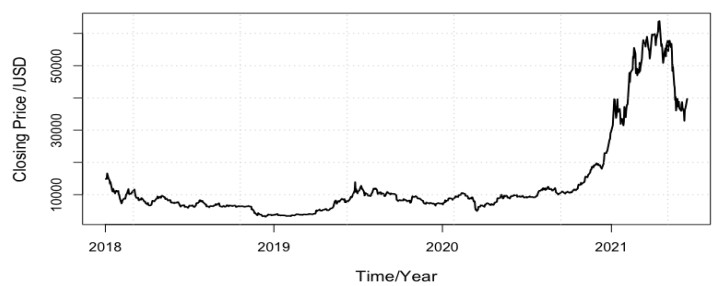
The Bitcoin futures market is growing and, as such, becoming more sophisticated. A small change in price may therefore have a large impact on the market. This paper investigates the propensity of 18 different competing GARCH family models and error distributions to model and forecast the volatility of Bitcoin futures returns. The study employs two different time periods (from January 2, 2018 to June 14, 2021; and March 11, 2020 to June 14, 2021). From the results, iGARCH(1, 1)-Students't-distribution (STD) is selected as the best performing model among the constructed models for the first period. By fitting the best three models from the first period to the second period, the iGARCH(1, 1)-STD is again selected as the optimal model. However, the iGARCH(1, 1)-normal inverse Gaussian (NIG) provides a significant variance forecast when used for in-sample and out-of-sample forecasts before the financial crisis and during the financial crisis, respectively. Our results indicate the impacts of past squared shocks on squared returns of Bitcoin futures and the ability of iGARCH(1, 1)-STD to capture such innovations and the propensity of iGARCH(1, 1)-NIG to optimally forecast the variance of Bitcoin futures returns.
Citation: Samuel Asante Gyamerah, Collins Abaitey. Modelling and forecasting the volatility of bitcoin futures: the role of distributional assumption in GARCH models[J]. Data Science in Finance and Economics, 2022, 2(3): 321-334. doi: 10.3934/DSFE.2022016
[1] | Nitesha Dwarika . The risk-return relationship in South Africa: tail optimization of the GARCH-M approach. Data Science in Finance and Economics, 2022, 2(4): 391-415. doi: 10.3934/DSFE.2022020 |
[2] | Moses Khumalo, Hopolang Mashele, Modisane Seitshiro . Quantification of the stock market value at risk by using FIAPARCH, HYGARCH and FIGARCH models. Data Science in Finance and Economics, 2023, 3(4): 380-400. doi: 10.3934/DSFE.2023022 |
[3] | Changlin Wang . Different GARCH model analysis on returns and volatility in Bitcoin. Data Science in Finance and Economics, 2021, 1(1): 37-59. doi: 10.3934/DSFE.2021003 |
[4] | Deniz Sevinç . Volatility spillovers among MIST stock markets. Data Science in Finance and Economics, 2022, 2(2): 80-95. doi: 10.3934/DSFE.2022004 |
[5] | Changlin Wang . Correction: Different GARCH models analysis of returns and volatility in Bitcoin. Data Science in Finance and Economics, 2022, 2(3): 205-208. doi: 10.3934/DSFE.2022010 |
[6] | Katleho Makatjane, Ntebogang Moroke . Examining stylized facts and trends of FTSE/JSE TOP40: a parametric and Non-Parametric approach. Data Science in Finance and Economics, 2022, 2(3): 294-320. doi: 10.3934/DSFE.2022015 |
[7] | Morten Risstad, Mathias Holand . On the relevance of realized quarticity for exchange rate volatility forecasts. Data Science in Finance and Economics, 2024, 4(4): 514-530. doi: 10.3934/DSFE.2024021 |
[8] | Kuo-Shing Chen . Interlinkages between Bitcoin, green financial assets, oil, and emerging stock markets. Data Science in Finance and Economics, 2024, 4(1): 160-187. doi: 10.3934/DSFE.2024006 |
[9] | Wojciech Kuryłek . Can we profit from BigTechs' time series models in predicting earnings per share? Evidence from Poland. Data Science in Finance and Economics, 2024, 4(2): 218-235. doi: 10.3934/DSFE.2024008 |
[10] | Xiaozheng Lin, Meiqing Wang, Choi-Hong Lai . A modification term for Black-Scholes model based on discrepancy calibrated with real market data. Data Science in Finance and Economics, 2021, 1(4): 313-326. doi: 10.3934/DSFE.2021017 |
The Bitcoin futures market is growing and, as such, becoming more sophisticated. A small change in price may therefore have a large impact on the market. This paper investigates the propensity of 18 different competing GARCH family models and error distributions to model and forecast the volatility of Bitcoin futures returns. The study employs two different time periods (from January 2, 2018 to June 14, 2021; and March 11, 2020 to June 14, 2021). From the results, iGARCH(1, 1)-Students't-distribution (STD) is selected as the best performing model among the constructed models for the first period. By fitting the best three models from the first period to the second period, the iGARCH(1, 1)-STD is again selected as the optimal model. However, the iGARCH(1, 1)-normal inverse Gaussian (NIG) provides a significant variance forecast when used for in-sample and out-of-sample forecasts before the financial crisis and during the financial crisis, respectively. Our results indicate the impacts of past squared shocks on squared returns of Bitcoin futures and the ability of iGARCH(1, 1)-STD to capture such innovations and the propensity of iGARCH(1, 1)-NIG to optimally forecast the variance of Bitcoin futures returns.
The first Bitcoin futures were offered on December 18, 2017 by the Chicago Board of Options Exchange (CBOE) Cheng (2017) and have already gained control in the market. Investors who could not hold spot positions in the Bitcoin market because of compliance regulations can now trade on the Bitcoin futures market because they are traded on an exchange regulated market with very low margin requirements (Baur and Dimpfl, 2019). Bitcoin futures are cash settled without the need for a Bitcoin wallet and do serve as a hedging instrument. Similarly to commodities/stocks futures, Bitcoin futures permit investors to speculate on falling prices ("go short"). According to Shiller (2017), the inability to go short was the reason for the bubble-like evolution of Bitcoin price in 2017. In 2017, the price of Bitcoin doubled four times leading to uncertainty in the price of Bitcoin. Volatility which relates to uncertainty about an assets value is therefore significant when modelling the price of an asset. The concept of volatility is applied in different financial and economic areas such as asset pricing, monetary policy, derivatives trading, and risk management. Volatility in the case of market uncertainty serves as a measure of consumers' and investors' confidence in the market. Volatility modelling and forecasting is therefore important in decision-making process of most investors on the futures market. The Bitcoin futures market is growing and as such becoming more sophisticated. A small change in price may therefore have large impact on the market.
