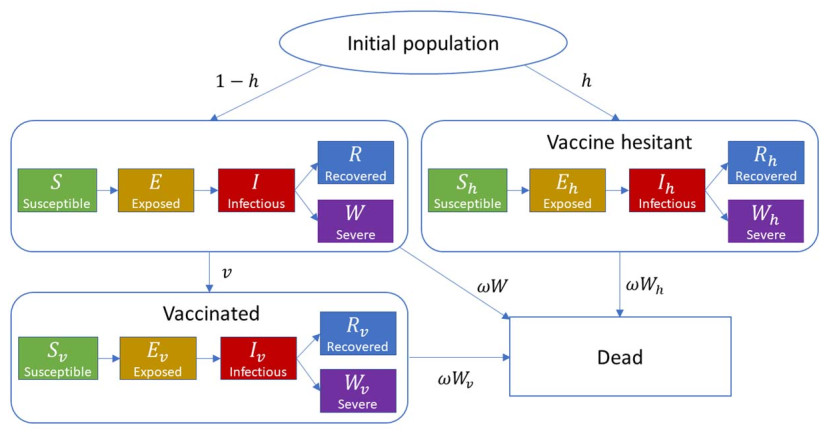
In this paper, we construct an age-structured epidemic model to analyze the optimal vaccine allocation strategy in an epidemic. We focus on two topics: the first one is the optimal vaccination interval between the first and second doses, and the second one is the optimal vaccine allocation ratio between young and elderly people. On the first topic, we show that the optimal interval tends to become longer as the relative efficacy of the first dose to the second dose (RE) increases. On the second topic, we show that the heterogeneity in the age-dependent susceptibility (HS) affects the optimal allocation ratio between young and elderly people, whereas the heterogeneity in the contact frequency among different age groups (HC) tends to affect the effectiveness of the vaccination campaign. A counterfactual simulation suggests that the epidemic wave in the summer of 2021 in Japan could have been greatly mitigated if the optimal vaccine allocation strategy had been taken.
Citation: Toshikazu Kuniya, Taisuke Nakata, Daisuke Fujii. Optimal vaccine allocation strategy: Theory and application to the early stage of COVID-19 in Japan[J]. Mathematical Biosciences and Engineering, 2024, 21(6): 6359-6371. doi: 10.3934/mbe.2024277
[1] | Tetsuro Kobayashi, Hiroshi Nishiura . Prioritizing COVID-19 vaccination. Part 2: Real-time comparison between single-dose and double-dose in Japan. Mathematical Biosciences and Engineering, 2022, 19(7): 7410-7424. doi: 10.3934/mbe.2022350 |
[2] | Pannathon Kreabkhontho, Watchara Teparos, Thitiya Theparod . Potential for eliminating COVID-19 in Thailand through third-dose vaccination: A modeling approach. Mathematical Biosciences and Engineering, 2024, 21(8): 6807-6828. doi: 10.3934/mbe.2024298 |
[3] | Giulia Luebben, Gilberto González-Parra, Bishop Cervantes . Study of optimal vaccination strategies for early COVID-19 pandemic using an age-structured mathematical model: A case study of the USA. Mathematical Biosciences and Engineering, 2023, 20(6): 10828-10865. doi: 10.3934/mbe.2023481 |
[4] | Amira Bouhali, Walid Ben Aribi, Slimane Ben Miled, Amira Kebir . Impact of immunity loss on the optimal vaccination strategy for an age-structured epidemiological model. Mathematical Biosciences and Engineering, 2024, 21(6): 6372-6392. doi: 10.3934/mbe.2024278 |
[5] | Eunha Shim . Optimal strategies of social distancing and vaccination against seasonal influenza. Mathematical Biosciences and Engineering, 2013, 10(5&6): 1615-1634. doi: 10.3934/mbe.2013.10.1615 |
[6] | Yuto Omae, Yohei Kakimoto, Makoto Sasaki, Jun Toyotani, Kazuyuki Hara, Yasuhiro Gon, Hirotaka Takahashi . SIRVVD model-based verification of the effect of first and second doses of COVID-19/SARS-CoV-2 vaccination in Japan. Mathematical Biosciences and Engineering, 2022, 19(1): 1026-1040. doi: 10.3934/mbe.2022047 |
[7] | Ayako Suzuki, Hiroshi Nishiura . Transmission dynamics of varicella before, during and after the COVID-19 pandemic in Japan: a modelling study. Mathematical Biosciences and Engineering, 2022, 19(6): 5998-6012. doi: 10.3934/mbe.2022280 |
[8] | Yuta Okada, Hiroshi Nishiura . Vaccine-induced reduction of COVID-19 clusters in school settings in Japan during the epidemic wave caused by B.1.1.529 (Omicron) BA.2, 2022. Mathematical Biosciences and Engineering, 2024, 21(9): 7087-7101. doi: 10.3934/mbe.2024312 |
[9] | Fang Wang, Lianying Cao, Xiaoji Song . Mathematical modeling of mutated COVID-19 transmission with quarantine, isolation and vaccination. Mathematical Biosciences and Engineering, 2022, 19(8): 8035-8056. doi: 10.3934/mbe.2022376 |
[10] | Ahmed Alshehri, Saif Ullah . A numerical study of COVID-19 epidemic model with vaccination and diffusion. Mathematical Biosciences and Engineering, 2023, 20(3): 4643-4672. doi: 10.3934/mbe.2023215 |
