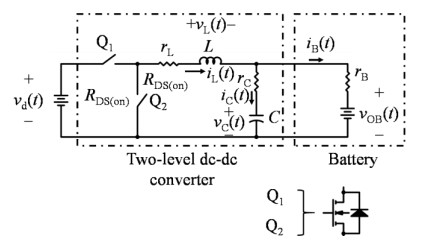
Objective: Autism spectrum disorder (ASD) is usually characterised by altered social skills, repetitive behaviours, and difficulties in verbal/nonverbal communication. It has been reported that electroencephalograms (EEGs) in ASD are characterised by atypical complexity. The most commonly applied method in studies of ASD EEG complexity is multiscale entropy (MSE), where the sample entropy is evaluated across several scales. However, the accuracy of MSE-based classifications between ASD and neurotypical EEG activities is poor owing to several shortcomings in scale extraction and length, the overlap between amplitude and frequency information, and sensitivity to frequency. The present study proposes a novel, nonlinear, non-stationary, adaptive, data-driven, and accurate method for the classification of ASD and neurotypical groups based on EEG complexity and entropy without the shortcomings of MSE. Approach: The proposed method is as follows: (a) each ASD and neurotypical EEG (122 subjects × 64 channels) is decomposed using empirical mode decomposition (EMD) to obtain the intrinsic components (intrinsic mode functions). (b) The extracted components are normalised through the direct quadrature procedure. (c) The Hilbert transforms of the components are computed. (d) The analytic counterparts of components (and normalised components) are found. (e) The instantaneous frequency function of each analytic normalised component is calculated. (f) The instantaneous amplitude function of each analytic component is calculated. (g) The Shannon entropy values of the instantaneous frequency and amplitude vectors are computed. (h) The entropy values are classified using a neural network (NN). (i) The achieved accuracy is compared to that obtained with MSE-based classification. (j) The consistency of the results of entropy 3D mapping with clinical data is assessed. Main results: The results demonstrate that the proposed method outperforms MSE (accuracy: 66.4%), with an accuracy of 93.5%. Moreover, the entropy 3D mapping results are more consistent with the available clinical data regarding brain topography in ASD. Significance: This study presents a more robust alternative to MSE, which can be used for accurate classification of ASD/neurotypical as well as for the examination of EEG entropy across brain zones in ASD.
Citation: Enas Abdulhay, Maha Alafeef, Hikmat Hadoush, V. Venkataraman, N. Arunkumar. EMD-based analysis of complexity with dissociated EEG amplitude and frequency information: a data-driven robust tool -for Autism diagnosis- compared to multi-scale entropy approach[J]. Mathematical Biosciences and Engineering, 2022, 19(5): 5031-5054. doi: 10.3934/mbe.2022235
[1] | Rasool M. Imran, Kadhim Hamzah Chalok, Siraj A. M. Nasrallah . Innovative two-stage thermal control of DC-DC converter for hybrid PV-battery system. AIMS Electronics and Electrical Engineering, 2025, 9(1): 26-45. doi: 10.3934/electreng.2025002 |
[2] | Mohamed M. Albarghot, Mohamed T. Iqbal, Kevin Pope, Luc Rolland . Dynamic modeling and simulation of the MUN Explorer autonomous underwater vehicle with a fuel cell system. AIMS Electronics and Electrical Engineering, 2020, 4(1): 114-131. doi: 10.3934/ElectrEng.2020.1.114 |
[3] | Suliana Ab-Ghani, Hamdan Daniyal, Abu Zaharin Ahmad, Norazila Jaalam, Norhafidzah Mohd Saad, Nur Huda Ramlan, Norhazilina Bahari . Adaptive online auto-tuning using Particle Swarm optimized PI controller with time-variant approach for high accuracy and speed in Dual Active Bridge converter. AIMS Electronics and Electrical Engineering, 2023, 7(2): 156-170. doi: 10.3934/electreng.2023009 |
[4] | Fadhil A. Hasan, Lina J. Rashad . Combining fractional-order PI controller with field-oriented control based on maximum torque per ampere technique considering iron loss of induction motor. AIMS Electronics and Electrical Engineering, 2024, 8(3): 380-403. doi: 10.3934/electreng.2024018 |
[5] | Saqib J Rind, Saba Javed, Yawar Rehman, Mohsin Jamil . Sliding mode control rotor flux MRAS based speed sensorless induction motor traction drive control for electric vehicles. AIMS Electronics and Electrical Engineering, 2023, 7(4): 354-379. doi: 10.3934/electreng.2023019 |
[6] | Dipak R. Swain, Sunita S. Biswal, Pravat Kumar Rout, P. K. Ray, R. K. Jena . Optimal fractional sliding mode control for the frequency stability of a hybrid industrial microgrid. AIMS Electronics and Electrical Engineering, 2023, 7(1): 14-37. doi: 10.3934/electreng.2023002 |
[7] | Anjan Ku. Sahoo, Ranjan Ku. Jena . Improved DTC strategy with fuzzy logic controller for induction motor driven electric vehicle. AIMS Electronics and Electrical Engineering, 2022, 6(3): 296-316. doi: 10.3934/electreng.2022018 |
[8] | Tarun Naruka, Debasis Tripathy, Prangya Mohanty . Power quality enhancement by a solar photovoltaic-based distribution static compensator. AIMS Electronics and Electrical Engineering, 2025, 9(2): 192-214. doi: 10.3934/electreng.2025010 |
[9] | Shimin V V, Varsha A Shah, Makarand M Lokhande . An advanced semiconductor material selection for switching devices in electric vehicles using three multiple attribute decision making methods. AIMS Electronics and Electrical Engineering, 2020, 4(4): 369-388. doi: 10.3934/ElectrEng.2020.4.369 |
[10] | I.E.S. Naidu, S. Srikanth, A. Siva sarapakara Rao, Adabala Venkatanarayana . A novel mine blast optimization algorithm (MBOA) based MPPT controlling for grid-PV systems. AIMS Electronics and Electrical Engineering, 2023, 7(2): 135-155. doi: 10.3934/electreng.2023008 |
Objective: Autism spectrum disorder (ASD) is usually characterised by altered social skills, repetitive behaviours, and difficulties in verbal/nonverbal communication. It has been reported that electroencephalograms (EEGs) in ASD are characterised by atypical complexity. The most commonly applied method in studies of ASD EEG complexity is multiscale entropy (MSE), where the sample entropy is evaluated across several scales. However, the accuracy of MSE-based classifications between ASD and neurotypical EEG activities is poor owing to several shortcomings in scale extraction and length, the overlap between amplitude and frequency information, and sensitivity to frequency. The present study proposes a novel, nonlinear, non-stationary, adaptive, data-driven, and accurate method for the classification of ASD and neurotypical groups based on EEG complexity and entropy without the shortcomings of MSE. Approach: The proposed method is as follows: (a) each ASD and neurotypical EEG (122 subjects × 64 channels) is decomposed using empirical mode decomposition (EMD) to obtain the intrinsic components (intrinsic mode functions). (b) The extracted components are normalised through the direct quadrature procedure. (c) The Hilbert transforms of the components are computed. (d) The analytic counterparts of components (and normalised components) are found. (e) The instantaneous frequency function of each analytic normalised component is calculated. (f) The instantaneous amplitude function of each analytic component is calculated. (g) The Shannon entropy values of the instantaneous frequency and amplitude vectors are computed. (h) The entropy values are classified using a neural network (NN). (i) The achieved accuracy is compared to that obtained with MSE-based classification. (j) The consistency of the results of entropy 3D mapping with clinical data is assessed. Main results: The results demonstrate that the proposed method outperforms MSE (accuracy: 66.4%), with an accuracy of 93.5%. Moreover, the entropy 3D mapping results are more consistent with the available clinical data regarding brain topography in ASD. Significance: This study presents a more robust alternative to MSE, which can be used for accurate classification of ASD/neurotypical as well as for the examination of EEG entropy across brain zones in ASD.
