2.5, 3.6, 3.11, 3.27, 2.87, 1.47, 3.11, 4.42, 2.41, 3.19, 3.22, 1.69, 3.28, 3.09, 1.87, 3.15, 4.9, 3.75, 2.43, 2.95, 2.97, 3.39, 2.96, 2.53, 2.67, 2.93, 3.22, 3.39, 2.81, 4.2, 3.33, 2.55, 3.31, 3.31, 2.85, 2.56, 3.56, 3.15, 2.35, 2.55, 2.59, 2.38, 2.81, 2.77, 2.17, 2.83, 1.92, 1.41, 3.68, 2.97, 1.36, 0.98, 2.76, 4.91, 3.68, 1.84, 1.59, 3.19, 1.57, 0.81, 5.56, 1.73, 1.59, 2, 1.22, 1.12, 1.71, 2.17, 1.17, 5.08, 2.48, 1.18, 3.51, 2.17, 1.69, 1.25, 4.38, 1.84, 0.39, 3.68, 2.48, 0.85, 1.61, 2.79, 4.7, 2.03, 1.8, 1.57, 1.08, 2.03, 1.61, 2.12, 1.89, 2.88, 2.82, 2.05, 3.655.
Due to the importance of the studies about the Covid-19 in the last years, we considered two sets of Covid-19 related to Algeria and Saudi Arabia in various times. The third data set is the Covid-19 data for the daily new cases in Algeria from 12 August 2020 to 09 November 2020 and it is available on the following electronic address https://sehhty.com/dz-covid/. It is given as follows
Data Set 3: 642,670,581,631,642,548,405,302,330,291,319,306,320,287,276,263,250,273,276,252,213,214,199,205,221,193,185,174,153,132,136,146,138,121,129,134,141,148,157,160,162,155,146,153,160,175,179,186,191,197,203,210,219,228,232,238,242,247,255,264,272,278,285,289,293,298,304,311,325,339,348,365,378,387,397,391,370,398,392,401,409,411,403,419,442,450,469,477,488,495.
The box and TTT plots for the above data are given in Figures 7 and 8, respectively.
The fourth data set is calling the Covid-19 data which present the daily new cases in Saudi Arabia from 24 March 2020 to 24 April 2020 and it is given by
Data Set 4: 1, 1, 1, 0, 1, 4, 0, 2, 6, 5, 4, 4, 5, 4, 3, 0, 3, 3, 5, 7, 6, 8, 6, 4, 4, 5, 5, 6, 6, 5, 7, 6. The descriptive statistics for the data is given in Table 8.
n | Min | Max | Mean | Median | St derivation | Kurtosis | Skweness | |
Data Set 1: | 100 | 60.000 | 463.000 | 175.670 | 158.000 | 83.166 | 1.713 | 1.316 |
Data Set 2: | 100 | 0.390 | 5.560 | 2.621 | 2.700 | 1.013 | 0.043 | 0.362 |
Data Set 3: | 90 | 121.000 | 670.000 | 294.322 | 274.500 | 131.618 | 0.369 | 0.927 |
Data Set 4: | 32 | 0 | 8 | 3.97 | 4 | 2.24 | -0.98 | -3.35 |
The WGQLD distribution is fitted to these two real data sets and compared with the following models:
● The generalized Quasi Lindley distribution: f(x)=θ2(θ2x36+αθx2+α2x)e−θx(α+1)2.
● Quasi Lindley distribution: f(x)=θ(α+xθ)α+1e−θx.
● The Pareto distribution: f(x)=αθαxα+1.
● Two-parameter Sujatha distribution: f(x)=θ3(x2+αx+1)e−θxθ2+αθ+2.
To choose the best model fitting, we considered Akaike information criterion (AIC) introduced by [1], Baysian information criterion (BIC) proposed by [30], Hannan Quinn Information Criterion (HQIC) suggested by [21], Consistent Akaike Information Criterion (CAIC) by [10], Kolmogorov-Smirnov (KS), where AIC = −2L+k,, CAIC = −2L+22knn−k−1, HQIC = 2loglog(n)[k−2L], BIC = −2L+klog(n), KS = sup|Fn(x)−F(x)|,Fn(x)=1n∑niłxi≤x, where k is the number of parameters and n is the sample size and L is the value of maximum log-likelihood function.
Based on the results reported in Tables 9, 10, 11 and 12, we observe that the WGQLD provides the better fit with the smallest values of AIC, AICc, BIC, HQIC and K-S with maximum P-values as compared to its competitive models considered in this study. Figures 9, 10, 11 and 12 support this claim.
Model | AIC | CAIC | BIC | HQIC | K-S | p-value |
WGQLD | 1142.145 | 1142.268 | 1147.355 | 1144.253 | 0.059902 | 0.865573 |
GQLD | 1145.844 | 1145.968 | 1151.055 | 1147.953 | 0.095349 | 0.323229 |
QLD | 1180.179 | 1180.303 | 1185.389 | 1182.288 | 0.216170 | 0.000174 |
PD | 1237.721 | 1237.845 | 1242.932 | 1239.83 | 0.340971 | 1.59e-10 |
TSPD | 1156.301 | 1156.425 | 1161.511 | 1158.41 | 0.143704 | 0.032159 |
Model | AIC | AICc | BIC | HQIC | K-S | p-value |
WGQLD | 295.1091 | 295.2328 | 300.3194 | 297.2178 | 0.105898 | 0.212049 |
GQLD | 306.1634 | 306.2871 | 311.3737 | 308.2721 | 0.123234 | 0.095915 |
QLD | 346.108 | 346.2317 | 351.3183 | 348.2167 | 0.223871 | 8.86e-05 |
PD | 396.7418 | 396.8655 | 401.9522 | 398.8505 | 0.320272 | 2.46-09 |
TSPD | 350.3233 | 350.447 | 355.5336 | 352.432 | 0.220935 | 0.000115 |
Model | AIC | AICc | BIC | HQIC | K-S | p-value |
WGQLD | 1118.42 | 1118.558 | 1123.420 | 1120.436 | 0.0606885 | 0.8946827 |
GQLD | 1122.41 | 1122.548 | 1127.410 | 1124.426 | 0.0900125 | 0.4593636 |
QLD | 1154.697 | 1154.835 | 1159.696 | 1156.713 | 0.2081433 | 0.0008209 |
PD | 1207.242 | 1207.38 | 1212.241 | 1209.258 | 0.3435296 | 1.190e-09 |
TSPD | 1132.559 | 1132.697 | 1137.559 | 1134.575 | 0.1352032 | 0.0744752 |
Model | AIC | AICc | BIC | HQIC | K-S | p-value |
WGQLD | 161.0522 | 161.4966 | 163.8546 | 161.9487 | 0.3077698 | 0.006804395 |
GQLD | 166.9975 | 167.4419 | 169.7999 | 167.894 | 0.3318577 | 0.00269967 |
In this article, we proposed the WGQLD distribution along with some of its properties such as, stochastic ordering, Median deviation, Harmonic mean, some plots of the pdf and cdf, Bonferroni and Lorenz curves and Gini index moments, coefficient of variation, coefficient of skewness and coefficient of kurtosis. Also, the hazard rate function, reliability function, reversed hazard rate and odds functions are presented. The maximum likelihood estimates is computed as well as the maximum product of spacing's, ordinary least squares, weighted least squares, Cramer-von-Mises, and Anderson-Darling methods are obtained. The results show that the best method of estimation is the MLE method. Applications of various real data sets are analyzed for illustration. It is proved that the WGQLD is empirically better than other competitors models considered in this study including the base GQLD. Therefore, in the future, the authors intend to investigate the performance of different estimators of the WGQLD based on ranked set sampling method and its modifications, see [3,4,19,20,32].
The author declare that they have no conflict of interest
The authors extend their appreciation to the deputy ship for research and innovation, "Ministry of Education" in Saudi Arabia for funding this research work through project No. IFKSURG-1438-086.
