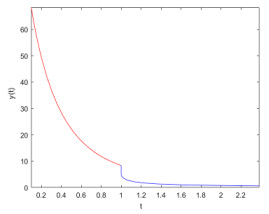
Neutrophils play a crucial role in the innate immune response as a first line of defense in many diseases, including cancer. Tumor-associated neutrophils (TANs) can either promote or inhibit tumor growth in various steps of cancer progression via mutual interactions with cancer cells in a complex tumor microenvironment (TME). In this study, we developed and analyzed mathematical models to investigate the role of natural killer cells (NK cells) and the dynamic transition between N1 and N2 TAN phenotypes in killing cancer cells through key signaling networks and how adjuvant therapy with radiation can be used in combination to increase anti-tumor efficacy. We examined the complex immune-tumor dynamics among N1/N2 TANs, NK cells, and tumor cells, communicating through key extracellular mediators (Transforming growth factor (TGF-β), Interferon gamma (IFN-γ)) and intracellular regulation in the apoptosis signaling network. We developed several tumor prevention strategies to eradicate tumors, including combination (IFN-γ, exogenous NK, TGF-β inhibitor) therapy and optimally-controlled ionizing radiation in a complex TME. Using this model, we investigated the fundamental mechanism of radiation-induced changes in the TME and the impact of internal and external immune composition on the tumor cell fate and their response to different treatment schedules.
Citation: Donggu Lee, Sunju Oh, Sean Lawler, Yangjin Kim. Bistable dynamics of TAN-NK cells in tumor growth and control of radiotherapy-induced neutropenia in lung cancer treatment[J]. Mathematical Biosciences and Engineering, 2025, 22(4): 744-809. doi: 10.3934/mbe.2025028
[1] | Deniz Sevinç . Volatility spillovers among MIST stock markets. Data Science in Finance and Economics, 2022, 2(2): 80-95. doi: 10.3934/DSFE.2022004 |
[2] | Nurun Najwa Bahari, Hafizah Bahaludin, Munira Ismail, Fatimah Abdul Razak . Network, correlation, and community structure of the financial sector of Bursa Malaysia before, during, and after COVID-19. Data Science in Finance and Economics, 2024, 4(3): 362-387. doi: 10.3934/DSFE.2024016 |
[3] | Moses Khumalo, Hopolang Mashele, Modisane Seitshiro . Quantification of the stock market value at risk by using FIAPARCH, HYGARCH and FIGARCH models. Data Science in Finance and Economics, 2023, 3(4): 380-400. doi: 10.3934/DSFE.2023022 |
[4] | Jiamin Cheng, Yuanying Jiang . How can carbon markets drive the development of renewable energy sector? Empirical evidence from China. Data Science in Finance and Economics, 2024, 4(2): 249-269. doi: 10.3934/DSFE.2024010 |
[5] | Kuo-Shing Chen . Interlinkages between Bitcoin, green financial assets, oil, and emerging stock markets. Data Science in Finance and Economics, 2024, 4(1): 160-187. doi: 10.3934/DSFE.2024006 |
[6] | Dachen Sheng, Opale Guyot . Market power, internal and external monitoring, and firm distress in the Chinese market. Data Science in Finance and Economics, 2024, 4(2): 285-308. doi: 10.3934/DSFE.2024012 |
[7] | Zimei Huang, Zhenghui Li . What reflects investor sentiment? Empirical evidence from China. Data Science in Finance and Economics, 2021, 1(3): 235-252. doi: 10.3934/DSFE.2021013 |
[8] | Katleho Makatjane, Ntebogang Moroke . Examining stylized facts and trends of FTSE/JSE TOP40: a parametric and Non-Parametric approach. Data Science in Finance and Economics, 2022, 2(3): 294-320. doi: 10.3934/DSFE.2022015 |
[9] | Samuel Asante Gyamerah, Collins Abaitey . Modelling and forecasting the volatility of bitcoin futures: the role of distributional assumption in GARCH models. Data Science in Finance and Economics, 2022, 2(3): 321-334. doi: 10.3934/DSFE.2022016 |
[10] | Nitesha Dwarika . The risk-return relationship in South Africa: tail optimization of the GARCH-M approach. Data Science in Finance and Economics, 2022, 2(4): 391-415. doi: 10.3934/DSFE.2022020 |
Neutrophils play a crucial role in the innate immune response as a first line of defense in many diseases, including cancer. Tumor-associated neutrophils (TANs) can either promote or inhibit tumor growth in various steps of cancer progression via mutual interactions with cancer cells in a complex tumor microenvironment (TME). In this study, we developed and analyzed mathematical models to investigate the role of natural killer cells (NK cells) and the dynamic transition between N1 and N2 TAN phenotypes in killing cancer cells through key signaling networks and how adjuvant therapy with radiation can be used in combination to increase anti-tumor efficacy. We examined the complex immune-tumor dynamics among N1/N2 TANs, NK cells, and tumor cells, communicating through key extracellular mediators (Transforming growth factor (TGF-β), Interferon gamma (IFN-γ)) and intracellular regulation in the apoptosis signaling network. We developed several tumor prevention strategies to eradicate tumors, including combination (IFN-γ, exogenous NK, TGF-β inhibitor) therapy and optimally-controlled ionizing radiation in a complex TME. Using this model, we investigated the fundamental mechanism of radiation-induced changes in the TME and the impact of internal and external immune composition on the tumor cell fate and their response to different treatment schedules.
When searching the literature, we noticed that several propositions have been made by several researchers to find kernels that can be used to obtain fractional differential operators. The main reason for this is that, real world problems show signs of processes resembling behaviors of some mathematical functions. Riemann, Liouville, Cauchy and Abel works have led to a fractional calculus with a power law kernel. Their work was later modified by Caputo; this version has been used in many fields of science because of its ability to allow classical initial conditions [1]. Prabhakar suggested a different kernel as a product of power-law and the generalized Mittag-Leffler function with three parameters. This version has also attracted the attention of many researchers, studies have been done on theory as well as on applications. Indeed, the two kernels have their specific values, for example, power-law helps only to replicate processes exhibiting power-law behavior, while the product of the power-law and the generalized three-parameters Mittag-Leffler has also its field of application [2]. As nature is complex, a new kernel was suggested by Caputo and Fabrizio, a special exponential kernel with Delta Dirac properties. A differential operator that is in fashion nowadays due to its ability to replicate processes following fading memory. Indeed, this kernel brought a new shift into fractional calculus, as the concept of the fractional derivative with a non-singular kernel was introduced [3]. A point made by some researcher about the non-fractionality of the kernel led to a new kernel, the generalized Mittag-Leffler function with one parameter. This version was suggested by Atangana and Baleanu, constituting another new step forward in the field of fractional calculus. The operators have been used in several fields of studies with great success [4]. In [5], the basic theory of fractional differentiation, existence-uniqueness theorems and analytical numerical methods of solution of fractional differential equations are presented. In [6], the authors examined Noether's theorems of fractional generalized Birkhoffian systems in terms of classical and combined Caputo derivatives. The development of the time-fractional damage model for hyperelastic body is considered in [7]. While looking at nature and its complexities, one can with no doubt conclude that these suggested kernels are not enough to predict all complex behaviors of our universe. On this note, one will proceed to search for a different kernel or modified kernel, or a class of functions that will be used to introduce new differential operators. Sabatier recently presented some variants of kernels that will also open new doors for investigation [8]. In addition to these outstanding contributions, several other ideas have been suggested, for example the concept of short memory was suggested and a fractional derivative in the Caputo sense has been defined for different values of fractional orders. The idea is to have a different type of variable-order derivative unlike the well-known version that considers a fractional orders. The idea is initiated to have a different type of variable order derivative unlike the well-known version that considers a fractional-order to be a function of time. This case was suggested by Wu et al. [9] and applied in chaos. On the other hand, researchers have noticed that several real-world problems exhibit processes with different behaviors as a function of time and space. A particular case is a passage from deterministic to stochastic, or from power law to exponential decay. It was noted that existing differential operators may not be able to account for these behaviors, thus piecewise differential and integral operators were introduced to deal with problems exhibiting crossover behaviors [10]. The main aim of this note is to provide a critical analysis of the possible applications, advantages, and disadvantages of these two concepts.
We will illustrate the motivation with some examples.
Death body decay in different temperatures. Consider a corpse found in a snowy place, and assume that such body has been found after 20 days. The corpse is taken and brought to the house and kept in a normal temperature for a few days and later put in a mortuary and buried. The main aim here is to replicate the process of decay. The first part will provide a very low decay. The second part will provide a fast decay, the third part will again be a fast process and finally, a slow process. Indeed, slow and fast processes can be characterized by some mathematical functions. The power-law function is
t−α. | (2.1) |
The exponential decay function with a Dirac delta property is
11−αexp[−α1−αt]. | (2.2) |
However, a crossover from fast to slow decay processes can be modeled using the following Mittag-Leffler function with Delta-Dirac property
11−αEα[−α1−αtα]. | (2.3) |
The process can be divided into several intervals to capture each behavior. In the first part, one can have
C0Dαty(t)=−λ1y(t)if0<t≤T1. | (2.4) |
The second processes can be
CFT1Dαty(t)=−λ2y(t)ifT1<t≤T2. | (2.5) |
The two last parts will be characterized by
ABCT2Dαty(t)=−λ3y(t)ifT2<t≤T. | (2.6) |
Thus the whole process will be a system with the following crossover behaviors
{C0Dαty(t)=−λ1y(t)if0<t≤T1,CFT1Dαty(t)=−λ2y(t)ifT1<t≤T2,ABCT2Dαty(t)=−λ3y(t)ifT2<t≤T. | (2.7) |
Therefore in general the concept of piecewise derivative has been introduced.
In this section, we present the definitions of both short memory and piecewise differentiation. For the short memory case, the idea has already been discussed; for example a paper published by Deng in 2007 has already discussed the short memory principle [11], which has also been called the fixed memory principle and logarithmic memory principle. Wu et al. [9] presented the concept of a fractional variable-order derivative where the fractional order changes within an interval. The suggested definition is given below as:
{Ct0Dα0ty(t)=f(y,t) for t∈[t0,t1],Ct1Dα1ty(t)=f(y,t) for t∈[t1,t2],Ct2Dα2ty(t)=f(y,t) for t∈[t2,t3]. | (3.1) |
Here Caputo power law derivative is used, which is known to have a singularity at the origin for a class of functions. The author did not give explicitly space of the functions as the derivative was defined in general, therefore, if we assume a class of the functions for which dydt is continuous then at each t=ti, f(y,t) should be zero.
On the other hand, the piecewise concept is defined as follows.
1) A piecewise derivative of f with classical, power-law and stochastic processes is given by
{Dy(t)=f(t,y(t))if0<t≤t1,Ct1Dαty(t)=f(t,y(t))ift1<t≤t2,dy(t)=f(t,y(t))dt+σy(t)dB(t)ift2<t≤t3. | (3.2) |
2) A piecewise derivative of f with power, exponential and Mittag-Leffler laws is given as
{C0Dαty(t)=f(t,y(t))if0<t≤t1,CFt1Dαty(t)=f(t,y(t))ift1<t≤t2,ABCt1Dαty(t)=f(t,y(t))ift2<t≤t3. | (3.3) |
Several definitions can be found in [10].
In the next section, we will provide a deep analysis of these concepts.The aim of the section is to present advantages and disadvantages of these two independent concepts. We note that
C0Dαtf(t)=1Γ(1−α)t∫0ddτf(τ)(t−τ)−αdτ, | (3.4) |
CF0Dαtf(t)=11−αt∫0ddτf(τ)exp[−α1−α(t−τ)]dτ, | (3.5) |
ABC0Dαtf(t)=11−αt∫0ddτf(τ)Eα[−α1−α(t−τ)α]dτ. | (3.6) |
Here, we present a deep analysis that will help the readers to see the difference between the two concepts. Let us start with short memory. A deep look inside the short memory concept defined by gives the following:
1) The short memory principle considers a change in constant variable order; however, it uses a single kernel. Indeed, this can be viewed as variable order where the order is changed within shorter intervals. Nonetheless, it describes some process and in the case of Caputo derivation, this process will only describe a power-law process that is, this process will not have crossover behavior.
2) The concept is unable to capture classical processes because the fractional order should change in each interval.
On a serious note, however, if we have f(t,y(t))≠0,∀t∈[0,T]. So at each tj
limt→tjCtjDαty(t)=f(t,y(t))∣t=tj. | (4.1) |
Sincedydt is continuous,
limt→tjCtjDαty(t)=f(tj,y(tj))≠0. | (4.2) |
However the right hand side produces f(t,y(t))≠0, which is a contradiction. It infers that f(tj,y(tj))=0. This may be used to explain the process with a renewal force that follows the power-law process. For example, the trajectory of a flea with a constant jump. There are fewest real world problems that present these behaviors. However, due to the power-law singularity the definition suggested by Wu may have some problems at the boundaries. Nonetheless, as a result of using non-singular kernels, the concept of short memory will be well-posed since the conditions at the boundaries will be well controlled and the renewal processes would be well-posed
On the other hand, however, the concept of piecewise was introduced for different purposes. The following example will give light to the situation. We consider evaluating the velocity of water within a geological formation with fractures. We record the velocity as a function of time. The velocity will obviously be low in the rock's matrix; however as the water reaches the fracture, there will be a crossover behavior because the velocity will suddenly increase. The first part of this process follows behaviors resembling a declining process and, later, an almost constant high velocity. Now if one was to withdraw water from such a geological formation and record the water level, one will observe a fading groundwater level in earlier times; then when water is being taken from the fracture, we observe a steady groundwater level. Thus, there is a crossover behavior from the fading process to long range, which can be captured using an exponential function, then the power law. Therefore, the differential operator able to replicate this process is
{C0Dαth(r,t)=f(t,r,h(r,t))if0<t≤T1,CFT1Dαth(r,t)=f1(t,r,h(r,t))ifT1<t≤T. | (4.3) |
Therefore, one can see a clear difference and objectives of both concepts. Piecewise differential operators are thus conceived to capture processes exhibiting different patterns, or crossover behaviors. Here the order does not change within an interval as changing order does not change the process but only the memory with the same pattern, rather, the kernels change to bring into the mathematical formulation the crossover behaviors by each kernel.
