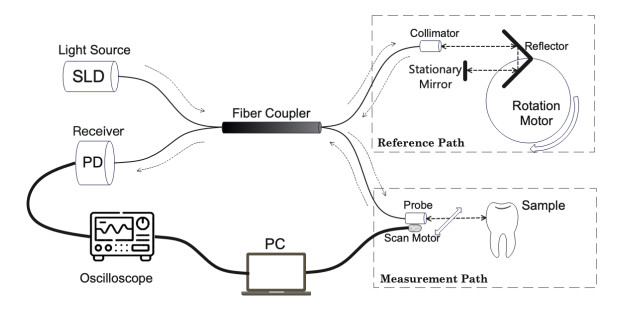
Optical coherence tomography (OCT) has been widely used for the diagnosis of dental demineralization. Most methods rely on extracting optical features from OCT echoes for evaluation or diagnosis. However, due to the diversity of biological samples and the complexity of tissues, the separability and robustness of extracted optical features are inadequate, resulting in a low diagnostic efficiency. Given the widespread utilization of entropy analysis in examining signals from biological tissues, we introduce a dental demineralization diagnosis method using OCT echoes, employing multiscale entropy analysis. Three multiscale entropy analysis methods were used to extract features from the OCT one-dimensional echo signal of normal and demineralized teeth, and a probabilistic neural network (PNN) was used for dental demineralization diagnosis. By comparing diagnostic efficiency, diagnostic speed, and parameter optimization dependency, the multiscale dispersion entropy-PNN (MDE-PNN) method was found to have comprehensive advantages in dental demineralization diagnosis with a diagnostic efficiency of 0.9397. Compared with optical feature-based dental demineralization diagnosis methods, the entropy features-based analysis had better feature separability and higher diagnostic efficiency, and showed its potential in dental demineralization diagnosis with OCT.
Citation: Ziqi Peng, Seiroh Okaneya, Hongzi Bai, Chuangxing Wu, Bei Liu, Tatsuo Shiina. Proposal of dental demineralization diagnosis with OCT echo based on multiscale entropy analysis[J]. Mathematical Biosciences and Engineering, 2024, 21(3): 4421-4439. doi: 10.3934/mbe.2024195
[1] | Xueyan Wang . A fuzzy neural network-based automatic fault diagnosis method for permanent magnet synchronous generators. Mathematical Biosciences and Engineering, 2023, 20(5): 8933-8953. doi: 10.3934/mbe.2023392 |
[2] | Dehua Feng, Xi Chen, Xiaoyu Wang, Xuanqin Mou, Ling Bai, Shu Zhang, Zhiguo Zhou . Predicting effectiveness of anti-VEGF injection through self-supervised learning in OCT images. Mathematical Biosciences and Engineering, 2023, 20(2): 2439-2458. doi: 10.3934/mbe.2023114 |
[3] | Huanhai Yang, Shue Liu . A prediction model of aquaculture water quality based on multiscale decomposition. Mathematical Biosciences and Engineering, 2021, 18(6): 7561-7579. doi: 10.3934/mbe.2021374 |
[4] | Dae Hyeon Kim, Jin-Oh Park, Dae-Young Lee, Young-Seok Choi . Multiscale distribution entropy analysis of short epileptic EEG signals. Mathematical Biosciences and Engineering, 2024, 21(4): 5556-5576. doi: 10.3934/mbe.2024245 |
[5] | Enas Abdulhay, Maha Alafeef, Hikmat Hadoush, V. Venkataraman, N. Arunkumar . EMD-based analysis of complexity with dissociated EEG amplitude and frequency information: a data-driven robust tool -for Autism diagnosis- compared to multi-scale entropy approach. Mathematical Biosciences and Engineering, 2022, 19(5): 5031-5054. doi: 10.3934/mbe.2022235 |
[6] | Javad Hassannataj Joloudari, Faezeh Azizi, Issa Nodehi, Mohammad Ali Nematollahi, Fateme Kamrannejhad, Edris Hassannatajjeloudari, Roohallah Alizadehsani, Sheikh Mohammed Shariful Islam . Developing a Deep Neural Network model for COVID-19 diagnosis based on CT scan images. Mathematical Biosciences and Engineering, 2023, 20(9): 16236-16258. doi: 10.3934/mbe.2023725 |
[7] | Zijian Wang, Yaqin Zhu, Haibo Shi, Yanting Zhang, Cairong Yan . A 3D multiscale view convolutional neural network with attention for mental disease diagnosis on MRI images. Mathematical Biosciences and Engineering, 2021, 18(5): 6978-6994. doi: 10.3934/mbe.2021347 |
[8] | Yue Li, Hongmei Jin, Zhanli Li . A weakly supervised learning-based segmentation network for dental diseases. Mathematical Biosciences and Engineering, 2023, 20(2): 2039-2060. doi: 10.3934/mbe.2023094 |
[9] | Akansha Singh, Krishna Kant Singh, Michal Greguš, Ivan Izonin . CNGOD-An improved convolution neural network with grasshopper optimization for detection of COVID-19. Mathematical Biosciences and Engineering, 2022, 19(12): 12518-12531. doi: 10.3934/mbe.2022584 |
[10] | Shaojun Zhu, Jinhui Zhao, Yating Wu, Qingshan She . Intermuscular coupling network analysis of upper limbs based on R-vine copula transfer entropy. Mathematical Biosciences and Engineering, 2022, 19(9): 9437-9456. doi: 10.3934/mbe.2022439 |
Optical coherence tomography (OCT) has been widely used for the diagnosis of dental demineralization. Most methods rely on extracting optical features from OCT echoes for evaluation or diagnosis. However, due to the diversity of biological samples and the complexity of tissues, the separability and robustness of extracted optical features are inadequate, resulting in a low diagnostic efficiency. Given the widespread utilization of entropy analysis in examining signals from biological tissues, we introduce a dental demineralization diagnosis method using OCT echoes, employing multiscale entropy analysis. Three multiscale entropy analysis methods were used to extract features from the OCT one-dimensional echo signal of normal and demineralized teeth, and a probabilistic neural network (PNN) was used for dental demineralization diagnosis. By comparing diagnostic efficiency, diagnostic speed, and parameter optimization dependency, the multiscale dispersion entropy-PNN (MDE-PNN) method was found to have comprehensive advantages in dental demineralization diagnosis with a diagnostic efficiency of 0.9397. Compared with optical feature-based dental demineralization diagnosis methods, the entropy features-based analysis had better feature separability and higher diagnostic efficiency, and showed its potential in dental demineralization diagnosis with OCT.
Dental caries is a chronic bacterial infection of the enamel, manifested by the demineralization of inorganic matter and decomposition of organic matter. The disease progresses from a change in color to the development of substantial lesions over time. In addition to secondary pulpitis and periapical inflammation, dental caries may also cause alveolar bone and jaw inflammation. Lesions continue to develop without timely treatment, forming cavities, and eventually, the dental crown is destroyed and lost [1,2]. The most common method of checking dental caries is using a metal probe to scratch the surface of the tooth and examine for cavities. The examination is accompanied by pain and discomfort associated with tooth decay. Additionally, oral X-rays and oral CT imaging are widely used for the examination of dental caries as non-contact and non-destructive methods. Using oral X-ray imaging, researchers investigated a variety of clinical dental problems [3,4]. However, oral X-ray imaging technology involves radiation, which imposes a physical and psychological burden on patients. An optical coherence tomography (OCT) is a new type of tomography based on the principle of an optical interferometer. The optical properties of biological tissue are evaluated by detecting reflected or backscattered signals within about 1mm depth and scanning allows for the acquisition of two-dimensional or three-dimensional images of biological tissues. As a typical measurement application, OCT can be utilized to image bacterial biofilms for the monitoring of their growth dynamics and destructions [5,6].
