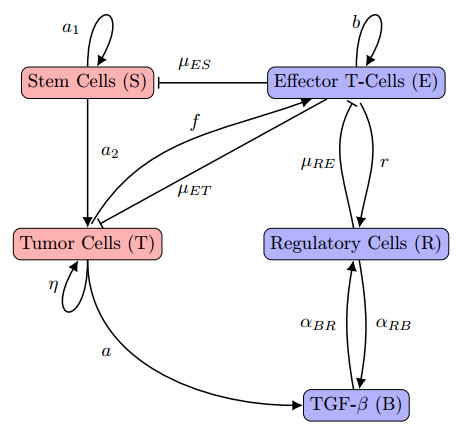
Citation: Samantha L Elliott, Emek Kose, Allison L Lewis, Anna E Steinfeld, Elizabeth A Zollinger. Modeling the stem cell hypothesis: Investigating the effects of cancer stem cells and TGF−β on tumor growth[J]. Mathematical Biosciences and Engineering, 2019, 16(6): 7177-7194. doi: 10.3934/mbe.2019360
[1] | J. Ignacio Tello . On a mathematical model of tumor growth based on cancer stem cells. Mathematical Biosciences and Engineering, 2013, 10(1): 263-278. doi: 10.3934/mbe.2013.10.263 |
[2] | Ghassen Haddad, Amira Kebir, Nadia Raissi, Amira Bouhali, Slimane Ben Miled . Optimal control model of tumor treatment in the context of cancer stem cell. Mathematical Biosciences and Engineering, 2022, 19(5): 4627-4642. doi: 10.3934/mbe.2022214 |
[3] | Donggu Lee, Sunju Oh, Sean Lawler, Yangjin Kim . Bistable dynamics of TAN-NK cells in tumor growth and control of radiotherapy-induced neutropenia in lung cancer treatment. Mathematical Biosciences and Engineering, 2025, 22(4): 744-809. doi: 10.3934/mbe.2025028 |
[4] | Katrine O. Bangsgaard, Morten Andersen, Vibe Skov, Lasse Kjær, Hans C. Hasselbalch, Johnny T. Ottesen . Dynamics of competing heterogeneous clones in blood cancers explains multiple observations - a mathematical modeling approach. Mathematical Biosciences and Engineering, 2020, 17(6): 7645-7670. doi: 10.3934/mbe.2020389 |
[5] | Qiaojun Situ, Jinzhi Lei . A mathematical model of stem cell regeneration with epigenetic state transitions. Mathematical Biosciences and Engineering, 2017, 14(5&6): 1379-1397. doi: 10.3934/mbe.2017071 |
[6] | Jihong Yang, Hao Xu, Congshu Li, Zhenhao Li, Zhe Hu . An explorative study for leveraging transcriptomic data of embryonic stem cells in mining cancer stemness genes, regulators, and networks. Mathematical Biosciences and Engineering, 2022, 19(12): 13949-13966. doi: 10.3934/mbe.2022650 |
[7] | Abeer S. Alnahdi, Muhammad Idrees . Nonlinear dynamics of estrogen receptor-positive breast cancer integrating experimental data: A novel spatial modeling approach. Mathematical Biosciences and Engineering, 2023, 20(12): 21163-21185. doi: 10.3934/mbe.2023936 |
[8] | Mohammad A. Tabatabai, Wayne M. Eby, Karan P. Singh, Sejong Bae . T model of growth and its application in systems of tumor-immunedynamics. Mathematical Biosciences and Engineering, 2013, 10(3): 925-938. doi: 10.3934/mbe.2013.10.925 |
[9] | Evans K. Afenya . Using Mathematical Modeling as a Resource in Clinical Trials. Mathematical Biosciences and Engineering, 2005, 2(3): 421-436. doi: 10.3934/mbe.2005.2.421 |
[10] | Hsiu-Chuan Wei . Mathematical modeling of tumor growth: the MCF-7 breast cancer cell line. Mathematical Biosciences and Engineering, 2019, 16(6): 6512-6535. doi: 10.3934/mbe.2019325 |
It's been almost 20 years since the renewed hypothesis and subsequent confirmation of cancer stem cells has fundamentally changed the way we view the development and treatment of tumors [1,2]. Soon after this discovery, cancer stem cells were confirmed in many tumor models (including both solid tumors and leukemias), cultured cell lines, and primary human tumors [3,4,5,6,7,8,9,10,11]. Cancer stem cell theory describes tumors as comprised of a heterogeneous population of cells, in which there is a subpopulation of tumor cells that behave similarly to the haematopoetic stem cells in normal tissues (reviewed in [12,13]). Tumor cells with this "stemness" property have low proliferation rates, but when they do divide they can undergo symmetric (creation of two identical daughter stem cells) or asymmetric cell division [14,15]. Asymmetric division creates one daughter cell that retains its "stemness" to become any type of cell and seed new tumors, while the other daughter cell becomes slightly more differentiated (yet still capable of great plasticity) to regenerate the tumor tissue [13,16]. Whether these cells arise from mutations of haematopoetic stem cells within adult tissue, de-differentiated tumor cells due to environmental pressures [16,17,18], former immune cells [19], or another mechanism, it is clear that-cells with cancer stem cell phenotypes contribute to drug and radiation resistance of tumors [20,21] as well as increased metastatic potential [22,23]. Recent approaches indicate that targeting cancer stem cells as part of a combined therapeutic regime may increase patient survival outcomes [24,25,26].
Recent advances have also elucidated the critical importance of transforming growth factor β (TGF-β) in the progression of cancer, as both a tumor repressor and later as a driver of metastasis and immune evasion (reviewed in [27,28]). The TGF-β pathway is typically responsible for signaling cellular senescence and apoptosis, making it a key anti-tumor agent and powerful immune suppressor. Paradoxically, TGF-β also promotes angiogenesis and metastasis, thus promoting tumor invasiveness. TGF-β is often upregulated within the tumor microenvironment, and can impact not only survival of tumor cells but suppression of the immune response to tumor cells. For example, TGF-β secreted by tumor cells specifically prevents CD8+ t-cell release of perforin and granzymes [29], TGF-β secreted by fibroblasts block CD8+ t-cells from entering the tumor microenvironment [30], and TGF-β signaling may be a mechanism that keeps cancer stem cells dormant and resistant to immune recognition [31].