The accelerating adoption of electric vehicles (EVs) is largely motivated by the global urgency to mitigate climate change, with fossil fuel–based transportation being a major contributor to greenhouse gas emissions [1,2]. Governments and the private sector are working together to advance EV technologies, aiming to make EVs as viable and accessible as conventional vehicles. A critical factor influencing EV efficiency and performance is the battery system, which governs key metrics like driving range, reliability, and lifespan. The battery is managed by a battery management system (BMS), which ensures safe operation by monitoring thermal and electrical parameters, balancing cell charge, and coordinating other auxiliary functions [3].
Apart from batteries, the efficiency of EVs relies heavily on power converters, specifically the traction inverter and the battery charger (BC). While the inverter controls power flow between the battery and motor, the BC regulates energy transfer from the grid (or alternative sources) to the battery, impacting attributes such as charging speed, range, and battery longevity [4]. BCs are classified based on factors like charging type (conductive vs. inductive), installation location (on-board vs. off-board), and power flow capability (unidirectional vs. bidirectional). Established standards such as IEC 61851-1 and SAE J1772 further distinguish BC types based on charging modes, levels, and safety protocols [1].
DC fast chargers and their associated power converter topologies, such as single-phase and three-phase isolated and non-isolated converters, are widely implemented in EV charging infrastructure. For instance, on-board battery chargers (OBCs) commonly integrate an EMI filter, an AC-DC converter with power factor correction (PFC), and a DC-DC converter. Variants of these topologies allow bidirectional power flow to support vehicle-to-grid interactions, enhancing grid flexibility [2]. However, while existing works cover a range of converter configurations and performance optimization methods [2,5,6], most omit the impact of non-idealities inherent in switching devices and energy storage components. These non-idealities, associated with parameters like switch resistance and inductor characteristics, significantly affect converter efficiency, dynamic response, and control accuracy.
In this study, we investigate a two-level DC-DC battery charger (2L-BC) with a focus on modeling and compensating for these non-idealities. Prior literature on DC-DC converters includes insightful contributions regarding fast-charging infrastructure and converter configurations [7,8,9,10]. Yet, few works address the non-ideal behavior of converters in EV battery charging contexts. For instance, research on general DC-DC converters has examined the impact of non-idealities in switch and inductor behavior, although without direct application to EV charging systems [11,12,13].
This article presents a comprehensive study of a 2L-BC model that includes the effects of non-idealities in switches and energy storage components, which is an area not commonly addressed in depth within the literature. The analysis focuses on how these non-ideal parameters influence essential operating points and performance metrics under both steady-state and dynamic conditions, offering critical insights for enhancing EV charger design and efficiency.
A key part of this study examines the equilibrium voltage VC and its sensitivity to series resistances rL and RDS(on), revealing that as these parasitic resistances increase, VC decreases significantly. This finding highlights the impact of non-idealities on output stability and efficiency. The analysis further investigates the inductance L and capacitance C under varying non-ideal conditions, showing that L decreases as both rL and RDS(on) decrease, establishing a direct relationship that influences energy transfer and response time in the converter. In contrast, the capacitance C remains nearly constant despite variations in rL and rC, confirming its stabilizing role in smoothing voltage ripples in the system.
Additionally, this study highlights the effects of non-idealities on charger efficiency (η). The analysis shows that efficiency peaks under ideal conditions, where parasitic resistances are minimized, while increased parasitic resistances cause a notable drop in performance. These findings emphasize the importance of accounting for non-ideal effects in charger design, providing essential considerations for optimizing both steady-state and transient behaviors in EV charging systems.
The paper is organized as follows: Sections 2 and 3 present the topology and mathematical model of the studied charger. Sections 4 and 5 explore the charger's efficiency and sensitivity to non-idealities incorporated in the model. Sections 6 and 7 focus on the dimensioning of energy storage components, such as the inductor and capacitor, and the synthesis of the linear output-feedback compensator. Finally, Sections 8 and 9 present the simulation results and conclusions of the study.
The proposed battery charger topology (2L-BC) is illustrated in Figure 1. It is based on a two-level DC-DC converter, with the load modeled as a standard battery [14]. The 2L-BC topology consists of two switches, labeled Q1 and Q2, which operate complementarily [15]. These switches are implemented using MOSFET devices, each incorporating its associated RDS(on) resistance and driving resistors.
In addition to the switches, the 2L-BC model includes the series resistances of the inductor (rL) and capacitor (rC), which reflect the non-idealities in these components. The battery is represented by its internal voltage, vOB(t), and its internal resistance, rB. The system variables include the input voltage, vd(t), the inductor voltage, vL(t), and the capacitor voltage, vC(t). The currents flowing through the inductor, capacitor, and battery are denoted as iL(t), iC(t), and iB(t), respectively.
It is assumed that the 2L-BC operates in continuous conduction mode, ensuring a stable operating regime for the converter under all conditions.
This section presents the modeling of the 2L-BC charger in both steady-state and dynamic regimes.
As a preliminary step in the modeling process, it is essential to identify the switching strategy employed by the converter switches (Q1 and Q2). This enables the determination of the switching function of the system in a steady state, which is represented by the variable sf(t). The switching frequency of the 2L-BC is labeled as fs, and the switching period is defined as Ts = 1/fs. It is known that both switches operate in a complementary manner, and thus, the following strategy is derived and defined as follows: in the first half-cycle of the switching period, i.e., when 0 ≤ t < D∙Ts, switches Q1 and Q2 operate as closed and open, respectively. Subsequently, for the second half-cycle, that is, when D∙Ts ≤ t < Ts, Q1 and Q2 operate as open and closed, respectively. With this information, it is possible to define sf(t) as follows [17]:
sf(t)={1,0≤t<D⋅Ts0,D⋅Ts≤t<Ts | (1) |
It should be noted that D is the duty cycle under stationary conditions.