In this paper, we construct an age-structured epidemic model to analyze the optimal vaccine allocation strategy in an epidemic. We focus on two topics: the first one is the optimal vaccination interval between the first and second doses, and the second one is the optimal vaccine allocation ratio between young and elderly people. On the first topic, we show that the optimal interval tends to become longer as the relative efficacy of the first dose to the second dose (RE) increases. On the second topic, we show that the heterogeneity in the age-dependent susceptibility (HS) affects the optimal allocation ratio between young and elderly people, whereas the heterogeneity in the contact frequency among different age groups (HC) tends to affect the effectiveness of the vaccination campaign. A counterfactual simulation suggests that the epidemic wave in the summer of 2021 in Japan could have been greatly mitigated if the optimal vaccine allocation strategy had been taken.
In the early stage of the coronavirus disease outbreak 2019 (COVID-19), people had to rely on non-pharmaceutical interventions (NPIs) such as lockdown. Although lockdowns were effective in temporarily suppressing the epidemic [1], they brought serious damage to the socio-economic systems in many countries [2]. Vaccination is thought to be one of the most cost-effective pharmaceutical interventions and is often regarded as a game-changer that has returned our lives to less restrictive ones.
In the early stages of COVID-19, the number of available vaccines was limited in many countries. Hence, designing how to allocate them from the viewpoint of minimizing the epidemic damage was important [3]. To design the optimal vaccine allocation, one can consider two ways of optimization: the first is to optimize the interval between the first and second doses, and the second is to optimize the ratio of allocation among different age groups. In this study, we deal with these two optimization problems by using a mathematical model and construct a general theoretical framework to assess the validity of vaccine allocation strategies in cases of epidemics.
For COVID-19, the vaccination interval between the first and second doses was set at 3–4 weeks in many countries, including the US. In the UK, this interval was set at 12 weeks in order to distribute the first doses to many people quickly [4]. Some papers constructed mathematical models to study how the vaccination interval affects the epidemic size [5,6]. In [5], it was shown that the relative efficacy (RE) of the first dose to the second one plays an important role in determining the optimal interval to minimize the total number of deaths. In [6], it was shown that the 12-week interval was highly beneficial to preventing the disease. A clinical study [7] showed that extending the vaccination interval from 3 weeks to 11–12 weeks boosts the peak antibody response by 3.5-fold in elderly people. In this paper, we propose a new age-structured mathematical model to investigate the optimal interval to minimize the total number of cases, deaths, and deaths weighted by the average life expectancy. By using this model, we will show that the long interval as adopted in the UK can be justified if the RE is sufficiently high.
The ratio of vaccine allocation among different age groups is also important because the symptoms and mortality of the disease and the pattern of social interactions during the epidemic highly depend on the individual's age. Thus, some previous studies have focused on this topic [8,9,10,11,12]. For COVID-19, prioritizing elderly people seems optimal because the mortality of COVID-19 is much higher in elderly people [9]. However, prioritizing young people also seems optimal because they tend to spread the infection to many people [10]. Some other studies focused on optimal allocation among not only age groups but also geographical regions [11] and occupations [12]. In this paper, our model will be applied to investigate the optimal ratio of allocation among young and elderly people. We will show that, to reduce the number of deaths as well as the number of deaths weighted by the average life expectancy, prioritizing young people is optimal if the heterogeneity in the age-dependent susceptibility (HS) is high, whereas prioritizing elderly people is optimal if the HS is low. In addition, we will show that the heterogeneity in the contact frequency among different age classes (HC) affects the effectiveness of the vaccination campaign for a fixed basic reproduction number [13].
