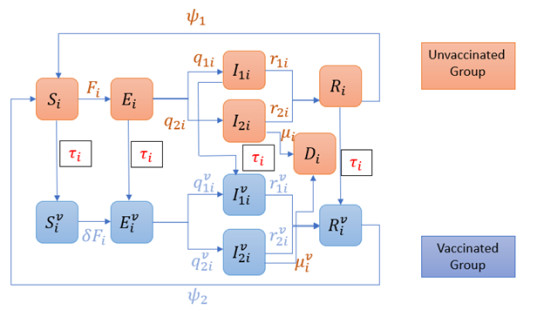
Citation: Amira Bouhali, Walid Ben Aribi, Slimane Ben Miled, Amira Kebir. Impact of immunity loss on the optimal vaccination strategy for an age-structured epidemiological model[J]. Mathematical Biosciences and Engineering, 2024, 21(6): 6372-6392. doi: 10.3934/mbe.2024278
[1] | Eunha Shim . Optimal strategies of social distancing and vaccination against seasonal influenza. Mathematical Biosciences and Engineering, 2013, 10(5&6): 1615-1634. doi: 10.3934/mbe.2013.10.1615 |
[2] | Pannathon Kreabkhontho, Watchara Teparos, Thitiya Theparod . Potential for eliminating COVID-19 in Thailand through third-dose vaccination: A modeling approach. Mathematical Biosciences and Engineering, 2024, 21(8): 6807-6828. doi: 10.3934/mbe.2024298 |
[3] | Giulia Luebben, Gilberto González-Parra, Bishop Cervantes . Study of optimal vaccination strategies for early COVID-19 pandemic using an age-structured mathematical model: A case study of the USA. Mathematical Biosciences and Engineering, 2023, 20(6): 10828-10865. doi: 10.3934/mbe.2023481 |
[4] | Eunha Shim . Prioritization of delayed vaccination for pandemic influenza. Mathematical Biosciences and Engineering, 2011, 8(1): 95-112. doi: 10.3934/mbe.2011.8.95 |
[5] | Rinaldo M. Colombo, Mauro Garavello . Optimizing vaccination strategies in an age structured SIR model. Mathematical Biosciences and Engineering, 2020, 17(2): 1074-1089. doi: 10.3934/mbe.2020057 |
[6] | Holly Gaff, Elsa Schaefer . Optimal control applied to vaccination and treatment strategies for various epidemiological models. Mathematical Biosciences and Engineering, 2009, 6(3): 469-492. doi: 10.3934/mbe.2009.6.469 |
[7] | Tetsuro Kobayashi, Hiroshi Nishiura . Prioritizing COVID-19 vaccination. Part 2: Real-time comparison between single-dose and double-dose in Japan. Mathematical Biosciences and Engineering, 2022, 19(7): 7410-7424. doi: 10.3934/mbe.2022350 |
[8] | Diána H. Knipl, Gergely Röst . Modelling the strategies for age specific vaccination scheduling during influenza pandemic outbreaks. Mathematical Biosciences and Engineering, 2011, 8(1): 123-139. doi: 10.3934/mbe.2011.8.123 |
[9] | Ayako Suzuki, Hiroshi Nishiura . Transmission dynamics of varicella before, during and after the COVID-19 pandemic in Japan: a modelling study. Mathematical Biosciences and Engineering, 2022, 19(6): 5998-6012. doi: 10.3934/mbe.2022280 |
[10] | Toshikazu Kuniya, Taisuke Nakata, Daisuke Fujii . Optimal vaccine allocation strategy: Theory and application to the early stage of COVID-19 in Japan. Mathematical Biosciences and Engineering, 2024, 21(6): 6359-6371. doi: 10.3934/mbe.2024277 |
The quest for effective vaccination strategies against COVID-19, coupled with the challenges posed by immunity loss and evolving epidemiological dynamics, constitutes a multifaceted endeavour at the forefront of global public health efforts. This endeavour encompasses various facets, ranging from the durability of vaccine-induced immunity to the emergence of new viral variants and the imperative of sustained vaccination efforts [1].
The optimisation of control strategies, particularly for vaccination allocation in the context of COVID-19, has been extensively studied and discussed. Research has varied, with some focusing on managing the pandemic through non-pharmaceutical interventions (NPIs) like social distancing and mask-wearing [2,3], while others have concentrated on vaccination strategies. Various studies offer insights into optimal vaccination strategies under different conditions. For instance, Gonzales-Parra, Cogollo, and Arenas [4] examined strategies considering factors like case fatality rates and population age structure. Hogan et al. [5] suggested targeting the elderly when vaccine supplies are scarce but recommended focusing on key transmitters to protect the susceptible population when supplies are plentiful and other interventions are ongoing. The study in [6] addressed the cost-effectiveness of combining vaccination with social distancing. Further research includes [7], which investigated disease control through combined vaccination, isolation, and treatment strategies. The authors of [8] explored the efficacy of single-dose vaccines under conditions of low transmission and high single-dose efficacy (SDE), showing that such a strategy could prevent up to 22% more deaths compared to a two-dose strategy prioritising the elderly. The impact of various control strategies on SARS-CoV-2 and simultaneous influenza infections was analyzed in [9]. Additionally, the implications of limited vaccine supplies and different vaccination rates and efficacies on virus transmission were explored in several studies, including those that assume a limited availability of effective COVID-19 rapid tests [10]. Urban et al. [11] assessed five vaccination approaches considering restricted vaccine access, daily rates, and efficacy. Finally, Zhou et al. [12] investigated the spatial heterogeneity of COVID-19 transmission in urban areas, optimising vaccine distribution strategies based on spatial priorities.
Central to this discourse is the concept of classical herd immunity, rooted in mathematical models that delineate the relationship between vaccination coverage and epidemic control. Defined as hC=1–1/R0, where R0 denotes the basic reproduction number, classical herd immunity underscores the pivotal role of vaccination in curtailing transmission dynamics [13].
However, the influence of age structure on epidemic transmission dynamics cannot be overstated. Age-dependent variations in susceptibility, transmission, and disease severity, as evidenced by empirical studies, underscore the need for tailored vaccination strategies that account for demographic nuances [14]. While younger individuals may exhibit lower risks of severe illness, they can serve as significant vectors of viral spread within communities. Conversely, older populations are at heightened risk of severe outcomes, necessitating prioritized vaccination efforts to safeguard vulnerable cohorts [15,16,17].
Age-related factors extend beyond susceptibility and severity to encompass vaccine response dynamics, necessitating tailored dosing regimens and booster strategies to optimise long-term protection, particularly in older adults [18,19]. Consequently, a comprehensive vaccination approach must intricately navigate these age-related nuances to optimise population-level immunity and mitigate the overall impact of COVID-19 [20].
Existing studies [20,21,22] have elucidated the role of age heterogeneity in achieving herd immunity thresholds and optimising vaccination prioritization; the transition to an endemic state underscores the need for sustained, long-term vaccination strategies. This necessitates a nuanced understanding of immunity loss dynamics, vaccine distribution logistics, and healthcare system constraints across diverse countries.
Addressing these challenges requires an interdisciplinary approach that integrates mathematical modeling and optimisation [23,24]. Optimal control theory offers a principled framework for devising vaccination strategies that balance epidemiological efficacy with practical constraints. Previous research [6,21] has explored short-term vaccination optimisation under resource constraints taking into consideration the age structure of the population. In [6], the authors presented an age-structured model to optimise vaccination and distancing for Brazil. However, several limitations arise. The vaccination compartment, condensed into a single equation, neglects the nuanced interplay of infection and recovery among vaccinated individuals. Additionally, assuming vaccination solely prevents infection without affecting transmission dynamics oversimplifies the complex relationship between vaccination and transmission. In [21], we provided a model to optimise the short-term vaccination strategy for the three age distributions where the vaccinated compartment undergoes the same epidemiological process of infection with different parameters. This study extends this paradigm to investigate the long-term implications of age and immunity loss on vaccination strategies.
