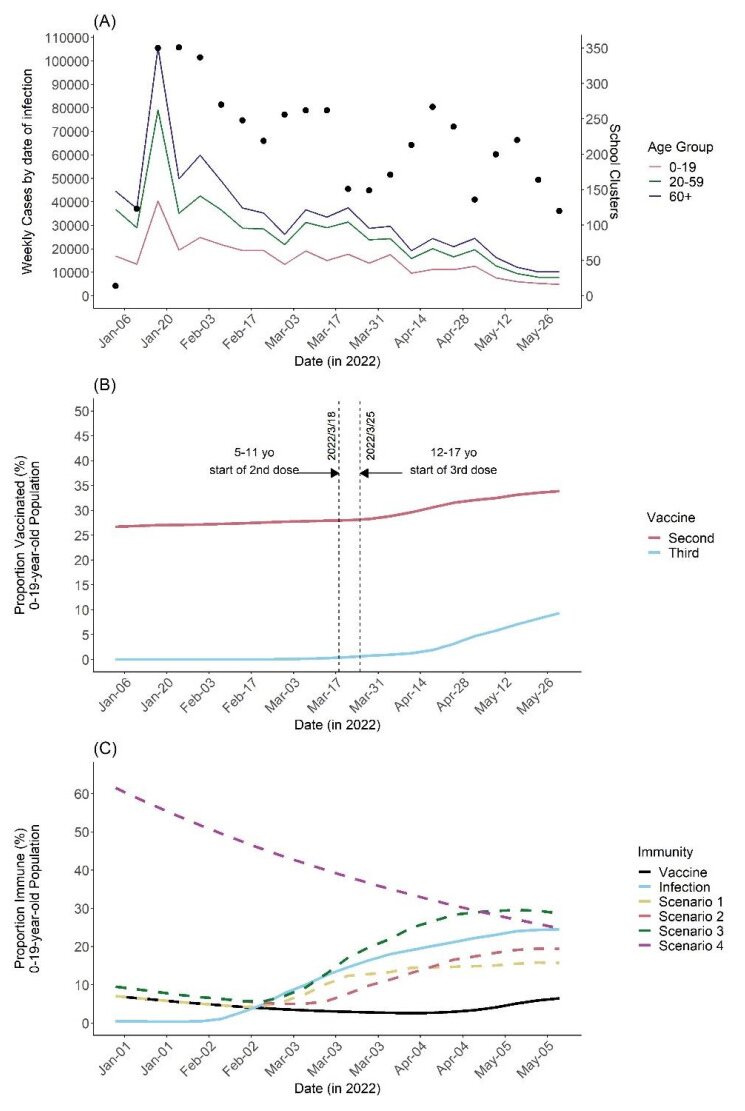
Clusters of COVID-19 in high-risk settings, such as schools, have been deemed a critical driving force of the major epidemic waves at the societal level. In Japan, the vaccination coverage among students remained low up to early 2022, especially for 5–11-year-olds. The vaccination of the student population only started in February 2022. Given this background and considering that vaccine effectiveness against school transmission has not been intensively studied, this paper proposes a mathematical model that links the occurrence of clustering to the case count among populations aged 0–19, 20–59, and 60+ years of age. We first estimated the protected (immune) fraction of each age group either by infection or vaccination and then linked the case count in each age group to the number of clusters via a time series regression model that accounts for the time-varying hazard of clustering per infector. From January 3 to May 30, 2022, there were 4,722 reported clusters in school settings. Our model suggests that the immunity offered by vaccination averted 226 (95% credible interval: 219–232) school clusters. Counterfactual scenarios assuming elevated vaccination coverage with faster roll-out reveal that additional school clusters could have been averted. Our study indicates that even relatively low vaccination coverage among students could substantially lower the risk of clustering through vaccine-induced immunity. Our results also suggest that antigenically updated vaccines that are more effective against the variant responsible for the ongoing epidemic may greatly help decrease not only the incidence but also the unnecessary loss of learning opportunities among school-age students.
Citation: Yuta Okada, Hiroshi Nishiura. Vaccine-induced reduction of COVID-19 clusters in school settings in Japan during the epidemic wave caused by B.1.1.529 (Omicron) BA.2, 2022[J]. Mathematical Biosciences and Engineering, 2024, 21(9): 7087-7101. doi: 10.3934/mbe.2024312
[1] | Sarafa A. Iyaniwura, Rabiu Musa, Jude D. Kong . A generalized distributed delay model of COVID-19: An endemic model with immunity waning. Mathematical Biosciences and Engineering, 2023, 20(3): 5379-5412. doi: 10.3934/mbe.2023249 |
[2] | Ryo Kinoshita, Sung-mok Jung, Tetsuro Kobayashi, Andrei R. Akhmetzhanov, Hiroshi Nishiura . Epidemiology of coronavirus disease 2019 (COVID-19) in Japan during the first and second waves. Mathematical Biosciences and Engineering, 2022, 19(6): 6088-6101. doi: 10.3934/mbe.2022284 |
[3] | Tetsuro Kobayashi, Hiroshi Nishiura . Prioritizing COVID-19 vaccination. Part 2: Real-time comparison between single-dose and double-dose in Japan. Mathematical Biosciences and Engineering, 2022, 19(7): 7410-7424. doi: 10.3934/mbe.2022350 |
[4] | Pannathon Kreabkhontho, Watchara Teparos, Thitiya Theparod . Potential for eliminating COVID-19 in Thailand through third-dose vaccination: A modeling approach. Mathematical Biosciences and Engineering, 2024, 21(8): 6807-6828. doi: 10.3934/mbe.2024298 |
[5] | Ugo Avila-Ponce de León, Angel G. C. Pérez, Eric Avila-Vales . Modeling the SARS-CoV-2 Omicron variant dynamics in the United States with booster dose vaccination and waning immunity. Mathematical Biosciences and Engineering, 2023, 20(6): 10909-10953. doi: 10.3934/mbe.2023484 |
[6] | Allison Fisher, Hainan Xu, Daihai He, Xueying Wang . Effects of vaccination on mitigating COVID-19 outbreaks: a conceptual modeling approach. Mathematical Biosciences and Engineering, 2023, 20(3): 4816-4837. doi: 10.3934/mbe.2023223 |
[7] | Natalie M. Linton, Sung-mok Jung, Hiroshi Nishiura . Not all fun and games: Potential incidence of SARS-CoV-2 infections during the Tokyo 2020 Olympic Games. Mathematical Biosciences and Engineering, 2021, 18(6): 9685-9696. doi: 10.3934/mbe.2021474 |
[8] | Fang Wang, Lianying Cao, Xiaoji Song . Mathematical modeling of mutated COVID-19 transmission with quarantine, isolation and vaccination. Mathematical Biosciences and Engineering, 2022, 19(8): 8035-8056. doi: 10.3934/mbe.2022376 |
[9] | Weike Zhou, Aili Wang, Fan Xia, Yanni Xiao, Sanyi Tang . Effects of media reporting on mitigating spread of COVID-19 in the early phase of the outbreak. Mathematical Biosciences and Engineering, 2020, 17(3): 2693-2707. doi: 10.3934/mbe.2020147 |
[10] | Sherry Towers, Katia Vogt Geisse, Chia-Chun Tsai, Qing Han, Zhilan Feng . The impact of school closures on pandemic influenza: Assessing potential repercussions using a seasonal SIR model. Mathematical Biosciences and Engineering, 2012, 9(2): 413-430. doi: 10.3934/mbe.2012.9.413 |
Clusters of COVID-19 in high-risk settings, such as schools, have been deemed a critical driving force of the major epidemic waves at the societal level. In Japan, the vaccination coverage among students remained low up to early 2022, especially for 5–11-year-olds. The vaccination of the student population only started in February 2022. Given this background and considering that vaccine effectiveness against school transmission has not been intensively studied, this paper proposes a mathematical model that links the occurrence of clustering to the case count among populations aged 0–19, 20–59, and 60+ years of age. We first estimated the protected (immune) fraction of each age group either by infection or vaccination and then linked the case count in each age group to the number of clusters via a time series regression model that accounts for the time-varying hazard of clustering per infector. From January 3 to May 30, 2022, there were 4,722 reported clusters in school settings. Our model suggests that the immunity offered by vaccination averted 226 (95% credible interval: 219–232) school clusters. Counterfactual scenarios assuming elevated vaccination coverage with faster roll-out reveal that additional school clusters could have been averted. Our study indicates that even relatively low vaccination coverage among students could substantially lower the risk of clustering through vaccine-induced immunity. Our results also suggest that antigenically updated vaccines that are more effective against the variant responsible for the ongoing epidemic may greatly help decrease not only the incidence but also the unnecessary loss of learning opportunities among school-age students.