The organization of this paper is as follows: in Section 2, we give an outline of our mathematical model (see Supplementary for details). We formulate an age-structured susceptible-exposed-infectious-recovered (SEIR) model with a severe population (W). The vaccine efficacy is considered by introducing the class-age structure into the vaccinated populations. In Section 3, we investigate the optimal vaccination interval between the first and second doses. In Section 4, we investigate the optimal vaccine allocation between young and elderly age groups. In Section 5, we perform a counterfactual simulation that shows how the total number of COVID-19-related deaths in Japan from May 15, 2021 to November 30, 2021 could have been reduced if the optimal vaccine strategy had been taken. Finally, Sections 6 and 7 are devoted to the discussion and conclusions, respectively.
Let S,E,I,R and W denote the susceptible, exposed, infectious, recovered, and severe populations, respectively. Let h and v be subscripts representing the vaccine hesitant and vaccinated, respectively. Let t,a and τ denote the time, chronological age, and vaccine age (time elapsed since the first vaccination shot), respectively. For example, Sv(t,a,τ) represents the susceptible population of age a at time t with vaccine age τ, and Ih(t,a) represents the infectious population with vaccine hesitancy of age a at time t. The transfer diagram of our model is shown in Figure 1.
For the full details of our model, see Supplementary. In our model, the units of time and vaccine age are set as 1 day, and the unit of age is set as 1 year. All independent variables t,a and τ are continuous and take values in [0,tmax], [0,amax] and [0,τmax], respectively. We assume tmax=τmax=232 so that t=0 corresponds to April 12, 2021, which is the day when the vaccination program for COVID-19 started in Japan, and t=232 corresponds to November 30, 2021, which is the last day when only the first and second doses are distributed in Japan1. We assume amax=100. To run the simulation program, we use the standard Euler forward method (see Figure S10 in Supplementary for the main part of our MATLAB code).
1 Of course, by changing parameters, our model can be applied to other cases not restricted to Japan.
The force of infection in our model is given by
λ(t,a)=∫amax0β(a,b)[I(t,b)+Ih(t,b)+∫τmax0Iv(t,b,τ)dτ]db, |
where β(a,b) denotes the transmission function between susceptible individuals aged a and infectious individuals aged b. We assume that β(a,b) is expressed as
β(a,b)=κβ1(a)β2(a−b), |
where κ is a scaling parameter adjusted to attain a fixed basic reproduction number R0 for different β1 and β2. β1=β1(a) is the susceptibility of those aged a, and β2=β2(x) is the contact frequency among individuals whose age difference is x. The HS is quantified by β1 as shown in Figure 2 (left). We assume that β1 is higher for young people than for elderly people aged over 65. We adopt this assumption because elderly people may be more likely to behave carefully and reduce their contact opportunities, taking into account the fact that the mortality rate of COVID-19 is higher for elderly people than for young people. The HC is quantified by β2 as shown in Figure 2 (right). We assume that β2(x) has five peaks at x=0,±30,±60. This assumption means that the contact frequency across (ⅰ) children, (ⅱ) their parents, and (ⅲ) grandparents is higher than for other pairs. For the exact definition of β1 and β2, see Supplementary.
We consider three parameter configurations regarding β1 and β2. They are shown in three lines in each panel of Figure 2. The relative value of β1 for young people (to that for elderly people) is larger in the "high HS" case than in the "low HS" case. People become more likely to contact those with similar ages—that is, the mixing becomes more heterogeneous—in the "high HC" case than in the "low HC" case.
The vaccine efficacies to reduce infection and mortality risks are represented by σ(τ)∈[0,1] and δ(τ)∈[0,1], respectively. They are assumed to be functions of vaccine age τ, and reduce the force of infection λ(t,a) and the ratio d(a) of which an infected individual becomes severe to [1−σ(τ)]λ(t,a) and [1−δ(τ)]d(a), respectively. Specifically, they are defined as follows:
σ(τ)={0,0≤τ<14,σ1[1−k(τ−14)],14≤τ<T,σ2[1−k(τ−T)],τ≥T,δ(τ)={0,0≤τ<14,δ1[1−k(τ−14)],14≤τ<T,δ2[1−k(τ−T)],τ≥T, |
where σi and δi(i=1,2) denote the efficacies of the i-th vaccination to reduce the infection and mortality risks, respectively. k denotes the waning rate of vaccine-induced immunity, and T denotes the length of the vaccination interval between the first and second doses. k is fixed as 1/600 so that the efficacies decrease to their half after about 300 days have passed [14]. σi, δi(i=1,2) and T are varied in the subsequent sections to discuss the optimal strategy.