Targeting resident CD8+ T-cells, as well as repurposing anti-viral CD8+ T-cells may be important in developing new cancer therapies [32,33]. However, the immune suppression of CD8+ T-cells by TGF-β, lymphocyte exhaustion, and the repression of antigen-specific targeting of both cancer cells and cancer stem cells must be overcome for these therapies to be effective [34,35,36,37]. To explore this, we have created a mathematical model that encompasses both the contributions of cancer stem cells and TGF-β in tumor growth and tumor progression, adding to the work done in [38,39]. We calibrate the model parameters using experimental data from [40], which present data for chemically induced skin papillomas, a type of HPV in mice where one randomized group of mice treated with 5-Fluorouracil (5-FU) and the control group were not treated.
Mathematical models have sought to help explain observed behaviors of tumor cell populations. Early mathematical models to include cancer stem cells were published in 2006 [41,42,43], were modified as more information was gathered [44,45,46,47] and remain a key component of tumor modeling today [38,39,48,49,50]. Similarly, mathematical modeling of the role of TGF-β in tumor progression, which began in 1991 [51], continues to be modified as new information emerges [52,53].
In order to understand the combined contributions of cancer stem cells and TGF-β on the progression of the cancer, we propose a coupled model that incorporates cancer stem cells (S), effector CD8+ T-cells (E), regulatory T-cells (R), tumor cells (T), and transforming growth factor TGF-β (B), which is produced by both regulatory T-cells (also called Tregs) and tumor cells in the model. The relationships between the five state variables are illustrated in Figure 1. We begin by describing the no-treatment model.
In order to model the dynamics between immune cells, tumor cells and cancer stem cells, several simplifying assumptions must be made. To effectively fight tumor cells, the immune system must balance the necessity of being non-reactive to self-tissues (or risk auto-immune reactions) with the essential task of recognizing subtle differences in tumor antigens to remove aberrant cancerous cells. The immune cells responsible for this tumor recognition are primarily lymphocytes: natural killer cells (NK), natural killer T-cells (NKT), and CD8+ T-cells, with help from other immune mediators (reviewed in [54,55,56]). However, chronic stimulation, such as those found in anti-viral and anti-tumor immune responses, can exhaust lymphocytes and prevent them from functioning [56,57].
In this model we consider activated CD8+ T-cells and regulatory T-cells as the primary lymphocytes in the system. This is due to the firmly established role of CD8+ T-cells in cancer therapies; research on NK and NKT-cells is still rapidly evolving [58,59]. Regulatory T-cells are the primary immune cells that produce TGF-β to suppress inappropriate immune responses [60], and are thus essential to our inclusion of TGF-β in the model.
As in [38], we assume that cancer stem cells have the potential to divide either symmetrically into two cancerous stem-cells, or asymmetrically into either a cancerous stem-cell and a non-stem cancer cell or two non-stem cancer cells, with respective probabilities a1,a2, and a3. We also assume that the typical population of cancer stem cells begins at the low end of ranges calculated through experimental data: approximately 1 percent of the total cancer cell population [14,15]. We denote the natural death rates of each population by δ, while the kill-terms resulting from various population interactions are denoted by μ. The following system of ordinary differential equations describes the changing populations of each of the five state variables.
dSdt=(a1−a3)S−μESES−δSS | (2.1) |
dEdt=bE+fET1+c3TB−rE−μRERE−δEE | (2.2) |
dBdt=aT2c2+T2+αRBR−δBB | (2.3) |
dRdt=rE+αBRRBc4+B−δRR | (2.4) |
dTdt=ηT(1−Tk)+(a2+2a3)S−μETET1+c1B−δTT | (2.5) |
Equation 2.1 describes the changes occuring in the stem cell population, S. The stem cell population grows at a rate dictated by the quantity a1−a3, which governs the number of daughter stem-cells produced from both symmetric and asymmetric stem cell division. Interactions between cancer cells and CD8+ T-cells result in cancer stem cell death, described by the μESES term. Finally, cancer stem cells die naturally at a rate of δS-we note that due to stem cell longevity, the natural death rate for stem cells is almost negligible.
Equation 2.2 describes the population change of the effector CD8+ T-cells, E. These cells have a basal growth rate of b and are recruited to the tumor site as the tumor population begins to swell, as described in [39]. The inhibitory effects of the TGF-β and the tumor on recruitment and proliferation are accounted for by the (1+c3TB)−1 term. The effector cells revert to regulatory T-cells at a rate of r per unit time, and the shutdown of the activated effector cells by the regulatory T-cells is expressed by the μRERE term.
The change in TGF-β concentration over time is expressed in Equation 2.3. The production of TGF-β is dependent on the tumor and Treg populations, as presented in [39]. We note that there are other existing models for describing TGF-β population. A recent example is by Khatibi et al. in [53]. Upregulation of TGF-β as a result of the increasing tumor cell population is given by the Michaelis-Menten term, aT2c2+T2. Conversely, the production of TGF-β by the Tregs is linear, as described in the second term.
As defined in Equation 2.4, the increase in regulatory T-cells occurs via two methods. First, reversal of the activated effector CD8+ T-cells contributes to an increasing Treg population. In addition, Tregs are activated by TGF-β; thus, an increasing concentration of TGF-β results in an increase in the Treg population as well, described by the αBRRBc4+B term.
The change in the tumor population over time is described in Equation 2.5. We assume that in the absence of immune cells, the tumor grows logistically. We note that another natural choice of model for tumor growth is the Gompertz Equation, but we found no significant change in the behavior of the populations when implementing this model and therefore opted to use the simpler logistic growth model. The cancerous stem cell division into non-stem cancer cells also contributes to the tumor population, governed by the (a2+2a3)S term. Interactions between the effector CD8+ T-cells and the tumor cells result in tumor apoptosis at a rate inversely proportional to the TGF-β production.