Contrary to the vast number of studies on volatility modeling and forecasting of Bitcoin spot returns (e.g. Katsiampa, 2017; Naimy and Hayek, 2018; Gyamerah, 2019) in the literature, there is scarcity of research on the volatility of Bitcoin futures return. In this paper, we contribute to the limited studies and literature on the volatility of Bitcoin futures returns Guo (2021a, b). Volatility modeling of Bitcoin return series using time-varying GARCH models has gained a lot of attention. Katsiampa (2017) explored the propensity of different generalized auto-regressive conditional heteroskedasticity (GARCH) type models to account for the volatility in Bitcoin price returns. They concluded that the AR-CGARCH model is the best fit model with respect to goodness-of-fit to the data. Naimy and Hayek (2018) estimated and compared the forecasting ability of different GARCH type models and concluded that the exponential GARCH(1, 1) outperforms standard GARCH(1, 1) model for both in-sample and out-of-sample forecast. Using Bitcoin return series from January 01, 2014 to August 16, 2014, Gyamerah (2019) concluded that threshold GARCH with normal inverse Gaussian error distribution is the optimal model to model and estimate the volatility in Bitcoin return series. By studying the volatility term structure, Guo (2021b) noticed that the price volatility of Bitcoin futures minimizes as the delivery date draws nearer. Their result is in contrast to what is normally observed in the commodity futures market, called the Samuelson effect. On the aspect of GARCH modeling, Guo (2021a) used GARCH type family models to explore the quantitative risk management of Bitcoin futures via Value at Risk. They captured the residuals of Bitcoin futures return using the normal inverse Gaussian distribution since it fully explained the heavy-tailed distribution of the residuals. In their study on application of GARCH models on Bitcoin futures option, Venter and Maré (2021) concluded that symmetric Heston-Nandi model is the optimal model for Bitcoin futures option.
This study therefore seeks to model and forecast the volatility of Bitcoin futures returns. Specifically, the study attempts to use different GARCH family models and different error distributions (Student's t distribution, normal inverse Gaussian, and generalized error distribution) to model and forecast the volatility of Bitcoin futures returns from January 02, 2018 to June 14, 2021, which includes the 2020 financial crisis. From previous studies explored in the literature and to the best of our knowledge, this paper is the first of its kind to comprehensively investigate the relative performance of six (6) different GARCH family models and three (3) different error distributions. This paper derives its motivation from the fact that Bitcoin futures as a financial asset are gaining a lot of attention in the cryptocurrency market. The interrupted transactions in futures contracts from December, 2017 with growing trading volumes affirm the exceptional influence of Bitcoin futures.
This paper is organized as follows: Section 2 discussues the methods used for the study; Section 3 presents the descriptive statistics analysis; Section 4 presents the empirical analysis; and section 5 concudes the study.
The data of Bitcoin futures are downloaded from Yahoo Finance (https://finance.yahoo.com/) and cover the period from the period 02/02/2018 to 14/06/2021. This period covers the first day that Bitcoin futures data was publicly available on Yahoo Finance to the time this study was conducted. Returns (logarithmic returns, Rt) are computed from the historical adjusted closing price of Bitcoin futures using Equation 1,
Rt=log(PtPt−1) | (1) |
Logarithmic returns are used because of their statistical properties such as ergocidity and stationarity. It should be noted that Bitcoin futures with the ticker BTC=F was taken because of the historical completeness of the data. The Shapiro-Wilk and Anderson-Darling tests are used to test for the normality of the residuals of the returns. The ARCH Lagrange multiplier (ARCH LM) and Ljung Box (LB) are employed to test for the auto-regressive conditional heteroskedasticity (ARCH) effects in the returns data of Bitcoin futures.
To specify the variance equation to model the presence of volatility in differenced logarithmic futures prices (i.e., futures price returns), we used six different GARCH family models, each of which has a different purpose. In particular, we modeled the variance for a constant mean equation using standard GARCH (sGARCH), threshold GARCH (tGARCH), integrated GARCH (iGARCH), exponential GARCH (eGARCH), asymmetric Power GARCH (apARCH) and Glosten-Jagannathan-Runkle GARCH (gjr-GARCH) models. The sensitivity of the estimation results of the models was checked by changing the distribution assumptions. More specifically, the sensitivity and appropriateness of the estimation results were observed by changing the distribution assumption to the Student's t-distribution (STD), generalized error distribution (GED), and the normal inverse Gaussian (NIG) distribution.
The standard GARCH (sGARCH) model is a generalization of ARCH model where it allows the conditional volatility to be dependent upon its own lags. Suppose p and q are the number of lagged squared return included and the number of lagged volatilities, then sGARCH(p, q) model is defined as
σ2t=ω+p∑m=1αmϵ2t−m+q∑n=1βnσ2t−n, | (2) |
where ω>0, αm>0, βn≥0 and for αm+βn<1, sGARCH(1, 1) is covariance stationary.
The conditional variance specification of tGARCH(p, q) is given as:
σ2t=ω+p∑m=1(αmϵ2t−m+γmIt−mϵ2t−m)+q∑n=1βnσ2t−n | (3) |
where
It−m={1,ifϵt−m<0(badnews)0,ifϵt−m≥0(goodnews) | (4) |
The effects of good news is αm and bad news is αm+γm. Leverage and asymmetry exist when γm>0 and γm≠0 respectively. The leverage effect in the model helps to differentiate negative and positive shocks.
The iGARCH model is persistent in variance due to the fact that present information remains important for different forecasting horizons.
σ2t=ω+p∑mβmσ2t−m+q∑n(1−βn)σ2t−n, | (5) |
where αm+βn=1
Developed by Developed by Nelson (1991), the Exponential GARCH (eGARCH) model integrate the asymmetries in how volatility reacts to past returns. An eGARCH(p, q) model assumes the form
log(σ2t)=ω+P∑m=1αm|bt−m|+γmbt−m+q∑n=1βnlog(σ2t−n), | (6) |
where bt=ϵt−mσt−m. γm is the leverage or asymmetric effect parameter. For eGARCH models, it is not necessary to impose non-negativity constraints since the modeling is done on the logarithm of the conditional volatility it is covariance stationary when |βn|<1.
Developed by Developed by Ding et al. (1993), apARCH is capable of capturing power effects, asymmetries, and different structures that exists in the data. A apARCH(p, q) model is given as:
σδt=ω+p∑m=1αm(|ϵt−m|−γmϵt−m)δ+q∑n=1βnσδt−n, | (7) |
where ω>0,δ≥0,αm≥0,βn≥0,−1≤γm≤1. δ and γm denote Box-Cox transformation of the conditional standard deviation and the leverage effect respectively. Negative innovation has an effect of αm+γm and positive innovation has an effect of αm.
Similar to the eGARCH model, the gjrGARCH model is also able to capture asymmetries in volatility. The gjrGARCH model was developed by Glosten et al. (1993) and it is an extension of sGARCH model with an added term which helps to capture any asymmetries. The extra term is zero if the return is greater than zero. Define a gjrGARCH(p, q) model as
σ2t=ω+p∑m=1(αmϵ2t−m+γmIt−mϵ2t−m)+q∑n=1βnσ2t−n | (8) |
where
It−m={1,ifϵt−1≤00,otherwise | (9) |
where ω>0,αm>0,βn≥0, αm+γm≥0 and gjrGARC(1, 1) is covariance stationary for γm<2(1−αm−βn)
In this study, maximum likelihood estimator is used to estimating the parameters of the GARCH models.
There is strong evidence that suggests that GARCH models with non-normal distributions are more robust in what comes to volatility forecasting than other historical models (Liu and Morley, 2009).
From Table 2, it is clear that the random shocks (ϵt) of Bitcoin futures return are not normally distributed. It is therefore appropriate to consider other types of distribution that can capture the non-normality in the residual data. In this study, three distributions are considered: Generalized Error Distribution, Student-t distribution and Normal Inverse Gaussian (NIG) types of distribution. These distributions are capable of capturing any asymmetric effect, fat tails, and peaks in the residual data (Gyamerah, 2019; Guo, 2021a).