The world has suffered substantially from the morbidity and mortality caused by severe acute respiratory syndrome coronavirus 2 (SARS-CoV-2), which originated in Wuhan, China, in late 2019. As a respiratory pathogen, the transmission of SARS-CoV-2 is mainly thought to occur through close contact between humans, although aerosol transmission has also attracted considerable attention [1,2,3]. high transmissibility and mode of transmission of SARS-CoV-2 have led to countless clusters throughout the (technically ongoing) pandemic [4,5,6,7,8]. Following an outbreak on a cruise ship that docked in Japan in February 2020, local chains of transmission of coronavirus disease 2019 (COVID-19) were gradually established, and it grew into an epidemic in March 2020. At that time, countermeasures in Japan focused on contact tracing, containment, and the prevention of clusters focusing on high-risk settings [9]. In early 2020, it was already evident that COVID-19 transmission tended to occur in high-risk settings that were heavily linked to nightlife and other indoor activities, which acted as the key driving force of the major epidemic [10,11,12]. This trend remained consistent in Japan until mid-2022 [13]. However, as the COVID-19 pandemic progressed, other types of clusters emerged, including school clusters [14]. The behavioral nature of students frequently leads to close contact and aggregations. One important factor that may explain the surge of infections in school settings is the relatively low vaccination rate among the school population. The low vaccination rate was presumably caused by parents' hesitancy toward vaccination and, in the case of Japan, the delayed approval process for the vaccination of school-age children [15,16].
One of the key public health concerns regarding school clusters is the number of clusters averted by vaccination. This is because clusters may not only harm the health of students or their families but also lead to the closure of classrooms or entire schools, thus disrupting school activities, which are important for students' physical and mental well-being [17,18]. For non-educational settings, Sasanami et al. built a mathematical model to elucidate the effectiveness of vaccination in preventing clusters in healthcare and welfare facilities [19]. Their study showed that vaccination played a substantial role in preventing clusters in such settings during the period before the emergence of the Omicron variant (B.1.1.529). However, in the school setting, the extent to which vaccine-induced protection prevented clusters in Japan has yet to be quantified.
The role of schools in COVID-19 transmission has been studied from various angles. Some studies have evaluated the impact of nationwide or regionwide school closures on COVID-19 incidence at the societal level, particularly during the early phases of the pandemic [20,21,22,23]. Though these studies do not strongly support the effectiveness of school closures against reductions in population-level COVID-19 incidences, these earlier results might not be applicable to the current situation under the larger epidemic size with Omicron variants. At a finer scale than societal perspectives, there are studies that quantified the risk of COVID-19 transmission itself, as well as preventive measures such as masking, physical distancing, screening, increased ventilation, and vaccination in school settings [24,25,26,27,28,29,30,31]. There have been studies focused on the occurrence of clusters within school settings [7,8,32]. Results from Tupper et al. in the pre-Omicron variant period in Canada suggest that larger clusters are responsible for more infections than smaller clusters, and that reduction of transmissibility and contact among students was effective in reducing total cases [7]. While other studies also investigated the risk of cluster occurrence as well as possible mitigation strategies in the school setting, these studies were conducted prior to the emergence of the Omicron variant. This makes it difficult to deduce similar results to the current situation in Japan, where a high number of cases have been caused by Omicron variants. Also, the abovementioned studies did not specifically address the vaccine effectiveness for preventing school clusters. Contrary to the relatively small contribution of the school-aged population to the entire COVID-19 incidence in 2020[10,33], COVID-19 transmissions in school settings played a growing role during the course of the pandemic [34]. The school clusters became more prominent since the spread of Omicron variants began in late 2021 in Japan. The original series of mRNA vaccines appeared to be less effective against Omicron than earlier circulating variants, and even before the emergence of Omicron variants, it was suggested that vaccinated students had a substantial risk of contracting COVID-19 [25]. Thus, a key question regarding COVID-19 transmission in schools concerns the impact of vaccination against the clustering of COVID-19 in school settings during the period in which Omicron variants were active.
