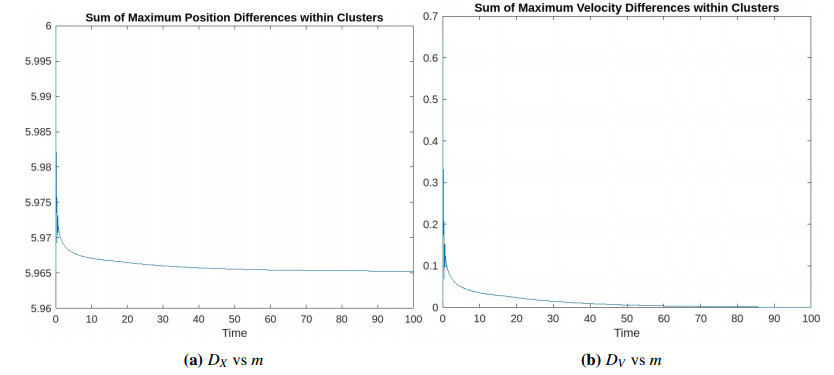
This paper demonstrates several sufficient frameworks for the multi-cluster flocking behavior of the fractional Cucker–Smale (CS) model. For this, we first employ the Caputo fractional derivative instead of the usual derivative to propose the fractional CS model with the memory effect. Then, using mathematical tools based on fractional calculus, we present suitable sufficient conditions in terms of properly separated initial data close to the multi-cluster, and well-prepared system parameters for the multi-cluster flocking of the fractional system to emerge. Finally, we offer several numerical simulations and compare them with the analytical results.
Citation: Hyunjin Ahn. On the multi-cluster flocking of the fractional Cucker–Smale model[J]. Mathematics in Engineering, 2024, 6(4): 607-647. doi: 10.3934/mine.2024024
[1] | Matteo Lapucci, Davide Pucci . Mixed-integer quadratic programming reformulations of multi-task learning models. Mathematics in Engineering, 2023, 5(1): 1-16. doi: 10.3934/mine.2023020 |
[2] | Jayme Vaz Jr., Edmundo Capelas de Oliveira . On the fractional Kelvin-Voigt oscillator. Mathematics in Engineering, 2022, 4(1): 1-23. doi: 10.3934/mine.2022006 |
[3] | Luca Azzolin, Luca Dedè, Antonello Gerbi, Alfio Quarteroni . Effect of fibre orientation and bulk modulus on the electromechanical modelling of human ventricles. Mathematics in Engineering, 2020, 2(4): 614-638. doi: 10.3934/mine.2020028 |
[4] | Karishma, Harendra Kumar . A novel hybrid model for task scheduling based on particle swarm optimization and genetic algorithms. Mathematics in Engineering, 2024, 6(4): 559-606. doi: 10.3934/mine.2024023 |
[5] | Federico Cluni, Vittorio Gusella, Dimitri Mugnai, Edoardo Proietti Lippi, Patrizia Pucci . A mixed operator approach to peridynamics. Mathematics in Engineering, 2023, 5(5): 1-22. doi: 10.3934/mine.2023082 |
[6] | Luca Formaggia, Alessio Fumagalli, Anna Scotti . A multi-layer reactive transport model for fractured porous media. Mathematics in Engineering, 2022, 4(1): 1-32. doi: 10.3934/mine.2022008 |
[7] | Zaffar Mehdi Dar, M. Arrutselvi, Chandru Muthusamy, Sundararajan Natarajan, Gianmarco Manzini . Virtual element approximations of the time-fractional nonlinear convection-diffusion equation on polygonal meshes. Mathematics in Engineering, 2025, 7(2): 96-129. doi: 10.3934/mine.2025005 |
[8] | Giuseppe Procopio, Massimiliano Giona . Bitensorial formulation of the singularity method for Stokes flows. Mathematics in Engineering, 2023, 5(2): 1-34. doi: 10.3934/mine.2023046 |
[9] | Ko-Shin Chen, Cyrill Muratov, Xiaodong Yan . Layered solutions for a nonlocal Ginzburg-Landau model with periodic modulation. Mathematics in Engineering, 2023, 5(5): 1-52. doi: 10.3934/mine.2023090 |
[10] | Anoumou Attiogbe, Mouhamed Moustapha Fall, El Hadji Abdoulaye Thiam . Nonlocal diffusion of smooth sets. Mathematics in Engineering, 2022, 4(2): 1-22. doi: 10.3934/mine.2022009 |
This paper demonstrates several sufficient frameworks for the multi-cluster flocking behavior of the fractional Cucker–Smale (CS) model. For this, we first employ the Caputo fractional derivative instead of the usual derivative to propose the fractional CS model with the memory effect. Then, using mathematical tools based on fractional calculus, we present suitable sufficient conditions in terms of properly separated initial data close to the multi-cluster, and well-prepared system parameters for the multi-cluster flocking of the fractional system to emerge. Finally, we offer several numerical simulations and compare them with the analytical results.
The term "flocking" refers to a phenomenon in which agents governed by an interacting many-body system converge at the same velocity while forming an appropriate group based on limited environmental information and simple laws. Flocking behaviors are ubiquitous in complex ecosystems. Examples include the flocks of birds, fish, sheep, spectators at sport events and the platooning of automated vehicles. Many mathematical models have been proposed in the mathematics community to describe flocking. Among them, the Cucker–Smale (CS) model introduced in [14] is a successful mathematical model representing flocking. The CS model is a Newtonian second-order system in terms of position-velocity, which is given by the following Cauchy problem concerning {(xi,vi)}Ni=1:
{dxidt=vi,t>0,i∈[N]:={1,…,N},dvidt=1NN∑j=1ϕ(‖xi−xj‖)(vj−vi),(xi(0),vi(0))∈Rd×Rd, | (1.1) |
where N and ‖⋅‖ denote the number of agents and the standard Euclidean l2-norm, respectively, and we set R+:=[0,∞) throughout the paper. In addition, ϕ:R+→R+ is a communication weight satisfying the following conditions:
0≤ϕ(r)≤ϕ(0)=1,(ϕ(r1)−ϕ(r2))(r1−r2)≤0,ϕ(⋅)∈C0,1loc(R+;R+). |
Due to the suitable dissipative structure regarding velocity in (1.1)2, the CS model and its variants have been actively studied from various perspectives. Examples include the mean-field limit [22,23], kinetic descriptions [7,25], hydrodynamic descriptions [17,27], time delay [13], stochastic analysis [8], relativistic correction [3,21], bi-cluster flocking [9], unit-speed constraint [1,10], temperature fields [16,24], collision avoidance [12] and extension to manifolds [2,4]. For the survey paper, refer to [11].
However, the literature on the CS model has only been investigated through a Markovian approach. Indeed, the behaviors of many agents (humans, animals, plants, etc.) in an ecosystem tend to be influenced by their memories and experiences, at least their short-term memory. Therefore, instead of the CS system (1.1) ignoring memory effects, we are primarily interested in studying the flocking system in a non-Markovian sense to investigate more realistic flocking mathematically. We consider the following fractional CS system by changing the usual time-derivative to the Caputo fractional derivative of order α∈(0,1):
{Dcαxi=vi,t>0,i∈[N],Dcαvi=1NN∑j=1ϕ(‖xi−xj‖)(vj−vi),(xi(0),vi(0))∈Rd×Rd, | (1.2) |
where Dcα denotes the Caputo fractional derivative of order α∈(0,1) (see Definition 2.1).
The Caputo fractional derivative is used in memory-preserving dynamic systems because it incorporates historical information into the derivative operator, reflecting the system's dependence on past states. This feature allows for more accurate modeling of systems with memory effects, such as viscoelastic materials, biological tissues, and economic systems. The flexibility of fractional derivatives enables the capture of complex temporal behaviors and nonlinear characteristics that integer-order derivatives cannot adequately describe. Consequently, the Caputo derivative is essential for representing the intricate dynamics of various natural and engineered systems.
Because dealing with a fractional derivative is more complicated than dealing with the usual derivative, (1.2) has been less lively studied than that in (1.1) (e.g., the discrete model [18,19], the Riemann–Liouville fractional derivative under a constant communication weight [29], optimal control problems [32,33], and mono-clustser flocking dynamics under a general communication weight [20] and under general network topologies [26]).
From the mentioned literature on (1.2), the multi-cluster flocking of the system (1.2) has not yet been addressed. Multi-cluster flocking behavior is often observed in nature and human society, for example, in social opinion disagreement, animal herding invaded by predators, K-means algorithms, flight multi-formation, and groups with members with similar characteristics.
Throughout this paper, we are primarily concerned with the following problem: Under what conditions in terms of initial data and system parameters does multi-cluster flocking occur in the proposed fractional system (1.2)?
Next, we provide concept definitions for the mono-, bi- and multi-cluster flocking of the system (1.2) as follows:
Definition 1.1. (n-cluster flocking) Let Z={(xi,vi)}Ni=1 be the solution of (1.2).
(1) The configuration Z exhibits n-cluster flocking behavior if there exist n-cluster groups Zβ={(xβi,vβi)}Nβi=1 such that the following assertions hold:
(i)|Zβ|=Nβ≥1,n∑β=1|Zβ|=n∑β=1Nβ=N,˙∪nβ=1Zβ=Z,(ii)(Flocking of the β-th cluster group)supt∈R+maxk,l∈[Nβ]‖xβk(t)−xβl(t)‖<∞,limt→∞maxk,l∈[Nβ]‖vβk(t)−vβl(t)‖=0,(iii)(Separation between different groups)lim supt→∞mink∈[Nβ],l∈[Nγ]‖xβk(t)−xγl(t)‖=∞,β≠γ. |
(2) The configuration Z exhibits mono-cluster flocking when n=1, bi-cluster flocking when n=2, and multi-cluster flocking when n≥3.
The rest of the paper is organized as follows: In Section 2, we briefly introduce basic materials to be used in Section 3 and Section 4 based on fractional calculus and present several basic estimates for (1.2). In Section 3, we study a sufficient framework guaranteeing the bi-cluster flocking of (1.2). In Section 4, we demonstrate a sufficient framework for the multi-cluster flocking of (1.2). In Section 5, we provide several numerical simulations and compare them with the analytical results. Finally, Section 6 is devoted to a brief summary of the main results and some discussion on the remaining issues to be investigated in future work.
Notation. We employ the following notations for concision:
(1)[l]:={1,…,l}forl∈N,Id:=d×didentity matrix,R+:=[0,∞),(2)Rm×n:=the set ofm×nmatrices,AT:=the transpose of A,⌊⋅⌋:=Gauss integer,(3)X:=(x1,…,xN)T∈RN×d,V:=(v1,…,vN)T∈RN×d,xi,vi∈Rd×1,(4)DZ:=maxi,j∈[N]‖zi−zj‖forZ=(z1,…,zN)T,‖⋅‖:=the standardl2-norm. |
In this section, we revisit basic materials for fractional calculus to be crucially employed in Sections 3 and 4. Subsequently, we present two preliminary lemmas to study the bi- and multi-cluster flocking of the proposed fractional system (1.2).
In this subsection, we briefly introduce basic concepts and the backgrounds based on fractional calculus, beginning with defining the Caputo fractional derivative.
Definition 2.1 (Caputo derivative). [31] For α∈(0,∞), if the right sides are well defined, the Caputo fractional derivative Dcαf of order α is defined as
Dcαf(t):={1Γ(⌊α⌋+1−α)∫t0f(⌊α⌋+1)(s)(t−s)α−⌊α⌋ds,ifα≠⌊α⌋,fα(t),ifα=⌊α⌋. |
Next, we recall the gamma function and beta function as follows:
Definition 2.2 (Gamma and beta functions). Let C and Z≤0 be the set of complex numbers and the set of nonpositive integers, respectively and let Re(w) be the real part of w∈C. The gamma function Γ=Γ(z) for z∈C and beta function B=B(z,w) for Re(z)>0 and Re(w)>0 are given by
(1) (Gamma function) Γ(z):=∫∞0e−ttz−1dt,z∈C.
(2) (Beta function) B(z,w):=∫10tz−1(1−t)w−1dt, for Re(z),Re(w)>0.
Thus, the following basic properties hold for Γ and B:
Remark 2.1. (Basic properties for Γ and B)
(1) Γ(1)=1,Γ(n)=(n−1)!for all positive integer n,Γ(z+1)=zΓ(z).
(2) B is symmetric, i.e., B(z,w)=B(w,z), B(z,w)=Γ(z)Γ(w)Γ(z+w).
The following text provides the basic concepts and essential relations for the Mittag-Leffler function. This function is often employed when addressing the convergence analysis of fractional ordinary differential equations, especially when deriving bi- and multi-flocking estimates.
Definition 2.3 (Mittag-Leffler function). For given α,β∈C, the Mittag-Leffler function, denoted by Eα,β, is defined as
Eα,β(z):=∞∑k=0zkΓ(αk+β). |
Proposition 2.1 (Basic facts (Ⅰ)). [20,31] For α,β,γ∈C, one has the following assertions:
(1) Eα,β(z)=zEα,α+β(z)+1Γ(β).
(2) For β,γ>0 and α,λ∈C,
1Γ(γ)∫t0(t−s)γ−1Eα,β(λsα)sβ−1ds=tβ+γ−1Eα,β+γ(λtα). |
(3) For α,y,z∈C, and β,γ>0,
∫t0sγ−1Eα,γ(ysα)(t−s)β−1Eα,β(z(t−s)α)ds=yEα,β+γ(ytα)−zEα,β+γ(ztα)y−ztβ+γ−1. |
The proposition below guarantees that the flocking estimates for Eα,β(⋅) that we obtain later are related to an algebraic decay rate.
Proposition 2.2 (Basic facts (Ⅱ)). [20,31] Suppose that α,β and γ satisfy
α∈(0,2),β∈R,πα2<γ<min(π,πα). |
Let N be the set of all natural numbers. Then, we have the following relations for n∈N:
(1) For |arg(z)|≤γ,
Eα,β(z)=1αexp(z1α)−n∑k=1z−kΓ(β−αk)+O(|z|−1−n),as|z|→∞. |
(2) For γ≤|arg(z)|≤π,
Eα,β(z)=−n∑k=1z−kΓ(β−αk)+O(|z|−1−n),as|z|→∞. |
(3) As a direct consequence of (2),
Eα,1(−Ctα)∼t−αCΓ(1−α),ast→∞,for every positive constantC. |
Subsequently, we define "completely monotone" to treat the fractional Caputo derivative of order α∈(0,1) in more detail.
Definition 2.4 (Completely monotone). [30] A C∞-function f is completely monotone if
(−1)kf(k)(t)≥0,t∈(0,∞),k∈N∪{0}. |
In addition, every completely monotone function is always nonnegative, monotonically decreasing, and convex.
Next, we present a previous result regarding the necessary and sufficient conditions for Eα,β(−t) to be completely monotone.
Proposition 2.3 (Complete monotonicity of Eα,β(−t)).[34] The term Eα,β(−t) is completely monotone on t∈R+−{0} if and only if α∈(0,1] and β≥α.
Next, for α∈(0,1) and T>0, we consider
Dαcx(t)=f(t,x(t)),t∈[0,T),x(0)=x0. | (2.1) |
Then, (2.1) can be transformed into the following integral equation when f is continuous:
x(t)=x0+D−αcf(t,x(t))=x0+1Γ(α)∫t0f(s,x(s))(t−s)1−αds. | (2.2) |
For a constant matrix A∈Rd×d and f(t,x(t))=Ax(t)+y(t), (2.1) is written as
Dαcx(t)=Ax(t)+y(t),t∈[0,T),x(0)=x0. | (2.3) |
In this case, under the appropriate conditions for y(t), a unique solution x(t) exists on [0,T]. Moreover, the solution can be expressed in a more specific form than (2.2).
Proposition 2.4 (Solution of (2.3)). [5,20] Let x(t) be the solution of (2.3) of order α∈(0,1) and let y(t) be an element of a space C1−α([0,T]), where C1−α([0,T]) is given by
C1−α([0,T]):={f(t)∈C0[0,T]|‖f‖C1−α:=supt∈[0,T]‖t1−αf(t)‖<∞}. |
Then, the solution of (2.3) uniquely exists and is expressed by
x(t)=Eα,1(Atα)x0+∫t0(t−s)α−1Eα,α(A(t−s)α)y(s)ds, |
where Eα,β(A) is defined as
Eα,β(A):=∞∑k=0AkΓ(αk+β),forA∈Rd×d. |
In the sequel, we briefly describe the Cauchy–Lipschitz theory for the fractional ODE of order α∈(0,1] as follows:
Proposition 2.5 (Cauchy–Lipschitz theory for a fractional ODE). [6] We consider the following fractional ODE system of order α∈(0,1]:
Dαcx(t)=f(t,x(t)),x(t0)=x0. | (2.4) |
If f is locally Lipschitz continuous in x and continuous in t, then for some strictly positive number ϵ, there exists a unique solution x(t) of (2.4) on the interval [t0−ϵ,t0+ϵ].
Finally, we state the comparison principle for the fractional ODE system (2.1).
Proposition 2.6 (Comparison principle for a fractional ODE). [28] Suppose that f(t,x) and F(t,x) are two continuous functions defined on G:=[0,T]×R satisfying
f(t,x(t))≤F(t,x(t))for(t,x)∈G. |
Next, assume that x=ψ(t) and x=ϕ(t) are the solutions of the following initial value problem for α∈(0,1):
(1) Dαcx(t)=f(t,x(t)),x(0)=x0,
(2) Dαcx(t)=F(t,x(t)),x(0)=x0, respectively.
Then, we have
ψ(t)≤ϕ(t),t∈[0,T]. |
For readers interested in several relations between the monotone function and the sign change of its Caputo derivative, we recommend [15].
In this subsection, we estimate the uniform boundedness of ‖V‖ in terms of the initial data motivated by the arguments employed in [20] and offer the previous lemma proven in [16]. First, we define the following functional:
Ψij(t):=ϕij(t)N+(1−∑Nk=1ϕik(t)N)δij, |
where δij denotes the Kronecker delta, and for simplicity, we set the following throughout the paper:
ϕij(t):=ϕ(‖xj(t)−xi(t)‖),i,j∈[N]. |
Then, it is straightforward to check that, for i,j∈[N], the functional Ψij satisfies
Ψij≥ϕijN,N∑j=1Ψij=1,1NN∑j=1ϕij(vj−vi)=N∑j=1Ψij(vj−vi). |
In the following text, we convert (1.2) into a matrix formulation in terms of X, V and the system parameters using the above functional Ψij, as follows:
DcαX(t)=V(t),DcαV(t)=−V(t)+Φ(X(t))V(t), | (2.5) |
where Φ(X(t))∈RN×N is given by
(Φ(X(t)))ij=Ψij(t)fori,j∈[N]. |
Then, combining (2.5) and Proposition 2.4 results in
V(t)=Eα,1(−tα)V(0)+∫t0(t−s)α−1Eα,α(−(t−s)α)Φ(X(s))V(s)ds. | (2.6) |
Next, we consider the following recurrence relation {V(i)}∞i=0 based on (2.6):
V(0)=0,i∈N∪{0},V(i+1)=Eα,1(−tα)V(0)+∫t0(t−s)α−1Eα,α(−(t−s)α)Φ(X(s))V(i)(s)ds. | (2.7) |
We observe that, for t∈R+,
sups∈[0,t]‖Φ(X(s))‖op≤1, | (2.8) |
where ‖⋅‖op denotes the operator norm of a matrix. Because Ψij≥0 and ∑Nj=1Ψij=1, we derive
‖Φ(X(s))‖op≤maxi∈[N]N∑j=1Ψ2ij(s)≤maxi∈[N](N∑j=1Ψij(s))2=1. |
Hence, we prove that ‖V‖ is uniformly bounded by ‖V(0)‖ from the above frameworks.