Different information criterion (Akaike Information Criterion (AIC) and Schwarz-Bayesian Information Criterion (BIC)) are used to compare the fit of the GARCH models. In selecting the best fit model among the GARCH models, the model with the lowest AIC or BIC values is selected as the best model.
In this study, two evaluation metrics(mean squared error (MSE) and mean absolute error (MAE)) are used to evaluate the forecasting accuracy of the best three GARCH models, each obtained from the error distributions. The lower the MAE value and MSE value, the better the forecasting accuracy of that model.
MAE=1NN∑i=1|σi−^σi| | (10) |
MSE=1NN∑i=1(σi−^σi)2, | (11) |
where N is the total number of forecasting horizon, σ and ^σi are the the observed volatility (absolute value of returns) and the forecast of the volatility.
Figure 1 shows the price dynamics between 02/01/2018 to 14/06/2021. The minimum and maximum futures prices are, respectively, 3145 and 63840. These values were recorded on 14/12/2018 and 15/04/2021 respectively. The maximum price occurred about a month after WHO declared COVID-19 as a pandemic. From Table 1, the skewness (2.211) and kurtosis (3.682) values of Bitcoin futures price indicate peakness in its price distribution. With a standard deviation of 13940, the price distribution is spread out over a wider range.
N | Min | Max | Mean | SE Mean | Median | Skewness | Kurtosis | SD | AD test | SW test | |
Bitcoin (BTCF)-Price | 860 | 3145 | 63840 | 13730 | 475.3 | 8932 | 2.211 | 3.682 | 13940 | p< 2e-16 | p< 2e-16 |
Bitcoin (BTCF)-Return | 859 | -0.267726 | 0.222358 | 0.001145 | 0.001650 | 0.000801 | -0.370932 | 4.537262 | 0.048363 | p< 2e-16 | p< 2e-16 |
N=number of sample; Min=Minimum; Max = Maximum; SE Mean =Standard Error of Mean; SD=Standard Deviation |
From Table 1, the returns of Bitcoin futures have a daily mean of 0.001145 and a daily volatility of 0.048363. That is, the daily mean is about 2.37% of the daily volatility. The kurtosis value is high for Bitcoin futures returns, indicating the presence of extreme values in the return distribution. The skewness value points toward asymmetrical properties of the returns' distribution. This is validated by the Anderson-Darling (AD) and Shapiro-Wilk (SW) tests (see Table 2). At 5% significance level, AD and SW tests reject the null hypothesis that the returns of Bitcoin futures are normally distributed. These indicate that the returns of Bitcoin futures are not likely to be normally distributed.
Shapiro-Wilk | Anderson-Darling | |
P-Value | <2e-16 | <2e-16 |
Test Statistics | 0.93 | 16 |
Test was conducted on 5% α level of significance |
Figure 2 presents the plot of the returns series and 95% confidence interval of the returns series. Clearly, we are 95% confident that the returns of Bitcoin futures will be between 0.09479 and −0.09479. For the period under consideration, the maximum positive and negative returns occurred on 13/05/2019 and 12/03/2020 and with values of 0.2223577 and −0.2677255 respectively. These values have been indicated in Figure 2 (right plot). From this, we can easily detect the financial crisis in 2020 with a sharp negative return. Clearly, there is also a pattern of volatility clustering in the returns series.
At 5% level of significance, the null hypothesis of normally distributed residuals is rejected using both the SW and AD tests (see Table 2). We can therefore conclude that the residuals are not normally distributed.
From Table 3, stationarity is rejected at 5% significance level. Using the ARCH LM test and at 5% level of significance, we can reject the null hypothesis of no ARCH effects. Also, at 5% level of significance, we can reject the null hypothesis of independently distributed squared residuals of Bitcoin futures return when the Ljung Box test is used. Both LM and Ljung Box test confirm the presence of ARCH effects in Bitcoin futures returns; hence we can employ GARCH-type family models to model the return series of Bitcoin futures.
ARCH Lagrange Multiplier (LM) | Ljung Box | ADF | |
P-Value | 0.00161 | 2e-08 | 0.01 |
Test Statistics | 54 | 1.1 | -20 |
To evaluate the performance of different GARCH models in capturing the volatility in Bitcoin futures returns, we tested the performance of the GARCH models in the time intervals 02/01/2018 to 14/06/2021 and from 11/03/2020 to 14/06/2021. The first time interval covers the first time that Bitcoin futures data was publicly available on Yahoo Finance from the time this study was conducted. The second time interval covers the period when the World Health Organization (WHO) declared COVID-19 as a pandemic, which caused the 2020 financial crisis. Since the ARCH effect was detected in the model, GARCH estimation was performed with different error specifications. The Tables' 4-6 present the estimation results of GARCH family models with the three distributional assumptions for the return series of Bitcoin futures. The maximum likelihood parameter estimates and the associated standard errors, AIC, and BIC values are reported in these tables. The GARCH models were compared relative to each other using the information criteria AIC and BIC. The BIC penalizes the number of parameters more heavily than the AIC and therefore favours simpler models. The standard errors of the estimates are presented in parentheses.
The sGARCH model was applied for the purpose of capturing variance dynamic. Clearly, from Table A1, the stationary condition of α1+β1<1 for sGARCH(1, 1) has been met, hence GARCH process for all the distribution assumptions are weakly stationary. The ARCH term (α1) and GARCH term (β1) are positive for all the distribution errors.
Table 4 reports the results of fitting a different GARCH(1, 1) model with conditionally t-distributed innovations for daily Bitcoin futures returns. For GARCH(1, 1) models with conditionally t-distributed errors, the iGARCH(1, 1) has the lowest AIC and BIC values. The QQ plot in Figure A1 is in congruous with earlier observational values of AIC and BIC for iGARCH(1, 1)-STD model. These results demonstrate that iGARCH(1, 1)-STD is optimal in modeling the effects of past squared shocks on the squared returns and to also capture the residuals distribution in the return series of Bitcoin futures. All the estimated parameters of iGARCH(1, 1)-STD model are statistically significant at 5% significance level. The sum of α1=0.106635 and β1=0.893365 is equal to 1 as required for any iGARCH model, which indicates persistence of volatility. With an α1=0.106635, Bitcoin futures return is jumpy. News of Bitcoin futures about past volatility have an explanatory power on current volatility. This is evident from the positive and significant coefficients of α1 and β1.