The per capita rate of vaccination in our model is defined by v=v(t,a)=v1(t)v2(a), where v1(t) is a value estimated by using the vaccination data in Japan [15]. v2(a) is a function defined by
v2(a)={0,0≤a<18,1−u,18≤a<65,u,a≥65, |
where u is the proportion of vaccine allocation to those aged over 65. Thus, a higher u means prioritizing the elderly. In the subsequent sections, u is also varied to investigate the optimal allocation ratio.
In this section, varying the vaccination interval between the first and second doses from 3 weeks (T=21) to 12 weeks (T=84), we investigate the optimal interval that minimizes the total number of cases, deaths, and deaths weighted by the average life expectancy. We assume that the basic reproduction number is fixed as R0=1.5, a sensible number for a disease like pandemic influenza [16] and set both of the HS and HC to medium (Figure 2). In reality, the contact matrix evolved over time, reflecting changes in policies and behaviors, but we abstracted from such time-variation in our analysis. We compare two cases where the allocation ratio is u=0.1 (prioritizing young people) and u=0.9 (prioritizing elderly people). To consider the relative efficacy (RE) of the first dose compared to the second one, we assume that σ2=δ2=1, and compare three cases where σ1=δ1=0.2(RE=0.2);0.5(RE=0.5);0.8(RE=0.8).
Figure 3 shows that the RE plays a central role in determining the optimal interval. In Figure 3, irrespective of the allocation ratio u, the optimal intervals for all objectives (cases, deaths, and deaths weighted by the average life expectancy) tend to become longer as the RE increases. We can interpret this as follows: if the RE is low, then the first dose is less effective, and thus, prioritizing the rollout of the second doses by shortening the interval is optimal; if the RE is high, then the first dose is sufficiently effective, and thus, prioritizing the rollout of the first doses by enlarging the interval is optimal.
We next consider a more special case where the vaccine efficacies are selected for vaccines of Pfizer and AstraZeneca. By taking the mean of the data in [17], we set
(σ1,σ2,δ1,δ2)={(0.63,0.90,0.80,0.94),Pfizer,(0.62,0.64,0.80,0.85),AstraZeneca. | (1) |
In both of these two cases, the long interval is optimal to maximize the reduction ratio of all objectives (Figure 4). This result suggests that the 12-week interval taken in the UK was reasonable. In addition, we can see from Figure 4 that the optimal intervals for Pfizer are shorter than those for AstraZeneca, reflecting the fact that the RE of AstraZeneca is higher than that of Pfizer.
In this section, varying the ratio u of vaccine allocation to the elderly people from 0.1 to 0.9, we investigate the optimal ratio that minimizes the total number of cases, deaths, and deaths weighted by the average life expectancy. We fix the basic reproduction number as R0=1.5, as in the previous section, and the vaccination interval between the first and second doses as 3 weeks. The vaccine efficacy is chosen as for Pfizer in Eq (1). We vary the HS and the HC (Figure 2), and investigate how they affect the optimal allocation ratio.
From Figure 5, A–C and Table 1, we see that, to reduce the total number of cases, prioritizing young people (u=0.1) is always optimal. On the other hand, from Figure 5, D–I, and Table 1, we see that the optimal ratio to maximize the reduction ratio of deaths and deaths weighted by the average life expectancy is more sensitive to the HS: if HS is high, then prioritizing young people (u=0.1) is optimal, whereas if HS is medium or low, then prioritizing elderly people (u=0.9) is optimal. This seems natural because if young people are more likely to be infected (the HS is high), then prioritizing them becomes optimal to stop the disease spread. If the HS is low, then prioritizing elderly people is optimal because they have a high mortality rate for COVID-19. In addition, from Figure 5 and Table 1, we can see that the HC does not affect the optimal allocation ratio in all cases. However, the effectiveness of the vaccination is reduced as the HC increases. From this result, we can conjecture that the vaccination program is more effective in a population well-mixed among different age groups. Here, note that the basic reproduction number R0 is fixed and just the contact frequency is changed in our simulation (see Supplementary for how to fix R0).