Figure 2 shows the behavior of each of the five state variable populations in the no-treatment case. Parameter values used for this simulation can be found in Table 1.
Name | Description | Estimate | Units | Reference |
a | Maximum rate of TGF-β production | 0.3 | days−1ng/ml | [39] |
a1 | Probability of symmetric division of a CSC | 0.01 | − | [38] |
a2 | Probability of asymmetric division of a CSC | 0.99 | − | [38] |
a3 | Probability of symmetric differentiation of a CSC | 1−a1−a2 | − | [38] |
αBR | Activation of Tregs by TGF-β | 0.07 | days−1 | [61] |
αRB | Production of TGF-β by Tregs | 10−6 | ng/ml ⋅ days−1⋅#−1 | Estimated |
b | Basal growth rate of effector cells | 0.1245 | days−1 | [62] |
c1 | TGF-β inhibitory parameter for induction of tumor death | 100 | ml/ng | [39] |
c2 | Steepness coefficient of TGF-β production | 3.036× 109 | #2 | [39] |
c3 | Magnitude of inhibition associated with tumor growth and TGF-β | 50 | ml/(ng #) | Estimated |
c4 | Activation constant half saturation | 0.5 | ng/ml | Estimated |
δB | Natural death rate of TGF-β | 0.44 | days−1 | Estimated |
δE | Natural death rate of effector cells | 0.15 | days−1 | Estimated |
δR | Natural death rate of regulatory cells | 10−5 | days−1 | [39] |
δS | Natural death rate of CSCs | 0.0005 | days−1 | [38] |
δT | Natural death rate of tumor cells | 0.886 | days−1 | Fit to [40] |
η | Proliferation rate of tumor cells | 0.997 | days−1 | Fit to [40] |
f | Tumor antigenicity | 0.62 | #−1days−1 | [39] |
γ | Decay coefficient for chemo drug | 49.9066 | days−1 | [63] |
k | Carrying capacity for tumor cells | 9.06865×108 | # | Fit to [40] |
KE | Death of effector cells due to chemo | 4.367 | days−1 | Estimated |
KR | Death of T-regulatory cells due to chemo | 4.367 | days−1 | Estimated |
KT | Death of tumor cells due to chemo | 8.734 | days−1 | Fit to [40] |
μES | Interactions of CSCs and effector cells leading to CSC death | 10−9 | (#∗time)−1 | Estimated |
μET | Effector T-cell induced tumor death rate/removal rate | 10−5 | #−1days−1 | [39] |
μRE | Regulatory T-cell induced effector cell death rate/removal rate | 10−5 | #−1days−1 | [39] |
νb(t) | Chemotherapy dosage concentration | Variable | mg/kg | [40] |
r | Rate at which effector cells become regulatory cells | 0.01 | days−1 | [39] |
In this section, we incorporate the effects of chemotherapy in the dynamics between the immune system, cancer stem cells and tumor cells. We assume that chemotherapy has a negative impact on CD8+ T-cells, tumor cells and regulatory T-cells, but that extermination rates may differ. We assume that chemotherapy does not affect the cancer stem cells; it is designed to target fast-cycling cells, while cancer stem cells are characterized by their slow division rates [14,15]. We let M denote the concentration of chemotherapy drug in the blood. The following system of equations describes the model with this chemotherapy regimen incorporated.
dSdt=(a1−a3)S−μESES−δSS | (2.6) |
dEdt=bE+fET1+c3TB−rE−μRERE−δEE−KE(1−e−M)E | (2.7) |
dBdt=aT2c2+T2+αRBR−δBB | (2.8) |
dRdt=rE+αBRRBc4+B−δRR−KR(1−e−M)R | (2.9) |
dTdt=ηT(1−Tk)+(a2+2a3)S | (2.10) |
−δTT−μETET1+c1B−KT(1−e−M)TdMdt=−γM+vb(t) | (2.11) |
In the updated model, the cancer stem cell and TGF-β equations remain unchanged from their no-treatment counterparts. The equations for the effector CD8+ T-cells, regulatory T-cells, and tumor cells each have an additional removal term to allow for the destruction of cells by the chemotherapy. Equation 2.11 describes the concentration of chemotherapy drug in the blood. We assume that drug concentration decays exponentially with time and include an impulse term, νb, to describe the discrete dosage administrations.
To ensure model alignment with experimental data, the tumor state equations were fit to data from [40], using the Delayed Rejection Adaptive Metropolis (DRAM) algorithm for Bayesian inference [64]. This variation of the Metropolis-Hastings algorithm allows for periodic updating of the covariance matrix used in the proposal distribution via the added adaptation step. Additionally, use of this algorithm results in better mixing of posterior chains via the inclusion of a delayed rejection procedure (which allows for selection of an alternate sample to be drawn from a narrower proposal distribution in place of outright rejection).
For the non-treatment model, the parameters δT, η and k were fit, with parameter samples drawn from the priors U(0,1), U(0,2) and U(108,1010), respectively. Uniform prior bounds were selected to ensure biologically realistic posterior values. All remaining model parameters were fixed at their literature values, or estimated where literature values were not available. See Table 1 for fixed parameter values. The data used for calibration was from the first observed tumor (left untreated) on mouse CM37 in [40]. The final fit of Equation 2.5 to the data is shown in Figure 3.
Upon completion of the calibration for the no-treatment model, a further calibration was performed upon the chemotherapy model, using data from mouse CM41 in [40]. This second mouse was treated with 5-Fluorouracil with a dosage of 50 mg/kg every seven days, beginning at day 26 when the tumor first exceeded 3 mm in size. Of the three tumors for CM41, we used the data from the second tumor as this tumor most closely mimicked the growth rate of the tumor chosen for the no-treatment case. With the assumption that proliferation rate, carrying capacity, and tumor death rate should be very similar in this second tumor, only KT-the kill rate for tumor cells due to chemotherapy-was estimated in this second calibration procedure. A uniform prior of U(0,20) was used to initialize the DRAM procedure. The chemotherapy decay rate, denoted by γ, was fixed at 49.9066 days−1 according to the half-life function, γ=ln(2)/t1/2, where the half-life of 5-Fluorouracil is t1/2=20 minutes [65]. The kill rates for CD8+ and T-regulatory cells, KE and KR, were chosen to be half of the determined KT value, as these rates should be smaller than KT but still on the same order of magnitude [63]. The final fit of Equation 2.10 to the data from [40] is shown in Figure 3.