Lemma 2.1 (Uniform boundedness of ‖V‖). In the recurrence relation (2.7), the following assertion holds for i∈N∪{0}:
‖V(i+1)−V(i)‖≤‖V(0)‖(sups∈[0,t]‖Φ(X(s))‖op)i∞∑j=0((j+i)Cj⋅(−1)jΓ(α(j+i)+1))⋅tα(j+i)=‖V(0)‖(−sups∈[0,t]‖Φ(X(s))‖op)i∞∑j=i(jCi⋅(−1)jΓ(αj+1))⋅tαj. |
Furthermore, if V is the solution of (2.5), we also demonstrate that, for t∈R+,
limi→∞V(i)=V,‖V‖≤‖V(0)‖. |
Proof. We employ an inductive argument to verify the assertion.
● (The case ofi=0): Definition 2.3 and (2.7) yield that
‖V(1)−V(0)‖≤‖V(0)‖Eα,1(−tα)=‖V(0)‖∞∑j=0((−1)jΓ(αj+1))⋅tαj. |
Therefore, we reach the desired assertion when i=0.
● (The case ofi>0): We assume that the assertion holds for k≤i, where k∈N∪{0}. Then, the inductive assumption, (2.7), (2.8), and Definition 2.3 imply that
‖V(i+2)−V(i+1)‖≤‖V(0)‖(sups∈[0,t]‖Φ(X(s))‖op)i+1×∫t0(t−s)α−1Eα,α(−(t−s)α)∞∑j=0((j+i)Cj⋅(−1)jΓ(α(j+i)+1))⋅sα(j+i)ds=‖V(0)‖(sups∈[0,t]‖Φ(X(s))‖op)i+1×∞∑k=0∞∑j=0((j+i)Cj⋅(−1)k+jΓ(α(k+1))Γ(α(j+i)+1))⋅∫t0(t−s)α(k+1)−1sα(j+i)ds. | (2.9) |
From the beta function (see Definition 2.2) and the second assertion of Remark 2.1, we immediately have that for z,w∈C satisfying Re(z)>0 and Re(w)>0,
Γ(z)Γ(w)Γ(z+w)=B(z,w):=∫10tz−1(1−t)w−1dt=∫t0(t−s)w−1sz−1tz+w−1ds, |
and we obtain
∞∑k=0∞∑j=0((j+i)Cj⋅(−1)k+jΓ(α(k+1))Γ(α(j+i)+1))⋅∫t0(t−s)α(k+1)−1sα(j+i)ds=∞∑k=0∞∑j=0((j+i)Cj⋅(−1)k+j⋅Γ(α(k+1))Γ(α(j+i)+1)Γ(α(k+1))Γ(α(j+i)+1)Γ(α(k+j+i+1)+1))⋅tα(k+j+i+1)=∞∑k=0∞∑j=0((j+i)Cj⋅(−1)k+jΓ(α(k+j+i+1)+1))⋅tα(k+j+i+1)=∞∑j=0∞∑l=j((j+i)Cj⋅(−1)lΓ(α(l+i+1)+1))⋅tα(l+i+1)=∞∑l=0l∑j=0(j+i)Cj((−1)lΓ(α(l+i+1)+1))⋅tα(l+i+1)=∞∑l=0((l+i+1)Cl⋅(−1)lΓ(α(l+i+1)+1))⋅tα(l+i+1)=(−1)i+1∞∑l=i+1(lCi+1⋅(−1)lΓ(αl+1))⋅tαl. | (2.10) |
Thus, we combine (2.9) and (2.10) to attain the desired assertion. Subsequently, we estimate from (2.10), Definition 2.3, Proposition 2.3, and (2.8) that
∞∑k=0‖V(k+1)−V(k)‖≤‖V(0)‖∞∑i=0∞∑j=i(−sup0≤s≤t‖Φ(X(s))‖op)i(jCi⋅(−1)jΓ(αj+1))⋅tαj=‖V(0)‖∞∑j=0(−1)jtαjΓ(αj+1)j∑i=0(−sup0≤s≤t‖Φ(X(s))‖op)ijCi=‖V(0)‖∞∑j=0(−1)jtαjΓ(αj+1)(1−sup0≤s≤t‖Φ(X(s))‖op)j=‖V(0)‖∞∑j=0tαjΓ(αj+1)(sup0≤s≤t‖Φ(X(s))‖op−1)j=‖V(0)‖Eα,1((sup0≤s≤t‖Φ(X(s))‖op−1)t)≤‖V(0)‖. |
The outcome induces that {V(i)}∞i=0 is a Cauchy sequence, and if we set V∞:=limi→∞V(i), then V∞ is the unique solution of (2.6) (i.e., V=V∞). Therefore, the desired lemma holds due to
‖V‖=‖V∞‖=∞∑k=0‖V(k+1)−V(k)‖≤‖V(0)‖. |
Moreover, we introduce the following crucial lemma to investigate the velocity alignment estimate in terms of DV in (1.2):
Lemma 2.2 (Key estimate). [16] Assume that A∈RN×N is a nonnegative stochastic matrix (i.e.,Aij≥0 and ∑Nj=1Aij=1 for i,j∈[N]) and Z,W∈RN×d are matrices satisfying
W=AZ. |
Then, it follows that
DW≤(1−μ(A))DZ, |
where μ(A) denotes the ergodicity coefficient of A∈RN×N defined by
μ(A):=mini,j∈[N]N∑k=1min(Aik,Ajk), |
and we set
Z:=(z1,…,zN)T,W:=(w1,…,wN)T,zi:=(z1i,…,zdi)T,wi:=(w1i,…,wdi)T. |
In this section, we demonstrate the bi-cluster flocking of the fractional system (1.2) under admissible data in terms of the initial data and system parameters. For this, we reorganize (1.2) to discriminate two cluster groups Z1={(x1i,v1i)}N1i=1 and Z2={(x2j,v2j)}N2j=1 from each other, where N1+N2=N and N1,N2≥1, as follows:
{Dcαx1i=v1i,Dcαx2j=v2j,t>0,i∈[N1],j∈[N2],Dcαv1i=1NN1∑k=1ϕ(‖x1i−x1k‖)(v1k−v1i)+1NN2∑k=1ϕ(‖x1i−x2k‖)(v2k−v1i),Dcαv2j=1NN2∑k=1ϕ(‖x2j−x2k‖)(v2k−v2j)+1NN1∑k=1ϕ(‖x2j−x1k‖)(v1k−v2j),(xk(0),vk(0))∈R2d,k∈[N]. | (3.1) |
In this subsection, we present several sufficient frameworks for the bi-cluster flocking of (3.1) and employ notation for simplicity. First, we set the following deviations and averages for position-velocity: For i∈[N1] and j∈[N2],
● (Position averages and deviations)
x1cen:=1N1N1∑i=1x1i,x2cen:=1N2N2∑j=1x2j,ˆx1i:=x1i−x1cen,ˆx2j:=x2j−x2cen. |
● (Velocity averages and deviations)
v1cen:=1N1N1∑i=1v1i,v2cen:=1N2N2∑j=1v2j,ˆv1i:=v1i−v1cen,ˆv2j:=v2j−v2cen. |
Then, we define the configuration vectors for each cluster group by
● (Configuration vectors for each cluster group)
X1:=(x11,…,x1N1),V1:=(v11,…,v1N1),X2:=(x21,…,x2N2),V2:=(v21,…,v2N2). |
Moreover, we set
● (Reordering of configuration vectors)
X:=(X1,X2)andV:=(V1,V2). |
Similarly, we let
● (Configuration vectors for the deviation of each cluster group)
ˆX1:=(ˆx11,…,ˆx1N1),ˆV1:=(ˆv11,…,ˆv1N1),ˆX2:=(ˆx21,…,ˆx2N2),ˆV2:=(ˆv21,…,ˆv2N2), |
and
● (Configuration vectors for deviation)
ˆX:=(ˆX1,ˆX2)andˆV:=(ˆV1,ˆV2). |
Next, we define Δv as
Δkv=vk1cen−vk2cen,k∈[d], |
where ak denotes the k-th component of a∈Rd. The functional Δv measures the difference between velocity averages in the two cluster groups Z1 and Z2.
Subsequently, we obtain basic estimates concerning the mentioned notation as follows:
Lemma 3.1 (Basic estimate). Suppose that (X,V) is the solution of the fractional system (3.1). Then, the following relations hold for t∈R+:
N1∑i=1‖ˆv1i‖2+N1‖v1cen‖2=‖V1‖2,N2∑j=1‖ˆv2j‖2+N2‖v2cen‖2=‖V2‖2. |
Proof. To prove the first assertion, from ∑N1i=1(v1i−v1cen)=0, we get
N1∑i=1‖ˆv1i‖2=N1∑i=1‖v1i−v1cen‖2=12N1N1∑i,j=1‖v1i−v1j‖2=N1∑i=1‖v1i‖2−1N1N1∑i,j=1⟨v1i,v1j⟩=‖V1‖2−N1‖v1cen‖2. |
Likewise, one has
N2∑j=1‖ˆv2j‖2+N2‖v2cen‖2=‖V2‖2. |
In what follows, we reinterpret the bi-cluster fractional system (3.1) in terms of position–velocity averages to later estimate Δkv and the rate at which the bi-cluster formation occurs.
Lemma 3.2 (Fractional systems for averages and deviations). Let (X,V) be the solution of the system (3.1). Then, (x1cen,v1cen), (x2cen,v2cen), (ˆx1i,ˆv1i), and (ˆx2j,ˆv2j) satisfy
{Dcαx1cen=v1cen,Dcαx2cen=v2cen,t>0,N1Dcαv1cen=1NN1∑i=1N2∑k=1ϕ(‖x1i−x2k‖)(v2k−v1i),N2Dcαv2cen=1NN2∑j=1N1∑k=1ϕ(‖x2j−x1k‖)(v1k−v2j) |
and
{Dcαˆx1i=ˆv1i,Dcαˆx2j=ˆv2j,t>0,i∈[N1],j∈[N2],Dcαˆv1i=−Dcαv1cen+1NN1∑k=1ϕ(‖x1i−x1k‖)(ˆv1k−ˆv1i)+1NN2∑k=1ϕ(‖x1i−x2k‖)(v2k−v1i),Dcαˆv2j=−Dcαv2cen+1NN2∑k=1ϕ(‖x2j−x2k‖)(ˆv2k−ˆv2j)+1NN1∑k=1ϕ(‖x2j−x1k‖)(v1k−v2j). |
Proof. We take ∑N1i=1 and ∑N2j=1 in (3.1)2 and (3.1)3, respectively, and then use the standard technique of interchanging i(j) and k and dividing by 2 to these, respectively, to reach the desired first equation. To get the second equation, we use (3.1) and the definitions of ˆx1i, ˆv1i, ˆx2j, and ˆv2j.
In the following, we define position-velocity diameters for each cluster group as follows:
● (The position-velocity diameter of each cluster group)
DX1:=maxi,j∈[N1]‖x1i−x1j‖,DV1:=maxi,j∈[N1]‖v1i−v1j‖,DX2:=maxi,j∈[N2]‖x2i−x2j‖,DV2:=maxi,j∈[N2]‖v2i−v2j‖. |
In addition, we set
● (Total position-velocity diameter)
DX:=DX1+DX2,DV:=DV1+DV2. |
Before we end this subsection, we provide several sufficient frameworks concerning the initial data and system parameters to derive the bi-cluster flocking estimate of the fractional system (3.1). Now, we display the admissible set (F) as follows:
(F):={(X(0),V(0))∈R2dN|(F1)−(F3)hold}. |
⋄ (F1)(Separated initial data): For some k∈[d], there is a nonnegative number r0 satisfying that initial position configurations are selected to be split suitably as below:
0≤r0<mini∈[N1],j∈[N2](xk1i(0)−xk2j(0)). |
⋄ (F2)(Assumption for group formation): There exists a nonnegative number D∞X≥0 such that
DX(0)+DV(0)ϕ(D∞X)Γ(α)+22α√2‖V(0)‖ϕ(D∞X)Γ(α)∫∞0ϕ(Δkv(0)4Γ(α+1)sα+r0)ds+2√2‖V(0)‖ϕ(D∞X)Γ(α)supt∈R+∫t0ϕ(Δkv(0)4Γ(α+1)(s2)α+r0)(t−s)1−αds≤D∞X. |
⋄ (F3)(Closeness to the bi-cluster): The initial data are in a formation state close to the bi-cluster as follows: For β∈[2],
∘Δkv(0)4>√2(N1−1)DˆV1(0)+√2(N2−1)DˆV2(0),≥√N‖V(0)‖2√N1N2Γ(α)supt∈R+(∫t0ϕ(Δkv(0)4Γ(α+1)sα+r0)(t−s)1−αds),∘2√2(N−Nβ)‖V(0)‖Nϕ(D∞X)supt∈R+(∫t0ϕ(Δkv(0)4Γ(α+1)sα+r0)(t−s)1−αds)≤DˆVβ(0). |
Herein, we briefly comment on the described sufficient framework (F). In (F1), the initial positions of the two clusters Z1 and Z2 are sufficiently far apart to attain the desired bi-cluster flocking. In addition, (F2) is the condition ensuring the group formation of each cluster group, and (F3) is the condition that the initial state of (3.1) is close to a bi-cluster. Finally, we notice that (F) is not empty when we take a sufficiently large r0 and appropriate initial data and system parameters, for instance, ϕ with a compact support or an exponential decay rate, etc.
In this subsection, we verify the bi-cluster flocking of (3.1) under the sufficient framework (F). To do this, we begin with demonstrating that the distance between two cluster groups is greater than some function proportional to tα for t∈R+. Throughout this subsection, we define the following:
ϕM(t):=maxi∈[N1],j∈[N2]ϕ(‖x2j−x1i‖). |
Lemma 3.3 (Estimate for Dcα(Δkv(t))). Suppose that (X, V) is the solution of (3.1). Then, it follows that, for t\in \mathbb{R}_+ ,
\begin{align*} D_{\alpha}^c(\Delta_v^k) \geq -\frac{\sqrt{N}{\|V(0)\|}}{\sqrt{N_1N_2}}\phi_M. \end{align*} |
Proof. By Lemma 3.2, we have
\begin{align*} D_{\alpha}^c(\Delta_v^k)& = D_{\alpha}^c(v^k_{1cen}-v^k_{2cen})\\ & = \frac{1}{NN_1}\sum\limits_{i = 1}^{N_1}\sum\limits_{j = 1}^{N_2}\phi(\|x_{1i}-x_{2j}\|)\left({v_{2j}^k}-{v_{1i}^k}\right)+ \frac{1}{NN_2}\sum\limits_{i = 1}^{N_1}\sum\limits_{j = 1}^{N_2}\phi(\|x_{1i}-x_{2j}\|)\left({v_{2j}^k}-{v_{1i}^k}\right)\\ & = -\frac{1}{N_1N_2}\sum\limits_{i = 1}^{N_1}\sum\limits_{j = 1}^{N_2}\phi(\|x_{2j}-x_{1i}\|)(v^k_{1i}-v^k_{2j}). \end{align*} |
Then, we employ Cauchy–Schwarz's inequality and Lemma 2.1 to find
\begin{align*} D_{\alpha}^c(\Delta_v^k) &\geq -\frac{1}{N_1N_2}\phi_M\sum\limits_{i = 1}^{N_1}\sum\limits_{j = 1}^{N_2}|v^k_{1i}-v^k_{2j}|\\ &\geq -\frac{1}{N_1N_2}\phi_M\sum\limits_{i = 1}^{N_1}\sum\limits_{j = 1}^{N_2}(|v^k_{1i}|+|v^k_{2j}|)\\ & = -\frac{1}{N_1N_2}\phi_M\left(N_2\sum\limits_{i = 1}^{N_1}|v^k_{1i}|+N_1\sum\limits_{j = 1}^{N_2}|v^k_{2j}|\right)\\ &\geq-\frac{1}{N_1N_2}\phi_M\left(\sqrt{N_1}N_2{\|V_1\|}+\sqrt{N_2}N_1{\|V_2\|}\right)\\ & = -\frac{1}{\sqrt{N_1N_2}}\phi_M\cdot (\sqrt{N_2}{\|V_1\|}+\sqrt{N_1}{\|V_2\|})\\ &\geq -\frac{\sqrt{N}\|V\|}{\sqrt{N_1N_2}}\phi_M\geq -\frac{\sqrt{N}{\|V(0)\|}}{\sqrt{N_1N_2}}\phi_M. \end{align*} |
Thus, we conclude the desired lemma.
Next, we set
S_1: = \sup\left\{s > 0\; \bigg|\; D_{X(t)}\leq D_X^\infty, \quad t\in [0, s)\right\}, |
S_1^*: = \sup S_1 and
S: = \sup\left\{s > 0\; \bigg|\; \min\limits_{i\in [N_1], j\in [N_2]}\|x_{1i}(t)-x_{2j}(t)\|\geq \frac{\Delta^k_v(0)}{4\Gamma(\alpha+1)}t^\alpha+r_0, \quad t\in [0, s), \quad s\leq S_1^*\right\}, |
S^*: = \sup S . From (\mathcal{F}_2) and the continuity of D_X , we observe that S_1\neq \emptyset and S_1^*\in (0, \infty] . In addition, since (\mathcal{F}_1) yields that
0\leq r_0 < \min\limits_{i\in [N_1], j\in [N_2]}(x_{1i}^k(0)-x_{2j}^k(0))\leq \min\limits_{i\in [N_1], j\in [N_2]}\|x_{1i}(0)-x_{2j}(0)\| |
and \min_{i\in [N_1], j\in [N_2]}\|x_{1i}-x_{2j}\| is continuous, we can obtain that S\neq \emptyset and S^*\in (0, S_1^*] .