The governing equations for the voltage of L [vL(t)] and the current of C [iC(t)] are derived by applying Kirchhoff's laws of voltage and current. These equations are functions of the variation of the topology as the switching half-cycles occur. The topology in the first switching half-cycle is shown in Figure 2(a), and the expressions for vL(t) and iC(t) are given by:
vL(t)=vd(t)−(rB||rCrC)⋅vd(t)−(rB||rCrB)⋅vOB(t)−(Rin+rB||rC)⋅iL(t) | (2) |
iC(t)=(rB||rCrC)⋅iL(t)−(rB||rCrB⋅rC)⋅vC(t)+(rB||rCrB⋅rC)⋅vOB(t) | (3) |
where Rin = RDS(on) + rL. In contrast, the small ripple approximation (SRA) principle is applied to (2) and (3), assuming that the ripple of the voltages vd(t), vC(t), and vOB(t) and of the current iL(t) is sufficiently small to be neglected, a common assumption in practice [15,16]. The revised versions of (2) and (3) following the application of the SRA are presented in (4) and (5).
vL(t)≈Vd−(rB||rCrC)⋅VC−(rB||rCrB)⋅VOB−(Rin+rB||rC)⋅IL | (4) |
iC(t)≈(rB||rCrC)⋅IL−(rB||rCrB⋅rC)⋅VC+(rB||rCrB⋅rC)⋅VOB | (5) |
The topology of the system when operating in the second half-cycle is depicted in Figure 2(b), and the expressions of vL(t) and iC(t) are defined as follows:
vL(t)=−(rB||rCrC)⋅vC(t)−(rB||rCrB)⋅vOB(t)−(Rin+rB||rC)⋅iL(t) | (6) |
iC(t)=(rB||rCrC)⋅iL(t)−(rB||rCrB⋅rC)⋅vC(t)+(rB||rCrB⋅rC)⋅vOB(t) | (7) |
Then, applying the SRA to (6) and (7), the new equations related to this switching half-cycle are given by:
vL(t)≈−(rB||rCrC)⋅VC−(rB||rCrB)⋅VOB−(Rin+rB||rC)⋅IL | (8) |
iC(t)≈(rB||rCrC)⋅IL−(rB||rCrB⋅rC)⋅VC+(rB||rCrB⋅rC)⋅VOB | (9) |
In light of the fact that the 2L-BC operates in a state of steady-state, the principles of inductor volt-second balance and capacitor charge balance are applied to the system. With regard to the former, it is demonstrated that the average voltage of L over one switching period (Ts), i.e., < vL(t) > Ts, must be zero [15]. Consequently, the inductor volt-second balance is calculated from (4) and (8) and stated as follows:
D⋅Vd−(rB||rCrC)⋅VC−(rB||rCrB)⋅VOB−(Rin+rB||rC)⋅IL=0 | (10) |
Conversely, the capacitor charge balance principle is applied according to (5) and (9), resulting in the verification that equation < iC(t) > Ts = 0, as given by [15]:
rB⋅IL−VC+VOB=0 | (11) |
It should be noted that (10) and (11) represent the model of the charger in equilibrium (steady state). For this system, the unknown variables are VC and IL, while the known variables are D and VOB. Solving for VC and IL yields the steady state variables given by:
{IL=rB||RinrB⋅Rin(D⋅Vd−rB||rCrBVOB)VC=rB||RinRin⋅(D⋅Vd−(rB||rCrB−RinrB||Rin)⋅VOB) | (12) |
From (11), it can be observed that the VC dynamics under steady-state conditions exhibit a slight dependence on the charger non-idealities, particularly with regard to RDS(on) and rL. This is due to the fact that, in practice, rB is significantly larger than Rin, resulting in rB || Rin ≈ rB. Therefore, the equilibrium state of the system may change if such resistances are not considered. The model of the charger in steady-state is illustrated in Figure 3.
Figure 2 and (1)−(3), (8), and (9) provide the 2L-BC switching model defined by
ddt[iL(t)vC(t)]=[−Rin+rB||rCL−rB||rCrC⋅LrB||rCrC⋅CrB||rCrB⋅rC⋅C]⋅[iL(t)vC(t)]+[1L⋅sf(t)−rB||rCrB⋅L0rB||rCrB⋅rC⋅C]⋅[vd(t)vOB(t)]iB(t)=[1−rB||rCrC⋅C1rB]⋅[iL(t)vC(t)]−1rB⋅vOB(t) | (13) |
Subsequently, the averaging operator ⟨x(t)⟩Ts=1Ts⋅∫tt−Tsx(τ)⋅dτ is applied to (13) over a Ts, resulting in the averaged model of the 2L-BC, defined as follows [15,17]:
ddt[⟨iL(t)⟩Ts⟨vC(t)⟩Ts]=[−Rin+rB||rCL−rB||rCrC⋅LrB||rCrC⋅CrB||rCrB⋅rC⋅C]⋅[⟨iL(t)⟩Ts⟨vC(t)⟩Ts]+[1L⋅d(t)−rB||rCrB⋅L0rB||rCrB⋅rC⋅C]⋅[⟨vd(t)⟩Ts⟨vOB(t)⟩Ts]⟨iB(t)⟩Ts=[1−rB||rCrC⋅C1rB]⋅[⟨iL(t)⟩Ts⟨vC(t)⟩Ts]−1rB⋅⟨vOB(t)⟩Ts | (14) |
Finally, the model in (14) is linearized using the Taylor series and the perturbation of the variables around the points of the charger [11,18] in steady-state. The equilibrium model of the charger, as represented in (12) [15,17], is used to calculate the steady-state values. It should be noted that in the switched model of the charger described in (13), the switching function is replaced by its averaged version, with the addition of a small disturbance in AC, i.e., d(t). The linearization of the charger results in the derivation of the small-signal state-space model, shown as follows:
{ddtx(t)=Am⋅x(t)+Bm⋅u(t)y(t)=Cm⋅x(t)+Dm⋅u(t) | (15) |
It should be noted that the variables with hats are the small-signal variables, which are assumed to be much smaller than their steady-state variables [15]. The state vector, defined in (15) as x(t)=[ˆiL(t),ˆvC(t)]T, groups the state variables. The input vector, defined as u(t)=[ˆvd(t),ˆvOB(t),ˆd(t)]T, groups the input variables. Finally, the output vector, defined as y(t) = [x(t), ˆiB(t)]T, groups the input variables. In this charger, the outputs have been defined as the state variables plus the load current. Furthermore, x(t) ∈ {R2} and {u(t), y(t)} ∈ {R3}. Conversely, the matrices of the model in (15) are defined as follows:
Am=[−Rin+rB||rCL−rB||rCrC⋅LrB||rCrC⋅CrB||rCrB⋅rC⋅C],Bm=[DL−rB||rCrB⋅LVdL0rB||rCrB⋅rC⋅C0],Cm=[1001rB||rCrBrB||rCrB⋅rC],Dm=[0000000−rB||rCrB⋅rC⋅C0] | (16) |
From here, Am, Bm, Cm, and Dm are the state, input, output, and direct transmission matrices, respectively. Also, Am M2x2 ∈ {Κ}, Bm M2x3 ∈ {Κ}, Cm, M3x2 ∈ {Κ}, and Dm M3x3 ∈ {Κ}.