Many clinical fields have benefited from OCT, which was initially applied in clinical and preclinical ophthalmology [7,8,9,10,11], followed by relevant applications in dermatology [12,13] and dentistry. Particularly in dentistry, Oliveira used OCT image to study root cracks diagnosis [14] and Shimada used OCT image to study caries, tooth fracture diagnosis, and interfacial space detection [15,16,17]. Most of the evaluation or diagnostic methods based on OCT rely on the optical features extracted from OCT echo signals. For example, Chan and Kang evaluated the degree of demineralization and remineralization, respectively, using the reflectivity integration of OCT echo signals as features [18,19]. Popescu used another optical feature, the attenuation of OCT echoes, to assess dental demineralization [20]. Although these studies provided abundant evidence for the correlation between the variations of optical features and the degree of demineralization, the separability and robustness of extracted optical features are inadequate. Moreover, the approximate fitting process used to solve for optical features also introduce errors to the extracted features. Therefore, using these optical features for dental demineralization diagnosis cannot provide good performance in diagnostic efficiency [20]. It is expected that a more effective feature extraction method can be developed to improve the separability and robustness of the extracted features, which can help to significantly enhance diagnostic efficiency.
Entropy analysis, as a common nonlinear dynamic analysis method, has been widely applied to the feature extraction of nonlinear signals, such as permutation entropy (PE) [21], fuzzy entropy (FE) [22], and dispersion entropy (DE) [23]. Unlike approximate fitting solutions for features, entropy analysis can comprehensively and accurately reflect the complexity of nonlinear signals. The effectiveness of entropy analysis methods in feature analysis of nonlinear signals has been extensively demonstrated. However, when extracting features from signals of highly complex biological tissues, it may not achieve a good diagnostic result. Multiscale analysis is proposed as a way of improving the reliability of features provided by entropy analysis. It is capable of reflecting the complexity of signals more comprehensively and providing more information about the signal from biological tissue. Feature extraction from complex biological signals such as electroencephalogram (EEG) and electrocardiogram (ECG) is widely performed using the entropies based on multiscale analysis. Multiscale fuzzy entropy (MFE) was used by Li to extract features from motor imagery EEG data [24,25]. Ahmed applied MFE to uterine electromyography analysis [26]. Liu analyzed ECG signals in patients with congestive heart failure, young healthy adults, and the elderly [27]. Using multiscale permutation entropy (MPE), Li studied the effects of sevoflurane anesthesia on the cerebral cortex [28]. MPE was employed by Ouyang to identify epilepsy and differentiate among normal, pre-seizure, and seizure states [29]. Despite the widespread use of these multiscale entropy (ME) methods in medicine, there are still some limitations. As MFE calculation is complex and time consuming, it is not suitable for long time series. Despite the simplicity of MPE, it fails to take into account the magnitude relationship between the amplitudes. In contrast, multiscale dispersion entropy (MDE) overcomes the aforementioned limitations and offers advantages such as anti-noise and robustness, fast computation speed, less impact from abrupt signals, and amplitude information when analyzing the complexity of time series [23]. A study conducted by Azami demonstrated the superiority of the MDE in the analysis of biological signals [30]. MDE analysis was conducted by Liu to evaluate the characteristics of denatured tissue during ultrasound treatment [31]. Sukriti monitors epileptic symptoms by analyzing EEG signals in epileptic patients using MDE [32].
Another important step in the diagnostic process is the use of classifiers to make decisions about extracted features. Machine learning, as an artificial intelligence algorithm, plays a crucial role not only in system optimization [33] but is also frequently employed as a classifier in the applications of bio signal recognition. Sangeetha used image processing technology and support vector machines to detect and classify periapical dental X-ray images [34]. Justiawan used a comparative analysis of color matching system and K-nearest neighbors to classify and identify dental images [35]. Hashem et al. used an improved recurrent neural network to examine the longevity of dental restoration [36]. Kaya et al. proposed a convolutional neural network method for primary and permanent tooth detection and enumeration on pediatric dental radiographs [37]. A probabilistic neural network (PNN) is an artificial intelligence algorithm based on Bayesian decision theory and the Parzen window probability density function [38]. In comparison with classifiers, PNN offers the advantages of simple computing mode, fast convergence rate, fast training rate, and always converges to the Bayesian optimal solution. Moreover, PNN is commonly used for multi-objective classification, diagnosis, and prediction of denatured biological tissues, with extremely high stability [39].
In view of widespread application of entropy analysis in the diagnosis of biological tissue signals, a dental demineralization diagnosis with OCT echo based on multiscale entropy analysis is proposed in this study. Through evaluating the diagnostic results of dental demineralization using this method, the effectiveness of multiscale entropy analysis in OCT-based biological tissue signal diagnosis can be demonstrated, thus providing a new analytical approach for OCT-based biological tissue signal diagnosis. In this study, three multiscale entropy analysis methods were employed to extract entropy features from dental OCT echo signals and PNN was used to diagnose dental demineralization based on the extracted features. Then, the practicability of the three methods in dental demineralization diagnosis was evaluated comprehensively. On this basis, the entropy analysis method with the best performance was selected and compared with the optical feature analysis method in performance.
Essentially, this paper is organized as follows: The first section provides an overview of OCT in the diagnosis of dental demineralization, as well as the use of multiscale entropy analysis and classifiers in the diagnosis of biological tissues. The second section describes the principle of MDE and PNN. The third section addresses the principle of OCT measurement, the OCT equipment used for measurement, and the experimental data acquisition. The fourth section presents the results of feature extraction and dental demineralization diagnosis based on three multiscale entropy analysis methods, as well as performance comparisons. The superiority of entropy analysis methods compared to optical feature analysis is also discussed in this section. The fifth section is a conclusion.
Despite the fact that MFE, MPE, and MDE methods were used in this study, this section only provides a detail of the algorithm employing MDE and PNN, as it demonstrated superior performance in diagnosing dental demineralization. However, MFE and MPE are widely utilized in various applications, and the specific algorithms can be referred to [24,25,26,27,28,29].
DE can be calculated by the time series X={x1,x2,⋯,xn} through the following steps [23]:
(a). Mapping X={x1,x2,⋯,xn} to Y={y1,y2,⋯,yn} by normal cumulative distribution function (NCDF).
yj=1σ√2π∫xj−∞e−(t−μ)22σ2dt, | (1) |
where σ is the standard deviation and μ is the average amplitude of time series.
(b). Mapping Y={y1,y2,⋯,yn} to Z={zc1,zc2,⋯,zcn}, which is set from 1 to c. Each element of the mapped signal is written as:
zcj=round(c·yj+0.5), | (2) |
where zcj is the jth element of the classified time series and round is the rounding operation.