In this paper, we propose a modeling framework for quantifying the effect of vaccination programs on preventing clusters in school settings throughout the time period in which Omicron BA.2 was predominant in Japan, namely from January to May 2022. First, we deploy mathematical models to estimate the population-level immunity against Omicron BA.2 and back-calculate the case count using surveillance data arranged by date of reporting [35]. Subsequently, we apply a time series regression model to account for the relationship between infection counts in three age groups (0–19, 20–59, and 60+ years) and clustering in a time-varying manner, considering the population-level immunity among those aged from 0 to 19 years. Finally, we consider counterfactual scenarios to clarify the further effect of vaccination on the occurrence of school clusters.
The present study used both the epidemiological data of COVID-19 as well as the record of vaccination at the national level. Our epidemiological data mainly consisted of cluster data available from the website of the Ministry of Health, Labour and Welfare [36] and the daily case count data in each age group from Health Center Real-Time Information-sharing System on COVID-19 (HER-SYS). The cluster data were subclassified into incidences in various settings including clusters in schools, which is the outcome variable in the subsequent analysis. Additional epidemiological data used in our study include the open data from the Tokyo Metropolitan government, which provide anonymized individual-level information on the date of onset, date of diagnosis, or date of report for some portion of cases [37].
As for the vaccination record, we retrieved the data from the Vaccination Record System (VRS). This record includes information on the daily number of vaccinations, classified by 5-year age groups. Other than the original datasets from Japan, we referred to published estimates of the generation time as well as the incubation period of SARS-CoV-2 Omicron variants [38,39].
As a setup for the main analysis of cluster incidence, we estimated the population-level immunity (i.e., protected fraction of the population by age and time) following the methodology described by Sasanami et al. [35] While Sasanami et al. estimated the population-level immunity for the adult population, we also conducted an estimation for the younger 0–19-year-old age group. Otherwise, our analysis is based on the same methodology and data (HER-SYS for case counts and VRS for vaccination records. For simplicity, we used the median of estimates of population-level immunity for subsequent analysis.
To estimate the incidence by the date of infection, we first quantified the delay distribution from infection to reporting as follows. Let D be the dataset consisting of all case records from Jan 1, 2022, to May 31, 2022, with complete information on the date of illness onset. Assuming that the delay distribution from symptom to onset is sufficiently described by some parametric probability density function with parameter θ, the discretized probability mass function as a function of time since the onset of illness can be described as follows:
pOnset→Report(t|θ)=CDFOnset→Report(t+1|θ)−CDFOnset→Report(t|θ). | (1) |
The likelihood function for observing D can then be described as
L(θ|D)=∏i∈DpOnset→Report(ti,report−ti,onset|θ)pOnset→Report(T−ti,onset|θ), | (2) |
where T is May 31, 2022, which was the last date of observation. Assuming a Weibull-distributed delay function and using maximum likelihood estimation on L(θ|D), we obtained the best fit estimate ˆθ. Using pOnset→Report(t|ˆθ) together with the reported incubation period of Omicron variants[39], the relationship between the incidence by date of reporting Yt and that by date of infection It can be described as follows:
Yt=∞∑T=0It−TT∑τ=0pincubation(τ)pOnset→Report(T−τ) | (3) |
Using this relationship, we estimated It as the daily case count by means of nonparametric back-calculation using the R package "surveillance" [40].
Based on the abovementioned preparation, we built a time-varying regression model to estimate the effect of vaccine-induced immunity on the incidence of clustered transmission events. The model is as follows:
Ncluster,t∼Poisson(λt), | (4) |
λt=κtΣβaJLa,t, | (5) |
where Ncluster,t, the number of clusters in week t, is assumed to follow a Poisson distribution with expected value λt. The back-calculated infection count by date of infection is denoted as Ia,t (a:0–19, 20–59, or 60+ years), and the number of infected individuals in age group a contributing to infection in week t is described as Ja,t using the renewal equation [41,42,43] (described in Supplementary methods). Equation (5) describes the expected number of clusters, λt, as a linear combination of JLa,t (Ja,t lagged by L weeks) with a fixed coefficient βa scaled by κt. For subsequent analysis, we fixed L to 1 week based on a cross-correlation analysis between the time series of cluster numbers and Ja,t. The temporal variation of κt was modeled as a random walk with standard deviation σ, as described in Supplementary methods. The parameters Θ=(βa,κt,σ) were estimated using a Bayesian framework via the Markov Chain Monte Carlo (MCMC) method. The priors we used are available in the supplementary material. For each estimation, we generated 1,500 MCMC samples from four chains, discarding 500 warm-up iterations from each chain. Convergence was confirmed by an R-hat value below 1.01, and no divergent chains were observed in the trace plots.
Based on the estimates from MCMC, the expected number, λt(S,A), and expected difference, Δλ(S,A), in the number of clusters compared with the number of observed clusters λt, which corresponds to counterfactual scenarios on vaccination programs, were calculated as follows:
λt(S,A)=λt1−vSvax−Avinf1−vvax−Avinf, | (6) |
Δλ(S,A)=∑tλt(S,A)−λt, | (7) |
where A accounts for the ascertainment ratio of the true infection count compared with the reported case count, and S denotes counterfactual scenarios for vaccination programs and vaccine-induced immunity. In equation (6), the denominator of the second term is for removing the effect of population-level immunity in the observation, and the numerator is for reflecting the population-level immunity in counterfactual scenarios. (Note that Avinf is the population-level immunity offered by true infections as observed in epidemiological data, so is not involved in counterfactual analysis.) Thus, using equation (6), we can obtain counterfactual predictions of the number of clusters for each counterfactual scenario S. The cumulative difference between the counterfactual number of clusters and observed clusters throughout the study period can be obtained by equation (7). Following an estimate of the ascertainment rate of COVID-19 cases in Japan, we assumed A=4 [44].