[1] | Simon AK, Hollander GA, McMichael A (2015) Evolution of the immune system in humans from infancy to old age. P Roy Soc B-Biol Sci 282: 20143085. https://doi.org/10.1098/rspb.2014.3085 |
[2] |
Nikolich-Žugich J (2018) The twilight of immunity: emerging concepts in aging of the immune system. Nat Immunol 19: 10-19. https://doi.org/10.1038/s41590-017-0006-x ![]() |
[3] |
Weiskopf D, Weinberger B, Grubeck-Loebenstein B (2009) The aging of the immune system. Transplant Int 22: 1041-1050. https://doi.org/10.1111/j.1432-2277.2009.00927.x ![]() |
[4] |
Weyand CM, Goronzy JJ (2016) Aging of the immune system. Mechanisms and therapeutic targets. Ann Am Thorac Soc 13: S422-S428. https://doi.org/10.1513/AnnalsATS.201602-095AW ![]() |
[5] |
Vasto S, Caruso C (2004) Immunity & ageing: a new journal looking at ageing from an immunological point of view. Immun Ageing 1: 1-4. https://doi.org/10.1186/1742-4933-1-1 ![]() |
[6] |
Shaw AC, Joshi S, Greenwood H, et al. (2010) Aging of the innate immune system. Curr Opin Immunol 22: 507-513. https://doi.org/10.1016/j.coi.2010.05.003 ![]() |
[7] | Hossain Z, Reza AHMM, Qasem WA, et al. (2022) Development of the immune system in the human embryo. Pediatr Res 2022: 1-5. https://doi.org/10.1038/s41390-022-01940-0 |
[8] |
Márquez EJ, Chung CH, Marches R, et al. (2020) Sexual-dimorphism in human immune system aging. Nat Commun 11: 751. https://doi.org/10.1038/s41467-020-14396-9 ![]() |
[9] |
Rosenthal KS (2018) Immune monitoring of the body's borders. AIMS Allergy Immunol 2: 148-164. https://doi.org/10.3934/Allergy.2018.3.148 ![]() |
[10] |
Rosenthal KS (2017) Dealing with garbage is the immune system's main job. MOJ Immunol 5: 00174. https://doi.org/10.15406/moji.2017.05.00174 ![]() |
[11] |
Melville JM, Moss TJM (2013) The immune consequences of preterm birth. Front Neurosci 7: 79. https://doi.org/10.3389/fnins.2013.00079 ![]() |
[12] |
Herzenberg LA, Tung JW (2006) B cell lineages: documented at last!. Nat Immunol 7: 225-226. https://doi.org/10.1038/ni0306-225 ![]() |
[13] |
Hornef MW, Torow N (2020) ‘Layered immunity’ and the ‘neonatal window of opportunity’—timed succession of non-redundant phases to establish mucosal host–microbial homeostasis after birth. Immunology 159: 15-25. https://doi.org/10.1111/imm.13149 ![]() |
[14] |
Filias A, Theodorou GL, Mouzopoulou S, et al. (2011) Phagocytic ability of neutrophils and monocytes in neonates. BMC Pediatr 11: 29. https://doi.org/10.1186/1471-2431-11-29 ![]() |
[15] |
Hebel K, Weinert S, Kuropka B, et al. (2014) CD4+ T cells from human neonates and infants are poised spontaneously to run a nonclassical IL-4 program. J Immunol 192: 5160-5170. https://doi.org/10.4049/jimmunol.1302539 ![]() |
[16] |
Leeansyah E, Loh L, Nixon DF, et al. (2014) Acquisition of innate-like microbial reactivity in mucosal tissues during human fetal MAIT-cell development. Nat Commun 5: 3143. https://doi.org/10.1038/ncomms4143 ![]() |
[17] |
Silva-Santos B, Schamel WW, Fisch P, et al. (2012) γδ T-cell conference 2012: close encounters for the fifth time. Eur J Immunol 42: 3101-3105. https://doi.org/10.1002/eji.201270101 ![]() |
[18] |
Gibbons D, Fleming P, Virasami A, et al. (2014) Interleukin-8 (CXCL8) production is a signatory T cell effector function of human newborn infants. Nat Med 20: 1206-1210. https://doi.org/10.1038/nm.3670 ![]() |
[19] |
Gibbons DL, Haque SF, Silberzahn T, et al. (2009) Neonates harbor highly active γδ T cells with selective impairments in preterm infants. Eur J Immunol 39: 1794-1806. https://doi.org/10.1002/eji.200939222 ![]() |
[20] |
Pou C, Nkulikiyimfura D, Henckel E, et al. (2019) The repertoire of maternal anti-viral antibodies in human newborns. Nat Med 25: 591-596. https://doi.org/10.1038/s41591-019-0392-8 ![]() |
[21] |
Sarvas H, Seppälä I, Kurikka S, et al. (1993) Half-life of the maternal IgG1 allotype in infants. J Clin Immunol 13: 145-151. https://doi.org/10.1007/BF00919271 ![]() |
[22] |
Fouda GG, Martinez DR, Swamy GK, et al. (2018) The Impact of IgG transplacental transfer on early life immunity. Immunohorizons 2: 14-25. https://doi.org/10.4049/immunohorizons.1700057 ![]() |
[23] |
Katherine Z, Sanidad KZ, Amir M, et al. (2022) Maternal gut microbiome-induced IgG regulates neonatal gut microbiome and immunity. Sci Immunol 7: eabh3816. https://doi.org/10.1126/sciimmunol.abh3816 ![]() |
[24] |
Kalliomäki M, Ouwehand A, Arvilommi H, et al. (1999) Transforming growth factor-β in breast milk: a potential regulator of atopic disease at an early age. J Allergy Clin Immunol 104: 1251-1257. https://doi.org/10.1016/S0091-6749(99)70021-7 ![]() |
[25] |
Brodin P (2022) Immune-microbe interactions early in life: A determinant of health and disease long term. Science 376: 945-950. https://doi.org/10.1126/science.abk2189 ![]() |
[26] |
Olin A, Henckel E, Chen Y, et al. (2018) Stereotypic immune system development in newborn children. Cell 174: 1277-1292. https://doi.org/10.1016/j.cell.2018.06.045 ![]() |
[27] |
Johnson JL, Jones MB, Cobb BA (2015) Polysaccharide A from the capsule of Bacteroides fragilis induces clonal CD4+ T cell expansion. J Biol Chem 290: 5007-5014. https://doi.org/10.1074/jbc.M114.621771 ![]() |
[28] |
Kelchtermans H, Billiau A, Matthys P (2008) How interferon-γ keeps autoimmune diseases in check. Trends Immunol 29: 479-486. https://doi.org/10.1016/j.it.2008.07.002 ![]() |
[29] |
Stiemsma LT, Reynolds LA, Turvey SE, et al. (2015) The hygiene hypothesis: current perspectives and future therapies. Immunotargets Ther 4: 143-157. https://doi.org/10.2147/ITT.S61528 ![]() |
[30] |
Okada H, Kuhn C, Feillet H, et al. (2010) The ‘hygiene hypothesis’ for autoimmune and allergic diseases: an update. Clin Exp Immunol 160: 1-9. https://doi.org/10.1111/j.1365-2249.2010.04139.x ![]() |
[31] |
Hill DA, Spergel JM (2018) The atopic march: Critical evidence and clinical relevance. Ann Allerg Asthma Im 120: 131-137. https://doi.org/10.1016/j.anai.2017.10.037 ![]() |
[32] |
Neu N, Duchon J, Zachariah P (2015) TORCH Infections. Clin Perinatol 42: 77-103. https://doi.org/10.1016/j.clp.2014.11.001 ![]() |
[33] |
Menzies D (2000) What does tuberculin reactivity after bacille Calmette–Guérin vaccination tell us?. Clin Infect Dis 31: S71-S74. https://doi.org/10.1086/314075 ![]() |
[34] |
Sherwood ER, Burelbach KR, McBride MA, et al. (2022) Innate immune memory and the host response to infection. J Immunol 208: 785-792. https://doi.org/10.4049/jimmunol.2101058 ![]() |
[35] |
Loske J, Röhmel J, Lukassen S, et al. (2022) Pre-activated antiviral innate immunity in the upper airways controls early SARS-CoV-2 infection in children. Nat Biotechnol 40: 319-324. https://doi.org/10.1038/s41587-021-01037-9 ![]() |
[36] |
Azzi T, Lünemann A, Murer A, et al. (2014) Role for early-differentiated natural killer cells in infectious mononucleosis. Blood 124: 2533-2543. https://doi.org/10.1182/blood-2014-01-553024 ![]() |
[37] |