Model | Estimated Parameters | |||||||||
μ | ω | α1 | β1 | η11 | γ1 | δ | shape | AIC | BIC | |
sGARCH(1, 1) | 0.001664∗ | 0.000060∗ | 0.105875 | 0.893125 | - | - | - | 2.999262 | -3.5373 | -3.5096 |
(0.001069) | (0.000033) | (0.026727) | (0.021343) | (-) | (-) | (-) | (0.294213) | |||
tGARCH(1, 1) | 0.001252 | 0.001258 | 0.163539 | 0.886712 | -0.086243 | - | - | 2.672477 | -3.5390 | -3.5058 |
(0.001254) | (0.000716) | (0.037106) | (0.021241) | (0.113943) | (-) | (0.277959) | (0.277959) | |||
iGARCH(1, 1) | 0.001663∗ | 0.000059∗∗∗ | 0.106635 | 0.893365 | - | - | - | 2.990437 | -3.5397 | -3.5175 |
(0.001068) | (0.000030) | (0.020543) | (-) | (-) | (-) | (-) | (0.213747) | |||
eGARCH(1, 1)) | 0.001303 | -0.112149 | 0.015673∗ | 0.980604 | - | 0.278848 | - | 2.721974 | -3.5427 | -3.5095 |
(0.000289) | (0.041624) | (0.030645) | (0.007757) | (-) | (0.067328) | (-) | (0.305861) | |||
apARCH(1, 1)) | 0.001340∗ | 0.000588∗ | 0.163180 | 0.890757 | - | −0.071496∗ | 1.213898∗ | 2.694736 | -3.5371 | -3.4983 |
(0.001107) | (0.001094) | (0.045643) | (0.017330) | (-) | (0.130387) | (0.590110) | (0.311436) | |||
gjrGARCH(1, 1)) | 0.001676∗ | 0.000059∗∗ | 0.108898∗∗∗ | 0.893594 | - | −0.006984∗ | - | 3.005712 | -3.5350 | -3.5018 |
(0.001084) | (0.000034) | (0.034112) | (0.021714) | (-) | (0.038282) | (-) | (0.293892) |
With the lowest AIC and BIC values of −3.5353 and −3.5132 respectively, iGARCH(1, 1)-GED is the optimal model among all the GARCH(1, 1)-GED models (See Table 5).
Model | Estimated Parameters | |||||||||
μ | ω | α1 | β1 | η11 | γ1 | δ | shape | AIC | BIC | |
sGARCH(1, 1) | 0.000681 | 0.000076∗∗∗ | 0.086968 | 0.884133 | - | - | - | 0.900692 | -3.5359 | -3.5082 |
(0.000154) | (0.000034) | (0.023399) | (0.027653) | (-) | (-) | (-) | (0.053196) | |||
TGARCH(1, 1) | 0.000000∗ | 0.002391∗∗∗ | 0.128371 | 0.862617 | −0.015097∗ | - | - | 0.876118 | -3.5318 | -3.4986 |
(0.000048) | (0.000939) | (0.028177) | (0.031352) | (0.129982) | (-) | (-) | (0.052555) | |||
iGARCH(1, 1) | 0.000000∗ | 0.000053∗∗∗ | 0.111739 | 0.888261 | - | - | - | 0.848831 | -3.5353 | -3.5132 |
(0.000044) | (0.000024) | (0.026253) | (-) | (-) | (-) | (-) | (0.047908) | |||
eGARCH(1, 1)) | 0.00000∗ | -0.29120 | −0.00426∗ | 0.95283 | - | 0.22056 | - | 0.87908 | -3.5349 | -3.5017 |
(0.000049) | (0.101340) | (0.026079) | (0.016309) | (-) | (0.043414) | (-) | (0.052749) | |||
apARCH(1, 1)) | 0.000493∗∗∗ | 0.000187∗ | 0.100026 | 0.879429 | - | 0.022567∗ | 1.737045 | 0.898400 | -3.5315 | -3.4927 |
(0.000203) | (0.000240) | (0.030610) | (0.028709) | (-) | (0.104785) | (0.391820) | (0.053134) | |||
gjrGARCH(1, 1)) | 0.000000∗ | 0.000079∗∗∗ | 0.082188 | 0.882615 | - | 0.011581∗ | - | 0.891387 | -3.5339 | -3.5007 |
(0.000053) | (0.000035) | (0.028770) | (0.028068) | (-) | (0.034613) | (-) | (0.053999) |
The best performing model among the different GARCH-NIG models is iGARCH-NIG. This is evident from its lowest AIC and BIC values of −3.5416 and −3.5139 respectively.
The best GARCH model from Table 4(iGARCH(1, 1)-STD), Table 5(iGARCH(1, 1)-GED) and Table 6(iGARCH(1, 1)- NIG) are fitted to daily Bitcoin futures from 11/03/2020 to 14/06/2021. This is necessary to help select the optimal model that can effectively model the volatility and capture the residuals of Bitcoin futures return during financial crisis. From Table 7, iGARCH(1, 1)-STD is selected as the optimal model since it has the lowest AIC and BIC values. The propensity of the student-t distribution to capture the residual series of Bitcoin futures return are in congruence to the results obtained by Wilhelmsson (2006). Wilhelmsson (2006) concluded in their studies that using leptokurtic error distribution to capture the residuals in Standard and Poor's 500 index futures return is appropriate in variance forecast as compared to normal distribution.
Model | Estimated Parameters | ||||||||||
μ | ω | α1 | β1 | η11 | γ1 | δ | skew | shape | AIC | BIC | |
sGARCH(1, 1) | 0.001215∗ | 0.000059∗∗∗ | 0.096789 | 0.890399 | - | - | - | −0.027705∗ | 0.422959 | -3.5398 | -3.5066 |
(0.001427) | (0.000028) | (0.024861) | (0.023035) | (-) | (-) | (-) | (0.074202) | (0.088495) | |||
TGARCH(1, 1) | 0.001220∗ | 0.000058∗∗∗ | 0.099548 | 0.890794 | - | −0.005154∗ | - | −0.028101∗ | 0.422188 | -3.5375 | -3.4988 |
(0.001634) | (0.000033) | (0.038197) | (0.018084) | (-) | (0.044743) | (-) | (0.080220) | (0.104442) | |||
iGARCH(1, 1) | 0.001169∗ | 0.000049∗∗∗ | 0.107657 | 0.892343 | - | - | - | −0.028807∗ | 0.390402 | -3.5416 | -3.5139 |
(0.001457) | (0.000022) | (.022101) | (-) | (-) | (-) | (-) | (0.077171) | (0.069449) | |||
eGARCH(1, 1) | 0.000542∗ | -0.191458 | 0.009095∗ | 0.968640 | - | 0.232120 | - | −0.055214∗ | 0.397532 | -3.5416 | -3.5028 |
(0.001412) | (0.073537) | (0.025764) | (0.011902) | (-) | (0.042221) | (-) | (0.075690) | (0.082720) | |||
apARCH(1, 1) | 0.000653∗ | 0.000668∗ | 0.129881 | 0.884082 | - | −0.050379∗ | 1.252465 | −0.051396∗ | 0.398624 | -3.5373 | -3.4930 |
(0.001459) | (0.001080) | (0.030803) | (0.025187) | (-) | (0.116375) | (0.450200) | (0.077003) | (0.084866) | |||
gjrGARCH(1, 1) | 0.001220∗ | 0.000058∗∗∗ | 0.099548 | 0.890794 | - | −0.005154∗ | - | −0.028101∗ | 0.422188 | -3.5375 | -3.4988 |
(0.001427) | (0.000029) | (0.032236) | (0.023174) | (-) | (0.037028) | (-) | (0.074273) | (0.088494) |
Model | Estimated Parameters | |||||||
μ | ω | α1 | β1 | skew | shape | AIC | BIC | |
iGARCH(1, 1)-STD | 0.005470 | 0.000043∗ | 0.111565 | 0.888435 | - | 4.027443 | -3.4869 | -3.4392 |
(0.001965) | (0.000031) | (0.032094) | (-) | (-) | (0.779175) | |||
iGARCH(1, 1)-GED | 0.004706∗ | 0.000033∗ | 0.104315 | 0.895685 | - | 1.118081 | -3.4652 | -3.4176 |
(0.002971) | (0.000023) | (0.034135) | (-) | (-) | (0.107730) | |||
iGARCH(1, 1)-NIG | 0.004391∗∗ | 0.000040∗ | 0.109908 | 0.890092 | −0.112198∗ | 0.881824 | -3.4769 | -3.4173 |
(0.002338) | (0.000027) | (0.032952) | (-) | (0.120317) | (0.303135) |
The in-sample comparison tells us how well a model fits the entire data set.