Subsequently, for \beta\in [2] , let
\phi_{\beta ij}(t): = \phi(\|x_{\beta j}(t)-x_{\beta i}(t)\|), \quad i, j \in [N_\beta], |
and we employ the following functional:
\Psi_{\beta ij}(t): = \frac{\phi_{\beta ij}(t)}{N_\beta}+\left(1-\frac{\sum\nolimits_{k = 1}^{N_\beta}\phi_{\beta ik}(t)}{N_\beta}\right)\delta_{ij}. |
Then, it is straightforward to check that
\Psi_{\beta ij}\geq \frac{\phi_{\beta ij}}{N_\beta}, \qquad \sum\limits_{j = 1}^{N_\beta}\Psi_{\beta ij} = 1, \qquad \sum\limits_{j = 1}^{N_\beta}\Psi_{\beta ij}(\hat{v}_{\beta j}-\hat{v}_{\beta i}) = \sum\limits_{j = 1}^{N_\beta}\frac{\phi_{\beta ij}}{N_\beta}(\hat{v}_{\beta j}-\hat{v}_{\beta i}). |
Based on the recurrence Eq (2.7) and motivated by the second assertion of Lemma 3.2 with the definitions of \Psi_{\beta ij} , \hat{V}_1 and \hat{V}_2 , we consider the following recurrence relation with respect to \{\hat{V}_\beta^{(i)}\}_{i = 0}^\infty for \beta\in [2] :
\begin{align} \begin{aligned} &\hat{V}_\beta^{(0)} = 0, \quad i\in \mathbb{N}\cup \{0\}, \quad \beta\in [2], \\ &\hat{V}_\beta^{(i+1)} = E_{\alpha, 1}(-t^\alpha)\hat{V}_\beta(0)+\int_{0}^t (t-s)^{\alpha-1}E_{\alpha, \alpha}(-(t-s)^\alpha)\Phi_\beta(X(s)) \hat{V}_\beta^{(i)}(s) ds\\ & \quad \quad\quad\quad\quad+\int_{0}^t (t-s)^{\alpha-1}E_{\alpha, \alpha}(-(t-s)^\alpha)\hat{\mathcal{R}}_\beta\big(X(s), V_\beta^{(i)}(s)\big)ds, \end{aligned} \end{align} | (3.2) |
where \hat{V}_\beta^{(i)}(s)\in \mathbb{R}^{N_\beta\times d} and \Phi_\beta(X(s))\in \mathbb{R}^{N_\beta\times N_\beta} are given by
\begin{equation*} \hat{V}_\beta^{(i)}(s): = \left(v^{(i)}_{\beta 1}-\frac{1}{N_\beta}\sum\limits_{j = 1}^{N_\beta}v^{(i)}_{\beta j}, \ldots, v^{(i)}_{\beta N_\beta}-\frac{1}{N_\beta}\sum\limits_{j = 1}^{N_\beta}v^{(i)}_{\beta j}\right)^T, \quad (\Phi_\beta(X(s)))_{ij}: = \Psi_{\beta ij}(s), \quad i, j\in [N_\beta]. \end{equation*} |
Furthermore, \hat{\mathcal{R}}_\beta\big(X(s), V_\beta^{(i)}(s)\big)\in \mathbb{R}^{N_\beta\times d} is written by
\begin{equation*} \hat{\mathcal{R}}_\beta\big(X(s), V_\beta^{(i)}(s)\big): = \begin{bmatrix} \frac{1}{N}\sum\nolimits_{k = 1}^{N_\gamma}\phi(\|x_{\beta 1}-x_{\gamma k}\|)\left({v^{(i)}_{\gamma k}}-{v^{(i)}_{\beta 1}}\right)^T-\mathcal{Q}_\beta(V^{(i)})\\ \vdots\\ \frac{1}{N}\sum\nolimits_{k = 1}^{N_\gamma}\phi(\|x_{\beta N_\beta}-x_{\gamma k}\|)\left({v^{(i)}_{\gamma k}}-{v^{(i)}_{\beta N_{\beta}}}\right)^T-\mathcal{Q}_\beta(V^{(i)}) \end{bmatrix}, \; \text{where} \quad \gamma\neq \beta, \end{equation*} |
and \mathcal{Q}_\beta(V^{(i)})\in \mathbb{R}^{1\times d} is defined as
\frac{1}{NN_\beta}\sum\limits_{j = 1}^{N_\beta}\sum\limits_{k = 1}^{N_\gamma}\phi(\|x_{\beta j}-x_{\gamma k}\|)\left({v^{(i)}_{\gamma k}}-{v^{(i)}_{\beta j}}\right)^T, \quad \text{where} \quad \gamma\neq \beta. |
Now, we study the uniform boundedness of D_{\hat{V}_1} and D_{\hat{V}_2} .
Lemma 3.4 (Estimates of D_{\hat{V}_1} and D_{\hat{V}_2} ). Assume that (X, V) is the solution of (3.1) such that (\mathcal{F}_1) – (\mathcal{F}_3) hold. Then, for \beta\in [2] and t\in [0, S^*] ,
D_{\hat{V}_\beta}\leq 2D_{\hat{V}_\beta(0)}. |
Proof. We divide the proof into several steps.
● (Step A: Estimate of \mu(\Phi_\beta(X(s))) ) For t\in [0, S^*] , because the following assertions hold:
D_{X(t)}\leq D_X^\infty\quad \text{and} \quad \Psi_{\beta ij}(t)\geq \frac{\phi_{\beta ij}}{N_\beta}, |
it follows from the definitions of the ergodicity coefficient (see Lemma 2.2) and (\Phi_\beta(X(t)))_{ij} and the monotonicity of \phi that, for t\in [0, S^*] ,
\begin{align} \mu\left(\Phi_\beta(X(t))\right)\geq \phi(D_X^\infty), \quad \beta\in [2]. \end{align} | (3.3) |
● (Step B: Recurrence relation) We take a diameter to (3.2) and apply (3.3) and Lemma 2.2 to this to get that, for t\in [0, S^*] ,
\begin{align*} D_{\hat{V}_\beta^{(i+1)}}&\leq E_{\alpha, 1}(-t^\alpha)D_{\hat{V}_\beta(0)}\\ &\qquad +\int_{0}^t (t-s)^{\alpha-1}E_{\alpha, \alpha}(-(t-s)^\alpha)\left[D_{\Phi_\beta(X(s)) \hat{V}_\beta^{(i)}(s)}+D_{\hat{\mathcal{R}}_\beta\big(X(s), V_\beta^{(i)}(s)\big)}\right] ds\\ &\leq E_{\alpha, 1}(-t^\alpha)D_{\hat{V}_\beta(0)}\\ &\qquad+\int_{0}^t (t-s)^{\alpha-1}E_{\alpha, \alpha}(-(t-s)^\alpha)\left[(1-\phi(D_X^\infty))D_{\hat{V}_\beta^{(i)}(s)}+D_{\hat{\mathcal{R}}_\beta\big(X(s), V_\beta^{(i)}(s)\big)}\right] ds. \end{align*} |
To estimate D_{\hat{\mathcal{R}}_\beta\big(X(s), V_\beta^{(i)}(s)\big)} , we note that, for j\in [N_\beta] and \gamma\neq \beta ,
\left\|\frac{1}{N}\sum\limits_{k = 1}^{N_\gamma}\phi(\|x_{\beta j}-x_{\gamma k}\|)\left({v^{(i)}_{\gamma k}}-{v^{(i)}_{\beta j}}\right)^T \right\|\leq \frac{\sqrt{2}N_\gamma \|V(0)\|}{N}\phi_M, |
where we used the proof of Lemma 2.1 to employ that
\begin{align} \begin{aligned} \|{v^{(i)}_{\gamma k}}-{v^{(i)}_{\beta j}}\|\leq \sqrt{2}\|V^{(i)}\|\leq \sqrt{2}\|V(0)\|. \end{aligned} \end{align} | (3.4) |
Then, D_{\hat{\mathcal{R}}_\beta\big(X(s), V_\beta^{(i)}(s)\big)} can be estimated as follows:
\begin{align*} D_{\hat{\mathcal{R}}_\beta\big(X(s), V_\beta^{(i)}(s)\big)}\leq \frac{2\sqrt{2}N_\gamma \|V(0)\|}{N}\phi_M. \end{align*} |
Thus, we combine the above relations and Proposition 2.3 to obtain that, for t\in [0, S^*] ,
\begin{align*} D_{\hat{V}_\beta^{(i+1)}} &\leq E_{\alpha, 1}(-t^\alpha)D_{\hat{V}_\beta(0)}\\ &\qquad +\int_{0}^t (t-s)^{\alpha-1}E_{\alpha, \alpha}(-(t-s)^\alpha)(1-\phi(D_X^\infty))D_{\hat{V}_\beta^{(i)}(s)} ds\\ &\qquad + \frac{2\sqrt{2}N_\gamma \|V(0)\|}{N}\int_{0}^t (t-s)^{\alpha-1}E_{\alpha, \alpha}(-(t-s)^\alpha)\phi_M(s) ds\\ &\leq E_{\alpha, 1}(-t^\alpha)D_{\hat{V}_\beta(0)}\\ &\qquad +\int_{0}^t (t-s)^{\alpha-1}E_{\alpha, \alpha}(-(t-s)^\alpha)(1-\phi(D_X^\infty))D_{\hat{V}_\beta^{(i)}(s)} ds\\ &\qquad + \frac{2\sqrt{2}N_\gamma \|V(0)\|}{N}\sup\limits_{t\in \mathbb{R}_+}\int_{0}^t (t-s)^{\alpha-1}\phi\left(\frac{\Delta^k_v(0)}{4\Gamma(\alpha+1)}s^\alpha+r_0\right) ds. \end{align*} |
From the above estimate, we construct the following recurrence relation for \{\hat{W}^{(i)}\}_{i = 0}^\infty :
\begin{align} \begin{aligned} &\hat{W}^{(0)} = 0, \quad i\in \mathbb{N}\cup \{0\}, \\ &\hat{W}^{(i+1)} = E_{\alpha, 1}(-t^\alpha)D_{\hat{V}_\beta(0)}+\int_{0}^t (t-s)^{\alpha-1}E_{\alpha, \alpha}(-(t-s)^\alpha)(1-\phi(D_X^\infty)){\hat{W}^{(i)}(s)}ds\\ &\qquad\qquad+ \frac{2\sqrt{2}N_\gamma \|V(0)\|}{N}\sup\limits_{t\in \mathbb{R}_+}\int_{0}^t (t-s)^{\alpha-1}\phi\left(\frac{\Delta^k_v(0)}{4\Gamma(\alpha+1)}s^\alpha+r_0\right) ds. \end{aligned} \end{align} | (3.5) |
In (3.5), we can immediately know that, for each i\in \mathbb{N}\cup\{0\} ,
D_{\hat{V}_\beta^{(i)}}\leq \hat{W}^{(i)}. |
● (Step C: Iterative method) We claim that
\begin{align} \begin{aligned} &|\hat{W}^{(i+1)}-\hat{W}^{(i)}|\\ &\leq D_{\hat{V}_\beta(0)}\left(1-\phi(D_X^\infty)\right)^{i}\sum\limits_{j = 0}^\infty \left(\frac{{}_{(j+i)}C_{j}\cdot(-1)^j}{\Gamma(\alpha (j+i)+1)}\right)\cdot t^{\alpha (j+i)}\\ &\quad+ \frac{2\sqrt{2}N_\gamma \|V(0)\|(1-\phi(D_X^\infty))^i}{N}\sup\limits_{t\in \mathbb{R}_+}\int_{0}^t (t-s)^{\alpha-1}\phi\left(\frac{\Delta^k_v(0)}{4\Gamma(\alpha+1)}s^\alpha+r_0\right) ds\\ & = D_{\hat{V}_\beta(0)}\left(-(1-\phi(D_X^\infty))\right)^{i}\sum\limits_{j = i}^\infty \left(\frac{{}_{j}C_{i}\cdot(-1)^j}{\Gamma(\alpha j+1)}\right)\cdot t^{\alpha j}\\ &\quad+ \frac{2\sqrt{2}N_\gamma \|V(0)\|(1-\phi(D_X^\infty))^i}{N}\sup\limits_{t\in \mathbb{R}_+}\int_{0}^t (t-s)^{\alpha-1}\phi\left(\frac{\Delta^k_v(0)}{4\Gamma(\alpha+1)}s^\alpha+r_0\right) ds. \end{aligned} \end{align} | (3.6) |
Proof of (3.6). We use induction to prove the desired claim.
\diamond\; (\mbox{The case of}\; i = 0) : From Definition 2.3 and (3.5), we have that
\begin{align*} &|\hat{W}^{(1)}-\hat{W}^{(0)}|\\ & = D_{\hat{V}_\beta(0)}E_{\alpha, 1}(-t^\alpha)+\frac{2\sqrt{2}N_\gamma \|V(0)\|}{N} \sup\limits_{t\in \mathbb{R}_+}\int_{0}^t (t-s)^{\alpha-1}\phi\left(\frac{\Delta^k_v(0)}{4\Gamma(\alpha+1)}s^\alpha+r_0\right) ds\\ & = D_{\hat{V}_\beta(0)}\sum\limits_{j = 0}^\infty \left(\frac{(-1)^j}{\Gamma(\alpha j+1)}\right)\cdot t^{\alpha j}+ \frac{2\sqrt{2}N_\gamma \|V(0)\|}{N}\sup\limits_{t\in \mathbb{R}_+}\int_{0}^t (t-s)^{\alpha-1}\phi\left(\frac{\Delta^k_v(0)}{4\Gamma(\alpha+1)}s^\alpha+r_0\right). \end{align*} |
\diamond\; (\mbox{The case of}\; i > 0) : Suppose that the desired assertion holds for 0\leq k\leq i , and we obtain from (3.5) that, for i\in \mathbb{N}\cup\{0\} ,
\begin{align*} \begin{aligned} &|\hat{W}^{(i+2)}-\hat{W}^{(i+1)}|\\ &\leq D_{\hat{V}_\beta(0)}\left(1-\phi(D_X^\infty)\right)^{i+1}\int_{0}^t (t-s)^{\alpha-1} E_{\alpha, \alpha}(-(t-s)^{\alpha})\sum\limits_{j = 0}^\infty\left(\frac{{}_{(j+i)}C_{j}\cdot(-1)^j}{\Gamma(\alpha(j+i)+1)}\right)\cdot s^{\alpha (j+i)}ds\\ &\quad+ \frac{2\sqrt{2}N_\gamma \|V(0)\|(1-\phi(D_X^\infty))^{i+1}}{N}\sup\limits_{t\in \mathbb{R}_+}\int_{0}^t (t-s)^{\alpha-1} \phi\left(\frac{\Delta^k_v(0)}{4\Gamma(\alpha+1)}s^\alpha+r_0\right) ds, \end{aligned} \end{align*} |
where we applied the following relation induced by the first and third assertions of Propositions 2.1 and 2.3:
\begin{align*} \int_{0}^{t} (t-s)^{\alpha-1}E_{\alpha, \alpha}(-(t-s)^\alpha) ds = E_{\alpha, \alpha+1}(-t^\alpha)t^\alpha = 1-E_{\alpha, 1}(-t^\alpha)\leq 1. \end{align*} |
Next, if we employ the same methods as (2.9) and (2.10), then we estimate that
\begin{align*} &|\hat{W}^{(i+2)}-\hat{W}^{(i+1)}|\\ &\leq D_{\hat{V}_\beta(0)}\left(1-\phi(D_X^\infty)\right)^{i+1}\sum\limits_{j = 0}^\infty \left(\frac{{}_{(j+i+1)}C_{j}\cdot(-1)^j}{\Gamma(\alpha (j+i+1)+1)}\right)\cdot t^{\alpha (j+i+1)}\\ &\quad+ \frac{2\sqrt{2}N_\gamma \|V(0)\|(1-\phi(D_X^\infty))^{i+1}}{N}\sup\limits_{t\in \mathbb{R}_+}\int_{0}^t (t-s)^{\alpha-1}\phi\left(\frac{\Delta^k_v(0)}{4\Gamma(\alpha+1)}s^\alpha+r_0\right) ds. \end{align*} |
Hence, we reach the desired claim.
● (Step D: Desired result) Using Lemma 2.1 and (3.6) yields that
\begin{align*} \begin{aligned} D_{\hat{V}_\beta}& = \lim\limits_{i\to\infty}D_{\hat{V}_\beta^{(i)}}\leq \lim\limits_{i\to\infty} \hat{W}^{(i)}\leq \sum\limits_{i = 0}^\infty|\hat{W}^{(i+1)}-\hat{W}^{(i)}|\\ &\leq D_{\hat{V}_\beta(0)}\sum\limits_{i = 0}^\infty\sum\limits_{j = i}^\infty \left(-(1-\phi(D_X^\infty))\right)^{i} \left(\frac{{}_{j}C_{i}\cdot(-1)^j}{\Gamma(\alpha j+1)}\right)\cdot t^{\alpha j}\\ &\qquad+ \frac{2\sqrt{2}N_\gamma \|V(0)\|}{N\phi(D_X^\infty)}\sup\limits_{t\in \mathbb{R}_+}\int_{0}^t (t-s)^{\alpha-1}\phi\left(\frac{\Delta^k_v(0)}{4\Gamma(\alpha+1)}s^\alpha+r_0\right) ds. \end{aligned} \end{align*} |
Because
\begin{align} \begin{aligned} &\sum\limits_{i = 0}^\infty\sum\limits_{j = i}^\infty \left(-(1-\phi(D_X^\infty))\right)^{i} \left(\frac{{}_{j}C_{i}\cdot(-1)^j}{\Gamma(\alpha j+1)}\right)\cdot t^{\alpha j}\\ & = \sum\limits_{j = 0}^\infty \frac{\left(-1\right)^jt^{\alpha j}}{\Gamma(\alpha j+1)} \sum\limits_{i = 0}^j \left(-(1-\phi(D_X^\infty))\right)^{i}{}_{j}C_{i}\\ & = \sum\limits_{j = 0}^\infty \frac{\left(-1\right)^jt^{\alpha j}}{\Gamma(\alpha j+1)} \left(-(1-\phi(D_X^\infty))+1\right)^j\\ & = \sum\limits_{j = 0}^\infty \frac{\left(-1\right)^jt^{\alpha j}}{\Gamma(\alpha j+1)}\cdot\frac{(-\phi(D_X^\infty))^j}{(-1)^j} = E_{\alpha, 1}\left(-\phi(D_X^\infty) t^\alpha\right), \end{aligned} \end{align} | (3.7) |
it follows from Proposition 2.3 and (\mathcal{F}_3) that, for t\in [0, S^*] ,
\begin{align*} \begin{aligned} D_{\hat{V}_\beta} &\leq D_{\hat{V}_\beta(0)}E_{\alpha, 1}\left(-\phi(D_X^\infty) t^\alpha\right)\\ &\qquad+ \frac{2\sqrt{2}N_\gamma \|V(0)\|}{N\phi(D_X^\infty)}\sup\limits_{t\in \mathbb{R}_+}\int_{0}^t (t-s)^{\alpha-1}\phi\left(\frac{\Delta^k_v(0)}{4\Gamma(\alpha+1)}s^\alpha+r_0\right) ds\\ &\leq D_{\hat{V}_\beta(0)}+\frac{2\sqrt{2}N_\gamma \|V(0)\|}{N\phi(D_X^\infty)}\sup\limits_{t\in \mathbb{R}_+}\int_{0}^t (t-s)^{\alpha-1}\phi\left(\frac{\Delta^k_v(0)}{4\Gamma(\alpha+1)}s^\alpha+r_0\right) ds\leq 2D_{\hat{V}_\beta(0)}. \end{aligned} \end{align*} |
Finally, we conclude the desired lemma.
From Lemmas 3.3 and 3.4, we can show that \min_{i\in [N_1], j\in [N_2]}\|x_{1i}-x_{2j}\| is greater than a function that increases proportionally to t^\alpha under (\mathcal{F}_1) – (\mathcal{F}_3) .