An interesting figure of merit to characterize the charger is the expression of the performance parameter (η) [15,19,20,21]. It is known that η = Po/Pi, where Pi and Po are the input and output power of the charger, respectively. According to Figure 3, Pi and Po are given by
{Pi=D⋅(Av1rB||Rin−DRin)⋅(Av2−1)⋅V2dPo=rB⋅(Av1rB||Rin−DRin)2⋅V2d | (17) |
From (17), Av1 and Av2 are defined as steady-state voltage gains, specifically Av1 = VC/Vd and Av2 = VC/VOB. Then, the expression of η is calculated and shown as follows:
η=rB⋅(Av1rB||Rin−DRin)⋅(1(Av2−1)⋅D) | (18) |
It is interesting to note that this expression is of the nonlinear type, mainly due to the presence of Av1 and Av2. Furthermore, it can be seen that the efficiency is strongly dependent on the non-idealities RDS(on) and rL, and it can be confirmed from (18) that this dependence is of the hyperbolic type.
Some observations can be drawn from the latter:
● The efficiency is highly sensitive to the non-idealities of the charger, such as the resistance RDS(on) and the inductor resistance rL.
● The dependence on these resistances follows a hyperbolic type, emphasizing that as the losses increase (due to higher RDS(on) or rL), the efficiency decreases.
This section highlights the importance of these non-idealities in the performance of the charger and how they affect its overall efficiency. The equation provides a framework to optimize the design by minimizing these losses to maximize efficiency.
The goal of the sensitivity analysis is to evaluate how variations in the non-ideal resistive parameters—specifically rL, rC, and RDS(on)—affect the efficiency η of the 2L-BC. By computing partial derivatives of the efficiency equation with respect to each of these parameters [22], insights are obtained into the individual and relative impacts of each non-ideality on system efficiency.
The analysis of sensitivity with respect to the inductor resistance rL reveals its significant influence on the overall efficiency η of the 2L-BC. As rL directly contributes to the input resistance Rin (where Rin = RDS(on) + rL), an increase in rL raises Rin, thereby affecting the voltage gain terms Av1 and Av2, both of which depend on Rin. The partial derivative of efficiency with respect to rL is given by:
∂η∂rL=∂η∂Rin⋅∂Rin∂rL⇒∂η∂rL=−(Av1−D(Av2−1)⋅D)⋅rBRin2 | (19) |
Equation (19) indicates that the sensitivity of η to rL is inversely proportional to Rin2. This hyperbolic relationship implies that an increase in rL leads to a decrease in efficiency, highlighting rL as a critical parameter. Reducing rL by selecting low-resistance inductors or optimizing inductor design can mitigate resistive losses and enhance charger efficiency. Thus, managing inductor resistance is essential for optimizing overall system performance, particularly in high-efficiency applications
The capacitor resistance rC influences the voltage gain Av2, defined as the ratio of the capacitor voltage VC to the output voltage VOB. Changes in rC modify Av2, which in turn affects efficiency. The partial derivative of η with respect to rC is expressed as:
∂η∂rC=∂η∂Av2⋅∂Av2∂rC⇒∂η∂rC=(1D⋅(Av2−1)2)⋅(rB||RinRin)⋅(rB||rCrC)2⋅(Av1rB||Rin−DRin) | (20) |
Expression (20) shows that the sensitivity of η to rC is influenced by rC's interactions with rB and Rin. This complex relationship implies that increasing rC can significantly affect efficiency due to its quadratic effect on the gain term, reducing η more strongly as rC rises. Consequently, minimizing rC through capacitor selection or design adjustments can enhance efficiency, especially in systems where resistive losses are critical. This emphasizes the importance of keeping rC low for optimal performance in high-efficiency charging applications.
The MOSFET on-resistance RDS(on) also contributes to Rin, thus influencing both voltage gains Av1 and Av2, which impact efficiency. The sensitivity of η to RDS(on) is derived by differentiating the efficiency equation with respect to RDS(on):
∂η∂RDS(on)=∂η∂Rin⋅∂Rin∂RDS(on)⇒∂η∂RDS(on)=−(Av1−D(Av2−1)⋅D)⋅rBRin2 | (21) |
Expression (21) reveals that RDS(on) and rL produce identical sensitivity effects on η due to their combined role in Rin. The analysis indicates that increases in either RDS(on) or rL lead to efficiency reductions, as both raise Rin, which in turn lowers Av1 and Av2, thus decreasing η. This result underscores the importance of minimizing both RDS(on) and rL to improve efficiency, especially in high-performance charger designs where resistive losses need to be minimized.
In order to calculate the energy storage elements, L and C, the slopes (m) of iL(t) and vC(t) during the first switching half-cycle, i.e., in the time interval 0 ≤ t < D∙Ts, are considered. These slopes are obtained from (4) and (5), respectively. Additionally, the slopes of iL(t) and vC(t) are depicted in Figure 4(a) and (b), respectively. Based on Figure 4 and the aforementioned equations, the values of L and C are calculated and presented as follows:
L≥12⋅D⋅TsΔIL⋅[Vd−(rB+RinrB)⋅VC+RinrB⋅VOB] | (22) |
C≥12⋅D⋅Ts⋅rB||rCrC⋅ΔVC⋅[IL−1rB⋅VC+1rB⋅VOB] | (23) |
From (22) and (23), the maximum allowable ripple percentages with respect to VC and IL, i.e., ΔVC and ΔIL respectively, can be calculated. It can also be seen that both ΔVC and ΔIL represent the constraints imposed on 2L-BC, which must be met. It is once again evident that the non-idealities of the charger affect the sizing of the energy storage elements, specifically at L and C. Nevertheless, as indicated by (22) and (23), the sizing of L is more significantly influenced by the variation of RDS(on) and rL than by the sizing of C.
The linear model in (15) is considered, and the Laplace transform (s) is applied to obtain the s-domain model shown as follows:
Y(s)=(Cm⋅(s⋅I−Am)−1⋅Bm+Dm)⋅U(s) | (24) |
In (24), I is an identity matrix of dimension 2×2. From (24), it follows that Y(s) = [IL(s), VC(s), IB(s)]T and U(s) = [Vd(s), VOB(s), D(s)]T are the complex output and input vectors, respectively. These vectors are defined as {U(s), Y(s)} ∈ .