(c). Reconstructing the embedding vector zm,ci with the embedding dimension m, number of classes c and the delay time d as follow:
zm,ci={zci,zci+d,...,zci+(m−1)d},i=1,2,...N−(m−1)d. | (3) |
(d). Mapping zm,ci to dispersion patterns πv0v1...vm−1, where zci=v0,zci+d=v1,...,zci+(m−1)d=vm−1. The total number of possible dispersion patterns of each zm,ci is equal cm.
(e). The relative frequency of each dispersion pattern can be calculated as follow:
p(πv0v1...vm−1)=Number(πv0v1...vm−1)N−(m−1)d, | (4) |
where Number(πv0v1...vm−1) is the mapping number of πv0v1...vm−1.
The DE can be calculated using Shannon's entropy as follow:
DE(x,m,c,d)=−∑cmπ=1p(πv0v1...vm−1)ln(p(πv0v1...vm−1)). | (5) |
The calculation of MDE consists of two parts: the coarse-grained process of time series and the entropy calculation of each coarse-grained sequence using any single scale entropy estimation algorithm.
For original signal X={x1,x2,⋯,xn}, it is divided into different segments based on the parameter τ known as the scale factor. Data points in each segment are averaged to produce coarse-grained time series, according to Eq (6).
M(τ)k,j=1τ∑jτ+k−1n=(j−1)τ+kxn,1≤j≤Nτ,1≤k≤τ. | (6) |
Repeat process (a)–(d) to calculate DE of each coarse-grained time series. The MDE can be expressed as follow [31]:
MDE(x,m,c,d,τ)=DE(M(τ)k,m,c,d). | (7) |
PNN is a radial basis network based on Parzen window estimation of probability density functions and Bayesian decision theory. The diagnosis process of PNN is as follows [39]:
(ⅰ). Normalizing the sample matrix, and deriving Euclidean distance between the normalized sample and the training sample as:
E=[E11E12E21E22…E1m…E2m⋮⋮Ep1Ep2⋱⋮…Epm]=[√∑nk=1|d1k−c1k|2√∑nk=1|d1k−c2k|2√∑nk=1|d2k−c1k|2√∑nk=1|d2k−c2k|2…√∑nk=1|d1k−cmk|2…√∑nk=1|d1k−cmk|2⋮⋮√∑nk=1|dpk−c1k|2√∑nk=1|dpk−c2k|2⋱⋮…√∑nk=1|dpk−cmk|2]. | (8) |
Calculating the initial probability matrix P by utilizing the radial basis function as an activation function. Where σ is the smoothing factor.
P=[P11P12P21P22…P1m…P2m⋮⋮Pp1Pp2⋱⋮…Ppm]=[exp−E112σ2exp−E122σ2exp−E212σ2exp−E222σ2…exp−E1m2σ2…exp−E2m2σ2⋮⋮exp−Ep12σ2exp−Ep12σ2⋱⋮…exp−Epm2σ2]. | (9) |
(ⅱ). According to the result from Eq (9), calculating the initial probability sum of the diagnosis type belonging to the test sample in probabilistic neural networks.
S=[S11S12S21S22…S1m…S2m⋮⋮Sp1Sp2⋱⋮…Spm]=[∑kl=1P1l∑k+1l=2P1l∑kl=1P2l∑k+1l=2P2l…∑ml=m−k+1P1l…∑ml=m−k+1P2l⋮⋮∑kl=1Ppl∑k+1l=2Ppl⋱⋮…∑ml=m−k+1Ppl]. | (10) |
(ⅲ). Calculating the maximum probability of the ith sample to be diagnosed as the class j.
OCT works in a similar way to the optical interferometer. Using the low coherence light source, tomography is obtained by interfering with the reference path and the measurement path. Figure 1 shows the schematic diagram of the time-domain (TD) OCT used in this study. Light from a superluminescent diode (SLD) (Anritsu, AS3E113HJ10M, Center wavelength: 1.32 μm, Bandwidth: 43 nm, Coherence length: 17.8 μm, Power: 1.4 mW) enters the fiber and is separated into two paths by a 2 × 2 fiber coupler. The reference light is reflected by the mirror and returned to the fiber coupler, and the measurement light is backscattered in the sample and also returned to the fiber coupler. Two paths of light interfere and are detected by a photodiode (PD). The interference occurs when the difference in optical paths between the references path and measurement path is smaller than the coherence length of the light source. The output of PD is proportioned to the amount of backscattering on the sample. It is possible to obtain interference signals between measurement light and reference light coming from different depths by moving the reflector. An optical characteristic of the sample at different depths (z-axis) can be determined by the position of the reflector and the intensity of the interference signal. In combination with rotation scanning of the measurement light in different directions (x, y-axis), it is possible to determine the 3D optical characteristics of the sample by computer processing. Figure 2 depicts the image of the incisor being measured with OCT system.
In traditional TD-OCT, a reflecting mirror is placed at the end of the reference path, and the scanning process is realized by linear movement of the mirror. This mechanism, however, requires the mirror to be returned from the scanning end point to the starting point between two successive scans. The rapid inversion of motors can lead to errors that affect the accuracy of depth direction of OCT. Using a rotating motor (25 r/s) and a right-angle mirror can avoid the precision error caused by reversing the speed motor. Referring to Figure 1, the reflecting mechanism can reflect the light emitted from the collimator back to the collimator position. In the range of ± 20 degrees in rotational motion, displacement of the reflector and time can be approximated linearly, which is equivalent to the effect of linear scanning [40,41,42]. A benefit of this rotating reflection mechanism is that it does not require adjusting the position of the mirror through the motor reversal motion between two consecutive scans, which can reduce the errors caused by the motor reversal motion.
Figure 3 illustrates the results of teeth measurements using OCT. The system has a depth resolution of 3 μm, while the scanning resolution is 0.2 mm. Figure 3(a) illustrates the result of a typical tooth scan and illustrates enamel and dentin, two important components of the tooth. Enamel is located in the outer layer of the tooth and is approximately 1.5 mm thick (the average is about 2 mm), and the dentin is located below the enamel. Because of the strong scattering of light in teeth, only part of the dentin signal is detected by OCT. During the measurement, the measurement beam should be adjusted perpendicular to the sample surface to increase backscattering to ensure that OCT can obtain deeper backscattered signals. Nevertheless, since the surface of the tooth is irregularly curved, it is difficult to ensure that the measurement beam is always perpendicular to it. Thus, the measurement beam cannot be well transmitted to the inside of the sample, reducing the distance of remote sensing. Figure 3(b), (c) shows OCT measurements of normal and decalcified teeth, respectively. Due to the large surface curvature of the tooth and the fact that the measurement light does not penetrate deep into the tooth, only the echoes of the enamel are measured in both of these results. Since, dental demineralization is primarily characterized by changes in the optical properties of the enamel. Hence, even if only the echo signal of tooth enamel can be used as a diagnostic basis for dental demineralization. Figure 3(d) illustrates the A-scan (Axial-scan) signals extracted from the position pointed by the dotted arrows in Figure 3(b), (c), respectively. An echo signal is generated at the interface between air and enamel in both cases, and it decreases with increasing depth. Echoes from enamel vary depending on the state of the enamel. However, it is difficult to represent the changing trend uniformly in the time domain waveform due to the difference of optical properties and surface curvature of individual teeth. Thus, it is necessary to extract a feature that is more effective than optics in order to facilitate the improvement of dental demineralization diagnosis.
