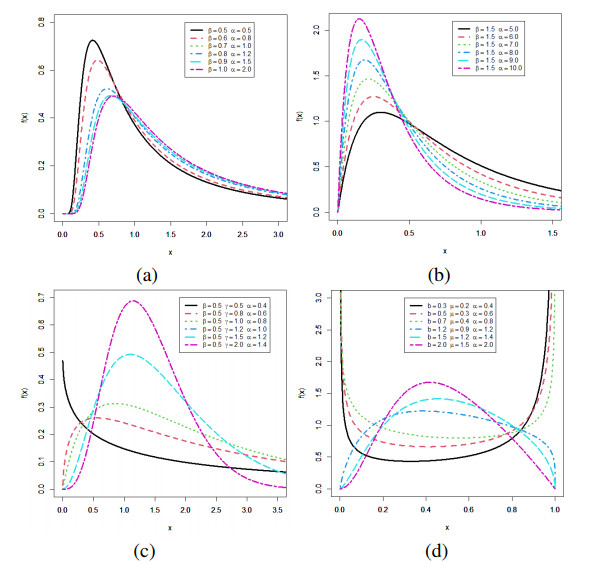
Citation: Jade Sternberg, Miriam Wankell, V. Nathan Subramaniam, Lionel W. Hebbard. The functional roles of T-cadherin in mammalian biology[J]. AIMS Molecular Science, 2017, 4(1): 62-81. doi: 10.3934/molsci.2017.1.62
[1] | Said G. Nassr, Amal S. Hassan, Rehab Alsultan, Ahmed R. El-Saeed . Acceptance sampling plans for the three-parameter inverted Topp–Leone model. Mathematical Biosciences and Engineering, 2022, 19(12): 13628-13659. doi: 10.3934/mbe.2022636 |
[2] | Walid Emam, Ghadah Alomani . Predictive modeling of reliability engineering data using a new version of the flexible Weibull model. Mathematical Biosciences and Engineering, 2023, 20(6): 9948-9964. doi: 10.3934/mbe.2023436 |
[3] | Manal M. Yousef, Rehab Alsultan, Said G. Nassr . Parametric inference on partially accelerated life testing for the inverted Kumaraswamy distribution based on Type-II progressive censoring data. Mathematical Biosciences and Engineering, 2023, 20(2): 1674-1694. doi: 10.3934/mbe.2023076 |
[4] | M. Nagy, Adel Fahad Alrasheedi . The lifetime analysis of the Weibull model based on Generalized Type-I progressive hybrid censoring schemes. Mathematical Biosciences and Engineering, 2022, 19(3): 2330-2354. doi: 10.3934/mbe.2022108 |
[5] | Walid Emam, Khalaf S. Sultan . Bayesian and maximum likelihood estimations of the Dagum parameters under combined-unified hybrid censoring. Mathematical Biosciences and Engineering, 2021, 18(3): 2930-2951. doi: 10.3934/mbe.2021148 |
[6] | Lernik Asserian, Susan E. Luczak, I. G. Rosen . Computation of nonparametric, mixed effects, maximum likelihood, biosensor data based-estimators for the distributions of random parameters in an abstract parabolic model for the transdermal transport of alcohol. Mathematical Biosciences and Engineering, 2023, 20(11): 20345-20377. doi: 10.3934/mbe.2023900 |
[7] | Wael S. Abu El Azm, Ramy Aldallal, Hassan M. Aljohani, Said G. Nassr . Estimations of competing lifetime data from inverse Weibull distribution under adaptive progressively hybrid censored. Mathematical Biosciences and Engineering, 2022, 19(6): 6252-6275. doi: 10.3934/mbe.2022292 |
[8] | M. G. M. Ghazal, H. M. M. Radwan . A reduced distribution of the modified Weibull distribution and its applications to medical and engineering data. Mathematical Biosciences and Engineering, 2022, 19(12): 13193-13213. doi: 10.3934/mbe.2022617 |
[9] | Raquel Caballero-Águila, María J. García-Ligero, Aurora Hermoso-Carazo, Josefa Linares-Pérez . Unreliable networks with random parameter matrices and time-correlated noises: distributed estimation under deception attacks. Mathematical Biosciences and Engineering, 2023, 20(8): 14550-14577. doi: 10.3934/mbe.2023651 |
[10] | Ibrahim Alkhairy . Classical and Bayesian inference for the discrete Poisson Ramos-Louzada distribution with application to COVID-19 data. Mathematical Biosciences and Engineering, 2023, 20(8): 14061-14080. doi: 10.3934/mbe.2023628 |
Weighted distributions (WDs) provide an approach to deal with model specification and data interpretations problems. They adjust the probabilities of the actual occurrence of events to arrive at a specification of the probabilities when those events are recorded. Reference [1] extended the basic ideas of the methods of ascertainment upon the estimation of frequencies in [2]. The author defined a unifying concept of the WDs and described several sample conditions that the WDs can model. The usefulness and applications of the WDs in various areas, including medicine, ecology, reliability, and branching processes, can also be seen in [3,4,5]. Important findings on the WDs have been reported by several research. For examples, reference [6] suggested a weighted x-gamma distribution, reference [7] derived a new generalized weighted Weibull distribution, reference [8] introduced the weighted exponential-Gompertz distribution, reference [9] studied the new weighted inverse Rayleigh distribution, reference [10] introduced a weighted version of the generalized inverse Weibull distribution, reference [11] proposed a bounded weighted exponential distribution, reference [12] derived a new weighted exponential distribution, reference [13] proposed a weighted power Lomax distribution, reference [14] derived a new generalized weighted exponential distribution, reference [15] introduced a new version of the weighted Weibull distribution, reference [16] proposed the modified weighted exponential distribution, and reference [17] proposed a weighted Nwikpe distribution, reference [18] introduced a new version of the double weighted quasi Lindley distribution and reference [19] proposed the modified length-biased weighted Lomax distribution.
In contrast, statistical models have the capacity to depict and predict real-world phenomena. Over the past few decades, numerous extended distributions have been extensively utilized in data modeling. Recent progress has been centered on the development of novel distribution families that not only enhance existing distributions but also offer significant versatility in practical data modeling. Engineering, economics, biology, and environmental science are particular examples of this. Regarding this, a number of writers suggested some of the created families of continuous distributions, (see for example [20,21,22]). Our interest here is in the same scheme used for the beta-G (B-G) family prepared in [23]. The following is the cumulative distribution function (cdf) for the B-G family:
F(x)=∫G(x)0r(t)dt, | (1.1) |
where G(x) is a cdf of a continuous distribution and r(t) is the probability density function (pdf) of the beta distribution. Naturally, any new family can be created by taking another pdf for r(t) with support [0,1] (see reference [23]).
As a matter of fact, few works about the weighted-G family have been proposed in the literature. For example, reference [24] studied the weighted exponential-G family, reference [25] introduced the weighted exponentiated family, reference [26] proposed a weighted general family, and reference [27] developed a weighted Topp-Leone-G family.
The primary purpose of this study is to introduce the length-biased truncated Lomax-G (LBTLo-G) family. The following arguments give enough motivation to study it:
1) The LBTLo-G family is very flexible and simple.
2) The LBTLo-G family contains some new distributions.
3) The shapes of the pdfs of the generated distributions can be unimodal, decreasing, bathtub, right-skewed, and symmetric. Also, the hazard rate function (hrf) shapes for these distributions can be increasing, decreasing, U-shaped, upside-down-shaped, or J- shaped.
After emphasizing these important aspects, some statistical and mathematical properties of the newly suggested family are discussed. The maximum likelihood (ML) method of estimation is used to estimate the LBTLo Weibull (LBTLoW) model parameters based on complete and type Ⅱ censoring (T2C).
The variability of the LBTLoW distribution is demonstrated through four authentic data sets. The first data set describes age data on rest times (in minutes) for analgesic patients. The second data set shows the percentage of natural gas reserves in 44 countries in 2020. The third authentic data set listed the top 20 countries by oil reserves. Proven reserves refer to the quantities of petroleum that can be predicted as commercially recoverable from known reservoirs, based on the analysis of geological and engineering data. These estimates are made considering existing economic conditions and are projected from a specific period onwards. The fourth data set displays the top 100 central banks in terms of gold reserves. This gold reserve data, collected from IMF IFS figures, tracks central banks' reported gold purchases and sales as a percentage of their international reserves. The application results show that the LBTLoW distribution can indeed match the data better than other competing distributions.
The following is the structure for this article: Section 2 defines the crucial functions of the LBTLo-G family and provides four special distributions of the family. In Section 3, some statistical properties of the LBTLo-G family are provided. Section 4 deals with the ML estimates (MLEs) of the unknown parameters. A simulation study to examine the theoretical performance of MLEs for the LBTLoW distribution is studied in Section 5. Section 6 presents the applicability and goodness of fit of the proposed models using four real data sets. The paper ends with a few last observations, as may be seen in Section 7.
Here, we suggest a new weighted family based on the weighted version of the truncated Lomax distribution, which is called the LBTLo distribution [28]. The cdf and pdf of the LBTLo distribution are, respectively, given by
G(t;α)=Λ(α)[(1+t)−α(1+αt)−1],0<t<1,α>0, | (2.1) |
g(t;α)=α(1−α)Λ(α)t(1+t)−(α+1), | (2.2) |
where Λ(α)=[2−α(1+α)−1]−1. For these functions, it is assumed the standard complementary values for t≤0 and t≥1.
As mentioned in [28], the following advantages of the LBTLo distribution are outlined: (ⅰ) It depends on only one parameter; (ⅱ) the pdf has only one maximum point with a relatively sharp peak and a heavy tail; (ⅲ) the hrf has increasing behavior or is N-shaped; and (ⅳ) it outperforms some other competing models in real-world applications to medical data and the percentage of household spending on education out of total household expenditure from the Household Income, Expenditure, and Consumption Survey data for North Sinai Governorate.
In light of these merits, the LBTLo distribution is a great choice to use in various fields. As a consequence, we present a novel generated family that is based on the LBTLo distribution. In order to define the LBTLo-G family, let G(x;ζ) and g(x;ζ) be the baseline cdf and pdf, respectively, of a continuous distribution, and ζ is a vector of parameters. The generalized B-G generator specified in (1.1) and the LBTLo distribution (2.2) are combined to generate the cdf of the LBTLo-G family:
F(x;α,ζ)=α(1−α)Λ(α)∫G(x;ζ)0t(1+t)−α−1dt=Λ(α)[(1+G(x;ζ))−α(1+αG(x;ζ))−1],x∈R,α>0, | (2.3) |
where α is a shape parameter. Therefore, the pdf of the LBTLo-G family is given by
f(x;α,ζ)=α(1−α)Λ(α)g(x;ζ)G(x;ζ)(1+G(x;ζ))−α−1,x∈R,α>0. | (2.4) |
A random variable X with the pdf (2.4) is designated as X∼ LBTLo-G from here on out. The complementary cdf (ccdf), and hrf, are, provided by
S(x;α,ζ)=1−Λ(α)[(1+G(x;ζ))−α(1+αG(x;ζ))−1], |
h(x;α,ζ)=α(1−α)Λ(α)g(x)G(x)(1+G(x))−α−11−Λ(α)[(1+G(x;ζ))−α(1+αG(x;ζ))−1]. |
We create four new LBTLo-G family sub-distributions in the subsections that follow: LBTLo-inverse exponential, LBTLo-uniform, LBTLo-Weibull, and LBTLo-Kumaraswamy distributions.
The cdf and pdf of the LBTLo-inverse exponential (LBTLoIE) distribution are obtained from (2.3) and (2.4) for G(x;β)=e−(β/x),β,x>0, as follows:
F(x;α,β)=Λ(α)[(1+e−(β/x))−α(1+αe−(β/x))−1],x>0,α,β>0, |
f(x;α,β)=α(1−α)Λ(α)βx−2e−2(β/x)(1+e−(β/x))−α−1. |
Further, the hrf is as follows:
h(x;α,β)=α(1−α)Λ(α)βx−2e−2(β/x)(1+e−(β/x))−α−11−Λ(α)[(1+e−(β/x))−α(1+αe−(β/x))−1]. |
The cdf and pdf of the LBTLo-uniform (LBTLoU) distribution are derived from (2.3) and (2.4) by taking G(x;β)=β−1x,0<x<β, as follows:
F(x;α,β)=[(1+αβ−1x)(1+β−1x)−α−1]Λ(α),0<x<β,α,β>0, |
f(x;α,β)=αβ−2x(1+β−1x)−α−1(1−α)Λ(α). |
Further, the hrf is as follows:
h(x;α,β)=αβ−2x(1+β−1x)−α−1(1−α)Λ(α)1−Λ(α)[(1+αβ−1x)(1+β−1x)−α−1]. |
The cdf and pdf of the LBTLoW distribution are derived from (2.3) and (2.4) taking G(x;β,γ)=1−e−βxγ,x,β,γ>0, as follows:
F(x;α,β,γ)=[(2−e−βxγ)−α(1+α−αe−βxγ)−1]Λ(α),x>0,α,β,γ>0, | (2.5) |
f(x;α,β,γ)=αβγ(1−α)xγ−1e−βxγ(1−e−βxγ)(2−e−βxγ)−α−1Λ(α). | (2.6) |
Further, the hrf is:
h(x;α,β,γ)=αβγ(1−α)xγ−1e−βxγ(1−e−βxγ)(2−e−βxγ)−α−1Λ(α)1−Λ(α)[(2−e−βxγ)−α(1+α−αe−βxγ)−1]. |
The cdf and pdf of the LBTLo- Kumaraswamy (LBTLoKw) distribution are obtained from (2.3) and (2.4) by taking G(x;μ,b)=1−(1−xμ)b,0<x<1,b,μ>0, as follows:
F(x;α,μ,b)=[(2−(1−xμ)b)−α(1+α−α(1−xμ)b)−1]Λ(α),0<x<1,α,μ,b>0, |
f(x;α,μ,b)=αμb(1−α)xμ−1(1−xμ)b−1(1−(1−xμ)b)×(2−(1−xμ)b)−α−1Λ(α). |
Further, the hrf is as follows:
h(x;α,μ,b)=αμb(1−α)xμ−1(1−xμ)b−1(1−(1−xμ)b)Λ(α)(2−(1−xμ)b)−α−11−Λ(α)[(2−(1−xμ)b)−α(1+α−α(1−xμ)b)−1]. |
The plots of pdf and hrf for the LBTLoIE, LBTLoU, LBTLoW and LBTLoKw distributions are given in Figures 1 and 2, respectively.
The pdfs of the investigated distributions can have a variety of forms, including right- and left-skewed, bathtub, uni-modal, declining, and symmetric shapes, as shown in Figure 1. The corresponding hrf can take any form, including U, J, reverse J, growing, or decreasing, as seen in Figure 1.
In this part, we give some statistical properties of the LBTLo-G family.
The LBTLo-G family representations in pdf and cdf format are displayed here. The generalized binomial theorem says that
(1+z)−β=∞∑i=0(−1)i(β+i−1i)zi, | (3.1) |
for |z|<1. Hence, by using (3.1) in (2.4), the pdf of the LBTLo-G family can be written as follows:
f(x;α,ζ)=∞∑i=0ϑig(x;ζ)G(x;ζ)i+1,x∈R, | (3.2) |
where ϑi=(−1)iα(1−α)Λ(α)(α+ii). For example, the expansion of pdf of the LBTLoW distribution is derived from (3.2) as follows:
f(x;α,β,γ)=βγ∞∑i=0ϑixγ−1e−βxγ(1−e−βxγ)i+1,x>0,α,β,γ>0. | (3.3) |
But, in the special case where b is a positive integer, the standard generalized binomial theorem says that
(1−z)b=b∑ν=0(−1)ν(bν)zν. | (3.4) |
Then using the binomial expansion (3.4) in (3.3), we get
f(x;α,β,γ)=∞∑i=0i+1∑ν=0ϑi,νxγ−1e−β(ν+1)xγ, | (3.5) |
where ϑi,ν=βγϑi(−1)ν(i+1ν). In what follows, an expansion for F(x;α,ζ)his derived, for h is an integer, again, the exponential and the binomial expansions are worked out:
F(x;α,ζ)h=Λ(α)h[(1+G(x;ζ))−α(1+αG(x;ζ))−1]h. | (3.6) |
Using the binomial expansion (3.4) in (3.6), we get
F(x;α,ζ)h=Λ(α)hh∑j=0(−1)h−j(hj)(1+G(x;ζ))−αj(1+αG(x;ζ))j. | (3.7) |
Using the binomial expansion (3.1), we obtain
F(x;α,ζ)h=Λ(α)h∞∑d=0h∑j=0(−1)d+h−j(hj)(αj+d−1d)×G(x;ζ)d(1+αG(x;ζ))j. | (3.8) |
By using (3.4) in (3.8), we obtain
F(x;α,ζ)h=∞∑d=0ϖd,j,mG(x;ζ)d+m, | (3.9) |
where ϖd,j,m=∑hj=0∑jm=0(−1)d+m+h−jαm(hj)(jm)(αj+d−1d)Λ(α)h.
For example, the expansion of the cdf of the LBTLoW distribution is derived from (3.9), where G(x,ζ)=1−e−βxγ, as follows:
F(x;α,β,γ)h=∞∑d=0ϖd,j,m(1−e−βxγ)d+m. |
By using the binomial expansion (3.4) in the last term of the previous equation, we get
F(x;α,β,γ)h=∞∑d=0d+m∑l=0(−1)l(d+ml)ϖd,j,me−βlxγ. | (3.10) |
The above representations are of interest to express various important moment measures as series. By truncating the index of summation, we can have a precise approximation with a reasonable computation cost.
As a special class of moments, the probability weighted moments (PWMs) have been proposed in [29]. This class is used to derive estimates of the parameters and quantiles of distributions expressible in inverse form. Let X be a random variable with pdf and cdf f(x) and F(x), respectively, and r and q be non-negative integers. Then, the (r,q)th PWM of X, denoted by πr,q, can be calculated through the following relation:
πr,q=E[XrF(X)q]=∫∞−∞xrf(x)F(x)qdx. | (3.11) |
On this basis, the (r,q)th PMW of X with pdf and cdf of the LBTLo-G family is obtained by substituting (3.2) and (3.9) into (3.11), as follows:
πr,q=E[XrF(X;α,ζ)q]=∫∞−∞∞∑i,d=0ϑiϖd,j,mxrg(x;ζ)[G(x;ζ)]i+d+m+1dx. |
Then, provided that the interchange of the integral and sum is valid, depending on the definitions of g(x;ζ) and G(x;ζ), we have
πr,q=∞∑i,d=0ϑiϖd,j,mρr,i+d+m+1, |
where
ρr,i+d+m+1=∫∞−∞xrg(x;ζ)[G(x;ζ)]i+d+m+1dx. |
For example, the (r,q)th of a random variable X that follows the LBTLoW distribution can be obtained by substituting (3.5) and (3.10) into (3.11), and replacing h with q. We thus obtain
πr,q=∞∑i,d=0i+1∑v=0d+m∑l=0(−1)lϑi,vϖd,j,m(β(ν+l+1))rγ+1(d+ml)Γ(rγ+1), |
where Γ(.) stands for gamma function.
In this part, for any non-negative integer r, the rth moment associated with the LBTLo-G family is derived.
Let X be a random variable having the pdf of the LBTLo-G family. Then, the rth moment of X is obtained as follows:
μ′r=E(Xr)=∫∞−∞∞∑i=0ϑixrg(x;ζ)[G(x;ζ)]i+1dx=∞∑i=0ϑiυr,i+1, |
where υr,i+1 is the (r,i+1)th PWM of the baseline distribution. For example, after some developments, the rth moment associated with LBTLoW distribution is given by
μ′r=∞∑i=0i+1∑ν=0ϑi,ν(β(ν+1))rγ+1Γ(rγ+1). |
Tables 1–3 show the numerical values of the first four moments μ′1, μ′2, μ′3, μ′4, also the numerical values of variance (σ2), coefficient of skewness (CS), coefficient of kurtosis (CK) and coefficient of variation (CV) associated with the LBTLoW and LBTLoIE distribution.
γ | α | μ′1 | μ′2 | μ′3 | μ′4 | σ2 | CS | CK | CV |
0.4 | 0.2 | 2.629 | 8.323 | 30.531 | 126.387 | 1.413 | 0.729 | 3.596 | 0.452 |
0.6 | 0.5 | 1.558 | 2.937 | 6.430 | 15.922 | 0.508 | 0.746 | 3.627 | 0.457 |
0.8 | 0.8 | 1.184 | 1.700 | 2.847 | 5.403 | 0.300 | 0.765 | 3.660 | 0.463 |
1.1 | 1.2 | 0.925 | 1.044 | 1.380 | 2.074 | 0.189 | 0.793 | 3.717 | 0.470 |
1.3 | 1.5 | 0.805 | 0.794 | 0.920 | 1.216 | 0.146 | 0.814 | 3.758 | 0.475 |
1.7 | 1.8 | 0.705 | 0.615 | 0.634 | 0.748 | 0.117 | 0.856 | 3.853 | 0.486 |
1.9 | 2.0 | 0.655 | 0.532 | 0.513 | 0.568 | 0.103 | 0.878 | 3.906 | 0.491 |
2.4 | 2.3 | 0.582 | 0.425 | 0.371 | 0.374 | 0.086 | 0.937 | 4.058 | 0.503 |
2.7 | 2.6 | 0.530 | 0.355 | 0.285 | 0.266 | 0.073 | 0.974 | 4.162 | 0.511 |
3.2 | 3.0 | 0.469 | 0.280 | 0.203 | 0.172 | 0.060 | 1.038 | 4.359 | 0.522 |
γ | α | μ′1 | μ′2 | μ′3 | μ′4 | σ2 | CS | CK | CV |
0.4 | 0.2 | 1.964 | 4.277 | 10.154 | 25.945 | 0.420 | 0.380 | 3.017 | 0.330 |
0.6 | 0.5 | 1.347 | 2.017 | 3.298 | 5.813 | 0.202 | 0.395 | 3.026 | 0.334 |
0.8 | 0.8 | 1.105 | 1.360 | 1.829 | 2.658 | 0.139 | 0.411 | 3.037 | 0.337 |
1.1 | 1.2 | 0.924 | 0.954 | 1.081 | 1.325 | 0.100 | 0.435 | 3.058 | 0.343 |
1.3 | 1.5 | 0.836 | 0.783 | 0.805 | 0.897 | 0.084 | 0.453 | 3.075 | 0.346 |
1.7 | 1.8 | 0.760 | 0.649 | 0.612 | 0.627 | 0.072 | 0.489 | 3.116 | 0.353 |
1.9 | 2.0 | 0.720 | 0.584 | 0.524 | 0.511 | 0.066 | 0.508 | 3.140 | 0.356 |
2.4 | 2.3 | 0.661 | 0.494 | 0.411 | 0.374 | 0.058 | 0.557 | 3.213 | 0.365 |
2.7 | 2.6 | 0.617 | 0.433 | 0.339 | 0.290 | 0.052 | 0.588 | 3.265 | 0.369 |
3.2 | 3.0 | 0.565 | 0.364 | 0.263 | 0.209 | 0.045 | 0.641 | 3.366 | 0.377 |
β | α | μ′1 | μ′2 | μ′3 | μ′4 | σ2 | CS | CK | CV |
1.5 | 0.2 | 0.029 | 0.026 | 0.023 | 0.021 | 0.025 | 5.273 | 29.151 | 5.386 |
0.5 | 0.032 | 0.028 | 0.025 | 0.023 | 0.027 | 5.014 | 26.463 | 5.138 | |
0.8 | 0.035 | 0.031 | 0.028 | 0.025 | 0.030 | 4.772 | 24.069 | 4.906 | |
1.2 | 0.039 | 0.035 | 0.031 | 0.028 | 0.033 | 4.472 | 21.275 | 4.622 | |
1.5 | 0.043 | 0.038 | 0.033 | 0.03 | 0.036 | 4.264 | 19.441 | 4.424 | |
1.8 | 0.046 | 0.041 | 0.036 | 0.033 | 0.038 | 4.069 | 17.801 | 4.241 | |
2 | 0.049 | 0.043 | 0.038 | 0.034 | 0.040 | 3.946 | 16.806 | 4.125 | |
2.3 | 0.053 | 0.046 | 0.041 | 0.037 | 0.043 | 3.771 | 15.445 | 3.961 | |
2.6 | 0.056 | 0.049 | 0.044 | 0.039 | 0.046 | 3.607 | 14.225 | 3.809 | |
3 | 0.062 | 0.054 | 0.048 | 0.043 | 0.050 | 3.405 | 12.794 | 3.621 | |
2.5 | 0.2 | 0.006 | 0.005 | 0.005 | 0.004 | 0.005 | 12.420 | 156.328 | 12.403 |
0.5 | 0.007 | 0.006 | 0.006 | 0.005 | 0.006 | 11.672 | 138.195 | 11.665 | |
0.8 | 0.007 | 0.007 | 0.006 | 0.006 | 0.007 | 10.981 | 122.434 | 10.984 | |
1.2 | 0.009 | 0.008 | 0.007 | 0.007 | 0.008 | 10.140 | 104.548 | 10.156 | |
1.5 | 0.01 | 0.009 | 0.008 | 0.007 | 0.009 | 9.563 | 93.121 | 9.589 | |
1.8 | 0.011 | 0.010 | 0.009 | 0.008 | 0.010 | 9.030 | 83.140 | 9.066 | |
2 | 0.012 | 0.011 | 0.010 | 0.009 | 0.010 | 8.697 | 77.190 | 8.739 | |
2.3 | 0.013 | 0.012 | 0.011 | 0.010 | 0.012 | 8.227 | 69.194 | 8.279 | |
2.6 | 0.014 | 0.013 | 0.012 | 0.011 | 0.013 | 7.792 | 62.179 | 7.853 | |
3 | 0.017 | 0.015 | 0.014 | 0.013 | 0.015 | 7.261 | 54.128 | 7.334 |
It can be seen from Tables 1–3 that, when the value of α,γ increases for a fixed value of β, the first four moments and σ2 decrease, while the CS, CK, and CV measures increase. When the value of β increases for a fixed value of α and γ, we observe that the first four moments and σ decrease and then increase, while the CS, CK, and CV measures increase. The LBTLoW distribution is skewed to the right by leptokurtic curves.