As of May 30, 2022, the approximate cumulative proportions that had already received a second vaccine dose were 11.1% among children aged 5–11 years and 65.7% among children aged 12–17 years. By the same date, 18.5% of the 12–17-year-olds had already received a third booster dose. This proportion was calculated using an approximate population count derived from the 5-year age class population data as of June 1, 2022 [45]. Based on these figures, we tested the counterfactual scenarios listed below (for a summary of all counterfactual scenarios, see supplementary material):
- Scenario 0: No vaccination.
- Scenario 1: Accelerating the second-dose vaccination program among children aged 5–11 years, completing it 8 weeks earlier and elevating the daily dose by a factor of eight.
- Scenario 2: Relatively elevating the second-dose vaccination coverage among 12–17-year-olds by 50%, as well as accelerating the third-dose vaccination program for the same population by 8 weeks and elevating the daily doses by a factor of four.
- Scenario 3: Combination of scenarios 1 and 2.
- Scenario 4: An extreme scenario where all 0–19-year-olds received the third booster vaccine on December 18, 2021. (Peak population-level immunity reached on January 1, 2022.)
All statistical and numerical analyses were performed using R version 4.2.2 (The R Project for Statistical Computing, Vienna, Austria) and Cmdstan version 2.33.0 [46,47,48]. Information on the prior distributions is presented in the supplementary material.
In Figure 1, panel (A) shows the back-calculated weekly infection counts by age groups (left y-axis), which are overlaid with the weekly number of reported school clusters (right y-axis). Panel (B) shows the cumulative vaccination coverage and estimated immune fraction against symptomatic COVID-19 infection among those aged 0–19 years. During the first epidemic wave caused by the Omicron variant, vaccination coverage was maintained at a rather low level among school children. Thus, mainly because of infection-acquired immunity and partly because of vaccine-acquired immunity, the immune fraction was elevated during the course of the corresponding epidemic wave as shown in panel (C) of Figure 2.
Based on these descriptive and preliminary analyses, we estimated the coefficients for the infector population of each age group, βa, in equation (5). Coefficients βa were estimated at 4.388 [95% credible interval (CrI): 3.776, 4.820] among those aged 0–19 years, 0.145 (95% CrI: 0.040, 0.385) among those aged 20–59 years, and 0.133 (95% CrI: 0.034, 0.361) among those aged 60+. As for the interpretation of βa, it can be seen as the per-person contribution by infectors in age group a. The highest per-person contribution was seen in the youngest population, but a small yet substantial contribution from those aged 20–59 and 60+ was also noted. See Supplementary Materials for results of scaling factor κt, contributions to cluster occurrence by each age group, and standard deviation σκ.
The expected number of school clusters is shown in Figure 2. We compare those based on (A) counterfactual scenario 0, (B) scenario 1, (C) scenario 2, (D) scenario 3, and (E) scenario 4. In line with the estimated difference between observed versus counterfactual vaccine-induced immune levels, panel (A) shows that when the vaccination effect was nullified, the number of clusters could have been higher in each time step. In contrast, panels (B), (C), and (D) show that accelerated vaccination programs with increased vaccination coverage among children aged 5–11 years or 12–19 years could have led to a gradual decrease in the number of school clusters compared with the actual observation.
Table 1 summarizes Δλ(S,A), the expected cumulative difference in school clusters for counterfactual scenarios compared with the observed number of clusters. The number of clusters was expected to increase in scenario 0 by 222.8 (95% CrI: 216.6,229.1), whereas in scenarios 1, 2, 3, and 4, accelerating the vaccination schedule and elevating the coverage among children aged 5–11 years or 12–19 years could have reduced school transmission clusters substantially throughout the study period. In scenarios 1, 2, and 3, the expected numbers of averted clusters were estimated as 310.6 (95% CrI: 299.7,322.1), 335.8 (95% CrI: 324.1,348.2), and 646.5 (95%CrI: 624.3,670.1), respectively. In addition to these results, the extreme assumption in scenario 4 led to aversion of 2101 (95% CrI: 2044, 2161) clusters, which amounts to about 44% reduction from the total of 4722 clusters that were observed during the study period. See supplementary material for results from other counterfactual scenarios.
Scenarios | Δλ(S,A) (95% CrI) |
0 | 222.8 (216.6,229.1) |
1 | -310.6 (-322.1, -299.7) |
2 | -335.8 (-348.2, -324.1) |
3 | -646.5 (-670.1, -624.3) |
4 | -2101 (-2161, -2044) |
The present study employed a statistical model that accounts for vaccination coverage in relation to the number of clustering events in school settings in Japan. Unfortunately, the second-dose vaccination coverage among students aged below 20 years was only 33.9% as of May 30, 2022. By fitting the statistical model to the empirical data and exploring counterfactual scenarios with potentially elevated vaccination coverage, we have shown that vaccine-induced immunity led to substantial aversion of school clusters during the BA.1 epidemic wave in 2022.
The importance of our study lies not only in the revelation of vaccine effectiveness against school clusters but also in the implication that higher vaccination coverage would have prevented a substantial number of school clusters, substantially averting both negative health impacts and loss of learning opportunities. Our counterfactual scenario 4 suggests that 100% coverage of third vaccination among those aged 0–19 years just before the arrival of Omicron variants could have prevented around 44% of the observed clusters, which should lead to a substantial reduction in terms of infection counts among this population. Other scenario analyses also suggest that vaccination campaigns could have prevented about 5%–14% of observed clusters in school settings even with lower vaccination rates and increasing infection-acquired immunity. Despite the relatively low effectiveness and rapid decay of vaccine-induced immunity elicited by mRNA vaccines that used the original Wuhan strain, these results highlight the importance of vaccination for stopping the occurrence of school clusters and averting a substantial number of infections among students. This finding is in line with studies that demonstrated the role of vaccination in minimizing school closure[31] and adds to studies that highlighted the importance of reducing transmissibility and contact among students to reduce school clusters and school closures [7,32]. Given that the school-aged population largely contributes to the COVID-19 transmission dynamic than in the earlier pandemic periods [34], our result strongly suggests the importance of vaccination among this population.