A total of 26 healthy teeth, including molars, premolars, canines and incisors, were used in this study, all obtained through wisdom tooth surgery or orthodontic surgery. Echo signals from various parts of the dental crown was used to verify the applicability of the diagnostic method for demineralization proposed in this study. A total of 78 tooth sectional images were obtained using OCT B-scan on the anterior, lateral, and top parts of healthy teeth, and 314 echo signal time series of normal teeth were extracted randomly from the 78 sectional images. To simulate different levels of demineralization on the surface of teeth, the four teeth were immersed in acetic acid solutions with concentration of 4.5% for 3 hours, 6 hours, and 12 hours, respectively [43,44]. OCT echoes of the demineralized teeth at different time points were measured using the same method, and a total of 628 sequences were obtained. The OCT echoes mentioned in this study refer to the A-scan signals of OCT in Figure 3(d).
In the depth direction, the sequence length of the original echo signal is 2500 points (resolution 3 μm, depth 7.5 mm). As can be seen in Figures 2(a)–(c), the OCT echo signals in the depth direction are concentrated in the middle of the image, while the remainder is primarily background noise. Since this study focused on the status of enamel demineralization, the echo of enamel layer can reflect the characteristics of demineralization well. It is important to note that if the sequence contains too much background noise, this will affect the separability of the extracted features. Thus, 300 points (mainly enamel, 0.9 mm) were selected for feature extraction and demineralization diagnosis in this study.
MPE, MFE, and MDE were used to extract the features of normal and decalcified teeth, respectively. Based on three feature extraction methods after parameter optimization, Figure 4 shows the mean value and standard deviation (error bars) of feature entropy under various scale factors. X-axis represents the scale factor, y-axis represents the entropy calculated using various methods, and the error bar represents the variance of the entropies. In all three methods, the average entropy of normal teeth is higher than that of decalcified teeth. Taking the signal intensity at the enamel interface as a reference, as displayed in Figures 3(b), (c), the signal intensity deep in the enamel of normal teeth is higher than those of demineralized teeth. Consequently, the chaos of the signal from normal teeth increases, which is reflected in the increase in entropy. This is consistent with the results of the three entropy analyses.
In terms of feature extraction, MPE shown in Figure 4(a) has the lowest performance among the three methods. As the scale factor is 1–4, the mean MPE for the two types of echoes are distinguishable; however, as the scale factor is increased, the complexity difference between the two types of echoes gradually disappears, and the mean MPE of the two types of echoes gradually overlapped. The intersection of the normal curve and the decalcification curve may be a cross-over artifact in the multiscale entropy analysis [45]. The reason may be that the length of coarse-grained time series in MPE became short with the increase of scale factor, resulting in the loss of time series information. At most of the scales, MFE shown in Figure 4(b) is able to distinguish the features between the two types of teeth. The MFE of the two types of samples are stable and well separable when the scale factor is 3–15, which can be utilized as a diagnostic indicator of dental demineralization. Nevertheless, when the scale factor is greater than 15, the fluctuation of MFE of normal sample echo signal increases with the increase of scale factor, and the separability becomes low. In the case of large-scale factors, MFE is not suitable for the diagnosis of dental demineralization. Additionally, compared to the other two methods, MFE has a higher time consumption. Despite the fact that MDE shown in Figure 4(c) fluctuates within an acceptable range at all scale factors, its separability is superior. The MDE feature extraction has a good performance at all scales when applied to the diagnosis of dental demineralization.
In this study, PNN was used to diagnose dental demineralization based on extracted features. Based on the preprocessed data discussed in Section 3.2,150 normal and demineralization samples were randomly selected, and three entropy analysis methods were used to calculate the entropy features, and then a PNN was used to train the model. Excluding the training data, more 150 normal and demineralization samples were randomly selected from the preprocessed data, and the PNN diagnostic test was carried out after the calculating the entropy features using the three entropy analysis methods. To eliminate the influence of data randomness on the results, the diagnostic process was repeated 50 times, with parameter optimization conducted for different data in each iteration. The average of the 50 results was taken as the diagnostic outcome.
We defined TP as true positive, TN as true negative, FP as false positive, and FN as false negative. Sensitivity, specificity, and diagnostic efficiency were calculated using PNN. Table 1 shows the comparison of the diagnostic results of dental demineralization by three methods based on single scale entropy feature. The three parameters of diagnostic results based on MPE entropy analysis method are only about 0.75, which indicates the result of poor separability of MPE features mentioned in Section 4.1. On the other hand, the methods based on MFE and MDE both perform well in diagnostic efficiency entropy, which are 0.9235 and 0.9118, respectively. The sensitivity is 0.9682 and 0.9758, and the specificity is 0.8844 and 0.8478, respectively. The sensitivity of both diagnostic methods is higher than the specificity, which is because the entropy features of decalcified samples are more stable, which can be demonstrated by the entropy feature distribution in Figure 4. Comparatively to MPE, the features extracted from MFE and MDE more accurately reflected the state of enamel tissue. The diagnosis of dental demineralization by OCT is based on the optical properties of tooth enamel, and the amplitude and attenuation of optical signals are important indicators of tooth status. In the calculation of MPE, however, the probability is based on the tendency of the signal sequence arrangement. Probability and entropy cannot be affected by changes in signal amplitude and decay rate in the same arrangement trend. MPE is an entropy feature that ignores the amplitude and the rate of change of the signal and is not suitable for diagnostic applications based on OCT echo signals. On the other hand, MFE and MDE take into account the amplitude of the signal when calculating fuzzy membership degree Dmi,j and NCDF, as well as the signal decay rate. Thus, MFE and MDE features can be used to achieve higher diagnostic efficiency in the diagnosis of dental decalcification.
Parameters | MPE-PNN | MFE-PNN | MDE-PNN |
Sensitivity (TP / (TP + FN)) | 0.7470 | 0.9682 | 0.9758 |
Specificity (TN / (TN + FP)) | 0.7562 | 0.8844 | 0.8478 |
Diagnostic Efficiency((TP+TN) / Total) | 0.7516 | 0.9263 | 0.9118 |
Entropy analysis methods (MPE, MFE, and MDE) at different scales have different abilities to characterize sample features. Figure 5 illustrates the diagnostic efficiency of dental demineralization diagnosis using different scales calculated by the three methods (as shown in Figure 4). MPE-PNN has a low diagnostic efficiency at all scales in diagnosis of dental demineralization. As described in Section 4.2, because the signal amplitude is ignored in the calculation of the entropy of MPE, the calculated entropy cannot reflect the amplitude characteristics of the sample. MPE feature extraction is not appropriate for diagnostic applications using OCT signals. In contrast to MPE, MFE, and MDE are able to accurately capture the sample features on most scales, resulting in higher diagnostic efficiency. As mentioned in Section 4.2, in MFE and MDE, signal amplitude is incorporated into the calculation of the fuzzy membership degree Dmi,j and NCDF. Thus, MFE-PNN and MDE-PNN are able to achieve a higher diagnostic efficiency in the diagnosis of dental demineralization. For single feature diagnosis, when the scale factor is 5, the MFE-PNN method provides the highest diagnostic efficiency, 0.9263. As the scale factor increases, however, the diagnostic efficiency of MFE-PNN gradually decreases and becomes unstable. In comparison to MFE-PNN, MDE-PNN stabilized its diagnostic efficiency about 0.91 within the range of scale factors 1–20.