Figure 5 presents the proposed control diagram for the 2L-BC, structured with a standard output feedback configuration [18]. The primary control goal is to regulate iB(t) using a PI compensator, chosen for its simplicity and well-established performance in power electronics applications. The PI compensator, extensively studied in the literature, is widely recognized for its effectiveness in reducing steady-state errors and ensuring stable transient responses, making it highly suitable for systems like the 2L-BC.
In this configuration, the PI controller outputs the duty cycle d(t), which is then fed into the charger modulator. The modulator produces the switching function sf(t), which manages the states of switches Q1 and Q2 according to (1). This approach leverages the robustness of PI control to handle non-idealities in the 2L-BC, thereby enhancing system stability and performance across variable load conditions.
The transfer function of interest for this control system is obtained from (24), which corresponds to:
IB(s)D(s)|Vd=0VOB=0=k⋅(1+sωz)(1+sωp1)⋅(1+sωp2) | (25) |
The steady-state gain (k), the zero (ωz), and the poles (ωp1 and ωp2) are defined as follows:
k=VdL⋅C⋅(rB+rC)⋅ωp1⋅ωp2,ωp1=−0.5⋅Reff1+√(0.5⋅Reff1)2−Reff2,ωp1=−0.5⋅Reff1−√(0.5⋅Reff1)2−Reff2,Reff1=rB||rCrB⋅rC⋅C+IL⋅(Rin+rB||rC)Reff2=(rB||rC)2rC⋅C⋅(1L⋅rC+ILrB)+IL⋅Rin⋅rB||rCC⋅rC⋅rB | (26) |
The controller described in Figure 5 is designed using the phase-margin test (PMT) [15]. As illustrated in Figure 5, and in accordance with the extensive literature on PI compensators, this compensator can be modeled as follows [15]:
Gc(s)=kp⋅(1+1/τi/s) | (27) |
where kp and τi are the proportional and integral constants, respectively. The block diagram associated with this loop is illustrated in Figure 6. On the other hand, the loop gain of the system is defined by:
Ts(s)=Gs(s)⋅k⋅(1+sωz)(1+sωp1)⋅(1+sωp2) | (28) |
On the other hand, the modulator is based on pulse-width modulation (PWM), and its design and operation are described in Figure 7 [15,16]. Its Laplace model is given by M(s) = 1/VM, where, in this case, VM = 1 V. The high-frequency carry function is represented by the following model:
sf(t)={1,d(t)≥vcarry(t)0,d(t)<vcarry(t) | (29) |
According to the PMT, it is necessary to study the frequency behavior of the loop gain where the compensator Gc(s) is not involved, assuming that Gc(s) ≈ 1. This loop gain [Tu(s)] is given by:
Tu(s)=k⋅(1+sωz)(1+sωp1)⋅(1+sωp2) | (30) |
Table 1 provides a list of the parameters associated with the charger under study [23]. Table 1 indicates that the frequency response of (30) can be obtained and illustrated in Figure 8. With regard to the PMT, the crossover frequency (fc) of the frequency response of Tu(s) can be considered to be equal to the switching frequency of the charger, that is, fc = fs = 27 kHz. Consequently, Figure 8 reveals that fc ≈ 13.2 kHz. Consequently, a compensator is necessary to bring the fc of the uncompensated system as close as possible to fs. As part of the procedure, the compensated loop gain of the system has been defined in (25). However, by making the change of variable s = j∙ω, assuming ω > > 1, defining that ωc = ωs, and considering (24):
T(j⋅ω)=−j⋅k⋅kpωc | (31) |
valid for high frequencies. The PMT can be applied to yield the following [15]:
abs(T(j⋅ω)|@highfrequencyω=ωc)=1⇒k⋅kpωc=1⇒kp=ωsk|ωs=ωc | (32) |
and, to ensure good stability, τi = 100/ωs is defined [15]. Consequently, the PI compensator has already been designed. In order to verify that the PI has been properly designed, (28) must be evaluated. Figure 9 illustrates the frequency response of the compensated loop gain.
Parameter | Value | Parameter | Value |
RDS(on) | 35 [mΩ] | C | 100 [ηF] |
rL | 1 [Ω] | vOB | 450 [V] |
rC | 1.5 [Ω] | D | 0.9 |
rC | 1 [Ω] | ΔVC | 5 [%] |
L | 9.5 [mH] | ΔIL | 5 [%] |
From Figure 9, it can be established that the fc (≈ 29 kHz) is close to fs, which indicates that the design of the PI compensator is adequate.
The simulation of the switched and averaged 2L-BC models defined in (13) and (14), respectively, is performed using MATLAB Simulink and Table 1. The control system proposed in Figure 5 is also implemented. The 2L-BC is supplied with a dc voltage Vd = 800 V and operates with an fs of 27 kHz.
The initial conditions of the 2L-BC are imposed on the battery and inductor currents, as well as on the capacitor voltage. This is indicated by the following values: iB0 = iL0 = 0 A and vC0 = 400 V. In contrast, the initial reference value of the load current is I*B = 30 A.
Figure 10 depicts the simulation results for the transient dynamic responses of iB(t), iL(t), vC(t), d(t), and vOB(t) in their switched version, i.e., iBsm(t), iLsm(t), vCsm(t), dsm(t), vOBsm(t), and in their averaged version, i.e., iBav(t), iLav(t), vCav(t), dav(t), and vOBav(t), respectively. Regarding the 2L-BC operation, at 60 ms, there is a step change in iB(t), reaching a new value of 40 A and subsequently entering a steady state. Additionally, at 90 ms, a change in the load is generated, resulting in a shift in the internal battery voltage, vOB(t), from 450 to 350 V. This disturbance in the system dynamics is observed at 90 ms.
Figure 10(a) illustrates that iB(t) behaves as if it were a first-order system, exhibiting no overshoot and zero steady-state errors. The settling times are notably brief, with iB(t) reaching its steady state after startup in just 30 ms. Following the step change in iB*(t), iB(t) reaches its final steady-state value in 4 ms. Furthermore, upon the occurrence of a change in load, a transient is generated in iB(t) of a very short duration (resembling a pulse, as described by [18]), with a duration of approximately 1.5 µs, after which it returns to its steady state value of 40 A. This situation serves to illustrate the optimal design of the PI compensator, while also demonstrating the adequate stability of the system.
Conversely, it can be demonstrated that the sizing of L and C is adequate and meets the ripple requirement. That is, the ripple of iL(t) and vC(t) is lower than ΔIL and ΔVC, respectively.
As illustrated in Figure 10(b) and (c), it can be observed that the values of ΔIL and ΔVC reach 0.16% and 2.4%, respectively.
In addition, the dynamics of iL(t) and vC(t) are observed to work correctly with a step change in iB*(t) and a change in load. After starting, vC(t) takes the value of 480 V and iL(t) takes the value of 30 A.