Moreover, our study also shows that, on a weekly scale, infector populations in older age groups contributed to the elevated incidence of school clusters. Though their contribution was smaller than those of school students, it seems substantial especially at around the peak of the epidemic wave (mid-January to mid-February). The contribution by infectors in older age groups may account both for direct transmission in the school setting and for indirect transmission outside of the school setting. Considering that the mean generation interval of Omicron variants may lie in the order of 3 days [38], it is possible that older age groups contributed mostly in an indirect manner. However, it is also possible that teachers or classroom assistants belonging to the older population may have played a substantial role in the occurrence of school clusters. Regarding this finding, exploring the effect of vaccination in older age groups will be the scope of future research.
Beyond the abovementioned revelations and insights from our results, the simplicity of our model is of key importance in the present study. This simple time-varying regression model revealed the age-group-specific contribution to school cluster incidence in a readily interpretable manner. Additionally, when the immunity level among student populations is estimable, our model provides a straightforward means of implementing counterfactual analyses. Although situations regarding immune profiles in the present day against COVID-19 are becoming increasingly complex because of the seamless emergence of SARS-CoV-2 variants and infections, and vaccination histories are becoming more intricate, if combined with social or serological surveys, our framework may still be applicable in the future.
There are four key limitations of our study. First, the data used in our study did not include background details including the cluster size and chronological process of cluster identification. That is, while we handled each cluster as having an equivalent incidence, the nature of transmission in different clusters might be completely different, even provided all clusters in discussion were in schools [7]. However, our model did at least account for the random variability of the mechanism (nature) of transmission by the time-varying scaling factor, accounting for the average overdispersion of transmission at the national level. Second, we only considered the direct impact of vaccination on the occurrence of school clusters in our counterfactual scenarios. In reality, changes in vaccination strategies might substantially change the transmission dynamics for the entire population. Elucidating the roles of schools in modifying the transmission dynamics of COVID-19 of the entire population is the key to success in appropriately quantifying the impact of vaccination in schools [49]. Third, our model did not consider the reduction of transmissibility by vaccination at an individual level [50]. We were not able to separate this effect from others in the model because the case count data were not accompanied by the vaccination status for each individual, so the suppressive effect of vaccination on school clusters may have been even larger than suggested by our modeling. Fourth, epidemiological approaches to counterfactual scenarios for factors that alter transmission are yet subject to technical debates. For instance, while the direct impact of vaccination can be evaluated via hypothesis testing by comparing risks in vaccinated and unvaccinated individuals, the entire impact (i.e., direct plus indirect effects) of vaccination programs requires comparing the transmission dynamics in actual and hypothetical (theoretical) scenarios, and conducting comparative assessment has yet to be formalized.
Despite these limitations, the present study revealed that school clusters may have been substantially reduced if the vaccination rate in the school population had been higher during the BA.1 epidemic in Japan in 2022. The resulting classroom closures caused a substantial loss of learning opportunities for students, and the burden of COVID-19, including post-acute sequelae of COVID-19 and the overwhelmed healthcare system, has been disastrous. Beyond these implications, our simple yet interpretable model is important in that it is readily applicable to the analysis of clusters in other settings if relevant data on clusters and population-level immunity are available.
In the present study, we built and deployed a time-varying regression model to reveal the impact of vaccine-induced immunity on the incidence of school clusters. Our counterfactual scenarios revealed that vaccination programs contributed substantially to the reduction of school clusters, despite the relatively low vaccination coverage among school-aged populations. Additionally, intensifying and accelerating the vaccination program could have averted more clusters, even without considering the effect of the vaccination program on the population-level COVID-19 dynamics. Even in the fifth year of the COVID-19 pandemic, a substantial proportion of the student population remains vaccine-naïve in Japan.
Y.O. received funding from the Kyoto University Foundation, SECOM Science and Technology Foundation, and The Fujiwara Memorial Foundation. H.N. received funding from Health and Labour Sciences Research Grants (grant numbers 20CA2024, 21HB1002, 21HA2016, and 23HA2005), the Japan Agency for Medical Research and Development (grant numbers JP23fk0108612 and JP23fk0108685), JSPS KAKENHI (grant numbers 21H03198 and 22K19670), the Environment Research and Technology Development Fund (grant number JPMEERF20S11804) of the Environmental Restoration and Conservation Agency of Japan, Kao Health Science Research, the Daikin GAP Fund of Kyoto University, the Japan Science and Technology Agency SICORP program (grant numbers JPMJSC20U3 and JPMJSC2105), and the RISTEX program for Science, Technology, and Innovation Policy (grant number JPMJRS22B4). The funders had no role in the study design, data collection and analysis, decision to publish, or preparation of the manuscript. We also thank Stuart Jenkinson, PhD, from Edanz (https://jp.edanz.com/ac) for editing a draft of this manuscript.
The authors declare they have not used Artificial Intelligence (AI) tools in the creation of this article.
Hiroshi Nishiura is a special issue editor for Mathematical Biosciences and Engineering and was not involved in the editorial review or the decision to publish this article. All authors declare that there are no competing interests.
Ethical approval was not required because none of the data used in the present study include any personally identifiable information.
Access to data from HER-SYS was granted to us exclusively for epidemiological analysis of COVID-19 and no data were disclosable to any third parties. Other than data from HER-SYS, the data used in this study are publicly available from the website of the Ministry of Health, Labour or Welfare (https://www.mhlw.go.jp/stf/covid-19/open-data.html).