Table 2 shows the maximum diagnostic efficiency of the three methods in single feature diagnostic mode under different embedding dimension m. Due to the inadequacy of MPE-PNN for diagnosis of OCT signals, parameter optimization does not work in improving diagnosis efficiency. The MFE-PNN method achieves the highest diagnostic efficiency of 0.9263 at m = 2, however, its diagnostic efficiency rapidly decreases as m is increased. The diagnostic efficiency of MFE-PNN is highly dependent upon the optimization of parameters. In contrast, adjusting the parameter near the optimal parameter has little effect on the diagnostic efficiency of the MDE method, which is always around 0.91 The feature extraction of MDE does not require much parameter optimization.
Embedded dimension | m = 2 | m = 3 | m = 4 |
MPE-PNN | 0.7516 | 0.5378 | 0.5306 |
MFE-PNN | 0.9263 | 0.8966 | 0.8384 |
MDE-PNN | 0.9118 | 0.9112 | 0.9090 |
Differences in stability between MFE and MDE are primarily due to the amplitude mapping process. Using the maximum difference in amplitude between reconstructed sequences, MFE maps amplitude information to fuzzy membership degree Dmi,j. The amplitude mapping of MDE takes the form of NCDF, and the integral calculation can keep the output entropy more robust. Despite the fact that MFE feature extraction may achieve the highest diagnostic efficiency at a few scales with proper parameter optimization, its robustness is not as good as MDE. The MDE feature extraction method has a low dependency on parameter optimization and is more suitable for biological tissue signals with high volatility and randomness.
In diagnostic applications, multiple features are often combined to improve diagnostic efficiency when diagnosis with a single feature is inefficient. Several features (shown in Figure 4) were selected based on the sequence of scale factors for the diagnosis of dental demineralization, as shown in Figure 6. In the multi-feature diagnosis mode, the diagnostic efficiency of MPE-PNN converges to about 0.82 with the increase in the number of features. Due to the fact that MPE cannot correctly characterize the TD-OCT echo signal at the high scale factor, the diagnostic efficiency of multi-feature diagnosis is low. In the MFE-PNN method, the features extracted at low scale are not accurate, resulting in a diagnostic efficiency of only 0.85. As the number of features increases, diagnostic efficiency improves rapidly and converges to approximately 0.95. Compared with the maximum diagnostic efficiency of 0.9263 under single feature diagnosis mode, the diagnostic efficiency increased to 0.9497 through multi-feature diagnosis. The multi-feature diagnosis based on MDE-PNN has a high diagnostic efficiency of 0.9118 at low scale. With the increase of feature number, the diagnostic efficiency gradually converges to around 0.94. The multi-feature based MDE-PNN diagnostic efficiency converges relatively slowly, which also proves the stability of MDE-PNN feature extraction. Despite the fact that multiple features can improve diagnostic efficiency, calculating them at different scales requires more time. It is also important to consider the time consumption of clinical applications.
A comparison of the computational execution times of three diagnostic methods for dental demineralization was conducted on the same PC (CPU: Intel, Core i7-12700H, RAM: 16 G-4800 Hz, GPU: RTX3500-4 GB), as shown in Table 3. Feature extraction time refers to the time required to calculate features under 20 scales. MPE and MDE are calculated in 1.79 and 1.45 s, respectively. MFE consumes the most time with 15.19 s and has the lowest computing efficiency. The diagnosis time for single feature diagnosis and multi-feature diagnosis modes was investigated and the multi-feature diagnosis was performed with 9 features. Despite the fact that multi-feature diagnosis takes longer than single feature diagnosis, the percentage increase in time is small. It is recommended to utilize the multi-feature diagnosis mode on the premise that the differences in features between the scales are obvious, which can enhance diagnostic efficiency at a low time cost.
Method | Feature Extraction (20 scales) | Diagnosis (Single feature) | Diagnosis (Multiple features) |
MPE-PNN | 1.79 s | 1.05 s | 1.12 s |
MFE-PNN | 15.19 s | 1.03 s | 1.08 s |
MDE-PNN | 1.49 s | 0.88 s | 1.06 s |
As a result of many aspects of analysis, MDE-PNN has low dependence on parameter optimization, stable high diagnostic efficiency, and low time consumption, making it suitable for the diagnosis of dental demineralization in OCT measurement.
Using the feature extraction method described in reference [21], optical features (attenuation rate) were extracted from the 600 one-dimensional OCT echo series mentioned in Section 4.2. To evaluate the separability of entropy feature, the entropy feature extracted by MDE was compared with optical features on various parameters. Table 4 shows the comparison of parameters derived from the features extracted with the different two methods. The separability of the features is defined as follow:
sp=(avgn−avgd)/[(sdn+sdd)/2], | (11) |
where avg is the mean of the feature, sd is the standard deviation, and n and d subscripts represent normal and demineralization states, respectively. A higher sp value indicates better separability of the features between normal and demineralization states. The sp of entropy features is 1.7658, while the sp of optical features is 1.0000, indicating that the entropy feature has the better separability, which helps to achieve higher diagnostic efficiency. In addition, the upper quartile and lower quartile of the two features were compared. It is found that the lower quartile of the entropy feature in the normal state is greater than the upper quartile of the entropy feature in the demineralization state, with no overlap between the quartiles. On the other hand, the lower quartile of the optical feature in the normal state is smaller than the upper quartile of the entropy feature in the demineralization state, with overlap between the quartiles. This also indicates that the separability of the entropy feature is better than that of the optical feature.
Parameters | Entropy features | Optical features (Attenuation rate) (μm-1) | ||
Normal | Demineralization | Normal | Demineralization | |
Average | 1.0438 | 0.4473 | 0.0240 | 0.0167 |
Standard deviation | 0.5651 | 0.1905 | 0.0088 | 0.0058 |
Upper quartile | 1.5369 | 0.4778 | 0.0306 | 0.0202 |
Lower quartile | 0.4943 | 0.3244 | 0.0169 | 0.0109 |
The dental demineralization diagnosis was performed using PNN based on the extracted optical features, and compared with the diagnostic results of entropy features extracted by MDE, as shown in Table 5. Similar to the diagnostic results of MDE entropy features, the diagnosis of dental demineralization using optical features was the average of 100 diagnostic results. The diagnostic results of the two features were evaluated in terms of sensitivity, specificity, and diagnostic efficiency. It can be seen that the diagnostic method based on MDE feature extraction is superior to the diagnosis based on optical feature extraction in all three parameters.
Parameters | Entropy feature analysis (Single feature) | Optical feature analysis (Single feature) |
Sensitivity | 0.9758 | 0.8250 |
Specificity | 0.8478 | 0.7084 |
Diagnostic efficiency | 0.9118 | 0.7667 |
The dental demineralization diagnosis based on multiscale entropy analysis has shown good performance in feature separation, diagnostic efficiency, and diagnostic speed. However, this method still faces challenges on OCT data availability and reproducibility when it is applied to clinical diagnosis.