For example, using the piecewise derivative the decay equation
{y′(t)=−λy(t)if0<t≤T1,CT1Dαty(t)=−λy(t)ifT1<t≤T2,dy(t)=−λy(t)dt+σy(t)dB(t)ifT2<t≤T | (4.4) |
which will lead to decay with crossover behaviors. From 0<t<T1, we shall observe a normal decay process. From T1<t<T2, we shall observe power-law decay or the Mittag-Leffler decay. From T2<t<T, we shall observe decay with randomness. On the other hand, with the short memory
{C0Dα1ty(t)=−λy(t)if0<t≤T1,CT1Dα2ty(t)=−λy(t)ifT1<t≤T2,CT2Dα3ty(t)=−λy(t)ifT2<t≤T | (4.5) |
which leads only to a power-law process or the Mittag-Leffler process.
In this section, we shall present clearly the difference between the two concepts by applying them to some problems. We shall start with a simple problem about the decay problem with two intervals.
Example 1. We consider a simple decay model within [0,T1] and [T1,T2]. In the case of short memory, we have
{C0Dα1ty(t)=−λy(t)ift∈[0,T1],CT1Dα2ty(t)=−λy(t)ift∈[T1,T2]. | (5.1) |
In the case of piecewise, we can consider the following
{dy(t)dt=−λy(t)ift∈[0,T1],CT1Dαty(t)=−λy(t)ift∈[T1,T2] | (5.2) |
or
{ABC0Dαty(t)=−λy(t)ift∈[0,T1],dy(t)dt=−λy(t)ift∈[T1,T2] | (5.3) |
or
{CF0Dαty(t)=−λy(t)ift∈[0,T1],CT1Dαty(t)=−λy(t)ift∈[T1,T2]. | (5.4) |
Using the Laplace transform on the above system yields in the case of short memory
{y(t)=y(0)Eα1[−λtα1]ift∈[0,T1],y(t)=y(T1)Eα2[−λ(t−T1)α2]ift∈[T1,T2]. | (5.5) |
In the case of piecewise, we have the following solution
{y(t)=y(0)exp[−λt]ift∈[0,T1],y(t)=y(T1)Eα[−λ(t−T1)α]ift∈[T1,T2] | (5.6) |
or
{y(t)=y(0)ift=0,y(t)=y(0)1+λ(1−α)Eα[−λα1+λ(1−α)tα]bift∈]0,T1],y(t)=y(T1)exp[−λ(t−T1)]ift∈[T1,T2] | (5.7) |
or
{y(t)=y(0)ift=0,y(t)=y(0)1+λ(1−α)exp[−λα1+λ(1−α)tα]bift∈]0,T1],y(t)=y(T1)Eα[−λ(t−T1)α]ift∈[T1,T2]. | (5.8) |
T1=1 where the crossover occurs and T2=2.4 is the final time. The numerical simulations are presented in Figures 1–4 below.
Example 2. We consider the spread of an infectious disease. We consider a Susceptible, Exposed, Infected and Recovered (SEIR) model. It was proven by Atangana and Seda that such a model may not be able to predict waves [12]. Thus to introduce waves, in particular two waves, we consider the spread to be in the periods [0,T1] and [T1,T2]. In the case of short memory, we have
{C0DαtS=μN−μS−βSINC0DαtE=βSIN−(μ+a)EC0DαtI=aE−(γ+μ)IC0DαtR=γI−μRift∈[0,T1],{CT1DβtS=μN−μS−βSINCT1DβtE=βSIN−(μ+a)ECT1DβtI=aE−(γ+μ)ICT1DβtR=γI−μRift∈[T1,T2]. | (5.9) |
In the case of piecewise several scenarios can be obtained. So we can have
{⋅S=μN−μS−βSIN⋅E=βSIN−(μ+a)E⋅I=aE−(γ+μ)I⋅R=γI−μRift∈[0,T1],{dS=(μN−μS−βSIN)dt+σ1SdB1(t)dE=(βSIN−(μ+a)E)dt+σ2EdB2(t)dI=(aE−(γ+μ)I)dt+σ3IdB3(t)dR=(γI−μR)dt+σ4RdB4(t)ift∈[T1,T2] | (5.10) |
or
{dS=(μN−μS−βSIN)dt+σ1SdB1(t)dE=(βSIN−(μ+a)E)dt+σ2EdB2(t)dI=(aE−(γ+μ)I)dt+σ3IdB3(t)dR=(γI−μR)dt+σ4RdB4(t)ift∈[0,T1],{CT1DαtS=μN−μS−βSINCT1DαtE=βSIN−(μ+a)ECT1DαtI=aE−(γ+μ)ICT1DαtR=γI−μRift∈[T1,T2]. | (5.11) |
Noting that several more scenarios can be considered, however, we will only consider these two in the case of piecewise. Before proceeding with the analysis of these models, we shall first provide an interpolation of each case in terms of wave behaviors. In the case of short memory, the first and second waves show the non-Gaussian distribution associated to the power-law variables t−α1 and t−α2. Therefore, one will observe different long tails for t−α1 and t−α2. On the other hand, however, the first considered model shows that the first wave has a normal distribution, while the second wave has random behavior. The second model shows that the first wave has a long tail spread while the second has random behavior.
We now present the numerical solutions of the considered models. We shall consider the numerical scheme suggested by Ghanbari et al. [13] for fractional cases. The short memory case can be simplified to
{C0Dαtyi(t)=Fi(t,yi(t))ift∈[0,T1],CT1Dβtyi(t)=Fi(t,yi(t))ift∈[T1,T2]. | (5.12) |
Applying the Ghanbari method yields
{yn1i=yi(0)+hα(˜a(α)n1Fi(t0,yi(t0))+n1∑k=2˜a(α)n1−kFi(tk,yi(tk)))ift∈[0,T1],yn2i=yi(T1)+hβ(˜a(β)n2Fi(T1,yi(T1))+n2∑j=n1+2a(β)n2−jFi(tj,yi(tj)))ift∈[T1,T2] | (5.13) |
where
˜a(α)n1=(n1−1)α+1−nα1(n1−α−1)Γ(α+2),a(β)n2={1Γ(β+2)ifn=0,(n2−1)β+1−2nβ+12+(n2+1)β+1Γ(β+2)ifn≥1. | (5.14) |
and
˜a(β)n2=(n2−1)β+1−nβ2(n2−β−1)Γ(β+2),θ(β)k=(k−1)β+1−2kβ+1+(k+1)β+1Γ(β+2) if k=n1+1,,..,n2−1. | (5.15) |
In the case of the piecewise first model, we have
{yn1i=yi(0)+∑n1j=2[2312Fi(tj,yi(tj))Δt−43Fi(tj−1,yi(tj−1))Δt+512Fi(tj−2,yi(tj−2))Δt] if t∈[0,T1],{yn2i=y(T1)+∑n2j=n1+3[2312Fi(tj,yi(tj))Δt−43Fi(tj−1,yi(tj−1))Δt+512Fi(tj−2,yi(tj−2))Δt] if t∈[T1,T2].+σiyi(Bn2+1i−Bn2i) | (5.16) |
For Case 2, we obtain
{{yn1i=yi(0)+∑n1j=2[2312Fi(tj,yi(tj))Δt−43Fi(tj−1,yi(tj−1))Δt+512Fi(tj−2,yi(tj−2))Δt]+σi∑n1j=1yj(Bji−Bj−1i)ift∈[0,T1],yn2i=yi(T1)+hα(˜a(α)n1Fi(tn1,yi(tn1))+∑n2k=0˜a(α)n1+kFi(tk,yi(tk)))ift∈[T1,T2]. | (5.17) |
The numerical solutions for short memory are depicted in Figure 5 with the following parameters
N=1000,μ=0.01,β=0.6,a=0.2,γ=0.03, | (5.18) |
and the following initial conditions
S(0)=1000,E(0)=25,I(50)=22,R(50)=5,S(50)=39E(50)=67,I(50)=505,R(50)=390 | (5.19) |
The numerical solutions for Cases 1 and 2 are respectively depicted in Figures 6 and 7 with the following parameters
N=1000,μ=0.01,β=0.6,a=0.2,γ=0.03, | (5.20) |
and the following initial conditions
S(0)=1000,E(0)=25,I(50)=22,R(50)=5,S(50)=35E(50)=44,I(50)=424,R(50)=527,α=0.7 | (5.21) |
Figure 5 demonstrates the short memory effect, where the first and second parts present different long tails behaviors characterized by α1 and α2; however, both clearly depict the same process which is the power law process. On the other hand, Figures 6 and 7 show the clear effects of the piecewise differential operators; the first part in Figure 6 shows normal distribution, which is characteristic of the classical differential operator, while the second part clearly shows the effect of randomness. The complete model therefore shows crossover behavior from deterministic to stochastic with no steady state. In Figure 6, the process is different. We start with randomness then end up with long tail behaviors as a result of the power law kernel.
Example 3. We now consider a chaotic problem in particular the 8-wing attractor [14]. This model has been studied by several researchers [15], the model is given as
⋅x=a(y−x)+f(t)yz⋅y=cx+dy−xz⋅z=−bz+xy | (5.22) |
where the function f(t)=M sgn(sin(wt))+K. The constant w is known to be a switch frequency and M and K are constant parameters.
In the case of short memory, we have
{C0Dα1tx=a(y−x)+f(t)yzC0Dα1ty=cx+dy−xzC0Dα1tz=−bz+xyift∈[0,T1],{CT1Dα2tx=a(y−x)+f(t)yzCT1Dα2ty=cx+dy−xzCT1Dα2tz=−bz+xyift∈[T1,T2]. | (5.23) |
In the case of piecewise, we can consider three cases. For Case 1, we can write
{ABC0Dαtx=a(y−x)+f(t)yzABC0Dαty=cx+dy−xzABC0Dαtz=−bz+xyift∈[0,T1],{dx=(a(y−x)+f(t)yz)dt+σ1xdB1(t)dy=(cx+dy−xz)dt+σ2ydB2(t)dz=(−bz+xy)dt+σ3zdB3(t)ift∈[T1,T2]. | (5.24) |
For Case 2, we have
{C0Dαtx=a(y−x)+f(t)yzC0Dαty=cx+dy−xzC0Dαtz=−bz+xyift∈[0,T1],{dx=(a(y−x)+f(t)yz)dt+σ1xdB1(t)dy=(cx+dy−xz)dt+σ2ydB2(t)dz=(−bz+xy)dt+σ3zdB3(t)ift∈[T1,T2]. | (5.25) |
For Case 3, we can get
{⋅x=a(y−x)+f(t)yz⋅y=cx+dy−xz⋅z=−bz+xyift∈[0,T1],{dx=(a(y−x)+f(t)yz)dt+σ1xdB1(t)dy=(cx+dy−xz)dt+σ2ydB2(t)dz=(−bz+xy)dt+σ3zdB3(t)ift∈[T1,T2]. | (5.26) |
We shall now present the numerical solution of each case. In the case of short memory, one can use the numerical method based on Lagrange interpolation [16]. We can first simplify the model as follows.
{C0Dα1tMi(t)=Π(t,Mi(t))ift∈[0,T1],CT1Dα2tMi(t)=Π(t,Mi(t))ift∈[T1,T2]. | (5.27) |
Thus, applying such method to the above system yields
{Mn1i=Mi(0)+[(Δt)α1Γ(α1+2)n1∑q=0Π(tq,Mqi)×[(n1−q+1)α1(n1−q+2+α1)−(n1−q)α1(n1−q+2+2α1)]−(Δt)α1Γ(α1+2)n1∑q=1Π(tq−1,Mq−1i)×[(n1−q+1)α1+1−(n1−q)α1(n1−q+1+α1)]]ift∈[0,T1],Mn2i=Mi(T1)+[(Δt)α2Γ(α2+2)n2∑k=n1+1Π(tk,Mki)×[(n2−q+1)α2(n2−q+2+α2)−(n2−q)α2(n2−q+2+2α2)]−(Δt)α2Γ(α2+2)n2∑k=n1+2Π(tk−1,Mk−1i)×[(n2−q+1)α2+1−(n2−q)α2(n2−q+1+α2)]]ift∈[T1,T2]. | (5.28) |
With the piecewise case, we obtain the following numerical solution by using a scheme based on Newton polynomial interpolation [17]. Also, we simplify the model as follows.
{ABC0Dα1tMi(t)=Π(t,Mi(t))ift∈[0,T1],dMi(t)=Π(t,Mi(t))dt+σiMidBi(t)ift∈[T1,T2]. | (5.29) |
Then applying this method yields
Mn1i=Mi(0)+1−αAB(α)Π(tn1,Mn1i)+α(Δt)αAB(α)Γ(α+1)n1∑k=2Π(tk−2,Mk−2i)×{(n1−k+1)α−(n1−k)α}+α(Δt)αAB(α)Γ(α+2)n1∑k=2[Π(tk−1,Mk−1i)−Π(tk−2,Mk−2i)]×{(n1−k+1)α(n1−k+3+2α)−(n1−k)α(n1−k+3+3α)}+α(Δt)α2AB(α)Γ(α+3)n1∑k=2[Π(tk,Mki)−2Π(tk−1,Mk−1i)+Π(tk−2,Mk−2i)]×{(n1−k+1)α[2(n1−k)2+(3α+10)(n1−k)+2α2+9α+12]−(n1−k)α[2(n1−k)2+(5α+10)(n1−k)+6α2+18α+12]},0≤t≤T1Mn2+4i=Mn2+3i+n3∑j3=n1+3[2312Π(tn2+3,Mn2+3i)−43Π(tn2+2,Mn2+2i)+512Π(tn2+1,Mn2+1i)]Δt+σiMn2i(Bn2+1i−Bn2+1i),T1≤t≤T2 | (5.30) |
For Case 2, we again apply the scheme with the Newton polynomial to obtain
{Mn1i=Mi(0)+(Δt)αΓ(α+1)n1∑k=2Π(tk−2,Mk−2i)×{(n1−k+1)α−(n1−k)α}+(Δt)αΓ(α+2)n1∑k=2[Π(tk−1,Mk−1i)−Π(tk−2,Mk−2i)],×{(n1−k+1)α(n1−k+3+2α)−(n1−k)α(n1−k+3+3α)}+(Δt)α2Γ(α+3)n1∑k=2[Π(tk,Mki)−2Π(tk−1,Mk−1i)+Π(tk−2,Mk−2i)]×{(n1−k+1)α[2(n1−k)2+(3α+10)(n1−k)+2α2+9α+12]−(n1−k)α[2(n1−k)2+(5α+10)(n1−k)+6α2+18α+12]},0≤t≤T1Mn2+4i=Mn2+3i+n3∑j3=n2+3[2312Π(tn2+3,Mn2+3i)−43Π(tn2+2,Mn2+2i)+512Π(tn2+1,Mn2+1i)]Δt+σiMi(Cn2)(Bn2+1i−Bn2+1i),T1≤t≤T2 | (5.31) |
where Cn2∈[tn2+4,tn2+3]. For Case 3, we use the simple Adams-Bashforth methods as follows
{Mn2+3i=Mn2+2i+[2312Π(tn2+2,Mn2+2i)−43Π(tn2+1,Mn2+1i)+512Π(tn2,Mn2i)]Δt,0≤t≤T1Mn2+4i=Mn2+3i+[2312Π(tn2+3,Mn2+3i)−43Π(tn2+2,Mn2+2i)+512Π(tn2+1,Mn2+1i)]Δt+σiMi(Cn2)(Bn2+1i−Bn2i),T1≤t≤T2 | (5.32) |
where Cn2∈[tn2+4,tn2+3].