Takahashi T, Iwasaki A (2021) Sex differences in immune responses. Science 371: 347-348. https://doi.org/10.1126/science.abe7199 ![]() |
[38] |
Klein SL, Flanagan KL (2016) Sex differences in immune responses. Nat Rev Immunol 16: 626-638. https://doi.org/10.1038/nri.2016.90 ![]() |
[39] |
Klein SL, Jedlicka A, Pekosz A (2010) The Xs and Y of immune responses to viral vaccines. Lancet Infect Dis 10: 338-349. https://doi.org/10.1016/S1473-3099(10)70049-9 ![]() |
[40] |
Pinheiro I, Dejager L, Libert C (2011) X-chromosome-located microRNAs in immunity: Might they explain male/female differences?. Bioessays 33: 791-802. https://doi.org/10.1002/bies.201100047 ![]() |
[41] |
Griesbeck M, Ziegler S, Laffont S, et al. (2015) Sex differences in plasmacytoid dendritic cell levels of IRF5 drive higher IFN-α production in women. J Immunol 195: 5327-5336. https://doi.org/10.4049/jimmunol.1501684 ![]() |
[42] |
Haitao T, Vermunt JV, Abeykoon J, et al. (2020) COVID-19 and sex differences: mechanisms and biomarkers. Mayo Clin Proc 95: 2189-2203. https://doi.org/10.1016/j.mayocp.2020.07.024 ![]() |
[43] |
Asano T, Boisson B, Onodi F, et al. (2021) X-linked recessive TLR7 deficiency in ~1% of men under 60 years old with life-threatening COVID-19. Sci Immunol 6: eabl4348. https://doi.org/10.1126/sciimmunol.abl4348 ![]() |
[44] |
Aomatsu M, Kato T, Kasahara E, et al. (2013) Gender difference in tumor necrosis factor-α production in human neutrophils stimulated by lipopolysaccharide and interferon-γ. Biochem Bioph Res Co 441: 220-225. https://doi.org/10.1016/j.bbrc.2013.10.042 ![]() |
[45] |
Brunsing RL, Owens KS, Prossnitz ER (2013) The G protein-coupled estrogen receptor (GPER) agonist G-1 expands the regulatory T-cell population under TH17-polarizing conditions. J Immunother 36: 190-196. https://doi.org/10.1097/CJI.0b013e31828d8e3b ![]() |
[46] |
Jaillon S, Berthenet K, Garlanda C (2019) Sexual dimorphism in innate immunity. Clin Rev Allerg Immu 56: 308-321. https://doi.org/10.1007/s12016-017-8648-x ![]() |
[47] |
Arruvito L, Sanz M, Banham AH, et al. (2007) Expansion of CD4+CD25+ and FOXP3+ regulatory T cells during the follicular phase of the menstrual cycle: implications for human reproduction. J Immunol 178: 2572-2578. https://doi.org/10.4049/jimmunol.178.4.2572 ![]() |
[48] |
Mor G, Aldo P, Alvero A (2017) The unique immunological and microbial aspects of pregnancy. Nat Rev Immunol 17: 469-482. https://doi.org/10.1038/nri.2017.64 ![]() |
[49] |
Krop J, Heidt S, Claas FHJ, et al. (2020) Regulatory T cells in pregnancy: it is not all about FoxP3. Front Immunol 11: 1182. https://doi.org/10.3389/fimmu.2020.01182 ![]() |
[50] |
Hanna J, Goldman-Wohl D, Hamani Y, et al. (2006) Decidual NK cells regulate key developmental processes at the human fetal-maternal interface. Nat Med 12: 1065-1074. https://doi.org/10.1038/nm1452 ![]() |
[51] |
Le Bouteiller P (2013) Human decidual NK cells: unique and tightly regulated effector functions in healthy and pathogen-infected pregnancies. Front Immunol 4: 404. https://doi.org/10.3389/fimmu.2013.00404 ![]() |
[52] |
Sojka DK, Yang L, Yokoyama WM (2019) Uterine natural killer cells. Front Immunol 10: 960. https://doi.org/10.3389/fimmu.2019.00960 ![]() |
[53] |
Yang SL, Wang HY, Li DJ, et al. (2019) Role of decidual natural killer cells at the maternal–fetal interface during pregnancy. Reprod Dev Med 3: 165-169. https://doi.org/10.4103/2096-2924.268161 ![]() |
[54] |
Piccinni MP, Lombardelli L, Logiodice F, et al. (2016) How pregnancy can affect autoimmune diseases progression?. Clin Mol Allergy 14: 11. https://doi.org/10.1186/s12948-016-0048-x ![]() |
[55] |
Bupp MRG, Jorgensen TN (2018) Androgen-induced immunosuppression. Front Immunol 9: 794. https://doi.org/10.3389/fimmu.2018.00794 ![]() |
[56] |
Trigunaite A, Dimo J, Jørgensen TN (2015) Suppressive effects of androgens on the immune system. Cellular Immunol 294: 87-94. https://doi.org/10.1016/j.cellimm.2015.02.004 ![]() |
[57] |
Angele MK, Pratschke S, Hubbard WJ, et al. (2014) Gender differences in sepsis. Virulence 5: 12-19. https://doi.org/10.4161/viru.26982 ![]() |
[58] |
Meier A, Chang J, Chan E, et al. (2009) Sex differences in the Toll-like receptor-mediated response of plasmacytoid dendritic cells to HIV-1. Nat Med 15: 955-959. https://doi.org/10.1038/nm.2004 ![]() |
[59] |
Meester I, Manilla-Muñoz E, León-Cachón, RBR, et al. (2020) SeXY chromosomes and the immune system: reflections after a comparative study. Biol Sex Differ 11: 1-13. https://doi.org/10.1186/s13293-019-0278-y ![]() |
[60] |
Russi AE, Walker-Caulfield ME, Ebel ME, et al. (2015) Cutting edge: c-Kit signaling differentially regulates type 2 innate lymphoid cell accumulation and susceptibility to central nervous system demyelination in male and female SJL mice. J Immunol 194: 5609-5613. https://doi.org/10.4049/jimmunol.1500068 ![]() |
[61] |
Zhang MA, Rego D, Moshkova M, et al. (2012) Peroxisome proliferator-activated receptor (PPAR)α and -γ regulate IFNγ and IL-17A production by human T cells in a sex-specific way. P Natl Acad Sci USA 109: 9505-9510. https://doi.org/10.1073/pnas.1118458109 ![]() |
[62] |
Tadount F, Doyon-Plourde P, Rafferty E, et al. (2020) Is there a difference in the immune response, efficacy, effectiveness and safety of seasonal influenza vaccine in males and females?—A systematic review. Vaccine 38: 444-459. https://doi.org/10.1016/j.vaccine.2019.10.091 ![]() |
[63] |
Gee J, Marquez P, Su J, et al. (2021) First month of COVID-19 vaccine safety monitoring—United States, December 14, 2020-January 13. MMWR Morb Mortal Wkly Rep 70: 283-288. https://doi.org/10.15585/mmwr.mm7008e3 ![]() |
[64] |
López-Otín C, Blasco MA, Partridge L, et al. (2013) The hallmarks of aging. Cell 153: 1194-1217. https://doi.org/10.1016/j.cell.2013.05.039 ![]() |
[65] |
Cuervo AM, Macian F (2014) Autophagy and the immune function in aging. Curr Opin Immunol 29: 97-104. https://doi.org/10.1016/j.coi.2014.05.006 ![]() |
[66] |
Gustafson CE, Kim C, Weyand CM, et al. (2020) Influence of immune aging on vaccine responses. J Allergy Clin Immunol 145: 1309-1321. https://doi.org/10.1016/j.jaci.2020.03.017 ![]() |
[67] |
Ciabattini A, Nardini C, Santoro F, et al. (2018) Vaccination in the elderly: The challenge of immune changes with aging. Semin Immunol 40: 83-94. https://doi.org/10.1016/j.smim.2018.10.010 ![]() |
[68] | Fülöp T, Foris G, Worum I, et al. (1985) Age-dependent alterations of Fcγ receptor-mediated effector functions of human polymorphonuclear leucocytes. Clin Exp Immunol 61: 425-432. |
[69] |
Butcher S, Chahel H, Lord JM (2000) Ageing and the neutrophil: No appetite for killing?. Immunology 100: 411-416. https://doi.org/10.1046/j.1365-2567.2000.00079.x ![]() |
[70] |
Solana R, Tarazona R, Gayoso I, et al. (2012) Innate immunosenescence: Effect of aging on cells and receptors of the innate immune system in humans. Semin Immunol 24: 331-341. https://doi.org/10.1016/j.smim.2012.04.008 ![]() |
[71] |