1). Reproducibility of data acquisition: In a laboratory setting, the OCT data of the front, top, and side of teeth can be obtained using a mechanical scanning device. In clinical practice, it may be more reliant on OCT echo data from the front or inside of teeth for diagnostic purposes. Importantly, it is necessary to ensure that the output beam from OCT is incident perpendicular to the tooth surface to obtain strong backscattered echo signals. This is also a significant challenge for dental practitioners using handheld probes to acquire data. It may be necessary to use OCT with a longer measurement depth and a probe fixation device to ensure that dentist can obtain OCT echo signals suitable for demineralization diagnosis.
2). Data preprocessing for sample diversity: The diversity in the shape and condition of tooth samples can lead to significant variations in data structure and numerical differences, even when using the same OCT device, probe fixation, and measurement parameters. Eliminating the data variability caused by external factors other than demineralization is also another challenge in clinical practice. Designing an algorithm to normalize the original OCT data is also a crucial task for enhancing clinical practicality.
3). The applicability of the algorithm to sample diversity: More than 600 data sets used in this study come from different locations of 26 tooth samples, and these data are not completely independent. However, in clinical diagnostics, the echo signals from patients' teeth are considered completely independent samples. There may be differences in diagnostic efficiency between clinical practice and the experimentally study. Addressing this issue requires ongoing accumulation of independent sample numbers in subsequent studies to further validate the applicability of the diagnostic algorithm to independent data.
To address the limitations of low feature separability and low robustness in optical feature analysis for dental demineralization diagnosis with OCT, a dental demineralization diagnostic method with OCT based on multiscale entropy analysis is proposed in this study. The features extraction from OCT dental echo signals was performed using MFE, MPE, and MDE methods, and the PNN was used to diagnose simulated dental demineralization of extracted features. According to the comparisons of diagnostic efficiency, time consumption, and dependence on parameter optimization, it was found that although the MFE-PNN method can achieve the highest diagnostic efficiency under specific parameters and modes, it is dependent upon the optimization of multiple key parameters and requires a large amount of time. In contrast, the MDE-PNN method not only has good feature separability, but also has a high diagnostic efficiency of 0.9397, low time costs, and low parameter optimization requirements. The results derived using MDE-PNN were also compared with those derived using optical feature analysis method. The comparison indicates that the entropy analysis method performs better than the optical feature analysis method in terms of feature separation and diagnostic efficiency. It can be considered that multiscale entropy analysis has potential in the diagnosis of dental demineralization, and this study also provides a viable approach for other applications of OCT in the diagnosis of biological signals. However, it is necessary to collect more biological samples to verify the applicability of this method on the diversity of biological samples.
However, the optical features of OCT echo are an important index in the diagnosis of early dental demineralization. In future work, to further improve the diagnostic efficiency of dental demineralization diagnosis, it would be beneficial to extract more kinds of features including optical features from OCT echo and combine them with entropy analysis for joint diagnosis.
The authors declare that they have not used Artificial Intelligence (AI) tools in the creation of this article.
This study was supported by Scientific Research Fund of Hunan Provincial Education Department (20B405, 22B0694), and Natural Science Youth Foundation of Hunan Province (2023JJ40462).
The authors declare that there are no conflicts of interest.
[1] |
R. H. Selwitz, A. I. Ismail, N. B. Pitts, Dental caries, Lancet, 369 (2007), 51–59. https://doi.org/10.1016/S0140-6736(07)60031-2 doi: 10.1016/S0140-6736(07)60031-2
![]() |
[2] |
J. D. Featherstone, Dental caries: a dynamic disease process, Aust. Dent. J., 53 (2008), 286–291. https://doi.org/10.1111/j.1834-7819.2008.00064.x doi: 10.1111/j.1834-7819.2008.00064.x
![]() |
[3] | A. L. A. S. Farhan, The modern X-ray imaging manners for diagnosis of the dental diseases, Eurasian J. Phys. Chem. Math., 7 (2022), 138–148. |
[4] |
A. E. Rad, M. S. M. Rahim, H. Kolivand, A. Norouzi, Automatic computer-aided caries detection from dental x-ray images using intelligent level set, Multimed.Tools Appl., 77 (2018), 28843–28862. https://doi.org/10.1007/s11042-018-6035-0 doi: 10.1007/s11042-018-6035-0
![]() |
[5] |
R. K. Meleppat, C. Shearwood, L. K. Seah, M. V. Matham, Quantitative optical coherence microscopy for the in situ investigation of the biofilm, J. Biomed. Opt., 21 (2016), 127002. https://doi.org/10.1117/1.JBO.21.12.127002 doi: 10.1117/1.JBO.21.12.127002
![]() |
[6] | R. K. Meleppat, M. V. Matham, L. K. Seah, C. Shearwood, Quantification of biofilm thickness using a swept source based optical coherence tomography system, in 3rd International Conference on Optical and Photonic Engineering, icOPEN 2015, Singapore, 2015. https://doi.org/10.1117/12.2190106 |
[7] |
G. Rebolleda, L. Diez-Alvarez, A. Casado, C. Sánchez-Sánchez, E. de Dompablo, J. J. González-López, et al., OCT: new perspectives in neuro-ophthalmology, Saudi J. Ophthalmol., 29 (2015), 9–25. https://doi.org/10.1016/j.sjopt.2014.09.016 doi: 10.1016/j.sjopt.2014.09.016
![]() |
[8] |
J. G. Fujimoto, W. Drexler, J. S. Schuman, C. K. Hitzenberger, Optical Coherence Tomography (OCT) in ophthalmology: introduction, Opt. Express, 17 (2009), 3978–3979. https://doi.org/10.1364/oe.17.003978 doi: 10.1364/oe.17.003978