Then, for the step change in iB(t), a logical increase in both variables [in iL(t) and vC(t), respectively] is generated. Finally, when the disturbance is generated in the load, iL(t) takes its maximum value of 45.43 A and, after 13 ms, it returns to its value of 40 A. On the other hand, vC(t) suffers a significant decrease, taking a transient value of 395.4 V, and after 10 ms, it reaches its final steady state value of 390 V. Such a condition generated in both variables after the load disturbance can be verified by analyzing expressions (2), (3), (6), and (7).
In Figure 10(d), it is observed that after system startup, the duty ratio d(t) takes the value of 0.64. After the step change in iB(t), d(t) takes a new steady state value of 0.66 and finally, when the load change occurs, d(t) undergoes an impulse type of disturbance and reaches its minimum value of 0.01 for a time of 1.5 μs [similar to iB(t)]. It also assumes a new steady state value of 0.54. On the other hand, a stable and unsaturated behavior is observed at d(t), which implies a greater slack in the control of iB(t). In addition, a small overshoot in d(t) is observed in response to the step change in iB(t). It is also observed that the switching frequency fs is transferred to d(t) due to the operation of the switches according to the switching strategy described in (1).
Figure 11 presents a series of surface plots analyzing the effects of variations in non-ideal parameters on the equilibrium point VC and the values of inductance L and capacitance C. Specifically, Figure 11(a) shows the equilibrium voltage VC as a function of inductor resistance rL and MOSFET on-resistance RDS(on); Figure 11(b) illustrates the variation of L as a function of rL and RDS(on); and Figure 11(c) depicts the variation of C as a function of rL and capacitor resistance rC. The constant parameters and respective variables are listed in Table 2.
Parameter | VC = f(RDS(on), rL) | L = f(RDS(on), rL) | C = f(rC, rL) |
Vd | 800 [V] | 800 [V] | 800 [V] |
rC | 1.5 [Ω] | 1.5 [Ω] | 10−6: 1: 103 [Ω] |
rB | 1 [Ω] | 1 [Ω] | 1 [Ω] |
VOB | 450 [V] | 450 [V] | 450 [V] |
D | 0.9 | 0.9 | 0.9 |
ΔVC | 0.02 [V] | 0.02 [V] | 0.02 [V] |
ΔIL | 0.14 [A] | 0.14 [A] | 0.14 [A] |
IB | 30 [A] | 30 [A] | 30 [A] |
Vc | - | 400 [V] | 400 [V] |
RDS(on) | 10−6:1:103 [Ω] | 10−6:1: 103 [Ω] | 35 [mΩ] |
rL | 10−6: 1: 103 [Ω] | 10−6: 1: 103 [Ω] | 10−6: 1: 103 [Ω] |
In Figure 11(a), the expression for VC follows (12), while the expressions for L and C in Figures 11(b) and 11(c) are given by (22) and (23), respectively. From Figure 11(a), it is evident that VC decreases significantly with increases in rL and RDS(on), indicating an inverse relationship. This finding aligns with the sensitivity analysis, which showed that higher rL and RDS(on) reduce efficiency due to increased input resistance Rin, impacting the voltage gain terms Av1 and Av2.
In Figure 11(b), the value of L decreases as both rL and RDS(on) decrease, demonstrating a direct relationship between L and these parameters. This relationship is consistent with the overall system's sensitivity to resistive elements, suggesting that minimizing rL and RDS(on) can mitigate resistive losses and positively influence system performance.
In contrast, Figure 11(c) indicates that the magnitude of C remains nearly constant despite variations in rL and rC, confirming the observations in Section 6. The value of C appears close to zero in the plot for scaling purposes, although it is approximately 100 nF.
Figure 12 illustrates the sensitivity of efficiency η as a function of rL and RDS(on), derived from (18). The surface plot reveals that charger performance is maximized under ideal conditions, where rL = RDS(on) = 0. As these non-ideal parameters increase, efficiency η declines sharply, consistent with the sensitivity analysis that showed an inverse relationship between η and both rL and RDS(on). This trend underscores the critical importance of minimizing these resistive losses when designing high-efficiency chargers. Table 3 lists the constant parameters and the variables relevant to this analysis.
Case | Parameter | Value |
η = f(RDS(on), rL) | Vd | 800 [V] |
rC | 1.5 [Ω] | |
rB | 1 [Ω] | |
VOB | 450 [V] | |
D | 0.9 | |
ΔVC | 0.02 [V] | |
ΔIL | 0.14 [A] | |
IB | 30 [A] | |
RDS(on) | l0−6: 1: 103 [Ω] | |
rL | 10−6: 1: 103 [Ω] |
These observations provide essential design insights, highlighting that reducing non-idealities rL, RDS(on), and rC is imperative for maintaining high efficiency in charger applications.
Figure 13 depicts the surfaces plotted in relation to the sensitivity expressions derived in (19)−(21). From this, it can be demonstrated that as rL and RDS(on) increase, the efficiency (η) decreases significantly until a saturated value is reached [see Figure 13(a)]. Additionally, Figure 13(b) illustrates that as rC increases, η also decreases significantly. This further verifies the strong dependence of these non-idealities on the behavior of η.
Figure 14 illustrates the step response of the loop gain in (25). From this figure, it can be observed that the dynamics under transient of (25) behave as a first-order system. Moreover, it can be identified that the system reaches a steady state at approximately 20 ms, demonstrating that the system responds rapidly to disturbances of this nature.
Finally, Figure 15 depicts the root locus diagram of (28). From this figure, it can be concluded that the loop gain exhibits a totally stable behavior, as all its poles are in the left half-plane of the s-plane. Furthermore, it is demonstrated that the system in question (28) has two zeros and two poles.
This work presents a detailed analysis of the two-level battery charger (2L-BC) performance using both switched and averaged models, with simulations conducted to validate the proposed control system and its design. The simulation results demonstrate that the system performs effectively under a range of operating conditions, with robust transient responses and well-behaved dynamic characteristics. Specifically, the battery current, inductor current, and capacitor voltage exhibit first-order dynamics, with settling times of just 30 ms after startup and 4 ms following step changes in load. These results confirm the rapid response capabilities of the system, highlighting the efficient performance of the PI compensator in maintaining minimal steady-state errors and overshoot.
Ripple analysis further supports the adequacy of the system design, with the ripple in inductor current and capacitor voltage kept well below the specified limits, verifying that the inductance and capacitance values chosen meet the performance requirements. The duty cycle shows stable behavior even during load changes, further illustrating the control strategy's effectiveness in maintaining system stability under dynamic conditions. These findings underscore the importance of a well-designed control system that can manage real-time variations while minimizing ripple and ensuring efficient power conversion.
Sensitivity analyses on non-ideal parameters, including inductor resistance, MOSFET on-resistance, and capacitor resistance, reveal their significant impact on system performance, particularly efficiency and equilibrium voltage. As these resistive losses increase, efficiency declines due to higher losses in the power stages. The equilibrium voltage decreases with higher values of inductor resistance and MOSFET on-resistance, highlighting the importance of minimizing these resistances to maintain high efficiency. Additionally, the analysis confirms that the sizing of the inductor and capacitor is well-calibrated to meet ripple requirements without compromising performance.