In this study, we leverage mathematical optimisation techniques to formulate vaccination strategies tailored to the age demographics and immunity dynamics of three distinct age distributions. By categorizing age distributions into expansive, stationary, and constrictive pyramids, represented respectively by Senegal, USA, and Tunisia, we illuminate the profound influence of population age structure and immunity loss on disease transmission dynamics and optimal age-dependent vaccination [25].
In fact, we explore the impact of varying maximal vaccination rates on optimal vaccination strategies, ranging from uniform rates across all age groups to age-dependent approaches reminiscent of World Health Organization (WHO) guidelines [26]. Through rigorous mathematical analysis and numerical simulations, we elucidate the implications of these strategies on the optimal age-dependent vaccination strategy and the number of critical infections and vaccination frequencies, offering actionable insights for policymakers and healthcare professionals alike.
The remainder of this paper is structured as follows: Section 2 presents the formulation of the optimal control problem and underlying assumptions. In Section 3, we conduct mathematical analysis to establish the well-posedness of the model and characterise the optimal control. Section 4 delves into the analysis and discussion of numerical simulation results, while Section 5 concludes with key insights and avenues for future research.
This paper extends previous work [21] to explore the influence of age distribution and immunity loss on the optimal vaccination strategies over the long term. In our previous work [21], the model was developed to optimise an age-dependent vaccination in the short term, accounting for vaccine shortages. Building upon this foundation, we now introduce modifications to the model to incorporate immunity loss and vaccination across all compartments except for severely infected and deceased individuals.
In fact, similarly to the previous work, the population is divided into n age classes, with each age class i,1≤i≤n divided into vaccinated and unvaccinated groups. Considering that vaccination reduces infection and death rates rather than stopping it, it is assumed that both the vaccinated and unvaccinated groups go through the same epidemiological process: individuals leave the susceptible compartments Si (respectively vaccinated Svi), toward the exposed Ei (respectively vaccinated Evi) through contact with either exposed or infected individuals, whether they are vaccinated or not and of any age class j. The exposed can either develop or not a severe form of infection moving to one of the infected compartments I1i (respectively vaccinated Iv1i) for the non-severe form and I2i (respectively vaccinated Iv2i) for the severe form. Subsequently, they either move toward the recovered compartments Ri (respectively vaccinated Rvi) or the dead one Di. However, we assume that for each age group i, all epidemiological compartments except severely infected and dead are subjected to vaccination at a time-dependent rate τi. Additionally, we incorporate the concept that a segment of recovered individuals, both from vaccinated and unvaccinated groups, lose their immunity with respective rates ψ1 and ψ2, and revert to the susceptible compartment. As both vaccine-induced immunity and infection-induced one are lost with similar time scales, we choose not to differentiate between the types and merely use the term immunity regardless of the gaining way. This aspect is depicted in Figure 1.
The model proposed is given by:
˙Si=−n∑j=1pj(βiEjNj+βviEvjNj+α1iI1jNj+α2iI2jNj+αv1iIv1jNj+αv2iIv2jNj)dijSi−τiSi+ψ1Ri+ψ2Rvi | (2.1) |
˙Ei=n∑j=1pj(βiEjNj+βviEvjNj+α1iI1jNj+α2iI2jNj+αv1iIv1jNj+αv2iIv2jNj)dijSi−τiEi−(q1i+q2i)Ei | (2.2) |
˙I1i=q1iEi−τiI1i−r1iI1i | (2.3) |
˙I2i=q2iEi−r2iI2i−μiI2i | (2.4) |
˙Ri=r1iI1i+r2iI2i−τiRi−ψ1Ri | (2.5) |
˙Svi=τiSi−δn∑j=1pj(βiEjNj+βviEvjNj+α1iI1jNj+α2iI2jNj+αv1iIv1jNj+αv2iIv2jNj)dijSvi | (2.6) |
˙Evi=δn∑j=1pj(βiEjNj+βviEvjNj+α1iI1jNj+α2iI2jNj+αv1iIv1jNj+αv2iIv2jNj)dijSvi+τiEi−(qv1i+qv2i)Evi | (2.7) |
˙Iv1i=qv1iEvi+τiI1i−r1iIv1i | (2.8) |
˙Iv2i=qv2iEvi−r2iIv2i−μviIv2i | (2.9) |
˙Rvi=r1iIv1i+r2iIv2i+τiRi−ψ2Rvi | (2.10) |
˙Di=μiI2i+μviIv2i | (2.11) |
subject to positive initial conditions:
Si(0)>0,Ei(0)>0,I1i(0)>0,I2i(0)>0,Ri(0)>0, Svi(0)≥0,Evi(0)≥0,Iv1i(0)≥0,Iv2i(0)≥0 and Rvi(0)≥0, for all 1≤i≤n.
The parameters of the model for all 1≤i≤n are:
● (dij)1≤i,j≤n is the contact matrix, and each coefficient dij>0,∀i,j∈{1,…,n}, denotes the probability that an individual of age i contacts an individual of age j.
● Ni is the total number of individuals of age class i given by: Ni=Si+Ei+I1i+I2i+Ri+Svi+Evi+Ivi1+Ivi2+Rvi+Di.
● pi is the probability of an individual to be of age class i. (i.e., pi=NiN(0)), where N(0)=n∑i=1Ni is the total population.
● βi,βvi,α1,i,α2,i,αv1,i and αv2,i are the infection rates of Ei, Evi, I1i, I2i, Iv1i and Iv2i, respectively.
● δ denotes the infection reduction rate.
● q1i,q2i,qv1i,qv2i are the rates at which an exposed develops severe or non-severe forms of infection, respectively, for vaccinated and unvaccinated groups. Noteworthy the rate of developing a severe infection is higher among the non-vaccinated than the vaccinated i.e.,q2i>qv2i.
● r1i, rv1i, r2i and rv2i are the recovery rates for vaccinated and unvaccinated individuals, respectively.
● μi and μvi are disease-induced mortality rates for the vaccinated and unvaccinated, respectively.
All parameters of the model are assumed to be positive constants. Additionally, the demographic process is not taken into account for this model. Therefore, the population size for each age class i, Ni, and the total population size, N, are supposed constant.
Considering that vaccines do not stop infection, but rather reduce the rate of severe forms of it among the population, and that we are setting a long-term vaccination schedule, we aim to minimise the number of severe infections (vaccinated or not) and the cost of vaccination by controlling the age-dependent vaccination rate. Therefore, the objective function is given by:
J(τ)=∫T0A1(||I2(t)||22+||Iv2(t)||22)+A2||τ(t)||22dt. | (2.12) |
where:
● I2=(I2i)1≤i≤n is the vector of unvaccinated critical infected and Iv2=(Iv2i)1≤i≤n is the vector of vaccinated critical infected individuals subject to ((2.1)–(2.11))
● τ=(τi)1≤i≤n is the control variables vector.
● T denotes the time horizon of control.
● A1 and A2 are cost balancing factors and ||||2 stands for the Euclidean norm.
Minimising this function results in minimising the number of critical infections among both vaccinated and unvaccinated individuals, ||I2(t)||22+||Iv2(t)||22, with a minimal vaccination effort, ||τ(t)||22. Deaths are not explicitly present in the objective function, as minimising the number of critically infected individuals in hospitals has a direct impact on the minimisation of the number of disease-induced deaths. Due to the limitations that face various healthcare systems, we assume that there is a maximal threshold for the vaccination rate. And since we aim to control the vaccination rate according to age, we assume that the maximal vaccination rates are age-dependent, and then 0≤τi(t)≤τmaxi for all i∈{1,…,n} and for all t∈[0,T].