Mylotte JM, Naughton B, Saludades C, et al. (1998) Validation and application of the pneumonia prognosis index to nursing home residents with pneumonia. J Am Geriatr Soc 46: 1538-1544. https://doi.org/10.1111/j.1532-5415.1998.tb01539.x ![]() |
[72] |
Hasegawa T, Feng Z, Yan Z, et al. (2020) Reduction in human epidermal Langerhans cells with age is associated with decline in CXCL14-mediated recruitment of CD14+ monocytes. J Invest Dermatol 140: 1327-1334. https://doi.org/10.1016/j.jid.2019.11.017 ![]() |
[73] |
Zegarska B, Pietkun K, Giemza-Kucharska P, et al. (2017) Changes of Langerhans cells during skin ageing. Postepy Dermatol Alergol 34: 260-267. https://doi.org/10.5114/ada.2017.67849 ![]() |
[74] |
Salimi S, Hamlyn JM (2020) COVID-19 and crosstalk with the hallmarks of aging. J Gerontol A-Biol 75: e34-e41. https://doi.org/10.1093/gerona/glaa149 ![]() |
[75] |
Becklund B, Purton J, Ramsey C, et al. (2016) The aged lymphoid tissue environment fails to support naïve T cell homeostasis. Sci Rep 6: 30842. https://doi.org/10.1038/srep30842 ![]() |
[76] |
Thompson HL, Smithey MJ, Surh CD, et al. (2017) Functional and homeostatic impact of age-related changes in lymph node stroma. Front Immunol 8: 706. https://doi.org/10.3389/fimmu.2017.00706 ![]() |
[77] |
Naylor K, Li G, Vallejo AN, et al. (2005) The influence of age on T cell generation and TCR diversity. J Immunol 174: 7446-7452. https://doi.org/10.4049/jimmunol.174.11.7446 ![]() |
[78] |
Goronzy JJ, Lee WW, Weyand CM (2007) Aging and T-cell diversity. Exp Gerontol 42: 400-406. https://doi.org/10.1016/j.exger.2006.11.016 ![]() |
[79] |
Moro-García MA, Alonso-Arias R, López-Vázquez A, et al. (2012) Relationship between functional ability in older people, immune system status, and intensity of response to CMV. Age 34: 479-495. https://doi.org/10.1007/s11357-011-9240-6 ![]() |
[80] |
Pangrazzi L, Weinberger BZ (2020) T cells, aging and senescence. Exp Gerontol 134: 110887. https://doi.org/10.1016/j.exger.2020.110887 ![]() |
[81] |
Solana R, Tarazona R, Aiello AE, et al. (2012) CMV and Immunosenescence: from basics to clinics. Immun Ageing 9: 23. https://doi.org/10.1186/1742-4933-9-23 ![]() |
[82] |
Frasca D, Blomberg BB, Fuldner R, et al. (2018) “Aging and immunity” symposium: Meeting report. Exp Gerontol 105: 1-3. https://doi.org/10.1016/j.exger.2017.12.004 ![]() |
[83] |
Goronzy J, Weyand C (2013) Understanding immunosenescence to improve responses to vaccines. Nat Immunol 14: 428-436. https://doi.org/10.1038/ni.2588 ![]() |
[84] |
Feehan J, Tripodi N, Apostolopoulos V (2021) The twilight of the immune system: The impact of immunosenescence in aging. Maturitas 147: 7-13. https://doi.org/10.1016/j.maturitas.2021.02.006 ![]() |
[85] |
Raynor J, Lages CS, Shehata H, et al. (2012) Homeostasis and function of regulatory T cells in aging. Curr Opin Immunol 24: 482-487. https://doi.org/10.1016/j.coi.2012.04.005 ![]() |
[86] |
Neiman M, Hellström C, Just D, et al. (2019) Individual and stable autoantibody repertoires in healthy individuals. Autoimmunity 52: 1-11. https://doi.org/10.1080/08916934.2019.1581774 ![]() |
[87] |
Khan MWA, Al Otaibi A, Sherwani S, et al. (2020) Glycation and oxidative stress increase autoantibodies in the elderly. Molecules 25: 3675. https://doi.org/10.3390/molecules25163675 ![]() |
[88] |
Barcenas-Morales G, Cortes-Acevedo P, Doffinger R (2019) Anticytokine autoantibodies leading to infection: early recognition, diagnosis and treatment options. Curr Opin Infect Dis 32: 330-336. https://doi.org/10.1097/QCO.0000000000000561 ![]() |
[89] |
Merkel PA, Lebo T, Knight V (2019) Functional analysis of anti-cytokine autoantibodies using flow cytometry. Front Immunol 10: 1517. https://doi.org/10.3389/fimmu.2019.01517 ![]() |
[90] |
Bastard P, Gervais A, Le Voyeret T, et al. (2021) Autoantibodies neutralizing type I IFNs are present in ~4% of uninfected individuals over 70 years old and account for ~20% of COVID-19 deaths. Sci Immunol 6: eabl4340. https://doi.org/10.1126/sciimmunol.abl4340 ![]() |
[91] |
Franceschi C, Salvioli S, Garagnani P, et al. (2017) Immunobiography and the heterogeneity of immune responses in the elderly: a focus on inflammaging and trained immunity. Front Immunol 8: 982. https://doi.org/10.3389/fimmu.2017.00982 ![]() |
[92] | Fulop T, Larbi A, Pawelec G, et al. (2021) Immunology of aging: the birth of inflammaging. Clin Rev Allerg Immu 2021: 1-14. https://doi.org/10.1007/s12016-021-08899-6 |
[93] |
Cai D, Liu T (2012) Inflammatory cause of metabolic syndrome via brain stress and NF-κB. Aging 4: 98-115. https://doi.org/10.18632/aging.100431 ![]() |
[94] |
Reed RG (2019) Stress and immunological aging. Curr Opin Behav Sci 28: 38-43. https://doi.org/10.1016/j.cobeha.2019.01.012 ![]() |
[95] |
Klopack ET, Crimmins EM, Cole SW, et al. (2022) Social stressors associated with age-related T lymphocyte percentages in older US adults: Evidence from the US Health and Retirement Study. P Natl Acad Sci USA 119: e2202780119. https://doi.org/10.1073/pnas.2202780119 ![]() |
[96] |
Gouin JP, Hantsoo L, Kiecolt-Glaser JK (2008) Immune dysregulation and chronic stress among older adults: A review. Neuroimmunomodulat 15: 251-259. https://doi.org/10.1159/000156468 ![]() |
[97] |
Minciullo PL, Catalano A, Mandraffino G, et al. (2015) Inflammaging and anti-inflammaging: the role of cytokines in extreme longevity. Arch Immunol Ther Ex 64: 111-126. https://doi.org/10.1007/s00005-015-0377-3 ![]() |
[98] |
Tajbakhsh A, Farahani N, Gheibihayat SM, et al. (2021) Autoantigen-specific immune tolerance in pathological and physiological cell death: Nanotechnology comes into view. Int Immunopharmacol 90: 107177. https://doi.org/10.1016/j.intimp.2020.107177 ![]() |
[99] |
Giefing-Kröll C, Berger P, Lepperdinger G, et al. (2015) How sex and age affect immune responses, susceptibility to infections, and response to vaccination. Aging Cell 14: 309-321. https://doi.org/10.1111/acel.12326 ![]() |
[100] |
Kauffman CA, Yoshikawa TT (2001) Fungal infections in older adults. Clin Infect Dis 33: 550-555. https://doi.org/10.1086/322685 ![]() |
[101] |
Shea KM, Kammerer JS, Winston CA, et al. (2014) Estimated rate of reactivation of latent tuberculosis infection in the United States, overall and by population subgroup. Am J Epidemiol 179: 216-225. https://doi.org/10.1093/aje/kwt246 ![]() |
[102] |
Nikolich-Zugich J, Knox KS, Rios CT, et al. (2020) SARS-CoV-2 and COVID-19 in older adults: what we may expect regarding pathogenesis, immune responses, and outcomes. Geroscience 42: 505-514. https://doi.org/10.1007/s11357-020-00186-0 ![]() |
[103] |
Boraschi D, Italiani P (2014) Immunosenescence and vaccine failure in the elderly: Strategies for improving response. Immunol Lett 162: 346-353. https://doi.org/10.1016/j.imlet.2014.06.006 ![]() |
[104] |
Kogut I, Scholz JL, Cancro MP, et al. (2012) B cell maintenance and function in aging. Semin Immunol 24: 342-349. https://doi.org/10.1016/j.smim.2012.04.004 ![]() |
[105] |