object | (HS, HC) | u in optimal | reduction ratio in optimal (%) |
cases | (high, high) | 0.1 (young) | 48.63 |
cases | (high, medium) | 0.1 (young) | 62.58 |
cases | (high, low) | 0.1 (young) | 66.02 |
cases | (medium, high) | 0.1 (young) | 52.04 |
cases | (medium, medium) | 0.1 (young) | 62.99 |
cases | (medium, low) | 0.1 (young) | 65.84 |
cases | (low, high) | 0.1 (young) | 53.12 |
cases | (low, medium) | 0.1 (young) | 62.92 |
cases | (low, low) | 0.1 (young) | 65.26 |
deaths | (high, high) | 0.1 (young) | 62.89 |
deaths | (high, medium) | 0.1 (young) | 69.67 |
deaths | (high, low) | 0.1 (young) | 70.76 |
deaths | (medium, high) | 0.9 (elderly) | 77.39 |
deaths | (medium, medium) | 0.9 (elderly) | 80.36 |
deaths | (medium, low) | 0.9 (elderly) | 81.33 |
deaths | (low, high) | 0.9 (elderly) | 81.21 |
deaths | (low, medium) | 0.9 (elderly) | 82.38 |
deaths | (low, low) | 0.9 (elderly) | 82.77 |
weighted deaths | (high, high) | 0.1 (young) | 64.25 |
weighted deaths | (high, medium) | 0.1 (young) | 71.56 |
weighted deaths | (high, low) | 0.1 (young) | 72.72 |
weighted deaths | (medium, high) | 0.9 (elderly) | 71.87 |
weighted deaths | (medium, medium) | 0.9 (elderly) | 76.37 |
weighted deaths | (medium, low) | 0.9 (elderly) | 77.90 |
weighted deaths | (low, high) | 0.9 (elderly) | 76.98 |
weighted deaths | (low, medium) | 0.9 (elderly) | 79.23 |
weighted deaths | (low, low) | 0.9 (elderly) | 80.08 |
In this section, fitting our model to the real data of reported cases in Japan from April 12, 2021 to November 30, 2021 [18], we estimate the time-varying transmission rate. Specifically, we compute the transmission rate on each day that minimizes the sum of the squares error between the real data of the newly reported cases in the past 7 days and its simulation counterparts. To the best of our knowledge, no previous study has used a similar method. In the estimation, we made the following assumptions (see Supplementary for more details):
● The HS and the HC are medium;
● The vaccination interval is 3 weeks;
● The vaccine allocation ratio is u=0.9 (prioritizing elderly people);
● The detection ratio (the ratio at which a newly infected individual is finally reported) is 0.5.
We then change the vaccination interval and the allocation ratio and investigate how the total number of deaths could be reduced in the optimal case. The curve fitted to the daily number of newly reported deaths is shown in Figure 6, bottom (see the red curve). In this case, the total number of deaths is 8,793. On the other hand, our simulation shows that the total number of deaths is minimized when the vaccination interval is 9 weeks and the allocation ratio is u=0.9 (see Figure 6, top). If such an optimal strategy is taken, then the total number of deaths is reduced to 7,176. In particular, the blue curve in the bottom panel of Figure 6 suggests that the epidemic wave of August 2021 could have been avoided if the optimal strategy had been taken.
In this paper, we have proposed an age-structured epidemic model and investigated the optimal vaccination interval between the first and second doses and the optimal vaccine allocation among young and elderly people. Although we used the data on COVID-19 in Japan in this paper, our model can be applied to various infectious diseases in countries not limited to Japan. The methodology constructed in this paper would help us to design an appropriate vaccine rollout program promptly in case of a future pandemic.
Our result on the optimal interval between the first and second doses has indicated that the RE plays the central role in determining the optimal interval: the short interval is optimal if the RE is low, whereas the long interval is optimal if the RE is high. This result is in line with the previous result in [5] that a longer interval can be optimal to minimize the number of deaths if the first dose presents a higher level of relative efficacy in the case of limited vaccine supply.
Moreover, for both cases of the Pfizer and AstraZeneca vaccines, our simulation has suggested that the optimal interval is longer or equal to 8 weeks. More precisely, an 8-9 weeks interval can be optimal for the Pfizer vaccine, and a 10-12 weeks interval can be optimal for the AstraZeneca vaccine to reduce the number of cases and deaths as well as the number of deaths weighted by the average life expectancy. This result is in line with the previous result in [6] for the data in the UK that the 12-week interval can be highly beneficial to reduce the number of hospital admissions and deaths. Hence, the 12-week interval taken in the UK for COVID-19 seems sensible. In the event of a future pandemic, it is important to have accurate information on vaccine efficacy to design efficient vaccine allocation strategies. If the number of available vaccines is limited and the efficacy of the first dose is not low, then it would be worth considering prolonging the vaccination interval.