Furthermore, if X is a random variable having the pdf of the LBTLo-G family, then the rth incomplete moment of X is obtained as follows:
φr(t)=E(XrI{X≤t})=∫t−∞xrf(x;α,ζ)dx=∫t−∞∞∑i=0ϑixrg(x;ζ)G(x;ζ)i+1dx. |
For example, after some developments, the rth incomplete moment associated with the LBTLoW distribution is given by
φr(t)=∞∑i=0i+1∑ν=0ϑi,ν[β(ν+1)]rγ+1Γ(rγ+1,β(ν+1)tγ), |
where Γ(.,x) is the lower incomplete gamma function.
Here, some uncertainty measures of the LBTLo-G family are derived. Then, these measures are specialized to the LBTLoW distribution. To begin, the Rényi entropy (RE), presented in [30], associated with a distribution with pdf f(x), is defined by
IR(ε)=11−εlog[∫∞−∞f(x)εdx],ε≠1,ε>0. |
A numerical study with integral calculus is possible; here, we focus on a series expansion. In what follows, an expansion for f(x;α,ζ)ε is derived, for ε is a non-integer (again, the generalized binomial expansion is worked out):
f(x;α,ζ)ε=∞∑i=0Δig(x;ζ)εG(x;ζ)i+ε, |
where
Δi=(−1)i[α(1−α)Λ(α)]ε(ε(α+1)+i−1i). |
Then, the RE associated with the LBTLo-G family is given by
IR(ε)=(1−ε)−1log{∫∞−∞∞∑i=0Δig(x;ζ)εG(x;ζ)i+εdx}. |
For example, the RE associated with the LBTLoW distribution can be obtained as follows:
IR(ε)=(1−ε)−1log{∞∑i,j=0Δi,jγ[β(ε+j)]−ε+(ε/γ)−(1/γ)Γ(ε−εγ+1γ)}. |
The Havrda and Charvát entropy (HaCE) (see [31]) associated with a distribution with pdf f(x) is defined by
HCR(ε)=121−ε−1({∫∞−∞f(x)εdx}1/ε−1),ε≠1,ε>0. |
Hence, the HaCE of the LBTLo-G family is given by
HCR(ε)=121−ε−1({∫∞−∞∞∑i=0Δig(x;ζ)εG(x;ζ)i+εdx}1/ε−1). |
For example, the HaCE of the LBTLoW distribution can be obtained as follows:
HCR(ε)=121−ε−1({∞∑i,j=0Δi,jγ[β(ε+j)]−ε+(ε/γ)−(1/γ)Γ(ε−εγ+1γ)}1/ε−1). |
The Arimoto entropy (ArE) (see [32]) associated with a distribution with pdf f(x) is defined by
AR(ε)=ε1−ε({∫∞−∞f(x)εdx}1/ε−1),ε≠1,ε>0. |
Hence, the ArE of the LBTLo-G family is given by
AR(ε)=ε1−ε({∫∞−∞∞∑i=0Δig(x;ζ)εG(x;ζ)i+εdx}1/ε−1). |
For example, the ArE of the LBTLoW distribution can be obtained as follows:
AR(ε)=ε1−ε({∞∑i,j=0Δi,jγ[β(ε+j)]−ε+(ε/γ)−(1/γ)Γ(ε−εγ+1γ)}1/ε−1). |
The Tsallis entropy (TsE) (see [33]) associated with a distribution with pdf f(x), is defined by
TR(ε)=1ε−1{1−∫∞−∞f(x)εdx},ε≠1,ε>0. |
Hence, the TsE of the LBTLo-G family is obtained as follows:
TR(ε)=1ε−1{1−∫∞−∞∞∑i=0Δig(x;ζ)εG(x;ζ)i+εdx}. |
For example, the TsE of the LBTLoW distribution can be obtained as follows:
TR(ε)=1ε−1{1−∞∑i,j=0Δi,jγ[β(ε+j)]−ε+(ε/γ)−(1/γ)Γ(ε−εγ+1γ)}. |
Some numerical values for the proposed entropy measures are obtained for the LBTLoW and LBTLoIE distribution in Tables 4 and 5.
ε | β | α | γ | RE | HaCE | ArE | TsE |
1.5 | 0.25 | 0.2 | 0.4 | 3.331 | 3.340 | 2.767 | 1.957 |
0.5 | 0.6 | 3.252 | 3.333 | 2.753 | 1.953 | ||
0.8 | 0.8 | 3.099 | 3.318 | 2.722 | 1.944 | ||
1.2 | 1.1 | 2.875 | 3.290 | 2.670 | 1.927 | ||
1.5 | 1.3 | 2.716 | 3.264 | 2.627 | 1.912 | ||
1.8 | 1.7 | 2.524 | 3.227 | 2.568 | 1.891 | ||
2.0 | 1.9 | 2.415 | 3.203 | 2.53 | 1.876 | ||
2.3 | 2.4 | 2.229 | 3.152 | 2.458 | 1.846 | ||
2.6 | 2.7 | 2.084 | 3.104 | 2.394 | 1.818 | ||
3.0 | 3.2 | 1.889 | 3.026 | 2.296 | 1.773 | ||
0.5 | 0.2 | 0.4 | 1.930 | 3.044 | 2.318 | 1.783 | |
0.5 | 0.6 | 1.924 | 3.042 | 2.315 | 1.782 | ||
0.8 | 0.8 | 1.824 | 2.996 | 2.260 | 1.755 | ||
1.2 | 1.1 | 1.660 | 2.909 | 2.161 | 1.704 | ||
1.5 | 1.3 | 1.541 | 2.835 | 2.081 | 1.661 | ||
1.8 | 1.7 | 1.392 | 2.726 | 1.969 | 1.597 | ||
2.0 | 1.9 | 1.308 | 2.657 | 1.901 | 1.556 | ||
2.3 | 2.4 | 1.161 | 2.517 | 1.770 | 1.475 | ||
2.6 | 2.7 | 1.051 | 2.397 | 1.661 | 1.404 | ||
3.0 | 3.2 | 0.903 | 2.207 | 1.500 | 1.293 | ||
2.0 | 0.25 | 0.2 | 0.4 | 2.180 | 1.987 | 1.837 | 0.993 |
0.5 | 0.6 | 2.167 | 1.986 | 1.835 | 0.993 | ||
0.8 | 0.8 | 2.053 | 1.982 | 1.812 | 0.991 | ||
1.2 | 1.1 | 1.876 | 1.973 | 1.769 | 0.987 | ||
1.5 | 1.3 | 1.753 | 1.965 | 1.734 | 0.982 | ||
1.8 | 1.7 | 1.595 | 1.949 | 1.681 | 0.975 | ||
2.0 | 1.9 | 1.510 | 1.938 | 1.648 | 0.969 | ||
2.3 | 2.4 | 1.358 | 1.912 | 1.581 | 0.956 | ||
2.6 | 2.7 | 1.247 | 1.887 | 1.524 | 0.943 | ||
3.0 | 3.2 | 1.098 | 1.840 | 1.435 | 0.920 | ||
0.5 | 0.2 | 0.4 | 1.210 | 1.877 | 1.503 | 0.938 | |
0.5 | 0.6 | 1.279 | 1.895 | 1.541 | 0.947 | ||
0.8 | 0.8 | 1.210 | 1.877 | 1.503 | 0.938 | ||
1.2 | 1.1 | 1.080 | 1.834 | 1.423 | 0.917 | ||
1.5 | 1.3 | 0.987 | 1.794 | 1.358 | 0.897 | ||
1.8 | 1.7 | 0.861 | 1.725 | 1.258 | 0.862 | ||
2.0 | 1.9 | 0.795 | 1.679 | 1.199 | 0.840 | ||
2.3 | 2.4 | 0.672 | 1.574 | 1.077 | 0.787 | ||
2.6 | 2.7 | 0.587 | 1.482 | 0.982 | 0.741 | ||
3.0 | 3.2 | 0.470 | 1.323 | 0.836 | 0.661 |
ε | β | α | RE | HaCE | ArE | TsE |
1.5 | 0.25 | 0.2 | 7.001 | 3.311 | 2.709 | 1.94 |
0.5 | 7.075 | 3.315 | 2.716 | 1.942 | ||
0.8 | 7.156 | 3.319 | 2.724 | 1.944 | ||
1.2 | 7.274 | 3.324 | 2.735 | 1.947 | ||
1.5 | 7.372 | 3.329 | 2.743 | 1.95 | ||
1.8 | 7.476 | 3.333 | 2.752 | 1.952 | ||
2 | 7.55 | 3.336 | 2.758 | 1.954 | ||
2.3 | 7.667 | 3.34 | 2.767 | 1.957 | ||
2.6 | 7.792 | 3.345 | 2.777 | 1.959 | ||
3 | 7.971 | 3.351 | 2.79 | 1.963 | ||
0.4 | 0.2 | 6.441 | 3.278 | 2.65 | 1.92 | |
0.5 | 6.452 | 3.279 | 2.651 | 1.921 | ||
0.8 | 6.469 | 3.28 | 2.653 | 1.921 | ||
1.2 | 6.503 | 3.282 | 2.657 | 1.923 | ||
1.5 | 6.536 | 3.284 | 2.66 | 1.924 | ||
1.8 | 6.577 | 3.287 | 2.665 | 1.925 | ||
2 | 6.608 | 3.289 | 2.668 | 1.927 | ||
2.3 | 6.661 | 3.292 | 2.674 | 1.928 | ||
2.6 | 6.721 | 3.296 | 2.681 | 1.931 | ||
3 | 6.813 | 3.301 | 2.69 | 1.934 | ||
2.0 | 0.25 | 0.2 | 4.376 | 1.975 | 1.776 | 0.987 |
0.5 | 4.429 | 1.976 | 1.782 | 0.988 | ||
0.8 | 4.487 | 1.977 | 1.788 | 0.989 | ||
1.2 | 4.57 | 1.979 | 1.796 | 0.99 | ||
1.5 | 4.639 | 1.981 | 1.803 | 0.99 | ||
1.8 | 4.713 | 1.982 | 1.81 | 0.991 | ||
2 | 4.765 | 1.983 | 1.815 | 0.991 | ||
2.3 | 4.847 | 1.984 | 1.823 | 0.992 | ||
2.6 | 4.934 | 1.986 | 1.83 | 0.993 | ||
3 | 5.058 | 1.987 | 1.841 | 0.994 | ||
0.4 | 0.2 | 3.975 | 1.962 | 1.726 | 0.981 | |
0.5 | 3.987 | 1.963 | 1.728 | 0.981 | ||
0.8 | 4.003 | 1.963 | 1.73 | 0.982 | ||
1.2 | 4.031 | 1.965 | 1.734 | 0.982 | ||
1.5 | 4.058 | 1.965 | 1.737 | 0.983 | ||
1.8 | 4.09 | 1.967 | 1.741 | 0.983 | ||
2 | 4.114 | 1.967 | 1.744 | 0.984 | ||
2.3 | 4.154 | 1.969 | 1.749 | 0.984 | ||
2.6 | 4.199 | 1.97 | 1.755 | 0.985 | ||
3 | 4.267 | 1.972 | 1.763 | 0.986 |
We can see from these tables that, as the value of ε rises, all entropy values decrease, providing more information. For a fixed value of β, as the values of α and γ rise, we infer that all entropy metrics decrease, indicating that there is less fluctuation. Additionally, we deduce that all entropies have less variability as the values of α, γ and β increase. When compared to other measures, the TsE measure values typically have the smallest values.
Let x(1)≤x(2)≤…≤x(n) be a T2C of size r resulting from a life test on n items whose lifetimes are described by the LBTLo-G family with a given set of parameters α and ζ, see [34,35,36,37]. The log-likelihood function of r failures and (n−r) censored values, is given by
logL(α,ζ)=rlogα+rlog(1−α)+rlogΛ(α)+r∑i=1logg(xi;ζ)+r∑i=1logG(xi;ζ)−(α+1)r∑i=1log(1+G(xi;ζ))+(n−r)log[Ar(α,ζ)], |
where Ar(α,ζ)=1−Λ(α)[(1+G(xr;ζ))−α(1+αG(xr;ζ))−1], and we write x(i)=xi for simplified form.
By maximizing the previous likelihood function, the MLEs of unknown parameters are determined. To achieve this, we can first compute the first derivative of the score function (Uα,Uζk), given as follows:
Uα=rα−r1−α+rΛ(α)(∂∂αΛ(α))−r∑i=1log(1+G(xi;ζ))+(n−r)Ar(α,ζ)(∂∂αAr(α,ζ)), |
Uζk=−(α+1)r∑i=111+G(xi;ζ)∂∂ζk(G(xi;ζ))+(n−r)Ar(α,ζ)∂∂ζkAr(α,ζ), |
where
∂∂αΛ(α)=[Λ(α)]22−α[(1+α)log2−1], |
∂∂αAr(α,ζ)=−∂∂αΛ(α)[(1+G(xr;ζ))−α(1+αG(xr;ζ))−1]+Λ(α)[(1+G(xr;ζ))−α{(1+αG(xr;ζ))log(1+G(xr;ζ))−G(xr;ζ)}], |
and
∂∂ζk(Ar(α,ζ))=α(α−1)Λ(α)G(xr;ζ)(1+G(xr;ζ))−α−1∂∂ζkG(xr;ζ). |
By putting Uα and Uζk equal to zero and solving these equations simultaneously, the MLEs of the LBTLo-G family are found. These equations are not amenable to analytical solution, however they are amenable to numerical solution by iterative techniques utilizing statistical software.
The confidence interval (CI) of the vector of the unknown parameters ξ=(α,ζ) could be obtained from the asymptotic distribution of the MLEs of the parameters as (ˆξMLE−ξ)→N2(0,I−1(ˆξMLE)), where I(ξ) is the Fisher information matrix. Under particular regularity conditions, the two-sided 100(1−v), asymptotic CI for the vector of unknown parameters ξ can be acquired in the following ways: ˆξMLE±zv/2√var(ˆξ), where var(ˆξ) is the element of the main diagonal of the asymptotic variance-covariance matrix I−1(ˆξMLE) and zv/2 is the upper vth/2 percentile of the standard normal distribution.
This section includes a simulation study to evaluate the performance of the MLEs for the LBTLoW model (α,β,γ), for complete and T2C. The Mathematica 9 package is used to get the mean squared error (MSE), lower bound (LB) of CI, upper bound (UB) of CI, average length (AL) of 95%, and coverage probability (CP) of 95% of the estimated values of α, β and γ. The algorithm is developed in the way described below:
1) From the LBTLoW distribution, 5000 random samples of sizes n = 50,100,150, and 200 are created.
2) Values of the unknown parameters (α,β,γ) are selected as Set 1 =(α=0.5,β=0.5,γ=0.5), Set 2 =(α=0.7,β=0.5,γ=0.25), Set 3 =(α=0.7,β=0.7,γ=0.5), and Set 4 =(α=0.6,β=0.3,γ=0.5).
3) Three levels of censorship are chosen: r = 70%, 80% (T2C), and 100% (complete sample).
4) The MLEs, Biases, and MSEs for all sample sizes and for all selected sets of parameters are computed. Furthermore, the LB, UB, AL, and CP with a confidence level of 0.95 for all sample sizes and for all selected sets of parameters are calculated.
5) Numerical outcomes are reported in Table 6. Based on complete and T2C samples, we can detect the following about the performance of the estimated parameters.
n | r | Set1 (α = 0.5, β = 0.5, γ = 0.5) | |||||||
MLE | Bias | MSE | LB | UB | AL | CP | |||
50 | 70% | α | 0.4204 | 0.0796 | 0.0064 | 0.0019 | 0.839 | 0.8370 | 97.4% |
β | 0.7041 | 0.2041 | 0.0471 | 0.5036 | 0.9046 | 0.4010 | 96.9% | ||
γ | 0.4201 | 0.0799 | 0.0069 | 0.3023 | 0.5379 | 0.2356 | 96.0% | ||
80% | α | 0.4218 | 0.0782 | 0.0061 | 0.0191 | 0.8245 | 0.8053 | 94.8% | |
β | 0.6382 | 0.1382 | 0.0242 | 0.4508 | 0.8256 | 0.3748 | 95.8% | ||
γ | 0.4386 | 0.0614 | 0.0053 | 0.3282 | 0.5490 | 0.2208 | 97.1% | ||
100% | α | 0.4234 | 0.0766 | 0.0059 | 0.0357 | 0.8111 | 0.7754 | 95.4% | |
β | 0.5177 | 0.0177 | 0.0056 | 0.3661 | 0.6694 | 0.3033 | 95.5% | ||
γ | 0.5316 | 0.0316 | 0.0027 | 0.4303 | 0.6328 | 0.2025 | 96.0% | ||
100 | 70% | α | 0.4213 | 0.0787 | 0.0062 | 0.0844 | 0.7583 | 0.6740 | 96.2% |
β | 0.6750 | 0.1750 | 0.0312 | 0.5375 | 0.8125 | 0.2750 | 95.9% | ||
γ | 0.4237 | 0.0763 | 0.0065 | 0.3389 | 0.5084 | 0.1694 | 96.0% | ||
80% | α | 0.4230 | 0.0770 | 0.0061 | 0.2099 | 0.6360 | 0.4262 | 96.2% | |
β | 0.6099 | 0.1099 | 0.0127 | 0.4819 | 0.7379 | 0.2560 | 96.1% | ||
γ | 0.4487 | 0.0513 | 0.0033 | 0.3652 | 0.5321 | 0.1669 | 97.3% | ||
100% | α | 0.4238 | 0.0762 | 0.0058 | 0.2501 | 0.5975 | 0.3473 | 95.6% | |
β | 0.4683 | 0.0317 | 0.0027 | 0.3558 | 0.5807 | 0.2249 | 95.8% | ||
γ | 0.4967 | 0.0033 | 0.0025 | 0.4199 | 0.5734 | 0.1535 | 96.0% | ||
150 | 70% | α | 0.4217 | 0.0783 | 0.0061 | 0.2710 | 0.5725 | 0.3015 | 95.2% |
β | 0.6626 | 0.1626 | 0.0281 | 0.5577 | 0.7675 | 0.2097 | 95.6% | ||
γ | 0.4277 | 0.0723 | 0.0058 | 0.3571 | 0.4983 | 0.1412 | 97.3% | ||
80% | α | 0.4236 | 0.0764 | 0.0059 | 0.3005 | 0.5466 | 0.2461 | 95.7% | |
β | 0.5977 | 0.0977 | 0.0113 | 0.4957 | 0.6997 | 0.2040 | 96.2% | ||
γ | 0.4649 | 0.0351 | 0.0022 | 0.3972 | 0.5325 | 0.1353 | 97.0% | ||
100% | α | 0.4238 | 0.0762 | 0.0058 | 0.3010 | 0.5467 | 0.2457 | 95.6% | |
β | 0.4766 | 0.0234 | 0.0023 | 0.3784 | 0.5749 | 0.1965 | 96.4% | ||
γ | 0.5277 | 0.0277 | 0.0015 | 0.4659 | 0.5894 | 0.1236 | 96.9% | ||
200 | 70% | α | 0.4219 | 0.0781 | 0.0061 | 0.3154 | 0.5285 | 0.2132 | 96.1% |
β | 0.6592 | 0.1592 | 0.0268 | 0.5675 | 0.7510 | 0.1835 | 96.3% | ||
γ | 0.4375 | 0.0625 | 0.0046 | 0.3789 | 0.4962 | 0.1173 | 96.7% | ||
80% | α | 0.4239 | 0.0761 | 0.0058 | 0.3236 | 0.5242 | 0.2006 | 96.3% | |
β | 0.5912 | 0.0912 | 0.0099 | 0.5074 | 0.6750 | 0.1676 | 97.0% | ||
γ | 0.4667 | 0.0333 | 0.0020 | 0.4101 | 0.5233 | 0.1132 | 97.5% | ||
100% | α | 0.4240 | 0.0760 | 0.0058 | 0.3372 | 0.5109 | 0.1737 | 96.5% | |
β | 0.4905 | 0.0095 | 0.0009 | 0.4167 | 0.5642 | 0.1475 | 96.7% | ||
γ | 0.5035 | 0.0035 | 0.0006 | 0.4524 | 0.5546 | 0.1022 | 97.1% | ||
n | r | Set2 (α = 0.7, β = 0.5, γ = 0.25) | |||||||
MLE | Bias | MSE | LB | UB | AL | CP | |||
50 | 70% | α | 0.4206 | 0.2794 | 0.0782 | 0.0055 | 0.8358 | 0.8304 | 97.7% |
β | 0.7016 | 0.2016 | 0.0462 | 0.5015 | 0.9016 | 0.4001 | 96.5% | ||
γ | 0.2172 | 0.0328 | 0.0017 | 0.1568 | 0.2776 | 0.1208 | 100% | ||
80% | α | 0.4214 | 0.2786 | 0.0776 | 0.2084 | 0.6345 | 0.4261 | 97.9% | |
β | 0.6361 | 0.1361 | 0.0243 | 0.4490 | 0.8231 | 0.3741 | 98.5% | ||
γ | 0.2297 | 0.0203 | 0.0010 | 0.1719 | 0.2874 | 0.1155 | 100% | ||
100% | α | 0.4234 | 0.2766 | 0.0765 | 0.2497 | 0.5970 | 0.3473 | 98.3% | |
β | 0.5161 | 0.0161 | 0.0062 | 0.3649 | 0.6673 | 0.3025 | 97.6% | ||
γ | 0.2565 | 0.0065 | 0.0007 | 0.2044 | 0.3086 | 0.1043 | 100% | ||
100 | 70% | α | 0.4210 | 0.2790 | 0.0779 | 0.0208 | 0.8212 | 0.8004 | 96.4% |
β | 0.7006 | 0.2006 | 0.0431 | 0.5593 | 0.8419 | 0.2826 | 98.0% | ||
γ | 0.2141 | 0.0359 | 0.0016 | 0.1720 | 0.2562 | 0.0842 | 100% | ||
80% | α | 0.4215 | 0.2785 | 0.0776 | 0.2708 | 0.5721 | 0.3013 | 97.2% | |
β | 0.6357 | 0.1357 | 0.0214 | 0.5035 | 0.7680 | 0.2645 | 97.7% | ||
γ | 0.2270 | 0.0230 | 0.0008 | 0.1866 | 0.2673 | 0.0807 | 100% | ||
100% | α | 0.4234 | 0.2766 | 0.0765 | 0.3006 | 0.5462 | 0.2456 | 97.3% | |
β | 0.5158 | 0.0158 | 0.0033 | 0.4088 | 0.6227 | 0.2140 | 98.2% | ||
γ | 0.2540 | 0.0040 | 0.0003 | 0.2176 | 0.2905 | 0.0729 | 100% | ||
150 | 70% | α | 0.4212 | 0.2788 | 0.0778 | 0.0330 | 0.8093 | 0.7763 | 97.7% |
β | 0.7000 | 0.2000 | 0.0419 | 0.5847 | 0.8153 | 0.2306 | 97.7% | ||
γ | 0.2122 | 0.0378 | 0.0016 | 0.1781 | 0.2463 | 0.0682 | 100% | ||
80% | α | 0.4215 | 0.2785 | 0.0776 | 0.2985 | 0.5445 | 0.2460 | 98.8% | |
β | 0.6350 | 0.1350 | 0.0203 | 0.5271 | 0.7430 | 0.2159 | 98.1% | ||
γ | 0.2259 | 0.0241 | 0.0008 | 0.1931 | 0.2587 | 0.0656 | 96.0% | ||
100% | α | 0.4234 | 0.2766 | 0.0765 | 0.3232 | 0.5237 | 0.2005 | 97.2% | |
β | 0.5151 | 0.0151 | 0.0023 | 0.4278 | 0.6024 | 0.1746 | 97.0% | ||
γ | 0.2529 | 0.0029 | 0.0002 | 0.2232 | 0.2825 | 0.0593 | 95.4% | ||
200 | 70% | α | 0.4209 | 0.2791 | 0.0779 | 0.0849 | 0.7569 | 0.6720 | 100% |
β | 0.6981 | 0.1981 | 0.0405 | 0.5984 | 0.7978 | 0.1994 | 97.2% | ||
γ | 0.2118 | 0.0382 | 0.0016 | 0.1823 | 0.2412 | 0.0589 | 97.3% | ||
80% | α | 0.4215 | 0.2785 | 0.0776 | 0.3150 | 0.5280 | 0.2131 | 100% | |
β | 0.6331 | 0.1331 | 0.0191 | 0.5398 | 0.7265 | 0.1867 | 98.2% | ||
γ | 0.2256 | 0.0244 | 0.0007 | 0.1973 | 0.2540 | 0.0567 | 98.0% | ||
100% | α | 0.4234 | 0.2766 | 0.0765 | 0.3366 | 0.5103 | 0.1737 | 100% | |
β | 0.5136 | 0.0136 | 0.0016 | 0.4381 | 0.5891 | 0.1510 | 98.8% | ||
γ | 0.2523 | 0.0023 | 0.0002 | 0.2267 | 0.2779 | 0.0512 | 100% | ||
n | r | Set3 (α = 0.7, β = 0.7, γ = 0.5) | |||||||
MLE | Bias | MSE | LB | UB | AL | CP | |||
50 | 70% | α | 0.4178 | 0.2822 | 0.0797 | 0.1937 | 0.6419 | 0.4482 | 96.2% |
β | 0.8994 | 0.1994 | 0.0477 | 0.6064 | 1.1923 | 0.5859 | 95.9% | ||
γ | 0.6151 | 0.1151 | 0.0239 | 0.3763 | 0.8540 | 0.4776 | 95.0% | ||
80% | α | 0.4193 | 0.2807 | 0.0788 | 0.2255 | 0.6131 | 0.3875 | 95.9% | |