This problem can be written as follows:
minτ∈UJ |
subject to ((2.1)–(2.11)) where
U={τ=(τ1,…,τn)∈l∞([0,T],Rn):0≤τi(t)≤τmaxi∀i∈{1,…,n}and∀t∈[0,T]} | (2.13) |
To ensure that the model is biologically meaningful, it is important to prove that all solutions with non-negative initial conditions will remain non-negative at all positive times. The following proposition shows that the model is suitable to study living populations. Let X(t)=(X1(t),…,Xn(t)) be the vector of state variables, where
Xi(t)=(Si(t),Ei(t),I1i(t),I2i(t),Ri(t),Svi(t),Evi(t),Iv1i(t),Iv2i(t),Rvi(t),Di(t))=(Xji)1≤j≤11 |
for all i∈{1,…,n} and for all t>0.
Proposition 1. For any non-negative initial condition Xi(0), the solution Xi(t) remains positive, for all t>0 and i∈{1,…,n}. Furthermore, the set Ω given by:
Ω={X=(X1,…,Xn)∈(R11)n:0<Xji≤Ni(0)∀1≤j≤11and1≤i≤n} |
is positively invariant under system ((2.1)–(2.11)).
Hint of the proof. [1)]
Existence and uniqueness of the solution: The right-hand side functions of the system are all of class C1. Thus, the mere application of the Cauchy-Lipchitz theorem yields the existence and uniqueness of the solution.
● Positivity: For all 1≤j≤11, if Xji=0 and all other variables are positive i.e., Xki≥0 for k≠j, ˙Xji≥0. Then, the vector field is always either pointing to the inside of the positive region or invariant on each of the previously mentioned hyperplanes. Consequently, the solution can never leave the positive region.
● Boundedness: For all t≥0, Xji(t)≤Ni(t)=11∑j=1Xji(t). And since ˙Ni(t)=0, one has Ni constant and consequently equals Ni(0) as the age classes are supposed constant. Then, one has Xji(t)≤Ni(0).
This proposition deals with both mathematical and biological existence problems. The first part makes sure that the model is well-posed in terms of having a solution whereas the second part of it deals with its suitability for application to living populations.
Considering the loss of immunity, we formulate an optimal control problem to find the optimal vaccination rate under given constraints.
The optimal control problem consists of finding a piece-wise continuous control
τ∗(t)=(τ∗1(t),τ∗2(t),…,τ∗n(t)) |
and the associated state variables X∗=(S∗i,E∗i,I∗1i,I∗2i,R∗i,Sv∗i,Ev∗i,Iv∗1i,Iv∗2i,Rv∗i,D∗i),fori∈{1,…,n} to minimise the given objective functional (2.12), i.e.,
J(τ∗)=minτ∈UJ(u)=minτ∈U∫T0||I2(t)||22+||Iv2(t)||22+||τ(t)||22dt. |
subject to ((2.1)–(2.11)) and their initial conditions.
The following proposition concerns the existence of an optimal control for the vaccination.
Proposition 2. There exists an optimal control variable τ∗∈U such that J(τ∗)=minτ∈UJ(τ), subject to the controlled system ((2.1)–(2.11)).
Hint of the proof. To prove the existence of the optimal control, we use the results by Fleming and Rishel (1975) and by Lukes (1982) [27]. One can easily verify that:
1) The set of controls and corresponding state variables is nonempty.
2) The admissible set U is convex and closed.
3) The right-hand side of the state variables system ((2.1)–(2.11)) is bounded by a linear function in the state and control variables.
4) The integrand of the objective functional J is convex on U and there exist constants ω1>0,ω2>0 and ρ>1 such that
J(τ)≤ω2+ω1(n∑i=1|τi|2)ρ2 |
where ρ=2,ω1=ϵ2√nτmax+1, ω2=ϵ2 and ϵ=n∑i=1(I22i(0)+Iv22i(0))e−2r2iT.
The previous proposition answers the mathematical requirements of not characterising the control before ensuring its existence. Once the existence of optimal control has been proven, we use the minimum principle of Pontryagin [28], which transforms the optimality problem into a problem of minimisation of the Hamiltonian H with respect to the controls τ, to characterise this optimal control.
Therefore, we define the Hamiltonian given by:
H(t,τ,X,λ)=<λ(t),˙X(t)>+A1(||I2(t)||22+||Iv2(t)||22)+A2||τ(t)||22. |
Proposition 3. Given an optimal control τ∗∈U and solution X∗=(S∗i,E∗i,I∗1i,I∗2i,R∗i,Sv∗i,Ev∗i,Iv∗1i,Iv∗2i,Rv∗i,D∗i), i=(1,…,n) of the corresponding state system ((2.1)–(2.11)), there exists a vector of adjoint variables λ=(λ1i,…,λ11i), for all i∈{1,…,n}, satisfying:
{dλ1idt=λ1i(n∑j=1pjFijdij+τi)−λ2in∑j=1pjFijdij−λ6iτidλ2idt=λ2i(τi++q1,i+q2,i)−λ3iq1,i−λ4iq2,i−λ7iτi+βiNdi,i(λ1iSi−λ2iSi+δλ6iSvi−δλ7iSvi)dλ3idt=λ3i(τi+r1i)−λ5ir1i−λ8iτi+α1,iNdi,i(λ1iSi−λ2iSi+δλ6iSvi−δλ7iSvi)dλ4idt=−2A1I2,i+λ4i(r2i+μi)−λ5ir2i−λ11iμi+α2,iNdi,i(λ1iSi−λ2iSi+δλ6iSvi−δλ7iSvi)dλ5idt=−λ1iψ1+λ5i(τi+ψ1)−λ10iτidλ6idt=δn∑j=1pjFijdij(λ6i−λ7i)dλ7idt=λ7i(qv1,i+qv2,i)−λ8iqv1,i−λ9iqv2,i+βviNdi,i(λ1iSi−λ2iSi+δλ6iSvi−δλ7iSvi)dλ8idt=r1i(λ8i−λ10i)+αv1,iNdi,i(λ1iSi−λ2iSi+δλ6iSvi+δλ7iSvi)dλ9idt=−2A1Iv2,i+λ9i(r2i+μvi)−λ10ir2i−λ11iμvi+αv2,iNdi,i(λ1iSi−λ2iSi+δλ6iSvi−δλ7iSvi)dλ10idt=ψ2(λ10i−λ1i)dλ11idt=0 | (3.1) |
with transversality conditions
λji(T)=0,i∈{1,…,n},j∈{1,…,11}. |
Furthermore, the optimal control τ∗=(τ1,…,τn) is given by:
τ∗i=min{max{0,12A2[Si(λ1i−λ6i)+Ei(λ2i−λ7i)+I1,i(λ3i−λ8i)+Ri(λ5i−λ10i)]};τi,max},∀i∈{1,…,n}. | (3.2) |
Hint of the proof. The adjoint equations and transversality conditions are determined using Pontryagin's minimum principle such that:
˙Xji(t)=dHdλji(t,X(t),λ(t),τ(t)) | (3.3) |
˙λji(t)=−dHdXji(t,X(t),λ(t),τ(t)) | (3.4) |
where Xi=(Xji)T1≤j≤11=(Si,Ei,I1i,I2i,Ri,Svi,Evi,Iv1i,Iv2i,Rvi,Di)T, λi=(λji)T for all i∈{1,…,n}.
By minimising the Hamiltonian H, for all i∈{1,…,n}, the optimal control τ∗i can be obtained from the optimality condition:
dHdτi=0 |
In fact, since the optimal control τ∗∈U, it is easy to obtain τ∗ in the form given in (3.2).
The objective of this section is to investigate the impact of different age distributions and immunity loss on the optimal vaccination strategy of model ((2.1)–(2.11)). Age distributions are classified into three major categories *. The first category is expansive, where most of the population is constituted of young individuals and the older classes get, the fewer individuals there are. The second type is the stationary age distribution. Such distribution tends to remain constant, exhibiting a more or less equal distribution for all age categories. And the third type is the constrictive one, where middle-aged individuals form the majority of the population and fewer individuals are to be found in the oldest and youngest classes. For that, for the numerical simulations, we have chosen three countries presenting the previous three different age distributions: Senegal, the USA, and Tunisia (See Figure 2 for age class distribution per country).