Our result on the vaccine allocation among young and elderly people has shown that the optimal allocation ratio to minimize the number of deaths as well as the number of deaths weighted by the average life expectancy depends, importantly, on the HS. Prioritizing young people can be justified if the HS is high; that is, young people are more likely to be infected as they tend to go out more. In [10], it was shown that prioritizing high-transmission (younger) age groups can minimize the number of deaths if the vaccine efficacy is higher than 60%. Our result is consistent with the previous result in [10] because the vaccine efficacy in our simulation is higher than 60% (see Eq (1)). Our result has also shown that the effectiveness of the vaccination campaign increases as the HC decreases. This result has suggested that the vaccination program can be more effective in a population well-mixed among different age groups.
Our counterfactual simulation has suggested that the epidemic wave in the summer of 2021 in Japan could have been avoided if the optimal vaccine strategy had been taken. In our simulation, we assumed that the HS and HC are medium and the detection ratio is 0.5. Comparing the number of deaths in simulation for different vaccination intervals and allocation ratios, our optimal strategy to minimize the number of deaths recommended the 9-week interval and prioritizing elderly people (u=0.9).
We end our discussion by pointing out several limitations of our study.
First, as in any simulation study, the optimal strategy would depend on the assumed parameter values. For example, the number of available vaccines would affect the optimal interval because if there are many vaccines so that all people can get vaccinated twice soon, then shortening the interval to prioritize the second shot would be optimal.
Second, we focus on the optimal allocation in a short time period (232 days from April 12, 2021, to November 30, 2021 in Japan) for the sake of simplicity. An extended optimization over a longer time period could be useful in designing a suitable vaccination strategy, even though we might have to take into account the effects of booster shots and the simulation might have to become more complex.
Third, we abstracted from how the vaccination interval affects the immune response. However, as a clinical study [7] suggested, a long interval could help increase the peak of the antibody response. Thus, the long vaccination interval could be justified from not only mathematical but also clinical points of view.
Fourth, we estimated the infection rate in our counterfactual simulation for Japan in 2021 using data from a fixed past period. If we had taken an alternative vaccination strategy and the number of reported cases and deaths had changed as a result, people's behavior could have also changed. In general, our assumption that the detection rate of infection was fixed over time is a useful starting point for analysis, but the infection rate can change according to policies, social norms, seasonality, and virus mutation.
The conclusions of this study are as follows:
● We have constructed an age-structured epidemic model to evaluate the vaccination interval between the first and second doses and the vaccine allocation strategy between young and elderly age groups.
● The RE plays an important role in determining the optimal vaccination interval. The optimal interval tends to become longer as the RE increases.
● The HS tends to affect the optimal allocation between young and elderly people. On the other hand, the HC tends to affect the effectiveness of the vaccination campaign.
● The counterfactual simulation for COVID-19 in Japan in 2021 has indicated that the epidemic wave in the summer of 2021 in Japan could have been avoided if the optimal vaccine allocation strategy had been taken.
The authors declare they have not used Artificial Intelligence (AI) tools in the creation of this article.
Toshikazu Kuniya is supported by JSPS Grant-in-Aid for Scientific Research (KAKENHI), Project Numbers 19K14594, 23K03214, and JST, PREST Grant Number JPMJPR23R5, Japan. Taisuke Nakata is supported by JSPS Grant-in-Aid for Scientific Research (KAKENHI), Project Number 22H04927, the Research Institute of Science and Technology for Society at the Japan Science and Technology Agency, the Center for Advanced Research in Finance at the University of Tokyo.
The authors declare there is no conflict of interest.