β | 0.8238 | 0.1238 | 0.0218 | 0.5471 | 1.1006 | 0.5535 | 95.9% | ||
γ | 0.5695 | 0.0695 | 0.0182 | 0.3447 | 0.7943 | 0.4496 | 96.7% | ||
100% | α | 0.4211 | 0.2789 | 0.0778 | 0.2480 | 0.5942 | 0.3461 | 96.8% | |
β | 0.7612 | 0.0612 | 0.0104 | 0.5012 | 1.0213 | 0.5201 | 97.0% | ||
γ | 0.5425 | 0.0425 | 0.0163 | 0.3395 | 0.7456 | 0.4061 | 95.0% | ||
100 | 70% | α | 0.4174 | 0.2826 | 0.0798 | 0.2439 | 0.5910 | 0.3470 | 95.0% |
β | 0.8787 | 0.1787 | 0.0353 | 0.6426 | 1.1148 | 0.4722 | 96.3% | ||
γ | 0.5696 | 0.0696 | 0.0201 | 0.3697 | 0.7696 | 0.3998 | 96.0% | ||
80% | α | 0.4191 | 0.2809 | 0.0789 | 0.2690 | 0.5691 | 0.3001 | 95.5% | |
β | 0.7802 | 0.0802 | 0.0134 | 0.5600 | 1.0004 | 0.4404 | 95.7% | ||
γ | 0.5479 | 0.0479 | 0.0091 | 0.3814 | 0.7144 | 0.3330 | 96.0% | ||
100% | α | 0.4206 | 0.2794 | 0.0781 | 0.2866 | 0.5546 | 0.2680 | 95.6% | |
β | 0.7146 | 0.0146 | 0.0068 | 0.5069 | 0.9223 | 0.4154 | 95.7% | ||
γ | 0.5597 | 0.0597 | 0.0074 | 0.4063 | 0.7131 | 0.3067 | 96.0% | ||
150 | 70% | α | 0.4176 | 0.2824 | 0.0798 | 0.2949 | 0.5403 | 0.2454 | 95.8% |
β | 0.8697 | 0.1697 | 0.0305 | 0.7056 | 1.0338 | 0.3282 | 96.2% | ||
γ | 0.5924 | 0.0924 | 0.0174 | 0.4449 | 0.7399 | 0.2950 | 97.1% | ||
80% | α | 0.4193 | 0.2807 | 0.0788 | 0.3131 | 0.5254 | 0.2122 | 96.2% | |
β | 0.8023 | 0.1023 | 0.0124 | 0.6492 | 0.9555 | 0.3063 | 96.1% | ||
γ | 0.5524 | 0.0524 | 0.0058 | 0.4332 | 0.6716 | 0.2384 | 97.0% | ||
100% | α | 0.4209 | 0.2791 | 0.0779 | 0.3261 | 0.5156 | 0.1895 | 95.8% | |
β | 0.7374 | 0.0374 | 0.0032 | 0.5932 | 0.8817 | 0.2885 | 96.3% | ||
γ | 0.5507 | 0.0507 | 0.0041 | 0.4447 | 0.6568 | 0.2121 | 96.9% | ||
200 | 70% | α | 0.4175 | 0.2825 | 0.0798 | 0.3173 | 0.5177 | 0.2004 | 96.1% |
β | 0.8406 | 0.1406 | 0.0265 | 0.7075 | 0.9736 | 0.2661 | 97.2% | ||
γ | 0.5683 | 0.0683 | 0.0069 | 0.4569 | 0.6798 | 0.2230 | 96.9% | ||
80% | α | 0.4193 | 0.2807 | 0.0788 | 0.3327 | 0.5059 | 0.1733 | 96.3% | |
β | 0.8043 | 0.1043 | 0.0123 | 0.6790 | 0.9295 | 0.2505 | 96.6% | ||
γ | 0.5502 | 0.0502 | 0.0041 | 0.4533 | 0.6471 | 0.1938 | 97.0% | ||
100% | α | 0.4209 | 0.2791 | 0.0779 | 0.3435 | 0.4983 | 0.1548 | 96.1% | |
β | 0.7411 | 0.0411 | 0.0030 | 0.6229 | 0.8592 | 0.2362 | 97.0% | ||
γ | 0.5463 | 0.0463 | 0.0041 | 0.4589 | 0.6338 | 0.1749 | 96.2% | ||
n | r | Set4 (α = 0.6, β = 0.3, γ = 0.5) | |||||||
MLE | Bias | MSE | LB | UB | AL | CP | |||
50 | 70% | α | 0.4197 | 0.1803 | 0.0325 | 0.2456 | 0.5939 | 0.3482 | 98.1% |
β | 0.5719 | 0.2719 | 0.0744 | 0.3742 | 0.7696 | 0.3954 | 97.0% | ||
γ | 0.2990 | 0.2010 | 0.0406 | 0.1769 | 0.4211 | 0.2442 | 98.0% | ||
80% | α | 0.4221 | 0.1779 | 0.0317 | 0.2716 | 0.5725 | 0.3009 | 98.4% | |
β | 0.4934 | 0.1934 | 0.0384 | 0.3126 | 0.6742 | 0.3616 | 97.4% | ||
γ | 0.3593 | 0.1407 | 0.0200 | 0.2383 | 0.4803 | 0.2421 | 98.2% | ||
100% | α | 0.4246 | 0.1754 | 0.0308 | 0.2903 | 0.5589 | 0.2686 | 97.9% | |
β | 0.4198 | 0.1198 | 0.0150 | 0.2576 | 0.5820 | 0.3244 | 97.4% | ||
γ | 0.4172 | 0.0828 | 0.0078 | 0.2968 | 0.5376 | 0.2408 | 98.4% | ||
100 | 70% | α | 0.4198 | 0.1802 | 0.0325 | 0.2966 | 0.5429 | 0.2463 | 97.2% |
β | 0.5674 | 0.2674 | 0.0717 | 0.4278 | 0.7069 | 0.2791 | 97.7% | ||
γ | 0.3137 | 0.1863 | 0.0351 | 0.2278 | 0.3995 | 0.1717 | 98.0% | ||
80% | α | 0.4222 | 0.1778 | 0.0316 | 0.3157 | 0.5286 | 0.2128 | 97.9% | |
β | 0.4857 | 0.1857 | 0.0350 | 0.3584 | 0.6130 | 0.2546 | 97.9% | ||
γ | 0.3669 | 0.1331 | 0.0179 | 0.2818 | 0.4519 | 0.1701 | 98.7% | ||
100% | α | 0.4248 | 0.1752 | 0.0307 | 0.3242 | 0.5253 | 0.2011 | 98.1% | |
β | 0.4082 | 0.1082 | 0.0125 | 0.2944 | 0.5221 | 0.2277 | 97.8% | ||
γ | 0.4171 | 0.0829 | 0.0074 | 0.3349 | 0.4993 | 0.1644 | 98.3% | ||
150 | 70% | α | 0.4198 | 0.1802 | 0.0325 | 0.3248 | 0.5148 | 0.1899 | 97.2% |
β | 0.5653 | 0.2653 | 0.0706 | 0.4518 | 0.6788 | 0.2270 | 98.3% | ||
γ | 0.3140 | 0.1860 | 0.0350 | 0.2421 | 0.3860 | 0.1439 | 99.3% | ||
80% | α | 0.4222 | 0.1778 | 0.0316 | 0.3353 | 0.5091 | 0.1738 | 97.7% | |
β | 0.4832 | 0.1832 | 0.0338 | 0.3797 | 0.5866 | 0.2069 | 98.2% | ||
γ | 0.3677 | 0.1323 | 0.0178 | 0.2996 | 0.4358 | 0.1363 | 99.0% | ||
100% | α | 0.4248 | 0.1752 | 0.0307 | 0.3377 | 0.5119 | 0.1742 | 97.3% | |
β | 0.4018 | 0.1018 | 0.0107 | 0.3032 | 0.5004 | 0.1972 | 97.9% | ||
γ | 0.4248 | 0.0752 | 0.0061 | 0.3608 | 0.4888 | 0.1280 | 99.7% | ||
200 | 70% | α | 0.4198 | 0.1802 | 0.0325 | 0.3423 | 0.4973 | 0.1551 | 99.0% |
β | 0.5650 | 0.2650 | 0.0705 | 0.4738 | 0.6561 | 0.1823 | 99.1% | ||
γ | 0.3256 | 0.1744 | 0.0309 | 0.2637 | 0.3875 | 0.1239 | 98.7% | ||
80% | α | 0.4222 | 0.1778 | 0.0316 | 0.3470 | 0.4975 | 0.1505 | 99.6% | |
β | 0.4781 | 0.1781 | 0.0321 | 0.3882 | 0.5679 | 0.1798 | 99.3% | ||
γ | 0.3733 | 0.1267 | 0.0173 | 0.3129 | 0.4336 | 0.1206 | 99.5% | ||
100% | α | 0.4250 | 0.1750 | 0.0306 | 0.3578 | 0.4921 | 0.1343 | 98.7% | |
β | 0.3904 | 0.0904 | 0.0088 | 0.3106 | 0.4703 | 0.1596 | 99.6% | ||
γ | 0.4262 | 0.0738 | 0.0060 | 0.3681 | 0.4843 | 0.1162 | 100% |
A. For almost all the true values, the MSE of all the estimates decreases as the sample sizes and the censoring level r increase, demonstrating that the various estimates are consistent (see Table 6 and Figure 3).
B. For all true parameter values, the ALs of all the estimates decrease as the sample sizes and the censoring level r increase (see Table 6 and Figure 4).
C. For all true parameter values, the CP of all the estimates increases as the sample sizes and the censoring level r increase (see Table 6).
D. The MSE of the estimate of α at the true value of Set1 yields the lowest values in comparison to the other actual parameter values for all sample sizes (see Table 6 and Figure 5).
E. At all actual values, the MSE of the estimate of β produces the largest results for all sample sizes (see Table 6 and Figure 6). Also, it is evident that except for n=50 and 200, the MSE of β estimates obtains the smallest values for the actual value of Set1 compared to the other actual sets at the censoring level 70%. At the censoring level 80%, the MSE of β estimates gets the smallest values at all sets of parameters except at n=50.
F. The MSE of the estimate of γ at the true value of Set2 gets the smallest values in comparison to the other actual parameter values for all sample sizes (see Table 6 and Figure 7).
G. The MSEs, biases, and ALs of γ are smaller than the other estimates of α and β in almost all of the cases.
H. As n rises, the CI's lengths get shorter.
I. As n increases, parameter estimates grow increasingly accurate, suggesting that they are asymptotically unbiased.
J. For the parameter values examined, the CI's overall performance is fairly strong.
Here, we provide applications to four real data sets to illustrate the importance and potentiality of the LBTLoW distribution. The goodness-of-fit statistics for these distributions and other competitive distributions are compared, and the MLEs of their parameters are provided.
The first real data set [38] on the relief times of twenty patients receiving an analgesic is 1.1, 1.4, 3, 1.7, 2.3, 1.4, 1.3, 1.7, 2.2, 1.7, 2.7, 4.1, 1.8, 1.5, 1.9, 1.8, 1.6, 1.2, 1.6, 2.
The second dataset illustrates the proportion of global reserves of natural gas in various countries as of the year 2020. In contrast to other nations, Russia possesses the largest natural gas reserves globally and maintains its position as the leading exporter of natural gas. Iran, on the other hand, ranks second in terms of natural gas reserves worldwide. Qatar, although holding slightly over 13% of the total global natural gas reserves, also plays a significant role in the natural gas market. Lastly, Saudi Arabia possesses the fifth-largest natural gas reserves globally. The electronic address from which it was taken is as follows: https://worldpopulationreview.com/. The data set is reported in Table 7.
Rank | Country | % Global Reserves | Rank | Country | % Global Reserves |
1 | Russia | 19.9 | 23 | Ukraine | 0.6 |
2 | Iran | 17.1 | 24 | Malaysia | 0.5 |
3 | Qatar | 13.1 | 25 | Uzbekistan | 0.4 |
4 | Turkmenistan | 7.2 | 26 | Oman | 0.4 |
5 | United States | 6.7 | 27 | Vietnam | 0.3 |
6 | China | 4.5 | 28 | Israel | 0.3 |
7 | Venezuela | 3.3 | 29 | Argentina | 0.2 |
8 | Saudi Arabia | 3.2 | 30 | Pakistan | 0.2 |
9 | United Arab Emirates | 3.2 | 31 | Trinidad | 0.2 |
10 | Nigeria | 2.9 | 32 | Brazil | 0.2 |
11 | Iraq | 1.9 | 33 | Myanmar | 0.2 |
12 | Canada | 1.3 | 34 | United Kingdom | 0.1 |
13 | Australia | 1.3 | 35 | Thailand | 0.1 |
14 | Azerbaijan | 1.3 | 36 | Mexico | 0.1 |
15 | Algeria | 1.2 | 37 | Bangladesh | 0.1 |
16 | Kazakhstan | 1.2 | 38 | Netherlands | 0.1 |
17 | Egypt | 1.1 | 39 | Bolivia | 0.1 |
18 | Kuwait | 0.9 | 40 | Brunei | 0.1 |
19 | Norway | 0.8 | 41 | Peru | 0.1 |
20 | Libya | 0.8 | 42 | Syria | 0.1 |
21 | Indonesia | 0.7 | 43 | Yemen | 0.1 |
22 | India | 0.7 | 44 | Papua New Guinea | 0.1 |
The third dataset pertains to the Top 20 Countries with the Largest Oil Reserves, measured in thousand million barrels. Crude oil serves as the predominant fuel source globally and is the primary source of energy on a wide scale. In the year 2020, global oil consumption reached around 88.6 million barrels per day, or 30.1% of the overall primary energy consumption. Venezuela possesses the largest oil reserves globally, over 300 billion barrels in total. Saudi Arabia holds the world's second-largest oil reserves, with 297.5 billion barrels. The United States is the world's leading producer of oil as well as the world's greatest user of oil, necessitating additional imports from dozens of other oil-producing countries. Despite having the world's highest oil production, the United States is only 9th in the world in terms of available oil reserves. It was obtained from the following electronic address: https://worldpopulationreview.com/. The data set is reported in Table 8.
Rank | Country | reserves2020 | Rank | Country | reserves2020 |
1 | Venezuela | 303.8 | 11 | Nigeria | 36.9 |
2 | Saudi Arabia | 297.5 | 12 | Kazakhstan | 30 |
3 | Canada | 168.1 | 13 | China | 26 |
4 | Iran | 157.8 | 14 | Qatar | 25.2 |
5 | Iraq | 145 | 15 | Algeria | 12.2 |
6 | Russia | 107.8 | 16 | Brazil | 11.9 |
7 | Kuwait | 101.5 | 17 | Norway | 7.9 |
8 | United Arab Emirates | 97.8 | 18 | Angola | 7.8 |
9 | United States | 68.8 | 19 | Azerbaijan | 7 |
10 | Libya | 48.4 | 20 | Mexico | 6.1 |
The fourth data set represents the Top 100 central banks that owned the largest gold Reserves (in thousand tons). Because of its safety, liquidity, and return qualities-the three major investment objectives for central banks-gold is an essential component of central bank reserves. As such, they are significant gold holders, accounting for around one-fifth of all gold extracted throughout history. They present gold reserve data derived using IMF IFS figures to help comprehend this sector of the gold market, which records central banks' (and other official institutions, when appropriate) reported purchases and sales of gold as a percentage of their international reserves. It was obtained from the following electronic address: https://www.gold.org/. The data set is reported in Table 9.
Rank | Country | Reserves of Gold | Rank | Country | Reserves of Gold | Rank | Country | Reserves of Gold |
1 | USA | 8.1335 | 35 | LBY | 0.1166 | 68 | CYP | 0.0139 |
2 | DEU | 3.3585 | 36 | GRC | 0.1141 | 69 | CUW | 0.0131 |
3 | IMF | 2.814 | 37 | ROK | 0.1045 | 70 | MUS | 0.0124 |
4 | ITA | 2.4518 | 38 | ROU | 0.1036 | 71 | IRL | 0.012 |
5 | FRA | 2.4365 | 39 | BIS | 0.102 | 72 | CZE | 0.0109 |
6 | RUS | 2.2985 | 40 | IRQ | 0.0964 | 73 | KGZ | 0.0102 |
7 | CHN | 1.9483 | 41 | HUN | 0.0945 | 74 | GHA | 0.0087 |
8 | CHE | 1.04 | 42 | AUS | 0.0798 | 75 | PRY | 0.0082 |
9 | JPN | 0.846 | 43 | KWT | 0.079 | 76 | NPL | 0.008 |
10 | IND | 0.7604 | 44 | IDN | 0.0786 | 77 | MNG | 0.0076 |
11 | NLD | 0.6125 | 45 | DNK | 0.0666 | 78 | MMR | 0.0073 |
12 | ECB | 0.5048 | 46 | PAK | 0.0647 | 79 | GTM | 0.0069 |
13 | TUR | 0.4311 | 47 | ARG | 0.0617 | 80 | MKD | 0.0069 |
14 | TAI | 0.4236 | 48 | ARE | 0.0553 | 81 | TUN | 0.0068 |
15 | PRT | 0.3826 | 49 | BLR | 0.0535 | 82 | LVA | 0.0067 |
16 | KAZ | 0.3681 | 50 | QAT | 0.0513 | 83 | LTU | 0.0058 |
17 | UZB | 0.3375 | 51 | KHM | 0.0504 | 84 | COL | 0.0047 |
18 | SAU | 0.3231 | 52 | FIN | 0.049 | 85 | BHR | 0.0047 |
19 | GBR | 0.3103 | 53 | JOR | 0.0435 | 86 | BRN | 0.0046 |
20 | LBN | 0.2868 | 54 | BOL | 0.0425 | 87 | GIN | 0.0042 |
21 | ESP | 0.2816 | 55 | BGR | 0.0408 | 88 | MOZ | 0.0039 |
22 | AUT | 0.28 | 56 | MYS | 0.0389 | 89 | SVN | 0.0032 |
23 | THA | 0.2442 | 57 | SRB | 0.0378 | 90 | ABW | 0.0031 |
24 | POL | 0.2287 | 58 | WAEMU | 0.0365 | 91 | BIH | 0.003 |
25 | BEL | 0.2274 | 59 | PER | 0.0347 | 92 | ALB | 0.0028 |
26 | DZA | 0.1736 | 60 | SVK | 0.0317 | 93 | LUX | 0.0022 |
27 | VEN | 0.1612 | 61 | UKR | 0.0271 | 94 | HKG | 0.0021 |
28 | PHL | 0.1563 | 62 | SYR | 0.0258 | 95 | ISL | 0.002 |
29 | SGP | 0.1537 | 63 | MAR | 0.0221 | 96 | TTO | 0.0019 |
30 | BRA | 0.1297 | 64 | ECU | 0.0219 | 97 | HTI | 0.0018 |
31 | SWE | 0.1257 | 65 | AFG | 0.0219 | 98 | YEM | 0.0016 |
32 | ZAF | 0.1254 | 66 | NGA | 0.0215 | 99 | SUR | 0.0015 |
33 | EGY | 0.125 | 67 | BGD | 0.014 | 100 | SLV | 0.0014 |
34 | MEX | 0.1199 |
The descriptive analysis of all the data sets is reported in Table 10.
n | Mean | Median | Skewness | Kurtosis | Range | Min | Max | Sum | |
Data1 | 20 | 1.900 | 1.700 | 1.860 | 4.185 | 3.000 | 1.100 | 4.100 | 38.000 |
Data2 | 44 | 2.248 | 0.650 | 2.990 | 8.864 | 19.800 | 0.100 | 19.900 | 98.900 |
Data3 | 20 | 83.375 | 42.650 | 1.430 | 1.420 | 297.700 | 6.100 | 303.800 | 1667.500 |
Data4 | 100 | 0.347 | 0.050 | 5.590 | 38.257 | 8.130 | 0.001 | 8.133 | 34.676 |
These real data sets are utilized to assess the goodness of fit of the LBTLoW distribution. The suggested model is compared with exponentiated transmuted generalized Rayleigh (ETGR) [39], beta Weibull (BW) [40], transmuted Lindley (T-Li) [41], McDonald log-logistic (McLL) [42], new modified Weibull (NMW) [43], weighted exponentiated inverted Weibull (WEIW) [44], transmuted complementary Weibull geometric (TCWG) [45], transmuted modified Weibull (TMW) [46], exponentiated Kumaraswamy Weibull (EKW) [47] and Weibull (W) models.
The maximum likelihood estimators (MLEs) and standard errors (SEs) of the model parameters are computed. In order to assess the distribution models, various criteria are taken into account, including the Akaike information criterion (AIC), correct AIC (CAIC), Bayesian IC (BIC), Hannan-Quinn IC (HQIC), Kolmogorov-Smirnov (KS) test, and p-value (PV) test. In contrast, the broader dissemination is associated with reduced values of AIC, CAIC, BIC, HQIC, KS, and the highest magnitude of PV. The maximum likelihood estimators (MLEs) of the competitive models, along with their standard errors (SEs) and values of AIC, CAIC, BIC, HQIC, PV, and KS for the suggested data sets, are displayed in Tables 11-18. It has been observed that the LBTLoW distribution, characterized by three parameters, exhibits superior goodness of fit compared to alternative models. This distribution exhibits the lowest values of AIC, CAIC, BIC, HQIC, and KS, and the highest value of PV among the distributions under consideration in this analysis. Furthermore, Figures 8-15 exhibit the graphical representations of the estimated pdf, cdf, ccdf, and probability-probability (PP) plots for the competitive model applied to the given data sets.