[1] |
N. Banholzer, K. Zürcher, P. Jent, P. Bittel, L. Furrer, M. Egger, et al., SARS-CoV-2 transmission with and without mask wearing or air cleaners in schools in Switzerland: A modeling study of epidemiological, environmental, and molecular data, PLoS Med., 20 (2023), e1004226. https://doi.org/10.1371/journal.pmed.1004226 doi: 10.1371/journal.pmed.1004226
![]() |
[2] |
E.A. Meyerowitz, A. Richterman, R.T. Gandhi, P.E. Sax, Transmission of SARS-CoV-2: A review of viral, host, and environmental factors, Ann. Intern. Med., 174 (2021), 69–79. https://doi.org/10.7326/M20-5008 doi: 10.7326/M20-5008
![]() |
[3] |
M. Cevik, J.L. Marcus, C. Buckee, T.C. Smith, Severe Acute Respiratory Syndrome Coronavirus 2 (SARS-CoV-2) transmission dynamics should inform policy, Clin. Infect. Dis., 73 (2021), S170–S176. https://doi.org/10.1093/cid/ciaa1442 doi: 10.1093/cid/ciaa1442
![]() |
[4] |
D.C. Adam, P. Wu, J.Y. Wong, E.H.Y. Lau, T.K. Tsang, S. Cauchemez, et al., Clustering and superspreading potential of SARS-CoV-2 infections in Hong Kong, Nat Med., 26 (2020), 1714–1719. https://doi.org/10.1038/s41591-020-1092-0 doi: 10.1038/s41591-020-1092-0
![]() |
[5] |
T. Liu, D. Gong, J. Xiao, J. Hu, G. He, Z. Rong, W. Ma, Cluster infections play important roles in the rapid evolution of COVID-19 transmission: A systematic review, Int. J. Infect. Dis., 99 (2020), 374–380. https://doi.org/10.1016/j.ijid.2020.07.073 doi: 10.1016/j.ijid.2020.07.073
![]() |
[6] |
Y. Furuse, N. Tsuchiya, R. Miyahara, I. Yasuda, E. Sando, Y.K. Ko, et al., COVID-19 case-clusters and transmission chains in the communities in Japan, J. Infect., 84 (2022), 248–288. https://doi.org/10.1016/j.jinf.2021.08.016 doi: 10.1016/j.jinf.2021.08.016
![]() |
[7] |
P. Tupper, S. Pai, COVID Schools Canada, C. Colijn, COVID-19 cluster size and transmission rates in schools from crowdsourced case reports, ELife, 11 (2022), e76174. https://doi.org/10.7554/eLife.76174 doi: 10.7554/eLife.76174
![]() |
[8] |
M. Ueda, K. Hayashi, H. Nishiura, Identifying High-Risk Events for COVID-19 Transmission: Estimating the Risk of Clustering Using Nationwide Data, Viruses, 15 (2023), 456. https://doi.org/10.3390/v15020456 doi: 10.3390/v15020456
![]() |
[9] |
H. Oshitani, L.A.W.J. The Expert Members of The National COVID-19 Cluster Taskforce at The Ministry of Health, Cluster-Based Approach to Coronavirus Disease 2019 (COVID-19) Response in Japan, from February to April 2020, Jpn. J. Infect. Dis., 73 (2020), 491–493. https://doi.org/10.7883/yoken.JJID.2020.363 doi: 10.7883/yoken.JJID.2020.363
![]() |
[10] |
T. Imamura, A. Watanabe, Y. Serizawa, M. Nakashita, M. Saito, M. Okada, et al., Transmission of COVID-19 in Nightlife, Household, and Health Care Settings in Tokyo, Japan, in 2020, JAMA Netw Open., 6 (2023), e230589. https://doi.org/10.1001/jamanetworkopen.2023.0589 doi: 10.1001/jamanetworkopen.2023.0589
![]() |
[11] |
S. Nagata, T. Nakaya, Y. Adachi, T. Inamori, K. Nakamura, D. Arima, et al., Mobility change and COVID-19 in Japan: Mobile data analysis of locations of infection, J. Epidemiol., 31 (2021), 387–391. https://doi.org/10.2188/jea.JE20200625 doi: 10.2188/jea.JE20200625
![]() |
[12] |
M. Nakanishi, R. Shibasaki, S. Yamasaki, S. Miyazawa, S. Usami, H. Nishiura, et al., On-site dining in Tokyo during the COVID-19 pandemic: Time series analysis using mobile phone location data, JMIR MHealth UHealth., 9 (2021), e27342. https://doi.org/10.2196/27342 doi: 10.2196/27342
![]() |
[13] |
Y. Okada, S. Yamasaki, A. Nishida, R. Shibasaki, H. Nishiura, Night-time population consistently explains the transmission dynamics of coronavirus disease 2019 in three megacities in Japan, Front Public Health., 11 (2023). https://doi.org/10.3389/fpubh.2023.1163698 doi: 10.3389/fpubh.2023.1163698
![]() |
[14] | Ministry of Education Culture Sports Science and Technology, Surveys on the situation in schools, case studies of initiatives (Japanese), Ministry of Education, Culture, Sports, Science and Technology. https://www.mext.go.jp/a_menu/coronavirus/mext_00007.html (accessed September 4, 2024) |
[15] |
A. Takamatsu, H. Honda, T. Miwa, T. Tabuchi, K. Taniguchi, K. Shibuya, et al., Factors associated with COVID-19 booster vaccine hesitancy: A nationwide, cross-sectional survey in Japan, Public Health, 223 (2023), 72–79. https://doi.org/10.1016/j.puhe.2023.07.02 doi: 10.1016/j.puhe.2023.07.02
![]() |
[16] |
Y. Takahashi, K. Ishitsuka, M. Sampei, S. Okawa, Y. Hosokawa, A. Ishiguro, et al., COVID-19 vaccine literacy and vaccine hesitancy among pregnant women and mothers of young children in Japan, Vaccine, 40 (2022), 6849–6856. https://doi.org/10.1016/j.vaccine.2022.09.094 doi: 10.1016/j.vaccine.2022.09.094
![]() |
[17] |