Figure 4 shows the dynamics of the six populations over the chemotherapy administration period outlined in [40], which is administering 50 mg/kg of 5-Fluorouracil (5-FU) per week to mice with skin carcinomas, beginning when the tumor reached 3-4 mm in size. It can be observed that while this treatment regimen reduces the rate at which the tumor develops, chemotherapy at this dosage level is still ultimately unsuccessful in eliminating the tumor. Another regimen Loizides et al experiment with is to administer mice with 100 mg/kg 5-FU weekly for 12 weeks. The result of this course of treatment can be seen in Figure 5.
5-FU is a very commonly used drug to treat various cancers including breast cancer, rectal cancer, cancers of head and neck and skin cancer. It is particularly effective for colorectal cancer [66]. Smith et al. find that continuous infusional 5-FU chemotherapy is more effective on colorectal cancer than conventional chemotherapy in [67]. In the trial that is addressed in the work of Smith et al., 5-FU is administered continuously by an ambulatory pump for 18 weeks at a dose of 200 mg/m2. When this human dosage is converted to mice, we see the resulting cell populations in Figure 6. Smith et al. report that toxicity was well tolerated however, lethargy, diarrhea and vomiting occurred more frequently in the group treated with 5-FU than the group who received conventional chemotherapy.
We experimented with the two weekly doses, 50 mg/kg and 100 mg/kg of 5-FU as in the study of Loizides by administering the chemotherapy twice a week, half the dose each time. The 100/2 mg/kg twice weekly 5-FU keeps the tumor load lower until about day 100, even though the outcome remains the same. The behavior of the tumor in both of this regimens can be seen in Figures 7 and 8.
Despite experimenting with the dosage and the frequency of drug administration, we have not been able to eliminate the tumor using chemotherapy, only. More specifically, we have simulated: 100/7 mg/kg, daily for 12 weeks, 100/7 mg/kg daily for 24 weeks and 450 mg/m2 daily for five days, as presented in [66]. Caroli et al discuss the serious adverse effects of a high dose of 5-FU, more specifically at a level of 2600 mg/m2 which is equivalent to 70 mg/kg for humans, administered weekly [68]. Therefore, we did not exceed 100 mg/kg in our simulations.
Sensitivity analysis was performed on both the non-treatment and chemotherapy models using Partial Rank Correlation Coefficients (PRCC) as a metric. Partial correlation metrics measure the effect of an input xj on an output y after removing the effects of all other inputs on y. Specifically, the partial correlation coefficient between an input xj and an associated scalar output y is computed by measuring the correlation between the residuals xj−ˆxj and y−ˆy, where
ˆxj=c0+k∑p=1,p≠jcpxp and ˆy=b0+k∑p=1,p≠jbpxp. | (5.1) |
We use the rank-transformed partial rank correlation coefficients as these tend to perform better in cases where the relationship between the parameter and output is nonlinear but monotonic [69]. Note that parameters to which the output is more sensitive have PRCCs close to 1 or -1, where a negative score indicates that the parameter and the quantity of interest are inversely related. The scalar quantity of interest (QoI) was defined to be the number of cancer stem cells (S) plus the number of tumor cells (T) at the final day of the data observation period. For the computation of the PRCC scores, 1000 parameter samples were generated by sampling each parameter uniformly from an interval ranging from ±25% of the nominal parameter value (listed in Table 1).
Figure 9 illustrates the results of the sensitivity analysis for both models. In both cases, the QoI is highly sensitive and inversely related to the tumor cell death rate (δT), and also highly sensitive but positively related to the tumor cell proliferation rate (η). In comparison, the QoI has negligible sensitivity to all other parameters.
Using the no-treatment model (2.1)-(2.5), we analyze the stability of the system. With five highly-coupled states and 23 parameters, the Jacobian matrix cannot be determined symbolically, so we proceed using the parameter values from Table 1. Our system of nonlinear differential equations yields 18 equilibrium points, only two of which are real, nonnegative, and biologically relevant. The first, (S,E,B,R,T)=(0,0,0,0,0), represents the tumor-free equilibrium, while (S,E,B,R,T)=(5.999998213,0,0,0,1.009648946×108) represents the equilibrium with the tumor near maximum capacity. The lack of additional biologically relevant equilibria suggests that there is no set of state variables at which a small, nonlethal tumor can be controlled by an effective immune system. We investigate the stability of our two equilibrium points below.
Linearization of the system of equations (2.1)-(2.5) yields the Jacobian matrix
[0.009500000−0.035500000−0.0510−9000.010−10−500.010000.111], | (6.1) |
with eigenvalues
λ1=0.111, λ2=0.0095, λ3=−0.05, λ4=−10−5, λ5=−0.0355. |
The existence of three negative and two positive eigenvalues at the origin indicates that this equilibrium is a saddle point. To gain insight into directions which are stable versus unstable, we investigate the eigenvectors corresponding to each of the eigenvalues given as columns of the following matrix:
[0−0.9950000000−0.9630012.00×10−81.87×10−80001.0000.271−10.098000]. |
Based on the eigenvectors, it can be seen that the two unstable directions align roughly with the S and T axes, while the three stable directions exist in E−B−R space. That is, a solution trajectory approaching the origin will eventually veer away towards a non-zero tumor state.