Distributions | MLE and SE | ||||
α | β | γ | λ | θ | |
LBTLoW | 8.648 | 3.074 | 0.042 | ||
(3.545) | (0.474) | (0.025) | |||
ETGR | 0.103 | 0.692 | 23.539 | -0.342 | |
(0.436) | (0.086) | (105.137) | (1.971) | ||
BW | 0.831 | 0.613 | 29.947 | 11.632 | |
(0.954) | (0.340) | (40.414) | (21.900) | ||
T-Li | 0.665 | 0.359 | |||
(0.332) | (0.048) | ||||
McLL | 0.881 | 2.070 | 1.926 | 19.225 | 32.033 |
(0.109) | (3.693) | (5.165) | (22.341) | (43.081) | |
NMW | 0.121 | 2.784 | 2.787 | 0.003 | 0.008 |
(0.056) | (20.370) | (0.428) | (0.025) | (0.002) | |
W | 0.122 | 2.787 | |||
(0.056) | (0.427) |
Distributions | AIC | CAIC | BIC | HQIC | KS | PV |
LBTLoW | 40.140 | 41.640 | 38.040 | 40.720 | 0.146 | 0.790 |
ETGR | 44.860 | 47.520 | 42.060 | 45.630 | 0.190 | 0.465 |
BW | 42.400 | 45.060 | 39.600 | 43.170 | 0.160 | 0.683 |
T-Li | 65.730 | 66.440 | 64.330 | 66.120 | 0.380 | 0.006 |
McLL | 43.850 | 48.140 | 40.360 | 44.830 | 0.147 | 0.734 |
NMW | 51.170 | 55.460 | 47.680 | 52.150 | 0.190 | 0.501 |
W | 45.170 | 45.880 | 43.780 | 45.560 | 0.180 | 0.509 |
Distributions | MLE and SE | ||||
α | β | γ | λ | θ | |
LBTLoW | 6.268 | 0.623 | 0.484 | ||
(2.631) | (0.066) | (0.210) | |||
ETGR | 0.055 | 0.071 | 8.773 | 0.947 | |
(0.027) | (0.029) | (7.043) | (0.081) | ||
TCWG | 34.076 | 0.802 | 0.005 | 1.12 | |
(81.023) | (0.021) | (0.013) | (0.285) | ||
EKW | 0.221 | 400.298 | 5.215 | 1 | 3.823 |
(0.038) | (718.99) | (0.649) | (0.004) | (3.036) | |
TMW | 0.851 | 1.159 | -0.554 | 0.519 | |
(0.163) | (1.026) | (0.985) | (0.379) | ||
BW | 2.861 | 0.075 | 78.550 | 42.576 | |
(69.095) | (0.090) | (167.320) | (187.300) | ||
T-Li | 0.604 | 0.671 | |||
(0.155) | (0.074) | ||||
McLL | 0.181 | 1.565 | 1.286 | 21.234 | 28.124 |
(0.193) | (9.254) | (5.432) | (34.701) | (45.757) | |
NMW | 6.8 x 10−8 | 0.680 | 0.223 | 0.015 | 0.806 |
(0.623) | (0.110) | (617.48) | (0.015) | (0.418) | |
W | 0.799 | 0.621 | |||
(0.136) | (0.068) |
Distributions | AIC | CAIC | BIC | HQIC | KS | PV |
LBTLoW | 132.210 | 132.810 | 131.140 | 134.200 | 0.130 | 0.425 |
ETGR | 143.470 | 144.490 | 142.040 | 146.110 | 0.180 | 0.118 |
TCWG | 137.690 | 138.710 | 136.260 | 140.330 | 0.150 | 0.251 |
EKW | 133.890 | 135.470 | 132.110 | 140.330 | 0.140 | 0.355 |
TMW | 140.900 | 142.480 | 139.120 | 144.210 | 0.150 | 0.276 |
BW | 133.180 | 134.200 | 131.750 | 135.820 | 0.130 | 0.408 |
T-Li | 174.360 | 174.660 | 173.650 | 175.690 | 0.200 | 0.057 |
McLL | 134.830 | 136.410 | 133.040 | 138.130 | 0.130 | 0.419 |
NMW | 143.780 | 145.360 | 142.000 | 147.090 | 0.160 | 0.243 |
W | 138.650 | 138.940 | 137.940 | 139.970 | 0.170 | 0.139 |
Distributions | MLE and SE | ||||
α | β | γ | λ | θ | |
LBTLoW | 2.515 | 0.756 | 0.040 | ||
(3.877) | (0.166) | (0.048) | |||
WEIW | 0.909 | 0.871 | 7.225 | ||
(106700) | (0.152) | (384700) | |||
TMW | 0.998 | 0.459 | -0.443 | 0.202 | |
(0.081) | (18.537) | (18.537) | (0.769) | ||
T-Li | 0.021 | 0.384 | |||
(0.345) | (0.004) | ||||
McLL | 0.208 | 93.978 | 1.279 | 24.759 | 32.815 |
(0.499) | (1721) | (19.272) | (142.806) | (161.611) | |
NMW | 10.7 x 10−8 | 0.930 | 0.859 | 7.46 x 10−8 | 0.017 |
(0.001) | (0.250) | (1.216) | (0.002) | (0.017) | |
EKW | 0.167 | 261.64 | 45.725 | 1.201 | 2.138 |
(0.079) | (1709) | (219.725) | (0.741) | (7.209) |
Distributions | AIC | CAIC | BIC | HQIC | KS | PV |
LBTLoW | 221.690 | 223.190 | 219.600 | 222.280 | 0.135 | 0.857 |
WEIW | 223.400 | 224.900 | 221.300 | 223.980 | 0.157 | 0.708 |
TMW | 226.410 | 230.690 | 222.910 | 227.380 | 0.153 | 0.734 |
T-Li | 230.480 | 231.180 | 229.080 | 230.870 | 0.265 | 0.120 |
McLL | 225.990 | 230.280 | 222.500 | 222.500 | 0.146 | 0.789 |
NMW | 226.570 | 230.860 | 223.080 | 227.540 | 0.140 | 0.826 |
EKW | 226.290 | 230.570 | 222.790 | 229.300 | 0.148 | 0.776 |
Distributions | MLE and SE | ||||
α | β | γ | λ | θ | |
LBTLoW | 6.498 | 0.482 | 1.490 | ||
(2.301) | (0.034) | (0.573) | |||
EKW | 0.221 | 1096 | 4.424 | 1 | 1.717 |
(0.030) | (1376) | (1.817) | (0.001) | (0.901) | |
TMW | 0.596 | 2.612 | 0.588 | -0.523 | |
(0.057) | (0.689) | (0.256) | (0.346) | ||
BW | 134.832 | 0.073 | 49.149 | 22.930 | |
(956.622) | (0.060) | (74.497) | (46.500) | ||
WEIW | 27.512 | 0.549 | 0.094 | ||
(3272000) | (0.042) | (856.967) | |||
W | 2.648 | 0.489 | |||
(0.281) | (0.035) |
Distributions | AIC | CAIC | BIC | HQIC | KS | PV |
LBTLoW | –170.510 | –170.260 | –170.510 | –167.350 | 0.070 | 0.704 |
ETGR | –167.710 | –167.070 | –167.710 | –157.710 | 0.078 | 0.584 |
BW | –158.180 | –157.540 | –158.180 | –152.910 | 0.077 | 0.593 |
T-Li | –170.220 | –169.800 | –170.220 | –166.010 | 0.071 | 0.703 |
McLL | –170.200 | –169.950 | –170.200 | –167.040 | 0.091 | 0.383 |
W | –157.390 | –157.260 | –157.390 | –155.280 | 0.100 | 0.269 |
From the previous figures, we conclude that the LBTLoW model clearly gives the best overall fit and so may be picked as the most appropriate model for explaining data.
The LBTLo-G family of distributions is explored in this article. The LBTLo-G family of probability distributions has a number of desirable characteristics, including being very flexible and simple, containing a number of new distributions, the ability for the generated distributions' pdfs to be unimodal, decreasing, bathtub-shaped, right-skewed, and symmetric, and the ability for their hrf shapes to be increasing, decreasing, U-shaped, upside-down-shaped, or J-shaped. These include discussion of the characteristics of the LBTLo-G family, including expansion for the density function, moments, incomplete moments, and certain entropy metrics. Estimating the model parameters is done using the ML technique. A simulation study demonstrated that the estimates of the model parameters are not far from their true values. Also, the biases and mean squared errors of estimates based on censored samples are larger than those based on complete samples. As the censoring levels and sample sizes increase, the coverage probability of estimates increases in approximately most cases.
As one distribution of the LBTLo-G family, the real datasets for global reserves of oil, gold, and natural gas were chosen to fit the LBTLoW distribution. The first data set proposed was the lifetime data relating to relief times (in minutes) of patients receiving an analgesic. The second data set provides the percent of global reserves of natural gas for 44 countries. We have considered the third real data analysis of the countries with the largest oil reserves in 20 countries. We consider another real-data analysis of the central bank owning the largest gold reserves in 100 countries. This gold reserve data, compiled using international monetary funds and international financial statistics, tracks central banks' reported purchases and sales of gold as a percentage of their international reserves. The LBTLoW model typically provides superior fits in comparison to certain other alternative models, as shown by real-world data applications.
The authors declare that they have not used artificial intelligence tools in the creation of this article.
Researchers Supporting Project number (RSPD2023R548), King Saud University, Riyadh, Saudi Arabia.
The authors declare that there are no conflicts of interest.
[1] | Berx G, van Roy F (2009) Involvement of members of the cadherin superfamily in cancer. Cold Spring Harb Perspect Biol 1: a003129. |
[2] |
Ranscht B, Dours-Zimmermann MT (1991) T-cadherin, a novel cadherin cell adhesion molecule in the nervous system lacks the conserved cytoplasmic region. Neuron 7: 391-402. doi: 10.1016/0896-6273(91)90291-7
![]() |
[3] |
Pfaff D, Philippova M, Kyriakakis E, et al. (2011) Paradoxical effects of T-cadherin on squamous cell carcinoma: up- and down-regulation increase xenograft growth by distinct mechanisms. J Pathol 225: 512-524. doi: 10.1002/path.2900
![]() |
[4] | Fredette BJ, Ranscht B (1994) T-cadherin expression delineates specific regions of the developing motor axon-hindlimb projection pathway. J Neurosci 14: 7331-7346. |
[5] |
Philippova M, Joshi MB, Kyriakakis E, et al. (2009) A guide and guard: the many faces of T-cadherin. Cell Signal 21: 1035-1044. doi: 10.1016/j.cellsig.2009.01.035
![]() |
[6] |
Rubina KA, Surkova EI, Semina EV, et al. (2015) T-Cadherin Expression in Melanoma Cells Stimulates Stromal Cell Recruitment and Invasion by Regulating the Expression of Chemokines, Integrins and Adhesion Molecules. Cancers (Basel) 7: 1349-1370. doi: 10.3390/cancers7030840
![]() |
[7] | Wyder L, Vitaliti A, Schneider H, et al. (2000) Increased expression of H/T-cadherin in tumor-penetrating blood vessels. Cancer Res 60: 4682-4688. |
[8] |
Dames SA, Bang E, Haussinger D, et al. (2008) Insights into the low adhesive capacity of human T-cadherin from the NMR structure of Its N-terminal extracellular domain. J Biol Chem 283: 23485-23495. doi: 10.1074/jbc.M708335200
![]() |
[9] |
Teng MS, Hsu LA, Wu S, et al. (2015) Association of CDH13 genotypes/haplotypes with circulating adiponectin levels, metabolic syndrome, and related metabolic phenotypes: the role of the suppression effect. PLoS One 10: e0122664. doi: 10.1371/journal.pone.0122664
![]() |
[10] |
Org E, Eyheramendy S, Juhanson P, et al. (2009) Genome-wide scan identifies CDH13 as a novel susceptibility locus contributing to blood pressure determination in two European populations. Hum Mol Genet 18: 2288-2296. doi: 10.1093/hmg/ddp135
![]() |
[11] | Angst BD, Marcozzi C, Magee AI (2001) The cadherin superfamily: diversity in form and function. J Cell Sci 114: 629-641. |
[12] |
Sato M, Mori Y, Sakurada A, et al. (1998) The H-cadherin (CDH13) gene is inactivated in human lung cancer. Hum Genet 103: 96-101. doi: 10.1007/s004390050790
![]() |
[13] | Behrens J, Lowrick O, Klein-Hitpass L, et al. (1991) The E-cadherin promoter: functional analysis of a G.C-rich region and an epithelial cell-specific palindromic regulatory element. Proc Natl Acad Sci U S A 88: 11495-11499. |
[14] | Jarrard DF, Paul R, van Bokhoven A, et al. (1997) P-Cadherin is a basal cell-specific epithelial marker that is not expressed in prostate cancer. Clin Cancer Res 3: 2121-2128. |
[15] |
Bromhead C, Miller JH, McDonald FJ (2006) Regulation of T-cadherin by hormones, glucocorticoid and EGF. Gene 374: 58-67. doi: 10.1016/j.gene.2006.01.013
![]() |
[16] |
Niermann T, Schmutz S, Erne P, et al. (2003) Aryl hydrocarbon receptor ligands repress T-cadherin expression in vascular smooth muscle cells. Biochem Biophys Res Commun 300: 943-949. doi: 10.1016/S0006-291X(02)02970-4
![]() |
[17] |
Ellmann L, Joshi MB, Resink TJ, et al. (2012) BRN2 is a transcriptional repressor of CDH13 (T-cadherin) in melanoma cells. Lab Invest 92: 1788-1800. doi: 10.1038/labinvest.2012.140
![]() |
[18] |
Kuzmenko YS, Stambolsky D, Kern F, et al. (1998) Characteristics of smooth muscle cell lipoprotein binding proteins (p105/p130) as T-cadherin and regulation by positive and negative growth regulators. Biochem Biophys Res Commun 246: 489-494. doi: 10.1006/bbrc.1998.8645
![]() |
[19] |
Ciatto C, Bahna F, Zampieri N, et al. (2010) T-cadherin structures reveal a novel adhesive binding mechanism. Nat Struct Mol Biol 17: 339-347. doi: 10.1038/nsmb.1781
![]() |
[20] |
Vestal DJ, Ranscht B (1992) Glycosyl phosphatidylinositol--anchored T-cadherin mediates calcium-dependent, homophilic cell adhesion. J Cell Biol 119: 451-461. doi: 10.1083/jcb.119.2.451
![]() |
[21] |
Sacristan MP, Vestal DJ, Dours-Zimmermann MT, et al. (1993) T-cadherin 2: molecular characterization, function in cell adhesion, and coexpression with T-cadherin and N-cadherin. J Neurosci Res 34: 664-680. doi: 10.1002/jnr.490340610
![]() |
[22] |
Harrison OJ, Bahna F, Katsamba PS, et al. (2010) Two-step adhesive binding by classical cadherins. Nat Struct Mol Biol 17: 348-357. doi: 10.1038/nsmb.1784
![]() |
[23] |
Winterhalter PR, Lommel M, Ruppert T, et al. (2013) O-glycosylation of the non-canonical T-cadherin from rabbit skeletal muscle by single mannose residues. FEBS Lett 587: 3715-3721. doi: 10.1016/j.febslet.2013.09.041
![]() |
[24] |
Stambolsky DV, Kuzmenko YS, Philippova MP, et al. (1999) Identification of 130 kDa cell surface LDL-binding protein from smooth muscle cells as a partially processed T-cadherin precursor. Biochim Biophys Acta 1416: 155-160. doi: 10.1016/S0005-2736(98)00218-1
![]() |
[25] |
Mavroconstanti T, Johansson S, Winge I, et al. (2013) Functional properties of rare missense variants of human CDH13 found in adult attention deficit/hyperactivity disorder (ADHD) patients. PLoS One 8: e71445. doi: 10.1371/journal.pone.0071445
![]() |
[26] |
Wang Y, Lam KS, Yau MH, et al. (2008) Post-translational modifications of adiponectin: mechanisms and functional implications. Biochem J 409: 623-633. doi: 10.1042/BJ20071492
![]() |
[27] |
Fruebis J, Tsao TS, Javorschi S, et al. (2001) Proteolytic cleavage product of 30-kDa adipocyte complement-related protein increases fatty acid oxidation in muscle and causes weight loss in mice. Proc Natl Acad Sci U S A 98: 2005-2010. doi: 10.1073/pnas.98.4.2005
![]() |
[28] |
Pajvani UB, Hawkins M, Combs TP, et al. (2004) Complex distribution, not absolute amount of adiponectin, correlates with thiazolidinedione-mediated improvement in insulin sensitivity. J Biol Chem 279: 12152-12162. doi: 10.1074/jbc.M311113200
![]() |
[29] |
Scherer PE, Williams S, Fogliano M, et al. (1995) A novel serum protein similar to C1q, produced exclusively in adipocytes. J Biol Chem 270: 26746-26749. doi: 10.1074/jbc.270.45.26746
![]() |
[30] |
Yamauchi T, Kamon J, Ito Y, et al. (2003) Cloning of adiponectin receptors that mediate antidiabetic metabolic effects. Nature 423: 762-769. doi: 10.1038/nature01705
![]() |
[31] |
Yamauchi T, Kadowaki T (2013) Adiponectin receptor as a key player in healthy longevity and obesity-related diseases. Cell Metab 17: 185-196. doi: 10.1016/j.cmet.2013.01.001
![]() |
[32] |
Hebbard L, Ranscht B (2014) Multifaceted roles of adiponectin in cancer. Best Pract Res Clin Endocrinol Metab 28: 59-69. doi: 10.1016/j.beem.2013.11.005
![]() |
[33] |
Hug C, Wang J, Ahmad NS, et al. (2004) T-cadherin is a receptor for hexameric and high-molecular-weight forms of Acrp30/adiponectin. Proc Natl Acad Sci U S A 101: 10308-10313. doi: 10.1073/pnas.0403382101
![]() |
[34] |
Hebbard LW, Garlatti M, Young LJ, et al. (2008) T-cadherin supports angiogenesis and adiponectin association with the vasculature in a mouse mammary tumor model. Cancer Res 68: 1407-1416. doi: 10.1158/0008-5472.CAN-07-2953
![]() |
[35] |
Denzel MS, Scimia MC, Zumstein PM, et al. (2010) T-cadherin is critical for adiponectin-mediated cardioprotection in mice. J Clin Invest 120: 4342-4352. doi: 10.1172/JCI43464
![]() |
[36] |
Parker-Duffen JL, Nakamura K, Silver M, et al. (2013) T-cadherin is essential for adiponectin-mediated revascularization. J Biol Chem 288: 24886-24897. doi: 10.1074/jbc.M113.454835
![]() |
[37] |
Rivero O, Sich S, Popp S, et al. (2013) Impact of the ADHD-susceptibility gene CDH13 on development and function of brain networks. Eur Neuropsychopharmacol 23: 492-507. doi: 10.1016/j.euroneuro.2012.06.009
![]() |
[38] | Fredette BJ, Miller J, Ranscht B (1996) Inhibition of motor axon growth by T-cadherin substrata. Development 122: 3163-3171. |
[39] |
Hayano Y, Zhao H, Kobayashi H, et al. (2014) The role of T-cadherin in axonal pathway formation in neocortical circuits. Development 141: 4784-4793. doi: 10.1242/dev.108290
![]() |
[40] |
Treutlein J, Cichon S, Ridinger M, et al. (2009) Genome-wide association study of alcohol dependence. Arch Gen Psychiatry 66: 773-784. doi: 10.1001/archgenpsychiatry.2009.83
![]() |
[41] |
Johnson C, Drgon T, Liu QR, et al. (2006) Pooled association genome scanning for alcohol dependence using 104,268 SNPs: validation and use to identify alcoholism vulnerability loci in unrelated individuals from the collaborative study on the genetics of alcoholism. Am J Med Genet B Neuropsychiatr Genet 141B: 844-853. doi: 10.1002/ajmg.b.30346
![]() |
[42] |
Arias-Vasquez A, Altink ME, Rommelse NN, et al. (2011) CDH13 is associated with working memory performance in attention deficit/hyperactivity disorder. Genes Brain Behav 10: 844-851. doi: 10.1111/j.1601-183X.2011.00724.x
![]() |
[43] |
Rivero O, Selten MM, Sich S, et al. (2015) Cadherin-13, a risk gene for ADHD and comorbid disorders, impacts GABAergic function in hippocampus and cognition. Transl Psychiatry 5: e655. doi: 10.1038/tp.2015.152
![]() |
[44] | Drgonova J, Walther D, Hartstein GL, et al. (2016) Cadherin 13: human cis-regulation and selectively-altered addiction phenotypes and cerebral cortical dopamine in knockout mice. Mol Med 22. |
[45] | Shen LH, Liao MH, Tseng YC (2012) Recent advances in imaging of dopaminergic neurons for evaluation of neuropsychiatric disorders. J Biomed Biotechnol 2012: 259349. |