Franceschi C, Capri M, Monti D, et al. (2007) Inflammaging and anti-inflammaging: A systemic perspective on aging and longevity emerged from studies in humans. Mech Ageing Dev 128: 92-105. https://doi.org/10.1016/j.mad.2006.11.016 ![]() |
1. | Saurabh L. Raikar, Dr. Rajesh S. Prabhu Gaonkar, Jaya algorithm in estimation of P[X > Y] for two parameter Weibull distribution, 2021, 7, 2473-6988, 2820, 10.3934/math.2022156 | |
2. | Lishamol Tomy, Veena G, Christophe Chesneau, Applications of the Sine Modified Lindley Distribution to Biomedical Data, 2022, 27, 2297-8747, 43, 10.3390/mca27030043 | |
3. | SidAhmed Benchiha, Amer Ibrahim Al-Omari, Ghadah Alomani, Goodness-of-Fit Tests for Weighted Generalized Quasi-Lindley Distribution Using SRS and RSS with Applications to Real Data, 2022, 11, 2075-1680, 490, 10.3390/axioms11100490 | |
4. | Amer Ibrahim Al-Omari, Rehab Alsultan, Ghadah Alomani, Asymmetric Right-Skewed Size-Biased Bilal Distribution with Mathematical Properties, Reliability Analysis, Inference and Applications, 2023, 15, 2073-8994, 1578, 10.3390/sym15081578 | |
5. | SidAhmed Benchiha, Laxmi Prasad Sapkota, Aned Al Mutairi, Vijay Kumar, Rana H. Khashab, Ahmed M. Gemeay, Mohammed Elgarhy, Said G. Nassr, A New Sine Family of Generalized Distributions: Statistical Inference with Applications, 2023, 28, 2297-8747, 83, 10.3390/mca28040083 |
μWGQLD | σWGQLD | SKWGQLD | KuWGQLD | CVWGQLD | |
α | θ=1.25 | ||||
0.1 | 3.889177 | 1.786969 | 0.897106 | 4.205054 | 0.459472 |
0.2 | 3.793939 | 1.782395 | 0.903213 | 4.217285 | 0.469801 |
0.3 | 3.711037 | 1.776240 | 0.910968 | 4.233681 | 0.478637 |
0.4 | 3.638095 | 1.769174 | 0.919440 | 4.252427 | 0.486291 |
0.5 | 3.573333 | 1.761615 | 0.928126 | 4.272400 | 0.492989 |
0.6 | 3.515385 | 1.753829 | 0.936749 | 4.292896 | 0.498901 |
0.7 | 3.463181 | 1.745987 | 0.945154 | 4.313464 | 0.504157 |
0.8 | 3.415873 | 1.738199 | 0.953260 | 4.333820 | 0.508859 |
0.9 | 3.372777 | 1.730537 | 0.961027 | 4.353780 | 0.513090 |
1 | 3.333333 | 1.723046 | 0.968437 | 4.373230 | 0.516914 |
1.1 | 3.297081 | 1.715755 | 0.975492 | 4.392101 | 0.520386 |
1.2 | 3.263636 | 1.708680 | 0.982196 | 4.410356 | 0.523551 |
1.3 | 3.232675 | 1.701828 | 0.988563 | 4.427977 | 0.526446 |
1.4 | 3.203922 | 1.695202 | 0.994608 | 4.444961 | 0.529102 |
1.5 | 3.177143 | 1.688801 | 1.000346 | 4.461313 | 0.531547 |
θ | α=2 | ||||
0.1 | 38.333333 | 20.749833 | 1.025012 | 4.534152 | 0.5413 |
0.2 | 19.166667 | 10.374916 | 1.025012 | 4.534152 | 0.5413 |
0.3 | 12.777778 | 6.916611 | 1.025012 | 4.534152 | 0.5413 |
0.4 | 9.583333 | 5.187458 | 1.025012 | 4.534152 | 0.5413 |
0.5 | 7.666667 | 4.149967 | 1.025012 | 4.534152 | 0.5413 |
0.6 | 6.388889 | 3.458305 | 1.025012 | 4.534152 | 0.5413 |
0.7 | 5.476190 | 2.964262 | 1.025012 | 4.534152 | 0.5413 |
0.8 | 4.791667 | 2.593729 | 1.025012 | 4.534152 | 0.5413 |
0.9 | 4.259259 | 2.305537 | 1.025012 | 4.534152 | 0.5413 |
1 | 3.833333 | 2.074983 | 1.025012 | 4.534152 | 0.5413 |
1.1 | 3.484848 | 1.886348 | 1.025012 | 4.534152 | 0.5413 |
1.2 | 3.194444 | 1.729153 | 1.025012 | 4.534152 | 0.5413 |
1.3 | 2.948718 | 1.596141 | 1.025012 | 4.534152 | 0.5413 |
1.4 | 2.738095 | 1.482131 | 1.025012 | 4.534152 | 0.5413 |
1.5 | 2.555556 | 1.383322 | 1.025012 | 4.534152 | 0.5413 |
α | HM(5,α) | α | HM(5,α) | α | HM(5,α) | θ | HM(θ,2) | θ | HM(θ,2) | θ | HM(θ,2) |
1 | 0.6000 | 16 | 0.4235 | 31 | 0.4125 | 1 | 2.6666 | 16 | 0.1666 | 31 | 0.0860 |
2 | 0.5333 | 17 | 0.4222 | 32 | 0.4121 | 2 | 1.3333 | 17 | 0.1568 | 32 | 0.0833 |
3 | 0.5000 | 18 | 0.4210 | 33 | 0.4117 | 3 | 0.8888 | 18 | 0.1481 | 33 | 0.0808 |
4 | 0.4800 | 19 | 0.4200 | 34 | 0.4114 | 4 | 0.6666 | 19 | 0.1403 | 34 | 0.0784 |
5 | 0.4666 | 20 | 0.4190 | 35 | 0.4111 | 5 | 0.5333 | 20 | 0.1333 | 35 | 0.0761 |
6 | 0.4571 | 21 | 0.4181 | 36 | 0.4108 | 6 | 0.4444 | 21 | 0.1269 | 36 | 0.0740 |
7 | 0.4500 | 22 | 0.4173 | 37 | 0.4105 | 7 | 0.3809 | 22 | 0.1212 | 37 | 0.0720 |
8 | 0.4444 | 23 | 0.4166 | 38 | 0.4102 | 8 | 0.3333 | 23 | 0.1159 | 38 | 0.0701 |
9 | 0.4400 | 24 | 0.4160 | 39 | 0.4100 | 9 | 0.2962 | 24 | 0.1111 | 39 | 0.0683 |
10 | 0.4363 | 25 | 0.4153 | 40 | 0.4097 | 10 | 0.2666 | 25 | 0.1066 | 40 | 0.0666 |
11 | 0.4333 | 26 | 0.4148 | 41 | 0.4095 | 11 | 0.2424 | 26 | 0.1025 | 41 | 0.0650 |
12 | 0.4307 | 27 | 0.4142 | 42 | 0.4093 | 12 | 0.2222 | 27 | 0.0987 | 42 | 0.0634 |
13 | 0.4285 | 28 | 0.4137 | 43 | 0.4090 | 13 | 0.2051 | 28 | 0.0952 | 43 | 0.0620 |
14 | 0.4266 | 29 | 0.4133 | 44 | 0.4088 | 14 | 0.1904 | 29 | 0.0919 | 44 | 0.0606 |
15 | 0.4250 | 30 | 0.4129 | 45 | 0.4086 | 15 | 0.1777 | 30 | 0.0888 | 45 | 0.0592 |
α | GI(α) | α | GI(α) | α | GI(α) |
1 | 0.2833333 | 16 | 0.3115732 | 31 | 0.3122193 |
2 | 0.2956578 | 17 | 0.3116666 | 32 | 0.3122354 |
3 | 0.3014769 | 18 | 0.3117464 | 33 | 0.3122502 |
4 | 0.3047121 | 19 | 0.3118154 | 34 | 0.3122637 |
5 | 0.3067016 | 20 | 0.3118753 | 35 | 0.3122762 |
6 | 0.3080137 | 21 | 0.3119276 | 36 | 0.3122877 |
7 | 0.3089251 | 22 | 0.3119736 | 37 | 0.3122984 |
8 | 0.3095841 | 23 | 0.3120143 | 38 | 0.3123082 |
9 | 0.3100762 | 24 | 0.3120505 | 39 | 0.3123174 |
10 | 0.3104533 | 25 | 0.3120828 | 40 | 0.3123259 |
11 | 0.3107487 | 26 | 0.3121117 | 41 | 0.3123339 |
12 | 0.3109844 | 27 | 0.3121377 | 42 | 0.3123413 |
13 | 0.3111755 | 28 | 0.3121611 | 43 | 0.3123482 |
14 | 0.3113326 | 29 | 0.3121824 | 44 | 0.3123547 |
15 | 0.3114633 | 30 | 0.3122017 | 45 | 0.3123607 |
Parameters | MLEs | MPS | OLS | WLS | CVEs | AD | ||||||
Es | MSE | Es | MSE | Es | MSE | Es | MSE | Es | MSE | Es | MSE | |
α=0.25 | 0.426 | 0.3075 | 0.377 | 0.2919 | 0.486 | 0.4311 | 0.472 | 0.3890 | 0.456 | 0.3717 | 0.463 | 0.3680 |
θ=1 | 1.010 | 0.0118 | 1.026 | 0.0129 | 1.006 | 0.0134 | 1.005 | 0.0127 | 1.008 | 0.0127 | 1.005 | 0.0121 |
α=0.5 | 0.781 | 1.0029 | 0.718 | 1.0197 | 0.835 | 1.1394 | 0.822 | 1.0732 | 0.804 | 1.0553 | 0.811 | 1.0250 |
θ=1.5 | 1.523 | 0.0288 | 1.550 | 0.0325 | 1.516 | 0.0335 | 1.515 | 0.0319 | 1.521 | 0.0321 | 1.515 | 0.0304 |
α=0.75 | 1.133 | 1.8082 | 1.075 | 1.9053 | 1.196 | 2.0488 | 1.177 | 1.9076 | 1.157 | 1.8866 | 1.167 | 1.8744 |
θ=2 | 2.031 | 0.0562 | 2.068 | 0.0626 | 2.018 | 0.0639 | 2.017 | 0.0604 | 2.025 | 0.0607 | 2.017 | 0.0583 |
α=1 | 1.485 | 2.5509 | 1.429 | 2.6727 | 1.552 | 2.7457 | 1.530 | 2.6005 | 1.511 | 2.5964 | 1.514 | 2.528 |
θ=1 | 1.016 | 0.0140 | 1.036 | 0.0159 | 1.012 | 0.0165 | 1.010 | 0.0156 | 1.015 | 0.0156 | 1.010 | 0.0148 |
α=0.3 | 0.496 | 0.4830 | 0.441 | 0.4607 | 0.546 | 0.5756 | 0.534 | 0.5275 | 0.518 | 0.5111 | 0.527 | 0.5156 |
θ=2 | 2.021 | 0.0487 | 2.053 | 0.0538 | 2.010 | 0.0551 | 2.008 | 0.0527 | 2.015 | 0.0528 | 2.009 | 0.0509 |
α=0.2 | 0.412 | 0.3789 | 0.368 | 0.3773 | 0.464 | 0.5029 | 0.455 | 0.4562 | 0.440 | 0.4340 | 0.445 | 0.4371 |
θ=3 | 3.046 | 0.1080 | 3.094 | 0.1213 | 3.034 | 0.1251 | 3.033 | 0.1202 | 3.044 | 0.1202 | 3.033 | 0.1134 |
α=0.8 | 1.202 | 2.0044 | 1.148 | 2.1251 | 1.266 | 2.2173 | 1.248 | 2.0845 | 1.228 | 2.0673 | 1.237 | 2.0439 |
θ=1 | 1.014 | 0.0141 | 1.034 | 0.0161 | 1.009 | 0.0166 | 1.009 | 0.0157 | 1.013 | 0.0157 | 1.009 | 0.0151 |