*https://populationeducation.org/what-are-different-types-population-pyramids/
For each one of the studied countries, the initial conditions were estimated based on data from its own population†. All populations were divided into six age groups : G1=[0,14], G2=[15,29], G3=[30,44], G4=[45,59], G5=[60,74], and G6≥75, according to data from WHO database‡.
†https://raw.githubusercontent.com/CSSEGISandData/.../time_series_covid19_confirmed_global.csv
Moreover, considering that these countries have distinct economical classification, their healthcare systems face disparate limitations. Hence, we would add to the age distribution the impact of maximal age-dependant vaccination rate τi,max, for all 1≤i≤n. We study four scenarios according to the value of τi,max. For the first three scenarios τi,max=τmax,∀1≤i≤6, is constant for all age classes and corresponds respectively to 0.005, 0.01, and 0.05, whereas the fourth is age-dependant as it is given by a vector (τi,max)1≤i≤6=(0,005;0.005;0.01;0.01;0.05;0.05).
The problem is solved numerically using the fourth-order Runge–Kutta method. The used parameters are listed in the Appendix A.
In what follows, we present the numerical simulations' results in two parts. The first one corresponds to the effect of constant maximal vaccination rate (τmax) and age distribution on the optimal vaccination strategy for the three studied age distributions, while the second part displays the impact of setting an age-dependant maximal vaccination rate.
In what follows, we evaluate the optimal vaccination strategy and its impact on the number of critical infections for the three countries and with three maximal vaccination rates, τmax=0.005,0.01, and 0.05. To do so, we plot the optimal vaccination (3.2) resulting from solving the optimal control problem ((2.1)–(2.11)) (Figure 3), and the total number of critical infections without vaccination, {τ=0}, and with an optimal vaccination (Figure 4).
For τmax=0.005, the optimal vaccination strategies for the three countries exhibit consistent features: they remain constant over time and are set equal to the maximal vaccination rate across all age classes. Indeed, Figure 3(a)–(c) shows that the age distribution does not affect the determination of an age-tailored optimal vaccination strategy.
This induces a first peak of critical infections among the unvaccinated individuals that mimics the no-vaccination peak (see Figure 4(a)–(c)). This peak is lowest in Senegal, gets higher for Tunisia, and is highest for the USA. However, in the case of Senegal, no second peak is observed, unlike Tunisia where its beginnings start to appear by the end of the year, or the USA where it is more clearly noticed. In fact, in the absence of vaccination, we notice that all countries register a high peak of infection followed by a lower one.
For τmax=0.01, (see Figure 3(d)–(f)), a slight difference in the vaccination strategies of the various age classes becomes noticeable. For the three countries, vaccination starts at the same time at its maximum simultaneously for all age classes. However, differences start to be noted as the various age classes start to show disparate decreasing times of vaccination curve corresponding to various age classes.
We observed that the optimal strategy is to vaccinate the most representative age groups for both Senegal and Tunisia. Indeed, in Senegal (see Figure 3(d)), older age groups are vaccinated at a maximum rate for a shorter duration compared to younger age groups. The duration of vaccination increases with age. Similarly, in Tunisia (refer to Figure 3(e)), a similar pattern is observed, with the duration of vaccination overlapping across age groups. However, priority is given to middle-aged groups (classes 2–4), which are the most represented in the country's constrictive population pyramid.
However, in the case of USA (see Figure 3(f)), despite doubling the maximal vaccination rate, we continue to observe a simultaneous and constant vaccination equal to its maximal rate over the whole vaccination time horizon.
Moreover, the first peak of unvaccinated critical infected individuals decreases considerably for the case of Senegal and Tunisia and a little less for the case of the USA, while the second peaks vanish for the three countries (see Figure 4(d)–(f)).
For a maximal vaccination rate of τmax=0.05 (Figure 3(g)–(i)), the previously forming disparities become even more visible.
Like in previous cases, Senegal and Tunisia exhibit similar behaviors in their vaccination strategies, with a shorter common vaccination period observed for both countries (see Figure 3(h), (g)). However, in Senegal, the optimal vaccination of the fifth and sixth age classes (the oldest) start decreasing since t=0.
Additionally, in the case of the USA, optimal vaccination curves begin to show a similar pattern to those of Senegal and Tunisia. However, priority is given to the fourth age class (aged between 45 and 59), with the next level of priority granted to age classes 2 and 3. This results in no peaks at all in Senegal, while a little bump being observed for Tunisia, and a considerably small peak noticed in the USA (see Figure 4(g)–(i)).
These findings underscore the common feature of emphasizing the vaccination of the most represented class among the population. This, also, implies that vaccinating the young age classes can protect old classes for old and middle-aged populations. However, the impact of the chosen maximal vaccination rate differs according to the age distribution considered. The older the population is, the higher the vaccination rate needed to control critical infections is.
Figure 5 displays a comparison between the age distribution of vaccinated individuals for the optimal strategy (in green) and the corresponding age distribution class (in blue) for each country and for the three different values of the maximal vaccination rate τmax. For τmax=0.005, the optimal vaccination proportions show a similar appearance to that of the age distribution, with frequencies that are nearly equal to twice the corresponding age classes for the three countries (see Figure 5(a)–(c)) with an emphasis on the over-represented class of the distribution. Setting higher τmax=0.01 and τmax=0.05 does not change this aspect but rather increases the proportions to three or four times the age class (see Figure 5(d)–(f) and (i)).
However, we notice that for the expansive age distribution and for τmax=0.05, the frequency of vaccinating the youngest age class is now twice that of the oldest class. The three constant maximal vaccination rates τmax generated an age-distribution-like schedule, prioritising not only vulnerable age classes but also giving significant attention to classes with the highest numbers of individuals.
The inclusion of an age-dependant maximal vaccination rate generates different responses, and the optimal strategy shows a drastic change (see Figure 6). We notice a similar vaccination strategy for Senegal and Tunisia (see Figure 6(a), (b)) despite the difference in their age distributions. In fact, as in the constant maximal vaccination scenario, we start by vaccinating all classes with a maximal rate; however, the common vaccination period is shorter for the two oldest classes. The vaccination of these two oldest classes stops nearly at the middle of the time horizon. Conversely, the vaccination curves for the USA show a constant vaccination rate equal to its maximum over the whole time horizon for all age classes, which can be explained by the stationary aspect of the age distribution (see Figure 6(c)).
The effect of optimal vaccination on the number of critical infections (see Figure 7(a)–(c)) shows, for the three studied countries, a peak higher than that of the scenario with τmax=0.05 (see Figure 4(g)–(i)), the scenario with the lowest peak among the four considered scenarios. Despite this fact, the age-dependent vaccination strategy manages to contain infections similarly to the maximal vaccination rate of 0.01. Therefore, it represents a viable alternative to the latter strategy, which helps avoid overwhelming medical staff and expanding the vaccination cost. These results suggest that, in the long term, age-dependent vaccination rates can be an effective alternative to maximal vaccination strategies in certain situations and constant maximal rates can be more suitable in others.
In the last figure (see Figure 8), we plot the proportion of the vaccinated individuals for each age class for the three countries using an age-dependent maximal vaccination rate. We observe a huge alteration in the proportions compared to the constant maximal vaccination rate. Vaccination frequencies across all countries exhibit a trend of decreasing frequency as individuals become younger, with the most pronounced effect observed in populations with stationary age distributions, where vaccination frequency for older classes is twice that of younger ones. These results indicate that the age-dependent maximal vaccination strategy aligns with a WHO-like approach.
In this endeavour, our objective is to investigate the impact of age demographics and immunity loss on long-term vaccination strategies. To achieve this goal, we established a controlled age-structured SEIRD mathematical model, ensuring its biological validity. This model provided the framework needed to scrutinise the repercussions of varying maximal vaccination rates on disease propagation across distinct age distributions.
Having established the mathematical validity of our model, we formulated an optimal control problem and utilised the Pontryagin maximum principle to delineate the optimal course of the control. Subsequently, through numerical simulations applied to three disparate age distributions—expansive, constrictive, and stationary—we contrasted the outcomes of three distinct constant maximal vaccination rates (0.005, 0.01, and 0.05) with an age-dependent vaccination rate for each country.