[1] |
S. Flaxman, S. Mishra, A. Gandy, H. J. T. Unwin, T. A. Mellan, H. Coupland, et al., Estimating the effects of non-pharmaceutical interventions on COVID-19 in Europe, Nature, 584 (2020), 257–261. https://doi.org/10.1038/s41586-020-2405-7 doi: 10.1038/s41586-020-2405-7
![]() |
[2] | M. Nicola, Z. Alsafi, C. Sohrabi, A. Kerwan, A. Al-Jabir, C. Iosifidis, et al., The socio-economic implications of the coronavirus pandemic (COVID-19): A review, Int. J. Surgery, 78 (2020), 185–193. https://doi.org/10.1016%2Fj.ijsu.2020.04.018 |
[3] | World Health Organization, WHO SAGE Roadmap for prioritizing uses of COVID-19 vaccines in the context of limited supply, 2020. Available from: https://www.who.int/docs/default-source/immunization/sage/covid/sage-prioritization-roadmap-covid19-vaccines.pdf. |
[4] | Joint Committee on Vaccination and Immunisation, Prioritising the first COVID-19 vaccine dose: JCVI statement, 2021. Available from: https://www.gov.uk/government/publications/prioritising-the-first-covid-19-vaccine-dose-jcvi-statement. |
[5] |
L. S. Ferreira, O. Canton, R. L. P. Silva, S. Poloni, V. Sudbrack, M. E. Borges, et al., Assessing the best time interval between doses in a two-dose vaccination regimen to reduce the number of deaths in an ongoing epidemic of SARS-CoV-2, PLoS Comput. Biol., 18 (2022), e10009978. https://doi.org/10.1371/journal.pcbi.1009978 doi: 10.1371/journal.pcbi.1009978
![]() |
[6] |
M. J. Keeling, S. Moore, B. S. Penman, E. M. Hill, The impacts of SARS-CoV-2 vaccine dose separation and targeting on the COVID-19 epidemic in England, Nat. Commun., 14 (2023), 740. https://doi.org/10.1038/s41467-023-35943-0 doi: 10.1038/s41467-023-35943-0
![]() |
[7] |
H. Parry, R. Bruton, C. Stephens, C. Bentley, K. Brown, G. Amirthalingam, et al., Extended interval BNT162b2 vaccination enhances peak antibody generation, NPJ Vaccines, 7 (2022), 14. https://doi.org/10.1038/s41541-022-00432-w doi: 10.1038/s41541-022-00432-w
![]() |
[8] |
K. M. Bubar, K. Reinholt, S. M. Kissler, M. Lipsitch, S. Cobey, Y. H. Grad, et al., Model-informed COVID-19 vaccine prioritization strategies by age and serostatus, Science, 371 (2021), 916–921. https://doi.org/10.1126/science.abe6959 doi: 10.1126/science.abe6959
![]() |
[9] |
B. H. Foy, B. Wahl, K. Mehta, A. Shet, G. I. Menon, C. Britto, Comparing COVID-19 vaccine allocation strategies in India: a mathematical modelling study, Int. J. Infect. Dis., 103 (2021), 431–438. https://doi.org/10.1016/j.ijid.2020.12.075 doi: 10.1016/j.ijid.2020.12.075
![]() |
[10] |
L. Matrajt, J. Eaton, T. Leung, E. R. Brown, Vaccine optimization for COVID-19: who to vaccinate first?, Sci. Adv., 7 (2021), eabf1374. https://doi.org/10.1126/sciadv.abf1374 doi: 10.1126/sciadv.abf1374
![]() |
[11] |
J. Molla, A. P. L. Chávez, T. Hiraoka, T. Ala-Nissila, M. Kivelä , L. Leskelä , Adaptive and optimized COVID-19 vaccination strategies across geographical regions and age groups, PLoS Comput. Biol., 18 (2022), e1009974. https://doi.org/10.1371/journal.pcbi.1009974 doi: 10.1371/journal.pcbi.1009974
![]() |
[12] |
A. Babus, S. Das, S. Lee, The optimal allocation of COVID-19 vaccines, Econom. Letters, 224 (2023) 111008. https://doi.org/10.1016/j.econlet.2023.111008 doi: 10.1016/j.econlet.2023.111008
![]() |
[13] |
O. Diekmann, J. A. P. Heesterbeek, J. A. J. Metz, On the definition and the computation of the basic reproduction ratio R0 in models for infectious diseases in heterogeneous populations, J. Math. Biol., 28 (1990), 365–382. https://doi.org/10.1007/BF00178324 doi: 10.1007/BF00178324
![]() |
[14] |
S. Kodera, E. A. Rashed, A. Hirata, Estimation of real-world vaccination effectiveness of mRNA COVID-19 vaccines against Delta and Omicron variants in Japan, Vaccines, 10 (2022), 430. https://doi.org/10.3390/vaccines10030430 doi: 10.3390/vaccines10030430
![]() |