This second equilibrium represents the system near maximum tumor capacity (note that tumor carrying capacity was defined to be 9.06865×108 cells in Section 3). The Jacobian matrix is given by
[0000.009500−0.0334000−0.05010−901.767×10−1500.010.064000−1.68000.010−0.111], |
with eigenvalues
λ1=0.0156+0.0271i, λ2=0.0156−0.0271i, λ3=−0.0313, λ4=−0.1110, λ5=−0.0334. |
The eigenvalues indicate stability in three directions, with a spiral source in the remaining two-dimensional plane. The corresponding eigenvectors are the columns of the following matrix:
[0.1314−0.2276i0.1314+0.2276i−0.2619−6.9585×10−11−0.02160000−0.04550.2098+0.3635i0.2098−0.3635i−0.4182−1.1929×10−10−0.03230.86620.86620.86301.6899×10−100.07610.0654−0.0140i0.0654+0.0140i0.108310.9953]. |
The dominance of the tumor state in the fourth eigenvector (corresponding to a negative eigenvalue), suggests that solution trajectories approaching the equilibrium along the T axis will be drawn towards a maximum tumor capacity state.
Overall, the stability analysis of this system suggests that unless the cancer stem cells and tumor cells are eradicated completely (S=T=0), simulation trajectories will inevitably converge to a maximum tumor capacity equilibrium.
In this work, we have modeled the role of cancer stem cells and TGF-β with no-treatment and with chemotherapy using experimental data from [40] to calibrate and validate the models. With no-treatment, the tumor grows as indicated by experimental data in [40], as can be seen in Figure 2. In this case, the tumor reaches its carrying capacity while the CD8+ T-cell population approaches zero. Although the CD8+ T-cells will never actually vanish, this behavior indicates that the CD8+ T-cell population is functionally zero, as they are shut-down by the TGF-β whose concentration reaches a maximum. Stability analysis of the no-treatment model reveals two unstable equilibria. An analysis of the tumor-free equilibrium predicts a growing tumor in the presence of tumor or cancer stem cells. The close to maximum tumor capacity equilibrium is stable in the direction of the tumor and hence, yields a similar predicament. In the presence of tumor or cancer stem cells, the tumor cells reach their maximum population.
It is shown that under chemotherapy, which targets only tumor cells but negatively affects the immune cells, the cancer will relapse. As chemotherapy does not target the cancer stem cells, we conclude that the tumor cells recover due to the presence of the cancer stem cells. This matches current experimental data [40,70,71]. This behavior is illustrated in Figure 4. The CD8+ T-cells become functionally zero, as in the no-treatment case, with maximum tumor capacity reached shortly after termination of the chemotherapy.
Due to the simplifying assumptions made, this model can only provide a theoretical framework for the actual physical system and reveal the impacts cancer stem cell populations have on the tumor and immune system from an abstract perspective.
This work was supported by NSF DMS [1239280]. EZ was supported by the St. Joseph's College Faculty Summer Research Grant.
The authors would like to thank Dr. Ami Radunskaya for valuable discussions.
All authors declare no conflicts of interest in this paper.
[1] | T. Reya, S. J. Morrison, M. F. Clark, et al., Stem cells, cancer, and cancer stem cells, Nature, 414 (2001), 105–111. |
[2] | M. Al-Hajj, M. S. Wicha, A. Benito-Hernandez, et al., Prospective identification of tumorigenic breast cancer cells, Proc. Nat. Acad. Sci., 100 (2003), 3983–3988. |
[3] | T. Kondo, T. Setoguchi and T. Taga, Persistence of a small subpopulation of cancer stem-like cells in the C6 glioma cell line, Proc. Nat. Acad. Sci., 101 (2004), 781–786. |
[4] | A. Cozzio, E. Passegué, P.M. Ayton, et al., Similar MLL-associated leukemias arising from self-renewing stem cells and short-lived myeloid progenitors, Gene Dev., 17 (2003), 3029–3035. |
[5] | S. K. Singh, I. D. Clarke, T. Hide, et al., Cancer stem cells in nervous system tumors, Oncogene, 23 (2004), 7267. |
[6] | X. Yuan, J. Curtin, Y. Xiong, et al., Isolation of cancer stem cells from adult glioblastoma multi-forme, Oncogene, 23 (2004), 9392. |
[7] | A. T. Collins, P. A. Berry, C. Hyde, et al., Prospective identification of tumorigenic prostate cancer stem cells, Cancer Res., 65 (2005), 10946–10951. |
[8] | , N. Haraguchi, H. Inoue, F. Tanaka, et al., Cancer stem cells in human gastrointestinal cancers, Human Cell, 19 (2006), 24–29. |
[9] | C. Li, D. G. Heidt, P. Dalerba, et al., Identification of pancreatic cancer stem cells, Cancer Res., 67 (2007), 1030–1037. |
[10] | I. Zucchi, S. Sanzone, S. Astigiano, et al., The properties of a mammary gland cancer stem cell, P. Natl. A. Sci., 104 (2007), 10476–10481. |
[11] | S. Ma, K. Chan, L. Hu, et al., Identification and characterization of tumorigenic liver cancer stem/progenitor cells, Gastroenterology, 132 (2007), 2542–2556. |
[12] | A. Kreso and J. Dick, Evolution of the cancer stem cell model, Cell Stem Cell, 14 (2014), 275–291. |
[13] | , K. Dzobo, D. A. Senthebane, A. Rowe, et al., Cancer stem cell hypothesis for therapeutic inno-vation in clinical oncology? Taking the root Out, not chopping the leaf, OMICS A J. Integ. Bio., 20 (2016), 681–691. |