We showed that the vaccination is most effective when applied to old and young age groups. However, our study shows also that in the long-term vaccination approach, two optimal strategies emerge depending on the chosen maximal vaccination rate: constant or age-dependent. In our previous work [21], the short-term vaccination strategy prioritised also the oldest age classes as well as the most significant age class, irrespective of any constraints. This was also confirmed by the age-structured model presented in [6], a study conducted for Brazil, which has a constrictive pyramid.
Furthermore, in this work, the results revealed that the optimal vaccination strategy is highly influenced by the choice of the maximal vaccination rate. When examining constant maximal vaccination rates, the significance of prioritising age groups based on population demographics became evident, with more attention given to the most represented age classes within each population. Moreover, augmented maximal vaccination rates led to a reduction in the number of critically infected individuals across all countries.
Conversely, an age-dependent approach yielded a WHO-like strategy, with infection peaks of greater magnitude than the maximal vaccination rate of 0.05 for all the nations under consideration. This suggests the need for careful consideration of both age distribution and vaccination strategy when designing public health interventions.
In summation, this research study contributes valuable insights into the field of optimal vaccination planning, emphasising the importance of considering the nuances of age groups and the impact of maximal vaccination efforts. The conclusions drawn from this research can inform policymakers and decision-makers in their ongoing efforts to reduce the spread of infectious diseases. As perspectives to this study, further exploration into the impact of memory on the dynamics of the model and the optimal control would provide valuable avenues for future research.
The authors declare they have not used Artificial Intelligence (AI) tools in the creation of this article.
We would like to thank Prof. Joel S. Brown of Moffitt Cancer Center, Department of Integrated Mathematical Oncology for conversations that contributed to enhancing the quality of this paper.
This work was supported, in part, by the Bill & Melinda Gates Foundation [INV-059607] and by Vaccine Impact Modelling Consortium (VIMC). Under the grant conditions of the Foundation, a Creative Commons Attribution 4.0 Generic License has already been assigned to the Author Accepted Manuscript version that might arise from this submission. At the time of analysis, the VIMC was jointly funded by Gavi, the Vaccine Alliance and the Bill & Melinda Gates Foundation (grant numbers INV-034281 and INV-009125/OPP1157270).
On behalf of all authors, the corresponding author states that there is no conflict of interest.
The parameters of the model are either based on literature or estimated using the data of the countries [32,33]. All these parameters are defined in Table A1.
Parameter Description | Country | ||
Tunisia | Senegal | USA | |
Population size [29] | 11818619 | 16743928 | 331002647 |
Age class distribution Pi [29] | 24, 3%, 21, 3%, | 42, 5%, 27, 3%, | 18%, 20%, |
23, 4%, 17, 7%, | 16, 7%, 8, 6%, | 20%, 19%, | |
10, 2%, 3, 1% | 4%, 0.7% | 16%, 7% | |
Infection rate α1i | 0, 326797 | 0, 326797 | 0, 326797 |
Infection rate α2i | 0,1.α1i | 0,1.α1i | 0,1.α1i |
Infection rate βi | (3/5).α1i | (3/5).α1i | (3/5).α1i |
Infection rate βvi | 0,8.βi | 0,8.βi | 0,8.βi |
Infection rate αv1i | 0,8.α1i | 0,8.α1i | 0,8.α1i |
Infection rate αv2i | 0,8.α2i | 0,8.α2i | 0,8.α2i |
Infection probability among δ | 0.3 | 0.3 | 0.3 |
Incubation rate λ [33] | 0.2 | 0.2 | 0.2 |
Hospitalization rate η [30] | 0.0027,0.0137,0.03, | 0.0027,0.0137,0.0301, | 0.0027,0.0137,0.03005, |
0.0792,0.1585,0.7158 | 0.0792,0.1584,0.7158 | 0.0792,0.1585,0.7158 | |
Infection rate q1 | 0.19999,0.19995,0.19988 | 0.19999,0.19995,0.19988 | 0.19999,0.19995,0.19988 |
0.19968,0.19937,0.19714 | 0.19968,0.19937,0.19714 | 0.19968,0.19937,0.19714 | |
Infection rate q2 | 1.0910−5,5.4610−5,1.20210−4 | 1.0910−5,5.4610−5,1.20210−4 | 1.0910−5,5.4610−5,1.20210−4 |
3.16910−4,6.33910−4,2.863410−3 | 3.16910−4,6.33910−4,2.863410−3 | 3.16910−4,6.33910−4,2.863410−3 | |
Infection rate qv1 | 0.199998,0.199995,0.199988 | 0.199998,0.199995,0.199988 | v |
0.199968,0.199937,0.199714 | 0.199968,0.199937,0.199714 | 0.199968,0.199937,0.199714 | |
Infection rate qv2 | 1.0910−6,5.4610−6,1.20210−5 | 1.0910−6,5.4610−6,1.20210−5 | 1.0910−6,5.4610−6,1.20210−5 |
3.16910−5,6.33910−5,2.863410−4 | 3.16910−5,6.33910−5,2.863410−4 | 3.16910−5,6.33910−5,2.863410−4 | |
Immunity loss rate ψ [33] | 1/180 | 1/180 | 1/180 |
Recovery rate of I1i, r1i [33] | 1/8 | 1/8 | 1/8 |
Recovery rate of I2, r2 [17] | 1/13.5,1/13.5,1/14, | 1/13.5,1/13.5,1/14, | 1/13.5,1/13.5,1/14, |
1/14.5,1/14.5,1/15 | 1/14.5,1/14.5,1/15 | 1/14.5,1/14.5,1/15 | |
Natural mortality rate [34] | 0,0,0.0001,0.0005, | 0,0,0.0001,0.0005, | 0,0,0.0001,0.0005, |
0.034,0.072 | 0.034,0.072 | 0.034,0.072 | |
Basic reproduction number | 2.1 | 2.1 | 2.1 |
Balancing cost factors A1 and A2 | 1000,N/2 | 1000,N/2 | 1000,N/2 |
τmax age-dependent | 0.005,0.005,0.01,0.01,0.05,0.05 | 0.005,0.005,0.01,0.01,0.05,0.05 | 0.005,0.005,0.01,0.01,0.05,0.05 |
Time horizon T | 1 year | 1 year | 1 year |
The infection rates by unvaccinated type-one infected α1i,1≤i≤n, were estimated, with respect to the value of R0 (R0=2.19), in a way to satisfy the following equations:
α1i+α2i+βi+αv1i+αv2i+βvi=1,1≤i≤n. | (A.1) |
α2i=0.1α1i,βi=0.6α1i,∀1≤i≤n | (A.2) |
αvki=0.8αki,k=1,2,βvi=0.8βi,∀1≤i≤n | (A.3) |
Noteworthy that R0 is not directly included in this study and is only used for parameter estimation. More details are available in [21].
For the three different countries, Tunisia, Senegal, and the USA, the contact matrices, D, used are estimated for 6 age groups based on [31].
The initial conditions used are estimated based on real data of the populations of the different countries mentioned, at the beginning of the vaccination campaign see Table A2. And using the age distribution of each country, the initial conditions for each infectious state and age per country are then defined in Table A3.