The best GARCH models from Tables 4-6 are used for in-sample and out-of-sample forecast in order to check their forecasting ability. The model with the lowest mean square error and mean absolute error is selected as the optimal model among the three models. The in-sample forecast is performed for the period between 02/01/2018 to 11/03/2020 (before financial crisis period) and an out-of-sample forecast is performed from the period between 11/03/2020 to 14/06/2021 (during financial crisis period).
Figure 3 shows the in-sample volatility of iGARCH-STD, iGARCH-GED, and iGARCH-NIG. The evaluation metrics (MAE of 0.016894 and MSE of 0.126090) values from Table 8 show that iGARCH-NIG model has a good forecasting ability pre-Covid period.
iGARCH(1, 1)-STD | iGARCH(1, 1)-GED | iGARCH(1, 1)-NIG | |
MSE | 0.019705 | 0.017984 | 0.016894 |
MAE | 0.135840 | 0.129950 | 0.126090 |
Out-of sample forecast are performed to verify the performance of three models during financial crisis period. For the purpose of the test, sample period from 11/03/2020 to 14/06/2021 (consisting of 314 data points) were considered for out-of sample forecast. From Table 9, iGARCH(1, 1)-NIG has the lowest mean square error and mean absolute error values of 0.002075 and 0.032820. Based on Tables 8 and 9, iGARCH(1, 1)-NIG is selected as the optimal model using forecasting ability as a measure of performance.
iGARCH(1, 1)-STD | iGARCH(1, 1)-GED | iGARCH(1, 1)-NIG | |
MSE | 0.002078 | 0.002088 | 0.002075 |
MAE | 0.032870 | 0.033000 | 0.032820 |
{Clearly, the NIG distribution is able to capture the distributional properties of the Bitcoin futures return.}
From Tables A4, there is a positive correlation between volatility and return of Bitcoin futures since the estimate for β1 is greater than zero for all eGARCH(1, 1) model with different error distributions. The presence of asymmetric response in the return series of Bitcoin futures is established given the non-zero asymmetric and leverage parameter values in all the gjrGARCH(1, 1) and eGARCH(1, 1) models. The positive and significant leverage effect parameter values of all the eGARCH models (eGARCH(1, 1)-STD = 0.278848, eGARCH(1, 1)-GED = 0.220560, eGARCH(1, 1)-NIG = 0.232120) demonstrate that positive shocks (good news) increase volatility more than negative shocks (bad news) of the same sign. The negative and significant{\footnote{significant at 1% and 5%}} leverage effect values in the gjrGARCH models (with the exception of gjrGARCH(1, 1)-GED) demonstrate that positive shocks (market advances) lead to increased volatility to a greater extend than negative shocks (market retreats) of the same magnitude. These results are consistent to the results of Kuhe (2018) who noted that the presence of leverage impacts and shocks persistence for different distributional assumptions in the Nigerial stock market. From Tables A1 and A6, the sum of parameters α1 and β1 for sGARCH and gjrGARCH is less than 1 for the three distributions. Nevertheless, α1<β1 in both GARCH models for the three different distributions. This signifies that negative shocks do not have a larger effect on conditional volatility than positive shocks of the same magnitude. These results is consistent to the findings of Thalassinos et al. (2015) who concluded in their studdies that volatility of returns on Czech Stock Market can be described by signinificant asymmetric and persistence impacts. Further, in most of the GARCH models, the estimate of γ1 is negative giving proof of an inverted reaction of the volatility. In general, GARCH models with conditionally t-distributed errors performed well as compared to GARCH models with generalized error distribution and normal inverse Gaussian. This is demonstrated from the low AIC and BIC values recorded for all GARCH-STD models. Generally, iGARCH models are the best performing GARCH model for modelling the volatility of Bitcoin futures return for the selected period (02/01, 2018 to 14/06/2021).
Similarly to the results obtained by Gyamerah (2019), the Normal Inverse Gaussian (NIG) error distribution captured adequately the leptokurtic and skewness in the bitcoin futures returns data. This indicates the efficiency of the NIG distribution.
In this paper, we investigate the relative performance of different GARCH family models (sGARCH, tGARCH, iGARCH, eGARCH, apARCH, and gjrGARCH) and different error distributions (student-t distribution (STD), generalized error distribution (GED), and normal inverse Gaussian (NIG)) in modeling and forecasting volatility of Bitcoin futures returns using two different time periods. The first period is from January 2, 2018 to June 14, 2021; the second period extends from March 11, 2020 to June 14, 2021. From the results, iGARCH(1, 1)-STD is selected as the best performing model among the 18 constructed models for the first period since it has the lowest AIC (−3.5397) and BIC (−3.5175) values. Using the three best models selected from the first period, iGARCH(1, 1)-STD is selected as the optimal model for the second period. These indicate the superiority of iGARCH(1, 1)-STD to model the volatility and error distribution of Bitcoin futures {returns. Our results indicate the effects of past squared shocks on the squared returns of Bitcoin futures and the ability of iGARCH(1, 1)-STD to model such innovations. }
Using the best 3 models (iGARCH(1, 1)-STD, iGARCH(1, 1)-GED, and iGARCH(1, 1)-NIG) among the 18 constructed models from the first period, we investigated the in-sample and out-of-sample predictive abilities. Based on two evaluation metrics (mean absolute error and mean square error), iGARCH(1, 1)-NIG is selected as the best performing model in-sample forecast for the period from January 02, 2018 to June 14, 2021. The model also performed well, with the lowest MSE and MAE values for out-of-sample forecast from 11 March, 2020 to 14 June, 2021 (during the COVID period). These results imply that the iGARCH-NIG model can be more advantageous to the other two models when carrying out risk management strategies for Bitcoin futures returns.
The authors declare that they have no competing interests.
Data for this work is available from the corresponding author upon request.