[14] | A. L. Allan, S. A. Vantyghem, A. B. Tuck, et al., Tumor dormancy and cancer stem cells: impli-cations for the biology and treatment of breast cancer metastasis, Breast Dis., 26 (2006), 87–98. |
[15] | D. Beier, P. Hau, M. Proescholdt, et al., CD133+ and CD133- glioblastoma-derived cancer stem cells show differential growth characteristics and molecular profiles, Cancer Res., 67 (2007), 4010–4015. |
[16] | , H. Lopez-Bertoni, Y. Li and J. Laterra, Cancer stem cells: dynamic entities in an ever-evolving paradigm, Bio. Med., 7 (2015), 1–10. |
[17] | P. Wang, W. Wan, S. Xiong, et al., Cancer stem-like cells can be induced through dedifferentia-tion under hypoxic conditions in glioma, hepatoma and lung cancer, Cell Death Discov., 3 (2017), 16105. |
[18] | Y. Xu, C. So, H. Lam, et al., Apoptosis reversal promotes cancer stem cell-like cell formation, Neoplasia, 20 (2018), 295–303. |
[19] | P. Grandics, The cancer stem cell: Evidence for its origin as an injured autoreactive T Cell, Molec. Cancer, 5 (2006), 1–6. |
[20] | H. Lou and M. Dean, Targeted therapy for cancer stem cells: the patched pathway and ABC transporters, Oncogene, 26 (2007), 1357. |
[21] | J. N. Rich, Cancer stem cells in radiation resistance, Cancer Res., 67 (2007), 8980–8984. |
[22] | N. V. Margaryan, H. Hazard-Jenkins, M. A. Salkeni, et al., The stem cell phenotype of aggressive breast cancer cells, Cancers, 11 (2019), 340. |
[23] | S. Pece, D. Disalvatore, D. Tosoni, et al., Identification and clinical validation of a multigene assay that interrogates the biology of cancer stem cells and predicts metastasis in breast cancer: A retrospective consecutive study, EBioMedicine, (2019), In press. |
[24] | G. L. Gravina, A. Mancini, A. Colapietro, et al., The small molecule ephrin receptor inhibitor, GLPG1790, reduces renewal capabilities of cancer stem cells, showing anti-tumour efficacy on preclinical glioblastoma models, Cancers, 11 (2019), 359. |
[25] | B. Bao, Z. Wang, S. Ali, et al., Metformin inhibits cell proliferation, migration and invasion by attenuating CSC function mediated by deregulating miRNAs in pancreatic cancer cells, Cancer Prevent. Res., 5 (2012), 355–364. |
[26] | L. MacDonagh, M. F. Gallagher, B. French, et al., Targeting the cancer stem cell marker, alde-hyde dehydrogenase 1, to circumvent cisplatin resistance in NSCLC, Oncotarget, 8 (2017), 72544–72563. |
[27] | J. J. Huang and G. C. Blobe, Dichotomous roles of TGF-β in human cancer, Biochem. Soc. Trans., 44 (2016), 1441–1454. |
[28] | A. Dahmani and J. Delisle, TGF-β in T cell biology: Implications for cancer immunotherapy, Cancers, 10 (2018), 194. |
[29] | D. A. Thomas and J. Massague, TGF-β directly targets cytotoxic T cell functions during tumor evasion of immune surveillance, Cancer Cell, 8 (2005), 369–380. |
[30] | S. Mariathasan, S. J. Turley, D. Nickles, et al., TGF-β attenuates tumour response to PD-L1 block-ade by contributing to exclusion of T cells, Nature, 554 (2018), 544. |
[31] | V. Ingangi, M. Minopoli, C. Ragone, et al., Role of microenvironment on the fate of disseminating cancer stem cells, Front. Onc., 9 (2019), 82. |
[32] | F. Mami-Chouaib, C. Blanc, S. Corgnac, et al., Resident memory T cells, critical components in tumor immunology, J. Immunother. Cancer , 6 (2018), 87. |
[33] | P. C. Rosato, S. Wijeyesinghe, J. M. Stolley, et al., Virus-specific memory T cells populate tumors and can be repurposed for tumor immunotherapy, Nat. Comm., 10 (2019), 567. |
[34] | N. Badrinath and S. Y. Yoo, Recent advances in cancer stem cell-targeted immunotherapy, Can- cers, 11 (2019), 310. |
[35] | F. Vahidian, P. H. G. Dujif, E. Safarzadeh, et al., Interactions between cancer stem cells, immune system and some environmental components: friends or foes?, Immunol. Lett., 208 (2019), 19–29. |
[36] | I. A. Voutsadakis, Immune ligands for cytotoxic T lymphocytes (CTLS) in cancer stem cells (CSCS), Front. Biosci., 23 (2018), 563–583. |
[37] | N. D. Price, G. Foltz, A. Madan, et al., Systems biology and cancer stem cells, J. Cell. Molec. Med., 12 (2008), 97–110. |
[38] | S. L. Weekes, B. Barker, S. Bober, et al., A multicompartment mathematical model of cancer stem cell-driven tumor growth dynamics, B. Math. Biol., 7 (2014), 1762–1782. |
[39] | S. Wilson and D. Levy, A mathematical model of the enhancement of tumor vaccine efficacy by immunotherapy, B. Math. Biol., 7 (2012), 1485–1500. |
[40] | C. Loizides, D. Iacovides, M. M. Hadjiandreou, et al., Model-based tumor growth dynamics and therapy response in a mouse model of De Novo Carcinogenesis, PLoS ONE, 10 (2015), e0143840. |
[41] | R. Ganguly and I. K. Puri, Mathematical model for the cancer stem cell hypothesis, Cell Prolif., 39 (2006), 3–14. |
[42] | A. L. Garner, Y. Y. Lau, D. W. Jordan, et al., Implications of a simple mathematical model to cancer cell population dynamics, Cell Prolif., 39 (2006), 15–28. |
[43] | D. Dingli and F. Michor, Successful therapy must eradicate cancer stem cells, Stem Cells, 4 (2006), 2603–2610. |
[44] | D. Dingli, A. Traulsen and J. M. Pacheco, Stochastic dynamics of hematopoietic tumor stem cells, Cell Cyc., 6 (2007), 461–466. |