[15] | Digital Agency, Vaccination Record System (VRS). Available from: https://info.vrs.digital.go.jp/opendata/ (Japanese). |
[16] |
C. Fraser, C. A. Donnelly, S. Cauchemez, W. P. Hanage, M. D. Kerkhove, T. D. Hollingsworth, et al., Pandemic potential of a strain of influenza A (H1N1): early findings, Science, 324 (2009) 1557–1561. https://doi.org/10.1126/science.1176062 doi: 10.1126/science.1176062
![]() |
[17] | SPI-M-O, Summary of further modelling of easing restrictions – Roadmap Step 2 (2021). Available from: https://assets.publishing.service.gov.uk/government/uploads/system/uploads/attachment_data/file/975909/S1182_SPI-M-O_Summary_of_modelling_of_easing_roadmap_step_2_restrictions.pdf. |
[18] | Ministry of Health, Labour and Welfare of Japan, Visualizing the data: information on COVID-19 infections. Available from: https://covid19.mhlw.go.jp/en/. |
![]() |
![]() |
1. | Asako Chiba, Taisuke Nakata, Thuy Linh Nguyen, Reo Takaku, COVID-19 risk perceptions in Japan: a cross-sectional study, 2024, 14, 2045-2322, 10.1038/s41598-024-67741-z |
object | (HS, HC) | u in optimal | reduction ratio in optimal (%) |
cases | (high, high) | 0.1 (young) | 48.63 |
cases | (high, medium) | 0.1 (young) | 62.58 |
cases | (high, low) | 0.1 (young) | 66.02 |
cases | (medium, high) | 0.1 (young) | 52.04 |
cases | (medium, medium) | 0.1 (young) | 62.99 |
cases | (medium, low) | 0.1 (young) | 65.84 |
cases | (low, high) | 0.1 (young) | 53.12 |
cases | (low, medium) | 0.1 (young) | 62.92 |
cases | (low, low) | 0.1 (young) | 65.26 |
deaths | (high, high) | 0.1 (young) | 62.89 |
deaths | (high, medium) | 0.1 (young) | 69.67 |
deaths | (high, low) | 0.1 (young) | 70.76 |
deaths | (medium, high) | 0.9 (elderly) | 77.39 |
deaths | (medium, medium) | 0.9 (elderly) | 80.36 |
deaths | (medium, low) | 0.9 (elderly) | 81.33 |
deaths | (low, high) | 0.9 (elderly) | 81.21 |
deaths | (low, medium) | 0.9 (elderly) | 82.38 |
deaths | (low, low) | 0.9 (elderly) | 82.77 |
weighted deaths | (high, high) | 0.1 (young) | 64.25 |
weighted deaths | (high, medium) | 0.1 (young) | 71.56 |
weighted deaths | (high, low) | 0.1 (young) | 72.72 |
weighted deaths | (medium, high) | 0.9 (elderly) | 71.87 |
weighted deaths | (medium, medium) | 0.9 (elderly) | 76.37 |
weighted deaths | (medium, low) | 0.9 (elderly) | 77.90 |
weighted deaths | (low, high) | 0.9 (elderly) | 76.98 |
weighted deaths | (low, medium) | 0.9 (elderly) | 79.23 |
weighted deaths | (low, low) | 0.9 (elderly) | 80.08 |
object | (HS, HC) | u in optimal | reduction ratio in optimal (%) |
cases | (high, high) | 0.1 (young) | 48.63 |
cases | (high, medium) | 0.1 (young) | 62.58 |
cases | (high, low) | 0.1 (young) | 66.02 |
cases | (medium, high) | 0.1 (young) | 52.04 |
cases | (medium, medium) | 0.1 (young) | 62.99 |
cases | (medium, low) | 0.1 (young) | 65.84 |
cases | (low, high) | 0.1 (young) | 53.12 |
cases | (low, medium) | 0.1 (young) | 62.92 |
cases | (low, low) | 0.1 (young) | 65.26 |
deaths | (high, high) | 0.1 (young) | 62.89 |
deaths | (high, medium) | 0.1 (young) | 69.67 |
deaths | (high, low) | 0.1 (young) | 70.76 |
deaths | (medium, high) | 0.9 (elderly) | 77.39 |
deaths | (medium, medium) | 0.9 (elderly) | 80.36 |
deaths | (medium, low) | 0.9 (elderly) | 81.33 |
deaths | (low, high) | 0.9 (elderly) | 81.21 |
deaths | (low, medium) | 0.9 (elderly) | 82.38 |
deaths | (low, low) | 0.9 (elderly) | 82.77 |
weighted deaths | (high, high) | 0.1 (young) | 64.25 |
weighted deaths | (high, medium) | 0.1 (young) | 71.56 |
weighted deaths | (high, low) | 0.1 (young) | 72.72 |
weighted deaths | (medium, high) | 0.9 (elderly) | 71.87 |
weighted deaths | (medium, medium) | 0.9 (elderly) | 76.37 |
weighted deaths | (medium, low) | 0.9 (elderly) | 77.90 |
weighted deaths | (low, high) | 0.9 (elderly) | 76.98 |
weighted deaths | (low, medium) | 0.9 (elderly) | 79.23 |
weighted deaths | (low, low) | 0.9 (elderly) | 80.08 |