Numerical solutions are obtained for the following parameters
a=14,b=43,c=−1,d=16,M=9,K=10,w=2π50. | (5.33) |
The numerical solutions for short memory are depicted in Figure 8 for the initial data x(0)=22.3,y(0)=4.9 and z(0)=2.6.
For piecewise, we consider the following stochastic-deterministic problem with power-law and Mittag-Leffler kernels:
{C0Dαtx=a(y−x)+f(t)yz+σ1xB′1(t)C0Dαty=cx+dy−xz+σ2yB′2(t)C0Dαtz=−bz+xy+σ3zB′3(t)ift∈[0,T1],{ABCT1Dαtx=a(y−x)+f(t)yzABCT1Dαty=cx+dy−xzABCT1Dαtz=−bz+xyift∈[T1,T2] | (5.34) |
with the stochastic constants
σ1=0.031;σ2=0.035;σ3=0.032. | (5.35) |
The numerical simulation for such a problem can be performed as illustrated in Figure 9 by using the same initial data and parameters.
For piecewise, we consider the following stochastic-deterministic problem with power-law and Mittag-Leffler kernels:
{ABC0Dαtx=a(y−x)+f(t)yz+σ1xB′1(t)ABC0Dαty=cx+dy−xz+σ2yB′2(t)ABC0Dαtz=−bz+xy+σ3zB′3(t)ift∈[0,T1],{CT1Dαtx=a(y−x)+f(t)yzCT1Dαty=cx+dy−xzCT1Dαtz=−bz+xyift∈[T1,T2] | (5.36) |
with the following stochastic constants
σ1=0.031;σ2=0.035;σ3=0.032. | (5.37) |
The numerical simulation for such a problem can be performed as shown in Figure 10 by using the same initial data and parameters.
Also, we consider the following deterministic-stochastic problem with power-law and Mittag-Leffler kernels:
{C0Dαtx=a(y−x)+f(t)yzC0Dαty=cx+dy−xzC0Dαtz=−bz+xyift∈[0,T1],{ABCT1Dαtx=a(y−x)+f(t)yz+σ1xB′1(t)ABCT1Dαty=cx+dy−xz+σ2yB′2(t)ABCT1Dαtz=−bz+xy+σ3zB′3(t)ift∈[T1,T2] | (5.38) |
with the following stochastic constants:
σ1=0.02;σ2=0.012;σ3=0.021. | (5.39) |
The numerical solutions for the above problem can be depicted as shown in Figure 11 using same initial data and parameters.
Figure 7 shows the results obtained by applying the power-law short memory concept. To show the difference within the interval, we have opted to consider the first part of the interval to be in blue and the second to be in red. However, a quick look at the results shows clearly the effect of a power law, no great change from one interval to another is observed since both depict long-tail behaviors as results of the power-law kernel. In this case, there is no crossover behavior, just a repetition of different long tail behaviors. On the other hand, in Figures 8 and 9, there is a clear change in patterns where the first patterns show deterministic behaviors in particular, long-tails due to power law in Figure 8, and the second part shows randomness, there is, therefore, a clear crossover from power law to randomness. While, in Figure 9, we have two crossovers, the first is due to the Mittag-Leffler kernel that shows a change from stretched exponential to power law and the second is stochastic.
Also, we consider deterministic-stochastic problem with power-law and Mittag-Leffler kernel
{ABC0Dαtx=a(y−x)+f(t)yzABC0Dαty=cx+dy−xzABC0Dαtz=−bz+xyift∈[0,T1],{CT1Dαtx=a(y−x)+f(t)yz+σ1xB′1(t)CT1Dαty=cx+dy−xz+σ2yB′2(t)CT1Dαtz=−bz+xy+σ3zB′3(t)ift∈[T1,T2] | (5.40) |
with the following stochastic constants:
σ1=0.02;σ2=0.012;σ3=0.021. | (5.41) |
The numerical solutions for the above problem can be depicted as shown in Figure 12 by using the same initial data and parameters.
In the last decades, researchers have devoted their attention to better understanding complex realworld problems even on a small scale. They have therefore developed several methods, different differential, and integral operators. To understand the process by which different long tails occur in different intervals, the concept of short memory was introduced. This concept defines power-law derivatives in different intervals and each interval has its own order. This order accounts for the long tail associated with that interval. However, the process is scale-invariant in terms of patterns. On the other hand, because there are many real-world problems that exhibit passages from one process to another, for example, a passage from power law to randomness, the concept of piecewise was introduced. In this work, a detailed analysis was given to show their different fields of applications and show also, when short memory and piecewise concepts can be applied. This paper thus helps researchers to identify what problem is suitable for short memory and piecewise.
The authors declare there is no conflict of interest.
[1] |
H. Sung, J. Ferlay, R. Siegel, M. Laversanne, I. Soerjomataram, A. Jemal, et al., Global cancer statistics 2020: GLOBOCAN estimates of incidence and mortality worldwide for 36 cancers in 185 countries, CA Cancer J. Clin., 71 (2021), 209–249. https://doi.org/10.3322/caac.21660 doi: 10.3322/caac.21660
![]() |
[2] |
J. Molina, P. Yang, S. Cassivi, S. Schild, A. Adjei, Non-small cell lung cancer: epidemiology, risk factors, treatment, and survivorship, Mayo Clin. Proc., 83 (2008), 584–594. https://doi.org/10.4065/83.5.584 doi: 10.4065/83.5.584
![]() |
[3] |
N. Altorki, G. Markowitz, D. Gao, J. Port, A. Saxena, B. Stiles, et al., The lung microenvironment: an important regulator of tumour growth and metastasis, Nat. Rev. Cancer, 19 (2019), 9–31. https://doi.org/10.1038/s41568-018-0081-9 doi: 10.1038/s41568-018-0081-9
![]() |
[4] |
X. Yan, S. Jiao, G. Zhang, Y. Guan, J. Wang, Tumor-associated immune factors are associated with recurrence and metastasis in non-small cell lung cancer, Cancer Gene Ther., 24 (2017), 57–63. https://doi.org/10.1038/cgt.2016.40 doi: 10.1038/cgt.2016.40
![]() |
[5] |
D. Lambrechts, E. Wauters, B. Boeckx, S. Aibar, D. Nittner, O. Burton, et al., Phenotype molding of stromal cells in the lung tumor microenvironment, Nat. Med., 24 (2018), 1277–1289. https://doi.org/10.1038/s41591-018-0096-5 doi: 10.1038/s41591-018-0096-5
![]() |
[6] |
A. Mantovani, S. Sozzani, M. Locati, P. Allavena, A. Sica, Macrophage polarization: tumor-associated macrophages as a paradigm for polarized M2 mononuclear phagocytes, Trends Immunol., 23 (2002), 549–555. https://doi.org/10.1016/S1471-4906(02)02302-5 doi: 10.1016/S1471-4906(02)02302-5
![]() |
[7] |
A. Kilic, R. Landreneau, J. Luketich, A. Pennathur, M. Schuchert, Density of tumor-infiltrating lymphocytes correlates with disease recurrence and survival in patients with large non-small-cell lung cancer tumors, J. Surg. Res., 167 (2011), 207–210. https://doi.org/10.1016/j.jss.2009.08.029 doi: 10.1016/j.jss.2009.08.029
![]() |
[8] |
Y. Kim, H. Jeon, H. Othmer, The role of the tumor microenvironment in glioblastoma: A mathematical model, IEEE Trans. Biomed. Eng., 64 (2017), 519–527. https://doi.org/10.1109/TBME.2016.2637828 doi: 10.1109/TBME.2016.2637828
![]() |
[9] |
A. Yuan, Y. Hsiao, H. Chen, H. Chen, C. Ho, Y. Chen, et al., Opposite effects of M1 and M2 macrophage subtypes on lung cancer progression, Sci. Rep., 5 (2015), 14273. https://doi.org/10.1038/srep14273 doi: 10.1038/srep14273
![]() |
[10] |
M. Shaul, L. Levy, J. Sun, I. Mishalian, S. Singhal, V. Kapoor, et al., Tumor-associated neutrophils display a distinct N1 profile following TGFbeta modulation: A transcriptomics analysis of pro- vs. antitumor TANs, Oncoimmunology, 5 (2016), e1232221. https://doi.org/10.1080/2162402X.2016.1232221 doi: 10.1080/2162402X.2016.1232221
![]() |
[11] |
C. Hagerling, Z. Werb, Neutrophils: Critical components in experimental animal models of cancer, Semin. Immunol., 28 (2016), 197–204. https://doi.org/10.1016/j.smim.2016.02.003 doi: 10.1016/j.smim.2016.02.003
![]() |
[12] |
R. Sionov, Z. Fridlender, Z. Granot, The multifaceted roles neutrophils play in the tumor microenvironment, Cancer Microenviron., 8 (2015), 125–158. https://doi.org/10.1007/s12307-014-0147-5 doi: 10.1007/s12307-014-0147-5
![]() |
[13] |
A. Swierczak, K. Mouchemore, J. Hamilton, R. Anderson, Neutrophils: important contributors to tumor progression and metastasis, Cancer Metast. Rev., 34 (2015), 735–751. https://doi.org/10.1007/s10555-015-9594-9 doi: 10.1007/s10555-015-9594-9
![]() |
[14] |
W. Liang, N. Ferrara, The complex role of neutrophils in tumor angiogenesis and metastasis, Cancer Immunol. Res., 4 (2016), 83–91. https://doi.org/10.1158/2326-6066.CIR-15-0313 doi: 10.1158/2326-6066.CIR-15-0313
![]() |
[15] |
Z. G. Fridlender, J. Sun, S. Kim, V. Kapoor, G. Cheng, L. Ling, et al., Polarization of tumor-associated neutrophil phenotype by TGF-beta: N1 versus N2 TAN, Cancer Cell, 16 (2009), 183–194, https://doi.org/10.1016/j.ccr.2009.06.017. doi: 10.1016/j.ccr.2009.06.017
![]() |
[16] | J. Foekens, C. Ries, M. Look, C. Gippner-Steppert, J. Klijn, M. Jochum, The prognostic value of polymorphonuclear leukocyte elastase in patients with primary breast cancer, Cancer Res., 63 (2003), 337–441. |
[17] | A. Bellocq, M. Antoine, A. Flahault, C. Philippe, B. Crestani, J. Bernaudin, et al., Neutrophil alveolitis in bronchioloalveolar carcinoma: induction by tumor-derived interleukin-8 and relation to clinical outcome, Am. J. Pathol., 152 (1998), 83–92. |
[18] |
J. Atzpodien, M. Reitz, Peripheral blood neutrophils as independent immunologic predictor of response and long-term survival upon immunotherapy in metastatic renal-cell carcinom, Cancer Biother. Radiopharm., 23 (2008), 129–134. https://doi.org/10.1089/cbr.2007.0429 doi: 10.1089/cbr.2007.0429
![]() |
[19] |
H. Schmidt, L. Bastholt, P. Geertsen, I. Christensen, S. Larsen, J. Gehl, et al., Elevated neutrophil and monocyte counts in peripheral blood are associated with poor survival in patients with metastatic melanoma: a prognostic model, Br. J. Cancer, 93 (2005), 273–278. https://doi.org/10.1038/sj.bjc.6602702 doi: 10.1038/sj.bjc.6602702
![]() |
[20] | A. J. Templeton, M. G. McNamara, B. Šeruga, F. Vera-Badillo, P. Aneja, A. Ocaña, et al., Prognostic role of neutrophil-to-lymphocyte ratio in solid tumors: a systematic review and meta-analysis, J. Natl. Cancer Inst., 106 (2014), dju124. |
[21] |
H. Maymani, K. Hess, R. Groisberg, D. Hong, A. Naing, S. Piha-Paul, et al., Predicting outcomes in patients with advanced non-small cell lung cancer enrolled in early phaseimmunotherapy trials, Lung Cancer, 120 (2018), 137–141. https://doi.org/10.1016/j.lungcan.2018.03.020 doi: 10.1016/j.lungcan.2018.03.020
![]() |
[22] |
J. Wang, Y. Jia, N. Wang, X. Zhang, B. Tan, G. Zhang, et al., The clinical significance of tumor-infiltrating neutrophils and neutrophil-to-CD8+ lymphocyte ratio in patients with resectable esophageal squamous cell carcinoma, J. Transl. Med., 12 (2014), 7. https://doi.org/10.1186/1479-5876-12-7 doi: 10.1186/1479-5876-12-7
![]() |
[23] |
R. Dolan, S. McSorley, J. Park, D. Watt, C. Roxburgh, P. Horgan, et al., The prognostic value of systemic inflammation in patients undergoing surgery for colon cancer: comparison of composite ratios and cumulative scores, Br. J. Cancer, 119 (2018), 40–51. https://doi.org/10.1038/s41416-018-0095-9 doi: 10.1038/s41416-018-0095-9
![]() |
[24] |
Y. Tao, L. Ding, G. Yang, J. Qiu, D. Wang, H. Wang, et al., Predictive impact of the inflammation-based indices in colorectal cancer patients with adjuvantchemotherapy, Cancer Med., 7 (2018), 2876–2886. https://doi.org/10.1002/cam4.1542 doi: 10.1002/cam4.1542