[46] |
Poliak S, Norovich AL, Yamagata M, et al. (2016) Muscle-type Identity of Proprioceptors Specified by Spatially Restricted Signals from Limb Mesenchyme. Cell 164: 512-525. doi: 10.1016/j.cell.2015.12.049
![]() |
[47] |
Huang ZY, Wu Y, Hedrick N, et al. (2003) T-cadherin-mediated cell growth regulation involves G2 phase arrest and requires p21(CIP1/WAF1) expression. Mol Cell Biol 23: 566-578. doi: 10.1128/MCB.23.2.566-578.2003
![]() |
[48] |
Matsuda K, Fujishima Y, Maeda N, et al. (2015) Positive feedback regulation between adiponectin and T-cadherin impacts adiponectin levels in tissue and plasma of male mice. Endocrinology 156: 934-946. doi: 10.1210/en.2014-1618
![]() |
[49] |
Niermann T, Kern F, Erne P, et al. (2000) The glycosyl phosphatidylinositol anchor of human T-cadherin binds lipoproteins. Biochem Biophys Res Commun 276: 1240-1247. doi: 10.1006/bbrc.2000.3465
![]() |
[50] | Joshi MB, Philippova M, Ivanov D, et al. (2005) T-cadherin protects endothelial cells from oxidative stress-induced apoptosis. FASEB J 19: 1737-1739. |
[51] |
Joshi MB, Ivanov D, Philippova M, et al. (2007) Integrin-linked kinase is an essential mediator for T-cadherin-dependent signaling via Akt and GSK3beta in endothelial cells. FASEB J 21: 3083-3095. doi: 10.1096/fj.06-7723com
![]() |
[52] |
Philippova M, Ivanov D, Joshi MB, et al. (2008) Identification of proteins associating with glycosylphosphatidylinositol- anchored T-cadherin on the surface of vascular endothelial cells: role for Grp78/BiP in T-cadherin-dependent cell survival. Mol Cell Biol 28: 4004-4017. doi: 10.1128/MCB.00157-08
![]() |
[53] |
Kyriakakis E, Philippova M, Joshi MB, et al. (2010) T-cadherin attenuates the PERK branch of the unfolded protein response and protects vascular endothelial cells from endoplasmic reticulum stress-induced apoptosis. Cell Signal 22: 1308-1316. doi: 10.1016/j.cellsig.2010.04.008
![]() |
[54] |
Resink TJ, Kuzmenko YS, Kern F, et al. (1999) LDL binds to surface-expressed human T-cadherin in transfected HEK293 cells and influences homophilic adhesive interactions. FEBS Lett 463: 29-34. doi: 10.1016/S0014-5793(99)01594-X
![]() |
[55] |
Rubina K, Talovskaya E, Cherenkov V, et al. (2005) LDL induces intracellular signalling and cell migration via atypical LDL-binding protein T-cadherin. Mol Cell Biochem 273: 33-41. doi: 10.1007/s11010-005-0250-5
![]() |
[56] |
Kipmen-Korgun D, Osibow K, Zoratti C, et al. (2005) T-cadherin mediates low-density lipoprotein-initiated cell proliferation via the Ca(2+)-tyrosine kinase-Erk1/2 pathway. J Cardiovasc Pharmacol 45: 418-430. doi: 10.1097/01.fjc.0000157458.91433.86
![]() |
[57] |
Ivanov D, Philippova M, Tkachuk V, et al. (2004) Cell adhesion molecule T-cadherin regulates vascular cell adhesion, phenotype and motility. Exp Cell Res 293: 207-218. doi: 10.1016/j.yexcr.2003.09.030
![]() |
[58] |
Ivanov D, Philippova M, Allenspach R, et al. (2004) T-cadherin upregulation correlates with cell-cycle progression and promotes proliferation of vascular cells. Cardiovasc Res 64: 132-143. doi: 10.1016/j.cardiores.2004.06.010
![]() |
[59] |
Philippova M, Banfi A, Ivanov D, et al. (2006) Atypical GPI-anchored T-cadherin stimulates angiogenesis in vitro and in vivo. Arterioscler Thromb Vasc Biol 26: 2222-2230. doi: 10.1161/01.ATV.0000238356.20565.92
![]() |
[60] |
Frismantiene A, Pfaff D, Frachet A, et al. (2014) Regulation of contractile signaling and matrix remodeling by T-cadherin in vascular smooth muscle cells: constitutive and insulin-dependent effects. Cell Signal 26: 1897-1908. doi: 10.1016/j.cellsig.2014.05.001
![]() |
[61] |
Kostopoulos CG, Spiroglou SG, Varakis JN, et al. (2014) Adiponectin/T-cadherin and apelin/APJ expression in human arteries and periadventitial fat: implication of local adipokine signaling in atherosclerosis? Cardiovasc Pathol 23: 131-138. doi: 10.1016/j.carpath.2014.02.003
![]() |
[62] | Fujishima Y, Maeda N, Matsuda K, et al. (2017) Adiponectin association with T-cadherin protects against neointima proliferation and atherosclerosis. FASEB J. |
[63] |
Philippova M, Suter Y, Toggweiler S, et al. (2011) T-cadherin is present on endothelial microparticles and is elevated in plasma in early atherosclerosis. Eur Heart J 32: 760-771. doi: 10.1093/eurheartj/ehq206
![]() |
[64] |
Tyrberg B, Miles P, Azizian KT, et al. (2011) T-cadherin (Cdh13) in association with pancreatic beta-cell granules contributes to second phase insulin secretion. Islets 3: 327-337. doi: 10.4161/isl.3.6.17705
![]() |
[65] | Andreeva AV, Kutuzov MA (2010) Cadherin 13 in cancer. Genes Chromosomes Cancer 49: 775-790. |
[66] |
Kong DD, Yang J, Li L, et al. (2015) T-cadherin association with clinicopathological features and prognosis in axillary lymph node-positive breast cancer. Breast Cancer Res Treat 150: 119-126. doi: 10.1007/s10549-015-3302-x
![]() |
[67] |
Lee SW (1996) H-cadherin, a novel cadherin with growth inhibitory functions and diminished expression in human breast cancer. Nat Med 2: 776-782. doi: 10.1038/nm0796-776
![]() |
[68] | Toyooka KO, Toyooka S, Virmani AK, et al. (2001) Loss of expression and aberrant methylation of the CDH13 (H-cadherin) gene in breast and lung carcinomas. Cancer Res 61: 4556-4560. |
[69] |
Miki Y, Katagiri T, Nakamura Y (1997) Infrequent mutation of the H-cadherin gene on chromosome 16q24 in human breast cancers. Jpn J Cancer Res 88: 701-704. doi: 10.1111/j.1349-7006.1997.tb00439.x
![]() |
[70] |
Celebiler Cavusoglu A, Kilic Y, Saydam S, et al. (2009) Predicting invasive phenotype with CDH1, CDH13, CD44, and TIMP3 gene expression in primary breast cancer. Cancer Sci 100: 2341-2345. doi: 10.1111/j.1349-7006.2009.01333.x
![]() |
[71] | Toyooka S, Toyooka KO, Harada K, et al. (2002) Aberrant methylation of the CDH13 (H-cadherin) promoter region in colorectal cancers and adenomas. Cancer Res 62: 3382-3386. |
[72] | Wei B, Shi H, Lu X, et al. (2015) Association between the expression of T-cadherin and vascular endothelial growth factor and the prognosis of patients with gastric cancer. Mol Med Rep 12: 2075-2081. |
[73] | Hibi K, Kodera Y, Ito K, et al. (2004) Methylation pattern of CDH13 gene in digestive tract cancers. Br J Cancer 91: 1139-1142. |
[74] |
Hibi K, Nakayama H, Kodera Y, et al. (2004) CDH13 promoter region is specifically methylated in poorly differentiated colorectal cancer. Br J Cancer 90: 1030-1033. doi: 10.1038/sj.bjc.6601647
![]() |
[75] | Scarpa M, Scarpa M, Castagliuolo I, et al. (2016) Aberrant gene methylation in non-neoplastic mucosa as a predictive marker of ulcerative colitis-associated CRC. Oncotarget 7: 10322-10331. |
[76] |
Ren JZ, Huo JR (2012) Correlation between T-cadherin gene expression and aberrant methylation of T-cadherin promoter in human colon carcinoma cells. Med Oncol 29: 915-918. doi: 10.1007/s12032-011-9836-9
![]() |
[77] | Zhong Y, Delgado Y, Gomez J, et al. (2001) Loss of H-cadherin protein expression in human non-small cell lung cancer is associated with tumorigenicity. Clin Cancer Res 7: 1683-1687. |
[78] |
Brock MV, Hooker CM, Ota-Machida E, et al. (2008) DNA methylation markers and early recurrence in stage I lung cancer. N Engl J Med 358: 1118-1128. doi: 10.1056/NEJMoa0706550
![]() |
[79] |
Zhou S, Matsuyoshi N, Liang SB, et al. (2002) Expression of T-cadherin in Basal keratinocytes of skin. J Invest Dermatol 118: 1080-1084. doi: 10.1046/j.1523-1747.2002.01795.x
![]() |
[80] |
Takeuchi T, Liang SB, Matsuyoshi N, et al. (2002) Loss of T-cadherin (CDH13, H-cadherin) expression in cutaneous squamous cell carcinoma. Lab Invest 82: 1023-1029. doi: 10.1097/01.LAB.0000025391.35798.F1
![]() |
[81] |
Mukoyama Y, Zhou S, Miyachi Y, et al. (2005) T-cadherin negatively regulates the proliferation of cutaneous squamous carcinoma cells. J Invest Dermatol 124: 833-838. doi: 10.1111/j.0022-202X.2005.23660.x
![]() |
[82] | Mukoyama Y, Utani A, Matsui S, et al. (2007) T-cadherin enhances cell-matrix adhesiveness by regulating beta1 integrin trafficking in cutaneous squamous carcinoma cells. Genes Cells 12: 787-796. |
[83] |
Pfaff D, Philippova M, Buechner SA, et al. (2010) T-cadherin loss induces an invasive phenotype in human keratinocytes and squamous cell carcinoma (SCC) cells in vitro and is associated with malignant transformation of cutaneous SCC in vivo. Br J Dermatol 163: 353-363. doi: 10.1111/j.1365-2133.2010.09801.x
![]() |
[84] |
Kyriakakis E, Maslova K, Philippova M, et al. (2012) T-Cadherin is an auxiliary negative regulator of EGFR pathway activity in cutaneous squamous cell carcinoma: impact on cell motility. J Invest Dermatol 132: 2275-2285. doi: 10.1038/jid.2012.131
![]() |
[85] |
Philippova M, Pfaff D, Kyriakakis E, et al. (2013) T-cadherin loss promotes experimental metastasis of squamous cell carcinoma. Eur J Cancer 49: 2048-2058. doi: 10.1016/j.ejca.2012.12.026
![]() |
[86] |
Wang XD, Wang BE, Soriano R, et al. (2007) Expression profiling of the mouse prostate after castration and hormone replacement: implication of H-cadherin in prostate tumorigenesis. Differentiation 75: 219-234. doi: 10.1111/j.1432-0436.2006.00135.x
![]() |
[87] | Dasen B, Vlajnic T, Mengus C, et al. (2016) T-cadherin in prostate cancer: relationship with cancer progression, differentiation and drug resistance. J Pathol Clin Res 3: 44-57. |
[88] |
Thomas G, Jacobs KB, Yeager M, et al. (2008) Multiple loci identified in a genome-wide association study of prostate cancer. Nat Genet 40: 310-315. doi: 10.1038/ng.91
![]() |
[89] |
Maslova K, Kyriakakis E, Pfaff D, et al. (2015) EGFR and IGF-1R in regulation of prostate cancer cell phenotype and polarity: opposing functions and modulation by T-cadherin. FASEB J 29: 494-507. doi: 10.1096/fj.14-249367
![]() |
[90] |
Lin Y, Sun G, Liu X, et al. (2011) Clinical significance of T-cadherin tissue expression in patients with bladder transitional cell carcinoma. Urol Int 86: 340-345. doi: 10.1159/000322962
![]() |
[91] |
Lin YL, Liu XQ, Li WP, et al. (2012) Promoter methylation of H-cadherin is a potential biomarker in patients with bladder transitional cell carcinoma. Int Urol Nephrol 44: 111-117. doi: 10.1007/s11255-011-9961-6
![]() |
[92] |
Lin YL, Sun G, Liu XQ, et al. (2011) Clinical significance of CDH13 promoter methylation in serum samples from patients with bladder transitional cell carcinoma. J Int Med Res 39: 179-186. doi: 10.1177/147323001103900119
![]() |
[93] |
Lin YL, Xie PG, Ma JG (2014) Aberrant methylation of CDH13 is a potential biomarker for predicting the recurrence and progression of non muscle invasive bladder cancer. Med Sci Monit 20: 1572-1577. doi: 10.12659/MSM.892130
![]() |
[94] |
Lin YL, He ZK, Li ZG, et al. (2013) Downregulation of CDH13 expression promotes invasiveness of bladder transitional cell carcinoma. Urol Int 90: 225-232. doi: 10.1159/000345054
![]() |
[95] |
Roman-Gomez J, Castillejo JA, Jimenez A, et al. (2003) Cadherin-13, a mediator of calcium-dependent cell-cell adhesion, is silenced by methylation in chronic myeloid leukemia and correlates with pretreatment risk profile and cytogenetic response to interferon alfa. J Clin Oncol 21: 1472-1479. doi: 10.1200/JCO.2003.08.166
![]() |
[96] |
Sakai M, Hibi K, Koshikawa K, et al. (2004) Frequent promoter methylation and gene silencing of CDH13 in pancreatic cancer. Cancer Sci 95: 588-591. doi: 10.1111/j.1349-7006.2004.tb02491.x
![]() |
[97] |
Jee SH, Sull JW, Lee JE, et al. (2010) Adiponectin concentrations: a genome-wide association study. Am J Hum Genet 87: 545-552. doi: 10.1016/j.ajhg.2010.09.004
![]() |
[98] |
Choi JR, Jang Y, Kim Yoon S, et al. (2015) The Impact of CDH13 Polymorphism and Statin Administration on TG/HDL Ratio in Cardiovascular Patients. Yonsei Med J 56: 1604-1612. doi: 10.3349/ymj.2015.56.6.1604
![]() |
[99] |
Wu Y, Li Y, Lange EM, et al. (2010) Genome-wide association study for adiponectin levels in Filipino women identifies CDH13 and a novel uncommon haplotype at KNG1-ADIPOQ. Hum Mol Genet 19: 4955-4964. doi: 10.1093/hmg/ddq423
![]() |
[100] | Nicolas A, Aubert R, Bellili-Munoz N, et al. (2016) T-cadherin gene variants are associated with type 2 diabetes and the Fatty Liver Index in the French population. Diabetes Metab. |
[101] |
Chung CM, Lin TH, Chen JW, et al. (2011) A genome-wide association study reveals a quantitative trait locus of adiponectin on CDH13 that predicts cardiometabolic outcomes. Diabetes 60: 2417-2423. doi: 10.2337/db10-1321
![]() |
[102] |
Fava C, Danese E, Montagnana M, et al. (2011) A variant upstream of the CDH13 adiponectin receptor gene and metabolic syndrome in Swedes. Am J Cardiol 108: 1432-1437. doi: 10.1016/j.amjcard.2011.06.068
![]() |
[103] |
Park J, Kim I, Jung KJ, et al. (2015) Gene-gene interaction analysis identifies a new genetic risk factor for colorectal cancer. J Biomed Sci 22: 73. doi: 10.1186/s12929-015-0180-9
![]() |
[104] | Kudrjashova E, Bashtrikov P, Bochkov V, et al. (2002) Expression of adhesion molecule T-cadherin is increased during neointima formation in experimental restenosis. Histochem Cell Biol 118: 281-290. |
1. | Najwan Alsadat, Amal S Hassan, Mohammed Elgarhy, Vasili B V Nagarjuna, Sid Ahmed Benchiha, Ahmed M Gemeay, A novel asymmetric extension of power XLindley distribution: properties, inference and applications to engineering data, 2024, 99, 0031-8949, 105262, 10.1088/1402-4896/ad77fa | |
2. | Mohammed Elgarhy, Arne Johannssen, Mohamed Kayid, An extended Rayleigh Weibull model with actuarial measures and applications, 2024, 10, 24058440, e32143, 10.1016/j.heliyon.2024.e32143 | |
3. | Naif Alotaibi, A.S. Al-Moisheer, Amal S. Hassan, Ibrahim Elbatal, Salem A. Alyami, Ehab M. Almetwally, Epidemiological modeling of COVID-19 data with Advanced statistical inference based on Type-II progressive censoring, 2024, 10, 24058440, e36774, 10.1016/j.heliyon.2024.e36774 | |
4. | Sara Almakhareez, Loai Alzoubi, Extension to Benrabia distribution with applications and parameter estimation, 2024, 2, 2768-4520, 10.1080/27684520.2024.2393597 | |
5. | Abdullah M. Alomair, Ayesha Babar, Muhammad Ahsan-ul-Haq, Saadia Tariq, An improved extension of Xgamma distribution: Its properties, estimation and application on failure time data, 2025, 24058440, e41976, 10.1016/j.heliyon.2025.e41976 | |
6. | Ahmed W. Shawki, Mohamed Kayid, Oluwafemi Samson Balogun, Tamer S. Helal, Modeling to radiotherapy, environmental and engineering data: Using a new approach to generating family of distributions, 2025, 18, 16878507, 101317, 10.1016/j.jrras.2025.101317 | |
7. | Mohammed Elgarhy, Amal S. Hassan, Najwan Alsadat, Oluwafemi Samson Balogun, Ahmed W. Shawki, Ibrahim E. Ragab, A Heavy Tailed Model Based on Power XLindley Distribution with Actuarial Data Applications, 2025, 142, 1526-1506, 2547, 10.32604/cmes.2025.058362 |
γ | α | μ′1 | μ′2 | μ′3 | μ′4 | σ2 | CS | CK | CV |
0.4 | 0.2 | 2.629 | 8.323 | 30.531 | 126.387 | 1.413 | 0.729 | 3.596 | 0.452 |
0.6 | 0.5 | 1.558 | 2.937 | 6.430 | 15.922 | 0.508 | 0.746 | 3.627 | 0.457 |
0.8 | 0.8 | 1.184 | 1.700 | 2.847 | 5.403 | 0.300 | 0.765 | 3.660 | 0.463 |
1.1 | 1.2 | 0.925 | 1.044 | 1.380 | 2.074 | 0.189 | 0.793 | 3.717 | 0.470 |
1.3 | 1.5 | 0.805 | 0.794 | 0.920 | 1.216 | 0.146 | 0.814 | 3.758 | 0.475 |
1.7 | 1.8 | 0.705 | 0.615 | 0.634 | 0.748 | 0.117 | 0.856 | 3.853 | 0.486 |
1.9 | 2.0 | 0.655 | 0.532 | 0.513 | 0.568 | 0.103 | 0.878 | 3.906 | 0.491 |
2.4 | 2.3 | 0.582 | 0.425 | 0.371 | 0.374 | 0.086 | 0.937 | 4.058 | 0.503 |
2.7 | 2.6 | 0.530 | 0.355 | 0.285 | 0.266 | 0.073 | 0.974 | 4.162 | 0.511 |
3.2 | 3.0 | 0.469 | 0.280 | 0.203 | 0.172 | 0.060 | 1.038 | 4.359 | 0.522 |
γ | α | μ′1 | μ′2 | μ′3 | μ′4 | σ2 | CS | CK | CV |
0.4 | 0.2 | 1.964 | 4.277 | 10.154 | 25.945 | 0.420 | 0.380 | 3.017 | 0.330 |
0.6 | 0.5 | 1.347 | 2.017 | 3.298 | 5.813 | 0.202 | 0.395 | 3.026 | 0.334 |
0.8 | 0.8 | 1.105 | 1.360 | 1.829 | 2.658 | 0.139 | 0.411 | 3.037 | 0.337 |
1.1 | 1.2 | 0.924 | 0.954 | 1.081 | 1.325 | 0.100 | 0.435 | 3.058 | 0.343 |
1.3 | 1.5 | 0.836 | 0.783 | 0.805 | 0.897 | 0.084 | 0.453 | 3.075 | 0.346 |
1.7 | 1.8 | 0.760 | 0.649 | 0.612 | 0.627 | 0.072 | 0.489 | 3.116 | 0.353 |
1.9 | 2.0 | 0.720 | 0.584 | 0.524 | 0.511 | 0.066 | 0.508 | 3.140 | 0.356 |
2.4 | 2.3 | 0.661 | 0.494 | 0.411 | 0.374 | 0.058 | 0.557 | 3.213 | 0.365 |
2.7 | 2.6 | 0.617 | 0.433 | 0.339 | 0.290 | 0.052 | 0.588 | 3.265 | 0.369 |
3.2 | 3.0 | 0.565 | 0.364 | 0.263 | 0.209 | 0.045 | 0.641 | 3.366 | 0.377 |
β | α | μ′1 | μ′2 | μ′3 | μ′4 | σ2 | CS | CK | CV |
1.5 | 0.2 | 0.029 | 0.026 | 0.023 | 0.021 | 0.025 | 5.273 | 29.151 | 5.386 |
0.5 | 0.032 | 0.028 | 0.025 | 0.023 | 0.027 | 5.014 | 26.463 | 5.138 | |
0.8 | 0.035 | 0.031 | 0.028 | 0.025 | 0.030 | 4.772 | 24.069 | 4.906 | |
1.2 | 0.039 | 0.035 | 0.031 | 0.028 | 0.033 | 4.472 | 21.275 | 4.622 | |