α=1 | 1.480 | 2.6725 | 1.429 | 2.8627 | 1.546 | 2.9849 | 1.519 | 2.8085 | 1.502 | 2.7973 | 1.511 | 2.7333 |
θ=3 | 3.043 | 0.1308 | 3.103 | 0.1497 | 3.027 | 0.154 | 3.023 | 0.1462 | 3.036 | 0.1464 | 3.024 | 0.1392 |
Parameters | MLEs | MPS | OLS | WLS | CVEs | AD | ||||||
Es | MSE | Es | MSE | Es | MSE | Es | MSE | Es | MSE | Es | MSE | |
α=0.25 | 0.315 | 0.0771 | 0.283 | 0.0674 | 0.335 | 0.0949 | 0.331 | 0.0878 | 0.323 | 0.0849 | 0.329 | 0.0868 |
θ=1 | 1.001 | 0.0047 | 1.010 | 0.0049 | 0.999 | 0.0053 | 0.999 | 0.0050 | 1.001 | 0.0050 | 0.999 | 0.0049 |
α=0.5 | 0.576 | 0.1816 | 0.534 | 0.1741 | 0.604 | 0.2142 | 0.596 | 0.1981 | 0.586 | 0.1943 | 0.595 | 0.1965 |
θ=1.5 | 1.505 | 0.0113 | 1.520 | 0.0120 | 1.504 | 0.0130 | 1.503 | 0.0123 | 1.506 | 0.0123 | 1.503 | 0.0121 |
α=0.75 | 0.875 | 0.4170 | 0.829 | 0.4199 | 0.902 | 0.4771 | 0.894 | 0.4451 | 0.884 | 0.4409 | 0.892 | 0.4370 |
θ=2 | 2.012 | 0.0217 | 2.033 | 0.0231 | 2.007 | 0.0256 | 2.007 | 0.0238 | 2.011 | 0.0239 | 2.007 | 0.0234 |
α=1 | 1.145 | 0.5971 | 1.101 | 0.6059 | 1.174 | 0.6490 | 1.163 | 0.6149 | 1.153 | 0.6132 | 1.162 | 0.6064 |
θ=1 | 1.004 | 0.0051 | 1.014 | 0.0054 | 1.002 | 0.0058 | 1.002 | 0.0055 | 1.004 | 0.0055 | 1.002 | 0.0054 |
α=0.3 | 0.363 | 0.0849 | 0.328 | 0.0754 | 0.389 | 0.1047 | 0.383 | 0.0972 | 0.375 | 0.0941 | 0.381 | 0.0957 |
θ=2 | 2.007 | 0.0180 | 2.025 | 0.0192 | 2.003 | 0.0211 | 2.003 | 0.0198 | 2.007 | 0.0198 | 2.003 | 0.0195 |
α=0.2 | 0.281 | 0.0654 | 0.252 | 0.0560 | 0.306 | 0.0862 | 0.300 | 0.0780 | 0.293 | 0.0748 | 0.298 | 0.0777 |
θ=3 | 3.015 | 0.0415 | 3.040 | 0.0441 | 3.010 | 0.0479 | 3.010 | 0.0452 | 3.015 | 0.0453 | 3.009 | 0.0444 |
α=0.8 | 0.935 | 0.4789 | 0.891 | 0.4883 | 0.958 | 0.5383 | 0.953 | 0.5067 | 0.943 | 0.5026 | 0.950 | 0.5011 |
θ=1 | 1.005 | 0.0054 | 1.016 | 0.0058 | 1.003 | 0.0062 | 1.003 | 0.0059 | 1.005 | 0.0059 | 1.003 | 0.0058 |
α=1 | 1.157 | 0.7315 | 1.115 | 0.7467 | 1.187 | 0.8219 | 1.174 | 0.7597 | 1.164 | 0.7562 | 1.175 | 0.7566 |
θ=3 | 3.010 | 0.0528 | 3.042 | 0.0561 | 3.004 | 0.0615 | 3.003 | 0.0577 | 3.009 | 0.0578 | 3.003 | 0.0570 |
Parameters | MLEs | MPS | OLS | WLS | CVEs | AD | ||||||
Es | MSE | Es | MSE | Es | MSE | Es | MSE | Es | MSE | Es | MSE | |
α=0.25 | 0.287 | 0.0343 | 0.265 | 0.0312 | 0.3042 | 0.0433 | 0.298 | 0.0393 | 0.294 | 0.0383 | 0.299 | 0.0395 |
θ=1 | 1.003 | 0.0023 | 1.008 | 0.0024 | 1.003 | 0.0026 | 1.002 | 0.0025 | 1.003 | 0.0025 | 1.002 | 0.0024 |
α=0.5 | 0.540 | 0.0819 | 0.511 | 0.0797 | 0.551 | 0.0967 | 0.547 | 0.0892 | 0.542 | 0.0882 | 0.548 | 0.0892 |
θ=1.5 | 1.504 | 0.0057 | 1.513 | 0.0059 | 1.503 | 0.0066 | 1.503 | 0.0062 | 1.504 | 0.0062 | 1.503 | 0.0061 |
α=0.75 | 0.797 | 0.1301 | 0.768 | 0.1295 | 0.811 | 0.1509 | 0.806 | 0.1397 | 0.801 | 0.1387 | 0.807 | 0.1392 |
θ=2 | 2.006 | 0.0099 | 2.018 | 0.0104 | 2.003 | 0.0116 | 2.003 | 0.0107 | 2.005 | 0.0107 | 2.003 | 0.0106 |
α=1 | 1.096 | 0.2412 | 1.069 | 0.2411 | 1.107 | 0.2800 | 1.104 | 0.2593 | 1.098 | 0.2578 | 1.104 | 0.2577 |
θ=1 | 1.005 | 0.0028 | 1.011 | 0.0029 | 1.003 | 0.0033 | 1.004 | 0.0030 | 1.005 | 0.0031 | 1.003 | 0.0030 |
α=0.3 | 0.326 | 0.0369 | 0.302 | 0.0344 | 0.336 | 0.0442 | 0.333 | 0.0412 | 0.329 | 0.0404 | 0.334 | 0.0411 |
θ=2 | 2.002 | 0.0092 | 2.012 | 0.0094 | 1.999 | 0.0102 | 1.999 | 0.0096 | 2.001 | 0.0096 | 1.999 | 0.0096 |
α=0.2 | 0.240 | 0.0262 | 0.220 | 0.0228 | 0.252 | 0.0320 | 0.248 | 0.0297 | 0.244 | 0.0288 | 0.249 | 0.0296 |
θ=3 | 3.009 | 0.0194 | 3.024 | 0.0201 | 3.005 | 0.0220 | 3.005 | 0.0207 | 3.008 | 0.0207 | 3.005 | 0.0205 |
α=0.8 | 0.846 | 0.1625 | 0.818 | 0.1621 | 0.854 | 0.1858 | 0.852 | 0.1748 | 0.847 | 0.1739 | 0.852 | 0.1725 |
θ=1 | 1.000 | 0.0027 | 1.007 | 0.0028 | 0.999 | 0.0032 | 0.999 | 0.0030 | 1.000 | 0.0030 | 0.999 | 0.0029 |
α=1 | 1.071 | 0.2538 | 1.044 | 0.2564 | 1.087 | 0.2868 | 1.080 | 0.2664 | 1.074 | 0.2651 | 1.081 | 0.2669 |
θ=3 | 3.006 | 0.0248 | 3.025 | 0.0258 | 3.003 | 0.0298 | 3.002 | 0.0274 | 3.006 | 0.0274 | 3.002 | 0.0273 |
Parameters | MLEs | MPS | OLS | WLS | CVEs | AD | ||||||
Es | MSE | Es | MSE | Es | MSE | Es | MSE | Es | MSE | Es | MSE | |
α=0.25 | 0.263 | 0.0162 | 0.249 | 0.0154 | 0.271 | 0.0204 | 0.268 | 0.0185 | 0.266 | 0.0183 | 0.269 | 0.0185 |
θ=1 | 1.000 | 0.0011 | 1.003 | 0.0011 | 1.000 | 0.0013 | 1.000 | 0.0012 | 1.001 | 0.0012 | 1.000 | 0.0012 |
α=0.5 | 0.512 | 0.0364 | 0.495 | 0.0362 | 0.519 | 0.0430 | 0.517 | 0.0397 | 0.514 | 0.0395 | 0.517 | 0.0397 |
θ=1.5 | 1.501 | 0.0028 | 1.506 | 0.0028 | 1.500 | 0.0032 | 1.500 | 0.0030 | 1.501 | 0.0030 | 1.500 | 0.0030 |
α=0.75 | 0.762 | 0.0563 | 0.745 | 0.0564 | 0.770 | 0.0637 | 0.767 | 0.0594 | 0.764 | 0.0592 | 0.767 | 0.0592 |
θ=2 | 2.000 | 0.0048 | 2.007 | 0.0049 | 1.999 | 0.0054 | 1.999 | 0.00511 | 2.000 | 0.0051 | 1.998 | 0.0050 |
α=1 | 1.026 | 0.0976 | 1.011 | 0.0979 | 1.034 | 0.1101 | 1.031 | 0.1031 | 1.028 | 0.1028 | 1.031 | 0.1033 |
θ=1 | 1.000 | 0.0013 | 1.004 | 0.0013 | 0.999 | 0.0015 | 1.000 | 0.0014 | 1.000 | 0.0014 | 1.000 | 0.0014 |
α=0.3 | 0.307 | 0.0191 | 0.291 | 0.0186 | 0.315 | 0.0229 | 0.313 | 0.0212 | 0.310 | 0.0210 | 0.313 | 0.0212 |
θ=2 | 2.000 | 0.0046 | 2.007 | 0.0047 | 1.999 | 0.0051 | 1.999 | 0.0048 | 2.000 | 0.0048 | 1.999 | 0.0048 |
α=0.2 | 0.215 | 0.0125 | 0.202 | 0.0114 | 0.222 | 0.0149 | 0.220 | 0.0138 | 0.217 | 0.0136 | 0.220 | 0.0138 |
θ=3 | 2.999 | 0.0100 | 3.008 | 0.0102 | 2.998 | 0.0113 | 2.998 | 0.0107 | 2.999 | 0.0107 | 2.998 | 0.0106 |
α=0.8 | 0.837 | 0.0749 | 0.821 | 0.0744 | 0.842 | 0.0846 | 0.841 | 0.0793 | 0.838 | 0.0790 | 0.842 | 0.0792 |
θ=1 | 1.002 | 0.0013 | 1.006 | 0.0014 | 1.001 | 0.0015 | 1.002 | 0.0014 | 1.002 | 0.0014 | 1.002 | 0.0014 |
α=1 | 1.030 | 0.0935 | 1.015 | 0.0935 | 1.040 | 0.1044 | 1.036 | 0.0978 | 1.033 | 0.0975 | 1.036 | 0.0976 |
θ=3 | 3.003 | 0.0116 | 3.014 | 0.0119 | 3.002 | 0.0133 | 3.002 | 0.0125 | 3.004 | 0.0125 | 3.001 | 0.0124 |
n | Min | Max | Mean | Median | St derivation | Kurtosis | Skweness | |
Data Set 1: | 100 | 60.000 | 463.000 | 175.670 | 158.000 | 83.166 | 1.713 | 1.316 |
Data Set 2: | 100 | 0.390 | 5.560 | 2.621 | 2.700 | 1.013 | 0.043 | 0.362 |
Data Set 3: | 90 | 121.000 | 670.000 | 294.322 | 274.500 | 131.618 | 0.369 | 0.927 |
Data Set 4: | 32 | 0 | 8 | 3.97 | 4 | 2.24 | -0.98 | -3.35 |
Model | AIC | CAIC | BIC | HQIC | K-S | p-value |
WGQLD | 1142.145 | 1142.268 | 1147.355 | 1144.253 | 0.059902 | 0.865573 |
GQLD | 1145.844 | 1145.968 | 1151.055 | 1147.953 | 0.095349 | 0.323229 |
QLD | 1180.179 | 1180.303 | 1185.389 | 1182.288 | 0.216170 | 0.000174 |
PD | 1237.721 | 1237.845 | 1242.932 | 1239.83 | 0.340971 | 1.59e-10 |
TSPD | 1156.301 | 1156.425 | 1161.511 | 1158.41 | 0.143704 | 0.032159 |
Model | AIC | AICc | BIC | HQIC | K-S | p-value |
WGQLD | 295.1091 | 295.2328 | 300.3194 | 297.2178 | 0.105898 | 0.212049 |
GQLD | 306.1634 | 306.2871 | 311.3737 | 308.2721 | 0.123234 | 0.095915 |
QLD | 346.108 | 346.2317 | 351.3183 | 348.2167 | 0.223871 | 8.86e-05 |
PD | 396.7418 | 396.8655 | 401.9522 | 398.8505 | 0.320272 | 2.46-09 |
TSPD | 350.3233 | 350.447 | 355.5336 | 352.432 | 0.220935 | 0.000115 |