[1] | Cheng E, Cme to launch bitcoin futures in three weeks after green light from regulator; bitcoin jumps, 2017. Available from: https://www.cnbctv18.com/market/cme-to-launch-bitcoin-futures-in-3-weeks-after-green-light-from-regulator-bitcoin-jumps-16131.htm |
[2] |
Baur DG, Dimpfl T (2019) Price discovery in bitcoin spot or futures? J Futures Mark 39: 803–817. https://doi.org/10.1002/fut.22004 doi: 10.1002/fut.22004
![]() |
[3] | Shiller RJ (2017) what is bitcoin really worth? don't even ask. N Y Times. |
[4] |
Katsiampa P (2017) Volatility estimation for bitcoin: A comparison of garch models. Econ Lett 158: 3–6. https://doi.org/10.1016/j.econlet.2017.06.023 doi: 10.1016/j.econlet.2017.06.023
![]() |
[5] |
Naimy VY, Hayek MR (2018) Modelling and predicting the bitcoin volatility using garch models. Int J Math Model Numer Optim 8: 197–215. https://doi.org/10.1504/IJMMNO.2018.088994 doi: 10.1504/IJMMNO.2018.088994
![]() |
[6] |
Gyamerah SA (2019) Modelling the volatility of bitcoin returns using garch models. Quant Financ Econ 3: 739–753. https://doi.org/10.3934/QFE.2019.4.739 doi: 10.3934/QFE.2019.4.739
![]() |
[7] |
Guo ZY (2021a) Risk management of bitcoin futures with garch models. Financ Res Lett 45: 102197. https://doi.org/10.1016/j.frl.2021.102197 doi: 10.1016/j.frl.2021.102197
![]() |
[8] |
Guo ZY (2021b) Price volatilities of bitcoin futures. Financ Res Lett 43: 102022. https://doi.org/10.1016/j.frl.2021.102022 doi: 10.1016/j.frl.2021.102022
![]() |
[9] |
Venter PJ, Maré E (2021) Univariate and multivariate garch models applied to bitcoin futures option pricing, J Risk Financ Manage 14: 261. https://doi.org/10.3390/jrfm14060261 doi: 10.3390/jrfm14060261
![]() |
[10] |
Nelson DB (1991) Conditional heteroskedasticity in asset returns: A new approach. Econometrica: J Econ Soc 347–370. https://doi.org/10.2307/2938260 doi: 10.2307/2938260
![]() |
[11] |
Ding Z, Granger CW, Engle RF (1993) A long memory property of stock market returns and a new model. J empirical financ 1: 83–106. https://doi.org/10.1016/0927-5398(93)90006-D doi: 10.1016/0927-5398(93)90006-D
![]() |
[12] |
Glosten LR, Jagannathan R, Runkle DE (1993) On the relation between the expected value and the volatility of the nominal excess return on stocks. J Financ 48: 1779–1801. https://doi.org/10.1111/j.1540-6261.1993.tb05128.x doi: 10.1111/j.1540-6261.1993.tb05128.x
![]() |
[13] |
Bollerslev T (1987) A conditionally heteroskedastic time series model for speculative prices and rates of return. Review Econ Stat 69: 542–547. https://doi.org/10.2307/1925546 doi: 10.2307/1925546
![]() |
[14] |
Kuhe DA (2018) Modeling volatility persistence and asymmetry with exogenous breaks in the nigerian stock returns. CBN J Appl Stat 9: 167–196. Available from: https://dc.cbn.gov.ng/jas/vol9/iss1/7 doi: 10.11114/aef.v2i1.608
![]() |
[15] |
Thalassinos I, Ugurlu E, Muratoglu Y, et al. (2015) Comparison of forecasting volatility in the czech republic stock market, Appl Econ Financ 2: 11–18. https://doi.org/10.11114/aef.v2i1.608 doi: 10.11114/aef.v2i1.608
![]() |
[16] |
Wilhelmsson A (2006) Garch forecasting performance under different distribution assumptions. J Forecast 25: 561–578. https://doi.org/10.1002/for.1009 doi: 10.1002/for.1009
![]() |
![]() |
![]() |
1. | Meghna Jayasankar, 2024, Chapter 3, 978-981-97-6241-5, 41, 10.1007/978-981-97-6242-2_3 | |
2. | Yi Xu, Jiajun Qi, Jilong Chen, Lingbing Feng, Kick down the ladder? The impact of cryptocurrency bans on company legal risks and employee structure, 2025, 15446123, 107186, 10.1016/j.frl.2025.107186 |
N | Min | Max | Mean | SE Mean | Median | Skewness | Kurtosis | SD | AD test | SW test | |
Bitcoin (BTCF)-Price | 860 | 3145 | 63840 | 13730 | 475.3 | 8932 | 2.211 | 3.682 | 13940 | p< 2e-16 | p< 2e-16 |
Bitcoin (BTCF)-Return | 859 | -0.267726 | 0.222358 | 0.001145 | 0.001650 | 0.000801 | -0.370932 | 4.537262 | 0.048363 | p< 2e-16 | p< 2e-16 |
N=number of sample; Min=Minimum; Max = Maximum; SE Mean =Standard Error of Mean; SD=Standard Deviation |
Shapiro-Wilk | Anderson-Darling | |
P-Value | <2e-16 | <2e-16 |
Test Statistics | 0.93 | 16 |
Test was conducted on 5% α level of significance |
ARCH Lagrange Multiplier (LM) | Ljung Box | ADF | |
P-Value | 0.00161 | 2e-08 | 0.01 |
Test Statistics | 54 | 1.1 | -20 |
Model | Estimated Parameters | |||||||||
μ | ω | α1 | β1 | η11 | γ1 | δ | shape | AIC | BIC | |
sGARCH(1, 1) | 0.001664∗ | 0.000060∗ | 0.105875 | 0.893125 | - | - | - | 2.999262 | -3.5373 | -3.5096 |
(0.001069) | (0.000033) | (0.026727) | (0.021343) | (-) | (-) | (-) | (0.294213) | |||
tGARCH(1, 1) | 0.001252 | 0.001258 | 0.163539 | 0.886712 | -0.086243 | - | - | 2.672477 | -3.5390 | -3.5058 |
(0.001254) | (0.000716) | (0.037106) | (0.021241) | (0.113943) | (-) | (0.277959) | (0.277959) | |||
iGARCH(1, 1) | 0.001663∗ | 0.000059∗∗∗ | 0.106635 | 0.893365 | - | - | - | 2.990437 | -3.5397 | -3.5175 |
(0.001068) | (0.000030) | (0.020543) | (-) | (-) | (-) | (-) | (0.213747) | |||