1.5 | 0.043 | 0.038 | 0.033 | 0.03 | 0.036 | 4.264 | 19.441 | 4.424 | |
1.8 | 0.046 | 0.041 | 0.036 | 0.033 | 0.038 | 4.069 | 17.801 | 4.241 | |
2 | 0.049 | 0.043 | 0.038 | 0.034 | 0.040 | 3.946 | 16.806 | 4.125 | |
2.3 | 0.053 | 0.046 | 0.041 | 0.037 | 0.043 | 3.771 | 15.445 | 3.961 | |
2.6 | 0.056 | 0.049 | 0.044 | 0.039 | 0.046 | 3.607 | 14.225 | 3.809 | |
3 | 0.062 | 0.054 | 0.048 | 0.043 | 0.050 | 3.405 | 12.794 | 3.621 | |
2.5 | 0.2 | 0.006 | 0.005 | 0.005 | 0.004 | 0.005 | 12.420 | 156.328 | 12.403 |
0.5 | 0.007 | 0.006 | 0.006 | 0.005 | 0.006 | 11.672 | 138.195 | 11.665 | |
0.8 | 0.007 | 0.007 | 0.006 | 0.006 | 0.007 | 10.981 | 122.434 | 10.984 | |
1.2 | 0.009 | 0.008 | 0.007 | 0.007 | 0.008 | 10.140 | 104.548 | 10.156 | |
1.5 | 0.01 | 0.009 | 0.008 | 0.007 | 0.009 | 9.563 | 93.121 | 9.589 | |
1.8 | 0.011 | 0.010 | 0.009 | 0.008 | 0.010 | 9.030 | 83.140 | 9.066 | |
2 | 0.012 | 0.011 | 0.010 | 0.009 | 0.010 | 8.697 | 77.190 | 8.739 | |
2.3 | 0.013 | 0.012 | 0.011 | 0.010 | 0.012 | 8.227 | 69.194 | 8.279 | |
2.6 | 0.014 | 0.013 | 0.012 | 0.011 | 0.013 | 7.792 | 62.179 | 7.853 | |
3 | 0.017 | 0.015 | 0.014 | 0.013 | 0.015 | 7.261 | 54.128 | 7.334 |
ε | β | α | γ | RE | HaCE | ArE | TsE |
1.5 | 0.25 | 0.2 | 0.4 | 3.331 | 3.340 | 2.767 | 1.957 |
0.5 | 0.6 | 3.252 | 3.333 | 2.753 | 1.953 | ||
0.8 | 0.8 | 3.099 | 3.318 | 2.722 | 1.944 | ||
1.2 | 1.1 | 2.875 | 3.290 | 2.670 | 1.927 | ||
1.5 | 1.3 | 2.716 | 3.264 | 2.627 | 1.912 | ||
1.8 | 1.7 | 2.524 | 3.227 | 2.568 | 1.891 | ||
2.0 | 1.9 | 2.415 | 3.203 | 2.53 | 1.876 | ||
2.3 | 2.4 | 2.229 | 3.152 | 2.458 | 1.846 | ||
2.6 | 2.7 | 2.084 | 3.104 | 2.394 | 1.818 | ||
3.0 | 3.2 | 1.889 | 3.026 | 2.296 | 1.773 | ||
0.5 | 0.2 | 0.4 | 1.930 | 3.044 | 2.318 | 1.783 | |
0.5 | 0.6 | 1.924 | 3.042 | 2.315 | 1.782 | ||
0.8 | 0.8 | 1.824 | 2.996 | 2.260 | 1.755 | ||
1.2 | 1.1 | 1.660 | 2.909 | 2.161 | 1.704 | ||
1.5 | 1.3 | 1.541 | 2.835 | 2.081 | 1.661 | ||
1.8 | 1.7 | 1.392 | 2.726 | 1.969 | 1.597 | ||
2.0 | 1.9 | 1.308 | 2.657 | 1.901 | 1.556 | ||
2.3 | 2.4 | 1.161 | 2.517 | 1.770 | 1.475 | ||
2.6 | 2.7 | 1.051 | 2.397 | 1.661 | 1.404 | ||
3.0 | 3.2 | 0.903 | 2.207 | 1.500 | 1.293 | ||
2.0 | 0.25 | 0.2 | 0.4 | 2.180 | 1.987 | 1.837 | 0.993 |
0.5 | 0.6 | 2.167 | 1.986 | 1.835 | 0.993 | ||
0.8 | 0.8 | 2.053 | 1.982 | 1.812 | 0.991 | ||
1.2 | 1.1 | 1.876 | 1.973 | 1.769 | 0.987 | ||
1.5 | 1.3 | 1.753 | 1.965 | 1.734 | 0.982 | ||
1.8 | 1.7 | 1.595 | 1.949 | 1.681 | 0.975 | ||
2.0 | 1.9 | 1.510 | 1.938 | 1.648 | 0.969 | ||
2.3 | 2.4 | 1.358 | 1.912 | 1.581 | 0.956 | ||
2.6 | 2.7 | 1.247 | 1.887 | 1.524 | 0.943 | ||
3.0 | 3.2 | 1.098 | 1.840 | 1.435 | 0.920 | ||
0.5 | 0.2 | 0.4 | 1.210 | 1.877 | 1.503 | 0.938 | |
0.5 | 0.6 | 1.279 | 1.895 | 1.541 | 0.947 | ||
0.8 | 0.8 | 1.210 | 1.877 | 1.503 | 0.938 | ||
1.2 | 1.1 | 1.080 | 1.834 | 1.423 | 0.917 | ||
1.5 | 1.3 | 0.987 | 1.794 | 1.358 | 0.897 | ||
1.8 | 1.7 | 0.861 | 1.725 | 1.258 | 0.862 | ||
2.0 | 1.9 | 0.795 | 1.679 | 1.199 | 0.840 | ||
2.3 | 2.4 | 0.672 | 1.574 | 1.077 | 0.787 | ||
2.6 | 2.7 | 0.587 | 1.482 | 0.982 | 0.741 | ||
3.0 | 3.2 | 0.470 | 1.323 | 0.836 | 0.661 |
ε | β | α | RE | HaCE | ArE | TsE |
1.5 | 0.25 | 0.2 | 7.001 | 3.311 | 2.709 | 1.94 |
0.5 | 7.075 | 3.315 | 2.716 | 1.942 | ||
0.8 | 7.156 | 3.319 | 2.724 | 1.944 | ||
1.2 | 7.274 | 3.324 | 2.735 | 1.947 | ||
1.5 | 7.372 | 3.329 | 2.743 | 1.95 | ||
1.8 | 7.476 | 3.333 | 2.752 | 1.952 | ||
2 | 7.55 | 3.336 | 2.758 | 1.954 | ||
2.3 | 7.667 | 3.34 | 2.767 | 1.957 | ||
2.6 | 7.792 | 3.345 | 2.777 | 1.959 | ||
3 | 7.971 | 3.351 | 2.79 | 1.963 | ||
0.4 | 0.2 | 6.441 | 3.278 | 2.65 | 1.92 | |
0.5 | 6.452 | 3.279 | 2.651 | 1.921 | ||
0.8 | 6.469 | 3.28 | 2.653 | 1.921 | ||
1.2 | 6.503 | 3.282 | 2.657 | 1.923 | ||
1.5 | 6.536 | 3.284 | 2.66 | 1.924 | ||
1.8 | 6.577 | 3.287 | 2.665 | 1.925 | ||
2 | 6.608 | 3.289 | 2.668 | 1.927 | ||
2.3 | 6.661 | 3.292 | 2.674 | 1.928 | ||
2.6 | 6.721 | 3.296 | 2.681 | 1.931 | ||
3 | 6.813 | 3.301 | 2.69 | 1.934 | ||
2.0 | 0.25 | 0.2 | 4.376 | 1.975 | 1.776 | 0.987 |
0.5 | 4.429 | 1.976 | 1.782 | 0.988 | ||
0.8 | 4.487 | 1.977 | 1.788 | 0.989 | ||
1.2 | 4.57 | 1.979 | 1.796 | 0.99 | ||
1.5 | 4.639 | 1.981 | 1.803 | 0.99 | ||
1.8 | 4.713 | 1.982 | 1.81 | 0.991 | ||
2 | 4.765 | 1.983 | 1.815 | 0.991 | ||
2.3 | 4.847 | 1.984 | 1.823 | 0.992 | ||
2.6 | 4.934 | 1.986 | 1.83 | 0.993 | ||
3 | 5.058 | 1.987 | 1.841 | 0.994 | ||
0.4 | 0.2 | 3.975 | 1.962 | 1.726 | 0.981 | |
0.5 | 3.987 | 1.963 | 1.728 | 0.981 | ||
0.8 | 4.003 | 1.963 | 1.73 | 0.982 | ||
1.2 | 4.031 | 1.965 | 1.734 | 0.982 | ||
1.5 | 4.058 | 1.965 | 1.737 | 0.983 | ||
1.8 | 4.09 | 1.967 | 1.741 | 0.983 | ||
2 | 4.114 | 1.967 | 1.744 | 0.984 | ||
2.3 | 4.154 | 1.969 | 1.749 | 0.984 | ||
2.6 | 4.199 | 1.97 | 1.755 | 0.985 | ||
3 | 4.267 | 1.972 | 1.763 | 0.986 |
n | r | Set1 (α = 0.5, β = 0.5, γ = 0.5) | |||||||
MLE | Bias | MSE | LB | UB | AL | CP | |||
50 | 70% | α | 0.4204 | 0.0796 | 0.0064 | 0.0019 | 0.839 | 0.8370 | 97.4% |
β | 0.7041 | 0.2041 | 0.0471 | 0.5036 | 0.9046 | 0.4010 | 96.9% | ||
γ | 0.4201 | 0.0799 | 0.0069 | 0.3023 | 0.5379 | 0.2356 | 96.0% | ||
80% | α | 0.4218 | 0.0782 | 0.0061 | 0.0191 | 0.8245 | 0.8053 | 94.8% | |
β | 0.6382 | 0.1382 | 0.0242 | 0.4508 | 0.8256 | 0.3748 | 95.8% | ||
γ | 0.4386 | 0.0614 | 0.0053 | 0.3282 | 0.5490 | 0.2208 | 97.1% | ||
100% | α | 0.4234 | 0.0766 | 0.0059 | 0.0357 | 0.8111 | 0.7754 | 95.4% | |
β | 0.5177 | 0.0177 | 0.0056 | 0.3661 | 0.6694 | 0.3033 | 95.5% | ||
γ | 0.5316 | 0.0316 | 0.0027 | 0.4303 | 0.6328 | 0.2025 | 96.0% | ||
100 | 70% | α | 0.4213 | 0.0787 | 0.0062 | 0.0844 | 0.7583 | 0.6740 | 96.2% |
β | 0.6750 | 0.1750 | 0.0312 | 0.5375 | 0.8125 | 0.2750 | 95.9% | ||
γ | 0.4237 | 0.0763 | 0.0065 | 0.3389 | 0.5084 | 0.1694 | 96.0% | ||
80% | α | 0.4230 | 0.0770 | 0.0061 | 0.2099 | 0.6360 | 0.4262 | 96.2% | |
β | 0.6099 | 0.1099 | 0.0127 | 0.4819 | 0.7379 | 0.2560 | 96.1% | ||
γ | 0.4487 | 0.0513 | 0.0033 | 0.3652 | 0.5321 | 0.1669 | 97.3% | ||
100% | α | 0.4238 | 0.0762 | 0.0058 | 0.2501 | 0.5975 | 0.3473 | 95.6% | |
β | 0.4683 | 0.0317 | 0.0027 | 0.3558 | 0.5807 | 0.2249 | 95.8% | ||
γ | 0.4967 | 0.0033 | 0.0025 | 0.4199 | 0.5734 | 0.1535 | 96.0% | ||
150 | 70% | α | 0.4217 | 0.0783 | 0.0061 | 0.2710 | 0.5725 | 0.3015 | 95.2% |
β | 0.6626 | 0.1626 | 0.0281 | 0.5577 | 0.7675 | 0.2097 | 95.6% | ||
γ | 0.4277 | 0.0723 | 0.0058 | 0.3571 | 0.4983 | 0.1412 | 97.3% | ||
80% | α | 0.4236 | 0.0764 | 0.0059 | 0.3005 | 0.5466 | 0.2461 | 95.7% | |
β | 0.5977 | 0.0977 | 0.0113 | 0.4957 | 0.6997 | 0.2040 | 96.2% | ||
γ | 0.4649 | 0.0351 | 0.0022 | 0.3972 | 0.5325 | 0.1353 | 97.0% | ||
100% | α | 0.4238 | 0.0762 | 0.0058 | 0.3010 | 0.5467 | 0.2457 | 95.6% | |
β | 0.4766 | 0.0234 | 0.0023 | 0.3784 | 0.5749 | 0.1965 | 96.4% | ||
γ | 0.5277 | 0.0277 | 0.0015 | 0.4659 | 0.5894 | 0.1236 | 96.9% | ||
200 | 70% | α | 0.4219 | 0.0781 | 0.0061 | 0.3154 | 0.5285 | 0.2132 | 96.1% |
β | 0.6592 | 0.1592 | 0.0268 | 0.5675 | 0.7510 | 0.1835 | 96.3% | ||
γ | 0.4375 | 0.0625 | 0.0046 | 0.3789 | 0.4962 | 0.1173 | 96.7% | ||
80% | α | 0.4239 | 0.0761 | 0.0058 | 0.3236 | 0.5242 | 0.2006 | 96.3% | |
β | 0.5912 | 0.0912 | 0.0099 | 0.5074 | 0.6750 | 0.1676 | 97.0% | ||
γ | 0.4667 | 0.0333 | 0.0020 | 0.4101 | 0.5233 | 0.1132 | 97.5% | ||
100% | α | 0.4240 | 0.0760 | 0.0058 | 0.3372 | 0.5109 | 0.1737 | 96.5% | |
β | 0.4905 | 0.0095 | 0.0009 | 0.4167 | 0.5642 | 0.1475 | 96.7% | ||
γ | 0.5035 | 0.0035 | 0.0006 | 0.4524 | 0.5546 | 0.1022 | 97.1% | ||
n | r | Set2 (α = 0.7, β = 0.5, γ = 0.25) | |||||||
MLE | Bias | MSE | LB | UB | AL | CP | |||
50 | 70% | α | 0.4206 | 0.2794 | 0.0782 | 0.0055 | 0.8358 | 0.8304 | 97.7% |
β | 0.7016 | 0.2016 | 0.0462 | 0.5015 | 0.9016 | 0.4001 | 96.5% | ||
γ | 0.2172 | 0.0328 | 0.0017 | 0.1568 | 0.2776 | 0.1208 | 100% | ||
80% | α | 0.4214 | 0.2786 | 0.0776 | 0.2084 | 0.6345 | 0.4261 | 97.9% | |
β | 0.6361 | 0.1361 | 0.0243 | 0.4490 | 0.8231 | 0.3741 | 98.5% | ||
γ | 0.2297 | 0.0203 | 0.0010 | 0.1719 | 0.2874 | 0.1155 | 100% | ||
100% | α | 0.4234 | 0.2766 | 0.0765 | 0.2497 | 0.5970 | 0.3473 | 98.3% | |
β | 0.5161 | 0.0161 | 0.0062 | 0.3649 | 0.6673 | 0.3025 | 97.6% | ||
γ | 0.2565 | 0.0065 | 0.0007 | 0.2044 | 0.3086 | 0.1043 | 100% | ||
100 | 70% | α | 0.4210 | 0.2790 | 0.0779 | 0.0208 | 0.8212 | 0.8004 | 96.4% |
β | 0.7006 | 0.2006 | 0.0431 | 0.5593 | 0.8419 | 0.2826 | 98.0% | ||
γ | 0.2141 | 0.0359 | 0.0016 | 0.1720 | 0.2562 | 0.0842 | 100% | ||
80% | α | 0.4215 | 0.2785 | 0.0776 | 0.2708 | 0.5721 | 0.3013 | 97.2% | |
β | 0.6357 | 0.1357 | 0.0214 | 0.5035 | 0.7680 | 0.2645 | 97.7% | ||
γ | 0.2270 | 0.0230 | 0.0008 | 0.1866 | 0.2673 | 0.0807 | 100% | ||
100% | α | 0.4234 | 0.2766 | 0.0765 | 0.3006 | 0.5462 | 0.2456 | 97.3% | |
β | 0.5158 | 0.0158 | 0.0033 | 0.4088 | 0.6227 | 0.2140 | 98.2% | ||
γ | 0.2540 | 0.0040 | 0.0003 | 0.2176 | 0.2905 | 0.0729 | 100% | ||
150 | 70% | α | 0.4212 | 0.2788 | 0.0778 | 0.0330 | 0.8093 | 0.7763 | 97.7% |
β | 0.7000 | 0.2000 | 0.0419 | 0.5847 | 0.8153 | 0.2306 | 97.7% | ||
γ | 0.2122 | 0.0378 | 0.0016 | 0.1781 | 0.2463 | 0.0682 | 100% | ||
80% | α | 0.4215 | 0.2785 | 0.0776 | 0.2985 | 0.5445 | 0.2460 | 98.8% | |
β | 0.6350 | 0.1350 | 0.0203 | 0.5271 | 0.7430 | 0.2159 | 98.1% | ||
γ | 0.2259 | 0.0241 | 0.0008 | 0.1931 | 0.2587 | 0.0656 | 96.0% | ||
100% | α | 0.4234 | 0.2766 | 0.0765 | 0.3232 | 0.5237 | 0.2005 | 97.2% | |
β | 0.5151 | 0.0151 | 0.0023 | 0.4278 | 0.6024 | 0.1746 | 97.0% | ||
γ | 0.2529 | 0.0029 | 0.0002 | 0.2232 | 0.2825 | 0.0593 | 95.4% | ||
200 | 70% | α | 0.4209 | 0.2791 | 0.0779 | 0.0849 | 0.7569 | 0.6720 | 100% |
β | 0.6981 | 0.1981 | 0.0405 | 0.5984 | 0.7978 | 0.1994 | 97.2% | ||
γ | 0.2118 | 0.0382 | 0.0016 | 0.1823 | 0.2412 | 0.0589 | 97.3% | ||
80% | α | 0.4215 | 0.2785 | 0.0776 | 0.3150 | 0.5280 | 0.2131 | 100% | |
β | 0.6331 | 0.1331 | 0.0191 | 0.5398 | 0.7265 | 0.1867 | 98.2% | ||
γ | 0.2256 | 0.0244 | 0.0007 | 0.1973 | 0.2540 | 0.0567 | 98.0% | ||
100% | α | 0.4234 | 0.2766 | 0.0765 | 0.3366 | 0.5103 | 0.1737 | 100% | |
β | 0.5136 | 0.0136 | 0.0016 | 0.4381 | 0.5891 | 0.1510 | 98.8% | ||
γ | 0.2523 | 0.0023 | 0.0002 | 0.2267 | 0.2779 | 0.0512 | 100% | ||
n | r | Set3 (α = 0.7, β = 0.7, γ = 0.5) | |||||||
MLE | Bias | MSE | LB | UB | AL | CP | |||
50 | 70% | α | 0.4178 | 0.2822 | 0.0797 | 0.1937 | 0.6419 | 0.4482 | 96.2% |
β | 0.8994 | 0.1994 | 0.0477 | 0.6064 | 1.1923 | 0.5859 | 95.9% | ||
γ | 0.6151 | 0.1151 | 0.0239 | 0.3763 | 0.8540 | 0.4776 | 95.0% | ||
80% | α | 0.4193 | 0.2807 | 0.0788 | 0.2255 | 0.6131 | 0.3875 | 95.9% | |
β | 0.8238 | 0.1238 | 0.0218 | 0.5471 | 1.1006 | 0.5535 | 95.9% | ||
γ | 0.5695 | 0.0695 | 0.0182 | 0.3447 | 0.7943 | 0.4496 | 96.7% | ||
100% | α | 0.4211 | 0.2789 | 0.0778 | 0.2480 | 0.5942 | 0.3461 | 96.8% | |
β | 0.7612 | 0.0612 | 0.0104 | 0.5012 | 1.0213 | 0.5201 | 97.0% | ||
γ | 0.5425 | 0.0425 | 0.0163 | 0.3395 | 0.7456 | 0.4061 | 95.0% | ||
100 | 70% | α | 0.4174 | 0.2826 | 0.0798 | 0.2439 | 0.5910 | 0.3470 | 95.0% |
β | 0.8787 | 0.1787 | 0.0353 | 0.6426 | 1.1148 | 0.4722 | 96.3% | ||
γ | 0.5696 | 0.0696 | 0.0201 | 0.3697 | 0.7696 | 0.3998 | 96.0% | ||
80% | α | 0.4191 | 0.2809 | 0.0789 | 0.2690 | 0.5691 | 0.3001 | 95.5% | |
β | 0.7802 | 0.0802 | 0.0134 | 0.5600 | 1.0004 | 0.4404 | 95.7% | ||
γ | 0.5479 | 0.0479 | 0.0091 | 0.3814 | 0.7144 | 0.3330 | 96.0% | ||
100% | α | 0.4206 | 0.2794 | 0.0781 | 0.2866 | 0.5546 | 0.2680 | 95.6% | |
β | 0.7146 | 0.0146 | 0.0068 | 0.5069 | 0.9223 | 0.4154 | 95.7% | ||
γ | 0.5597 | 0.0597 | 0.0074 | 0.4063 | 0.7131 | 0.3067 | 96.0% | ||
150 | 70% | α | 0.4176 | 0.2824 | 0.0798 | 0.2949 | 0.5403 | 0.2454 | 95.8% |
β | 0.8697 | 0.1697 | 0.0305 | 0.7056 | 1.0338 | 0.3282 | 96.2% | ||
γ | 0.5924 | 0.0924 | 0.0174 | 0.4449 | 0.7399 | 0.2950 | 97.1% | ||
80% | α | 0.4193 | 0.2807 | 0.0788 | 0.3131 | 0.5254 | 0.2122 | 96.2% | |
β | 0.8023 | 0.1023 | 0.0124 | 0.6492 | 0.9555 | 0.3063 | 96.1% | ||
γ | 0.5524 | 0.0524 | 0.0058 | 0.4332 | 0.6716 | 0.2384 | 97.0% | ||
100% | α | 0.4209 | 0.2791 | 0.0779 | 0.3261 | 0.5156 | 0.1895 | 95.8% | |
β | 0.7374 | 0.0374 | 0.0032 | 0.5932 | 0.8817 | 0.2885 | 96.3% | ||
γ | 0.5507 | 0.0507 | 0.0041 | 0.4447 | 0.6568 | 0.2121 | 96.9% | ||
200 | 70% | α | 0.4175 | 0.2825 | 0.0798 | 0.3173 | 0.5177 | 0.2004 | 96.1% |
β | 0.8406 | 0.1406 | 0.0265 | 0.7075 | 0.9736 | 0.2661 | 97.2% | ||
γ | 0.5683 | 0.0683 | 0.0069 | 0.4569 | 0.6798 | 0.2230 | 96.9% | ||
80% | α | 0.4193 | 0.2807 | 0.0788 | 0.3327 | 0.5059 | 0.1733 | 96.3% | |
β | 0.8043 | 0.1043 | 0.0123 | 0.6790 | 0.9295 | 0.2505 | 96.6% | ||
γ | 0.5502 | 0.0502 | 0.0041 | 0.4533 | 0.6471 | 0.1938 | 97.0% | ||
100% | α | 0.4209 | 0.2791 | 0.0779 | 0.3435 | 0.4983 | 0.1548 | 96.1% | |
β | 0.7411 | 0.0411 | 0.0030 | 0.6229 | 0.8592 | 0.2362 | 97.0% | ||
γ | 0.5463 | 0.0463 | 0.0041 | 0.4589 | 0.6338 | 0.1749 | 96.2% | ||
n | r | Set4 (α = 0.6, β = 0.3, γ = 0.5) | |||||||
MLE | Bias | MSE | LB | UB | AL | CP | |||
50 | 70% | α | 0.4197 | 0.1803 | 0.0325 | 0.2456 | 0.5939 | 0.3482 | 98.1% |
β | 0.5719 | 0.2719 | 0.0744 | 0.3742 | 0.7696 | 0.3954 | 97.0% | ||
γ | 0.2990 | 0.2010 | 0.0406 | 0.1769 | 0.4211 | 0.2442 | 98.0% | ||
80% | α | 0.4221 | 0.1779 | 0.0317 | 0.2716 | 0.5725 | 0.3009 | 98.4% | |
β | 0.4934 | 0.1934 | 0.0384 | 0.3126 | 0.6742 | 0.3616 | 97.4% | ||
γ | 0.3593 | 0.1407 | 0.0200 | 0.2383 | 0.4803 | 0.2421 | 98.2% | ||
100% | α | 0.4246 | 0.1754 | 0.0308 | 0.2903 | 0.5589 | 0.2686 | 97.9% | |
β | 0.4198 | 0.1198 | 0.0150 | 0.2576 | 0.5820 | 0.3244 | 97.4% | ||
γ | 0.4172 | 0.0828 | 0.0078 | 0.2968 | 0.5376 | 0.2408 | 98.4% | ||
100 | 70% | α | 0.4198 | 0.1802 | 0.0325 | 0.2966 | 0.5429 | 0.2463 | 97.2% |
β | 0.5674 | 0.2674 | 0.0717 | 0.4278 | 0.7069 | 0.2791 | 97.7% | ||
γ | 0.3137 | 0.1863 | 0.0351 | 0.2278 | 0.3995 | 0.1717 | 98.0% | ||
80% | α | 0.4222 | 0.1778 | 0.0316 | 0.3157 | 0.5286 | 0.2128 | 97.9% | |
β | 0.4857 | 0.1857 | 0.0350 | 0.3584 | 0.6130 | 0.2546 | 97.9% | ||
γ | 0.3669 | 0.1331 | 0.0179 | 0.2818 | 0.4519 | 0.1701 | 98.7% | ||
100% | α | 0.4248 | 0.1752 | 0.0307 | 0.3242 | 0.5253 | 0.2011 | 98.1% | |
β | 0.4082 | 0.1082 | 0.0125 | 0.2944 | 0.5221 | 0.2277 | 97.8% | ||
γ | 0.4171 | 0.0829 | 0.0074 | 0.3349 | 0.4993 | 0.1644 | 98.3% | ||
150 | 70% | α | 0.4198 | 0.1802 | 0.0325 | 0.3248 | 0.5148 | 0.1899 | 97.2% |
β | 0.5653 | 0.2653 | 0.0706 | 0.4518 | 0.6788 | 0.2270 | 98.3% | ||
γ | 0.3140 | 0.1860 | 0.0350 | 0.2421 | 0.3860 | 0.1439 | 99.3% | ||
80% | α | 0.4222 | 0.1778 | 0.0316 | 0.3353 | 0.5091 | 0.1738 | 97.7% | |
β | 0.4832 | 0.1832 | 0.0338 | 0.3797 | 0.5866 | 0.2069 | 98.2% | ||
γ | 0.3677 | 0.1323 | 0.0178 | 0.2996 | 0.4358 | 0.1363 | 99.0% | ||
100% | α | 0.4248 | 0.1752 | 0.0307 | 0.3377 | 0.5119 | 0.1742 | 97.3% | |
β | 0.4018 | 0.1018 | 0.0107 | 0.3032 | 0.5004 | 0.1972 | 97.9% | ||
γ | 0.4248 | 0.0752 | 0.0061 | 0.3608 | 0.4888 | 0.1280 | 99.7% | ||
200 | 70% | α | 0.4198 | 0.1802 | 0.0325 | 0.3423 | 0.4973 | 0.1551 | 99.0% |
β | 0.5650 | 0.2650 | 0.0705 | 0.4738 | 0.6561 | 0.1823 | 99.1% | ||
γ | 0.3256 | 0.1744 | 0.0309 | 0.2637 | 0.3875 | 0.1239 | 98.7% | ||
80% | α | 0.4222 | 0.1778 | 0.0316 | 0.3470 | 0.4975 | 0.1505 | 99.6% | |
β | 0.4781 | 0.1781 | 0.0321 | 0.3882 | 0.5679 | 0.1798 | 99.3% | ||
γ | 0.3733 | 0.1267 | 0.0173 | 0.3129 | 0.4336 | 0.1206 | 99.5% | ||
100% | α | 0.4250 | 0.1750 | 0.0306 | 0.3578 | 0.4921 | 0.1343 | 98.7% | |
β | 0.3904 | 0.0904 | 0.0088 | 0.3106 | 0.4703 | 0.1596 | 99.6% | ||
γ | 0.4262 | 0.0738 | 0.0060 | 0.3681 | 0.4843 | 0.1162 | 100% |
Rank | Country | % Global Reserves | Rank | Country | % Global Reserves |
1 | Russia | 19.9 | 23 | Ukraine | 0.6 |
2 | Iran | 17.1 | 24 | Malaysia | 0.5 |
3 | Qatar | 13.1 | 25 | Uzbekistan | 0.4 |
4 | Turkmenistan | 7.2 | 26 | Oman | 0.4 |
5 | United States | 6.7 | 27 | Vietnam | 0.3 |
6 | China | 4.5 | 28 | Israel | 0.3 |
7 | Venezuela | 3.3 | 29 | Argentina | 0.2 |
8 | Saudi Arabia | 3.2 | 30 | Pakistan | 0.2 |
9 | United Arab Emirates | 3.2 | 31 | Trinidad | 0.2 |
10 | Nigeria | 2.9 | 32 | Brazil | 0.2 |
11 | Iraq | 1.9 | 33 | Myanmar | 0.2 |
12 | Canada | 1.3 | 34 | United Kingdom | 0.1 |
13 | Australia | 1.3 | 35 | Thailand | 0.1 |
14 | Azerbaijan | 1.3 | 36 | Mexico | 0.1 |
15 | Algeria | 1.2 | 37 | Bangladesh | 0.1 |
16 | Kazakhstan | 1.2 | 38 | Netherlands | 0.1 |
17 | Egypt | 1.1 | 39 | Bolivia | 0.1 |
18 | Kuwait | 0.9 | 40 | Brunei | 0.1 |
19 | Norway | 0.8 | 41 | Peru | 0.1 |
20 | Libya | 0.8 | 42 | Syria | 0.1 |
21 | Indonesia | 0.7 | 43 | Yemen | 0.1 |
22 | India | 0.7 | 44 | Papua New Guinea | 0.1 |
Rank | Country | reserves2020 | Rank | Country | reserves2020 |
1 | Venezuela | 303.8 | 11 | Nigeria | 36.9 |
2 | Saudi Arabia | 297.5 | 12 | Kazakhstan | 30 |
3 | Canada | 168.1 | 13 | China | 26 |
4 | Iran | 157.8 | 14 | Qatar | 25.2 |
5 | Iraq | 145 | 15 | Algeria | 12.2 |
6 | Russia | 107.8 | 16 | Brazil | 11.9 |