Lemma 3.5. [Estimate of \min_{i\in [N_1], j\in [N_2]}\|x_{1i}-x_{2j}\| ] Assume that (X(0), V(0)) satisfies (\mathcal{F}_1) – (\mathcal{F}_3) , and let (X, V) be the solution of (3.1). Then, we can derive S^* = S_1^* , which leads to
\begin{align*} \min\limits_{i\in [N_1], j\in [N_2]}\|x_{1i}(t)-x_{2j}(t)\|\geq \frac{\Delta^k_v(0)}{4\Gamma(\alpha+1)}t^\alpha+r_0, \quad t\in [0, S_1^*]. \end{align*} |
Proof. For the proof by contradiction, suppose that S^* < S_1^* . Then, we notice that
\min\limits_{i\in [N_1], j\in [N_2]}\|x_{1i}(S^*)-x_{2j}(S^*)\| = \frac{\Delta^k_v(0)}{4\Gamma(\alpha+1)}(S^*)^\alpha+r_0. |
Thus, by the definition of \phi_M(t) and the monotonicity of \phi , we attain that, for t\in[0, S^*] ,
\phi_M(t)\leq \phi\left(\frac{\Delta^k_v(0)}{4\Gamma(\alpha+1)}t^\alpha+r_0\right). |
This relation and Lemme 3.3 induce that, for t\in[0, S^*] ,
\begin{align*} D_\alpha^c(\Delta_v^k) \geq -\frac{\sqrt{N}{\|V(0)\|}}{\sqrt{N_1N_2}}\phi_M\geq -\frac{\sqrt{N}{\|V(0)\|}}{\sqrt{N_1N_2}}\phi\left(\frac{\Delta^k_v(0)}{4\Gamma(\alpha+1)}t^\alpha+r_0\right). \end{align*} |
If we apply Proposition 2.6 and (2.2) to the above inequality, then we can estimate that, for t\in [0, S^*] ,
\Delta_v^k(t) \geq\Delta_v^k(0)-\frac{\sqrt{N}{\|V(0)\|}}{\sqrt{N_1N_2}\Gamma(\alpha)}\int_{0}^{t}\frac{\phi\left(\frac{\Delta^k_v(0)}{4\Gamma(\alpha+1)}s^\alpha+r_0\right)}{(t-s)^{1-\alpha}}ds. |
Using (\mathcal{F}_3) implies that, for t\in [0, S^*] ,
\begin{align*} \Delta_v^k(t) &\geq\Delta_v^k(0)-\frac{\sqrt{N}{\|V(0)\|}}{\sqrt{N_1N_2}\Gamma(\alpha)}\int_{0}^{t}\frac{\phi\left(\frac{\Delta^k_v(0)}{4\Gamma(\alpha+1)}s^\alpha+r_0\right)}{(t-s)^{1-\alpha}}ds\\ &\geq \Delta_v^k(0)-\frac{\sqrt{N}{\|V(0)\|}}{\sqrt{N_1N_2}\Gamma(\alpha)}\sup\limits_{t\in \mathbb{R}_+}\left(\int_{0}^{t}\frac{\phi\left(\frac{\Delta^k_v(0)}{4\Gamma(\alpha+1)}s^\alpha+r_0\right)}{(t-s)^{1-\alpha}}ds\right)\\ &\geq \Delta_v^k(0)-2\sqrt{2(N_1-1)}D_{\hat{V}_1(0)}-2\sqrt{2(N_2-1)}D_{\hat{V}_2(0)}\geq \frac{\Delta^k_v(0)}{2}. \end{align*} |
Therefore, from the above outcome, (\mathcal{F}_3) , and Lemma 3.4, we deduce that, for t\in [0, S^*] ,
\begin{align*} v^k_{1i}(t)-v^k_{2j}(t)& = \Delta_v^k(t)+\hat{v}^k_{1i}-\hat{v}^k_{2j}\geq \Delta_v^k(t)-|\hat{v}^k_{1i}|-|\hat{v}^k_{2j}|\\ &\geq \frac{\Delta^k_v(0)}{2}-\sqrt{\frac{N_1-1}{2}}D_{\hat{V}_1(t)}-\sqrt{\frac{N_2-1}{2}}D_{\hat{V}_2(t)}\\ &\geq \frac{\Delta^k_v(0)}{2}-\sqrt{2(N_1-1)}D_{\hat{V}_1(0)}-\sqrt{2(N_2-1)}D_{\hat{V}_2(0)}\\ & > \frac{\Delta^k_v(0)}{4}, \end{align*} |
where we applied the below relations:
|\hat{v}^k_{1i}|^2\leq \sum\limits_{i = 1}^{N_1}\|\hat{v}_{1i}\|^2 = \frac{1}{2N_1}\sum\limits_{i\neq j, i, j = 1}^{N_1}\|\hat{v}_{1i}-\hat{v}_{1j}\|^2\leq \frac{(N_1-1)D^2_{\hat{V}_1}}{2}. |
Because of the above relation, the first assertion of Remark 2.1, (\mathcal{F}_1) , (1.2)_1 , and (2.2), we reach the following inequality:
\begin{align*} \|x_{1i}(S^*)-x_{2j}(S^*)\|&\geq x_{1i}^k(S^*)-x_{2j}^k(S^*)\\ & = x_{1i}^k(0)-x_{2j}^k(0)+\frac{1}{\Gamma(\alpha)}\int_{0}^{S^*}\frac{(v_{1i}^k(s)-v_{2j}^k(s))}{(S^*-s)^{1-\alpha}}ds\\ & > r_0+\frac{\Delta^k_v(0)}{4\Gamma(\alpha)}\int_{0}^{S^*}\frac{1}{(S^*-s)^{1-\alpha}}ds\\ & = r_0+\frac{\Delta^k_v(0)}{4\Gamma(\alpha+1)}(S^*)^\alpha, \end{align*} |
which reveals that
\min\limits_{i\in [N_1], j\in [N_2]}\|x_{1i}(S^*)-x_{2j}(S^*)\| > \frac{\Delta^k_v(0)}{4\Gamma(\alpha+1)}(S^*)^\alpha+r_0. |
This results in a contradiction. Accordingly, S^* = S_1^* , and we prove the desired lemma.
In what follows, we study the velocity alignment estimate for each cluster group based on the iterative method dealt with in Section 2.2. For \beta\in [2] , we recall
\phi_{\beta ij}(t): = \phi(\|x_{\beta j}(t)-x_{\beta i}(t)\|), \quad i, j \in [N_\beta], |
and again employ the following functional:
\Psi_{\beta ij}(t): = \frac{\phi_{\beta ij}(t)}{N_\beta}+\left(1-\frac{\sum\nolimits_{k = 1}^{N_\beta}\phi_{\beta ik}(t)}{N_\beta}\right)\delta_{ij}. |
Then, we remind that
\Psi_{\beta ij}\geq \frac{\phi_{\beta ij}}{N_\beta}, \qquad \sum\limits_{j = 1}^{N_\beta}\Psi_{\beta ij} = 1, |
\sum\limits_{j = 1}^{N_\beta}\Psi_{\beta ij}(v_{\beta j}-v_{\beta i}) = \sum\limits_{j = 1}^{N_\beta}\frac{\phi_{\beta ij}}{N_\beta}(v_{\beta j}-v_{\beta i}). |
From the recurrence Eq (2.7) and \Psi_{\beta ij} with V_1 and V_2 defined in Section 3.1, we consider the following subrecurrence relation \{V_\beta^{(i)}\}_{i = 0}^\infty for \beta\in [2] :
\begin{align} \begin{aligned} &V_\beta^{(0)} = 0, \quad i\in \mathbb{N}\cup \{0\}, \quad \beta\in [2], \\ &V_\beta^{(i+1)} = E_{\alpha, 1}(-t^\alpha)V_\beta(0)+\int_{0}^t (t-s)^{\alpha-1}E_{\alpha, \alpha}(-(t-s)^\alpha)\Phi_\beta(X(s)) V_\beta^{(i)}(s) ds\\ & \quad \quad\quad\quad\quad+\int_{0}^t (t-s)^{\alpha-1}E_{\alpha, \alpha}(-(t-s)^\alpha)\mathcal{R}_\beta\big(X(s), V_\beta^{(i)}(s)\big)ds, \end{aligned} \end{align} | (3.8) |
where V_\beta^{(i)}(s)\in \mathbb{R}^{N_\beta\times d} , \Phi_\beta(X(s))\in \mathbb{R}^{N_\beta\times N_\beta} is given by
\begin{equation*} (\Phi_\beta(X(s)))_{ij}: = \Psi_{\beta ij}(s)\quad \text{for}\quad i, j\in [N_\beta], \end{equation*} |
and \mathcal{R}_\beta\big(X(s), V_\beta^{(i)}(s)\big)\in \mathbb{R}^{N_\beta\times d} is defined by
\begin{equation*} \mathcal{R}_\beta\big(X(s), V_\beta^{(i)}(s)\big): = \begin{bmatrix} \frac{1}{N}\sum\nolimits_{k = 1}^{N_\gamma}\phi(\|x_{\beta 1}-x_{\gamma k}\|)\left({v^{(i)}_{\gamma k}}-{v^{(i)}_{\beta 1}}\right)^T \\ \vdots\\ \frac{1}{N}\sum\nolimits_{k = 1}^{N_\gamma}\phi(\|x_{\beta N_\beta}-x_{\gamma k}\|)\left({v^{(i)}_{\gamma k}}-{v^{(i)}_{\beta N_{\beta}}}\right)^T \end{bmatrix}, \quad \text{where}\quad \gamma\neq \beta. \end{equation*} |
Next, for t\in [0, S_1^*] , we note that (3.3) holds in this case as follows:
\begin{align} \mu\left(\Phi_\beta(X(t))\right)\geq \phi(D_X^\infty), \quad \beta\in [n]. \end{align} | (3.9) |
Taking a diameter to (3.8), and then applying (3.9) and Lemma 2.2 to this derives that, for t\in [0, S_1^*] ,
\begin{align*} D_{V_\beta^{(i+1)}}&\leq E_{\alpha, 1}(-t^\alpha)D_{V_\beta(0)}\\ &\qquad +\int_{0}^t (t-s)^{\alpha-1}E_{\alpha, \alpha}(-(t-s)^\alpha)\left[D_{\Phi_\beta(X(s)) V_\beta^{(i)}(s)}+D_{\mathcal{R}_\beta\big(X(s), V_\beta^{(i)}(s)\big)}\right] ds\\ &\leq E_{\alpha, 1}(-t^\alpha)D_{V_\beta(0)}\\ &\qquad+\int_{0}^t (t-s)^{\alpha-1}E_{\alpha, \alpha}(-(t-s)^\alpha)\left[(1-\phi(D_X^\infty))D_{V_\beta^{(i)}(s)}+D_{\mathcal{R}_\beta\big(X(s), V_\beta^{(i)}(s)\big)}\right] ds. \end{align*} |
To estimate D_{\mathcal{R}_\beta\big(X(s), V_\beta^{(i)}(s)\big)} , we observe from (3.4) that, for j\in [N_\beta] ,
\left\|\frac{1}{N}\sum\limits_{k = 1}^{N_\gamma}\phi(\|x_{\beta j}-x_{\gamma k}\|)\left({v^{(i)}_{\gamma k}}-{v^{(i)}_{\beta j}}\right)^T \right\|\leq \frac{\sqrt{2}N_\gamma \|V(0)\|}{N}\phi_M. |
Then, D_{\mathcal{R}_\beta\big(X(s), V_\beta^{(i)}(s)\big)} can be estimated as follows:
\begin{align} D_{\mathcal{R}_\beta\big(X(s), V_\beta^{(i)}(s)\big)}\leq \frac{2\sqrt{2}N_\gamma \|V(0)\|}{N}\phi_M. \end{align} | (3.10) |
Hence, combining the above outcomes implies that, for t\in [0, S_1^*] ,
\begin{align*} D_{V_\beta^{(i+1)}} &\leq E_{\alpha, 1}(-t^\alpha)D_{V_\beta(0)}\\ &\qquad +\int_{0}^t (t-s)^{\alpha-1}E_{\alpha, \alpha}(-(t-s)^\alpha)(1-\phi(D_X^\infty))D_{V_\beta^{(i)}(s)} ds\\ &\qquad + \frac{2\sqrt{2}N_\gamma \|V(0)\|}{N}\int_{0}^t (t-s)^{\alpha-1}E_{\alpha, \alpha}(-(t-s)^\alpha)\phi_M(s) ds. \end{align*} |
Therefore, we have from Proposition 2.3 and Lemma 3.5 that, for t\in [0, S_1^*] ,
\begin{align} \begin{aligned} D_{V_\beta^{(i+1)}} &\leq E_{\alpha, 1}(-t^\alpha)D_{V_\beta(0)}+\int_{0}^t (t-s)^{\alpha-1}E_{\alpha, \alpha}(-(t-s)^\alpha)(1-\phi(D_X^\infty))D_{V_\beta^{(i)}(s)} ds\\ &\qquad + \frac{2\sqrt{2}N_\gamma \|V(0)\|}{N}\int_{0}^t (t-s)^{\alpha-1}E_{\alpha, \alpha}(-(t-s)^\alpha)\phi\left(\frac{\Delta^k_v(0)}{4\Gamma(\alpha+1)}s^\alpha+r_0\right) ds\\ & = E_{\alpha, 1}(-t^\alpha)D_{V_\beta(0)}+\int_{0}^t (t-s)^{\alpha-1}E_{\alpha, \alpha}(-(t-s)^\alpha)(1-\phi(D_X^\infty))D_{V_\beta^{(i)}(s)} ds\\ &\qquad + \frac{2\sqrt{2}N_\gamma \|V(0)\|}{N}\int_{0}^{\frac{t}{2}} (t-s)^{\alpha-1}E_{\alpha, \alpha}(-(t-s)^\alpha)\phi\left(\frac{\Delta^k_v(0)}{4\Gamma(\alpha+1)}s^\alpha+r_0\right) ds\\ &\qquad + \frac{2\sqrt{2}N_\gamma \|V(0)\|}{N}\int_{\frac{t}{2}}^{t} (t-s)^{\alpha-1}E_{\alpha, \alpha}(-(t-s)^\alpha)\phi\left(\frac{\Delta^k_v(0)}{4\Gamma(\alpha+1)}s^\alpha+r_0\right) ds \\ &\leq E_{\alpha, 1}(-t^\alpha)D_{V_\beta(0)}+\int_{0}^t (t-s)^{\alpha-1}E_{\alpha, \alpha}(-(t-s)^\alpha)(1-\phi(D_X^\infty))D_{V_\beta^{(i)}(s)} ds\\ &\qquad + \frac{2\sqrt{2}N_\gamma \|V(0)\|}{N}E_{\alpha, \alpha}\left(-\left(\frac{t}{2}\right)^\alpha\right)\left(\frac{t}{2}\right)^{\alpha-1}\int_{0}^{\frac{t}{2}} \phi\left(\frac{\Delta^k_v(0)}{4\Gamma(\alpha+1)}s^\alpha+r_0\right) ds\\ &\qquad + \frac{2\sqrt{2}N_\gamma \|V(0)\|}{N}\phi\left(\frac{\Delta^k_v(0)}{4\Gamma(\alpha+1)}\left(\frac{t}{2}\right)^\alpha+r_0\right)\int_{\frac{t}{2}}^{t} (t-s)^{\alpha-1}E_{\alpha, \alpha}(-(t-s)^\alpha) ds, \end{aligned} \end{align} | (3.11) |
which leads to
\begin{align} \begin{aligned} D_{V_\beta^{(i+1)}} &\leq E_{\alpha, 1}(-t^\alpha)D_{V_\beta(0)}+\int_{0}^t (t-s)^{\alpha-1}E_{\alpha, \alpha}(-(t-s)^\alpha)(1-\phi(D_X^\infty))D_{V_\beta^{(i)}(s)} ds\\ &\qquad + \frac{2\sqrt{2}N_\gamma \|V(0)\|}{N}E_{\alpha, \alpha}\left(-\left(\frac{t}{2}\right)^\alpha\right)\left(\frac{t}{2}\right)^{\alpha-1}\int_{0}^{\infty} \phi\left(\frac{\Delta^k_v(0)}{4\Gamma(\alpha+1)}s^\alpha+r_0\right) ds\\ &\qquad + \frac{2\sqrt{2}N_\gamma \|V(0)\|}{N}\phi\left(\frac{\Delta^k_v(0)}{4\Gamma(\alpha+1)}\left(\frac{t}{2}\right)^\alpha+r_0\right)\int_{0}^{t} (t-s)^{\alpha-1}E_{\alpha, \alpha}(-(t-s)^\alpha) ds. \end{aligned} \end{align} | (3.12) |
Since the first and third assertions of Proposition 2.1 yield that
\begin{align} \int_{0}^{t} (t-s)^{\alpha-1}E_{\alpha, \alpha}(-(t-s)^\alpha) ds = E_{\alpha, \alpha+1}(-t^\alpha)t^\alpha = 1-E_{\alpha, 1}(-t^\alpha)\leq 1, \end{align} | (3.13) |
for t\in [0, S_1^*] , one has
\begin{align*} D_{V_\beta^{(i+1)}}&\leq E_{\alpha, 1}(-t^\alpha)D_{V_\beta(0)}+\int_{0}^t (t-s)^{\alpha-1}E_{\alpha, \alpha}(-(t-s)^\alpha)(1-\phi(D_X^\infty))D_{V_\beta^{(i)}(s)} ds\\ &\qquad + \frac{2\sqrt{2}N_\gamma \|V(0)\|}{N}E_{\alpha, \alpha}\left(-\left(\frac{t}{2}\right)^\alpha\right)\left(\frac{t}{2}\right)^{\alpha-1}\int_{0}^{\infty} \phi\left(\frac{\Delta^k_v(0)}{4\Gamma(\alpha+1)}s^\alpha+r_0\right) ds\\ &\qquad + \frac{2\sqrt{2}N_\gamma \|V(0)\|}{N}\phi\left(\frac{\Delta^k_v(0)}{4\Gamma(\alpha+1)}\left(\frac{t}{2}\right)^\alpha+r_0\right). \end{align*} |
Now, we investigate D_{V_\beta^{(i)}} to demonstrate the velocity alignment of each cluster group. To do this, we address the following recurrence relation in terms of \{W^{(i)}\}_{i = 0}^\infty :
\begin{align} \begin{aligned} &W^{(0)} = 0, \quad i\in \mathbb{N}\cup \{0\}, \\ &W^{(i+1)} = E_{\alpha, 1}(-t^\alpha)D_{V_\beta(0)}+\int_{0}^t (t-s)^{\alpha-1}E_{\alpha, \alpha}(-(t-s)^\alpha)(1-\phi(D_X^\infty)){W^{(i)}(s)}ds\\ &\qquad\qquad+ \frac{2\sqrt{2}N_\gamma \|V(0)\|}{N}E_{\alpha, \alpha}\left(-\left(\frac{t}{2}\right)^\alpha\right)\left(\frac{t}{2}\right)^{\alpha-1}\int_{0}^{\infty} \phi\left(\frac{\Delta^k_v(0)}{4\Gamma(\alpha+1)}s^\alpha+r_0\right) ds\\ &\qquad \qquad + \frac{2\sqrt{2}N_\gamma \|V(0)\|}{N}\phi\left(\frac{\Delta^k_v(0)}{4\Gamma(\alpha+1)}\left(\frac{t}{2}\right)^\alpha+r_0\right). \end{aligned} \end{align} | (3.14) |
In (3.14), we can easily check that, for each i\in \mathbb{N}\cup\{0\} ,
D_{V_\beta^{(i)}}\leq W^{(i)}. |
Next, we estimate |W^{(i+1)}-W^{(i)}| for each i\in \mathbb{N}\cup \{0\} to deduce the decay rate of D_{V_\beta} .