The efficiency analysis indicates that maximum performance is achieved when resistive losses are minimized. As resistances such as inductor resistance, MOSFET on-resistance, and capacitor resistance increase, efficiency declines sharply, reinforcing the need for careful component selection to reduce these non-idealities. The results suggest that even small increases in these resistances can lead to significant efficiency losses, underscoring the importance of considering component tolerances and non-idealities during the design phase.
The loop gain analysis indicates that the system's dynamics behave as a first-order system, with fast transient responses and minimal overshoot. The system reaches steady-state conditions quickly, demonstrating its ability to respond efficiently to rapid disturbances. The root locus analysis confirms the stability of the control system, with all poles residing in the left half-plane of the s-plane, ensuring robust performance under various operating conditions.
Overall, the findings from this study offer valuable insights into the design and optimization of battery chargers, emphasizing the importance of selecting components with low resistances to maximize system efficiency. The proposed control system is shown to be effective in ensuring stable and efficient operation, providing an essential framework for future developments in high-efficiency power converters. Future research could explore further refinements in the control strategy and experimental validation, as well as the integration of more advanced control techniques, such as model predictive or sliding mode control, to enhance system robustness and transient response, especially in the presence of larger disturbances or component aging.
Conceptualization: JMCS; Formal analysis: JMCS, JLAL, and RF; Investigation: JMCS, JLAL, and RF; Methodology: JMCS and RF; Supervision: JMCS; Validation: JMCS, JLAL, and RF; Visualization: JLAL and RF; Writing − original draft: JMCS; Writing − review & editing: JLAL and RF.
The authors declare that there is no conflict of interest in this paper.
[1] |
T. Hirota, R. So, Y. S. Kim, B. Leventhal, R. A. Epstein, A systematic review of screening tools in non-young children and adults for autism spectrum disorder, Res. Dev. Disabil., 80 (2018), 1–12. https://doi.org/10.1016/j.ridd.2018.05.017 doi: 10.1016/j.ridd.2018.05.017
![]() |
[2] |
C. Lord, S. Risi, P.S. Dilavore, C. Shulman, A. Thurm, A. Pickles. Autism from 2 to 9 years of age. Arch. Gen. Psychiatry, 63 (2006), 694–701. https://doi.org/10.1001/archpsyc.63.6.694 doi: 10.1001/archpsyc.63.6.694
![]() |
[3] |
B. B. Sizoo, E. H. Horwitz, J. P. Teunisse, C. C. Kan, C. Vissers, E. Forceville, et al., Predictive validity of self-report questionnaires in the assessment of autism spectrum disorders in adults, Autism, 19 (2015), 842–849. https://doi.org/10.1177/1362361315589869 doi: 10.1177/1362361315589869
![]() |
[4] |
P. O. Towle, P. A. Patrick, Autism Spectrum Disorder Screening Instruments for Very Young Children: A Systematic Review, Autism. Res. Treat., 2016 (2016), 4624829. https://doi.org/10.1155/2016/4624829 doi: 10.1155/2016/4624829
![]() |
[5] |
D. Bone, S. Bishop, M. P. Black, M. S. Goodwin, C. Lord, S. S. Narayanan, Use of machine learning to improve autism screening and diagnostic instruments: effectiveness, efficiency, and multi-Instrument Fusion, J. Child. Psychol. Psychiatry, 57 (2017), 927–937. https://doi.org/10.1111/jcpp.12559 doi: 10.1111/jcpp.12559
![]() |
[6] |
J. A. Kosmicki, V. Sochat, M. Duda, D. P. Wall, Searching for a minimal set of behaviors for autism detection through feature selection-based machine learning, Transl. Psychiatry, 5 (2015), 514–517. https://doi.org/10.1038/tp.2015.7 doi: 10.1038/tp.2015.7
![]() |
[7] |
F. Thabtah, Machine learning in autistic spectrum disorder behavioral research: A review and ways forward, Informatics Heal. Soc. Care, 44 (2019), 278–297. https://doi.org/10.1080/17538157.2017.1399132 doi: 10.1080/17538157.2017.1399132
![]() |
[8] |
D. H. Oh, I. B. Kim, S. H. Kim, D. H. Ahn, Predicting autism spectrum disorder using blood-based gene expression signatures and machine learning, Clin. Psychopharmacol. Neurosci., 15 (2017), 47–52. https://doi.org/10.9758/cpn.2017.15.1.47 doi: 10.9758/cpn.2017.15.1.47
![]() |
[9] |
M. Duda, R. Ma, N. Haber, D. P. Wall, Use of machine learning for behavioral distinction of autism and ADHD, Transl. Psychiatry, 6 (2016), 732. https://doi.org/10.1038/tp.2015.221 doi: 10.1038/tp.2015.221
![]() |
[10] |
G. Li, O. Lee, H. Rabitz, High-efficiency classification of children with autism spectrum disorder, PLoS One, 13 (2018), 1–23. https://doi.org/10.1371/journal.pone.0192867 doi: 10.1371/journal.pone.0192867
![]() |
[11] |
Q. Tariq, S. L. Fleming, J. N. Schwartz, K. Dunlap, C. Corbin, P. Washington, et al., Detecting Developmental Delay and Autism Through Machine Learning Models Using Home Videos of Bangladeshi Children: Development and Validation Study, J. Med. Internet Res., 21 (2019), 13822. https://doi.org/10.2196/13822 doi: 10.2196/13822
![]() |
[12] | D. Eman, W. R. Emanuel, Machine Learning Classifiers for Autism Spectrum Disorder: A Review, 2019 4th Int. Conf. Inform. Technol. Inform. Syst. Electr. Eng. (ICITISEE), Yogyakarta, Indonesia, 2019. https://doi.org/10.1109/ICITISEE48480.2019.9003807 |
[13] |
X. Bi, Y. Wang, Q. Shu, Q. Sun, Q. Xu, Classification of autism spectrum disorder using random support vector machine cluster, Frontiers in Genetics, 6 (2018), 9–18. https://doi.org/10.3389/fgene.2018.00018 doi: 10.3389/fgene.2018.00018
![]() |
[14] |