eGARCH(1, 1)) | 0.001303 | -0.112149 | 0.015673∗ | 0.980604 | - | 0.278848 | - | 2.721974 | -3.5427 | -3.5095 |
(0.000289) | (0.041624) | (0.030645) | (0.007757) | (-) | (0.067328) | (-) | (0.305861) | |||
apARCH(1, 1)) | 0.001340∗ | 0.000588∗ | 0.163180 | 0.890757 | - | −0.071496∗ | 1.213898∗ | 2.694736 | -3.5371 | -3.4983 |
(0.001107) | (0.001094) | (0.045643) | (0.017330) | (-) | (0.130387) | (0.590110) | (0.311436) | |||
gjrGARCH(1, 1)) | 0.001676∗ | 0.000059∗∗ | 0.108898∗∗∗ | 0.893594 | - | −0.006984∗ | - | 3.005712 | -3.5350 | -3.5018 |
(0.001084) | (0.000034) | (0.034112) | (0.021714) | (-) | (0.038282) | (-) | (0.293892) |
Model | Estimated Parameters | |||||||||
μ | ω | α1 | β1 | η11 | γ1 | δ | shape | AIC | BIC | |
sGARCH(1, 1) | 0.000681 | 0.000076∗∗∗ | 0.086968 | 0.884133 | - | - | - | 0.900692 | -3.5359 | -3.5082 |
(0.000154) | (0.000034) | (0.023399) | (0.027653) | (-) | (-) | (-) | (0.053196) | |||
TGARCH(1, 1) | 0.000000∗ | 0.002391∗∗∗ | 0.128371 | 0.862617 | −0.015097∗ | - | - | 0.876118 | -3.5318 | -3.4986 |
(0.000048) | (0.000939) | (0.028177) | (0.031352) | (0.129982) | (-) | (-) | (0.052555) | |||
iGARCH(1, 1) | 0.000000∗ | 0.000053∗∗∗ | 0.111739 | 0.888261 | - | - | - | 0.848831 | -3.5353 | -3.5132 |
(0.000044) | (0.000024) | (0.026253) | (-) | (-) | (-) | (-) | (0.047908) | |||
eGARCH(1, 1)) | 0.00000∗ | -0.29120 | −0.00426∗ | 0.95283 | - | 0.22056 | - | 0.87908 | -3.5349 | -3.5017 |
(0.000049) | (0.101340) | (0.026079) | (0.016309) | (-) | (0.043414) | (-) | (0.052749) | |||
apARCH(1, 1)) | 0.000493∗∗∗ | 0.000187∗ | 0.100026 | 0.879429 | - | 0.022567∗ | 1.737045 | 0.898400 | -3.5315 | -3.4927 |
(0.000203) | (0.000240) | (0.030610) | (0.028709) | (-) | (0.104785) | (0.391820) | (0.053134) | |||
gjrGARCH(1, 1)) | 0.000000∗ | 0.000079∗∗∗ | 0.082188 | 0.882615 | - | 0.011581∗ | - | 0.891387 | -3.5339 | -3.5007 |
(0.000053) | (0.000035) | (0.028770) | (0.028068) | (-) | (0.034613) | (-) | (0.053999) |
Model | Estimated Parameters | ||||||||||
μ | ω | α1 | β1 | η11 | γ1 | δ | skew | shape | AIC | BIC | |
sGARCH(1, 1) | 0.001215∗ | 0.000059∗∗∗ | 0.096789 | 0.890399 | - | - | - | −0.027705∗ | 0.422959 | -3.5398 | -3.5066 |
(0.001427) | (0.000028) | (0.024861) | (0.023035) | (-) | (-) | (-) | (0.074202) | (0.088495) | |||
TGARCH(1, 1) | 0.001220∗ | 0.000058∗∗∗ | 0.099548 | 0.890794 | - | −0.005154∗ | - | −0.028101∗ | 0.422188 | -3.5375 | -3.4988 |
(0.001634) | (0.000033) | (0.038197) | (0.018084) | (-) | (0.044743) | (-) | (0.080220) | (0.104442) | |||
iGARCH(1, 1) | 0.001169∗ | 0.000049∗∗∗ | 0.107657 | 0.892343 | - | - | - | −0.028807∗ | 0.390402 | -3.5416 | -3.5139 |
(0.001457) | (0.000022) | (.022101) | (-) | (-) | (-) | (-) | (0.077171) | (0.069449) | |||
eGARCH(1, 1) | 0.000542∗ | -0.191458 | 0.009095∗ | 0.968640 | - | 0.232120 | - | −0.055214∗ | 0.397532 | -3.5416 | -3.5028 |
(0.001412) | (0.073537) | (0.025764) | (0.011902) | (-) | (0.042221) | (-) | (0.075690) | (0.082720) | |||
apARCH(1, 1) | 0.000653∗ | 0.000668∗ | 0.129881 | 0.884082 | - | −0.050379∗ | 1.252465 | −0.051396∗ | 0.398624 | -3.5373 | -3.4930 |
(0.001459) | (0.001080) | (0.030803) | (0.025187) | (-) | (0.116375) | (0.450200) | (0.077003) | (0.084866) | |||
gjrGARCH(1, 1) | 0.001220∗ | 0.000058∗∗∗ | 0.099548 | 0.890794 | - | −0.005154∗ | - | −0.028101∗ | 0.422188 | -3.5375 | -3.4988 |
(0.001427) | (0.000029) | (0.032236) | (0.023174) | (-) | (0.037028) | (-) | (0.074273) | (0.088494) |
Model | Estimated Parameters | |||||||
μ | ω | α1 | β1 | skew | shape | AIC | BIC | |
iGARCH(1, 1)-STD | 0.005470 | 0.000043∗ | 0.111565 | 0.888435 | - | 4.027443 | -3.4869 | -3.4392 |
(0.001965) | (0.000031) | (0.032094) | (-) | (-) | (0.779175) | |||
iGARCH(1, 1)-GED | 0.004706∗ | 0.000033∗ | 0.104315 | 0.895685 | - | 1.118081 | -3.4652 | -3.4176 |
(0.002971) | (0.000023) | (0.034135) | (-) | (-) | (0.107730) | |||
iGARCH(1, 1)-NIG | 0.004391∗∗ | 0.000040∗ | 0.109908 | 0.890092 | −0.112198∗ | 0.881824 | -3.4769 | -3.4173 |
(0.002338) | (0.000027) | (0.032952) | (-) | (0.120317) | (0.303135) |
iGARCH(1, 1)-STD | iGARCH(1, 1)-GED | iGARCH(1, 1)-NIG | |
MSE | 0.019705 | 0.017984 | 0.016894 |
MAE | 0.135840 | 0.129950 | 0.126090 |
iGARCH(1, 1)-STD | iGARCH(1, 1)-GED | iGARCH(1, 1)-NIG | |
MSE | 0.002078 | 0.002088 | 0.002075 |
MAE | 0.032870 | 0.033000 | 0.032820 |
N | Min | Max | Mean | SE Mean | Median | Skewness | Kurtosis | SD | AD test | SW test | |
Bitcoin (BTCF)-Price | 860 | 3145 | 63840 | 13730 | 475.3 | 8932 | 2.211 | 3.682 | 13940 | p< 2e-16 | p< 2e-16 |
Bitcoin (BTCF)-Return | 859 | -0.267726 | 0.222358 | 0.001145 | 0.001650 | 0.000801 | -0.370932 | 4.537262 | 0.048363 | p< 2e-16 | p< 2e-16 |
N=number of sample; Min=Minimum; Max = Maximum; SE Mean =Standard Error of Mean; SD=Standard Deviation |
Shapiro-Wilk | Anderson-Darling | |
P-Value | <2e-16 | <2e-16 |
Test Statistics | 0.93 | 16 |
Test was conducted on 5% α level of significance |
ARCH Lagrange Multiplier (LM) | Ljung Box | ADF | |
P-Value | 0.00161 | 2e-08 | 0.01 |
Test Statistics | 54 | 1.1 | -20 |