Model | AIC | AICc | BIC | HQIC | K-S | p-value |
WGQLD | 1118.42 | 1118.558 | 1123.420 | 1120.436 | 0.0606885 | 0.8946827 |
GQLD | 1122.41 | 1122.548 | 1127.410 | 1124.426 | 0.0900125 | 0.4593636 |
QLD | 1154.697 | 1154.835 | 1159.696 | 1156.713 | 0.2081433 | 0.0008209 |
PD | 1207.242 | 1207.38 | 1212.241 | 1209.258 | 0.3435296 | 1.190e-09 |
TSPD | 1132.559 | 1132.697 | 1137.559 | 1134.575 | 0.1352032 | 0.0744752 |
Model | AIC | AICc | BIC | HQIC | K-S | p-value |
WGQLD | 161.0522 | 161.4966 | 163.8546 | 161.9487 | 0.3077698 | 0.006804395 |
GQLD | 166.9975 | 167.4419 | 169.7999 | 167.894 | 0.3318577 | 0.00269967 |
μWGQLD | σWGQLD | SKWGQLD | KuWGQLD | CVWGQLD | |
α | θ=1.25 | ||||
0.1 | 3.889177 | 1.786969 | 0.897106 | 4.205054 | 0.459472 |
0.2 | 3.793939 | 1.782395 | 0.903213 | 4.217285 | 0.469801 |
0.3 | 3.711037 | 1.776240 | 0.910968 | 4.233681 | 0.478637 |
0.4 | 3.638095 | 1.769174 | 0.919440 | 4.252427 | 0.486291 |
0.5 | 3.573333 | 1.761615 | 0.928126 | 4.272400 | 0.492989 |
0.6 | 3.515385 | 1.753829 | 0.936749 | 4.292896 | 0.498901 |
0.7 | 3.463181 | 1.745987 | 0.945154 | 4.313464 | 0.504157 |
0.8 | 3.415873 | 1.738199 | 0.953260 | 4.333820 | 0.508859 |
0.9 | 3.372777 | 1.730537 | 0.961027 | 4.353780 | 0.513090 |
1 | 3.333333 | 1.723046 | 0.968437 | 4.373230 | 0.516914 |
1.1 | 3.297081 | 1.715755 | 0.975492 | 4.392101 | 0.520386 |
1.2 | 3.263636 | 1.708680 | 0.982196 | 4.410356 | 0.523551 |
1.3 | 3.232675 | 1.701828 | 0.988563 | 4.427977 | 0.526446 |
1.4 | 3.203922 | 1.695202 | 0.994608 | 4.444961 | 0.529102 |
1.5 | 3.177143 | 1.688801 | 1.000346 | 4.461313 | 0.531547 |
θ | α=2 | ||||
0.1 | 38.333333 | 20.749833 | 1.025012 | 4.534152 | 0.5413 |
0.2 | 19.166667 | 10.374916 | 1.025012 | 4.534152 | 0.5413 |
0.3 | 12.777778 | 6.916611 | 1.025012 | 4.534152 | 0.5413 |
0.4 | 9.583333 | 5.187458 | 1.025012 | 4.534152 | 0.5413 |
0.5 | 7.666667 | 4.149967 | 1.025012 | 4.534152 | 0.5413 |
0.6 | 6.388889 | 3.458305 | 1.025012 | 4.534152 | 0.5413 |
0.7 | 5.476190 | 2.964262 | 1.025012 | 4.534152 | 0.5413 |
0.8 | 4.791667 | 2.593729 | 1.025012 | 4.534152 | 0.5413 |
0.9 | 4.259259 | 2.305537 | 1.025012 | 4.534152 | 0.5413 |
1 | 3.833333 | 2.074983 | 1.025012 | 4.534152 | 0.5413 |
1.1 | 3.484848 | 1.886348 | 1.025012 | 4.534152 | 0.5413 |
1.2 | 3.194444 | 1.729153 | 1.025012 | 4.534152 | 0.5413 |
1.3 | 2.948718 | 1.596141 | 1.025012 | 4.534152 | 0.5413 |
1.4 | 2.738095 | 1.482131 | 1.025012 | 4.534152 | 0.5413 |
1.5 | 2.555556 | 1.383322 | 1.025012 | 4.534152 | 0.5413 |
α | HM(5,α) | α | HM(5,α) | α | HM(5,α) | θ | HM(θ,2) | θ | HM(θ,2) | θ | HM(θ,2) |
1 | 0.6000 | 16 | 0.4235 | 31 | 0.4125 | 1 | 2.6666 | 16 | 0.1666 | 31 | 0.0860 |
2 | 0.5333 | 17 | 0.4222 | 32 | 0.4121 | 2 | 1.3333 | 17 | 0.1568 | 32 | 0.0833 |
3 | 0.5000 | 18 | 0.4210 | 33 | 0.4117 | 3 | 0.8888 | 18 | 0.1481 | 33 | 0.0808 |
4 | 0.4800 | 19 | 0.4200 | 34 | 0.4114 | 4 | 0.6666 | 19 | 0.1403 | 34 | 0.0784 |
5 | 0.4666 | 20 | 0.4190 | 35 | 0.4111 | 5 | 0.5333 | 20 | 0.1333 | 35 | 0.0761 |
6 | 0.4571 | 21 | 0.4181 | 36 | 0.4108 | 6 | 0.4444 | 21 | 0.1269 | 36 | 0.0740 |
7 | 0.4500 | 22 | 0.4173 | 37 | 0.4105 | 7 | 0.3809 | 22 | 0.1212 | 37 | 0.0720 |
8 | 0.4444 | 23 | 0.4166 | 38 | 0.4102 | 8 | 0.3333 | 23 | 0.1159 | 38 | 0.0701 |
9 | 0.4400 | 24 | 0.4160 | 39 | 0.4100 | 9 | 0.2962 | 24 | 0.1111 | 39 | 0.0683 |
10 | 0.4363 | 25 | 0.4153 | 40 | 0.4097 | 10 | 0.2666 | 25 | 0.1066 | 40 | 0.0666 |
11 | 0.4333 | 26 | 0.4148 | 41 | 0.4095 | 11 | 0.2424 | 26 | 0.1025 | 41 | 0.0650 |
12 | 0.4307 | 27 | 0.4142 | 42 | 0.4093 | 12 | 0.2222 | 27 | 0.0987 | 42 | 0.0634 |
13 | 0.4285 | 28 | 0.4137 | 43 | 0.4090 | 13 | 0.2051 | 28 | 0.0952 | 43 | 0.0620 |
14 | 0.4266 | 29 | 0.4133 | 44 | 0.4088 | 14 | 0.1904 | 29 | 0.0919 | 44 | 0.0606 |
15 | 0.4250 | 30 | 0.4129 | 45 | 0.4086 | 15 | 0.1777 | 30 | 0.0888 | 45 | 0.0592 |
α | GI(α) | α | GI(α) | α | GI(α) |
1 | 0.2833333 | 16 | 0.3115732 | 31 | 0.3122193 |
2 | 0.2956578 | 17 | 0.3116666 | 32 | 0.3122354 |
3 | 0.3014769 | 18 | 0.3117464 | 33 | 0.3122502 |
4 | 0.3047121 | 19 | 0.3118154 | 34 | 0.3122637 |
5 | 0.3067016 | 20 | 0.3118753 | 35 | 0.3122762 |
6 | 0.3080137 | 21 | 0.3119276 | 36 | 0.3122877 |
7 | 0.3089251 | 22 | 0.3119736 | 37 | 0.3122984 |
8 | 0.3095841 | 23 | 0.3120143 | 38 | 0.3123082 |
9 | 0.3100762 | 24 | 0.3120505 | 39 | 0.3123174 |
10 | 0.3104533 | 25 | 0.3120828 | 40 | 0.3123259 |
11 | 0.3107487 | 26 | 0.3121117 | 41 | 0.3123339 |
12 | 0.3109844 | 27 | 0.3121377 | 42 | 0.3123413 |
13 | 0.3111755 | 28 | 0.3121611 | 43 | 0.3123482 |
14 | 0.3113326 | 29 | 0.3121824 | 44 | 0.3123547 |
15 | 0.3114633 | 30 | 0.3122017 | 45 | 0.3123607 |
Parameters | MLEs | MPS | OLS | WLS | CVEs | AD | ||||||
Es | MSE | Es | MSE | Es | MSE | Es | MSE | Es | MSE | Es | MSE | |
α=0.25 | 0.426 | 0.3075 | 0.377 | 0.2919 | 0.486 | 0.4311 | 0.472 | 0.3890 | 0.456 | 0.3717 | 0.463 | 0.3680 |
θ=1 | 1.010 | 0.0118 | 1.026 | 0.0129 | 1.006 | 0.0134 | 1.005 | 0.0127 | 1.008 | 0.0127 | 1.005 | 0.0121 |
α=0.5 | 0.781 | 1.0029 | 0.718 | 1.0197 | 0.835 | 1.1394 | 0.822 | 1.0732 | 0.804 | 1.0553 | 0.811 | 1.0250 |
θ=1.5 | 1.523 | 0.0288 | 1.550 | 0.0325 | 1.516 | 0.0335 | 1.515 | 0.0319 | 1.521 | 0.0321 | 1.515 | 0.0304 |
α=0.75 | 1.133 | 1.8082 | 1.075 | 1.9053 | 1.196 | 2.0488 | 1.177 | 1.9076 | 1.157 | 1.8866 | 1.167 | 1.8744 |
θ=2 | 2.031 | 0.0562 | 2.068 | 0.0626 | 2.018 | 0.0639 | 2.017 | 0.0604 | 2.025 | 0.0607 | 2.017 | 0.0583 |
α=1 | 1.485 | 2.5509 | 1.429 | 2.6727 | 1.552 | 2.7457 | 1.530 | 2.6005 | 1.511 | 2.5964 | 1.514 | 2.528 |
θ=1 | 1.016 | 0.0140 | 1.036 | 0.0159 | 1.012 | 0.0165 | 1.010 | 0.0156 | 1.015 | 0.0156 | 1.010 | 0.0148 |
α=0.3 | 0.496 | 0.4830 | 0.441 | 0.4607 | 0.546 | 0.5756 | 0.534 | 0.5275 | 0.518 | 0.5111 | 0.527 | 0.5156 |
θ=2 | 2.021 | 0.0487 | 2.053 | 0.0538 | 2.010 | 0.0551 | 2.008 | 0.0527 | 2.015 | 0.0528 | 2.009 | 0.0509 |
α=0.2 | 0.412 | 0.3789 | 0.368 | 0.3773 | 0.464 | 0.5029 | 0.455 | 0.4562 | 0.440 | 0.4340 | 0.445 | 0.4371 |
θ=3 | 3.046 | 0.1080 | 3.094 | 0.1213 | 3.034 | 0.1251 | 3.033 | 0.1202 | 3.044 | 0.1202 | 3.033 | 0.1134 |
α=0.8 | 1.202 | 2.0044 | 1.148 | 2.1251 | 1.266 | 2.2173 | 1.248 | 2.0845 | 1.228 | 2.0673 | 1.237 | 2.0439 |
θ=1 | 1.014 | 0.0141 | 1.034 | 0.0161 | 1.009 | 0.0166 | 1.009 | 0.0157 | 1.013 | 0.0157 | 1.009 | 0.0151 |
α=1 | 1.480 | 2.6725 | 1.429 | 2.8627 | 1.546 | 2.9849 | 1.519 | 2.8085 | 1.502 | 2.7973 | 1.511 | 2.7333 |
θ=3 | 3.043 | 0.1308 | 3.103 | 0.1497 | 3.027 | 0.154 | 3.023 | 0.1462 | 3.036 | 0.1464 | 3.024 | 0.1392 |
Parameters | MLEs | MPS | OLS | WLS | CVEs | AD | ||||||
Es | MSE | Es | MSE | Es | MSE | Es | MSE | Es | MSE | Es | MSE | |
α=0.25 | 0.315 | 0.0771 | 0.283 | 0.0674 | 0.335 | 0.0949 | 0.331 | 0.0878 | 0.323 | 0.0849 | 0.329 | 0.0868 |
θ=1 | 1.001 | 0.0047 | 1.010 | 0.0049 | 0.999 | 0.0053 | 0.999 | 0.0050 | 1.001 | 0.0050 | 0.999 | 0.0049 |
α=0.5 | 0.576 | 0.1816 | 0.534 | 0.1741 | 0.604 | 0.2142 | 0.596 | 0.1981 | 0.586 | 0.1943 | 0.595 | 0.1965 |