Lemma 3.6 (Estimate of |W^{(i+1)}-W^{(i)}| ). In the recurrence relation (3.14), the following assertion holds for i\in \mathbb{N}\cup\{0\} and t\in [0, S_1^*] :
\begin{align*} |W^{(i+1)}-W^{(i)}| &\leq D_{V_\beta(0)}\left(1-\phi(D_X^\infty)\right)^{i}\sum\limits_{j = 0}^\infty \left(\frac{{}_{(j+i)}C_{j}\cdot(-1)^j}{\Gamma(\alpha (j+i)+1)}\right)\cdot t^{\alpha (j+i)}\\ &\quad+ \frac{2\sqrt{2}N_\gamma \|V(0)\|(1-\phi(D_X^\infty))^i}{N}E_{\alpha, \alpha}\left(-\left(\frac{t}{2}\right)^\alpha\right)\left(\frac{t}{2}\right)^{\alpha-1}\int_{0}^{\infty} {\phi\left(\frac{\Delta^k_v(0)}{4\Gamma(\alpha+1)}s^\alpha+r_0\right)} ds\\ &\quad + \frac{2\sqrt{2}N_\gamma \|V(0)\|(1-\phi(D_X^\infty))^i}{N}\phi\left(\frac{\Delta^k_v(0)}{4\Gamma(\alpha+1)}\left(\frac{t}{2}\right)^\alpha+r_0\right)\\ & = D_{V_\beta(0)}\left(-(1-\phi(D_X^\infty))\right)^{i}\sum\limits_{j = i}^\infty \left(\frac{{}_{j}C_{i}\cdot(-1)^j}{\Gamma(\alpha j+1)}\right)\cdot t^{\alpha j}\\ &\quad+ \frac{2\sqrt{2}N_\gamma \|V(0)\|(1-\phi(D_X^\infty))^i}{N}E_{\alpha, \alpha}\left(-\left(\frac{t}{2}\right)^\alpha\right)\left(\frac{t}{2}\right)^{\alpha-1}\int_{0}^{\infty} {\phi\left(\frac{\Delta^k_v(0)}{4\Gamma(\alpha+1)}s^\alpha+r_0\right)} ds\\ &\quad + \frac{2\sqrt{2}N_\gamma \|V(0)\|(1-\phi(D_X^\infty))^i}{N}\phi\left(\frac{\Delta^k_v(0)}{4\Gamma(\alpha+1)}\left(\frac{t}{2}\right)^\alpha+r_0\right). \end{align*} |
Proof. We employ an inductive method to show the desired lemma.
● (\mbox{The case of}\; i = 0) : From (3.14), we directly attain that
\begin{align*} |W^{(1)}-W^{(0)}| & = D_{V_\beta(0)}E_{\alpha, 1}(-t^\alpha)\\ &\qquad+ \frac{2\sqrt{2}N_\gamma \|V(0)\|}{N}E_{\alpha, \alpha}\left(-\left(\frac{t}{2}\right)^\alpha\right)\left(\frac{t}{2}\right)^{\alpha-1}\int_{0}^{\infty} {\phi\left(\frac{\Delta^k_v(0)}{4\Gamma(\alpha+1)}s^\alpha+r_0\right)} ds\\ &\qquad + \frac{2\sqrt{2}N_\gamma \|V(0)\|}{N}\phi\left(\frac{\Delta^k_v(0)}{4\Gamma(\alpha+1)}\left(\frac{t}{2}\right)^\alpha+r_0\right)\\ & = D_{V_\beta(0)}\sum\limits_{j = 0}^\infty \left(\frac{(-1)^j}{\Gamma(\alpha j+1)}\right)\cdot t^{\alpha j} \\ &\qquad +\frac{2\sqrt{2}N_\gamma \|V(0)\|}{N}E_{\alpha, \alpha}\left(-\left(\frac{t}{2}\right)^\alpha\right)\left(\frac{t}{2}\right)^{\alpha-1}\int_{0}^{\infty} {\phi\left(\frac{\Delta^k_v(0)}{4\Gamma(\alpha+1)}s^\alpha+r_0\right)} ds\\ &\qquad + \frac{2\sqrt{2}N_\gamma \|V(0)\|}{N}\phi\left(\frac{\Delta^k_v(0)}{4\Gamma(\alpha+1)}\left(\frac{t}{2}\right)^\alpha+r_0\right). \end{align*} |
Then, we reach the desired lemma when i = 0 .
● (\mbox{The case of}\; i > 0) : Suppose that the desired assertion holds for 0\leq k\leq i , and we note from (3.14) that, for i\in \mathbb{N}\cup\{0\} ,
\begin{align*} |W^{(i+2)}-W^{(i+1)}|\leq \int_{0}^t (t-s)^{\alpha-1}E_{\alpha, \alpha}(-(t-s)^\alpha)(1-\phi(D_X^\infty))|W^{(i+1)}(s)-{W^{(i)}(s)}|ds. \end{align*} |
We employ the inductive assumption, Proposition 2.1, Definition 2.3, and (3.13) to estimate that
\begin{align*} |W^{(i+2)}-W^{(i+1)}| &\leq D_{V_\beta(0)}\left(1-\phi(D_X^\infty)\right)^{i+1}\int_{0}^t (t-s)^{\alpha-1} E_{\alpha, \alpha}(-(t-s)^{\alpha})\sum\limits_{j = 0}^\infty\left(\frac{{}_{(j+i)}C_{j}\cdot(-1)^j}{\Gamma(\alpha(j+i)+1)}\right)\cdot s^{\alpha (j+i)}ds\\ &\quad+ \frac{2\sqrt{2}N_\gamma \|V(0)\|(1-\phi(D_X^\infty))^{i+1}}{N}\\ &\quad\qquad \times \Bigg[E_{\alpha, \alpha}\left(-\left(\frac{t}{2}\right)^\alpha\right)\left(\frac{t}{2}\right)^{\alpha-1}\int_{0}^{\infty} {\phi\left(\frac{\Delta^k_v(0)}{4\Gamma(\alpha+1)}s^\alpha+r_0\right)} ds\\ & \quad +\phi\left(\frac{\Delta^k_v(0)}{4\Gamma(\alpha+1)}\left(\frac{t}{2}\right)^\alpha+r_0\right)\Bigg]\times \int_{0}^t (t-s)^{\alpha-1}E_{\alpha, \alpha}(-(t-s)^\alpha)ds\\ &\leq D_{V_\beta(0)}\left(1-\phi(D_X^\infty)\right)^{i+1}\int_{0}^t (t-s)^{\alpha-1} E_{\alpha, \alpha}(-(t-s)^{\alpha})\sum\limits_{j = 0}^\infty\left(\frac{{}_{(j+i)}C_{j}\cdot(-1)^j}{\Gamma(\alpha(j+i)+1)}\right)\cdot s^{\alpha (j+i)}ds\\ &\quad+ \frac{2\sqrt{2}N_\gamma \|V(0)\|(1-\phi(D_X^\infty))^{i+1}}{N}\\ &\quad\qquad \times \Bigg[E_{\alpha, \alpha}\left(-\left(\frac{t}{2}\right)^\alpha\right)\left(\frac{t}{2}\right)^{\alpha-1}\int_{0}^{\infty} {\phi\left(\frac{\Delta^k_v(0)}{4\Gamma(\alpha+1)}s^\alpha+r_0\right)} ds\\ & \quad +\phi\left(\frac{\Delta^k_v(0)}{4\Gamma(\alpha+1)}\left(\frac{t}{2}\right)^\alpha+r_0\right)\Bigg]\\ & = D_{V_\beta(0)}\left(1-\phi(D_X^\infty)\right)^{i+1}\sum\limits_{j, k = 0}^\infty \left(\frac{{}_{(j+i)}C_{j}\cdot(-1)^{k+j}}{\Gamma(\alpha(k+1) )\Gamma(\alpha(j+i)+1)}\right) \int_{0}^t (t-s)^{\alpha(k+1)-1}s^{\alpha(j+i)}ds\\ &\quad+ \frac{2\sqrt{2}N_\gamma \|V(0)\|(1-\phi(D_X^\infty))^{i+1}}{N}\\ &\quad\qquad \times \Bigg[E_{\alpha, \alpha}\left(-\left(\frac{t}{2}\right)^\alpha\right)\left(\frac{t}{2}\right)^{\alpha-1}\int_{0}^{\infty} {\phi\left(\frac{\Delta^k_v(0)}{4\Gamma(\alpha+1)}s^\alpha+r_0\right)} ds\\ & \quad +\phi\left(\frac{\Delta^k_v(0)}{4\Gamma(\alpha+1)}\left(\frac{t}{2}\right)^\alpha+r_0\right)\Bigg] \\ & = D_{V_\beta(0)}\left(1-\phi(D_X^\infty)\right)^{i+1}\sum\limits_{j = 0}^\infty \left(\frac{{}_{(j+i+1)}C_{j}\cdot(-1)^j}{\Gamma(\alpha (j+i+1)+1)}\right)\cdot t^{\alpha (j+i+1)}\\ &\quad+ \frac{2\sqrt{2}N_\gamma \|V(0)\|(1-\phi(D_X^\infty))^{i+1}}{N}\\ &\quad\qquad \times \Bigg[E_{\alpha, \alpha}\left(-\left(\frac{t}{2}\right)^\alpha\right)\left(\frac{t}{2}\right)^{\alpha-1}\int_{0}^{\infty} {\phi\left(\frac{\Delta^k_v(0)}{4\Gamma(\alpha+1)}s^\alpha+r_0\right)} ds\\ & \quad +\phi\left(\frac{\Delta^k_v(0)}{4\Gamma(\alpha+1)}\left(\frac{t}{2}\right)^\alpha+r_0\right)\Bigg], \end{align*} |
where we used the same methodology as in (2.10) to the last equation. Finally, we obtain the desired lemma.
As a direct consequence of Lemma 3.6, we verify from Lemma 2.1 and Definition 2.3 that, for t\in [0, S_1^*] ,
\begin{align*} \begin{aligned} D_{V_\beta}& = \lim\limits_{i\to\infty}D_{V_\beta^{(i)}}\leq \lim\limits_{i\to\infty} W^{(i)}\leq \sum\limits_{i = 0}^\infty|W^{(i+1)}-W^{(i)}|\\ &\leq D_{V_\beta(0)}\sum\limits_{i = 0}^\infty\sum\limits_{j = i}^\infty \left(-(1-\phi(D_X^\infty))\right)^{i} \left(\frac{{}_{j}C_{i}\cdot(-1)^j}{\Gamma(\alpha j+1)}\right)\cdot t^{\alpha j}\\ &\qquad+ \frac{2\sqrt{2}N_\gamma \|V(0)\|}{N\phi(D_X^\infty)}E_{\alpha, \alpha}\left(-\left(\frac{t}{2}\right)^\alpha\right)\left(\frac{t}{2}\right)^{\alpha-1}\int_{0}^{\infty} {\phi\left(\frac{\Delta^k_v(0)}{4\Gamma(\alpha+1)}s^\alpha+r_0\right)} ds\\ &\qquad + \frac{2\sqrt{2}N_\gamma \|V(0)\|}{N\phi(D_X^\infty)}\phi\left(\frac{\Delta^k_v(0)}{4\Gamma(\alpha+1)}\left(\frac{t}{2}\right)^\alpha+r_0\right). \end{aligned} \end{align*} |
Moreover, we see from (3.7) that, for t\in [0, S_1^*] ,
\begin{align*} \begin{aligned} D_{V_\beta} &\leq D_{V_\beta(0)}E_{\alpha, 1}\left(-\phi(D_X^\infty) t^\alpha\right)\\ &\qquad+ \frac{2\sqrt{2}N_\gamma \|V(0)\|}{N\phi(D_X^\infty)}E_{\alpha, \alpha}\left(-\left(\frac{t}{2}\right)^\alpha\right)\left(\frac{t}{2}\right)^{\alpha-1}\int_{0}^{\infty} {\phi\left(\frac{\Delta^k_v(0)}{4\Gamma(\alpha+1)}s^\alpha+r_0\right)} ds\\ &\qquad + \frac{2\sqrt{2}N_\gamma \|V(0)\|}{N\phi(D_X^\infty)}\phi\left(\frac{\Delta^k_v(0)}{4\Gamma(\alpha+1)}\left(\frac{t}{2}\right)^\alpha+r_0\right). \end{aligned} \end{align*} |
In conclusion, we deduce that, for t\in [0, S_1^*] ,
\begin{align} \begin{aligned} D_V& = \sum\limits_{\beta = 1}^2D_{V_\beta} \\ &\leq D_{V(0)}E_{\alpha, 1}\left(-\phi(D_X^\infty) t^\alpha\right) \\ &\qquad+ \frac{2\sqrt{2} \|V(0)\|}{\phi(D_X^\infty)}E_{\alpha, \alpha}\left(-\left(\frac{t}{2}\right)^\alpha\right)\left(\frac{t}{2}\right)^{\alpha-1}\int_{0}^{\infty} {\phi\left(\frac{\Delta^k_v(0)}{4\Gamma(\alpha+1)}s^\alpha+r_0\right)} ds \\ &\qquad + \frac{2\sqrt{2} \|V(0)\|}{\phi(D_X^\infty)}\phi\left(\frac{\Delta^k_v(0)}{4\Gamma(\alpha+1)}\left(\frac{t}{2}\right)^\alpha+r_0\right). \end{aligned} \end{align} | (3.15) |
Now, we are ready to demonstrate the bi-cluster flocking of the fractional system (3.1) by proving that S_1^* = \infty .
Theorem 3.1 (Bi-cluster flocking). Assume that (X(0), V(0)) satisfies (\mathcal{F}_1) – (\mathcal{F}_3) , and suppose that (X, V) is the solution of the system (3.1). Then, we get the following bi-cluster flocking estimate for t\in \mathbb{R}_+ :
(1) (Group formation and bi-cluster formation)
D_{X(t)}\leq D_X^\infty\quad \mathit{\text{and}} \quad \min\limits_{i\in [N_1], j\in [N_2]}\|x_{1i}(t)-x_{2j}(t)\|\geq \frac{\Delta^k_v(0)}{4\Gamma(\alpha+1)}t^\alpha+r_0. |
(2) (Velocity alignment)
\begin{align*} \begin{aligned} D_{V(t)} &\leq D_{V(0)}E_{\alpha, 1}\left(-\phi(D_X^\infty) t^\alpha\right)\\ &\qquad+ \frac{2\sqrt{2} \|V(0)\|}{\phi(D_X^\infty)}E_{\alpha, \alpha}\left(-\left(\frac{t}{2}\right)^\alpha\right)\left(\frac{t}{2}\right)^{\alpha-1}\int_{0}^{\infty} {\phi\left(\frac{\Delta^k_v(0)}{4\Gamma(\alpha+1)}s^\alpha+r_0\right)} ds\\ &\qquad + \frac{2\sqrt{2} \|V(0)\|}{\phi(D_X^\infty)}\phi\left(\frac{\Delta^k_v(0)}{4\Gamma(\alpha+1)}\left(\frac{t}{2}\right)^\alpha+r_0\right). \end{aligned} \end{align*} |
Proof. Due to Lemma 3.5 and (3.15), it suffices to derive that S_1^* = \infty . For the proof by contradiction, suppose that S_1^* < \infty . Then, we have
D_{X(S_1^*)} = D_X^\infty. |
Subsequently, from (2.2) and
D_{\alpha}^c X_1 = V_1\quad \text{and} \quad D_{\alpha}^c X_2 = V_2, |
one has
\begin{align*} &X_1(t) = X_1(0)+\frac{1}{\Gamma(\alpha)}\int_{0}^t \frac{V_1(s)}{(t-s)^{1-\alpha}} ds, \\ &X_2(t) = X_2(0)+\frac{1}{\Gamma(\alpha)}\int_{0}^t \frac{V_2(s)}{(t-s)^{1-\alpha}} ds. \end{align*} |
Thus, taking a diameter to the above relations leads to
\begin{align*} &D_{X_1(t)}\leq D_{X_1(0)}+\frac{1}{\Gamma(\alpha)}\int_{0}^t \frac{D_{V_1(s)}}{(t-s)^{1-\alpha}} ds, \\ &D_{X_2(t)}\leq D_{X_2(0)}+\frac{1}{\Gamma(\alpha)}\int_{0}^t \frac{D_{V_2(s)}}{(t-s)^{1-\alpha}} ds, \end{align*} |
and we obtain D_{X(t)}\leq D_{X(0)}+\frac{1}{\Gamma(\alpha)}\int_{0}^t \frac{D_{V(s)}}{(t-s)^{1-\alpha}} ds.