Parameter description | Country | ||
Tunisia | Senegal | USA | |
Date of first case | Mars 2, 2020 | Mars 2, 2020 | 26 February 2020 |
Date of first vaccination t0 | Mars 13, 2021 | Feb 22, 2021 | 10 December 2020 |
Number of cumulative cases at t0 | 242,124 | 16,529 | 16,217,128 |
Number of active case at t0 | 24,554 | 6451 | 9,669,611 |
Initial condition per country | Tunisia | Senegal | USA |
S(t0) | Pi−(272124)Pi/Pop | Pi−(23529)Pi/Pop | Pi−(25916216)Pi/Pop |
E(t0) | (30000Pi/Pop | (7000Pi/Pop | (10000000Pi/Pop |
I1(t0) | (1−0.02η)(24554)Pi/Pop | (1−0.02η)(6451)Pi/Pop | (1−0.02η)(9669611)Pi/Pop |
I2(t0) | 0.02η(24554)Pi/Pop | 0.02η(6451)Pi/Pop | 0.02η(9669611)Pi/Pop |
R(t0) | 217570Pi/Pop | 10078Pi/Pop | 6246605Pi/Pop |
Sv(t0) | (0, 0, 0, 0, 0, 0) | (0, 0, 0, 0, 0, 0) | (0, 0, 0, 0, 0, 0) |
Ev(t0) | (0, 0, 0, 0, 0, 0) | (0, 0, 0, 0, 0, 0) | (0, 0, 0, 0, 0, 0) |
Iv1(t0) | (0, 0, 0, 0, 0, 0) | (0, 0, 0, 0, 0, 0) | (0, 0, 0, 0, 0, 0) |
Iv2(t0) | (0, 0, 0, 0, 0, 0) | (0, 0, 0, 0, 0, 0) | (0, 0, 0, 0, 0, 0) |
Rv(t0) | (0, 0, 0, 0, 0, 0) | (0, 0, 0, 0, 0, 0) | (0, 0, 0, 0, 0, 0) |
D(t0) | (0, 0, 0, 0, 0, 0) | (0, 0, 0, 0, 0, 0) | (0, 0, 0, 0, 0, 0) |
[1] |
N. Wu, K. Joyal-Desmarais, P. A. B. Ribeiro, A. M. Vieira, J. Stojanovic, C. Sanuade, et al., Long-term effectiveness of COVID-19 vaccines against infections, hospitalizations, and mortality in adults: findings from a rapid living systematic evidence synthesis and meta-analysis up to December 2022, Lancet Respir. Med., 11 (2023), 439–452. https://doi.org/10.1016/S2213-2600(23)00015-2 doi: 10.1016/S2213-2600(23)00015-2
![]() |
[2] |
A. I. Abioye, O. J. Peter, H. A. Ogunseye, F. A. Oguntolu, K. Oshinubi, A. A. Ibrahim, et al., Mathematical model of COVID-19 in Nigeria with optimal control, Res. Phys., 28 (2021), 104598. https://doi.org/10.1016/j.rinp.2021.104598 doi: 10.1016/j.rinp.2021.104598
![]() |
[3] |
M. Diarra, A. Kebir, C. Talla, A. Barry, J. Faye, D. Louati, et al., Non-pharmaceutical interventions and COVID-19 vaccination strategies in Senegal: a modelling study, BMJ Glob. Health, 7 (2022), e007236. https://doi.org/10.1136/bmjgh-2021-007236 doi: 10.1136/bmjgh-2021-007236
![]() |
[4] |
G. Gonzalez-Parra, M. R. Cogollo, A. J. Arenas, Mathematical modeling to study optimal allocation of vaccines against COVID-19 using an age-structured population, Axioms, 11 (2022), 109. https://doi.org/10.3390/axioms11030109 doi: 10.3390/axioms11030109
![]() |
[5] |
A. B. Hogan, P. Winskill, O. J. Watson, P. G. T. Walker, C. Whittaker, M. Baguelin, et al., Within-country age-based prioritisation, global allocation, and public health impact of a vaccine against SARS-CoV-2: A mathematical modelling analysis, Vaccine, 39 (2021), 2995–3006. https://doi.org/10.1016/j.vaccine.2021.04.002 doi: 10.1016/j.vaccine.2021.04.002
![]() |
[6] |
P. Jia, J. Yang, X. Li, Optimal control and cost-effective analysis of an age-structured emerging infectious disease model, Inf. Dis. Modell., 1 (2021), 149–169. https://doi.org/10.1016/j.idm.2021.12.004 doi: 10.1016/j.idm.2021.12.004
![]() |
[7] | A. A. Khan, S. Ullah, R. Amin, Optimal control analysis of COVID-19 vaccine epidemic model: a case study, Eur. Phys. J. Plus, 137 (2022). https://doi.org/10.1140/epjp/s13360-022-02365-8 |
[8] |
L. Matrajt, J. Eaton, T. Leung, D. Dimitrov, J. T. Schiffer, D. A. Swan, et al., optimising vaccine allocation for COVID-19 vaccines shows the potential role of single-dose vaccination, Nat. Commun., 12 (2021), 3449. https://doi.org/10.1038/s41467-021-23761-1 doi: 10.1038/s41467-021-23761-1
![]() |
[9] |
M. M. Ojo, T. O. Benson, O. J. Peter, E. F. Doungmo Goufo, Nonlinear optimal control strategies for a mathematical model of COVID-19 and influenza co-infection, Phys. A: Stat. Mech. Appl., 607 (2022), 128173. https://doi.org/10.1016/j.physa.2022.128173 doi: 10.1016/j.physa.2022.128173
![]() |
[10] |
A. Olivares, E. Staffetti, Optimal control-based vaccination and testing strategies for COVID-19, Comput. Methods Programs Biomed., 211 (2021), 106411. https://doi.org/10.1016/j.cmpb.2021.106411 doi: 10.1016/j.cmpb.2021.106411
![]() |
[11] |
M. Urban, J. Jodlowska, J. Balbus, K. Kubica, Vaccination strategies based on a mathematical model of epidemics considering the age structure of the population, WSEAS Trans. Biol Biomed., 21 (2024), 29–39. https://doi.org/10.37394/23208.2024.21.4 doi: 10.37394/23208.2024.21.4
![]() |
[12] |
S. Zhou, S. Zhou, Z. Zheng, J. Lu, optimising spatial allocation of COVID-19 vaccine by agent-based spatiotemporal simulations, Geohealth, 6 (2021), e2021GH000427. https://doi.org/10.1029/2021GH000427 doi: 10.1029/2021GH000427
![]() |
[13] | O. Diekmann J. A. P. Heesterbeek, Mathematical Epidemiology of Infectious Diseases: Model Building, Analysis and Interpretation, John Wiley & Sons, 2000. |
[14] |
T. Britton, F. Ball, P. Trapman, A mathematical model reveals the influence of population heterogeneity on herd immunity to SARS-CoV-2, Science, 369 (2020), 846–849. https://doi.org/10.1126/science.abc6810 doi: 10.1126/science.abc6810
![]() |
[15] |
S. Bentout, A. Tridane, S. Djilali, T. M. Touaoula, Age-structured modeling of COVID-19 epidemic in the USA, UAE and Algeria, Alex. Eng. J., 60 (2021), 401–411. https://doi.org/10.1016/j.aej.2020.08.053 doi: 10.1016/j.aej.2020.08.053
![]() |
[16] |
J. Read, J. Lessler, S. Riley, S. Wang, L. Tan, K. Kwok, et al., Social mixing patterns in rural and urban areas of southern China, Proc. Biol. Sci., 281 (2014), 122. https://doi.org/10.1098/rspb.2014.0268 doi: 10.1098/rspb.2014.0268