![]() |
[25] |
T. Tham, Y. Bardash, S. Herman, P. Costantino, Neutrophil-to-lymphocyte ratio as a prognostic indicator in head and neck cancer: A systematicreview and meta-analysis, Head Neck, 40 (2018), 2546–2557. https://doi.org/10.1002/hed.25324 doi: 10.1002/hed.25324
![]() |
[26] |
Z. Huang, Y. Liu, C. Yang, X. Li, C. Pan, J. Rao, et al., Combined neutrophil/platelet/lymphocyte/differentiation score predicts chemosensitivity in advanced gastric cancer, BMC Cancer, 18 (2018), 515. https://doi.org/10.1186/s12885-018-4414-6 doi: 10.1186/s12885-018-4414-6
![]() |
[27] | D. Galun, A. Bogdanovic, J. D. Kovac, P. Bulajic, Z. Loncar, M. Zuvela, Preoperative neutrophil-to-lymphocyte ratio as a prognostic predictor after curative-intent surgeryfor hepatocellular carcinoma: experience from a developing country, Cancer Manage. Res., 10 (2018), 977–988. |
[28] | J. Kaiser, H. Li, S. North, R. Leibowitz-Amit, J. Seah, N. Morshed, et al., The prognostic role of the change in neutrophil-to-lymphocyte ratio during neoadjuvantchemotherapy in patients with muscle-invasive bladder cancer: A retrospective, multi-institutional study, Bladder Cancer, 4 (2018), 185–194. |
[29] |
M. Zhu, M. Feng, F. He, B. Han, K. Ma, X. Zeng, et al., Pretreatment neutrophil-lymphocyte and platelet-lymphocyte ratio predict clinical outcome and prognosis for cervical cancer, Clin. Chim. Acta, 483 (2018), 296–302. https://doi.org/10.1016/j.cca.2018.05.025 doi: 10.1016/j.cca.2018.05.025
![]() |
[30] | P. Xue, M. Kanai, Y. Mori, T. Nishimura, N. Uza, Y. Kodama, et al., Neutrophil-to-lymphocyte ratio for predicting palliative chemotherapy outcomes in advancedpancreatic cancer patients, Cancer Med., 3 (2014), 406–415. |
[31] |
W. Park, G. Lopes, Perspectives: Neutrophil-to-lymphocyte ratio as a potential biomarker in immune checkpoint inhibitor for non-small-cell lung cancer, Clin. Lung Cancer, 20 (2019), 143–147. https://doi.org/10.1016/j.cllc.2018.12.003 doi: 10.1016/j.cllc.2018.12.003
![]() |
[32] |
T. Tuting, K. de Visser, How neutrophils promote metastasis, Science, 352 (2016), 145–146. https://doi.org/10.1126/science.aaf7300 doi: 10.1126/science.aaf7300
![]() |
[33] |
E. Jalilian, F. Abolhasani-Zadeh, A. Afgar, A. Samoudi, H. Zeinalynezhad, L. Langroudi, Neutralizing tumor-related inflammation and reprogramming of cancer-associated fibroblasts by curcumin in breast cancer therapy, Sci. Rep., 13 (2023), 20770. https://doi.org/10.1038/s41598-023-48073-w doi: 10.1038/s41598-023-48073-w
![]() |
[34] |
G. Evan, E. Harrington, A. Fanidi, H. Land, B. Amati, M. Bennett, Integrated control of cell proliferation and cell death by the c-myc oncogene, Philos. Trans. R. Soc. Lond. B Biol. Sci., 345 (1994), 269–275. https://doi.org/10.1098/rstb.1994.0105 doi: 10.1098/rstb.1994.0105
![]() |
[35] |
T. Libermann, L. Zerbini, Targeting transcription factors for cancer gene therapy, Curr. Gene Ther., 6 (2006), 17–33. https://doi.org/10.2174/156652306775515501 doi: 10.2174/156652306775515501
![]() |
[36] |
M. Marin, A. Karis, P. Visser, F. Grosveld, S. Philipsen, Transcription factor Sp1 is essential for early embryonic development but dispensable for cell growth and differentiation, Cell, 89 (1997), 619–628. https://doi.org/10.1016/S0092-8674(00)80243-3 doi: 10.1016/S0092-8674(00)80243-3
![]() |
[37] |
S. Akira, Functional roles of stat family proteins: lessons from knockout mice, Stem Cells, 17 (1999), 138–146. https://doi.org/10.1002/stem.170138 doi: 10.1002/stem.170138
![]() |
[38] |
H. Yu, D. Pardoll, R. Jove, STATs in cancer inflammation and immunity: a leading role for STAT3, Nat. Rev. Cancer, 9 (2009), 798–809. https://doi.org/10.1038/nrc2734 doi: 10.1038/nrc2734
![]() |
[39] |
J. Chen, J. Zhao, L. Chen, N. Dong, Z. Ying, Z. Cai, et al., STAT1 modification improves therapeutic effects of interferons on lung cancer cells, J. Transl. Med., 13 (2015), 293. https://doi.org/10.1186/s12967-015-0656-0 doi: 10.1186/s12967-015-0656-0
![]() |
[40] |
J. Yang, Y. Liu, X. Mai, S. Lu, L. Jin, X. Tai, STAT1-induced upregulation of LINC00467 promotes the proliferation migration of lung adenocarcinoma cells by epigenetically silencing DKK1 to activate Wnt/β-catenin signaling pathway, Biochem. Biophys. Res. Commun., 514 (2019), 118–126. https://doi.org/10.1016/j.bbrc.2019.04.107 doi: 10.1016/j.bbrc.2019.04.107
![]() |
[41] | C. L. Yang, Y. Y. Liu, Y. G. Ma, Y. X. Xue, D. G. Liu, Y. Ren, et al., Curcumin blocks small cell lung cancer cells migration, invasion, angiogenesis, cell cycle and neoplasia through janus kinase-STAT3 signalling pathway, PLoS One, 7 (2012), e37960. |
[42] |
F. Pezzella, H. Turley, I. Kuzu, M. Tungekar, M. Dunnill, C. Pierce, et al., bcl-2 protein in non-small-cell lung carcinoma, N. Engl. J. Med., 329 (1993), 690–694. https://doi.org/10.1056/NEJM199309023291003 doi: 10.1056/NEJM199309023291003
![]() |
[43] |
J. Pawlowski, A. Kraft, Bax-induced apoptotic cell death, Proc. Natl. Acad. Sci. U.S.A., 97 (2000), 529–531. https://doi.org/10.1073/pnas.97.2.529 doi: 10.1073/pnas.97.2.529
![]() |
[44] | I. Porebska, E. Wyrodek, M. Kosacka, J. Adamiak, R. Jankowska, A. Harlozinska-Szmyrka, Apoptotic markers p53, Bcl-2 and Bax in primary lung cancer, In Vivo, 20 (2006), 599–604. |
[45] |
M. Nielsen, C. Kaestel, K. Eriksen, A. Woetmann, T. Stokkedal, K. Kaltoft, et al., Inhibition of constitutively activated Stat3 correlates with altered Bcl-2/Bax expression and induction of apoptosis in mycosis fungoides tumor cells, Leukemia, 13 (1999), 735–738. https://doi.org/10.1038/sj.leu.2401415 doi: 10.1038/sj.leu.2401415
![]() |
[46] | J. Massague, TGFbeta in cancer, Cell, 134 (2008), 215–230. |
[47] |
C. David, J. Massague, Contextual determinants of TGFβ action in development, immunity and cancer, Nat. Rev. Mol. Cell Biol., 19 (2018), 419–435. https://doi.org/10.1038/s41580-018-0007-0 doi: 10.1038/s41580-018-0007-0
![]() |
[48] |
R. Akhurst, A. Hata, Targeting the TGFβ signalling pathway in disease, Nat. Rev. Drug Discov., 11 (2012), 790–811. https://doi.org/10.1038/nrd3810 doi: 10.1038/nrd3810
![]() |
[49] |
R. Akhurst, R. Derynck, TGF-β signaling in cancer–a double-edged sword, Trends Cell Biol., 11 (2001), S44–S51. https://doi.org/10.1016/S0962-8924(01)82259-5 doi: 10.1016/S0962-8924(01)82259-5
![]() |
[50] |
M. Li, R. Flavell, TGF-β: a master of all T cell trades, Cell, 134 (2008), 392–404. https://doi.org/10.1016/j.cell.2008.07.025 doi: 10.1016/j.cell.2008.07.025
![]() |
[51] |
C. Bellomo, L. Caja, A. Moustakas, Transforming growth factor β as regulator of cancer stemness and metastasis, Br. J. Cancer, 115 (2016), 761–769. https://doi.org/10.1038/bjc.2016.255 doi: 10.1038/bjc.2016.255
![]() |
[52] |
O. Aktas, A. Ozturk, B. Erman, S. Erus, S. Tanju, S. Dilege, Role of natural killer cells in lung cancer, J. Cancer Res. Clin. Oncol., 144 (2018), 997–1003. https://doi.org/10.1007/s00432-018-2635-3 doi: 10.1007/s00432-018-2635-3
![]() |
[53] |
R. Castriconi, C. Cantoni, M. Chiesa, M. Vitale, E. Marcenaro, R. Conte, et al., Transforming growth factor β1 inhibits expression of NKp30 and NKG2D receptors: consequences for the NK-mediated killing of dendritic cells, Proc. Natl. Acad. Sci. U.S.A., 100 (2003), 4120–4125. https://doi.org/10.1073/pnas.0730640100 doi: 10.1073/pnas.0730640100
![]() |
[54] |
M. Shaul, Z. Fridlender, Cancer related circulating and tumor-associated neutrophils - subtypes, sources and function, FEBS J., 285 (2018), 4316–4342. https://doi.org/10.1111/febs.14524 doi: 10.1111/febs.14524
![]() |
[55] |
S. Saha, S. Biswas, Tumor-associated neutrophils show phenotypic and functional divergence in human lung cancer, Cancer Cell, 30 (2016), 11–13. https://doi.org/10.1016/j.ccell.2016.06.016 doi: 10.1016/j.ccell.2016.06.016
![]() |
[56] |
L. Shen, J. Smith, Z. Shen, M. Eriksson, C. Sentman, C. Wira, Inhibition of human neutrophil degranulation by transforming growth factor-β1, Clin. Exp. Immunol., 149 (2007), 155–161. https://doi.org/10.1111/j.1365-2249.2007.03376.x doi: 10.1111/j.1365-2249.2007.03376.x
![]() |
[57] |
L. Andzinski, N. Kasnitz, S. Stahnke, C. Wu, M. Gereke, M. von Kockritz-Blickwede, et al., Type Ⅰ IFNs induce anti-tumor polarization of tumor associated neutrophils in mice and human, Int. J. Cancer, 138 (2016), 1982–1993. https://doi.org/10.1002/ijc.29945 doi: 10.1002/ijc.29945
![]() |
[58] |
J. Jablonska, S. Leschner, K. Westphal, S. Lienenklaus, S. Weiss, Neutrophils responsive to endogenous IFN-β regulate tumor angiogenesis and growth in a mouse tumor model, J. Clin. Invest., 120 (2010), 1151–1164. https://doi.org/10.1172/JCI37223 doi: 10.1172/JCI37223
![]() |
[59] |
F. Wang, S. Zhang, R. Jeon, I. Vuckovic, X. Jiang, A. Lerman, et al., Interferon gamma induces reversible metabolic reprogramming of M1 macrophages to sustain cell viability and pro-inflammatory activity, EBioMedicine, 30 (2018), 303–316. https://doi.org/10.1016/j.ebiom.2018.02.009 doi: 10.1016/j.ebiom.2018.02.009
![]() |
[60] |
J. Catani, R. Medrano, A. Hunger, P. D. Valle, S. Adjemian, D. Zanatta, et al., Intratumoral immunization by p19Arf and interferon-β gene transfer in a heterotopic mouse model of lung carcinoma, Transl. Oncol., 9 (2016), 565–574. https://doi.org/10.1016/j.tranon.2016.09.011 doi: 10.1016/j.tranon.2016.09.011
![]() |
[61] |
J. Swann, Y. Hayakawa, N. Zerafa, K. Sheehan, B. Scott, R. Schreiber, et al., Type Ⅰ IFN contributes to NK cell homeostasis, activation, and antitumor function, J. Immunol., 178 (2007), 7540–7549. https://doi.org/10.4049/jimmunol.178.12.7540 doi: 10.4049/jimmunol.178.12.7540
![]() |
[62] |
Q. Lin, L. Rong, X. Jia, R. Li, B. Yu, J. Hu, et al., IFN-γ-dependent NK cell activation is essential to metastasis suppression by engineered salmonella, Nat. Commun., 12 (2021), 2537. https://doi.org/10.1038/s41467-021-22755-3 doi: 10.1038/s41467-021-22755-3
![]() |
[63] |
M. Masucci, M. Minopoli, M. Carriero, Tumor associated neutrophils. their role in tumorigenesis, metastasis, prognosis and therapy, Front. Oncol., 9 (2019), 1146. https://doi.org/10.3389/fonc.2019.01146 doi: 10.3389/fonc.2019.01146
![]() |
[64] |
Y. Gao, J. Yang, Y. Cai, S. Fu, N. Zhang, X. Fu, et al., IFN-γ-mediated inhibition of lung cancer correlates with PD‐L1 expression and is regulated by PI3K-AKT signaling, Int. J. Cancer, 143 (2018), 931–943. https://doi.org/10.1002/ijc.31357 doi: 10.1002/ijc.31357
![]() |
[65] |
D. Jorgovanovic, M. Song, L. Wang, Y. Zhang, Roles of IFN-γ in tumor progression and regression: a review, Biomark Res., 8 (2020), 49. https://doi.org/10.1186/s40364-020-00228-x doi: 10.1186/s40364-020-00228-x
![]() |
[66] |
J. Cong, H. Wei, Natural killer cells in the lungs, Front. Immunol., 10 (2019), 1416. https://doi.org/10.3389/fimmu.2019.01416 doi: 10.3389/fimmu.2019.01416
![]() |
[67] |