![]() |
[9] |
R. K. Meleppat, K. E. Ronning, S. J. Karlen, M. E. Burns, E. N. Pugh, R. J. Zawadzki, et al., In vivo multimodal retinal imaging of disease-related pigmentary changes in retinal pigment epithelium, Sci. Rep., 11 (2021), 16252. https://doi.org/10.1038/s41598-021-95320-z doi: 10.1038/s41598-021-95320-z
![]() |
[10] |
R. K. Meleppat, P. Zhang, M. J. Ju, S. K. Manna, Y. F. Jian, E. N. Pugh, et al., Directional optical coherence tomography reveals melanin concentration-dependent scattering properties of retinal pigment epithelium, J. Biomed. Opt., 24 (2019), 066011. https://doi.org/10.1117/1.JBO.24.6.066011 doi: 10.1117/1.JBO.24.6.066011
![]() |
[11] | R. K. Meleppat, E. B. Miller, S. K. Manna, P. F. Zhang, E. N. Pugh, R. J. Zawadzki, Multiscale Hessian filtering for enhancement of OCT angiography images, in Ophthalmic technologies XXIX, San Francisco, California, United States, 10858 (2019), 64–70. https://doi.org/10.1117/12.2511044 |
[12] |
J. Welzel, Optical coherence tomography in dermatology: a review, Skin Res. Technol., 7 (2001), 1–9. https://doi.org/10.1034/j.1600-0846.2001.007001001.x doi: 10.1034/j.1600-0846.2001.007001001.x
![]() |
[13] |
M. Mogensen, L. Thrane, T. M. Jørgensen, P. E. Andersen, G. B. Jemec, OCT imaging of skin cancer and other dermatological diseases, J. Biophotonics, 2 (2009), 442–451. https://doi.org/10.1002/jbio.200910020 doi: 10.1002/jbio.200910020
![]() |
[14] |
B. P. de Oliveira, A. C. Câmara, D. A. Duarte, A. S. L. Gomes, R. J. Heck, A. C. Dantas, et al., Detection of apical root cracks using spectral domain and swept-source optical coherence tomography, J. Endodont., 43 (2017), 1148–1151. https://doi.org/10.1016/j.joen.2017.01.019 doi: 10.1016/j.joen.2017.01.019
![]() |
[15] |
Y. Shimada, A. Sadr, Y. Sumi, J. Tagami, Application of optical coherence tomography (OCT) for diagnosis of caries, cracks, and defects of restorations, Curr. Oral Health Rep., 2 (2015), 73–80. https://doi.org/10.1007/s40496-015-0045-z doi: 10.1007/s40496-015-0045-z
![]() |
[16] |
Y. Shimada, A. Sadr, M. F. Burrow, J. Tagami, N. Ozawa, Y. Sumi, Validation of swept-source optical coherence tomography (SS-OCT) for the diagnosis of occlusal caries, J. Dent., 38 (2010), 655–665. https://doi.org/10.1016/j.jdent.2010.05.004 doi: 10.1016/j.jdent.2010.05.004
![]() |
[17] |
Y. Shimada, H. Nakagawa, A. Sadr, I. Wada, M. Nakajima, T. Nikaido, et al., Noninvasive cross‐sectional imaging of proximal caries using swept‐source optical coherence tomography (SS‐OCT) in vivo, J. Biophotonics, 7 (2014), 506–513. https://doi.org/10.1002/jbio.201200210 doi: 10.1002/jbio.201200210
![]() |
[18] |
K. H. Chan, A. C. Chan, W. A. Fried, J. C. Simon, C. L. Darling, D. Fried, Use of 2D images of depth and integrated reflectivity to represent the severity of demineralization in cross‐polarization optical coherence tomography, J. Biophotonics, 8 (2015), 36–45. https://doi.org/10.1002/jbio.201300137 doi: 10.1002/jbio.201300137
![]() |
[19] |
H. Kang, C. L. Darling, D. Fried, Nondestructive monitoring of the repair of enamel artificial lesions by an acidic remineralization model using polarization-sensitive optical coherence tomography, Dent. Mater., 28 (2012), 488–494. https://doi.org/10.1016/j.dental.2011.11.020 doi: 10.1016/j.dental.2011.11.020
![]() |
[20] |
D. P. Popescu, M. G. Sowa, M. D. Hewko, L. P. Choo-Smith, Assessment of early demineralization in teeth using the signal attenuation in optical coherence tomography images, J. Biomed. Opt., 13 (2008), 054053. https://doi.org/10.1117/1.2992129 doi: 10.1117/1.2992129
![]() |
[21] |
C. Bandt, B. Pompe, Permutation entropy: a natural complexity measure for time series, Phys. Rev. Lett., 88 (2002), 174102. https://doi.org/10.1103/PhysRevLett.88.174102 doi: 10.1103/PhysRevLett.88.174102
![]() |
[22] |
B. Kosko, Fuzzy entropy and conditioning, Inf. Sci, 40 (1986), 165–174. https://doi.org/10.1016/0020-0255(86)90006-X doi: 10.1016/0020-0255(86)90006-X
![]() |
[23] |
M. Rostaghi, H. Azami, Dispersion entropy: A measure for time-series analysis, IEEE Signal Process Lett., 23 (2016), 610–614. https://doi.org/10.1109/LSP.2016.2542881 doi: 10.1109/LSP.2016.2542881
![]() |
[24] |
M. A. Li, H. N. Liu, W. Zhu, J. F. Yang, Applying improved multiscale fuzzy entropy for feature extraction of MI-EEG, Appl. Sci., 7 (2017), 92. https://doi.org/10.3390/app7010092 doi: 10.3390/app7010092
![]() |
[25] |
M. G. Li, R. T. Wang, D. Q. Xu, An improved composite multiscale fuzzy entropy for feature extraction of MI-EEG, Entropy, 22 (2020), 1356. https://doi.org/10.3390/e22121356 doi: 10.3390/e22121356
![]() |
[26] |
M. U. Ahmed, T. Chanwimalueang, S. Thayyil, D. P. Mandic, A multivariate multiscale fuzzy entropy algorithm with application to uterine EMG complexity analysis, Entropy, 19 (2016), 2. https://doi.org/10.3390/e19010002 doi: 10.3390/e19010002
![]() |
[27] |
T. B. Liu, W. P. Yao, M. Wu, Z. R, Shi, J. Wang, X. B. Ning, Multiscale permutation entropy analysis of electrocardiogram, Phys. A, 471 (2017), 492–498. https://doi.org/10.1016/j.physa.2016.11.102 doi: 10.1016/j.physa.2016.11.102
![]() |
[28] |
D. Li, X. Li, Z. Liang, L. J. Voss, J. W. Sleigh, Multiscale permutation entropy analysis of EEG recordings during sevoflurane anesthesia, J. Neural Eng., 7 (2010), 046010. https://doi.org/10.1088/1741-2560/7/4/046010 doi: 10.1088/1741-2560/7/4/046010
![]() |
[29] |
G. X. Ouyang, J. Li, X. Z. Liu, X. L. Li, Dynamic characteristics of absence EEG recordings with multiscale permutation entropy analysis, Epilepsy Res., 104 (2013), 246–252. https://doi.org/10.1016/j.eplepsyres.2012.11.003 doi: 10.1016/j.eplepsyres.2012.11.003
![]() |
[30] |
H. Azami, M. Rostaghi, D. Abásolo, J. Escudero, Refined composite multiscale dispersion entropy and its application to biomedical signals, IEEE Trans. Biomed. Eng., 64 (2017), 2872–2879. https://doi.org/10.1109/TBME.2017.2679136 doi: 10.1109/TBME.2017.2679136
![]() |
[31] |
B. Liu, R. M. Wang, Z. Q. Peng, L. J. Qin, Identification of denatured biological tissues based on compressed sensing and improved multiscale dispersion entropy during HIFU treatment, Entropy, 22 (2020), 944. https://doi.org/10.3390/e22090944 doi: 10.3390/e22090944