D. Courtney, P. Watson, M. Battaglia, B.H. Mulsant, P. Szatmari, COVID-19 Impacts on Child and Youth Anxiety and Depression: Challenges and Opportunities, Can, J, Psychiatry., 65 (2020), 688–691. https://doi.org/10.1177/0706743720935646 doi: 10.1177/0706743720935646
![]() |
[18] |
R.E. Norman, M. Byambaa, R. De, A. Butchart, J. Scott, T. Vos, The long-term health consequences of child physical abuse, emotional abuse, and neglect: A systematic review and meta-analysis, PLoS Med., 9 (2012), e1001349. https://doi.org/10.1371/journal.pmed.1001349 doi: 10.1371/journal.pmed.1001349
![]() |
[19] |
M. Sasanami, T. Kayano, H. Nishiura, The number of COVID-19 clusters in healthcare and elderly care facilities averted by vaccination of healthcare workers in Japan, February–June 2021, Math. Biosci. Eng., 19 (2022), 2762–2773. https://doi.org/10.3934/mbe.2022126 doi: 10.3934/mbe.2022126
![]() |
[20] |
E. Abdollahi, M. Haworth-Brockman, Y. Keynan, J.M. Langley, S.M. Moghadas, Simulating the effect of school closure during COVID-19 outbreaks in Ontario, Canada, BMC Med., 18 (2020), 230. https://doi.org/10.1186/s12916-020-01705-8 doi: 10.1186/s12916-020-01705-8
![]() |
[21] |
K.A. Auger, S.S. Shah, T. Richardson, D. Hartley, M. Hall, A. Warniment, et al., Association Between Statewide School Closure and COVID-19 Incidence and Mortality in the US, JAMA, 324 (2020), 859–870. https://doi.org/10.1001/jama.2020.1434 doi: 10.1001/jama.2020.1434
![]() |
[22] |
K. Fukumoto, C.T. McClean, K. Nakagawa, No causal effect of school closures in Japan on the spread of COVID-19 in spring 2020, Nat. Med., 27 (2021), 2111–2119. https://doi.org/10.1038/s41591-021-01571-8 doi: 10.1038/s41591-021-01571-8
![]() |
[23] |
K. Iwata, A. Doi, C. Miyakoshi, Was school closure effective in mitigating coronavirus disease 2019 (COVID-19)? Time series analysis using Bayesian inference, Int. J. Infect. Dis., 99 (2020), 57–61. https://doi.org/10.1016/j.ijid.2020.07.052 doi: 10.1016/j.ijid.2020.07.052
![]() |
[24] |
T. Leng, E.M. Hill, A. Holmes, E. Southall, R.N. Thompson, M.J. Tildesley, et al., Quantifying pupil-to-pupil SARS-CoV-2 transmission and the impact of lateral flow testing in English secondary schools, Nat. Commun., 13 (2022), 1106. https://doi.org/10.1038/s41467-022-28731-9 doi: 10.1038/s41467-022-28731-9
![]() |
[25] |
K.E. Wiens, C.P. Smith, E. Badillo-Goicoechea, K.H. Grantz, M.K. Grabowski, A.S. Azman, et al., In-person schooling and associated COVID-19 risk in the United States over spring semester 2021, Sci Adv., 8 (2022), eabm9128. https://doi.org/10.1126/sciadv.abm9128 doi: 10.1126/sciadv.abm9128
![]() |
[26] |
C. Molina Grané, P. Mancuso, M. Vicentini, F. Venturelli, O. Djuric, M. Manica, et al., SARS-CoV-2 transmission patterns in educational settings during the Alpha wave in Reggio-Emilia, Italy, Epidemics, 44 (2023), 100712. https://doi.org/10.1016/j.epidem.2023.100712 doi: 10.1016/j.epidem.2023.100712
![]() |
[27] |
K.O. Zimmerman, I.C. Akinboyo, M.A. Brookhart, A.E. Boutzoukas, K.A. McGann, M.J. Smith, et al., Incidence and secondary transmission of SARS-CoV-2 infections in schools, Pediatrics, 147 (2021), e2020048090. https://doi.org/10.1542/peds.2020-048090 doi: 10.1542/peds.2020-048090
![]() |
[28] |
P. van den Berg, E.M. Schechter-Perkins, R.S. Jack, I. Epshtein, R. Nelson, E. Oster, et al., Effectiveness of 3 Versus 6 ft of Physical Distancing for Controlling Spread of Coronavirus Disease 2019 Among Primary and Secondary Students and Staff: A Retrospective, Statewide Cohort Study, Clin. Infect. Dis., 73 (2021), 1871–1878. https://doi.org/10.1093/cid/ciab230 doi: 10.1093/cid/ciab230
![]() |
[29] |
M. Hast, M. Swanson, C. Scott, E. Oraka, C. Espinosa, E. Burnett, et al., Prevalence of risk behaviors and correlates of SARS-CoV-2 positivity among in-school contacts of confirmed cases in a Georgia school district in the pre-vaccine era, December 2020–January 2021, BMC Public Health, 22 (2022), 101. https://doi.org/10.1186/s12889-021-12347-7 doi: 10.1186/s12889-021-12347-7
![]() |
[30] |
T. Akaishi, S. Kushimoto, Y. Katori, N. Sugawara, K. Igarashi, M. Fujita, et al., COVID-19 transmission at schools in Japan, Tohoku J. Exp. Med., 255 (2021), 239–246. https://doi.org/10.1620/tjem.255.239 doi: 10.1620/tjem.255.239
![]() |
[31] |
E. Colosi, G. Bassignana, D.A. Contreras, C. Poirier, P.-Y. Boëlle, S. Cauchemez, et al., Screening and vaccination against COVID-19 to minimise school closure: A modelling study, Lancet Infect. Dis., 22 (2022), 977–989. https://doi.org/10.1016/S1473-3099(22)00138-4 doi: 10.1016/S1473-3099(22)00138-4
![]() |
[32] |
P. Tupper, C. Colijn, COVID-19 in schools: Mitigating classroom clusters in the context of variable transmission, PLoS Comput. Biol., 17 (2021), e1009120. https://doi.org/10.1371/journal.pcbi.1009120 doi: 10.1371/journal.pcbi.1009120
![]() |
[33] |