M. Vitale, C. Cantoni, G. Pietra, M. Mingari, L. Moretta, Effect of tumor cells and tumor microenvironment on NK-cell function, Eur. J. Immunol., 44 (2014), 1582–1592. https://doi.org/10.1002/eji.201344272 doi: 10.1002/eji.201344272
![]() |
[68] |
D. Pardoll, The blockade of immune checkpoints in cancer immunotherapy, Nat. Rev. Cancer, 12 (2012), 252–264. https://doi.org/10.1038/nrc3239 doi: 10.1038/nrc3239
![]() |
[69] |
J. Gao, J. Ward, C. Pettaway, L. Shi, S. Subudhi, L. Vence, et al., Vista is an inhibitory immune checkpoint that is increased after ipilimumab therapy in patients with prostate cancer, Nat. Med., 23 (2017), 551–555. https://doi.org/10.1038/nm.4308 doi: 10.1038/nm.4308
![]() |
[70] |
R. Tallerico, C. Garofalo, E. Carbone, A new biological feature of natural killer cells: The recognition of solid tumor-derived cancer stem cells, Front. Immunol., 7 (2016), 179. https://doi.org/10.3389/fimmu.2016.00179 doi: 10.3389/fimmu.2016.00179
![]() |
[71] |
S. Viel, A. Marçais, F. Guimaraes, R. Loftus, J. Rabilloud, M. Grau, et al., TGF-β inhibits the activation and functions of NK cells by repressing the mTOR pathway, Sci. Signal, 9 (2016), ra19. https://doi.org/10.1126/scisignal.aad1884 doi: 10.1126/scisignal.aad1884
![]() |
[72] |
F. Otegbeye, E. Ojo, S. Moreton, N. Mackowski, D. Lee, M. de Lima, et al., Inhibiting TGF-beta signaling preserves the function of highly activated, in vitro expanded natural killer cells in aml and colon cancer models, PLoS One, 13 (2018), e0191358. https://doi.org/10.1371/journal.pone.0191358 doi: 10.1371/journal.pone.0191358
![]() |
[73] |
R. Sun, J. Luo, D. Li, Y. Shu, C. Luo, S. Wang, et al., Neutrophils with protumor potential could efficiently suppress tumor growth after cytokine priming and in presence of normal NK cells, Oncotarget, 5 (2014), 12621–12634. https://doi.org/10.18632/oncotarget.2181 doi: 10.18632/oncotarget.2181
![]() |
[74] |
Y. Kim, J. Yoo, T. Lee, J. Liu, J. Yu, M. Caligiuri, et al., Complex role of NK cells in regulation of oncolytic virus-bortezomib therapy, Proc. Natl. Acad. Sci. U.S.A., 115 (2018), 4927–4932. https://doi.org/10.1073/pnas.1715295115 doi: 10.1073/pnas.1715295115
![]() |
[75] |
S. Lim, T. Kim, J. Lee, C. Sonn, K. Kim, J. Kim, et al., Ex vivo expansion of highly cytotoxic human NK cells by cocultivation with irradiated tumor cells for adoptive immunotherapy, Cancer Res., 73 (2013), 2598–2607. https://doi.org/10.1158/0008-5472.CAN-12-2893 doi: 10.1158/0008-5472.CAN-12-2893
![]() |
[76] |
A. Aspirin, A. de Los Reyes V, Y. Kim, Polytherapeutic strategies with oncolytic virus-bortezomib and adjuvant NK cells in cancer treatment, J. R. Soc. Interface, 18 (2021), 20200669. https://doi.org/10.1098/rsif.2020.0669 doi: 10.1098/rsif.2020.0669
![]() |
[77] |
J. Sia, R. Szmyd, E. Hau, H. Gee, Molecular mechanisms of radiation-induced cancer cell death: A primer, Front. Cell Dev. Biol., 8 (2020), 41. https://doi.org/10.3389/fcell.2020.00041 doi: 10.3389/fcell.2020.00041
![]() |
[78] |
A. Koushik, K. Harish, H. Avinash, Principles of radiation oncology: a beams eye view for a surgeon, Indian J. Surg. Oncol., 4 (2013), 255–262. https://doi.org/10.1007/s13193-013-0231-1 doi: 10.1007/s13193-013-0231-1
![]() |
[79] |
J. Chen, X. Liu, Z. Zeng, J. Li, Y. Luo, W. Sun, et al., Immunomodulation of NK cells by ionizing radiation, Front. Oncol., 10 (2020), 874. https://doi.org/10.3389/fonc.2020.00874 doi: 10.3389/fonc.2020.00874
![]() |
[80] |
S. Formenti, S. Demaria, Combining radiotherapy and cancer immunotherapy: a paradigm shift, J. Natl. Cancer Inst., 105 (2013), 256–265. https://doi.org/10.1093/jnci/djs629 doi: 10.1093/jnci/djs629
![]() |
[81] |
X. Lai, A. Friedman, Mathematical modeling of cancer treatment with radiation and PD-L1 inhibitor, Sci. China Math., 63 (2020), 465–484. https://doi.org/10.1007/s11425-019-1648-6 doi: 10.1007/s11425-019-1648-6
![]() |
[82] |
D. Lonergan, A. Mikulec, M. Hanasono, M. Kita, R. Koch, Growth factor profile of irradiated human dermal fibroblasts using a serum-free method, Plast Reconstr. Surg., 111 (2003), 1960–1968. https://doi.org/10.1097/01.PRS.0000055065.41599.75 doi: 10.1097/01.PRS.0000055065.41599.75
![]() |
[83] |
J. Santibanez, M. Quintanilla, C. Bernabeu, TGF-β/TGF-β receptor system and its role in physiological and pathological conditions, Clin. Sci., 121 (2011), 233–251. https://doi.org/10.1042/CS20110086 doi: 10.1042/CS20110086
![]() |
[84] |
D. Powell, A. Huttenlocher, Neutrophils in the tumor microenvironment, Trends Immunol., 37 (2016), 41–52. https://doi.org/10.1016/j.it.2015.11.008 doi: 10.1016/j.it.2015.11.008
![]() |
[85] |
Y. Kim, D. Lee, J. Lee, S. Lee, S. Lawler, Role of tumor-associated neutrophils in regulation of tumor growth in lung cancer development: A mathematical model, PLoS One, 14 (2019), e0211041. https://doi.org/10.1371/journal.pone.0211041 doi: 10.1371/journal.pone.0211041
![]() |
[86] | E. Demidenko, Mixed Models: Theory and Applications with R, 2nd edition, Wiley Series, 2013. |
[87] | V. Collins, R. Loeffler, H. Tivey, Observations on growth rates of human tumors, Am. J. Roentgenol. Radium. Ther. Nucl. Med., 76 (1956), 988–1000. |
[88] |
A. Tsoularis, J. Wallace, Analysis of logistic growth models, Math. Biosci., 179 (2002), 21–55. https://doi.org/10.1016/S0025-5564(02)00096-2 doi: 10.1016/S0025-5564(02)00096-2
![]() |
[89] |
P. Charles, The gompertz curve as a growth curve, Proc. Natl. Acad. Sci. U.S.A., 18 (1932), 1–8. https://doi.org/10.1073/pnas.18.1.1 doi: 10.1073/pnas.18.1.1
![]() |
[90] |
H. Murphy, H. Jaafari, H. Dobrovolny, Differences in predictions of ode models of tumor growth: a cautionary example, BMC Cancer, 16 (2016), 163. https://doi.org/10.1186/s12885-016-2164-x doi: 10.1186/s12885-016-2164-x
![]() |
[91] |
Y. Kim, J. Wallace, F. Li, M. Ostrowski, A. Friedman, Transformed epithelial cells and fibroblasts/myofibroblasts interaction in breast tumor: a mathematical model and experiments, J. Math. Biol., 61 (2010), 401–421. https://doi.org/10.1007/s00285-009-0307-2 doi: 10.1007/s00285-009-0307-2
![]() |
[92] |
A. Stein, T. Demuth, D. Mobley, M. Berens, L. Sander, A mathematical model of glioblastoma tumor spheroid invasion in a three-dimensional in vitro experiment, Biophys. J., 92 (2007), 356–365. https://doi.org/10.1529/biophysj.106.093468 doi: 10.1529/biophysj.106.093468
![]() |
[93] |
H. Enderling, A. Anderson, M. Chaplain, A. Munro, J. Vaidya, Mathematical modelling of radiotherapy strategies for early breast cancer, J. Theor. Biol., 241 (2006), 158–71. https://doi.org/10.1016/j.jtbi.2005.11.015 doi: 10.1016/j.jtbi.2005.11.015
![]() |
[94] |
J. Weis, M. Miga, T. Yankeelov, Three-dimensional image-based mechanical modeling for predicting the response of breast cancer to neoadjuvant therapy, Comput. Methods Appl. Mech. Eng., 314 (2017), 494–512. https://doi.org/10.1016/j.cma.2016.08.024 doi: 10.1016/j.cma.2016.08.024
![]() |
[95] |
Y. Kim, J. Lee, C. Lee, S. Lawler, Role of senescent tumor cells in building a cytokine shield in the tumor microenvironment: mathematical modeling, J. Math. Biol., 86 (2022), 14. https://doi.org/10.1007/s00285-022-01850-z doi: 10.1007/s00285-022-01850-z
![]() |
[96] |
Y. Kim, D. Lee, S. Lawler, Collective invasion of glioma cells through OCT1 signalling and interaction with reactive astrocytes after surgery, Phil. Trans. R. Soc. B, 375 (2020), 20190390. https://doi.org/10.1098/rstb.2019.0390 doi: 10.1098/rstb.2019.0390
![]() |
[97] |
Y. Kim, J. Lee, D. Lee, H. Othmer, Synergistic effects of bortezomib-OV therapy and anti-invasive strategies in glioblastoma: a mathematical model, Cancers, 11 (2019), 215. https://doi.org/10.3390/cancers11020215 doi: 10.3390/cancers11020215
![]() |
[98] |
H. Enderling, M. Chaplain, A. Anderson, J. Vaidya, A mathematical model of breast cancer development, local treatment and recurrence, J. Theor. Biol., 246 (2007), 245–259. https://doi.org/10.1016/j.jtbi.2006.12.010 doi: 10.1016/j.jtbi.2006.12.010
![]() |
[99] |
D. Corwin, C. Holdsworth, R. Rockne, A. Trister, M. Mrugala, J. Rockhill, et al., Toward patient-specific, biologically optimized radiation therapy plans for the treatment of glioblastoma, PLoS One, 8 (2013), e79115. https://doi.org/10.1371/journal.pone.0079115 doi: 10.1371/journal.pone.0079115
![]() |
[100] |
Y. Kim, A. Friedman, Interaction of tumor with its microenvironment: A mathematical model, Bull. Math. Biol., 72 (2010), 1029–1068. https://doi.org/10.1007/s11538-009-9481-z doi: 10.1007/s11538-009-9481-z
![]() |
[101] | P. Maini, Modelling aspects of tumour metabolism, in Proceedings of the International Congress of Mathematicians 2010 (ICM 2010), (2011), 3091–3104. https://doi.org/10.1142/9789814324359_0181 |
[102] |
Y. Kim, H. Lee, N. Dmitrieva, J. Kim, B. Kaur, A. Friedman, Choindroitinase ABC Ⅰ-mediated enhancement of oncolytic virus spread and anti-tumor efficacy: A mathematical model, PLoS One, 9 (2014), e102499. https://doi.org/10.1371/journal.pone.0102499 doi: 10.1371/journal.pone.0102499
![]() |
[103] |
K. Swanson, E. Alvord, J. Murray, Virtual resection of gliomas: Effect of extent of resection on recurrence, Math. Comput. Modell., 37 (2003), 1177–1190. https://doi.org/10.1016/S0895-7177(03)00129-8 doi: 10.1016/S0895-7177(03)00129-8
![]() |
[104] |
Y. Kim, S. Roh, S. Lawler, A. Friedman, miR451 and AMPK/MARK mutual antagonism in glioma cells migration and proliferation, PLoS One, 6 (2011), e28293. https://doi.org/10.1371/journal.pone.0028293 doi: 10.1371/journal.pone.0028293
![]() |
[105] |
K. Kotredes, A. Gamero, Interferons as inducers of apoptosis in malignant cells, J. Interferon Cytokine Res., 33 (2013), 162–170. https://doi.org/10.1089/jir.2012.0110 doi: 10.1089/jir.2012.0110
![]() |
[106] |
A. Groeger, V. Esposito, A. D. Luca, R. Cassandro, G. Tonini, V. Ambrogi, et al., Prognostic value of immunohistochemical expression of p53, bax, Bcl‐2 and Bcl‐xL in resected non‐small‐cell lung cancers, Histopathology, 44 (2004), 54–63. https://doi.org/10.1111/j.1365-2559.2004.01750.x doi: 10.1111/j.1365-2559.2004.01750.x
![]() |
[107] |
J. Lee, D. Lee, Y. Kim, Mathematical model of stat signalling pathways in cancer development and optimal control approaches, R. Soc. Open Sci., 9 (2021), 210594. https://doi.org/10.1098/rsos.210594 doi: 10.1098/rsos.210594
![]() |
[108] |
C. Huang, C. Chung, T. Hu, J. Chen, P. Liu, C. Chen, Recent progress in TGF-β inhibitors for cancer therapy, Biomed. Pharmacother., 134 (2021), 111046. https://doi.org/10.1016/j.biopha.2020.111046 doi: 10.1016/j.biopha.2020.111046
![]() |
[109] | S. Herbertz, J. S. Sawyer, A. J. Stauber, I. Gueorguieva, K. E. Driscoll, S. T. Estrem, et al., Clinical development of galunisertib (LY2157299 monohydrate), a small molecule inhibitor of transforming growth factor-beta signaling pathway, Drug Des. Dev. Ther., 9 (2015), 4479–4499. |
[110] |
R. Kelley, E. Gane, E. Assenat, J. Siebler, P. Galle, P. Merle, et al., A phase 2 study of galunisertib (TGF-β1 receptor type Ⅰ inhibitor) and sorafenib in patients with advanced hepatocellular carcinoma, Clin. Transl. Gastroenterol., 10 (2019), e00056. https://doi.org/10.14309/ctg.0000000000000056 doi: 10.14309/ctg.0000000000000056