![]() |
[17] |
I. Voinsky, G. Baristaite, D. Gurwitz, Effects of age and sex on recovery from COVID-19: Analysis of 5769 Israeli patients, J. Infect., 2 (2020), e102–e103. https://doi.org/10.1016/j.jinf.2020.05.026 doi: 10.1016/j.jinf.2020.05.026
![]() |
[18] |
S. S. Shen-Orr, D. Furman, Variability in the immune system: of vaccine responses and immune states, Curr. Opin. Immunol., 4 (2013), 542–547. https://doi.org/10.1016/j.coi.2013.07.009 doi: 10.1016/j.coi.2013.07.009
![]() |
[19] |
L. L. Dietz, A. K. Juhl, O. S. Søgaard, J. Reekie, H. Nielsen, I. S. Johansen, et al., Impact of age and comorbidities on SARS-CoV-2 vaccine-induced T cell immunity, Commun. Med., 1 (2023), 58. https://doi.org/10.1038/s43856-023-00277-x doi: 10.1038/s43856-023-00277-x
![]() |
[20] |
B. Naffeti, W. Ben Aribi, A. Kebir, M. Diarra, M. Schoenhals, I. Vigan-Womas, et al., Comparative reconstruction of SARS-CoV-2 transmission in three African countries using a mathematical model integrating immunity data, IJID Reg., 10 (2024), 100–107. https://doi.org/10.1016/j.ijregi.2023.11.011 doi: 10.1016/j.ijregi.2023.11.011
![]() |
[21] | A. Bouhali, W. Ben Aribi, A. Kebir, S. Ben Miled, Age optimal vaccination strategy for respiratory infectious disease: a constraint-dependant approach, Authorea Prepr., 2023. https://doi.org/10.22541/au.169571176.62627085/v1 |
[22] |
E. H. Elbasha, A. B. Gumel, Vaccination and herd immunity thresholds in heterogeneous populations, J. Math. Biol., 83 (2021), 73. https://doi.org/10.1007/s00285-021-01686-z doi: 10.1007/s00285-021-01686-z
![]() |
[23] |
K. Hattaf, M. I. El Karimi, A. A. Mohsen, Z. Hajhouji, M. El Younoussi, N. Yousfi, Mathematical modeling and analysis of the dynamics of RNA viruses in presence of immunity and treatment: A case study of SARS-CoV-2, Vaccines, 11 (2023), 2076–393X. https://doi.org/10.3390/vaccines11020201 doi: 10.3390/vaccines11020201
![]() |
[24] |
B. Naffeti, S. Bourdin, W. Ben Aribi, A. Kebir, S. Ben Miled, Spatio-temporal evolution of the COVID-19 across African countries, Front. Public Health, 28 (2022), 1039925. https://doi.org/10.3389/fpubh.2022.1039925 doi: 10.3389/fpubh.2022.1039925
![]() |
[25] |
N. G. Davies, P. Klepac, Y. Liu, K. Prem, M. Jit, R. M. Eggo, Age-dependent effects in the transmission and control of COVID-19 epidemics, Nat. Med., 26 (2020), 1205–1211. https://doi.org/10.1038/s41591-020-0962-9 doi: 10.1038/s41591-020-0962-9
![]() |
[26] | WHO, Global-covid-19-vaccination-mid-2022, 2022. Available from: https://reliefweb.int/report/world/strategy-achieve-global-covid-19-vaccination-mid-2022. |
[27] | W. Fleming, R. Rishel, Deterministic and Stochastic Optimal Control, Springer-Verlag, New York Heidelberg Berlin, 1986. |
[28] | E. Trelat, Contrôle Optimal : Théorie et Applications, Université Pierre et Marie Curie (Paris 6) et Institut Universitaire de France, Paris, 2005. |
[29] | Population Pyramids of the World from 1950 to 2100, Population Pyramid.net, 2020. Available from: https://www.populationpyramid.net/tunisia/2020/. |
[30] | Stratégie Vaccinale Contre la COVID-19 en Tunisie, Report of the Tunisian Ministry of Public Health for COVID-19 Vaccination, 2021. Available from: http://www.santetunisie.rns.tn/images/strategie-vaccination-covid-19.pdf. |
[31] |
K. Prem, A. R. Cook, M. Jit, Projecting social contact matrices in 152 countries using contact surveys and demographic data, PLoS Comput. Biol., 13 (2017), e1005697. https://doi.org/10.1371/journal.pcbi.1005697 doi: 10.1371/journal.pcbi.1005697
![]() |
[32] | WHO, COVID-19 Weekly Epidemiological Update, Available from: https://www.who.int/publications/m/item/weekly-epidemiological-update-on-covid-19—10-august-2023. |
[33] | WHO, WHO COVID-19 dashboard, Available from: https://data.who.int/dashboards/covid19/cases?n = c. |
[34] | Our World in Data, Number of Deaths by Age, World, 2021. Available from: https://ourworldindata.org/grapher/annual-deaths-by-age. |
Parameter Description | Country | ||
Tunisia | Senegal | USA | |
Population size [29] | 11818619 | 16743928 | 331002647 |
Age class distribution Pi [29] | 24, 3%, 21, 3%, | 42, 5%, 27, 3%, | 18%, 20%, |
23, 4%, 17, 7%, | 16, 7%, 8, 6%, | 20%, 19%, | |
10, 2%, 3, 1% | 4%, 0.7% | 16%, 7% | |
Infection rate α1i | 0, 326797 | 0, 326797 | 0, 326797 |
Infection rate α2i | 0,1.α1i | 0,1.α1i | 0,1.α1i |
Infection rate βi | (3/5).α1i | (3/5).α1i | (3/5).α1i |
Infection rate βvi | 0,8.βi | 0,8.βi | 0,8.βi |
Infection rate αv1i | 0,8.α1i | 0,8.α1i | 0,8.α1i |
Infection rate αv2i | 0,8.α2i | 0,8.α2i | 0,8.α2i |
Infection probability among δ | 0.3 | 0.3 | 0.3 |
Incubation rate λ [33] | 0.2 | 0.2 | 0.2 |
Hospitalization rate η [30] | 0.0027,0.0137,0.03, | 0.0027,0.0137,0.0301, | 0.0027,0.0137,0.03005, |
0.0792,0.1585,0.7158 | 0.0792,0.1584,0.7158 | 0.0792,0.1585,0.7158 | |
Infection rate q1 | 0.19999,0.19995,0.19988 | 0.19999,0.19995,0.19988 | 0.19999,0.19995,0.19988 |
0.19968,0.19937,0.19714 | 0.19968,0.19937,0.19714 | 0.19968,0.19937,0.19714 | |
Infection rate q2 | 1.0910−5,5.4610−5,1.20210−4 | 1.0910−5,5.4610−5,1.20210−4 | 1.0910−5,5.4610−5,1.20210−4 |
3.16910−4,6.33910−4,2.863410−3 | 3.16910−4,6.33910−4,2.863410−3 | 3.16910−4,6.33910−4,2.863410−3 | |
Infection rate qv1 | 0.199998,0.199995,0.199988 | 0.199998,0.199995,0.199988 | v |
0.199968,0.199937,0.199714 | 0.199968,0.199937,0.199714 | 0.199968,0.199937,0.199714 | |
Infection rate qv2 | 1.0910−6,5.4610−6,1.20210−5 | 1.0910−6,5.4610−6,1.20210−5 | 1.0910−6,5.4610−6,1.20210−5 |