7 | Kuwait | 101.5 | 17 | Norway | 7.9 |
8 | United Arab Emirates | 97.8 | 18 | Angola | 7.8 |
9 | United States | 68.8 | 19 | Azerbaijan | 7 |
10 | Libya | 48.4 | 20 | Mexico | 6.1 |
Rank | Country | Reserves of Gold | Rank | Country | Reserves of Gold | Rank | Country | Reserves of Gold |
1 | USA | 8.1335 | 35 | LBY | 0.1166 | 68 | CYP | 0.0139 |
2 | DEU | 3.3585 | 36 | GRC | 0.1141 | 69 | CUW | 0.0131 |
3 | IMF | 2.814 | 37 | ROK | 0.1045 | 70 | MUS | 0.0124 |
4 | ITA | 2.4518 | 38 | ROU | 0.1036 | 71 | IRL | 0.012 |
5 | FRA | 2.4365 | 39 | BIS | 0.102 | 72 | CZE | 0.0109 |
6 | RUS | 2.2985 | 40 | IRQ | 0.0964 | 73 | KGZ | 0.0102 |
7 | CHN | 1.9483 | 41 | HUN | 0.0945 | 74 | GHA | 0.0087 |
8 | CHE | 1.04 | 42 | AUS | 0.0798 | 75 | PRY | 0.0082 |
9 | JPN | 0.846 | 43 | KWT | 0.079 | 76 | NPL | 0.008 |
10 | IND | 0.7604 | 44 | IDN | 0.0786 | 77 | MNG | 0.0076 |
11 | NLD | 0.6125 | 45 | DNK | 0.0666 | 78 | MMR | 0.0073 |
12 | ECB | 0.5048 | 46 | PAK | 0.0647 | 79 | GTM | 0.0069 |
13 | TUR | 0.4311 | 47 | ARG | 0.0617 | 80 | MKD | 0.0069 |
14 | TAI | 0.4236 | 48 | ARE | 0.0553 | 81 | TUN | 0.0068 |
15 | PRT | 0.3826 | 49 | BLR | 0.0535 | 82 | LVA | 0.0067 |
16 | KAZ | 0.3681 | 50 | QAT | 0.0513 | 83 | LTU | 0.0058 |
17 | UZB | 0.3375 | 51 | KHM | 0.0504 | 84 | COL | 0.0047 |
18 | SAU | 0.3231 | 52 | FIN | 0.049 | 85 | BHR | 0.0047 |
19 | GBR | 0.3103 | 53 | JOR | 0.0435 | 86 | BRN | 0.0046 |
20 | LBN | 0.2868 | 54 | BOL | 0.0425 | 87 | GIN | 0.0042 |
21 | ESP | 0.2816 | 55 | BGR | 0.0408 | 88 | MOZ | 0.0039 |
22 | AUT | 0.28 | 56 | MYS | 0.0389 | 89 | SVN | 0.0032 |
23 | THA | 0.2442 | 57 | SRB | 0.0378 | 90 | ABW | 0.0031 |
24 | POL | 0.2287 | 58 | WAEMU | 0.0365 | 91 | BIH | 0.003 |
25 | BEL | 0.2274 | 59 | PER | 0.0347 | 92 | ALB | 0.0028 |
26 | DZA | 0.1736 | 60 | SVK | 0.0317 | 93 | LUX | 0.0022 |
27 | VEN | 0.1612 | 61 | UKR | 0.0271 | 94 | HKG | 0.0021 |
28 | PHL | 0.1563 | 62 | SYR | 0.0258 | 95 | ISL | 0.002 |
29 | SGP | 0.1537 | 63 | MAR | 0.0221 | 96 | TTO | 0.0019 |
30 | BRA | 0.1297 | 64 | ECU | 0.0219 | 97 | HTI | 0.0018 |
31 | SWE | 0.1257 | 65 | AFG | 0.0219 | 98 | YEM | 0.0016 |
32 | ZAF | 0.1254 | 66 | NGA | 0.0215 | 99 | SUR | 0.0015 |
33 | EGY | 0.125 | 67 | BGD | 0.014 | 100 | SLV | 0.0014 |
34 | MEX | 0.1199 |
n | Mean | Median | Skewness | Kurtosis | Range | Min | Max | Sum | |
Data1 | 20 | 1.900 | 1.700 | 1.860 | 4.185 | 3.000 | 1.100 | 4.100 | 38.000 |
Data2 | 44 | 2.248 | 0.650 | 2.990 | 8.864 | 19.800 | 0.100 | 19.900 | 98.900 |
Data3 | 20 | 83.375 | 42.650 | 1.430 | 1.420 | 297.700 | 6.100 | 303.800 | 1667.500 |
Data4 | 100 | 0.347 | 0.050 | 5.590 | 38.257 | 8.130 | 0.001 | 8.133 | 34.676 |
Distributions | MLE and SE | ||||
α | β | γ | λ | θ | |
LBTLoW | 8.648 | 3.074 | 0.042 | ||
(3.545) | (0.474) | (0.025) | |||
ETGR | 0.103 | 0.692 | 23.539 | -0.342 | |
(0.436) | (0.086) | (105.137) | (1.971) | ||
BW | 0.831 | 0.613 | 29.947 | 11.632 | |
(0.954) | (0.340) | (40.414) | (21.900) | ||
T-Li | 0.665 | 0.359 | |||
(0.332) | (0.048) | ||||
McLL | 0.881 | 2.070 | 1.926 | 19.225 | 32.033 |
(0.109) | (3.693) | (5.165) | (22.341) | (43.081) | |
NMW | 0.121 | 2.784 | 2.787 | 0.003 | 0.008 |
(0.056) | (20.370) | (0.428) | (0.025) | (0.002) | |
W | 0.122 | 2.787 | |||
(0.056) | (0.427) |
Distributions | AIC | CAIC | BIC | HQIC | KS | PV |
LBTLoW | 40.140 | 41.640 | 38.040 | 40.720 | 0.146 | 0.790 |
ETGR | 44.860 | 47.520 | 42.060 | 45.630 | 0.190 | 0.465 |
BW | 42.400 | 45.060 | 39.600 | 43.170 | 0.160 | 0.683 |
T-Li | 65.730 | 66.440 | 64.330 | 66.120 | 0.380 | 0.006 |
McLL | 43.850 | 48.140 | 40.360 | 44.830 | 0.147 | 0.734 |
NMW | 51.170 | 55.460 | 47.680 | 52.150 | 0.190 | 0.501 |
W | 45.170 | 45.880 | 43.780 | 45.560 | 0.180 | 0.509 |
Distributions | MLE and SE | ||||
α | β | γ | λ | θ | |
LBTLoW | 6.268 | 0.623 | 0.484 | ||
(2.631) | (0.066) | (0.210) | |||
ETGR | 0.055 | 0.071 | 8.773 | 0.947 | |
(0.027) | (0.029) | (7.043) | (0.081) | ||
TCWG | 34.076 | 0.802 | 0.005 | 1.12 | |
(81.023) | (0.021) | (0.013) | (0.285) | ||
EKW | 0.221 | 400.298 | 5.215 | 1 | 3.823 |
(0.038) | (718.99) | (0.649) | (0.004) | (3.036) | |
TMW | 0.851 | 1.159 | -0.554 | 0.519 | |
(0.163) | (1.026) | (0.985) | (0.379) | ||
BW | 2.861 | 0.075 | 78.550 | 42.576 | |
(69.095) | (0.090) | (167.320) | (187.300) | ||
T-Li | 0.604 | 0.671 | |||
(0.155) | (0.074) | ||||
McLL | 0.181 | 1.565 | 1.286 | 21.234 | 28.124 |
(0.193) | (9.254) | (5.432) | (34.701) | (45.757) | |
NMW | 6.8 x 10−8 | 0.680 | 0.223 | 0.015 | 0.806 |
(0.623) | (0.110) | (617.48) | (0.015) | (0.418) | |
W | 0.799 | 0.621 | |||
(0.136) | (0.068) |
Distributions | AIC | CAIC | BIC | HQIC | KS | PV |
LBTLoW | 132.210 | 132.810 | 131.140 | 134.200 | 0.130 | 0.425 |
ETGR | 143.470 | 144.490 | 142.040 | 146.110 | 0.180 | 0.118 |
TCWG | 137.690 | 138.710 | 136.260 | 140.330 | 0.150 | 0.251 |
EKW | 133.890 | 135.470 | 132.110 | 140.330 | 0.140 | 0.355 |
TMW | 140.900 | 142.480 | 139.120 | 144.210 | 0.150 | 0.276 |
BW | 133.180 | 134.200 | 131.750 | 135.820 | 0.130 | 0.408 |
T-Li | 174.360 | 174.660 | 173.650 | 175.690 | 0.200 | 0.057 |
McLL | 134.830 | 136.410 | 133.040 | 138.130 | 0.130 | 0.419 |
NMW | 143.780 | 145.360 | 142.000 | 147.090 | 0.160 | 0.243 |
W | 138.650 | 138.940 | 137.940 | 139.970 | 0.170 | 0.139 |
Distributions | MLE and SE | ||||
α | β | γ | λ | θ | |
LBTLoW | 2.515 | 0.756 | 0.040 | ||
(3.877) | (0.166) | (0.048) | |||
WEIW | 0.909 | 0.871 | 7.225 | ||
(106700) | (0.152) | (384700) | |||
TMW | 0.998 | 0.459 | -0.443 | 0.202 | |
(0.081) | (18.537) | (18.537) | (0.769) | ||
T-Li | 0.021 | 0.384 | |||
(0.345) | (0.004) | ||||
McLL | 0.208 | 93.978 | 1.279 | 24.759 | 32.815 |
(0.499) | (1721) | (19.272) | (142.806) | (161.611) | |
NMW | 10.7 x 10−8 | 0.930 | 0.859 | 7.46 x 10−8 | 0.017 |
(0.001) | (0.250) | (1.216) | (0.002) | (0.017) | |
EKW | 0.167 | 261.64 | 45.725 | 1.201 | 2.138 |
(0.079) | (1709) | (219.725) | (0.741) | (7.209) |
Distributions | AIC | CAIC | BIC | HQIC | KS | PV |
LBTLoW | 221.690 | 223.190 | 219.600 | 222.280 | 0.135 | 0.857 |
WEIW | 223.400 | 224.900 | 221.300 | 223.980 | 0.157 | 0.708 |
TMW | 226.410 | 230.690 | 222.910 | 227.380 | 0.153 | 0.734 |
T-Li | 230.480 | 231.180 | 229.080 | 230.870 | 0.265 | 0.120 |
McLL | 225.990 | 230.280 | 222.500 | 222.500 | 0.146 | 0.789 |
NMW | 226.570 | 230.860 | 223.080 | 227.540 | 0.140 | 0.826 |
EKW | 226.290 | 230.570 | 222.790 | 229.300 | 0.148 | 0.776 |
Distributions | MLE and SE | ||||
α | β | γ | λ | θ | |
LBTLoW | 6.498 | 0.482 | 1.490 | ||
(2.301) | (0.034) | (0.573) | |||
EKW | 0.221 | 1096 | 4.424 | 1 | 1.717 |
(0.030) | (1376) | (1.817) | (0.001) | (0.901) | |
TMW | 0.596 | 2.612 | 0.588 | -0.523 | |
(0.057) | (0.689) | (0.256) | (0.346) | ||
BW | 134.832 | 0.073 | 49.149 | 22.930 | |
(956.622) | (0.060) | (74.497) | (46.500) | ||
WEIW | 27.512 | 0.549 | 0.094 | ||
(3272000) | (0.042) | (856.967) | |||
W | 2.648 | 0.489 | |||
(0.281) | (0.035) |
Distributions | AIC | CAIC | BIC | HQIC | KS | PV |
LBTLoW | –170.510 | –170.260 | –170.510 | –167.350 | 0.070 | 0.704 |
ETGR | –167.710 | –167.070 | –167.710 | –157.710 | 0.078 | 0.584 |
BW | –158.180 | –157.540 | –158.180 | –152.910 | 0.077 | 0.593 |
T-Li | –170.220 | –169.800 | –170.220 | –166.010 | 0.071 | 0.703 |
McLL | –170.200 | –169.950 | –170.200 | –167.040 | 0.091 | 0.383 |
W | –157.390 | –157.260 | –157.390 | –155.280 | 0.100 | 0.269 |
γ | α | μ′1 | μ′2 | μ′3 | μ′4 | σ2 | CS | CK | CV |
0.4 | 0.2 | 2.629 | 8.323 | 30.531 | 126.387 | 1.413 | 0.729 | 3.596 | 0.452 |
0.6 | 0.5 | 1.558 | 2.937 | 6.430 | 15.922 | 0.508 | 0.746 | 3.627 | 0.457 |
0.8 | 0.8 | 1.184 | 1.700 | 2.847 | 5.403 | 0.300 | 0.765 | 3.660 | 0.463 |
1.1 | 1.2 | 0.925 | 1.044 | 1.380 | 2.074 | 0.189 | 0.793 | 3.717 | 0.470 |
1.3 | 1.5 | 0.805 | 0.794 | 0.920 | 1.216 | 0.146 | 0.814 | 3.758 | 0.475 |
1.7 | 1.8 | 0.705 | 0.615 | 0.634 | 0.748 | 0.117 | 0.856 | 3.853 | 0.486 |
1.9 | 2.0 | 0.655 | 0.532 | 0.513 | 0.568 | 0.103 | 0.878 | 3.906 | 0.491 |
2.4 | 2.3 | 0.582 | 0.425 | 0.371 | 0.374 | 0.086 | 0.937 | 4.058 | 0.503 |
2.7 | 2.6 | 0.530 | 0.355 | 0.285 | 0.266 | 0.073 | 0.974 | 4.162 | 0.511 |
3.2 | 3.0 | 0.469 | 0.280 | 0.203 | 0.172 | 0.060 | 1.038 | 4.359 | 0.522 |
γ | α | μ′1 | μ′2 | μ′3 | μ′4 | σ2 | CS | CK | CV |
0.4 | 0.2 | 1.964 | 4.277 | 10.154 | 25.945 | 0.420 | 0.380 | 3.017 | 0.330 |
0.6 | 0.5 | 1.347 | 2.017 | 3.298 | 5.813 | 0.202 | 0.395 | 3.026 | 0.334 |
0.8 | 0.8 | 1.105 | 1.360 | 1.829 | 2.658 | 0.139 | 0.411 | 3.037 | 0.337 |
1.1 | 1.2 | 0.924 | 0.954 | 1.081 | 1.325 | 0.100 | 0.435 | 3.058 | 0.343 |
1.3 | 1.5 | 0.836 | 0.783 | 0.805 | 0.897 | 0.084 | 0.453 | 3.075 | 0.346 |
1.7 | 1.8 | 0.760 | 0.649 | 0.612 | 0.627 | 0.072 | 0.489 | 3.116 | 0.353 |
1.9 | 2.0 | 0.720 | 0.584 | 0.524 | 0.511 | 0.066 | 0.508 | 3.140 | 0.356 |
2.4 | 2.3 | 0.661 | 0.494 | 0.411 | 0.374 | 0.058 | 0.557 | 3.213 | 0.365 |
2.7 | 2.6 | 0.617 | 0.433 | 0.339 | 0.290 | 0.052 | 0.588 | 3.265 | 0.369 |
3.2 | 3.0 | 0.565 | 0.364 | 0.263 | 0.209 | 0.045 | 0.641 | 3.366 | 0.377 |
β | α | μ′1 | μ′2 | μ′3 | μ′4 | σ2 | CS | CK | CV |
1.5 | 0.2 | 0.029 | 0.026 | 0.023 | 0.021 | 0.025 | 5.273 | 29.151 | 5.386 |
0.5 | 0.032 | 0.028 | 0.025 | 0.023 | 0.027 | 5.014 | 26.463 | 5.138 | |
0.8 | 0.035 | 0.031 | 0.028 | 0.025 | 0.030 | 4.772 | 24.069 | 4.906 | |
1.2 | 0.039 | 0.035 | 0.031 | 0.028 | 0.033 | 4.472 | 21.275 | 4.622 | |
1.5 | 0.043 | 0.038 | 0.033 | 0.03 | 0.036 | 4.264 | 19.441 | 4.424 | |
1.8 | 0.046 | 0.041 | 0.036 | 0.033 | 0.038 | 4.069 | 17.801 | 4.241 | |
2 | 0.049 | 0.043 | 0.038 | 0.034 | 0.040 | 3.946 | 16.806 | 4.125 | |
2.3 | 0.053 | 0.046 | 0.041 | 0.037 | 0.043 | 3.771 | 15.445 | 3.961 | |
2.6 | 0.056 | 0.049 | 0.044 | 0.039 | 0.046 | 3.607 | 14.225 | 3.809 | |
3 | 0.062 | 0.054 | 0.048 | 0.043 | 0.050 | 3.405 | 12.794 | 3.621 | |
2.5 | 0.2 | 0.006 | 0.005 | 0.005 | 0.004 | 0.005 | 12.420 | 156.328 | 12.403 |
0.5 | 0.007 | 0.006 | 0.006 | 0.005 | 0.006 | 11.672 | 138.195 | 11.665 | |
0.8 | 0.007 | 0.007 | 0.006 | 0.006 | 0.007 | 10.981 | 122.434 | 10.984 | |
1.2 | 0.009 | 0.008 | 0.007 | 0.007 | 0.008 | 10.140 | 104.548 | 10.156 | |
1.5 | 0.01 | 0.009 | 0.008 | 0.007 | 0.009 | 9.563 | 93.121 | 9.589 | |
1.8 | 0.011 | 0.010 | 0.009 | 0.008 | 0.010 | 9.030 | 83.140 | 9.066 | |
2 | 0.012 | 0.011 | 0.010 | 0.009 | 0.010 | 8.697 | 77.190 | 8.739 | |
2.3 | 0.013 | 0.012 | 0.011 | 0.010 | 0.012 | 8.227 | 69.194 | 8.279 | |
2.6 | 0.014 | 0.013 | 0.012 | 0.011 | 0.013 | 7.792 | 62.179 | 7.853 | |
3 | 0.017 | 0.015 | 0.014 | 0.013 | 0.015 | 7.261 | 54.128 | 7.334 |
ε | β | α | γ | RE | HaCE | ArE | TsE |
1.5 | 0.25 | 0.2 | 0.4 | 3.331 | 3.340 | 2.767 | 1.957 |
0.5 | 0.6 | 3.252 | 3.333 | 2.753 | 1.953 | ||
0.8 | 0.8 | 3.099 | 3.318 | 2.722 | 1.944 | ||
1.2 | 1.1 | 2.875 | 3.290 | 2.670 | 1.927 | ||
1.5 | 1.3 | 2.716 | 3.264 | 2.627 | 1.912 | ||
1.8 | 1.7 | 2.524 | 3.227 | 2.568 | 1.891 | ||
2.0 | 1.9 | 2.415 | 3.203 | 2.53 | 1.876 | ||
2.3 | 2.4 | 2.229 | 3.152 | 2.458 | 1.846 | ||
2.6 | 2.7 | 2.084 | 3.104 | 2.394 | 1.818 | ||
3.0 | 3.2 | 1.889 | 3.026 | 2.296 | 1.773 | ||
0.5 | 0.2 | 0.4 | 1.930 | 3.044 | 2.318 | 1.783 | |
0.5 | 0.6 | 1.924 | 3.042 | 2.315 | 1.782 | ||
0.8 | 0.8 | 1.824 | 2.996 | 2.260 | 1.755 | ||
1.2 | 1.1 | 1.660 | 2.909 | 2.161 | 1.704 | ||
1.5 | 1.3 | 1.541 | 2.835 | 2.081 | 1.661 | ||
1.8 | 1.7 | 1.392 | 2.726 | 1.969 | 1.597 | ||
2.0 | 1.9 | 1.308 | 2.657 | 1.901 | 1.556 | ||
2.3 | 2.4 | 1.161 | 2.517 | 1.770 | 1.475 | ||
2.6 | 2.7 | 1.051 | 2.397 | 1.661 | 1.404 | ||
3.0 | 3.2 | 0.903 | 2.207 | 1.500 | 1.293 | ||
2.0 | 0.25 | 0.2 | 0.4 | 2.180 | 1.987 | 1.837 | 0.993 |
0.5 | 0.6 | 2.167 | 1.986 | 1.835 | 0.993 | ||
0.8 | 0.8 | 2.053 | 1.982 | 1.812 | 0.991 | ||
1.2 | 1.1 | 1.876 | 1.973 | 1.769 | 0.987 | ||
1.5 | 1.3 | 1.753 | 1.965 | 1.734 | 0.982 | ||
1.8 | 1.7 | 1.595 | 1.949 | 1.681 | 0.975 | ||
2.0 | 1.9 | 1.510 | 1.938 | 1.648 | 0.969 | ||
2.3 | 2.4 | 1.358 | 1.912 | 1.581 | 0.956 | ||
2.6 | 2.7 | 1.247 | 1.887 | 1.524 | 0.943 | ||
3.0 | 3.2 | 1.098 | 1.840 | 1.435 | 0.920 | ||
0.5 | 0.2 | 0.4 | 1.210 | 1.877 | 1.503 | 0.938 | |
0.5 | 0.6 | 1.279 | 1.895 | 1.541 | 0.947 | ||
0.8 | 0.8 | 1.210 | 1.877 | 1.503 | 0.938 | ||
1.2 | 1.1 | 1.080 | 1.834 | 1.423 | 0.917 | ||
1.5 | 1.3 | 0.987 | 1.794 | 1.358 | 0.897 | ||
1.8 | 1.7 | 0.861 | 1.725 | 1.258 | 0.862 | ||
2.0 | 1.9 | 0.795 | 1.679 | 1.199 | 0.840 | ||
2.3 | 2.4 | 0.672 | 1.574 | 1.077 | 0.787 | ||
2.6 | 2.7 | 0.587 | 1.482 | 0.982 | 0.741 | ||
3.0 | 3.2 | 0.470 | 1.323 | 0.836 | 0.661 |
ε | β | α | RE | HaCE | ArE | TsE |
1.5 | 0.25 | 0.2 | 7.001 | 3.311 | 2.709 | 1.94 |
0.5 | 7.075 | 3.315 | 2.716 | 1.942 | ||
0.8 | 7.156 | 3.319 | 2.724 | 1.944 | ||
1.2 | 7.274 | 3.324 | 2.735 | 1.947 | ||
1.5 | 7.372 | 3.329 | 2.743 | 1.95 | ||
1.8 | 7.476 | 3.333 | 2.752 | 1.952 | ||
2 | 7.55 | 3.336 | 2.758 | 1.954 | ||
2.3 | 7.667 | 3.34 | 2.767 | 1.957 | ||
2.6 | 7.792 | 3.345 | 2.777 | 1.959 | ||
3 | 7.971 | 3.351 | 2.79 | 1.963 | ||
0.4 | 0.2 | 6.441 | 3.278 | 2.65 | 1.92 | |
0.5 | 6.452 | 3.279 | 2.651 | 1.921 | ||
0.8 | 6.469 | 3.28 | 2.653 | 1.921 | ||
1.2 | 6.503 | 3.282 | 2.657 | 1.923 | ||
1.5 | 6.536 | 3.284 | 2.66 | 1.924 | ||
1.8 | 6.577 | 3.287 | 2.665 | 1.925 | ||
2 | 6.608 | 3.289 | 2.668 | 1.927 | ||
2.3 | 6.661 | 3.292 | 2.674 | 1.928 | ||
2.6 | 6.721 | 3.296 | 2.681 | 1.931 | ||
3 | 6.813 | 3.301 | 2.69 | 1.934 | ||
2.0 | 0.25 | 0.2 | 4.376 | 1.975 | 1.776 | 0.987 |
0.5 | 4.429 | 1.976 | 1.782 | 0.988 | ||
0.8 | 4.487 | 1.977 | 1.788 | 0.989 | ||
1.2 | 4.57 | 1.979 | 1.796 | 0.99 | ||
1.5 | 4.639 | 1.981 | 1.803 | 0.99 | ||
1.8 | 4.713 | 1.982 | 1.81 | 0.991 | ||
2 | 4.765 | 1.983 | 1.815 | 0.991 | ||
2.3 | 4.847 | 1.984 | 1.823 | 0.992 | ||
2.6 | 4.934 | 1.986 | 1.83 | 0.993 | ||
3 | 5.058 | 1.987 | 1.841 | 0.994 | ||
0.4 | 0.2 | 3.975 | 1.962 | 1.726 | 0.981 | |
0.5 | 3.987 | 1.963 | 1.728 | 0.981 | ||
0.8 | 4.003 | 1.963 | 1.73 | 0.982 | ||
1.2 | 4.031 | 1.965 | 1.734 | 0.982 | ||
1.5 | 4.058 | 1.965 | 1.737 | 0.983 | ||
1.8 | 4.09 | 1.967 | 1.741 | 0.983 | ||
2 | 4.114 | 1.967 | 1.744 | 0.984 | ||
2.3 | 4.154 | 1.969 | 1.749 | 0.984 | ||
2.6 | 4.199 | 1.97 | 1.755 | 0.985 | ||
3 | 4.267 | 1.972 | 1.763 | 0.986 |
n | r | Set1 (α = 0.5, β = 0.5, γ = 0.5) | |||||||
MLE | Bias | MSE | LB | UB | AL | CP | |||
50 | 70% | α | 0.4204 | 0.0796 | 0.0064 | 0.0019 | 0.839 | 0.8370 | 97.4% |
β | 0.7041 | 0.2041 | 0.0471 | 0.5036 | 0.9046 | 0.4010 | 96.9% | ||
γ | 0.4201 | 0.0799 | 0.0069 | 0.3023 | 0.5379 | 0.2356 | 96.0% | ||
80% | α | 0.4218 | 0.0782 | 0.0061 | 0.0191 | 0.8245 | 0.8053 | 94.8% | |
β | 0.6382 | 0.1382 | 0.0242 | 0.4508 | 0.8256 | 0.3748 | 95.8% | ||
γ | 0.4386 | 0.0614 | 0.0053 | 0.3282 | 0.5490 | 0.2208 | 97.1% | ||
100% | α | 0.4234 | 0.0766 | 0.0059 | 0.0357 | 0.8111 | 0.7754 | 95.4% | |
β | 0.5177 | 0.0177 | 0.0056 | 0.3661 | 0.6694 | 0.3033 | 95.5% | ||
γ | 0.5316 | 0.0316 | 0.0027 | 0.4303 | 0.6328 | 0.2025 | 96.0% | ||
100 | 70% | α | 0.4213 | 0.0787 | 0.0062 | 0.0844 | 0.7583 | 0.6740 | 96.2% |
β | 0.6750 | 0.1750 | 0.0312 | 0.5375 | 0.8125 | 0.2750 | 95.9% | ||
γ | 0.4237 | 0.0763 | 0.0065 | 0.3389 | 0.5084 | 0.1694 | 96.0% | ||
80% | α | 0.4230 | 0.0770 | 0.0061 | 0.2099 | 0.6360 | 0.4262 | 96.2% | |
β | 0.6099 | 0.1099 | 0.0127 | 0.4819 | 0.7379 | 0.2560 | 96.1% | ||
γ | 0.4487 | 0.0513 | 0.0033 | 0.3652 | 0.5321 | 0.1669 | 97.3% | ||
100% | α | 0.4238 | 0.0762 | 0.0058 | 0.2501 | 0.5975 | 0.3473 | 95.6% | |
β | 0.4683 | 0.0317 | 0.0027 | 0.3558 | 0.5807 | 0.2249 | 95.8% | ||
γ | 0.4967 | 0.0033 | 0.0025 | 0.4199 | 0.5734 | 0.1535 | 96.0% | ||
150 | 70% | α | 0.4217 | 0.0783 | 0.0061 | 0.2710 | 0.5725 | 0.3015 | 95.2% |
β | 0.6626 | 0.1626 | 0.0281 | 0.5577 | 0.7675 | 0.2097 | 95.6% | ||
γ | 0.4277 | 0.0723 | 0.0058 | 0.3571 | 0.4983 | 0.1412 | 97.3% | ||
80% | α | 0.4236 | 0.0764 | 0.0059 | 0.3005 | 0.5466 | 0.2461 | 95.7% | |
β | 0.5977 | 0.0977 | 0.0113 | 0.4957 | 0.6997 | 0.2040 | 96.2% | ||
γ | 0.4649 | 0.0351 | 0.0022 | 0.3972 | 0.5325 | 0.1353 | 97.0% | ||
100% | α | 0.4238 | 0.0762 | 0.0058 | 0.3010 | 0.5467 | 0.2457 | 95.6% | |
β | 0.4766 | 0.0234 | 0.0023 | 0.3784 | 0.5749 | 0.1965 | 96.4% | ||
γ | 0.5277 | 0.0277 | 0.0015 | 0.4659 | 0.5894 | 0.1236 | 96.9% | ||
200 | 70% | α | 0.4219 | 0.0781 | 0.0061 | 0.3154 | 0.5285 | 0.2132 | 96.1% |
β | 0.6592 | 0.1592 | 0.0268 | 0.5675 | 0.7510 | 0.1835 | 96.3% | ||
γ | 0.4375 | 0.0625 | 0.0046 | 0.3789 | 0.4962 | 0.1173 | 96.7% | ||
80% | α | 0.4239 | 0.0761 | 0.0058 | 0.3236 | 0.5242 | 0.2006 | 96.3% | |
β | 0.5912 | 0.0912 | 0.0099 | 0.5074 | 0.6750 | 0.1676 | 97.0% | ||
γ | 0.4667 | 0.0333 | 0.0020 | 0.4101 | 0.5233 | 0.1132 | 97.5% | ||
100% | α | 0.4240 | 0.0760 | 0.0058 | 0.3372 | 0.5109 | 0.1737 | 96.5% | |
β | 0.4905 | 0.0095 | 0.0009 | 0.4167 | 0.5642 | 0.1475 | 96.7% | ||