![]() |
[111] |
N. Bjorkstrom, H. Ljunggren, J. Michaelsson, Emerging insights into natural killer cells in human peripheral tissues, Nat. Rev. Immunol., 16 (2016), 310–320. https://doi.org/10.1038/nri.2016.34 doi: 10.1038/nri.2016.34
![]() |
[112] |
J. Lee, K. Park, J. Ryu, H. Bae, A. Choi, H. Lee, et al., Natural killer cell activity for IFN-gamma production as a supportive diagnostic marker for gastric cancer, Oncotarget, 8 (2017), 70431–70440. https://doi.org/10.18632/oncotarget.19712 doi: 10.18632/oncotarget.19712
![]() |
[113] |
Y. Rocca, M. Roberti, E. Julia, M. Pampena, L. Bruno, S. Rivero, et al., Phenotypic and functional dysregulated blood NK cells in colorectal cancer patients can be activated by cetuximab plus IL-2 or IL-15, Front. Immunol., 7 (2016), 413. https://doi.org/10.3389/fimmu.2016.00413 doi: 10.3389/fimmu.2016.00413
![]() |
[114] |
M. Lodoen, L. Lanier, Natural killer cells as an initial defense against pathogens, Curr. Opin. Immunol., 18 (2006), 391–398. https://doi.org/10.1080/08913810608443667 doi: 10.1080/08913810608443667
![]() |
[115] |
J. Siren, T. Sareneva, J. Pirhonen, M. Strengell, V. Veckman, I. Julkunen, et al., Cytokine and contact-dependent activation of natural killer cells by influenza a or sendai virus-infected macrophages, J. Gen. Virol., 85 (2004), 2357–2364. https://doi.org/10.1099/vir.0.80105-0 doi: 10.1099/vir.0.80105-0
![]() |
[116] |
A. Iversen, P. Norris, C. Ware, C. Benedict, Human NK cells inhibit cytomegalovirus replication through a noncytolytic mechanism involving lymphotoxin-dependent induction of IFN-beta, J. Immunol., 175 (2005), 7568–7574. https://doi.org/10.4049/jimmunol.175.11.7568 doi: 10.4049/jimmunol.175.11.7568
![]() |
[117] |
M. Studeny, F. Marini, J. Dembinski, C. Zompetta, M. Cabreira-Hansen, B. Bekele, et al., Mesenchymal stem cells: potential precursors for tumor stroma and targeted-delivery vehicles for anticancer agents, J. Natl. Cancer Inst., 96 (2004), 1593–603. https://doi.org/10.1093/jnci/djh299 doi: 10.1093/jnci/djh299
![]() |
[118] |
M. Chiantore, S. Vannucchi, R. Accardi, M. Tommasino, Z. Percario, G. Vaccari, et al., Interferon-β induces cellular senescence in cutaneous human papilloma virus-transformed human keratinocytes by affecting p53 transactivating activity, PLoS One, 7 (2012), e36909. https://doi.org/10.1371/journal.pone.0036909 doi: 10.1371/journal.pone.0036909
![]() |
[119] |
A. Takaoka, S. Hayakawa, H. Yanai, D. Stoiber, H. Negishi, H. Kikuchi, et al., Integration of interferon-α/β signalling to p53 responses in tumour suppression and antiviral defence, Nature, 424 (2003), 516–523. https://doi.org/10.1038/nature01850 doi: 10.1038/nature01850
![]() |
[120] |
F. Zhang, S. Sriram, Identification and characterization of the interferon-beta-mediated p53 signal pathway in human peripheral blood mononuclear cells, Immunology, 128 (2009), e905–e918. https://doi.org/10.1111/j.1365-2567.2009.03104.x doi: 10.1111/j.1365-2567.2009.03104.x
![]() |
[121] |
D. Ghosh, P. Parida, Interferon therapy in lung cancer: Current perspectives, Curr. Cancer Ther. Rev., 12 (2016), 237–245. https://doi.org/10.1016/j.explore.2016.04.001 doi: 10.1016/j.explore.2016.04.001
![]() |
[122] |
B. Liu, X. Zhu, L. Kong, M. Wang, C. Spanoudis, P. Chaturvedi, et al., Bifunctional TGF-β trap/IL-15 protein complex elicits potent NK cell and CD8+ T cell immunity against solid tumors, Mol. Ther., 29 (2021), 2949–2962. https://doi.org/10.1109/TFUZZ.2020.3009755 doi: 10.1109/TFUZZ.2020.3009755
![]() |
[123] |
J. Marcoe, J. Lim, K. Schaubert, N. Fodil-Cornu, M. Matka, A. McCubbrey, et al., TGF-β is responsible for NK cell immaturity during ontogeny and increased susceptibility to infection during mouse infancy, Nat. Immunol., 13 (2012), 843–850. https://doi.org/10.1038/ni.2388 doi: 10.1038/ni.2388
![]() |
[124] |
R. Rouce, H. Shaim, T. Sekine, G. Weber, B. Ballard, S. Ku, et al., The TGF-β/SMAD pathway is an important mechanism for NK cell immune evasion in childhood B-acute lymphoblastic leukemia, Leukemia, 30 (2016), 800–811. https://doi.org/10.1038/leu.2015.327 doi: 10.1038/leu.2015.327
![]() |
[125] |
S. Regis, A. Dondero, F. Caliendo, C. Bottino, R. Castriconi, NK cell function regulation by TGF-β-induced epigenetic mechanisms, Front. Immunol., 11 (2020), 311. https://doi.org/10.3389/fimmu.2020.00311 doi: 10.3389/fimmu.2020.00311
![]() |
[126] |
T. Laskowski, A. Biederstadt, K. Rezvani, Natural killer cells in antitumour adoptive cell immunotherapy, Nat. Rev. Cancer, 22 (2022), 557–575. https://doi.org/10.1038/s41568-022-00491-0 doi: 10.1038/s41568-022-00491-0
![]() |
[127] |
S. Marino, I. Hogue, C. Ray, D. Kirschner, A methodology for performing global uncertainty and sensitivity analysis in systems biology, J. Theor. Biol., 254 (2008), 178–196. https://doi.org/10.1016/j.jtbi.2008.04.011 doi: 10.1016/j.jtbi.2008.04.011
![]() |
[128] |
J. Fowler, The linear-quadratic formula and progress in fractionated radiotherapy, Br. J. Radiol., 62 (1989), 679–694. https://doi.org/10.1259/0007-1285-62-740-679 doi: 10.1259/0007-1285-62-740-679
![]() |
[129] | G. Wiernik, Fractionation in radiotherapy, Anticancer Res., 3 (1983), 283–297. |
[130] |
S. McMahon, The linear quadratic model: usage, interpretation and challenges, Phys. Med. Biol., 64 (2019), 01TR01. https://doi.org/10.1088/1361-6560/aaf26a doi: 10.1088/1361-6560/aaf26a
![]() |
[131] |
H. Enderling, M. Chaplain, P. Hahnfeldt, Quantitative modeling of tumor dynamics and radiotherapy, Acta Biotheor., 58 (2010), 341–353. https://doi.org/10.1007/s10441-010-9111-z doi: 10.1007/s10441-010-9111-z
![]() |
[132] |
J. Harrold, P. Gisleskog, I. Delor, P. Jacqmin, J. Perez-Ruixo, A. Narayanan, et al., Quantification of radiation injury on neutropenia and the link between absolute neutrophil count time course and overall survival in nonhuman primates treated with G-CSF, Pharm. Res., 37 (2020), 102. https://doi.org/10.1007/s11095-020-02839-3 doi: 10.1007/s11095-020-02839-3
![]() |
[133] |
A. Wisdom, C. Hong, A. Lin, Y. Xiang, D. Cooper, J. Zhang, et al., Neutrophils promote tumor resistance to radiation therapy, Proc. Natl. Acad. Sci. U.S.A., 116 (2019), 18584–18589. https://doi.org/10.1073/pnas.1901562116 doi: 10.1073/pnas.1901562116
![]() |
[134] |
H. Thames, S. Bentzen, I. Turesson, M. Overgaard, W. V. den Bogaert, Time-dose factors in radiotherapy: a review of the human data, Radiother. Oncol., 19 (1990), 219–235. https://doi.org/10.1016/0167-8140(90)90149-Q doi: 10.1016/0167-8140(90)90149-Q
![]() |
[135] |
P. Blake, A. Swart, J. Orton, H. Kitchener, T. Whelan, H. Lukka, et al., Adjuvant external beam radiotherapy in the treatment of endometrial cancer (MRC ASTEC and NCIC CTG EN. 5 randomised trials): pooled trial results, systematic review, and meta-analysis, Lancet, 373 (2009), 137–146. https://doi.org/10.1016/S0140-6736(08)61767-5 doi: 10.1016/S0140-6736(08)61767-5
![]() |
[136] |
S. Faria, W. Schlupp, H. Chiminazzo Jr, Radiotherapy in the treatment of vertebral hemangiomas, Int. J. Radiat. Oncol. Biol. Phys., 11 (1985), 387–390. https://doi.org/10.1016/0360-3016(85)90162-2 doi: 10.1016/0360-3016(85)90162-2
![]() |
[137] | S. Lenhart, J. Workman, Optimal Control Applied to Biological Models, 1st edition, Chapman and Hall/CRC, 2007. |
[138] |
A. Jarrett, D. Faghihi, D. Ii, E. Lima, J. Virostko, G. Biros, et al., Optimal control theory for personalized therapeutic regimens in oncology: Background, history, challenges, and opportunities, J. Clin. Med., 9 (2020), 1314. https://doi.org/10.3390/jcm9051314 doi: 10.3390/jcm9051314
![]() |
[139] |
K. Bahrami, M. Kim, Optimal control of multiplicative control systems arising from cancer therapy, IEEE Trans. Autom. Control, 20 (1975), 537–542. https://doi.org/10.1109/TAC.1975.1101019 doi: 10.1109/TAC.1975.1101019
![]() |
[140] |
G. W. Swan, T. L. Vincent, Optimal control analysis in the chemotherapy of IGG multiple myeloma, Bull. Math. Biol., 39 (1977), 317–337. https://doi.org/10.1016/S0092-8240(77)80070-0 doi: 10.1016/S0092-8240(77)80070-0
![]() |
[141] |
G. W. Swan, Optimal control applications in the chemotherapy of multiple myeloma, Math. Med. Biol., 2 (1985), 139–160. https://doi.org/10.1093/imammb/2.3.139 doi: 10.1093/imammb/2.3.139
![]() |
[142] |
A. Ergun, K. Camphausen, L. Wein, Optimal scheduling of radiotherapy and angiogenic inhibitors, Bull. Math. Biol., 65 (2003), 407–424. https://doi.org/10.1016/S0092-8240(03)00006-5 doi: 10.1016/S0092-8240(03)00006-5
![]() |
[143] |
D. Lee, A. de Los Reyes V, Y. Kim, Optimal strategies of oncolytic virus-bortezomib therapy via the apoptotic, necroptotic, and oncolysis signaling network, Math. Biosci. Eng., 21 (2024), 3876–3909. https://doi.org/10.3934/mbe.2024173 doi: 10.3934/mbe.2024173
![]() |
[144] |
E. Ratajczyk, U. Ledzewicz, H. Schattler, Optimal control for a mathematical model of glioma treatment with oncolytic therapy and TNF-α inhibitors, J. Optim. Theory Appl., 176 (2018), 456–477. https://doi.org/10.1007/s10957-018-1218-4 doi: 10.1007/s10957-018-1218-4
![]() |
[145] | H. Schattler, Y. Kim, U. Ledzewicz, A. los Reyes V, E. Jung, On the control of cell migration and proliferation in glioblastoma, in Proceeding of the IEEE Conference on Decision and Control, (2013), 1810–1815. https://doi.org/10.1109/CDC.2013.6760145 |
[146] |
E. Jung, A. los Reyes, K. Pumares, Y. Kim, Strategies in regulating glioblastoma signaling pathways and anti-invasion therapy, PLoS One, 14 (2019), e0215547. https://doi.org/10.1371/journal.pone.0215547 doi: 10.1371/journal.pone.0215547
![]() |
[147] |
A. L. Reyes, E. Jung, Y. Kim, Optimal control strategies of eradicating invisible glioblastoma cells after conventional surgery, J. Roy. Soc. Interface, 12 (2015), 20141392. https://doi.org/10.1098/rsif.2014.1392 doi: 10.1098/rsif.2014.1392
![]() |
[148] |
A. Reyes, Y. Kim, Optimal regulation of tumour-associated neutrophils in cancer progression, R. Soc. Open Sci., 9 (2022), 210705. https://doi.org/10.1098/rsos.210705 doi: 10.1098/rsos.210705
![]() |
[149] |
G. Boivin, P. Ancey, R. de Silly, P. Kalambaden, C. Contat, B. Petit, et al., Anti-Ly6G binding and trafficking mediate positive neutrophil selection to unleash the anti-tumor efficacy of radiation therapy, Oncoimmunology, 10 (2021), 1876597. https://doi.org/10.1080/2162402X.2021.1876597 doi: 10.1080/2162402X.2021.1876597
![]() |
[150] |
Z. Zhang, X. Liu, D. Chen, J. Yu, Radiotherapy combined with immunotherapy: the dawn of cancer treatment, Signal Transduction Targeted Ther., 7 (2022), 258. https://doi.org/10.1038/s41392-022-01102-y doi: 10.1038/s41392-022-01102-y
![]() |
[151] |
M. Grinde, J. Vik, K. Camilio, I. Martinez-Zubiaurre, T. Hellevik, Ionizing radiation abrogates the pro-tumorigenic capacity of cancer-associated fibroblasts co-implanted in xenografts, Sci. Rep., 7 (2017), 46714. https://doi.org/10.1038/srep46714 doi: 10.1038/srep46714