θ=1.5 | 1.505 | 0.0113 | 1.520 | 0.0120 | 1.504 | 0.0130 | 1.503 | 0.0123 | 1.506 | 0.0123 | 1.503 | 0.0121 |
α=0.75 | 0.875 | 0.4170 | 0.829 | 0.4199 | 0.902 | 0.4771 | 0.894 | 0.4451 | 0.884 | 0.4409 | 0.892 | 0.4370 |
θ=2 | 2.012 | 0.0217 | 2.033 | 0.0231 | 2.007 | 0.0256 | 2.007 | 0.0238 | 2.011 | 0.0239 | 2.007 | 0.0234 |
α=1 | 1.145 | 0.5971 | 1.101 | 0.6059 | 1.174 | 0.6490 | 1.163 | 0.6149 | 1.153 | 0.6132 | 1.162 | 0.6064 |
θ=1 | 1.004 | 0.0051 | 1.014 | 0.0054 | 1.002 | 0.0058 | 1.002 | 0.0055 | 1.004 | 0.0055 | 1.002 | 0.0054 |
α=0.3 | 0.363 | 0.0849 | 0.328 | 0.0754 | 0.389 | 0.1047 | 0.383 | 0.0972 | 0.375 | 0.0941 | 0.381 | 0.0957 |
θ=2 | 2.007 | 0.0180 | 2.025 | 0.0192 | 2.003 | 0.0211 | 2.003 | 0.0198 | 2.007 | 0.0198 | 2.003 | 0.0195 |
α=0.2 | 0.281 | 0.0654 | 0.252 | 0.0560 | 0.306 | 0.0862 | 0.300 | 0.0780 | 0.293 | 0.0748 | 0.298 | 0.0777 |
θ=3 | 3.015 | 0.0415 | 3.040 | 0.0441 | 3.010 | 0.0479 | 3.010 | 0.0452 | 3.015 | 0.0453 | 3.009 | 0.0444 |
α=0.8 | 0.935 | 0.4789 | 0.891 | 0.4883 | 0.958 | 0.5383 | 0.953 | 0.5067 | 0.943 | 0.5026 | 0.950 | 0.5011 |
θ=1 | 1.005 | 0.0054 | 1.016 | 0.0058 | 1.003 | 0.0062 | 1.003 | 0.0059 | 1.005 | 0.0059 | 1.003 | 0.0058 |
α=1 | 1.157 | 0.7315 | 1.115 | 0.7467 | 1.187 | 0.8219 | 1.174 | 0.7597 | 1.164 | 0.7562 | 1.175 | 0.7566 |
θ=3 | 3.010 | 0.0528 | 3.042 | 0.0561 | 3.004 | 0.0615 | 3.003 | 0.0577 | 3.009 | 0.0578 | 3.003 | 0.0570 |
Parameters | MLEs | MPS | OLS | WLS | CVEs | AD | ||||||
Es | MSE | Es | MSE | Es | MSE | Es | MSE | Es | MSE | Es | MSE | |
α=0.25 | 0.287 | 0.0343 | 0.265 | 0.0312 | 0.3042 | 0.0433 | 0.298 | 0.0393 | 0.294 | 0.0383 | 0.299 | 0.0395 |
θ=1 | 1.003 | 0.0023 | 1.008 | 0.0024 | 1.003 | 0.0026 | 1.002 | 0.0025 | 1.003 | 0.0025 | 1.002 | 0.0024 |
α=0.5 | 0.540 | 0.0819 | 0.511 | 0.0797 | 0.551 | 0.0967 | 0.547 | 0.0892 | 0.542 | 0.0882 | 0.548 | 0.0892 |
θ=1.5 | 1.504 | 0.0057 | 1.513 | 0.0059 | 1.503 | 0.0066 | 1.503 | 0.0062 | 1.504 | 0.0062 | 1.503 | 0.0061 |
α=0.75 | 0.797 | 0.1301 | 0.768 | 0.1295 | 0.811 | 0.1509 | 0.806 | 0.1397 | 0.801 | 0.1387 | 0.807 | 0.1392 |
θ=2 | 2.006 | 0.0099 | 2.018 | 0.0104 | 2.003 | 0.0116 | 2.003 | 0.0107 | 2.005 | 0.0107 | 2.003 | 0.0106 |
α=1 | 1.096 | 0.2412 | 1.069 | 0.2411 | 1.107 | 0.2800 | 1.104 | 0.2593 | 1.098 | 0.2578 | 1.104 | 0.2577 |
θ=1 | 1.005 | 0.0028 | 1.011 | 0.0029 | 1.003 | 0.0033 | 1.004 | 0.0030 | 1.005 | 0.0031 | 1.003 | 0.0030 |
α=0.3 | 0.326 | 0.0369 | 0.302 | 0.0344 | 0.336 | 0.0442 | 0.333 | 0.0412 | 0.329 | 0.0404 | 0.334 | 0.0411 |
θ=2 | 2.002 | 0.0092 | 2.012 | 0.0094 | 1.999 | 0.0102 | 1.999 | 0.0096 | 2.001 | 0.0096 | 1.999 | 0.0096 |
α=0.2 | 0.240 | 0.0262 | 0.220 | 0.0228 | 0.252 | 0.0320 | 0.248 | 0.0297 | 0.244 | 0.0288 | 0.249 | 0.0296 |
θ=3 | 3.009 | 0.0194 | 3.024 | 0.0201 | 3.005 | 0.0220 | 3.005 | 0.0207 | 3.008 | 0.0207 | 3.005 | 0.0205 |
α=0.8 | 0.846 | 0.1625 | 0.818 | 0.1621 | 0.854 | 0.1858 | 0.852 | 0.1748 | 0.847 | 0.1739 | 0.852 | 0.1725 |
θ=1 | 1.000 | 0.0027 | 1.007 | 0.0028 | 0.999 | 0.0032 | 0.999 | 0.0030 | 1.000 | 0.0030 | 0.999 | 0.0029 |
α=1 | 1.071 | 0.2538 | 1.044 | 0.2564 | 1.087 | 0.2868 | 1.080 | 0.2664 | 1.074 | 0.2651 | 1.081 | 0.2669 |
θ=3 | 3.006 | 0.0248 | 3.025 | 0.0258 | 3.003 | 0.0298 | 3.002 | 0.0274 | 3.006 | 0.0274 | 3.002 | 0.0273 |
Parameters | MLEs | MPS | OLS | WLS | CVEs | AD | ||||||
Es | MSE | Es | MSE | Es | MSE | Es | MSE | Es | MSE | Es | MSE | |
α=0.25 | 0.263 | 0.0162 | 0.249 | 0.0154 | 0.271 | 0.0204 | 0.268 | 0.0185 | 0.266 | 0.0183 | 0.269 | 0.0185 |
θ=1 | 1.000 | 0.0011 | 1.003 | 0.0011 | 1.000 | 0.0013 | 1.000 | 0.0012 | 1.001 | 0.0012 | 1.000 | 0.0012 |
α=0.5 | 0.512 | 0.0364 | 0.495 | 0.0362 | 0.519 | 0.0430 | 0.517 | 0.0397 | 0.514 | 0.0395 | 0.517 | 0.0397 |
θ=1.5 | 1.501 | 0.0028 | 1.506 | 0.0028 | 1.500 | 0.0032 | 1.500 | 0.0030 | 1.501 | 0.0030 | 1.500 | 0.0030 |
α=0.75 | 0.762 | 0.0563 | 0.745 | 0.0564 | 0.770 | 0.0637 | 0.767 | 0.0594 | 0.764 | 0.0592 | 0.767 | 0.0592 |
θ=2 | 2.000 | 0.0048 | 2.007 | 0.0049 | 1.999 | 0.0054 | 1.999 | 0.00511 | 2.000 | 0.0051 | 1.998 | 0.0050 |
α=1 | 1.026 | 0.0976 | 1.011 | 0.0979 | 1.034 | 0.1101 | 1.031 | 0.1031 | 1.028 | 0.1028 | 1.031 | 0.1033 |
θ=1 | 1.000 | 0.0013 | 1.004 | 0.0013 | 0.999 | 0.0015 | 1.000 | 0.0014 | 1.000 | 0.0014 | 1.000 | 0.0014 |
α=0.3 | 0.307 | 0.0191 | 0.291 | 0.0186 | 0.315 | 0.0229 | 0.313 | 0.0212 | 0.310 | 0.0210 | 0.313 | 0.0212 |
θ=2 | 2.000 | 0.0046 | 2.007 | 0.0047 | 1.999 | 0.0051 | 1.999 | 0.0048 | 2.000 | 0.0048 | 1.999 | 0.0048 |
α=0.2 | 0.215 | 0.0125 | 0.202 | 0.0114 | 0.222 | 0.0149 | 0.220 | 0.0138 | 0.217 | 0.0136 | 0.220 | 0.0138 |
θ=3 | 2.999 | 0.0100 | 3.008 | 0.0102 | 2.998 | 0.0113 | 2.998 | 0.0107 | 2.999 | 0.0107 | 2.998 | 0.0106 |
α=0.8 | 0.837 | 0.0749 | 0.821 | 0.0744 | 0.842 | 0.0846 | 0.841 | 0.0793 | 0.838 | 0.0790 | 0.842 | 0.0792 |
θ=1 | 1.002 | 0.0013 | 1.006 | 0.0014 | 1.001 | 0.0015 | 1.002 | 0.0014 | 1.002 | 0.0014 | 1.002 | 0.0014 |
α=1 | 1.030 | 0.0935 | 1.015 | 0.0935 | 1.040 | 0.1044 | 1.036 | 0.0978 | 1.033 | 0.0975 | 1.036 | 0.0976 |
θ=3 | 3.003 | 0.0116 | 3.014 | 0.0119 | 3.002 | 0.0133 | 3.002 | 0.0125 | 3.004 | 0.0125 | 3.001 | 0.0124 |
n | Min | Max | Mean | Median | St derivation | Kurtosis | Skweness | |
Data Set 1: | 100 | 60.000 | 463.000 | 175.670 | 158.000 | 83.166 | 1.713 | 1.316 |
Data Set 2: | 100 | 0.390 | 5.560 | 2.621 | 2.700 | 1.013 | 0.043 | 0.362 |
Data Set 3: | 90 | 121.000 | 670.000 | 294.322 | 274.500 | 131.618 | 0.369 | 0.927 |
Data Set 4: | 32 | 0 | 8 | 3.97 | 4 | 2.24 | -0.98 | -3.35 |
Model | AIC | CAIC | BIC | HQIC | K-S | p-value |
WGQLD | 1142.145 | 1142.268 | 1147.355 | 1144.253 | 0.059902 | 0.865573 |
GQLD | 1145.844 | 1145.968 | 1151.055 | 1147.953 | 0.095349 | 0.323229 |
QLD | 1180.179 | 1180.303 | 1185.389 | 1182.288 | 0.216170 | 0.000174 |
PD | 1237.721 | 1237.845 | 1242.932 | 1239.83 | 0.340971 | 1.59e-10 |
TSPD | 1156.301 | 1156.425 | 1161.511 | 1158.41 | 0.143704 | 0.032159 |
Model | AIC | AICc | BIC | HQIC | K-S | p-value |
WGQLD | 295.1091 | 295.2328 | 300.3194 | 297.2178 | 0.105898 | 0.212049 |
GQLD | 306.1634 | 306.2871 | 311.3737 | 308.2721 | 0.123234 | 0.095915 |
QLD | 346.108 | 346.2317 | 351.3183 | 348.2167 | 0.223871 | 8.86e-05 |
PD | 396.7418 | 396.8655 | 401.9522 | 398.8505 | 0.320272 | 2.46-09 |
TSPD | 350.3233 | 350.447 | 355.5336 | 352.432 | 0.220935 | 0.000115 |
Model | AIC | AICc | BIC | HQIC | K-S | p-value |
WGQLD | 1118.42 | 1118.558 | 1123.420 | 1120.436 | 0.0606885 | 0.8946827 |
GQLD | 1122.41 | 1122.548 | 1127.410 | 1124.426 | 0.0900125 | 0.4593636 |
QLD | 1154.697 | 1154.835 | 1159.696 | 1156.713 | 0.2081433 | 0.0008209 |
PD | 1207.242 | 1207.38 | 1212.241 | 1209.258 | 0.3435296 | 1.190e-09 |
TSPD | 1132.559 | 1132.697 | 1137.559 | 1134.575 | 0.1352032 | 0.0744752 |
Model | AIC | AICc | BIC | HQIC | K-S | p-value |
WGQLD | 161.0522 | 161.4966 | 163.8546 | 161.9487 | 0.3077698 | 0.006804395 |
GQLD | 166.9975 | 167.4419 | 169.7999 | 167.894 | 0.3318577 | 0.00269967 |