γ | 0.5035 | 0.0035 | 0.0006 | 0.4524 | 0.5546 | 0.1022 | 97.1% | ||
n | r | Set2 (α = 0.7, β = 0.5, γ = 0.25) | |||||||
MLE | Bias | MSE | LB | UB | AL | CP | |||
50 | 70% | α | 0.4206 | 0.2794 | 0.0782 | 0.0055 | 0.8358 | 0.8304 | 97.7% |
β | 0.7016 | 0.2016 | 0.0462 | 0.5015 | 0.9016 | 0.4001 | 96.5% | ||
γ | 0.2172 | 0.0328 | 0.0017 | 0.1568 | 0.2776 | 0.1208 | 100% | ||
80% | α | 0.4214 | 0.2786 | 0.0776 | 0.2084 | 0.6345 | 0.4261 | 97.9% | |
β | 0.6361 | 0.1361 | 0.0243 | 0.4490 | 0.8231 | 0.3741 | 98.5% | ||
γ | 0.2297 | 0.0203 | 0.0010 | 0.1719 | 0.2874 | 0.1155 | 100% | ||
100% | α | 0.4234 | 0.2766 | 0.0765 | 0.2497 | 0.5970 | 0.3473 | 98.3% | |
β | 0.5161 | 0.0161 | 0.0062 | 0.3649 | 0.6673 | 0.3025 | 97.6% | ||
γ | 0.2565 | 0.0065 | 0.0007 | 0.2044 | 0.3086 | 0.1043 | 100% | ||
100 | 70% | α | 0.4210 | 0.2790 | 0.0779 | 0.0208 | 0.8212 | 0.8004 | 96.4% |
β | 0.7006 | 0.2006 | 0.0431 | 0.5593 | 0.8419 | 0.2826 | 98.0% | ||
γ | 0.2141 | 0.0359 | 0.0016 | 0.1720 | 0.2562 | 0.0842 | 100% | ||
80% | α | 0.4215 | 0.2785 | 0.0776 | 0.2708 | 0.5721 | 0.3013 | 97.2% | |
β | 0.6357 | 0.1357 | 0.0214 | 0.5035 | 0.7680 | 0.2645 | 97.7% | ||
γ | 0.2270 | 0.0230 | 0.0008 | 0.1866 | 0.2673 | 0.0807 | 100% | ||
100% | α | 0.4234 | 0.2766 | 0.0765 | 0.3006 | 0.5462 | 0.2456 | 97.3% | |
β | 0.5158 | 0.0158 | 0.0033 | 0.4088 | 0.6227 | 0.2140 | 98.2% | ||
γ | 0.2540 | 0.0040 | 0.0003 | 0.2176 | 0.2905 | 0.0729 | 100% | ||
150 | 70% | α | 0.4212 | 0.2788 | 0.0778 | 0.0330 | 0.8093 | 0.7763 | 97.7% |
β | 0.7000 | 0.2000 | 0.0419 | 0.5847 | 0.8153 | 0.2306 | 97.7% | ||
γ | 0.2122 | 0.0378 | 0.0016 | 0.1781 | 0.2463 | 0.0682 | 100% | ||
80% | α | 0.4215 | 0.2785 | 0.0776 | 0.2985 | 0.5445 | 0.2460 | 98.8% | |
β | 0.6350 | 0.1350 | 0.0203 | 0.5271 | 0.7430 | 0.2159 | 98.1% | ||
γ | 0.2259 | 0.0241 | 0.0008 | 0.1931 | 0.2587 | 0.0656 | 96.0% | ||
100% | α | 0.4234 | 0.2766 | 0.0765 | 0.3232 | 0.5237 | 0.2005 | 97.2% | |
β | 0.5151 | 0.0151 | 0.0023 | 0.4278 | 0.6024 | 0.1746 | 97.0% | ||
γ | 0.2529 | 0.0029 | 0.0002 | 0.2232 | 0.2825 | 0.0593 | 95.4% | ||
200 | 70% | α | 0.4209 | 0.2791 | 0.0779 | 0.0849 | 0.7569 | 0.6720 | 100% |
β | 0.6981 | 0.1981 | 0.0405 | 0.5984 | 0.7978 | 0.1994 | 97.2% | ||
γ | 0.2118 | 0.0382 | 0.0016 | 0.1823 | 0.2412 | 0.0589 | 97.3% | ||
80% | α | 0.4215 | 0.2785 | 0.0776 | 0.3150 | 0.5280 | 0.2131 | 100% | |
β | 0.6331 | 0.1331 | 0.0191 | 0.5398 | 0.7265 | 0.1867 | 98.2% | ||
γ | 0.2256 | 0.0244 | 0.0007 | 0.1973 | 0.2540 | 0.0567 | 98.0% | ||
100% | α | 0.4234 | 0.2766 | 0.0765 | 0.3366 | 0.5103 | 0.1737 | 100% | |
β | 0.5136 | 0.0136 | 0.0016 | 0.4381 | 0.5891 | 0.1510 | 98.8% | ||
γ | 0.2523 | 0.0023 | 0.0002 | 0.2267 | 0.2779 | 0.0512 | 100% | ||
n | r | Set3 (α = 0.7, β = 0.7, γ = 0.5) | |||||||
MLE | Bias | MSE | LB | UB | AL | CP | |||
50 | 70% | α | 0.4178 | 0.2822 | 0.0797 | 0.1937 | 0.6419 | 0.4482 | 96.2% |
β | 0.8994 | 0.1994 | 0.0477 | 0.6064 | 1.1923 | 0.5859 | 95.9% | ||
γ | 0.6151 | 0.1151 | 0.0239 | 0.3763 | 0.8540 | 0.4776 | 95.0% | ||
80% | α | 0.4193 | 0.2807 | 0.0788 | 0.2255 | 0.6131 | 0.3875 | 95.9% | |
β | 0.8238 | 0.1238 | 0.0218 | 0.5471 | 1.1006 | 0.5535 | 95.9% | ||
γ | 0.5695 | 0.0695 | 0.0182 | 0.3447 | 0.7943 | 0.4496 | 96.7% | ||
100% | α | 0.4211 | 0.2789 | 0.0778 | 0.2480 | 0.5942 | 0.3461 | 96.8% | |
β | 0.7612 | 0.0612 | 0.0104 | 0.5012 | 1.0213 | 0.5201 | 97.0% | ||
γ | 0.5425 | 0.0425 | 0.0163 | 0.3395 | 0.7456 | 0.4061 | 95.0% | ||
100 | 70% | α | 0.4174 | 0.2826 | 0.0798 | 0.2439 | 0.5910 | 0.3470 | 95.0% |
β | 0.8787 | 0.1787 | 0.0353 | 0.6426 | 1.1148 | 0.4722 | 96.3% | ||
γ | 0.5696 | 0.0696 | 0.0201 | 0.3697 | 0.7696 | 0.3998 | 96.0% | ||
80% | α | 0.4191 | 0.2809 | 0.0789 | 0.2690 | 0.5691 | 0.3001 | 95.5% | |
β | 0.7802 | 0.0802 | 0.0134 | 0.5600 | 1.0004 | 0.4404 | 95.7% | ||
γ | 0.5479 | 0.0479 | 0.0091 | 0.3814 | 0.7144 | 0.3330 | 96.0% | ||
100% | α | 0.4206 | 0.2794 | 0.0781 | 0.2866 | 0.5546 | 0.2680 | 95.6% | |
β | 0.7146 | 0.0146 | 0.0068 | 0.5069 | 0.9223 | 0.4154 | 95.7% | ||
γ | 0.5597 | 0.0597 | 0.0074 | 0.4063 | 0.7131 | 0.3067 | 96.0% | ||
150 | 70% | α | 0.4176 | 0.2824 | 0.0798 | 0.2949 | 0.5403 | 0.2454 | 95.8% |
β | 0.8697 | 0.1697 | 0.0305 | 0.7056 | 1.0338 | 0.3282 | 96.2% | ||
γ | 0.5924 | 0.0924 | 0.0174 | 0.4449 | 0.7399 | 0.2950 | 97.1% | ||
80% | α | 0.4193 | 0.2807 | 0.0788 | 0.3131 | 0.5254 | 0.2122 | 96.2% | |
β | 0.8023 | 0.1023 | 0.0124 | 0.6492 | 0.9555 | 0.3063 | 96.1% | ||
γ | 0.5524 | 0.0524 | 0.0058 | 0.4332 | 0.6716 | 0.2384 | 97.0% | ||
100% | α | 0.4209 | 0.2791 | 0.0779 | 0.3261 | 0.5156 | 0.1895 | 95.8% | |
β | 0.7374 | 0.0374 | 0.0032 | 0.5932 | 0.8817 | 0.2885 | 96.3% | ||
γ | 0.5507 | 0.0507 | 0.0041 | 0.4447 | 0.6568 | 0.2121 | 96.9% | ||
200 | 70% | α | 0.4175 | 0.2825 | 0.0798 | 0.3173 | 0.5177 | 0.2004 | 96.1% |
β | 0.8406 | 0.1406 | 0.0265 | 0.7075 | 0.9736 | 0.2661 | 97.2% | ||
γ | 0.5683 | 0.0683 | 0.0069 | 0.4569 | 0.6798 | 0.2230 | 96.9% | ||
80% | α | 0.4193 | 0.2807 | 0.0788 | 0.3327 | 0.5059 | 0.1733 | 96.3% | |
β | 0.8043 | 0.1043 | 0.0123 | 0.6790 | 0.9295 | 0.2505 | 96.6% | ||
γ | 0.5502 | 0.0502 | 0.0041 | 0.4533 | 0.6471 | 0.1938 | 97.0% | ||
100% | α | 0.4209 | 0.2791 | 0.0779 | 0.3435 | 0.4983 | 0.1548 | 96.1% | |
β | 0.7411 | 0.0411 | 0.0030 | 0.6229 | 0.8592 | 0.2362 | 97.0% | ||
γ | 0.5463 | 0.0463 | 0.0041 | 0.4589 | 0.6338 | 0.1749 | 96.2% | ||
n | r | Set4 (α = 0.6, β = 0.3, γ = 0.5) | |||||||
MLE | Bias | MSE | LB | UB | AL | CP | |||
50 | 70% | α | 0.4197 | 0.1803 | 0.0325 | 0.2456 | 0.5939 | 0.3482 | 98.1% |
β | 0.5719 | 0.2719 | 0.0744 | 0.3742 | 0.7696 | 0.3954 | 97.0% | ||
γ | 0.2990 | 0.2010 | 0.0406 | 0.1769 | 0.4211 | 0.2442 | 98.0% | ||
80% | α | 0.4221 | 0.1779 | 0.0317 | 0.2716 | 0.5725 | 0.3009 | 98.4% | |
β | 0.4934 | 0.1934 | 0.0384 | 0.3126 | 0.6742 | 0.3616 | 97.4% | ||
γ | 0.3593 | 0.1407 | 0.0200 | 0.2383 | 0.4803 | 0.2421 | 98.2% | ||
100% | α | 0.4246 | 0.1754 | 0.0308 | 0.2903 | 0.5589 | 0.2686 | 97.9% | |
β | 0.4198 | 0.1198 | 0.0150 | 0.2576 | 0.5820 | 0.3244 | 97.4% | ||
γ | 0.4172 | 0.0828 | 0.0078 | 0.2968 | 0.5376 | 0.2408 | 98.4% | ||
100 | 70% | α | 0.4198 | 0.1802 | 0.0325 | 0.2966 | 0.5429 | 0.2463 | 97.2% |
β | 0.5674 | 0.2674 | 0.0717 | 0.4278 | 0.7069 | 0.2791 | 97.7% | ||
γ | 0.3137 | 0.1863 | 0.0351 | 0.2278 | 0.3995 | 0.1717 | 98.0% | ||
80% | α | 0.4222 | 0.1778 | 0.0316 | 0.3157 | 0.5286 | 0.2128 | 97.9% | |
β | 0.4857 | 0.1857 | 0.0350 | 0.3584 | 0.6130 | 0.2546 | 97.9% | ||
γ | 0.3669 | 0.1331 | 0.0179 | 0.2818 | 0.4519 | 0.1701 | 98.7% | ||
100% | α | 0.4248 | 0.1752 | 0.0307 | 0.3242 | 0.5253 | 0.2011 | 98.1% | |
β | 0.4082 | 0.1082 | 0.0125 | 0.2944 | 0.5221 | 0.2277 | 97.8% | ||
γ | 0.4171 | 0.0829 | 0.0074 | 0.3349 | 0.4993 | 0.1644 | 98.3% | ||
150 | 70% | α | 0.4198 | 0.1802 | 0.0325 | 0.3248 | 0.5148 | 0.1899 | 97.2% |
β | 0.5653 | 0.2653 | 0.0706 | 0.4518 | 0.6788 | 0.2270 | 98.3% | ||
γ | 0.3140 | 0.1860 | 0.0350 | 0.2421 | 0.3860 | 0.1439 | 99.3% | ||
80% | α | 0.4222 | 0.1778 | 0.0316 | 0.3353 | 0.5091 | 0.1738 | 97.7% | |
β | 0.4832 | 0.1832 | 0.0338 | 0.3797 | 0.5866 | 0.2069 | 98.2% | ||
γ | 0.3677 | 0.1323 | 0.0178 | 0.2996 | 0.4358 | 0.1363 | 99.0% | ||
100% | α | 0.4248 | 0.1752 | 0.0307 | 0.3377 | 0.5119 | 0.1742 | 97.3% | |
β | 0.4018 | 0.1018 | 0.0107 | 0.3032 | 0.5004 | 0.1972 | 97.9% | ||
γ | 0.4248 | 0.0752 | 0.0061 | 0.3608 | 0.4888 | 0.1280 | 99.7% | ||
200 | 70% | α | 0.4198 | 0.1802 | 0.0325 | 0.3423 | 0.4973 | 0.1551 | 99.0% |
β | 0.5650 | 0.2650 | 0.0705 | 0.4738 | 0.6561 | 0.1823 | 99.1% | ||
γ | 0.3256 | 0.1744 | 0.0309 | 0.2637 | 0.3875 | 0.1239 | 98.7% | ||
80% | α | 0.4222 | 0.1778 | 0.0316 | 0.3470 | 0.4975 | 0.1505 | 99.6% | |
β | 0.4781 | 0.1781 | 0.0321 | 0.3882 | 0.5679 | 0.1798 | 99.3% | ||
γ | 0.3733 | 0.1267 | 0.0173 | 0.3129 | 0.4336 | 0.1206 | 99.5% | ||
100% | α | 0.4250 | 0.1750 | 0.0306 | 0.3578 | 0.4921 | 0.1343 | 98.7% | |
β | 0.3904 | 0.0904 | 0.0088 | 0.3106 | 0.4703 | 0.1596 | 99.6% | ||
γ | 0.4262 | 0.0738 | 0.0060 | 0.3681 | 0.4843 | 0.1162 | 100% |
Rank | Country | % Global Reserves | Rank | Country | % Global Reserves |
1 | Russia | 19.9 | 23 | Ukraine | 0.6 |
2 | Iran | 17.1 | 24 | Malaysia | 0.5 |
3 | Qatar | 13.1 | 25 | Uzbekistan | 0.4 |
4 | Turkmenistan | 7.2 | 26 | Oman | 0.4 |
5 | United States | 6.7 | 27 | Vietnam | 0.3 |
6 | China | 4.5 | 28 | Israel | 0.3 |
7 | Venezuela | 3.3 | 29 | Argentina | 0.2 |
8 | Saudi Arabia | 3.2 | 30 | Pakistan | 0.2 |
9 | United Arab Emirates | 3.2 | 31 | Trinidad | 0.2 |
10 | Nigeria | 2.9 | 32 | Brazil | 0.2 |
11 | Iraq | 1.9 | 33 | Myanmar | 0.2 |
12 | Canada | 1.3 | 34 | United Kingdom | 0.1 |
13 | Australia | 1.3 | 35 | Thailand | 0.1 |
14 | Azerbaijan | 1.3 | 36 | Mexico | 0.1 |
15 | Algeria | 1.2 | 37 | Bangladesh | 0.1 |
16 | Kazakhstan | 1.2 | 38 | Netherlands | 0.1 |
17 | Egypt | 1.1 | 39 | Bolivia | 0.1 |
18 | Kuwait | 0.9 | 40 | Brunei | 0.1 |
19 | Norway | 0.8 | 41 | Peru | 0.1 |
20 | Libya | 0.8 | 42 | Syria | 0.1 |
21 | Indonesia | 0.7 | 43 | Yemen | 0.1 |
22 | India | 0.7 | 44 | Papua New Guinea | 0.1 |
Rank | Country | reserves2020 | Rank | Country | reserves2020 |
1 | Venezuela | 303.8 | 11 | Nigeria | 36.9 |
2 | Saudi Arabia | 297.5 | 12 | Kazakhstan | 30 |
3 | Canada | 168.1 | 13 | China | 26 |
4 | Iran | 157.8 | 14 | Qatar | 25.2 |
5 | Iraq | 145 | 15 | Algeria | 12.2 |
6 | Russia | 107.8 | 16 | Brazil | 11.9 |
7 | Kuwait | 101.5 | 17 | Norway | 7.9 |
8 | United Arab Emirates | 97.8 | 18 | Angola | 7.8 |
9 | United States | 68.8 | 19 | Azerbaijan | 7 |
10 | Libya | 48.4 | 20 | Mexico | 6.1 |
Rank | Country | Reserves of Gold | Rank | Country | Reserves of Gold | Rank | Country | Reserves of Gold |
1 | USA | 8.1335 | 35 | LBY | 0.1166 | 68 | CYP | 0.0139 |
2 | DEU | 3.3585 | 36 | GRC | 0.1141 | 69 | CUW | 0.0131 |
3 | IMF | 2.814 | 37 | ROK | 0.1045 | 70 | MUS | 0.0124 |
4 | ITA | 2.4518 | 38 | ROU | 0.1036 | 71 | IRL | 0.012 |
5 | FRA | 2.4365 | 39 | BIS | 0.102 | 72 | CZE | 0.0109 |
6 | RUS | 2.2985 | 40 | IRQ | 0.0964 | 73 | KGZ | 0.0102 |
7 | CHN | 1.9483 | 41 | HUN | 0.0945 | 74 | GHA | 0.0087 |
8 | CHE | 1.04 | 42 | AUS | 0.0798 | 75 | PRY | 0.0082 |
9 | JPN | 0.846 | 43 | KWT | 0.079 | 76 | NPL | 0.008 |
10 | IND | 0.7604 | 44 | IDN | 0.0786 | 77 | MNG | 0.0076 |
11 | NLD | 0.6125 | 45 | DNK | 0.0666 | 78 | MMR | 0.0073 |
12 | ECB | 0.5048 | 46 | PAK | 0.0647 | 79 | GTM | 0.0069 |
13 | TUR | 0.4311 | 47 | ARG | 0.0617 | 80 | MKD | 0.0069 |
14 | TAI | 0.4236 | 48 | ARE | 0.0553 | 81 | TUN | 0.0068 |
15 | PRT | 0.3826 | 49 | BLR | 0.0535 | 82 | LVA | 0.0067 |
16 | KAZ | 0.3681 | 50 | QAT | 0.0513 | 83 | LTU | 0.0058 |
17 | UZB | 0.3375 | 51 | KHM | 0.0504 | 84 | COL | 0.0047 |
18 | SAU | 0.3231 | 52 | FIN | 0.049 | 85 | BHR | 0.0047 |
19 | GBR | 0.3103 | 53 | JOR | 0.0435 | 86 | BRN | 0.0046 |
20 | LBN | 0.2868 | 54 | BOL | 0.0425 | 87 | GIN | 0.0042 |
21 | ESP | 0.2816 | 55 | BGR | 0.0408 | 88 | MOZ | 0.0039 |
22 | AUT | 0.28 | 56 | MYS | 0.0389 | 89 | SVN | 0.0032 |
23 | THA | 0.2442 | 57 | SRB | 0.0378 | 90 | ABW | 0.0031 |
24 | POL | 0.2287 | 58 | WAEMU | 0.0365 | 91 | BIH | 0.003 |
25 | BEL | 0.2274 | 59 | PER | 0.0347 | 92 | ALB | 0.0028 |
26 | DZA | 0.1736 | 60 | SVK | 0.0317 | 93 | LUX | 0.0022 |
27 | VEN | 0.1612 | 61 | UKR | 0.0271 | 94 | HKG | 0.0021 |
28 | PHL | 0.1563 | 62 | SYR | 0.0258 | 95 | ISL | 0.002 |
29 | SGP | 0.1537 | 63 | MAR | 0.0221 | 96 | TTO | 0.0019 |
30 | BRA | 0.1297 | 64 | ECU | 0.0219 | 97 | HTI | 0.0018 |
31 | SWE | 0.1257 | 65 | AFG | 0.0219 | 98 | YEM | 0.0016 |
32 | ZAF | 0.1254 | 66 | NGA | 0.0215 | 99 | SUR | 0.0015 |
33 | EGY | 0.125 | 67 | BGD | 0.014 | 100 | SLV | 0.0014 |
34 | MEX | 0.1199 |
n | Mean | Median | Skewness | Kurtosis | Range | Min | Max | Sum | |
Data1 | 20 | 1.900 | 1.700 | 1.860 | 4.185 | 3.000 | 1.100 | 4.100 | 38.000 |
Data2 | 44 | 2.248 | 0.650 | 2.990 | 8.864 | 19.800 | 0.100 | 19.900 | 98.900 |
Data3 | 20 | 83.375 | 42.650 | 1.430 | 1.420 | 297.700 | 6.100 | 303.800 | 1667.500 |
Data4 | 100 | 0.347 | 0.050 | 5.590 | 38.257 | 8.130 | 0.001 | 8.133 | 34.676 |
Distributions | MLE and SE | ||||
α | β | γ | λ | θ | |
LBTLoW | 8.648 | 3.074 | 0.042 | ||
(3.545) | (0.474) | (0.025) | |||
ETGR | 0.103 | 0.692 | 23.539 | -0.342 | |
(0.436) | (0.086) | (105.137) | (1.971) | ||
BW | 0.831 | 0.613 | 29.947 | 11.632 | |
(0.954) | (0.340) | (40.414) | (21.900) | ||
T-Li | 0.665 | 0.359 | |||
(0.332) | (0.048) | ||||
McLL | 0.881 | 2.070 | 1.926 | 19.225 | 32.033 |
(0.109) | (3.693) | (5.165) | (22.341) | (43.081) | |
NMW | 0.121 | 2.784 | 2.787 | 0.003 | 0.008 |
(0.056) | (20.370) | (0.428) | (0.025) | (0.002) | |
W | 0.122 | 2.787 | |||
(0.056) | (0.427) |
Distributions | AIC | CAIC | BIC | HQIC | KS | PV |
LBTLoW | 40.140 | 41.640 | 38.040 | 40.720 | 0.146 | 0.790 |
ETGR | 44.860 | 47.520 | 42.060 | 45.630 | 0.190 | 0.465 |
BW | 42.400 | 45.060 | 39.600 | 43.170 | 0.160 | 0.683 |
T-Li | 65.730 | 66.440 | 64.330 | 66.120 | 0.380 | 0.006 |
McLL | 43.850 | 48.140 | 40.360 | 44.830 | 0.147 | 0.734 |
NMW | 51.170 | 55.460 | 47.680 | 52.150 | 0.190 | 0.501 |
W | 45.170 | 45.880 | 43.780 | 45.560 | 0.180 | 0.509 |
Distributions | MLE and SE | ||||
α | β | γ | λ | θ | |
LBTLoW | 6.268 | 0.623 | 0.484 | ||
(2.631) | (0.066) | (0.210) | |||
ETGR | 0.055 | 0.071 | 8.773 | 0.947 | |
(0.027) | (0.029) | (7.043) | (0.081) | ||
TCWG | 34.076 | 0.802 | 0.005 | 1.12 | |
(81.023) | (0.021) | (0.013) | (0.285) | ||
EKW | 0.221 | 400.298 | 5.215 | 1 | 3.823 |
(0.038) | (718.99) | (0.649) | (0.004) | (3.036) | |
TMW | 0.851 | 1.159 | -0.554 | 0.519 | |
(0.163) | (1.026) | (0.985) | (0.379) | ||
BW | 2.861 | 0.075 | 78.550 | 42.576 | |
(69.095) | (0.090) | (167.320) | (187.300) | ||
T-Li | 0.604 | 0.671 | |||
(0.155) | (0.074) | ||||
McLL | 0.181 | 1.565 | 1.286 | 21.234 | 28.124 |
(0.193) | (9.254) | (5.432) | (34.701) | (45.757) | |
NMW | 6.8 x 10−8 | 0.680 | 0.223 | 0.015 | 0.806 |
(0.623) | (0.110) | (617.48) | (0.015) | (0.418) | |
W | 0.799 | 0.621 | |||
(0.136) | (0.068) |
Distributions | AIC | CAIC | BIC | HQIC | KS | PV |
LBTLoW | 132.210 | 132.810 | 131.140 | 134.200 | 0.130 | 0.425 |
ETGR | 143.470 | 144.490 | 142.040 | 146.110 | 0.180 | 0.118 |
TCWG | 137.690 | 138.710 | 136.260 | 140.330 | 0.150 | 0.251 |
EKW | 133.890 | 135.470 | 132.110 | 140.330 | 0.140 | 0.355 |
TMW | 140.900 | 142.480 | 139.120 | 144.210 | 0.150 | 0.276 |
BW | 133.180 | 134.200 | 131.750 | 135.820 | 0.130 | 0.408 |
T-Li | 174.360 | 174.660 | 173.650 | 175.690 | 0.200 | 0.057 |
McLL | 134.830 | 136.410 | 133.040 | 138.130 | 0.130 | 0.419 |
NMW | 143.780 | 145.360 | 142.000 | 147.090 | 0.160 | 0.243 |
W | 138.650 | 138.940 | 137.940 | 139.970 | 0.170 | 0.139 |
Distributions | MLE and SE | ||||
α | β | γ | λ | θ | |
LBTLoW | 2.515 | 0.756 | 0.040 | ||
(3.877) | (0.166) | (0.048) | |||
WEIW | 0.909 | 0.871 | 7.225 | ||
(106700) | (0.152) | (384700) | |||
TMW | 0.998 | 0.459 | -0.443 | 0.202 | |
(0.081) | (18.537) | (18.537) | (0.769) | ||
T-Li | 0.021 | 0.384 | |||
(0.345) | (0.004) | ||||
McLL | 0.208 | 93.978 | 1.279 | 24.759 | 32.815 |
(0.499) | (1721) | (19.272) | (142.806) | (161.611) | |
NMW | 10.7 x 10−8 | 0.930 | 0.859 | 7.46 x 10−8 | 0.017 |
(0.001) | (0.250) | (1.216) | (0.002) | (0.017) | |
EKW | 0.167 | 261.64 | 45.725 | 1.201 | 2.138 |
(0.079) | (1709) | (219.725) | (0.741) | (7.209) |
Distributions | AIC | CAIC | BIC | HQIC | KS | PV |
LBTLoW | 221.690 | 223.190 | 219.600 | 222.280 | 0.135 | 0.857 |
WEIW | 223.400 | 224.900 | 221.300 | 223.980 | 0.157 | 0.708 |
TMW | 226.410 | 230.690 | 222.910 | 227.380 | 0.153 | 0.734 |
T-Li | 230.480 | 231.180 | 229.080 | 230.870 | 0.265 | 0.120 |
McLL | 225.990 | 230.280 | 222.500 | 222.500 | 0.146 | 0.789 |
NMW | 226.570 | 230.860 | 223.080 | 227.540 | 0.140 | 0.826 |
EKW | 226.290 | 230.570 | 222.790 | 229.300 | 0.148 | 0.776 |
Distributions | MLE and SE | ||||
α | β | γ | λ | θ | |
LBTLoW | 6.498 | 0.482 | 1.490 | ||
(2.301) | (0.034) | (0.573) | |||
EKW | 0.221 | 1096 | 4.424 | 1 | 1.717 |
(0.030) | (1376) | (1.817) | (0.001) | (0.901) | |
TMW | 0.596 | 2.612 | 0.588 | -0.523 | |
(0.057) | (0.689) | (0.256) | (0.346) | ||
BW | 134.832 | 0.073 | 49.149 | 22.930 | |
(956.622) | (0.060) | (74.497) | (46.500) | ||
WEIW | 27.512 | 0.549 | 0.094 | ||
(3272000) | (0.042) | (856.967) | |||
W | 2.648 | 0.489 | |||
(0.281) | (0.035) |
Distributions | AIC | CAIC | BIC | HQIC | KS | PV |
LBTLoW | –170.510 | –170.260 | –170.510 | –167.350 | 0.070 | 0.704 |
ETGR | –167.710 | –167.070 | –167.710 | –157.710 | 0.078 | 0.584 |
BW | –158.180 | –157.540 | –158.180 | –152.910 | 0.077 | 0.593 |
T-Li | –170.220 | –169.800 | –170.220 | –166.010 | 0.071 | 0.703 |
McLL | –170.200 | –169.950 | –170.200 | –167.040 | 0.091 | 0.383 |
W | –157.390 | –157.260 | –157.390 | –155.280 | 0.100 | 0.269 |