Herein, (3.15), Propositions 2.1 and 2.3 yield that
\begin{align} \begin{aligned} D_{X(S_1^*)} &\leq D_{X(0)}+\frac{1}{\Gamma(\alpha)}\int_{0}^{S_1^*} \frac{D_{V(s)}}{(S_1^*-s)^{1-\alpha}} ds\\ &\leq D_{X(0)}+ \frac{D_{V(0)}}{\Gamma(\alpha)}\int_{0}^{S_1^*} \frac{E_{\alpha, 1}\left(-\phi(D_X^\infty) s^\alpha\right)}{(S_1^*-s)^{1-\alpha}} ds\\ &\quad+ \frac{2\sqrt{2} \|V(0)\|}{\phi(D_X^\infty)\Gamma(\alpha)}\int_{0}^{\infty} {\phi\left(\frac{\Delta^k_v(0)}{4\Gamma(\alpha+1)}s^\alpha+r_0\right)} ds\cdot\int_{0}^{S_1^*} \frac{E_{\alpha, \alpha}\left(-\left(\frac{s}{2}\right)^\alpha\right)\left(\frac{s}{2}\right)^{\alpha-1}}{(S_1^*-s)^{1-\alpha}} ds\\ &\quad + \frac{2\sqrt{2} \|V(0)\|}{\phi(D_X^\infty)\Gamma(\alpha)}\int_{0}^{S_1^*} \frac{\phi\left(\frac{\Delta^k_v(0)}{4\Gamma(\alpha+1)}\left(\frac{s}{2}\right)^\alpha+r_0\right)}{(S_1^*-s)^{1-\alpha}} ds\\ & = D_{X(0)}+ \frac{D_{V(0)}}{\phi(D_X^\infty)}\left(\frac{1}{\Gamma(\alpha)}-E_{\alpha, \alpha+1}(-\phi(D_X^\infty (S_1^*)^\alpha)\right)\\ &\quad+ \frac{2^\alpha\sqrt{2} \|V(0)\|(S_1^*)^{2\alpha-1}}{\phi(D_X^\infty)}\int_{0}^{\infty} {\phi\left(\frac{\Delta^k_v(0)}{4\Gamma(\alpha+1)}s^\alpha+r_0\right)} ds \cdot E_{2\alpha, \alpha}\left(-\left(\frac{1}{2}\right)^\alpha \cdot (S_1^*)^\alpha\right)\\ &\quad + \frac{2\sqrt{2} \|V(0)\|}{\phi(D_X^\infty)\Gamma(\alpha)}\int_{0}^{S_1^*} \frac{\phi\left(\frac{\Delta^k_v(0)}{4\Gamma(\alpha+1)}\left(\frac{s}{2}\right)^\alpha+r_0\right)}{(S_1^*-s)^{1-\alpha}} ds\\ & < D_{X(0)}+ \frac{D_{V(0)}}{\phi(D_X^\infty)\Gamma(\alpha)}\\ &\quad+ \frac{2^\alpha\sqrt{2} \|V(0)\|(S_1^*)^{2\alpha-1}}{\phi(D_X^\infty)}\int_{0}^{\infty} {\phi\left(\frac{\Delta^k_v(0)}{4\Gamma(\alpha+1)}s^\alpha+r_0\right)} ds \cdot E_{2\alpha, \alpha}\left(-\left(\frac{1}{2}\right)^\alpha \cdot (S_1^*)^{2\alpha-1}\right)\\ &\quad + \frac{2\sqrt{2} \|V(0)\|}{\phi(D_X^\infty)\Gamma(\alpha)}\sup\limits_{t\in \mathbb{R}_+}\int_{0}^{t} \frac{\phi\left(\frac{\Delta^k_v(0)}{4\Gamma(\alpha+1)}\left(\frac{s}{2}\right)^\alpha+r_0\right)}{(t-s)^{1-\alpha}} ds \\ &\quad = D_{X(0)}+ \frac{D_{V(0)}}{\phi(D_X^\infty)\Gamma(\alpha)}\\ &\qquad+ \frac{2^{2\alpha}\sqrt{2} \|V(0)\|}{\phi(D_X^\infty)}\int_{0}^{\infty} {\phi\left(\frac{\Delta^k_v(0)}{4\Gamma(\alpha+1)}s^\alpha+r_0\right)} ds \cdot \left[\frac{1}{\Gamma(\alpha)}-E_{\alpha, \alpha}\left(-\left(\frac{1}{2}\right)^\alpha (S_1^*)^{2\alpha-1}\right)\right] \\ &\qquad + \frac{2\sqrt{2} \|V(0)\|}{\phi(D_X^\infty)\Gamma(\alpha)}\sup\limits_{t\in \mathbb{R}_+}\int_{0}^{t} \frac{\phi\left(\frac{\Delta^k_v(0)}{4\Gamma(\alpha+1)}\left(\frac{s}{2}\right)^\alpha+r_0\right)}{(t-s)^{1-\alpha}} ds\\ &\quad < D_{X(0)}+ \frac{D_{V(0)}}{\phi(D_X^\infty)\Gamma(\alpha)}+ \frac{2^{2\alpha}\sqrt{2} \|V(0)\|}{\phi(D_X^\infty)\Gamma(\alpha)}\int_{0}^{\infty} {\phi\left(\frac{\Delta^k_v(0)}{4\Gamma(\alpha+1)}s^\alpha+r_0\right)} ds \\ &\qquad + \frac{2\sqrt{2} \|V(0)\|}{\phi(D_X^\infty)\Gamma(\alpha)}\sup\limits_{t\in \mathbb{R}_+}\int_{0}^{t} \frac{\phi\left(\frac{\Delta^k_v(0)}{4\Gamma(\alpha+1)}\left(\frac{s}{2}\right)^\alpha+r_0\right)}{(t-s)^{1-\alpha}} ds\leq D_X^\infty, \end{aligned} \end{align} | (3.16) |
which gives that
D_{X(S_1^*)} < D_X^\infty. |
This is contradictory to D_{X(S_1^*)} = D_X^\infty ; thus, S_1^* = \infty , and we demonstrate the desired bi-cluster flocking estimate.
In this section, we obtain the multi-cluster flocking of the fractional system (1.2) under admissible data in terms of the initial data and system parameters. As in the bi-cluster system (3.1), we reformulate (1.2) to distinguish several cluster groups Z_\beta = \{(x_{\beta i}, v_{\beta i})\}_{i = 1}^{N_\beta} for \beta\in [n] from Definition 1.1, where \sum_{\beta = 1}^n N_\beta = N and N_\beta\geq 1 , as follows:
\begin{equation} \begin{cases} D_{\alpha}^c{x}_{\beta i} = v_{\beta i}, \quad t > 0, \quad i\in [N_\beta], \quad \beta\in[n], \\ D_{\alpha}^c{v}_{\beta i} = \frac{1}{N}\sum\limits_{k = 1}^{N_\beta}\phi(\|x_{\beta i}-x_{\beta k}\|)\left({v_{\beta k}}-{v_{\beta i}}\right)+\frac{1}{N}\sum\limits_{\gamma\neq \beta}\sum\limits_{k = 1}^{N_\gamma}\phi(\|x_{\beta i}-x_{\gamma k}\|)\left({v_{\gamma k}}-{v_{\beta i}}\right), \\ (x_k(0), v_k(0))\in \mathbb{R}^{2d}, \quad k\in[N]. \end{cases} \end{equation} | (4.1) |
The methodology in this section is an extended version of that in Section 3, where n = 2 extends to a general n ; thus, the detailed proof and arguments are omitted.
In this subsection, we provide suitable sufficient frameworks guaranteeing the multi-cluster flocking of (4.1) and handy notation for brevity. Similar to Section 3.1, for \beta\in [n] , we define
● (Position averages and deviations)
x_{\beta cen}: = \frac{1}{N_\beta }\sum\limits_{i = 1}^{N_\beta }x_{\beta i}, \quad \hat{x}_{\beta i}: = x_{\beta i}-x_{\beta cen}. |
● (Velocity averages and deviations)
v_{\beta cen}: = \frac{1}{N_\beta}\sum\limits_{i = 1}^{N_\beta}v_{\beta i}, \quad \hat{v}_{\beta i}: = v_{\beta i}-v_{\beta cen}. |
● (Configuration vectors for each cluster group)
\begin{align*} X_{\beta}: = (x_{\beta 1}, \ldots , x_{\beta N_{\beta}}), \qquad V_{\beta}: = (v_{\beta 1}, \ldots , v_{\beta N_{\beta}}). \end{align*} |
● (Reordering of configuration vectors)
X: = (X_1, \ldots, X_n)\quad \text{and}\quad V: = (V_1, \ldots, V_n). |
● (Configuration vectors for the deviation of each cluster group)
\begin{align*} \hat{X}_{\beta}: = (\hat{x}_{\beta 1}, \ldots , \hat{x}_{\beta N_{\beta}}), \qquad \hat{V}_{\beta}: = (\hat{v}_{\beta 1}, \ldots , \hat{v}_{\beta N_{\beta}}). \end{align*} |
● (Configuration vectors for deviation)
\hat{X}: = (\hat{X}_1, \ldots, \hat{X}_{n})\quad\text{and} \quad \hat{V}: = (\hat{V}_1, \ldots, \hat{V}_{n}). |
● (Difference between velocity averages)
\Delta_{v, \beta, \gamma}^k = v^k_{\beta cen}-v^k_{\gamma cen}, \quad k\in [d]. |
● (The position-velocity diameters of each cluster group)
\begin{align*} D_{X_\beta}: = \max\limits_{i, j\in [N_\beta]}\|x_{\beta i}-x_{\beta j}\|, \quad D_{V_\beta}: = \max\limits_{i, j\in [N_\beta]}\|v_{\beta i}-v_{\beta j}\|. \end{align*} |
● (Total position-velocity diameters)
D_{X}: = \sum\limits_{\beta = 1}^n D_{X_\beta}, \quad D_{V}: = \sum\limits_{\beta = 1}^n D_{V_\beta}. |
Then, we describe the following two lemmas counterparts of Lemmas 3.1 and 3.2 without the proof:
Lemma 4.1 (Basic estimate). Suppose that (X, V) is the solution of the system (4.1). Then, we get the following assertions for \beta\in [n] and t\in \mathbb{R}_+ :
\begin{align*} \sum\limits_{i = 1}^{N_\beta}\|\hat{v}_{\beta i}\|^2+N_\beta\|v_{\beta cen}\|^2 = \|V_\beta\|^2. \end{align*} |
Lemma 4.2 (Fractional systems for averages and deviations). Let (X, V) be the solution of the system (4.1). Then, (x_{\beta cen}, v_{\beta cen}) and (\hat{x}_{\beta i}, \hat{v}_{\beta i}) satisfy
\begin{equation*} \begin{cases} D_{\alpha}^c{x}_{\beta cen} = v_{\beta cen}, \quad t > 0, \quad \beta\in [n], \\ N_\beta D_{\alpha}^c{v}_{\beta cen} = \frac{1}{N}\sum\limits_{\gamma\neq \beta}\sum\limits_{i = 1}^{N_\beta}\sum\limits_{j = 1}^{N_\gamma}\phi(\|x_{\beta i}-x_{\gamma j}\|)\left({v_{\gamma j}}-{v_{\beta i}}\right) \end{cases} \end{equation*} |
and
\begin{equation*} \begin{cases} D^c_{\alpha}\hat{x}_{\beta i} = \hat{v}_{\beta i}, \quad t > 0, \quad i\in [N_\beta], \quad \beta\in [n], \\ D^c_{\alpha}\hat{v}_{\beta i} = -D_\alpha^c{v}_{\beta cen} + \frac{1}{N}\sum\limits_{j = 1}^{N_\beta}\phi(\|x_{\beta i}-x_{\beta j}\|)(\hat{v}_{\beta j}-\hat{v}_{\beta i})\\ \quad \quad \quad + \frac{1}{N}\sum\limits_{\gamma\neq\beta}\sum\limits_{j = 1}^{N_\gamma}\phi(\|x_{\beta i}-x_{\gamma j}\|)(v_{\gamma j}-v_{\beta i}). \end{cases} \end{equation*} |
Finally, we present several sufficient frameworks (\mathcal {G}) in terms of the initial data and system parameters for the multi-cluster flocking of (4.1) as follows:
(\mathcal {G}): = \{(X(0), V(0))\in \mathbb{R}^{2dN}\; |\; (\mathcal{G}_1), (\mathcal{G}_2)\; \text{and}\; (\mathcal{G}_3)\; \text{hold}\}. |
\diamond (\mathcal{G}_1)\; (\text{Separated initial data}) : For some k\in [d] , there exists a nonnegative number r_0\geq 0 such that the initial position configurations are chosen to be split as follows:
0\leq r_0 < \min\limits_{1\leq \beta < \gamma\leq n}\min\limits_{i\in [N_\beta], j\in [N_\gamma]}(x_{\beta i}^k(0)-x_{\gamma j}^k(0)). |
\diamond (\mathcal{G}_2)\; (\text{Condition for group formation}) : There is a nonnegative number D_X^\infty\geq 0 satisfying
\begin{align*} &D_{X(0)}+ \frac{D_{V(0)}}{\phi(D_X^\infty)\Gamma(\alpha)}+ \frac{2^{2\alpha}\sqrt{2}(n-1)N \|V(0)\|}{\phi(D_X^\infty)\Gamma(\alpha)}\int_{0}^{\infty} {\phi\left(\frac{\min\limits_{1\leq \beta < \gamma\leq n}\Delta^k_{v, \beta, \gamma}(0)}{4\Gamma(\alpha+1)}s^\alpha+r_0\right)} ds \\ &\quad + \frac{2\sqrt{2}(n-1)N\|V(0)\|}{\phi(D_X^\infty)\Gamma(\alpha)}\sup\limits_{t\in \mathbb{R}_+}\int_{0}^{t} \frac{\phi\left(\frac{\min\limits_{1\leq \beta < \gamma\leq n}\Delta^k_{v, \beta, \gamma}(0)}{4\Gamma(\alpha+1)}\left(\frac{s}{2}\right)^\alpha+r_0\right)}{(t-s)^{1-\alpha}} ds\leq D_X^\infty. \end{align*} |
\diamond (\mathcal{G}_3)\; (\text{Closeness to the multi-cluster}) : The initial data are in a formation state close to the multi-cluster as follows: For \beta, \gamma \in [n] ,
\begin{align*} &\circ\frac{\min\nolimits_{1\leq \beta < \gamma\leq n} \Delta^k_{v, \beta, \gamma}(0)}{4} > \sqrt{2(N_\beta-1)}D_{\hat{V}_\beta(0)}+\sqrt{2(N_\gamma-1)}D_{\hat{V}_\gamma(0)}, \\ &\; \; \; \qquad \quad \quad\qquad\qquad\geq\frac{(2N-(N_\beta+N_\gamma)){\|V(0)\|}}{2N\Gamma(\alpha)}\sup\limits_{t\in \mathbb{R}_+}\left(\int_{0}^{t}\frac{\phi\left(\frac{\min\nolimits_{1\leq \beta < \gamma\leq n}\Delta^k_{v, \beta, \gamma}(0)}{4\Gamma(\alpha+1)}s^\alpha+r_0\right)}{(t-s)^{1-\alpha}}ds\right), \\ &\circ\frac{2\sqrt{2}(N-N_\beta) \|V(0)\|}{N\phi(D_X^\infty)}\sup\limits_{t\in \mathbb{R}_+}\left(\int_{0}^{t}\frac{\phi\left(\frac{\min\nolimits_{1\leq \beta < \gamma\leq n}\Delta^k_{v, \beta, \gamma}(0)}{4\Gamma(\alpha+1)}s^\alpha+r_0\right)}{(t-s)^{1-\alpha}}ds\right)\leq D_{\hat{V}_\beta(0)}. \end{align*} |
In this subsection, we demonstrate the multi-cluster flocking of (4.1), assuming (\mathcal {G}) . Throughout the subsection, we set the following:
\phi_M(t): = \max\limits_{1\leq \beta\neq\gamma\leq n}\max\limits_{i\in [N_\beta], j\in [N_\gamma]}\phi(\|x_{\gamma j}-x_{\beta i}\|). |
Next, we prove that the distance between two distinct cluster groups is greater than some function proportional to t^\alpha . To achieve this, we provide the following lemma counterpart of Lemma 3.3:
Lemma 4.3 (Estimate of D_\alpha^c(\Delta_{v, \beta, \gamma}^k(t)) ). Let (X, V) be the solution of the system (4.1). Then, we obtain that, for \beta, \gamma \in [n] and t\in \mathbb{R}_+ ,
\begin{align*} D_{\alpha}^c(\Delta_{v, \beta, \gamma}^k) \geq - \frac{\sqrt{2}(2N-(N_\beta+N_\gamma))\|V(0)\|}{N}\phi_M. \end{align*} |
Proof. Employing Lemma 4.2 gives that
\begin{align*} D_{\alpha}^c(\Delta_{v, \beta, \gamma}^k)& = D_{\alpha}^c(v^k_{\beta cen}-v^k_{\gamma cen})\\ & = \frac{1}{NN_\beta}\sum\limits_{i = 1}^{N_\beta}\sum\limits_{\theta\neq \beta}\sum\limits_{k = 1}^{N_\theta}\phi(\|x_{\beta i}-x_{\theta k}\|)\left({v_{\theta k}}-{v_{\beta i}}\right)\\ &\quad+ \frac{1}{NN_\gamma}\sum\limits_{i = 1}^{N_\gamma}\sum\limits_{\theta\neq \gamma}\sum\limits_{k = 1}^{N_\theta}\phi(\|x_{\gamma i}-x_{\theta k}\|)\left({v_{\gamma i}}-{v_{\theta k}}\right). \end{align*} |
Thus, we attain the following estimates from Cauchy–Schwarz's inequality and Lemma 2.1:
\begin{align*} D_{\alpha}^c(\Delta_{v, \beta, \gamma}^k) &\geq - \frac{1}{NN_\beta}\phi_M\sum\limits_{i = 1}^{N_\beta}\sum\limits_{\theta\neq \beta}\sum\limits_{k = 1}^{N_\theta}\left(|{v_{\theta k}}|+|{v_{\beta i}}|\right)- \frac{1}{NN_\gamma}\phi_M\sum\limits_{i = 1}^{N_\gamma}\sum\limits_{\theta\neq \gamma}\sum\limits_{k = 1}^{N_\theta}\left(|{v_{\gamma i}}|+|{v_{\theta k}}|\right)\\ &\geq - \frac{\sqrt{2}(N-N_\beta)\|V(0)\|}{N}\phi_M- \frac{\sqrt{2}(N-N_\gamma)\|V(0)\|}{N}\phi_M\\ & = - \frac{\sqrt{2}(2N-(N_\beta+N_\gamma))\|V(0)\|}{N}\phi_M. \end{align*} |
In conclusion, we get the desired lemma.
In what follows, as in Section 3, we consider
S_1: = \sup\left\{s > 0\; \bigg|\; D_X(t)\leq D_X^\infty, \quad t\in [0, s), \right\}, |
S_1^*: = \sup S_1 and
S: = \left\{s > 0\; \bigg|\; \min\limits_{\beta < \gamma}\min\limits_{i, j}\|x_{\beta i}-x_{\gamma j}\|\geq \frac{\min\limits_{\beta < \gamma}\Delta^k_{v, \beta, \gamma}(0)}{4\Gamma(\alpha+1)}t^\alpha+r_0, \quad t\in [0, s), \quad s\leq S_1^*\right\}, |
S^*: = \sup S . By the continuity of D_X and (\mathcal{G}_2) , one has S_1\neq \emptyset and S_1^*\in (0, \infty] . Moreover, because (\mathcal{G}_1) implies that
0\leq r_0 < \min\limits_{1\leq \beta < \gamma\leq n}\min\limits_{i\in [N_\beta], j\in [N_\gamma]}(x_{\beta i}^k(0)-x_{\gamma j}^k(0))\leq \min\limits_{1\leq \beta < \gamma\leq n}\min\limits_{i\in [N_\beta], j\in [N_\gamma]}\|x_{\beta i}(0)-x_{\gamma j}(0)\| |
and \min_{i\in [N_\beta], j\in [N_\gamma]}\|x_{\beta i}-x_{\gamma j}\| is continuous, it follows that S\neq \emptyset and S^*\in (0, S_1^*] .
Then, we can obtain the same result as Lemma 3.4 on [0, S^*] .
Lemma 4.4 (Estimate of D_{\hat{V}_\beta} ). Assume that (X(0), V(0)) satisfies (\mathcal{G}_1) – (\mathcal{G}_3) , and suppose that (X, V) is the solution of (4.1). Then, for \beta\in [n] and t\in [0, S^*] ,
D_{\hat{V}_\beta}\leq 2D_{\hat{V}_\beta(0)}. |
Proof. We omit this proof because it is similar to the proof of Lemma 3.4.