[45] | R. Ashkenazi, T. L. Jackson, G. Dontu, et al., Breast cancer stem cells-research opportunities utilizing mathematical modeling, Stem Cell Rev., 3 (2007), 176–182. |
[46] | R. Ganguly and I. K. Puri, Mathematical model for chemotherapeutic drug efficacy in arresting tumour growth based on the cancer stem cell hypothesis, Cell Prolif., 40 (2007), 338–354. |
[47] | S. E. Kern and D. Shibata, The fuzzy math of solid tumor stem cells: a perspective, Cancer Res., 67 (2007), 8985–8988. |
[48] | H. Zhong, S. Brown, S. Devpura, et al., Kinetic modeling of tumor regression incorporating the concept of cancer stem-like cells for patients with locally advanced lung cancer, Theor. Biol. Med. Model, 15 (2018), 23. |
[49] | M. E. Sehl and M. S. Wicha, Modeling of interactions between cancer stem cells and their mi-croenvironment: Predicting clinical response, Cancer Sys. Bio. Met. Prot. (ed. L. Von Stechow), Springer New York, 2018. |
[50] | S. A. M. Tonekaboni, A. Dhawan and M. Kohandel, Mathematical modelling of plasticity and phenotype switching in cancer cell populations, Math. Biosci., 283 (2017), 30–37. |
[51] | S. Michelson and J. Leith, Autocrine and paracrine growth factors in tumor growth: A mathemat-ical model, B. Math. Biol., 53 (1991), 639–656. |
[52] | G. Ascolani and P. Liò, Modeling TGF-β in early stages of cancer tissue dynamics, PLoS ONE, 9 (2014), 1–20. |
[53] | S. Khatibi, H. J. Zhu, J. Wagner, et al., Mathematical model of TGF-β signalling: Deedback coupling is consistent with signal switching, BMC Sys. Bio., 11 (2017), 48. |
[54] | D. Krijgsman, M. Hokland and P. J. Juppen, The role of natural killer T cells in cancer-A pheno-typical and functional approach, Front. Immun., 9 (2018), 367. |
[55] | E. Vivier, D. H. Raulet, A. Moretta, et al., Innate or adaptive immunity? The example of natural killer cells, Science, 331 (2011), 44–49. |
[56] | M. Hashimoto, A. O. Kamphorst, S. J. Im, et al., CD8 T cell exhaustion in chronic infection and cancer: opportunities for interventions, Ann. Rev. Med., 69 (2018), 301–318. |
[57] | I. P. da Silva, A. Gallois, S. Jimenez-Baranda, et al., Reversal of NK-cell exhaustion in advanced melanoma by Tim-3 blockade, Cancer Immunol. Res., 2 (2014), 410–422. |
[58] | E. Bae, H. Seo, I. Kim, et al., Roles of NKT cells in cancer immunotherapy, Arch. Pharm. Res., (2019), In press. |
[59] | A. Cerwenka and L. L. Lanier, Natural killers join the fight against cancer, Science, 359 (2018), 1460–1461. |
[60] | Y. Takeuchi and H. Nishikawa, Roles of regulatory T cells in cancer immunity, Int. Immunol., 28 (2016), 401–409. |
[61] | A. Friedman, Mathematical Biology: Modeling and Analysis, Amer. Math. Monthly, 127 (2018), ISBN: 978-1-4704-4715-1. |
[62] | J. C. Arciero, T. L. Jackson and D. E. Kirschner, A mathematical model of tumor-immune evasion and siRNA treatment, Disc. Cont. Dyn. Sys. Series B, 4 (2004), 39–58. |
[63] | L. G. de Pillis, W. Gu and A. E. Radunskaya, Mixed immunotherapy and chemotherapy of tumors: modeling, applications and biological interpretations, J. Theor. Biol., 238 (2006), 841–862. |
[64] | H. Haario, M. Laine, A. Mira, et al., Efficient adaptive MCMC, Stat. Comput., 26 (2006), 339–354. |
[65] | R. B. Diasio and B. B. Harris, Clinical pharmacology of 5-fluorouracil, Clin. Pharmoacokin., 16 (1989), 215–237. |
[66] | N. Petrelli, L. Herrera, Y. Rustum, et al., A prospective randomized trial of 5-fluorouracil ver-sus 5-fluorouracil and high-dose leucovorin versus 5-fluorouracil and methotrexate in previously untreated patients with advanced colorectal carcinoma. J. Clin. Onc., 5 (1987), 1559–1565. |
[67] | I. E. Smith, R. P. A'Hern, G. A. Coombes, et al., A novel continuous infusional 5-fluorouracil-based chemotherapy regimen compared with conventional chemotherapy in the neo-adjuvant treat-ment of early breast cancer: 5 year results of the TOPIC trial. Ann. Onc., 15 (2004), 751–758. |
[68] | F. X. Caroli-Bosc, J. L. Van Laethem, P. Michel, et al., A weekly 24-h infusion of high-dose 5-fluorouracil (5-FU)+ leucovorin and bi-weekly cisplatin (CDDP) was active and well tolerated in patients with non-colon digestive carcinomas, Eur. J. Cancer, 37 (2001), 1828–1832. |
[69] | S. Marino, I. B. Hogue, C. J. Ray, et al., A methodology for performing global uncertainty and sensitivity analysis in systems biology, J. Theor. Biol., 254 (2009), 178–196. |
[70] | L. Wu, W. Blum, C. Zhu, et al., Putative cancer stem cells may be the key target to inhibit cancer cell repopulation between the intervals of chemoradiation in murine mesothelioma, BMC Cancer, 18 (2018), 471. |
[71] | H. Liu, L. Lv and K. Yang, Chemotherapy targeting cancer stem cells, Am. J. Cancer Res., 5 (2015), 880–893. |
1. | Regina Padmanabhan, Nader Meskin, Ala-Eddin Al Moustafa, 2021, Chapter 8, 978-981-15-8639-2, 157, 10.1007/978-981-15-8640-8_8 | |
2. | Yan Chen, Meng Li, Jian Cao, Guohong Cai, Xiantao Li, Yuejiao Liu, Wen Chen, CTLA-4 promotes lymphoma progression through tumor stem cell enrichment and immunosuppression, 2021, 16, 2391-5412, 909, 10.1515/biol-2021-0094 | |