Model | Estimated Parameters | |||||||||
μ | ω | α1 | β1 | η11 | γ1 | δ | shape | AIC | BIC | |
sGARCH(1, 1) | 0.001664∗ | 0.000060∗ | 0.105875 | 0.893125 | - | - | - | 2.999262 | -3.5373 | -3.5096 |
(0.001069) | (0.000033) | (0.026727) | (0.021343) | (-) | (-) | (-) | (0.294213) | |||
tGARCH(1, 1) | 0.001252 | 0.001258 | 0.163539 | 0.886712 | -0.086243 | - | - | 2.672477 | -3.5390 | -3.5058 |
(0.001254) | (0.000716) | (0.037106) | (0.021241) | (0.113943) | (-) | (0.277959) | (0.277959) | |||
iGARCH(1, 1) | 0.001663∗ | 0.000059∗∗∗ | 0.106635 | 0.893365 | - | - | - | 2.990437 | -3.5397 | -3.5175 |
(0.001068) | (0.000030) | (0.020543) | (-) | (-) | (-) | (-) | (0.213747) | |||
eGARCH(1, 1)) | 0.001303 | -0.112149 | 0.015673∗ | 0.980604 | - | 0.278848 | - | 2.721974 | -3.5427 | -3.5095 |
(0.000289) | (0.041624) | (0.030645) | (0.007757) | (-) | (0.067328) | (-) | (0.305861) | |||
apARCH(1, 1)) | 0.001340∗ | 0.000588∗ | 0.163180 | 0.890757 | - | −0.071496∗ | 1.213898∗ | 2.694736 | -3.5371 | -3.4983 |
(0.001107) | (0.001094) | (0.045643) | (0.017330) | (-) | (0.130387) | (0.590110) | (0.311436) | |||
gjrGARCH(1, 1)) | 0.001676∗ | 0.000059∗∗ | 0.108898∗∗∗ | 0.893594 | - | −0.006984∗ | - | 3.005712 | -3.5350 | -3.5018 |
(0.001084) | (0.000034) | (0.034112) | (0.021714) | (-) | (0.038282) | (-) | (0.293892) |
Model | Estimated Parameters | |||||||||
μ | ω | α1 | β1 | η11 | γ1 | δ | shape | AIC | BIC | |
sGARCH(1, 1) | 0.000681 | 0.000076∗∗∗ | 0.086968 | 0.884133 | - | - | - | 0.900692 | -3.5359 | -3.5082 |
(0.000154) | (0.000034) | (0.023399) | (0.027653) | (-) | (-) | (-) | (0.053196) | |||
TGARCH(1, 1) | 0.000000∗ | 0.002391∗∗∗ | 0.128371 | 0.862617 | −0.015097∗ | - | - | 0.876118 | -3.5318 | -3.4986 |
(0.000048) | (0.000939) | (0.028177) | (0.031352) | (0.129982) | (-) | (-) | (0.052555) | |||
iGARCH(1, 1) | 0.000000∗ | 0.000053∗∗∗ | 0.111739 | 0.888261 | - | - | - | 0.848831 | -3.5353 | -3.5132 |
(0.000044) | (0.000024) | (0.026253) | (-) | (-) | (-) | (-) | (0.047908) | |||
eGARCH(1, 1)) | 0.00000∗ | -0.29120 | −0.00426∗ | 0.95283 | - | 0.22056 | - | 0.87908 | -3.5349 | -3.5017 |
(0.000049) | (0.101340) | (0.026079) | (0.016309) | (-) | (0.043414) | (-) | (0.052749) | |||
apARCH(1, 1)) | 0.000493∗∗∗ | 0.000187∗ | 0.100026 | 0.879429 | - | 0.022567∗ | 1.737045 | 0.898400 | -3.5315 | -3.4927 |
(0.000203) | (0.000240) | (0.030610) | (0.028709) | (-) | (0.104785) | (0.391820) | (0.053134) | |||
gjrGARCH(1, 1)) | 0.000000∗ | 0.000079∗∗∗ | 0.082188 | 0.882615 | - | 0.011581∗ | - | 0.891387 | -3.5339 | -3.5007 |
(0.000053) | (0.000035) | (0.028770) | (0.028068) | (-) | (0.034613) | (-) | (0.053999) |
Model | Estimated Parameters | ||||||||||
μ | ω | α1 | β1 | η11 | γ1 | δ | skew | shape | AIC | BIC | |
sGARCH(1, 1) | 0.001215∗ | 0.000059∗∗∗ | 0.096789 | 0.890399 | - | - | - | −0.027705∗ | 0.422959 | -3.5398 | -3.5066 |
(0.001427) | (0.000028) | (0.024861) | (0.023035) | (-) | (-) | (-) | (0.074202) | (0.088495) | |||
TGARCH(1, 1) | 0.001220∗ | 0.000058∗∗∗ | 0.099548 | 0.890794 | - | −0.005154∗ | - | −0.028101∗ | 0.422188 | -3.5375 | -3.4988 |
(0.001634) | (0.000033) | (0.038197) | (0.018084) | (-) | (0.044743) | (-) | (0.080220) | (0.104442) | |||
iGARCH(1, 1) | 0.001169∗ | 0.000049∗∗∗ | 0.107657 | 0.892343 | - | - | - | −0.028807∗ | 0.390402 | -3.5416 | -3.5139 |
(0.001457) | (0.000022) | (.022101) | (-) | (-) | (-) | (-) | (0.077171) | (0.069449) | |||
eGARCH(1, 1) | 0.000542∗ | -0.191458 | 0.009095∗ | 0.968640 | - | 0.232120 | - | −0.055214∗ | 0.397532 | -3.5416 | -3.5028 |
(0.001412) | (0.073537) | (0.025764) | (0.011902) | (-) | (0.042221) | (-) | (0.075690) | (0.082720) | |||
apARCH(1, 1) | 0.000653∗ | 0.000668∗ | 0.129881 | 0.884082 | - | −0.050379∗ | 1.252465 | −0.051396∗ | 0.398624 | -3.5373 | -3.4930 |
(0.001459) | (0.001080) | (0.030803) | (0.025187) | (-) | (0.116375) | (0.450200) | (0.077003) | (0.084866) | |||
gjrGARCH(1, 1) | 0.001220∗ | 0.000058∗∗∗ | 0.099548 | 0.890794 | - | −0.005154∗ | - | −0.028101∗ | 0.422188 | -3.5375 | -3.4988 |
(0.001427) | (0.000029) | (0.032236) | (0.023174) | (-) | (0.037028) | (-) | (0.074273) | (0.088494) |
Model | Estimated Parameters | |||||||
μ | ω | α1 | β1 | skew | shape | AIC | BIC | |
iGARCH(1, 1)-STD | 0.005470 | 0.000043∗ | 0.111565 | 0.888435 | - | 4.027443 | -3.4869 | -3.4392 |
(0.001965) | (0.000031) | (0.032094) | (-) | (-) | (0.779175) | |||
iGARCH(1, 1)-GED | 0.004706∗ | 0.000033∗ | 0.104315 | 0.895685 | - | 1.118081 | -3.4652 | -3.4176 |
(0.002971) | (0.000023) | (0.034135) | (-) | (-) | (0.107730) | |||
iGARCH(1, 1)-NIG | 0.004391∗∗ | 0.000040∗ | 0.109908 | 0.890092 | −0.112198∗ | 0.881824 | -3.4769 | -3.4173 |
(0.002338) | (0.000027) | (0.032952) | (-) | (0.120317) | (0.303135) |
iGARCH(1, 1)-STD | iGARCH(1, 1)-GED | iGARCH(1, 1)-NIG | |
MSE | 0.019705 | 0.017984 | 0.016894 |
MAE | 0.135840 | 0.129950 | 0.126090 |
iGARCH(1, 1)-STD | iGARCH(1, 1)-GED | iGARCH(1, 1)-NIG | |
MSE | 0.002078 | 0.002088 | 0.002075 |
MAE | 0.032870 | 0.033000 | 0.032820 |