In the same manner as the proof of Lemma 3.5, when the three frameworks (\mathcal{G}_1) – (\mathcal{G}_3) are assumed, we verify that for \beta\neq \gamma , \min_{i\in [N_\beta], j\in [N_\gamma]}\|x_{\beta i}-x_{\gamma j}\| is greater than a function that increases proportionally to t^\alpha , as follows:
Lemma 4.5. [Estimate of \min_{i\in [N_\beta], j\in [N_\gamma]}\|x_{\beta i}-x_{\gamma j}\| ] Suppose that (X(0), V(0)) satisfies (\mathcal{G}_1) – (\mathcal{G}_3) , and let (X, V) be the solution of (4.1). Then, S^* = S_1^* holds, which asserts that
\begin{align} \min\limits_{1\leq \beta < \gamma\leq n}\min\limits_{i\in [N_\beta], j\in [N_\gamma]}\|x_{\beta i}-x_{\gamma j}\|\geq \frac{\min\limits_{1\leq \beta < \gamma\leq n}\Delta^k_{v, \beta, \gamma}(0)}{4\Gamma(\alpha+1)}t^\alpha+r_0, \quad t\in [0, S_1^*]. \end{align} | (4.2) |
To derive the velocity alignment estimate concerning each cluster group based on the iterative method, we extend (3.8) to the multi-cluster setting. For this, for \beta\in [n] , we let
\phi_{\beta ij}(t): = \phi(\|x_{\beta j}(t)-x_{\beta i}(t)\|), \quad i, j \in [N_\beta], |
and set the following functional:
\Psi_{\beta ij}(t): = \frac{\phi_{\beta ij}(t)}{N_\beta}+\left(1-\frac{\sum\nolimits_{k = 1}^{N_\beta}\phi_{\beta ik}(t)}{N_\beta}\right)\delta_{ij}. |
Then, we immediately have that
\Psi_{\beta ij}\geq \frac{\phi_{\beta ij}}{N_\beta}, \qquad \sum\limits_{j = 1}^{N_\beta}\Psi_{\beta ij} = 1, \qquad \sum\limits_{j = 1}^{N_\beta}\Psi_{\beta ij}(v_{\beta j}-v_{\beta i}) = \sum\limits_{j = 1}^{N_\beta}\frac{\phi_{\alpha ij}}{N_\beta}(v_{\beta j}-v_{\beta i}). |
Similar to the construction of (3.8), we consider a subrecurrence relation \{V_\beta^{(i)}\}_{i = 0}^\infty for \beta\in [n] , as follows:
\begin{align} \begin{aligned} &V_\beta^{(0)} = 0, \quad i\in \mathbb{N}\cup \{0\}, \quad \beta\in [n], \\ &V_\beta^{(i+1)} = E_{\alpha, 1}(-t^\alpha)V_\beta(0)+\int_{0}^t (t-s)^{\alpha-1}E_{\alpha, \alpha}(-(t-s)^\alpha)\Phi_\beta(X(s)) V_\beta^{(i)}(s) ds\\ & \quad \quad\quad\quad+\int_{0}^t (t-s)^{\alpha-1}E_{\alpha, \alpha}(-(t-s)^\alpha)\mathcal{R}_\beta\big(X(s), V_\beta^{(i)}(s)\big)ds, \end{aligned} \end{align} | (4.3) |
where V_\beta^{(i)}(s)\in \mathbb{R}^{N_\beta\times d} , and \Phi_\beta(X(s))\in \mathbb{R}^{N_\beta\times N_\beta} is given by
\begin{equation*} (\Phi_\beta(X(s)))_{ij}: = \Psi_{\beta ij}(s)\quad \text{for}\quad i, j\in [N_\beta], \end{equation*} |
and \mathcal{R}_\beta\big(X(s), V_\beta^{(i)}(s)\big)\in \mathbb{R}^{N_\beta\times d} is written by
\begin{equation*} \mathcal{R}_\beta\big(X(s), V_\beta^{(i)}(s)\big): = \begin{bmatrix} \frac{1}{N}\sum\nolimits_{\gamma\neq \beta}\sum\nolimits_{k = 1}^{N_\gamma}\phi(\|x_{\beta 1}-x_{\gamma k}\|)\left({v^{(i)}_{\gamma k}}-{v^{(i)}_{\beta 1}}\right)^T \\ \vdots\\ \frac{1}{N}\sum\nolimits_{\gamma\neq \beta}\sum\nolimits_{k = 1}^{N_\gamma}\phi(\|x_{\beta N_\beta}-x_{\gamma k}\|)\left({v^{(i)}_{\gamma k}}-{v^{(i)}_{\beta N_{\beta}}}\right)^T \end{bmatrix}. \end{equation*} |
Subsequently, as in (3.9), it is easy to check that, for \beta\in[n] and t\in [0, S_1^*] ,
\begin{align} \mu\left(\Phi_\beta(X(t))\right)\geq \phi(D_X^\infty). \end{align} | (4.4) |
Hence, if we take a diameter to (4.3) and apply (4.4) and Lemma 2.2 to this, we reach that, for t\in [0, S_1^*] ,
\begin{align*} D_{V_\beta^{(i+1)}} &\leq E_{\alpha, 1}(-t^\alpha)D_{V_\beta(0)}\\ &\quad+\int_{0}^t (t-s)^{\alpha-1}E_{\alpha, \alpha}(-(t-s)^\alpha)\left[(1-\phi(D_X^\infty))D_{V_\beta^{(i)}(s)}+D_{\mathcal{R}_\beta\big(X(s), V_\beta^{(i)}(s)\big)}\right] ds\\ &\leq E_{\alpha, 1}(-t^\alpha)D_{V_\beta(0)}\\ &\quad+\int_{0}^t (t-s)^{\alpha-1}E_{\alpha, \alpha}(-(t-s)^\alpha)\left[(1-\phi(D_X^\infty))D_{V_\beta^{(i)}(s)}+\frac{2\sqrt{2}(N-N_\beta) \|V(0)\|}{N}\phi_M\right] ds, \end{align*} |
where D_{\mathcal{R}_\beta\big(X(s), V_\beta^{(i)}(s)\big)} can be estimated as follows, with the same argument as that for (3.10):
D_{\mathcal{R}_\beta\big(X(s), V_\beta^{(i)}(s)\big)}\leq \frac{2\sqrt{2}(N-N_\beta) \|V(0)\|}{N}\phi_M. |
Accordingly, under the frameworks (\mathcal{G}_1) – (\mathcal{G}_3) , using (3.13), (4.2), and the same methodologies employed in (3.11) and (3.12), we induce that, for t\in [0, S_1^*] ,
\begin{align*} &D_{V_\beta^{(i+1)}}\leq E_{\alpha, 1}(-t^\alpha)D_{V_\beta(0)}+\int_{0}^t (t-s)^{\alpha-1}E_{\alpha, \alpha}(-(t-s)^\alpha)(1-\phi(D_X^\infty))D_{V_\beta^{(i)}(s)} ds\\ &\qquad\qquad + \frac{2\sqrt{2}(N-N_\beta) \|V(0)\|}{N}\\ &\qquad\qquad\qquad\times E_{\alpha, \alpha}\left(-\left(\frac{t}{2}\right)^\alpha\right)\left(\frac{t}{2}\right)^{\alpha-1}\int_{0}^{\infty} \phi\left(\frac{\min\nolimits_{1\leq \beta < \gamma\leq n}\Delta^k_{v, \beta, \gamma}(0)}{4\Gamma(\alpha+1)}s^\alpha+r_0\right) ds\\ &\qquad\qquad + \frac{2\sqrt{2}(N-N_\beta) \|V(0)\|}{N}\phi\left(\frac{\min\nolimits_{1\leq \beta < \gamma\leq n}\Delta^k_{v, \beta, \gamma}(0)}{4\Gamma(\alpha+1)}\left(\frac{t}{2}\right)^\alpha+r_0\right). \end{align*} |
In the sequel, we consider the following recurrence relation concerning \{W^{(i)}\}_{i = 0}^\infty to study the velocity alignment estimate of (4.1):
\begin{align} \begin{aligned} &W^{(0)} = 0, \quad i\in \mathbb{N}\cup \{0\}, \\ \\ &W^{(i+1)} = E_{\alpha, 1}(-t^\alpha)D_{V_\beta(0)}+\int_{0}^t (t-s)^{\alpha-1}E_{\alpha, \alpha}(-(t-s)^\alpha)(1-\phi(D_X^\infty)){W^{(i)}(s)}ds\\ &\qquad\qquad + \frac{2\sqrt{2}(N-N_\beta) \|V(0)\|}{N}\\ &\qquad\qquad\qquad\times E_{\alpha, \alpha}\left(-\left(\frac{t}{2}\right)^\alpha\right)\left(\frac{t}{2}\right)^{\alpha-1}\int_{0}^{\infty} \phi\left(\frac{\min\nolimits_{1\leq \beta < \gamma\leq n}\Delta^k_{v, \beta, \gamma}(0)}{4\Gamma(\alpha+1)}s^\alpha+r_0\right) ds\\ &\qquad\qquad + \frac{2\sqrt{2}(N-N_\beta) \|V(0)\|}{N}\phi\left(\frac{\min\nolimits_{1\leq \beta < \gamma\leq n}\Delta^k_{v, \beta, \gamma}(0)}{4\Gamma(\alpha+1)}\left(\frac{t}{2}\right)^\alpha+r_0\right). \end{aligned} \end{align} | (4.5) |
Herein, we crucially notice that for each i\in \mathbb{N}\cup\{0\} , D_{V_\beta^{(i)}}\leq W^{(i)} .
Then, as in the proof of Lemma 3.6, the inductive argument yields a relation regarding |W^{(i+1)}-W^{(i)}| for i\in [N] as below:
Lemma 4.6 (Estimate for |W^{(i+1)}-W^{(i)}| ). In the recurrence relation (4.5), the following assertions hold for i\in \mathbb{N}\cup\{0\} and t\in [0, S_1^*] :
\begin{align*} |W^{(i+1)}-W^{(i)}| &\leq D_{V_\beta(0)}\left(-(1-\phi(D_X^\infty))\right)^{i}\sum\limits_{j = i}^\infty \left(\frac{{}_{j}C_{i}\cdot(-1)^j}{\Gamma(\alpha j+1)}\right)\cdot t^{\alpha j}\\ &\quad+ \frac{2\sqrt{2}(N-N_\beta) \|V(0)\|(1-\phi(D_X^\infty))^i}{N}E_{\alpha, \alpha}\left(-\left(\frac{t}{2}\right)^\alpha\right)\left(\frac{t}{2}\right)^{\alpha-1}\\ &\qquad\qquad\times\int_{0}^{\infty} {\phi\left(\frac{\min\nolimits_{1\leq \beta < \gamma\leq n}\Delta^k_{v, \beta, \gamma}(0)}{4\Gamma(\alpha+1)}s^\alpha+r_0\right)} ds\\ &\quad + \frac{2\sqrt{2}(N-N_\beta) \|V(0)\|(1-\phi(D_X^\infty))^i}{N}\phi\left(\frac{\min\nolimits_{1\leq \beta < \gamma\leq n}\Delta^k_{v, \beta, \gamma}(0)}{4\Gamma(\alpha+1)}\left(\frac{t}{2}\right)^\alpha+r_0\right). \end{align*} |
Therefore, due to (3.7) and Lemma 3.6, it follows from Lemma 2.1 and Definition 2.3 that, for t\in [0, S_1^*] ,
\begin{align*} \begin{aligned} &D_{V_\beta} = \lim\limits_{i\to\infty}D_{V_\beta^{(i)}}\leq \lim\limits_{i\to\infty} W^{(i)}\leq \sum\limits_{i = 0}^\infty|W^{(i+1)}-W^{(i)}|\\ &\leq D_{V_\beta(0)}E_{\alpha, 1}\left(-\phi(D_X^\infty) t^\alpha\right)\\ & \quad + \frac{2\sqrt{2}(N-N_\beta) \|V(0)\|}{N\phi(D_X^\infty)}E_{\alpha, \alpha}\left(-\left(\frac{t}{2}\right)^\alpha\right)\left(\frac{t}{2}\right)^{\alpha-1}\int_{0}^{\infty} {\phi\left(\frac{\min\nolimits_{1\leq \beta < \gamma\leq n}\Delta^k_{v, \beta, \gamma}(0)}{4\Gamma(\alpha+1)}s^\alpha+r_0\right)} ds\\ & \quad + \frac{2\sqrt{2}(N-N_\beta) \|V(0)\|}{N\phi(D_X^\infty)}\phi\left(\frac{\min\nolimits_{1\leq \beta < \gamma\leq n}\Delta^k_{v, \beta, \gamma}(0)}{4\Gamma(\alpha+1)}\left(\frac{t}{2}\right)^\alpha+r_0\right), \end{aligned} \end{align*} |
which implies that, for t\in [0, S_1^*] ,
\begin{align} \begin{aligned} D_V& = \sum\limits_{\beta = 1}^nD_{V_\beta}\\ &\leq D_{V(0)}E_{\alpha, 1}\left(-\phi(D_X^\infty) t^\alpha\right)\\ &\qquad+ \frac{2\sqrt{2}(n-1)N \|V(0)\|}{\phi(D_X^\infty)}E_{\alpha, \alpha}\left(-\left(\frac{t}{2}\right)^\alpha\right)\left(\frac{t}{2}\right)^{\alpha-1}\\ &\qquad\qquad\times\int_{0}^{\infty} {\phi\left(\frac{\min\nolimits_{1\leq \beta < \gamma\leq n}\Delta^k_{v, \beta, \gamma}(0)}{4\Gamma(\alpha+1)}s^\alpha+r_0\right)} ds\\ &\qquad + \frac{2\sqrt{2}(n-1)N \|V(0)\|}{\phi(D_X^\infty)}\phi\left(\frac{\min\nolimits_{1\leq \beta < \gamma\leq n}\Delta^k_{v, \beta, \gamma}(0)}{4\Gamma(\alpha+1)}\left(\frac{t}{2}\right)^\alpha+r_0\right). \end{aligned} \end{align} | (4.6) |
Finally, we prove the multi-cluster flocking of the system (4.1) under (\mathcal{G}_1) – (\mathcal{G}_3) .
Theorem 4.1 (Multi-cluster flocking). Suppose that (X(0), V(0)) satisfies (\mathcal{G}_1) – (\mathcal{G}_3) , and assume that (X, V) is the solution of (4.1). Then, the following multi-cluster flocking estimate holds for t\in \mathbb{R}_+ :
(1) (Group formation and multi-cluster formation)
\qquad D_{X(t)}\leq D_X^\infty\quad \mathit{\text{and}} \quad \min\limits_{1\leq \beta < \gamma\leq n}\min\limits_{i\in [N_\beta], j\in [N_\gamma]}\|x_{\beta i}-x_{\gamma j}\|\geq \frac{\min\nolimits_{1\leq \beta < \gamma\leq n}\Delta^k_{v, \beta, \gamma}(0)}{4\Gamma(\alpha+1)}t^\alpha+r_0. |
(2) (Velocity alignment)
\begin{align*} \begin{aligned} D_{V(t)} &\leq D_{V(0)}E_{\alpha, 1}\left(-\phi(D_X^\infty) t^\alpha\right)\\ &\qquad+ \frac{2\sqrt{2}(n-1)N \|V(0)\|}{\phi(D_X^\infty)}E_{\alpha, \alpha}\left(-\left(\frac{t}{2}\right)^\alpha\right)\left(\frac{t}{2}\right)^{\alpha-1}\\ &\qquad\qquad\times\int_{0}^{\infty} {\phi\left(\frac{\min\nolimits_{1\leq \beta < \gamma\leq n}\Delta^k_{v, \beta, \gamma}(0)}{4\Gamma(\alpha+1)}s^\alpha+r_0\right)} ds\\ &\qquad + \frac{2\sqrt{2}(n-1)N \|V(0)\|}{\phi(D_X^\infty)}\phi\left(\frac{\min\nolimits_{1\leq \beta < \gamma\leq n}\Delta^k_{v, \beta, \gamma}(0)}{4\Gamma(\alpha+1)}\left(\frac{t}{2}\right)^\alpha+r_0\right). \end{aligned} \end{align*} |
Proof. By Lemma 4.5 and (4.6), it suffices to demonstrate that S_1^* = \infty . For the proof by contradiction, suppose that S_1^* < \infty . Then,
D_{X(S_1^*)} = D_X^\infty. |
Similar to the proof of Theorem 3.1, one has
D_{X(t)}\leq D_{X(0)}+\frac{1}{\Gamma(\alpha)}\int_{0}^t \frac{D_{V(s)}}{(t-s)^{1-\alpha}} ds. |
Moreover, based on the arguments employed in (3.16), we can derive from (4.6), Propositions 2.1 and 2.3 that, for t\in [0, S_1^*] ,
\begin{align*} D_{X(S_1^*)}& < D_{X(0)}+ \frac{D_{V(0)}}{\phi(D_X^\infty)\Gamma(\alpha)}\\ &\quad+ \frac{2^{2\alpha}\sqrt{2}(n-1)N \|V(0)\|}{\phi(D_X^\infty)\Gamma(\alpha)}\int_{0}^{\infty} {\phi\left(\frac{\min\nolimits_{1\leq \beta < \gamma\leq n}\Delta^k_{v, \beta, \gamma}(0)}{4\Gamma(\alpha+1)}s^\alpha+r_0\right)} ds \\ &\quad + \frac{2\sqrt{2}(n-1)N\|V(0)\|}{\phi(D_X^\infty)\Gamma(\alpha)}\sup\limits_{t\in \mathbb{R}_+}\int_{0}^{t} \frac{\phi\left(\frac{\min\nolimits_{1\leq \beta < \gamma\leq n}\Delta^k_{v, \beta, \gamma}(0)}{4\Gamma(\alpha+1)}\left(\frac{s}{2}\right)^\alpha+r_0\right)}{(t-s)^{1-\alpha}} ds\\ &\leq D_X^\infty. \end{align*} |
This causes a contradiction to D_{X(S_1^*)} = D_X^\infty . Eventually, S_1^* = \infty , and we conclude the desired multi-cluster flocking of (4.1).