![]() |
[152] |
O. Al-Assar, F. Demiciorglu, S. Lunardi, M. Gaspar-Carvalho, W. McKenna, R. Muschel, et al., Contextual regulation of pancreatic cancer stem cell phenotype and radioresistance by pancreatic stellate cells, Radiother. Oncol., 111 (2014), 243–251. https://doi.org/10.1016/j.radonc.2014.03.014 doi: 10.1016/j.radonc.2014.03.014
![]() |
[153] |
C. Arteaga, J. Engelman, ERBB receptors: from oncogene discovery to basic science to mechanism-based cancer therapeutics, Cancer Cell, 25 (2014), 282–303. https://doi.org/10.1016/j.ccr.2014.02.025 doi: 10.1016/j.ccr.2014.02.025
![]() |
[154] |
S. Du, S. Bouquet, C. Lo, I. Pellicciotta, S. Bolourchi, R. Parry, et al., Attenuation of the dna damage response by transforming growth factor-beta inhibitors enhances radiation sensitivity of non-small-cell lung cancer cells in vitro and in vivo, Int. J. Radiat. Oncol. Biol. Phys., 91 (2015), 91–99. https://doi.org/10.1016/j.ijrobp.2014.09.026 doi: 10.1016/j.ijrobp.2014.09.026
![]() |
[155] |
E. Dieleman, A. Uitterhoeve, M. van Hoek, R. van Os, J. Wiersma, M. Koolen, et al., Concurrent daily cisplatin and high-dose radiation therapy in patients with stage iii non-small cell lung cancer, Int. J. Radiat. Oncol. Biol. Phys., 102 (2018), 543–551. https://doi.org/10.1016/j.ijrobp.2018.07.188 doi: 10.1016/j.ijrobp.2018.07.188
![]() |
[156] |
S. Vora, B. Daly, L. Blaszkowsky, J. McGrath, M. Bankoff, S. Supran, et al., High dose radiation therapy and chemotherapy as induction treatment for stage iii nonsmall cell lung carcinoma, Cancer, 89 (2000), 1946–1952. https://doi.org/10.1002/1097-0142(20001101)89:9<1946::AID-CNCR10>3.0.CO;2-1 doi: 10.1002/1097-0142(20001101)89:9<1946::AID-CNCR10>3.0.CO;2-1
![]() |
[157] |
F. Kong, R. T. Haken, M. Schipper, M. Sullivan, M. Chen, C. Lopez, et al., High-dose radiation improved local tumor control and overall survival in patients with inoperable/unresectable non-small-cell lung cancer: long-term results of a radiation dose escalation study, Int. J. Radiat. Oncol. Biol. Phys., 63 (2005), 324–333. https://doi.org/10.1016/j.ijrobp.2005.02.010 doi: 10.1016/j.ijrobp.2005.02.010
![]() |
[158] |
M. Manus, K. Lamborn, W. Khan, A. Varghese, L. Graef, S. Knox, Radiotherapy-associated neutropenia and thrombocytopenia: analysis of risk factors and development of a predictive model, Blood, 89 (1997), 2303–2310. https://doi.org/10.1182/blood.V89.7.2303 doi: 10.1182/blood.V89.7.2303
![]() |
[159] |
R. Prabhu, R. Cassidy, J. Landry, Radiation therapy and neutropenia, Curr. Probl. Cancer, 39 (2015), 292–296. https://doi.org/10.1016/j.currproblcancer.2015.07.010 doi: 10.1016/j.currproblcancer.2015.07.010
![]() |
[160] |
T. Smith, J. Khatcheressian, G. Lyman, H. Ozer, J. Armitage, L. Balducci, et al., 2006 update of recommendations for the use of white blood cell growth factors: an evidence-based clinical practice guideline, J. Clin. Oncol., 24 (2006), 3187–3205. https://doi.org/10.1200/JCO.2006.06.4451 doi: 10.1200/JCO.2006.06.4451
![]() |
[161] |
J. Crawford, C. Caserta, F. Roila, Hematopoietic growth factors: ESMO clinical practice guidelines for the applications, Ann. Oncol., 21 (2010), 248–251. https://doi.org/10.1093/annonc/mdq195 doi: 10.1093/annonc/mdq195
![]() |
[162] |
P. Mauch, L. Constine, J. Greenberger, W. Knospe, J. Sullivan, J. Liesveld, et al., Hematopoietic stem cell compartment: acute and late effects of radiation therapy and chemotherapy, Int. J. Radiat. Oncol. Biol. Phys., 31 (1995), 1319–1339. https://doi.org/10.1016/0360-3016(94)00430-S doi: 10.1016/0360-3016(94)00430-S
![]() |
[163] |
A. Farese, T. MacVittie, Filgrastim for the treatment of hematopoietic acute radiation syndrome, Drugs Today (Barc), 51 (2015), 537–548. https://doi.org/10.1358/dot.2015.51.9.2386730 doi: 10.1358/dot.2015.51.9.2386730
![]() |
[164] |
P. Cornes, P. Gascon, S. Chan, K. Hameed, C. Mitchell, P. Field, et al., Systematic review and meta-analysis of short- versus long-acting granulocyte colony-stimulating factors for reduction of chemotherapy-induced febrile neutropenia, Adv. Ther., 35 (2018), 1816–1829. https://doi.org/10.1007/s12325-018-0798-6 doi: 10.1007/s12325-018-0798-6
![]() |
[165] |
Y. Zeng, X. Lv, J. Du, Natural killer cell-based immunotherapy for lung cancer: Challenges and perspectives (review), Oncol. Rep., 46 (2021), 232. https://doi.org/10.3892/or.2021.8183 doi: 10.3892/or.2021.8183
![]() |
[166] |
P. Carrega, B. Morandi, R. Costa, G. Frumento, G. Forte, G. Altavilla, et al., Natural killer cells infiltrating human nonsmall-cell lung cancer are enriched in CD56 bright CD16(-) cells and display an impaired capability to kill tumor cells, Cancer, 112 (2008), 863–875. https://doi.org/10.1002/cncr.23239 doi: 10.1002/cncr.23239
![]() |
[167] |
S. Platonova, J. Cherfils-Vicini, D. Damotte, L. Crozet, V. Vieillard, P. Validire, et al., Profound coordinated alterations of intratumoral NK cell phenotype and function in lung carcinoma, Cancer Res., 71 (2011), 5412–5422. https://doi.org/10.1158/0008-5472.CAN-10-4179 doi: 10.1158/0008-5472.CAN-10-4179
![]() |
[168] |
Y. Laouar, F. Sutterwala, L. Gorelik, R. Flavell, Transforming growth factor-β controls T helper type 1 cell development through regulation of natural killer cell interferon-γ, Nat. Immunol., 6 (2005), 600–607. https://doi.org/10.1038/ni1197 doi: 10.1038/ni1197
![]() |
[169] |
B. Bassani, D. Baci, M. Gallazzi, A. Poggi, A. Bruno, L. Mortara, Natural killer cells as key players of tumor progression and angiogenesis: Old and novel tools to divert their pro-tumor activities into potent anti-tumor effects, Cancers (Basel), 11 (2019), 461. https://doi.org/10.3390/cancers11040461 doi: 10.3390/cancers11040461
![]() |
[170] |
M. Balsamo, W. Vermi, M. Parodi, G. Pietra, C. Manzini, P. Queirolo, et al., Melanoma cells become resistant to NK-cell-mediated killing when exposed to NK-cell numbers compatible with NK-cell infiltration in the tumor, Eur. J. Immunol., 42 (2012), 1833–1842. https://doi.org/10.1002/eji.201142179 doi: 10.1002/eji.201142179
![]() |
[171] |
T. Li, Y. Yang, X. Hua, G. Wang, W. Liu, C. Jia, et al., Hepatocellular carcinoma-associated fibroblasts trigger NK cell dysfunction via PGE2 and IDO, Cancer Lett., 318 (2012), 154–161. https://doi.org/10.1016/j.canlet.2011.12.020 doi: 10.1016/j.canlet.2011.12.020
![]() |
[172] |
T. Li, S. Yi, W. Liu, C. Jia, G. Wang, X. Hua, et al., Colorectal carcinoma-derived fibroblasts modulate natural killer cell phenotype and antitumor cytotoxicity, Med. Oncol., 30 (2013), 663. https://doi.org/10.1007/s12032-013-0663-z doi: 10.1007/s12032-013-0663-z
![]() |
[173] |
C. Granville, R. Memmott, A. Balogh, J. Mariotti, S. Kawabata, W. Han, et al., A central role for Foxp3+ regulatory T cells in K-Ras-driven lung tumorigenesis, PLoS One, 4 (2009), e5061. https://doi.org/10.1371/journal.pone.0005061 doi: 10.1371/journal.pone.0005061
![]() |
[174] |
M. J. Smyth, M. W. Teng, J. Swann, K. Kyparissoudis, D. I. Godfrey, Y. Hayakawa, CD4+ CD25+T regulatory cells suppress NK cell-mediated immunotherapy of cancer, J. Immunol., 176 (2006), 1582–1587. https://doi.org/10.4049/jimmunol.176.3.1582 doi: 10.4049/jimmunol.176.3.1582
![]() |
[175] |
F. Ghiringhelli, C. Menard, M. Terme, C. Flament, J. Taieb, N. Chaput, et al., CD4+CD25+ regulatory T cells inhibit natural killer cell functions in a transforming growth factor-beta-dependent manner, J. Exp. Med., 202 (2005), 1075–1085. https://doi.org/10.1084/jem.20051511 doi: 10.1084/jem.20051511
![]() |
[176] |
A. Page, N. Chuvin, J. Valladeau-Guilemond, S. Depil, Development of NK cell-based cancer immunotherapies through receptor engineering, Cell Mol. Immunol., 21 (2024), 315–331. https://doi.org/10.1038/s41423-024-01145-x doi: 10.1038/s41423-024-01145-x
![]() |
[177] |
J. Markert, P. Liechty, W. Wang, S. Gaston, E. Braz, M. Karrasch, et al., Phase Ib trial of mutant herpes simplex virus G207 inoculated pre-and post-tumor resection for recurrent GBM, Mol. Ther., 17 (2009), 199–207. https://doi.org/10.1038/mt.2008.228 doi: 10.1038/mt.2008.228
![]() |
[178] |
M. Grimes, B. Hall, L. Foltz, T. Levy, K. Rikova, J. Gaiser, et al., Integration of protein phosphorylation, acetylation, and methylation data sets to outline lung cancer signaling networks, Sci. Signal., 11 (2018), eaaq1087. https://doi.org/10.1126/scisignal.aaq1087 doi: 10.1126/scisignal.aaq1087
![]() |
[179] |
J. Qu, Y. Zhang, X. Chen, H. Yang, C. Zhou, N. Yang, Newly developed anti-angiogenic therapy in non-small cell lung cancer, Oncotarget, 9 (2017), 10147–10163. https://doi.org/10.18632/oncotarget.23755 doi: 10.18632/oncotarget.23755
![]() |
[180] |
S. Revels, J. Lee, Anti-angiogenic therapy in nonsquamous non-small cell lung cancer (NSCLC) with tyrosine kinase inhibition (TKI) that targets the VEGF receptor (VEGFR): perspective on phase Ⅲ clinical trials, J. Thorac. Dis., 10 (2018), 617–620. https://doi.org/10.21037/jtd.2018.01.105 doi: 10.21037/jtd.2018.01.105
![]() |
[181] |
M. Stratigos, A. Matikas, A. Voutsina, D. Mavroudis, V. Georgoulias, Targeting angiogenesis in small cell lung cancer, Transl. Lung Cancer Res., 5 (2016), 389–400. https://doi.org/10.21037/tlcr.2016.08.04 doi: 10.21037/tlcr.2016.08.04
![]() |
[182] |
S. Lee, H. Kwak, M. Kang, Y. Park, G. Jeong, Fibroblast-associated tumour microenvironment induces vascular structure-networked tumouroid, Sci. Rep., 8 (2018), 2365. https://doi.org/10.1038/s41598-018-20886-0 doi: 10.1038/s41598-018-20886-0
![]() |
[183] |
B. Lee, J. Konen, S. Wilkinson, A. Marcus, Y. Jiang, Local alignment vectors reveal cancer cell-induced ECM fiber remodeling dynamics, Sci. Rep., 7 (2017), 39498. https://doi.org/10.1038/srep39498 doi: 10.1038/srep39498
![]() |
[184] |
S. Pyonteck, L. Akkari, A. Schuhmacher, R. Bowman, L. Sevenich, D. Quail, et al., CSF-1R inhibition alters macrophage polarization and blocks glioma progression, Nat. Med., 19 (2013), 1264–1272. https://doi.org/10.1038/nm.3337 doi: 10.1038/nm.3337
![]() |
[185] |
S. Arelaki, A. Arampatzioglou, K. Kambas, C. Papagoras, P. Miltiades, I. Angelidou, et al., Gradient infiltration of neutrophil extracellular traps in colon cancer and evidence for their involvement in tumour growth, PLoS One, 11 (2016), e0154484. https://doi.org/10.1371/journal.pone.0154484 doi: 10.1371/journal.pone.0154484
![]() |
[186] |
A. Houghton, D. Rzymkiewicz, H. Ji, A. Gregory, E. Egea, H. Metz, et al., Neutrophil elastase-mediated degradation of IRS-1 accelerates lung tumor growth, Nat. Med., 16 (2010), 219–223. https://doi.org/10.1038/nm.2084 doi: 10.1038/nm.2084
![]() |
[187] |
J. Park, R. Wysocki, Z. Amoozgar, L. Maiorino, M. Fein, J. Jorns, et al., Cancer cells induce metastasis-supporting neutrophil extracellular DNA traps, Sci. Transl. Med., 8 (2016), 361ra138. https://doi.org/10.1126/scitranslmed.aag1711 doi: 10.1126/scitranslmed.aag1711
![]() |
[188] |