T. Imamura, M. Saito, Y.K. Ko, T. Imamura, K. Otani, H. Akaba, et al., Roles of children and adolescents in COVID-19 transmission in the community: A retrospective analysis of nationwide data in Japan, Front. Pediatr., 9 (2021). https://doi.org/10.3389/fped.2021.705882 doi: 10.3389/fped.2021.705882
![]() |
[34] |
T. Akaishi, T. Ishii, Coronavirus disease 2019 transmission and symptoms in young children during the severe acute respiratory syndrome coronavirus 2 Delta variant and Omicron variant outbreaks, J. Int. Med. Res., 50 (2022), 03000605221102079. https://doi.org/10.1177/03000605221102079 doi: 10.1177/03000605221102079
![]() |
[35] |
M. Sasanami, M. Fujimoto, T. Kayano, K. Hayashi, H. Nishiura, Projecting the COVID-19 immune landscape in Japan in the presence of waning immunity and booster vaccination, J. Theor. Biol., 559 (2023), 111384. https://doi.org/10.1016/j.jtbi.2022.111384 doi: 10.1016/j.jtbi.2022.111384
![]() |
[36] | Ministry of Health Labour and Welfare, Visualizing the data: information on COVID-19 infections, (n.d.). https://covid19.mhlw.go.jp/en/ (accessed September 4, 2024) |
[37] | Bureau of Social Welfare and Public Health Tokyo Metropolitan Government, Details of announcement of new coronavirus-positive patients in Tokyo, 2023, (n.d.). https://catalog.data.metro.tokyo.lg.jp/dataset/t000010d0000000068 |
[38] | Alex Selby, Estimating Generation Time Of Omicron - Covid-19, (2022). https://sonorouschocolate.com/covid19/index.php/Estimating_Generation_Time_Of_Omicron (accessed September 4, 2024) |
[39] | National Institute of Infectious Diseases, Estimation of Incubation Period for SARS-CoV-2 Mutant B.1.1.529 Strain (Omicron Strain): Preliminary Report, 2022, (2022). https://www.niid.go.jp/niid/ja/2019-ncov/2551-cepr/10903-b11529-period.html (accessed September 4, 2024) |
[40] |
M. Salmon, D. Schumacher, M. Höhle, Monitoring Count Time Series in R: Aberration detection in public health surveillance, J. Stat. Softw., 70 (2016), 1–35. https://doi.org/10.18637/jss.v070.i10 doi: 10.18637/jss.v070.i10
![]() |
[41] |
C. Fraser, Estimating individual and household reproduction numbers in an emerging epidemic, PLoS One, 2 (2007), e758. https://doi.org/10.1371/journal.pone.0000758 doi: 10.1371/journal.pone.0000758
![]() |
[42] |
H. Nishiura, Time variations in the transmissibility of pandemic influenza in Prussia, Germany, from 1918–19, Theor. Biol. Med. Model., 4 (2007), 20. https://doi.org/10.1186/1742-4682-4-20 doi: 10.1186/1742-4682-4-20
![]() |
[43] | H. Nishiura, G. Chowell, The effective reproduction number as a prelude to statistical estimation of time-dependent epidemic trends, in: G. Chowell, J.M. Hyman, L.M.A. Bettencourt, C. Castillo-Chavez (Eds.), Mathematical and Statistical Estimation Approaches in Epidemiology, Springer Netherlands, Dordrecht, 2009: pp. 103–121. https://doi.org/10.1007/978-90-481-2313-1_5 |
[44] |
T. Sanada, T. Honda, F. Yasui, K. Yamaji, T. Munakata, N. Yamamoto, et al., Serologic survey of IgG against SARS-CoV-2 among hospital visitors without a history of SARS-CoV-2 infection in Tokyo, 2020–2021, J. Epidemiol., 32 (2022), 105–111. https://doi.org/10.2188/jea.JE20210324 doi: 10.2188/jea.JE20210324
![]() |
[45] | Ministry of Internal Affairs and Communications Statistics Bureau, Statistics Bureau Homepage/Population Estimates/Population Estimates (as of October 1, 2022) - Nationwide: Age (each year), Population by Gender; Prefectures: Age (5-year age groups), Population by Gender. (in Japanese), 2023, (2023). https://www.stat.go.jp/data/jinsui/2022np/index.html (accessed September 4, 2024) |
[46] | R: The R Project for Statistical Computing, (2023). https://www.r-project.org/ (accessed September 4, 2024) |
[47] | J. Gabry, R. Češnovar, A. Johnson, S. Bronder, R Interface to CmdStan, (2023). https://mc-stan.org/cmdstanr/ (accessed September 4, 2024) |
[48] | Stan Development Team, Stan modeling language users guide and reference manual, Version 2.32, 2023, (2023). https://mc-stan.org/ (accessed September 4, 2024) |
[49] |
L. Casini, M. Roccetti, Reopening Italy's schools in September 2020: A Bayesian estimation of the change in the growth rate of new SARS-CoV-2 cases, BMJ Open, 11 (2021), e051458. https://doi.org/10.1136/bmjopen-2021-051458 doi: 10.1136/bmjopen-2021-051458
![]() |
[50] |
T. Braeye, L. Catteau, R. Brondeel, J.A.F. van Loenhout, K. Proesmans, L. Cornelissen, et al., Vaccine effectiveness against transmission of alpha, delta and omicron SARS-COV-2-infection, Belgian contact tracing, 2021–2022, Vaccine, 41 (2023), 3292–3300. https://doi.org/10.1016/j.vaccine.2023.03.069 doi: 10.1016/j.vaccine.2023.03.069
![]() |
![]() |
![]() |
Scenarios | Δλ(S,A) (95% CrI) |
0 | 222.8 (216.6,229.1) |
1 | -310.6 (-322.1, -299.7) |
2 | -335.8 (-348.2, -324.1) |
3 | -646.5 (-670.1, -624.3) |
4 | -2101 (-2161, -2044) |