In this section, we present several numerical descriptions using the Grunwald–Letnikov approximation to compare with the analytical result of Theorem 4.1. For all simulations, we set
\begin{align*} &N = {9}, \quad \Delta t = h = 0.1, \quad {t = mh\in[0, 1000]}, \quad m\in \{0, 1, 2, \ldots, {1000}\}, \\ & N_1 = N_2 = N_3 = 3, \quad n = 3, \quad \alpha = 0.5, \quad d = 2, \quad k = 1, \quad r_0 = 200, \quad D_X^\infty = 10, \end{align*} |
and choose the below communication weight:
\begin{align*} \begin{aligned} \phi(s) = 10\exp(-0.1s), \quad s\in\mathbb{R_+}. \end{aligned} \end{align*} |
Then, we select the following initial data satisfying
\begin{align*} &\{x_i(0)\}_{i = 1}^{9} = \bigg\{ (305, -1), \; (306, 0), \; (304, 0), \; (0, 1), \; (1, 0), \; (-1, 0), \\ & \quad \quad\quad\quad\quad\quad\quad (-305, -1), \; (-304, 0), \; (-306, 0)\bigg\}, \\ &\{v_i(0)\}_{i = 1}^{9} = \bigg\{ (6.1, -1), \; (5.9, -0.9), \; (6, -1), \; (0, 1.1), \; (0.1, 0.9), \; (0, 1), \\ & \quad \quad\quad\quad\quad\quad\quad(-5.9, -1), \; (-6.1, -1), \; (-6, -1)\bigg\}, \end{align*} |
and consider the 3 -cluster groups as follows:
\begin{align*} &Z_1: = \{(x_1, v_1), (x_2, v_2), (x_3, v_3)\}, \\ &Z_2: = \{(x_4, v_4), (x_5, v_5), (x_6, v_6)\}, \\ &Z_3: = \{(x_7, v_7), (x_8, v_8), (x_9, v_9)\}, \end{align*} |
where we denote
\begin{align*} &(x_{11}, v_{11}): = (x_1, v_1), \quad (x_{12}, v_{12}): = (x_2, v_2), \quad (x_{13}, v_{13}): = (x_3, v_3), \\ &(x_{21}, v_{21}): = (x_4, v_4), \quad (x_{22}, v_{22}): = (x_5, v_5), \quad (x_{23}, v_{23}): = (x_6, v_6), \\ &(x_{31}, v_{31}): = (x_7, v_7), \quad (x_{32}, v_{32}): = (x_8, v_8), \quad (x_{33}, v_{33}): = (x_9, v_9). \end{align*} |
Subsequently, we now briefly check that the above initial data and system parameters satisfy the sufficient framework (\mathcal{G}_1) – (\mathcal{G}_3) addressed in Section 4. To do this, we observe through direct calculation that
\begin{align*} &\Gamma(0.5) = \sqrt{\pi}, \quad \Gamma(1.5)\approx 0.88622, \quad D_{\hat{V}_1(0)} = D_{\hat{V}_2(0)} = \sqrt{0.05}, \quad D_{\hat{V}_3(0)} = 0.2, \\ & D_X(0) = 6, \quad D_V(0) = 0.2+\sqrt{0.2}, \quad \|V(0)\|\approx 14.97, \quad 2^\alpha = 2^{0.5} = \sqrt{2}, \quad \phi(10)\approx 3.68, \\ & r_0 = 200 < \min\limits_{1\leq \beta < \gamma\leq 3}\min\limits_{i\in [N_\beta], j\in [N_\gamma]}(x_{\beta i}^1(0)-x_{\gamma j}^1(0)), \quad \min\limits_{1\leq \beta < \gamma\leq 3} \Delta^1_{v, \beta, \gamma}(0)\approx 5.9667. \end{align*} |
Hence, it remains to verify the framework (\mathcal{G}_2) - (\mathcal{G}_3) . When using the Python code, it follows that the following relations hold:
\begin{align*} &\int_{0}^{\infty} {\phi\left(\frac{\min\nolimits_{1\leq \beta < \gamma\leq n}\Delta^k_{v, \beta, \gamma}(0)}{4\Gamma(\alpha+1)}s^\alpha+r_0\right)} ds \approx \int_{0}^{\infty}\phi\left(\frac{5.9667}{4\cdot 0.88622}s^{0.5}+200\right)ds < 10^{-6}, \\ &\sup\limits_{t\in \mathbb{R}_+}\left(\int_{0}^{t} \frac{\phi\left(\frac{\min\nolimits_{1\leq \beta < \gamma\leq n}\Delta^k_{v, \beta, \gamma}(0)}{4\Gamma(\alpha+1)}\left(\frac{s}{2}\right)^\alpha+r_0\right)}{(t-s)^{1-\alpha}} ds \right) < 10^{-5}, \\ &\sup\limits_{t\in \mathbb{R}_+}\left(\int_{0}^{t}\frac{\phi\left(\frac{\min\nolimits_{1\leq \beta < \gamma\leq n}\Delta^k_{v, \beta, \gamma}(0)}{4\Gamma(\alpha+1)}s^\alpha+r_0\right)}{(t-s)^{1-\alpha}}ds\right) < 10^{-5}. \end{align*} |
Therefore, it is straightforward to see that (\mathcal{G}_2) is satisfied because one has
\begin{align*} &D_{X(0)}+ \frac{D_{V(0)}}{\phi(D_X^\infty)\Gamma(\alpha)}+ \frac{2^{2\alpha}\sqrt{2}(n-1)N \|V(0)\|}{\phi(D_X^\infty)\Gamma(\alpha)}\int_{0}^{\infty} {\phi\left(\frac{\min\nolimits_{1\leq \beta < \gamma\leq n}\Delta^k_{v, \beta, \gamma}(0)}{4\Gamma(\alpha+1)}s^\alpha+r_0\right)} ds \\ &\quad + \frac{2\sqrt{2}(n-1)N\|V(0)\|}{\phi(D_X^\infty)\Gamma(\alpha)}\sup\limits_{t\in \mathbb{R}_+}\int_{0}^{t} \frac{\phi\left(\frac{\min\nolimits_{1\leq \beta < \gamma\leq n}\Delta^k_{v, \beta, \gamma}(0)}{4\Gamma(\alpha+1)}\left(\frac{s}{2}\right)^\alpha+r_0\right)}{(t-s)^{1-\alpha}} ds\\ & < 6+\frac{0.2+\sqrt{0.2}+4\sqrt{2}\cdot 9\cdot 14.97\cdot 10^{-6}+4\sqrt{2}\cdot 9\cdot 14.97\cdot 10^{-5}}{3.68\cdot\sqrt{\pi}}\approx 6.1 < D_X^\infty = 10. \end{align*} |
Furthermore, we can show that (\mathcal{G}_3) is satisfied due to for \beta, \gamma \in [3] ,
\begin{align*} &\frac{\min\nolimits_{1\leq \beta < \gamma\leq 3} \Delta^k_{v, \beta, \gamma}(0)}{4}\approx \frac{5.9667}{4} > 2\sqrt{0.05}+2\sqrt{0.05}\\ &\geq \sqrt{2(N_\beta-1)}D_{\hat{V}_\beta(0)}+\sqrt{2(N_\gamma-1)}D_{\hat{V}_\gamma(0)}\geq 2\cdot 0.2+2\sqrt{0.05}\approx 0.847 > \frac{12\cdot 14.97\cdot 10^{-5}}{2\cdot 9 \cdot \sqrt{\pi}}\\ &\geq\frac{(2N-(N_\beta+N_\gamma)){\|V(0)\|}}{2N\Gamma(\alpha)}\sup\limits_{t\in \mathbb{R}_+}\left(\int_{0}^{t}\frac{\phi\left(\frac{\min\nolimits_{1\leq \beta < \gamma\leq n}\Delta^k_{v, \beta, \gamma}(0)}{4\Gamma(\alpha+1)}s^\alpha+r_0\right)}{(t-s)^{1-\alpha}}ds\right), \end{align*} |
and for \beta\in [3] ,
\begin{align*} &\frac{2\sqrt{2}(N-N_\beta) \|V(0)\|}{N\phi(D_X^\infty)}\sup\limits_{t\in \mathbb{R}_+}\left(\int_{0}^{t}\frac{\phi\left(\frac{\min\nolimits_{1\leq \beta < \gamma\leq n}\Delta^k_{v, \beta, \gamma}(0)}{4\Gamma(\alpha+1)}s^\alpha+r_0\right)}{(t-s)^{1-\alpha}}ds\right)\\ & < \frac{2\sqrt{2}\cdot 6\cdot 14.97\cdot 10^{-5}}{9\cdot 3.68}\approx 7.67\cdot 10^{-5} < 0.2\leq D_{\hat{V}_\beta(0)}. \end{align*} |
Next, we describe several numerical results concerning the multi-cluster flocking result studied in Theorem 4.1.
First, Figure 1 shows the emergence of multi-cluster flocking, substantiating the analytical results of Theorem 4.1. Second, Figure 2 illustrates the emergence of multi-cluster formation, validating the analytical results of Theorem 4.1. In particular, Figure 2(B) indicates that
\min\limits_{1\leq \beta < \gamma\leq 3}\min\limits_{i\in [N_\beta], j\in [N_\gamma]}\|x_{\beta i}-x_{\gamma j}\| |
tends to increase as a constant multiple of t^{0.5} .
In this paper, we have studied the multi-cluster flocking of the fractional CS model with the Caputo fractional derivative. In the proposed fractional system, we offered appropriate admissible data consisting of well-separated initial data, well-prepared system parameters, and a communication weight satisfying suitable conditions to demonstrate the desired multi-cluster flocking. Afterward, we observed that multi-cluster flocking does not rapidly occur, unlike exponential decay. The rate of occurrence depends on the algebraic decay rate and communication weight. However, we are unsatisfied with the current results and still have some topics to explore in future research. Examples include the mean-field limit (i.e., N\to \infty ), extension to the Riemannian manifold, and collision avoidance of the fractional CS system. We leave these issues as future work.
The authors declare they have not used Artificial Intelligence (AI) tools in the creation of this article.
The work of H. Ahn was supported by the National Research Foundation of Korea (NRF) grant funded by the Korea government (MSIT) (2022R1C12007321).
The author declares no conflict of interest.
[1] |
H. Ahn, Emergent behaviors of thermodynamic Cucker–Smale ensemble with unit-speed constraint, Discrete Cont. Dyn. Syst.-Ser. B, 28 (2023), 4800–4825. https://doi.org/10.3934/dcdsb.2023042 doi: 10.3934/dcdsb.2023042
![]() |
[2] |
H. Ahn, S. Y. Ha, D. Kim, F. Schlöder, W. Shim, The mean-field limit of the Cucker–Smale model on Riemannian manifolds, Quart. Appl. Math., 80 (2022), 403–450. https://doi.org/10.1090/qam/1613 doi: 10.1090/qam/1613
![]() |
[3] |
H. Ahn, S. Y. Ha, J. Kim, Nonrelativistic limits of the relativistic Cucker–Smale model and its kinetic counterpart, J. Math. Phys., 63 (2022), 082701. https://doi.org/10.1063/5.0070586 doi: 10.1063/5.0070586
![]() |
[4] |
H. Ahn, S. Y. Ha, W. Shim, Emergent dynamics of a thermodynamic Cucker–Smale ensemble on complete Riemannian manifolds, Kinet. Relat. Models, 14 (2021), 323–351. https://doi.org/10.3934/krm.2021007 doi: 10.3934/krm.2021007
![]() |
[5] |
B. Bonilla, M. Rivero, J. J. Trujillo, On systems of linear fractional differential equations with constant coefficients, Appl. Math. Comput., 187 (2007), 68–78. https://doi.org/10.1016/j.amc.2006.08.104 doi: 10.1016/j.amc.2006.08.104
![]() |
[6] |
L. Bourdin, Cauchy–Lipschitz theory for fractional multi-order dynamics: state-transition matrices, Duhamel formulas and duality theorems, Differ. Integral Equ., 31 (2018), 559–594. https://doi.org/10.57262/die/1526004031 doi: 10.57262/die/1526004031
![]() |
[7] |
J. A. Carrillo, M. Fornasier, J. Rosado, G. Toscani, Asymptotic flocking dynamics for the kinetic Cucker–Smale model, SIAM. J. Math. Anal., 42 (2010), 218–236. https://doi.org/10.1137/090757290 doi: 10.1137/090757290
![]() |
[8] |
P. Cattiaux, F. Delebecque, L. Pédèches, Stochastic Cucker–Smale models: old and new, Ann. Appl. Probab., 28 (2018), 3239–3286. https://doi.org/10.1214/18-AAP1400 doi: 10.1214/18-AAP1400
![]() |
[9] |
J. Cho, S. Y. Ha, F. Huang, C. Jin, D. Ko, Emergence of bi-cluster flocking for the Cucker–Smale model, Math. Mod. Meth. Appl. Sci., 26 (2016), 1191–1218. https://doi.org/10.1142/S0218202516500287 doi: 10.1142/S0218202516500287
![]() |
[10] |
S. H. Choi, S. Y. Ha, Emergence of flocking for a multi-agent system moving with constant speed, Commun. Math. Sci., 14 (2016), 953–972. https://doi.org/10.4310/CMS.2016.v14.n4.a4 doi: 10.4310/CMS.2016.v14.n4.a4
![]() |
[11] | Y. P. Choi, S. Y. Ha, Z. Li, Emergent dynamics of the Cucker–Smale flocking model and its variants, In: N. Bellomo, P. Degond, E. Tadmor, Active particles, volume 1: advances in theory, models, and applications, Cham: Birkhäuser, 2017,299–331. https://doi.org/10.1007/978-3-319-49996-3_8 |
[12] |
Y. P. Choi, D. Kalsie, J. Peszek, A. Peters, A collisionless singular Cucker–Smale model with decentralized formation control, SIAM J. Appl. Dyn. Syst., 18 (2019), 1954–1981. https://doi.org/10.1137/19M1241799 doi: 10.1137/19M1241799
![]() |
[13] |
Y. P. Choi, Z. Li, Emergent behavior of Cucker–Smale flocking particles with heterogeneous time delays, Appl. Math. Lett., 86 (2018), 49–56. https://doi.org/10.1016/j.aml.2018.06.018 doi: 10.1016/j.aml.2018.06.018
![]() |
[14] |
F. Cucker, S. Smale, Emergent behavior in flocks, IEEE Trans. Automat. Control, 52 (2007), 852–862. https://doi.org/10.1109/TAC.2007.895842 doi: 10.1109/TAC.2007.895842
![]() |
[15] |
K. Diethelm, Monotonocity of functions and sign changes of their Caputo derivatives, Fract. Calc. Appl. Anal., 19 (2016), 561–566. https://doi.org/10.1515/fca-2016-0029 doi: 10.1515/fca-2016-0029
![]() |
[16] |
J. G. Dong, S. Y. Ha, D. Kim, Emergent behaviors of continuous and discrete thermomechanical Cucker–Smale models on general digraphs, Math. Mod. Meth. Appl. Sci., 29 (2019), 589–632. https://doi.org/10.1142/S0218202519400013 doi: 10.1142/S0218202519400013
![]() |
[17] |
A. Figalli, M. Kang, A rigorous derivation from the kinetic Cucker–Smale model to the pressureless Euler system with nonlocal alignment, Anal. PDE, 12 (2019), 843–866. https://doi.org/10.2140/apde.2019.12.843 doi: 10.2140/apde.2019.12.843
![]() |
[18] |
E. Girejko, D. Mozyrska, M. Wyrwas, Numerical analysis of behaviour of the Cucker–Smale type models with fractional operators, J. Comput. Appl. Math., 339 (2018), 111–123. https://doi.org/10.1016/j.cam.2017.12.013 doi: 10.1016/j.cam.2017.12.013
![]() |
[19] |
E. Girejko, D. Mozyrska, M. Wyrwas, On the fractional variable order Cucker–Smale type model, IFAC-PapersOnLine, 51 (2018), 693–697. https://doi.org/10.1016/j.ifacol.2018.06.184 doi: 10.1016/j.ifacol.2018.06.184
![]() |
[20] |
S. Y. Ha, J. Jung, P. Kuchling, Emergence of anomalous flocking in the fractional Cucker–Smale model, Discrete Cont. Dyn. Syst., 39 (2019), 5465–5489. https://doi.org/10.3934/dcds.2019223 doi: 10.3934/dcds.2019223
![]() |
[21] |
S. Y. Ha, J. Kim, T. Ruggeri, From the relativistic mixture of gases to the relativistic Cucker–Smale flocking, Arch. Rational Mech. Anal., 235 (2020), 1661–1706. https://doi.org/10.1007/s00205-019-01452-y doi: 10.1007/s00205-019-01452-y
![]() |
[22] |
S. Y. Ha, J. Kim, X. Zhang, Uniform stability of the Cucker–Smale model and its application to the mean-field limit, Kinet. Relat. Mod., 11 (2018), 1157–1181. https://doi.org/10.3934/KRM.2018045 doi: 10.3934/KRM.2018045
![]() |
[23] | S. Y. Ha, J. G. Liu, A simple proof of Cucker–Smale flocking dynamics and mean-field limit, Commun. Math. Sci., 7 (2009), 297–325. |
[24] |
S. Y. Ha, T. Ruggeri, Emergent dynamics of a thermodynamically consistent particle model, Arch. Rational Mech. Anal., 223 (2017), 1397–1425. https://doi.org/10.1007/s00205-016-1062-3 doi: 10.1007/s00205-016-1062-3
![]() |
[25] | S. Y. Ha, E. Tadmor, From particle to kinetic and hydrodynamic description of flocking, Kinet. Relat. Mod., 1 (2008), 415–435. |
[26] |
J. Jung, P. Kuchling, Emergent dynamics of the fractional Cucker–Smale model under general network topologies, Commun. Pure Appl. Anal., 21 (2022), 2831–2856. https://doi.org/10.3934/cpaa.2022077 doi: 10.3934/cpaa.2022077
![]() |
[27] |
T. K. Karper, A. Mellet, K. Trivisa, Hydrodynamic limit of the kinetic Cucker–Smale flocking model, Math. Mod. Meth. Appl. Sci., 25 (2015), 131–163. https://doi.org/10.1142/S0218202515500050 doi: 10.1142/S0218202515500050
![]() |
[28] |
Z. Lu, Y. Zhu, Comparison principle for fractional differential equations with the Caputo derivatives, Adv. Differ. Equ., 2018 (2018), 237. https://doi.org/10.1186/s13662-018-1691-y doi: 10.1186/s13662-018-1691-y
![]() |
[29] | A. B. Malinowska, T. Odzijewicz, E. Schmeidel, On the existence of optimal controls for the fractional continuous-time Cucker–Smale model, In: A. Babiarz, A. Czornik, J. Klamka, M. Niezabitowski, Theory and applications of non-integer order systems, Cham: Springer, 407 (2017), 227–240. https://doi.org/10.1007/978-3-319-45474-0_21 |
[30] | M. Merkle, Completely monotone functions: a digest, In: G. V. Milovanovi\grave{{\rm{c}}}, M. Rassias, Analytic number theory, approximation theory, and special functions, New York: Springer, 2014,347–364. https://doi.org/10.1007/978-1-4939-0258-3_12 |
[31] | I. Podlubny, Fractional differential equations: an introduction to fractional derivatives, fractional differential equations, to methods of their solution and some of their applications, Vol. 198, Mathematics in Science and Engineering, Elsevier, 1999. |
[32] |
A. Ricardo, K. Rafal, A. B. Malinowska, O. Tatiana, On the necessary optimality conditions for the fractioanl Cucker–Smale optimal control problems, Commun. Nonlinear Sci. Numer. Simul., 96 (2021), 105678. https://doi.org/10.1016/j.cnsns.2020.105678 doi: 10.1016/j.cnsns.2020.105678
![]() |
[33] |
A. Ricardo, K. Rafal, A. B. Malinowska, O. Tatiana, On the existence of optimal consensus control for the fractioanl Cucker–Smale model, Arch. Control Sci., 30 (2020), 625–651. https://doi.org/10.24425/acs.2020.135844 doi: 10.24425/acs.2020.135844
![]() |
[34] | W. R. Schneider, Completely monotone generalized Mittag-Leffler functions, Expo. Math., 14 (1996), 3–16. |