![]() |
[32] |
M. Chakraborty, D. Mitra, Automated detection of epileptic seizures using multiscale and refined composite multiscale dispersion entropy, Chaos Solitons Fract., 146 (2021), 110939. https://doi.org/10.1016/j.chaos.2021.110939 doi: 10.1016/j.chaos.2021.110939
![]() |
[33] |
X. L. Huang, X. M. Ma, F. Hu., Machine learning and intelligent communications, Mobile Network Appl., 23 (2018), 68–70. https://doi.org/10.1007/s11036-017-0962-2 doi: 10.1007/s11036-017-0962-2
![]() |
[34] |
M. Sangeetha, K. Kumar, A. A. Aljabr, Image processing techniques in periapical dental X-ray image detection and classification, Webology, 18 (2021), 42–53. https://doi.org/10.14704/WEB/V18SI02/WEB18011 doi: 10.14704/WEB/V18SI02/WEB18011
![]() |
[35] |
Justiawan, D. A. Wahjuningrum, R. P. Hadi, A. P. Nurhayati, K. Prayogo, R. Sigit, et al., Comparative analysis of color matching system for teeth recognition using color moment, Med. Devices: Evidence Res., 12 (2019), 497–504. https://doi.org/10.2147/MDER.S224280 doi: 10.2147/MDER.S224280
![]() |
[36] |
M. Hashem, A. A. Al-Kheraif, A. A. Wahba, Examining the longevity of dental restoration using Hebbian adversarial networks clustering with gradient boosting recurrent neural network, Measurement, 141 (2019), 313–323. https://doi.org/10.1016/j.measurement.2019.04.035 doi: 10.1016/j.measurement.2019.04.035
![]() |
[37] |
E. Kaya, H. G. Gunec, S. S. Gokyay, S. Kutal, S. Gulum, H. F. Ates, Proposing a CNN method for primary and permanent tooth detection and enumeration on pediatric dental radiographs, J. Clin. Pediatr. Dent., 46 (2022), 293–298. https://doi.org/10.22514/1053-4625-46.4.6 doi: 10.22514/1053-4625-46.4.6
![]() |
[38] |
D. F. Specht, Probabilistic neural networks, Neural Networks, 3 (1990), 109–118. https://doi.org/10.1016/0893-6080(90)90049-Q doi: 10.1016/0893-6080(90)90049-Q
![]() |
[39] |
B. Liu, X. Zhang, X. Zou, J. Cao, Z. Q. Peng, Biological tissue damage monitoring method based on IMWPE and PNN during HIFU treatment, Information, 12 (2021), 404. https://doi.org/10.3390/info12100404 doi: 10.3390/info12100404
![]() |
[40] |
T. Shiina, Y. Moritani, M. Ito, Y. Okamura, Long-optical-path scanning mechanism for optical coherence tomography, Appl. Opt., 42 (2003), 3795–3799. https://doi.org/10.1364/AO.42.003795 doi: 10.1364/AO.42.003795
![]() |
[41] |
K. Saeki, D. Huyan, M. Sawada, Y. Sun, A. Nakamura, M. Kimura, et al., Measurement algorithm for real front and back curved surfaces of contact lenses, Appl. Opt., 59 (2020), 9051–9059. https://doi.org/10.1364/AO.399190 doi: 10.1364/AO.399190
![]() |
[42] |
D. Huyan, N. Lagrosas, T. Shiina, Target imaging in scattering media using ghost imaging optical coherence tomography, APL Photonics, 7 (2022), 086104. https://doi.org/10.1063/5.0099638 doi: 10.1063/5.0099638
![]() |
[43] |
D. Fried, J. Xie, S. Shafi, J. D. Featherstone, T. M. Breunig, C. Q. Le, et al., Imaging caries lesions and lesion progression with polarization sensitive optical coherence tomography, J. Biomed. Opt., 7 (2002), 618–627. https://doi.org/10.1117/1.1509752 doi: 10.1117/1.1509752
![]() |
[44] |
M. T. Tsai, Y. L. Wang, T. W. Yeh, H. C. Lee, W. J. Chen, J. L. Ke, et al., Early detection of enamel demineralization by optical coherence tomography, Sci. Rep., 9 (2019), 17154. https://doi.org/10.1038/s41598-019-53567-7 doi: 10.1038/s41598-019-53567-7
![]() |
[45] |
Y. Li, J. Liu, C. Tang, W. Han, S. Y. Zhou, S. Q. Yang, et al., Multiscale entropy analysis of instantaneous frequency variation to overcome the cross-over artifact in rhythmic EEG, IEEE Access, 9 (2021), 12896–12905. https://doi.org/10.1109/ACCESS.2021.3051367 doi: 10.1109/ACCESS.2021.3051367
![]() |
Parameters | MPE-PNN | MFE-PNN | MDE-PNN |
Sensitivity (TP / (TP + FN)) | 0.7470 | 0.9682 | 0.9758 |
Specificity (TN / (TN + FP)) | 0.7562 | 0.8844 | 0.8478 |
Diagnostic Efficiency((TP+TN) / Total) | 0.7516 | 0.9263 | 0.9118 |
Embedded dimension | m = 2 | m = 3 | m = 4 |
MPE-PNN | 0.7516 | 0.5378 | 0.5306 |
MFE-PNN | 0.9263 | 0.8966 | 0.8384 |
MDE-PNN | 0.9118 | 0.9112 | 0.9090 |
Method | Feature Extraction (20 scales) | Diagnosis (Single feature) | Diagnosis (Multiple features) |
MPE-PNN | 1.79 s | 1.05 s | 1.12 s |
MFE-PNN | 15.19 s | 1.03 s | 1.08 s |
MDE-PNN | 1.49 s | 0.88 s | 1.06 s |
Parameters | Entropy features | Optical features (Attenuation rate) (μm-1) | ||
Normal | Demineralization | Normal | Demineralization | |
Average | 1.0438 | 0.4473 | 0.0240 | 0.0167 |
Standard deviation | 0.5651 | 0.1905 | 0.0088 | 0.0058 |
Upper quartile | 1.5369 | 0.4778 | 0.0306 | 0.0202 |
Lower quartile | 0.4943 | 0.3244 | 0.0169 | 0.0109 |
Parameters | Entropy feature analysis (Single feature) | Optical feature analysis (Single feature) |
Sensitivity | 0.9758 | 0.8250 |
Specificity | 0.8478 | 0.7084 |
Diagnostic efficiency | 0.9118 | 0.7667 |
Parameters | MPE-PNN | MFE-PNN | MDE-PNN |
Sensitivity (TP / (TP + FN)) | 0.7470 | 0.9682 | 0.9758 |
Specificity (TN / (TN + FP)) | 0.7562 | 0.8844 | 0.8478 |
Diagnostic Efficiency((TP+TN) / Total) | 0.7516 | 0.9263 | 0.9118 |
Embedded dimension | m = 2 | m = 3 | m = 4 |
MPE-PNN | 0.7516 | 0.5378 | 0.5306 |
MFE-PNN | 0.9263 | 0.8966 | 0.8384 |
MDE-PNN | 0.9118 | 0.9112 | 0.9090 |
Method | Feature Extraction (20 scales) | Diagnosis (Single feature) | Diagnosis (Multiple features) |
MPE-PNN | 1.79 s | 1.05 s | 1.12 s |
MFE-PNN | 15.19 s | 1.03 s | 1.08 s |
MDE-PNN | 1.49 s | 0.88 s | 1.06 s |
Parameters | Entropy features | Optical features (Attenuation rate) (μm-1) | ||
Normal | Demineralization | Normal | Demineralization | |
Average | 1.0438 | 0.4473 | 0.0240 | 0.0167 |
Standard deviation | 0.5651 | 0.1905 | 0.0088 | 0.0058 |
Upper quartile | 1.5369 | 0.4778 | 0.0306 | 0.0202 |
Lower quartile | 0.4943 | 0.3244 | 0.0169 | 0.0109 |
Parameters | Entropy feature analysis (Single feature) | Optical feature analysis (Single feature) |
Sensitivity | 0.9758 | 0.8250 |
Specificity | 0.8478 | 0.7084 |
Diagnostic efficiency | 0.9118 | 0.7667 |