A. Chapgier, S. Boisson-Dupuis, E. Jouanguy, G. Vogt, J. Feinberg, A. Prochnicka-Chalufour, et al., Novel STAT1 alleles in otherwise healthy patients with mycobacterial disease, PLoS Genet., 2 (2006), e131. https://doi.org/10.1371/journal.pgen.0020131 doi: 10.1371/journal.pgen.0020131
![]() |
[189] |
P. Klover, W. Muller, G. Robinson, R. Pfeiffer, D. Yamaji, L. Hennighausen, Loss of STAT1 from mouse mammary epithelium results in an increased Neu-induced tumor burden, Neoplasia, 12 (2010), 899–905. https://doi.org/10.1593/neo.10716 doi: 10.1593/neo.10716
![]() |
[190] |
R. Noy, J. Pollard, Tumor-associated macrophages: from mechanisms to therapy, Immunity, 41 (2014), 49–61. https://doi.org/10.1016/j.immuni.2014.06.010 doi: 10.1016/j.immuni.2014.06.010
![]() |
[191] |
A. Mantovani, S. Biswas, M. Galdiero, A. Sica, M. Locati, Macrophage plasticity and polarization in tissue repair and remodelling, J. Pathol., 229 (2013), 176–185. https://doi.org/10.1002/path.4133 doi: 10.1002/path.4133
![]() |
[192] |
B. Yan, J. Wei, Y. Yuan, R. Sun, D. Li, J. Luo, et al., IL-6 cooperates with G-CSF to induce protumor function of neutrophils in bone marrow by enhancing STAT3 activation, J. Immunol., 190 (2013), 5882–5893. https://doi.org/10.4049/jimmunol.1201881 doi: 10.4049/jimmunol.1201881
![]() |
[193] |
K. Steinbach, P. Schick, F. Trepel, H. Raffler, J. Dohrmann, G. Heilgeist, et al., Estimation of kinetic parameters of neutrophilic, eosinophilic, and basophilic granulocytes in human blood, Blut, 39 (1979), 27–38. https://doi.org/10.1007/BF01008072 doi: 10.1007/BF01008072
![]() |
[194] |
D. Dale, W. C. Liles, C. Llewellyn, E. Rodger, T. H. Price, Neutrophil transfusions: kinetics and functions of neutrophils mobilized with granulocyte-colony-stimulating factor and dexamethasone, Transfusion, 38 (1998), 713–721. https://doi.org/10.1046/j.1537-2995.1998.38898375509.x doi: 10.1046/j.1537-2995.1998.38898375509.x
![]() |
[195] |
Y. Kim, H. Othmer, A hybrid model of tumor-stromal interactions in breast cancer, Bull. Math. Biol., 75 (2013), 1304–1350. https://doi.org/10.1007/s11538-012-9787-0 doi: 10.1007/s11538-012-9787-0
![]() |
[196] |
J. Diao, E. Winter, C. Cantin, W. Chen, L. Xu, D. Kelvin, et al., In situ replication of immediate dendritic cell (DC) precursors contributes to conventional DC homeostasis in lymphoid tissue, J. Immunol., 176 (2006), 7196–7206. https://doi.org/10.4049/jimmunol.176.12.7196 doi: 10.4049/jimmunol.176.12.7196
![]() |
[197] |
P. Salmon, J. L. Cotonnec, A. Galazka, A. Abdul-Ahad, A. Darragh, Pharmacokinetics and pharmacodynamics of recombinant human interferon-beta in healthy male volunteers, J. Interferon Cytokine Res., 16 (1996), 759–964. https://doi.org/10.1089/jir.1996.16.759 doi: 10.1089/jir.1996.16.759
![]() |
[198] |
Y. Zhang, D. Wallace, C. de Lara, H. Ghattas, B. Asquith, A. Worth, et al., In vivo kinetics of human natural killer cells: the effects of ageing and acute and chronic viral infection, Immunology, 121 (2007), 258–265. https://doi.org/10.1111/j.1365-2567.2007.02573.x doi: 10.1111/j.1365-2567.2007.02573.x
![]() |
[199] |
K. Stringaris, Orphan NKs! the mystery of the self-renewing NK cells, Blood, 129 (2017), 1890–1891. https://doi.org/10.1182/blood-2016-12-755546 doi: 10.1182/blood-2016-12-755546
![]() |
[200] |
N. Stute, V. Santana, J. Rodman, M. Schell, J. Ihle, W. Evans, Pharmacokinetics of subcutaneous recombinant human granulocyte colony-stimulating factor in children, Blood, 79 (1992), 2849–2854. https://doi.org/10.1182/blood.V79.11.2849.2849 doi: 10.1182/blood.V79.11.2849.2849
![]() |
[201] |
E. Shochat, V. Rom-Kedar, L. Segel, G-CSF control of neutrophils dynamics in the blood, Bull. Math. Biol., 69 (2007), 2299–2338. https://doi.org/10.1007/s11538-007-9221-1 doi: 10.1007/s11538-007-9221-1
![]() |
[202] |
L. Deng, H. Liang, M. Xu, X. Yang, B. Burnette, A. Arina, et al., Sting-dependent cytosolic dna sensing promotes radiation-induced type Ⅰ interferon-dependent antitumor immunity in immunogenic tumors, Immunity, 41 (2014), 843–52. https://doi.org/10.1016/j.immuni.2014.10.019 doi: 10.1016/j.immuni.2014.10.019
![]() |
[203] |
J. Andrejeva, D. Young, S. Goodbourn, R. Randall, Degradation of STAT1 and STAT2 by the Ⅴ proteins of simian virus 5 and human parainfluenza virus type 2, respectively: consequences for virus replication in the presence of alpha/beta and gamma interferons, J. Virol., 76 (2002), 2159–2167. https://doi.org/10.1128/jvi.76.5.2159-2167.2002 doi: 10.1128/jvi.76.5.2159-2167.2002
![]() |
[204] | L. Yang, R. Wang, Z. Ma, Y. Xiao, Y. Nan, Y. Wang, et al., Porcine reproductive and respiratory syndrome virus antagonizes JAK/STAT3 signaling via nsp5 by inducing STAT3 degradation, J. Virol., 91 (2017), e02087–16. |
[205] |
R. Rooswinkel, B. van de Kooij, E. de Vries, M. Paauwe, R. Braster, et al., Antiapoptotic potency of Bcl-2 proteins primarily relies on their stability, not binding selectivity, Blood, 123 (2014), 2806–2815. https://doi.org/10.1182/blood-2013-08-519470 doi: 10.1182/blood-2013-08-519470
![]() |
[206] |
M. Blagosklonny, M. Alvarez, A. Fojo, L. Neckers, bcl-2 protein downregulation is not required for differentiation of multidrug resistant HL60 leukemia cells, Leuk. Res., 20 (1996), 101–107. https://doi.org/10.1016/0145-2126(95)00103-4 doi: 10.1016/0145-2126(95)00103-4
![]() |
[207] |
S. Magal, A. Jackman, S. Ish-Shalom, L. Botzer, P. Gonen, R. Schlegel, et al., Downregulation of Bax mRNA expression and protein stability by the E6 protein of human papillomavirus 16, J. Gen. Virol., 86 (2005), 611–621. https://doi.org/10.1099/vir.0.80453-0 doi: 10.1099/vir.0.80453-0
![]() |
[208] |
M. Xin, X. Deng, Nicotine inactivation of the proapoptotic function of Bax through phosphorylation, J. Biol. Chem., 280 (2005), 10781–10789. https://doi.org/10.1074/jbc.M500084200 doi: 10.1074/jbc.M500084200
![]() |
[209] |
E. Gaffney, K. Pugh, P. Maini, F. Arnold, Investigating a simple model of cutaneous wound healing angiogenesis, J. Math. Biol., 45 (2002), 337–374. https://doi.org/10.1007/s002850200161 doi: 10.1007/s002850200161
![]() |
[210] |
G. Pettet, H. Byrne, D. McElwain, J. Norbury, A model of wound-healing angiogenesis in soft tissue, Math. Biosci., 136 (1996), 35–63. https://doi.org/10.1016/0025-5564(96)00044-2 doi: 10.1016/0025-5564(96)00044-2
![]() |
[211] |
P. Moghe, R. Nelson, R. Tranquillo, Cytokine-stimulated chemotaxis of human neutrophils in a 3-D conjoined fibrin gel assay, J. Immunol. Methods, 180 (1995), 193–211. https://doi.org/10.1016/0022-1759(94)00314-M doi: 10.1016/0022-1759(94)00314-M
![]() |
[212] |
D. Brown, Dependence of neurones on astrocytes in a coculture system renders neurones sensitive to transforming growth factor beta1-induced glutamate toxicity, J. Neurochem., 72 (1999), 943–953. https://doi.org/10.1046/j.1471-4159.1999.0720943.x doi: 10.1046/j.1471-4159.1999.0720943.x
![]() |
[213] | S. Koka, J. Vance, G. Maze, Bone growth factors: potential for use as an osseointegration enhancement technique (OET), J. West Soc. Periodontol. Periodontal. Abstr., 43 (1995), 97–104. |
[214] |
E. Woodcock, S. Land, R. Andrews, A low affinity, low molecular weight endothelin-a receptor present in neonatal rat heart, Clin. Exp. Pharmacol. Physiol., 20 (1993), 331–334. https://doi.org/10.1111/j.1440-1681.1993.tb01697.x doi: 10.1111/j.1440-1681.1993.tb01697.x
![]() |
[215] |
M. Serizawa, T. Takahashi, N. Yamamoto, Y. Koh, Combined treatment with erlotinib and a transforming growth factor-β type Ⅰ receptor inhibitor effectively suppresses the enhanced motility of erlotinib-resistant non-small-cell lung cancer cells, J. Thorac. Oncol., 8 (2013), 259–269. https://doi.org/10.1097/JTO.0b013e318279e942 doi: 10.1097/JTO.0b013e318279e942
![]() |
[216] |
J. Maher, R. Zhang, G. Palanisamy, K. Perkins, L. Liu, P. Brassil, et al., Lung-restricted ALK5 inhibition avoids systemic toxicities associated with TGFβ pathway inhibition, Toxicol. Appl. Pharmacol., 438 (2022), 115905. https://doi.org/10.1016/j.taap.2022.115905 doi: 10.1016/j.taap.2022.115905
![]() |
[217] |
L. Spender, G. J. Ferguson, G. Hughes, B. Davies, F. Goldberg, B. Herrera, et al., Preclinical evaluation of AZ12601011 and AZ12799734, inhibitors of transforming growth factor β superfamily type 1 receptors, Mol. Pharmacol., 95 (2019), 222–234. https://doi.org/10.1124/mol.118.112946 doi: 10.1124/mol.118.112946
![]() |
[218] |
A. Friedman, J. P. Tian, G. Fulci, E. A. Chiocca, J. Wang, Glioma virotherapy: effects of innate immune suppression and increased viral replication capacity, Cancer Res., 66 (2006), 2314–2319. https://doi.org/10.1158/0008-5472.CAN-05-2661 doi: 10.1158/0008-5472.CAN-05-2661
![]() |
[219] |
X. Liu, Z. Wang, Y. Yang, L. Wang, R. Sun, Y. Zhao, et al., Active components with inhibitory activities on IFN-γ/STAT1 and IL-6/ATAT3 signaling pathways from caulis trachelospermi, Molecules, 19 (2014), 11560–11571. https://doi.org/10.3390/molecules190811560 doi: 10.3390/molecules190811560
![]() |
[220] |
L. Chen, S. Willis, A. Wei, B. Smith, J. Fletcher, M. Hinds, et al., Differential targeting of prosurvival Bcl-2 proteins by their BH3-only ligands allows complementary apoptotic function, Mol. Cell, 17 (2005), 393–403. https://doi.org/10.1016/j.molcel.2004.12.030 doi: 10.1016/j.molcel.2004.12.030
![]() |
[221] |
M. Shanmugam, H. Shen, F. Tang, F. Arfuso, M. Rajesh, L. Wang, et al., Potential role of genipin in cancer therapy, Pharmacol. Res., 133 (2018), 195–200. https://doi.org/10.1016/j.phrs.2018.05.007 doi: 10.1016/j.phrs.2018.05.007
![]() |
[222] |
P. Gascon, U. Fuhr, F. Sorgel, M. Kinzig-Schippers, A. Makhson, S. Balser, et al., Development of a new G-CSF product based on biosimilarity assessment, Ann. Oncol., 21 (2010), 1419–1429. https://doi.org/10.1093/annonc/mdp574 doi: 10.1093/annonc/mdp574
![]() |
[223] |
Z. Tekdemir, A. Seckin, T. Kacar, E. Yilmaz, S. Bekiroglu, Evaluation of structural, biological, and functional similarity of biosimilar granulocyte colony stimulating factor to its reference product, Pharm. Res., 37 (2020), 215. https://doi.org/10.1007/s11095-020-02932-7 doi: 10.1007/s11095-020-02932-7
![]() |
1. | Rodrigo Moreira, Larissa Ferreira Rodrigues Moreira, Flávio de Oliveira Silva, Brazilian Selic Rate Forecasting with Deep Neural Networks, 2024, 0927-7099, 10.1007/s10614-024-10597-2 | |
2. | Michele Bufalo, Claudia Ceci, Giuseppe Orlando, Addressing the financial impact of natural disasters in the era of climate change, 2024, 73, 10629408, 102152, 10.1016/j.najef.2024.102152 | |
3. | Guo-Hui Yang, Si-Qi Ma, Xiao-Dong Bian, Jiang-Cheng Li, Pierluigi Vellucci, The roles of liquidity and delay in financial markets based on an optimal forecasting model, 2023, 18, 1932-6203, e0290869, 10.1371/journal.pone.0290869 | |
4. | Giacomo Ascione, Michele Bufalo, Giuseppe Orlando, Modeling volatility of disaster-affected populations: A non-homogeneous geometric-skew Brownian motion approach, 2024, 130, 10075704, 107761, 10.1016/j.cnsns.2023.107761 |