3.16910−5,6.33910−5,2.863410−4 | 3.16910−5,6.33910−5,2.863410−4 | 3.16910−5,6.33910−5,2.863410−4 | |
Immunity loss rate ψ [33] | 1/180 | 1/180 | 1/180 |
Recovery rate of I1i, r1i [33] | 1/8 | 1/8 | 1/8 |
Recovery rate of I2, r2 [17] | 1/13.5,1/13.5,1/14, | 1/13.5,1/13.5,1/14, | 1/13.5,1/13.5,1/14, |
1/14.5,1/14.5,1/15 | 1/14.5,1/14.5,1/15 | 1/14.5,1/14.5,1/15 | |
Natural mortality rate [34] | 0,0,0.0001,0.0005, | 0,0,0.0001,0.0005, | 0,0,0.0001,0.0005, |
0.034,0.072 | 0.034,0.072 | 0.034,0.072 | |
Basic reproduction number | 2.1 | 2.1 | 2.1 |
Balancing cost factors A1 and A2 | 1000,N/2 | 1000,N/2 | 1000,N/2 |
τmax age-dependent | 0.005,0.005,0.01,0.01,0.05,0.05 | 0.005,0.005,0.01,0.01,0.05,0.05 | 0.005,0.005,0.01,0.01,0.05,0.05 |
Time horizon T | 1 year | 1 year | 1 year |
Parameter description | Country | ||
Tunisia | Senegal | USA | |
Date of first case | Mars 2, 2020 | Mars 2, 2020 | 26 February 2020 |
Date of first vaccination t0 | Mars 13, 2021 | Feb 22, 2021 | 10 December 2020 |
Number of cumulative cases at t0 | 242,124 | 16,529 | 16,217,128 |
Number of active case at t0 | 24,554 | 6451 | 9,669,611 |
Initial condition per country | Tunisia | Senegal | USA |
S(t0) | Pi−(272124)Pi/Pop | Pi−(23529)Pi/Pop | Pi−(25916216)Pi/Pop |
E(t0) | (30000Pi/Pop | (7000Pi/Pop | (10000000Pi/Pop |
I1(t0) | (1−0.02η)(24554)Pi/Pop | (1−0.02η)(6451)Pi/Pop | (1−0.02η)(9669611)Pi/Pop |
I2(t0) | 0.02η(24554)Pi/Pop | 0.02η(6451)Pi/Pop | 0.02η(9669611)Pi/Pop |
R(t0) | 217570Pi/Pop | 10078Pi/Pop | 6246605Pi/Pop |
Sv(t0) | (0, 0, 0, 0, 0, 0) | (0, 0, 0, 0, 0, 0) | (0, 0, 0, 0, 0, 0) |
Ev(t0) | (0, 0, 0, 0, 0, 0) | (0, 0, 0, 0, 0, 0) | (0, 0, 0, 0, 0, 0) |
Iv1(t0) | (0, 0, 0, 0, 0, 0) | (0, 0, 0, 0, 0, 0) | (0, 0, 0, 0, 0, 0) |
Iv2(t0) | (0, 0, 0, 0, 0, 0) | (0, 0, 0, 0, 0, 0) | (0, 0, 0, 0, 0, 0) |
Rv(t0) | (0, 0, 0, 0, 0, 0) | (0, 0, 0, 0, 0, 0) | (0, 0, 0, 0, 0, 0) |
D(t0) | (0, 0, 0, 0, 0, 0) | (0, 0, 0, 0, 0, 0) | (0, 0, 0, 0, 0, 0) |
Parameter Description | Country | ||
Tunisia | Senegal | USA | |
Population size [29] | 11818619 | 16743928 | 331002647 |
Age class distribution Pi [29] | 24, 3%, 21, 3%, | 42, 5%, 27, 3%, | 18%, 20%, |
23, 4%, 17, 7%, | 16, 7%, 8, 6%, | 20%, 19%, | |
10, 2%, 3, 1% | 4%, 0.7% | 16%, 7% | |
Infection rate α1i | 0, 326797 | 0, 326797 | 0, 326797 |
Infection rate α2i | 0,1.α1i | 0,1.α1i | 0,1.α1i |
Infection rate βi | (3/5).α1i | (3/5).α1i | (3/5).α1i |
Infection rate βvi | 0,8.βi | 0,8.βi | 0,8.βi |
Infection rate αv1i | 0,8.α1i | 0,8.α1i | 0,8.α1i |
Infection rate αv2i | 0,8.α2i | 0,8.α2i | 0,8.α2i |
Infection probability among δ | 0.3 | 0.3 | 0.3 |
Incubation rate λ [33] | 0.2 | 0.2 | 0.2 |
Hospitalization rate η [30] | 0.0027,0.0137,0.03, | 0.0027,0.0137,0.0301, | 0.0027,0.0137,0.03005, |
0.0792,0.1585,0.7158 | 0.0792,0.1584,0.7158 | 0.0792,0.1585,0.7158 | |
Infection rate q1 | 0.19999,0.19995,0.19988 | 0.19999,0.19995,0.19988 | 0.19999,0.19995,0.19988 |
0.19968,0.19937,0.19714 | 0.19968,0.19937,0.19714 | 0.19968,0.19937,0.19714 | |
Infection rate q2 | 1.0910−5,5.4610−5,1.20210−4 | 1.0910−5,5.4610−5,1.20210−4 | 1.0910−5,5.4610−5,1.20210−4 |
3.16910−4,6.33910−4,2.863410−3 | 3.16910−4,6.33910−4,2.863410−3 | 3.16910−4,6.33910−4,2.863410−3 | |
Infection rate qv1 | 0.199998,0.199995,0.199988 | 0.199998,0.199995,0.199988 | v |
0.199968,0.199937,0.199714 | 0.199968,0.199937,0.199714 | 0.199968,0.199937,0.199714 | |
Infection rate qv2 | 1.0910−6,5.4610−6,1.20210−5 | 1.0910−6,5.4610−6,1.20210−5 | 1.0910−6,5.4610−6,1.20210−5 |
3.16910−5,6.33910−5,2.863410−4 | 3.16910−5,6.33910−5,2.863410−4 | 3.16910−5,6.33910−5,2.863410−4 | |
Immunity loss rate ψ [33] | 1/180 | 1/180 | 1/180 |
Recovery rate of I1i, r1i [33] | 1/8 | 1/8 | 1/8 |
Recovery rate of I2, r2 [17] | 1/13.5,1/13.5,1/14, | 1/13.5,1/13.5,1/14, | 1/13.5,1/13.5,1/14, |
1/14.5,1/14.5,1/15 | 1/14.5,1/14.5,1/15 | 1/14.5,1/14.5,1/15 | |
Natural mortality rate [34] | 0,0,0.0001,0.0005, | 0,0,0.0001,0.0005, | 0,0,0.0001,0.0005, |
0.034,0.072 | 0.034,0.072 | 0.034,0.072 | |
Basic reproduction number | 2.1 | 2.1 | 2.1 |
Balancing cost factors A1 and A2 | 1000,N/2 | 1000,N/2 | 1000,N/2 |
τmax age-dependent | 0.005,0.005,0.01,0.01,0.05,0.05 | 0.005,0.005,0.01,0.01,0.05,0.05 | 0.005,0.005,0.01,0.01,0.05,0.05 |
Time horizon T | 1 year | 1 year | 1 year |
Parameter description | Country | ||
Tunisia | Senegal | USA | |
Date of first case | Mars 2, 2020 | Mars 2, 2020 | 26 February 2020 |
Date of first vaccination t0 | Mars 13, 2021 | Feb 22, 2021 | 10 December 2020 |
Number of cumulative cases at t0 | 242,124 | 16,529 | 16,217,128 |
Number of active case at t0 | 24,554 | 6451 | 9,669,611 |
Initial condition per country | Tunisia | Senegal | USA |
S(t0) | Pi−(272124)Pi/Pop | Pi−(23529)Pi/Pop | Pi−(25916216)Pi/Pop |
E(t0) | (30000Pi/Pop | (7000Pi/Pop | (10000000Pi/Pop |
I1(t0) | (1−0.02η)(24554)Pi/Pop | (1−0.02η)(6451)Pi/Pop | (1−0.02η)(9669611)Pi/Pop |
I2(t0) | 0.02η(24554)Pi/Pop | 0.02η(6451)Pi/Pop | 0.02η(9669611)Pi/Pop |
R(t0) | 217570Pi/Pop | 10078Pi/Pop | 6246605Pi/Pop |
Sv(t0) | (0, 0, 0, 0, 0, 0) | (0, 0, 0, 0, 0, 0) | (0, 0, 0, 0, 0, 0) |
Ev(t0) | (0, 0, 0, 0, 0, 0) | (0, 0, 0, 0, 0, 0) | (0, 0, 0, 0, 0, 0) |
Iv1(t0) | (0, 0, 0, 0, 0, 0) | (0, 0, 0, 0, 0, 0) | (0, 0, 0, 0, 0, 0) |
Iv2(t0) | (0, 0, 0, 0, 0, 0) | (0, 0, 0, 0, 0, 0) | (0, 0, 0, 0, 0, 0) |
Rv(t0) | (0, 0, 0, 0, 0, 0) | (0, 0, 0, 0, 0, 0) | (0, 0, 0, 0, 0, 0) |
D(t0) | (0, 0, 0, 0, 0, 0) | (0, 0, 0, 0, 0, 0) | (0, 0, 0, 0, 0, 0) |