3. | TUĞBA AKMAN YILDIZ, EMEK KÖSE, SAMANTHA L. ELLIOTT, MATHEMATICAL MODELING OF PANCREATIC CANCER TREATMENT WITH CANCER STEM CELLS, 2021, 29, 0218-3390, 799, 10.1142/S0218339021500182 | |
4. | Regina Padmanabhan, Nader Meskin, Ala-Eddin Al Moustafa, 2021, Chapter 2, 978-981-15-8639-2, 15, 10.1007/978-981-15-8640-8_2 | |
5. |
Ellen R. Swanson, Emek Köse, Elizabeth A. Zollinger, Samantha L. Elliott,
Mathematical Modeling of Tumor and Cancer Stem Cells Treated with CAR-T Therapy and Inhibition of TGF-β ,
2022,
84,
0092-8240,
10.1007/s11538-022-01015-5
|
|
6. | Yan Fu, Tian Lu, Meng Zhou, Dongwei Liu, Qihang Gan, Guowei Wang, Effect of color cross-correlated noise on the growth characteristics of tumor cells under immune surveillance, 2023, 20, 1551-0018, 21626, 10.3934/mbe.2023957 | |
7. | Haiwei Wang, Xinrui Wang, Liangpu Xu, Transforming growth factor-induced gene TGFBI is correlated with the prognosis and immune infiltrations of breast cancer, 2024, 22, 1477-7819, 10.1186/s12957-024-03301-z |
Name | Description | Estimate | Units | Reference |
a | Maximum rate of TGF-β production | 0.3 | days−1ng/ml | [39] |
a1 | Probability of symmetric division of a CSC | 0.01 | − | [38] |
a2 | Probability of asymmetric division of a CSC | 0.99 | − | [38] |
a3 | Probability of symmetric differentiation of a CSC | 1−a1−a2 | − | [38] |
αBR | Activation of Tregs by TGF-β | 0.07 | days−1 | [61] |
αRB | Production of TGF-β by Tregs | 10−6 | ng/ml ⋅ days−1⋅#−1 | Estimated |
b | Basal growth rate of effector cells | 0.1245 | days−1 | [62] |
c1 | TGF-β inhibitory parameter for induction of tumor death | 100 | ml/ng | [39] |
c2 | Steepness coefficient of TGF-β production | 3.036× 109 | #2 | [39] |
c3 | Magnitude of inhibition associated with tumor growth and TGF-β | 50 | ml/(ng #) | Estimated |
c4 | Activation constant half saturation | 0.5 | ng/ml | Estimated |
δB | Natural death rate of TGF-β | 0.44 | days−1 | Estimated |
δE | Natural death rate of effector cells | 0.15 | days−1 | Estimated |
δR | Natural death rate of regulatory cells | 10−5 | days−1 | [39] |
δS | Natural death rate of CSCs | 0.0005 | days−1 | [38] |
δT | Natural death rate of tumor cells | 0.886 | days−1 | Fit to [40] |
η | Proliferation rate of tumor cells | 0.997 | days−1 | Fit to [40] |
f | Tumor antigenicity | 0.62 | #−1days−1 | [39] |
γ | Decay coefficient for chemo drug | 49.9066 | days−1 | [63] |
k | Carrying capacity for tumor cells | 9.06865×108 | # | Fit to [40] |
KE | Death of effector cells due to chemo | 4.367 | days−1 | Estimated |
KR | Death of T-regulatory cells due to chemo | 4.367 | days−1 | Estimated |
KT | Death of tumor cells due to chemo | 8.734 | days−1 | Fit to [40] |
μES | Interactions of CSCs and effector cells leading to CSC death | 10−9 | (#∗time)−1 | Estimated |
μET | Effector T-cell induced tumor death rate/removal rate | 10−5 | #−1days−1 | [39] |
μRE | Regulatory T-cell induced effector cell death rate/removal rate | 10−5 | #−1days−1 | [39] |
νb(t) | Chemotherapy dosage concentration | Variable | mg/kg | [40] |
r | Rate at which effector cells become regulatory cells | 0.01 | days−1 | [39] |
Name | Description | Estimate | Units | Reference |
a | Maximum rate of TGF-β production | 0.3 | days−1ng/ml | [39] |
a1 | Probability of symmetric division of a CSC | 0.01 | − | [38] |
a2 | Probability of asymmetric division of a CSC | 0.99 | − | [38] |
a3 | Probability of symmetric differentiation of a CSC | 1−a1−a2 | − | [38] |
αBR | Activation of Tregs by TGF-β | 0.07 | days−1 | [61] |
αRB | Production of TGF-β by Tregs | 10−6 | ng/ml ⋅ days−1⋅#−1 | Estimated |
b | Basal growth rate of effector cells | 0.1245 | days−1 | [62] |
c1 | TGF-β inhibitory parameter for induction of tumor death | 100 | ml/ng | [39] |
c2 | Steepness coefficient of TGF-β production | 3.036× 109 | #2 | [39] |
c3 | Magnitude of inhibition associated with tumor growth and TGF-β | 50 | ml/(ng #) | Estimated |
c4 | Activation constant half saturation | 0.5 | ng/ml | Estimated |
δB | Natural death rate of TGF-β | 0.44 | days−1 | Estimated |
δE | Natural death rate of effector cells | 0.15 | days−1 | Estimated |
δR | Natural death rate of regulatory cells | 10−5 | days−1 | [39] |
δS | Natural death rate of CSCs | 0.0005 | days−1 | [38] |
δT | Natural death rate of tumor cells | 0.886 | days−1 | Fit to [40] |
η | Proliferation rate of tumor cells | 0.997 | days−1 | Fit to [40] |
f | Tumor antigenicity | 0.62 | #−1days−1 | [39] |
γ | Decay coefficient for chemo drug | 49.9066 | days−1 | [63] |
k | Carrying capacity for tumor cells | 9.06865×108 | # | Fit to [40] |
KE | Death of effector cells due to chemo | 4.367 | days−1 | Estimated |
KR | Death of T-regulatory cells due to chemo | 4.367 | days−1 | Estimated |
KT | Death of tumor cells due to chemo | 8.734 | days−1 | Fit to [40] |
μES | Interactions of CSCs and effector cells leading to CSC death | 10−9 | (#∗time)−1 | Estimated |
μET | Effector T-cell induced tumor death rate/removal rate | 10−5 | #−1days−1 | [39] |
μRE | Regulatory T-cell induced effector cell death rate/removal rate | 10−5 | #−1days−1 | [39] |
νb(t) | Chemotherapy dosage concentration | Variable | mg/kg | [40] |
r | Rate at which effector cells become regulatory cells | 0.01 | days−1 | [39] |