E. Grossi, C. Olivieri, M. Buscema, Diagnosis of autism through EEG processed by advanced computational algorithms: a pilot study, Comput. Methods Programs Biomed., 142 (2017), 73–79. https://doi.org/10.1016/j.cmpb.2017.02.002 doi: 10.1016/j.cmpb.2017.02.002
![]() |
[15] | M. L. Raja, M. Priya, Neural network based classification of EEG signals for diagnosis of autism spectrum disorder, Int. J. Pharm. Bio. Sci., 8 (2017), 1020–1026. |
[16] | L. Raja, M. M. Priyab, EEG based ASD diagnosis for children using auto-regressive features and FFNN, Int. J. Control Theo. App., 10 (2017), 27–32. |
[17] | L. Raja, M. M. Priya, EEG based diagnosis of autism spectrum disorder using static and dynamic neural networks, ARPN J. Eng. Appl. Sci., 12 (2017), 4653787. |
[18] |
R. Djemal, K. AlSharabi, S. Ibrahim, A. Alsuwailem, EEG-based computer aided diagnosis of autism spectrum disorder using wavelet, entropy, and ANN, BioMed. Res. Int., 2017 (2017), 1–9. https://doi.org/10.1155/2017/9816591 doi: 10.1155/2017/9816591
![]() |
[19] |
T. M. Heunis, C. Aldrich, P. J. Vries, Recent Advances in Resting-State Electroencephalography Biomarkers for Autism Spectrum Disorder-A Review of Methodological and Clinical Challenges, Rev. Pediatr. Neurol., 61 (2016), 28–37. https://doi.org/10.1016/j.pediatrneurol.2016.03.010 doi: 10.1016/j.pediatrneurol.2016.03.010
![]() |
[20] | N. P. Jordanova, J. P. Jordanov, Spectrum-weighted EEG frequency ("brain-rate") as a quantitative indicator of mental arousal. Prilozi, 26 (2005), 35–42. |
[21] |
E. Abdulhay, M. Alafeef, A. Abdelhay, A. Al-Bashir, Classification of Normal, Ictal and Inter-ictal EEG via Direct Quadrature and Random Forest Tree, J. Med. Biol. Eng., 37 (2017), 843–857. https://doi.org/10.1007/s40846-017-0239-z doi: 10.1007/s40846-017-0239-z
![]() |
[22] | Z. Dandan, D. Haiyan, H. Xinlin, L. Yunfeng, Z. Congle, Y. Datian, The Combination of Amplitude and Sample Entropy in EEG and its Application to Assessment of Cerebral Injuries in Piglets, 2008 Int. Conf. BioMed. Eng. Informatics, Sanya, China, 2008. https://doi.org/10.1109/BMEI.2008.12 |
[23] |
E. Abdulhay, M. Alafeef, L. Alzghoul, M. Al Momani, R. Al Abdi, N. Arunkumar, et al., Computer-aided autism diagnosis via second-order difference plot area applied to EEG empirical mode decomposition, Neural Comput. Appl., 32 (2020), 10947–10956. https://doi.org/10.1007/s00521-018-3738-0 doi: 10.1007/s00521-018-3738-0
![]() |
[24] |
R. J. Oweis, E. W. Abdulhay, Seizure classification in EEG signals utilizing Hilbert-Huang transform, Biomed. Eng. Online, 10 (2011), 38. https://doi.org/10.1186/1475-925X-10-38 doi: 10.1186/1475-925X-10-38
![]() |
[25] | E. Abdulhay, M. Alafeef, H. Hadoush, N. Alomari, M. Bashayreh, Frequency 3D Mapping and Inter-Channel Stability of EEG Intrinsic Function Pulsation: Indicators Towards Autism Spectrum Diagnosis, 2017 10th Jordanian Int. Electric. Electron. Eng. Conf. (JIEEEC), Amman, Jordan, 2017. https://doi.org/10.1109/JIEEEC.2017.8051416 |
[26] |
H. Hadoush, M. Alafeef, E. Abdulhay, Automated identification for autism severity level: EEG analysis using empirical mode decomposition and second order difference plot, Behavioural Brain Res., 362 (2019), 240–248. https://doi.org/10.1016/j.bbr.2019.01.018 doi: 10.1016/j.bbr.2019.01.018
![]() |
[27] |
E. Abdulhay, V. Elamaran, M. Chandrasekar, V. S. Balaji, and K. Narasimhan, Automated diagnosis of epilepsy from EEG signals using ensemble learning approach, Pattern Recognition Letters, 139 (2020), 174–181. https://doi.org/10.1016/j.patrec.2017.05.021 doi: 10.1016/j.patrec.2017.05.021
![]() |
[28] |
T. H. Pham, J. Vicnesh, J. K. Wei, S. J. Oh, N. Arunkumar, E. Abdulhay, et al., Autism spectrum disorder diagnostic system using HOS bispectrum with EEG signals, Int. J. Environ. Res. Public Health, 17 (2020), 1–14. https://doi.org/10.3390/ijerph17030971 doi: 10.3390/ijerph17030971
![]() |
[29] |
W. Bosl, A. Tierney, H. T. Flusberg, C. Nelson, EEG complexity as a biomarker for autism spectrum disorder risk, BMC Med., 9 (2011), 18. https://doi.org/10.1186/1741-7015-9-18 doi: 10.1186/1741-7015-9-18
![]() |
[30] |
F. H. Duffy, A. Heidelise, Autism, spectrum or clusters? An EEG coherence study, BMC Neurol., 19 (2019), 27. https://doi.org/10.1186/s12883-019-1254-1 doi: 10.1186/s12883-019-1254-1
![]() |
[31] | A. Sheikhani, H. Behnam, M. R. Mohammadi, M. Noroozian, Analysis of EEG background activity in Autsim disease patients with bispectrum and STFT measure, Proceedings of the 11th WSEAS Int. Conf. Commun., Agios Nikolaos, Greece, 2007. |
[32] |
J. Kang, H. Chen, X. Li, X. Li, EEG entropy analysis in autistic children, J. Clin. Neurosci., 62 (2019), 199–206. https://doi.org/10.1016/j.jocn.2018.11.027 doi: 10.1016/j.jocn.2018.11.027
![]() |
[33] |
L. Billeci, F. Sicca, K. Maharatna, F. Apicella, A. Narzisi, G. Campatelli, et al., On the application of quantitative EEG for characterizing autistic brain: a systematic review, Front. Hum. Neurosci., 7 (2013), 442. https://doi.org/10.3389/fnhum.2013.00442 doi: 10.3389/fnhum.2013.00442
![]() |
[34] |
M. Ahmadlou, H. Adeli, A. Adeli, Fractality and a wavelet-chaos-neural network methodology for EEG-based diagnosis of autistic spectrum disorder, J. Clin. Neurophysiol., 27 (2010), 328–333. https://doi.org/10.1097/WNP.0b013e3181f40dc8 doi: 10.1097/WNP.0b013e3181f40dc8
![]() |
[35] | B. B. Mandelbrot, The Fractal Geometry of Nature. New York: Freeman and Company (1977), 1–468. |
[36] |
M. Costa, A. L. Goldberger, C. K. Peng, Multiscale entropy analysis of biological signals. Phys. Rev. E., 71 (2005), 021906. https://doi.org/10.1103/PhysRevE.71.021906 doi: 10.1103/PhysRevE.71.021906
![]() |
[37] |
A. Namdari, Z. Li, A review of entropy measures for uncertainty quantification of stochastic processes, Adv. Mechanical Eng., 11 (2019), 1–14. https://doi.org/10.1177/1687814019857350 doi: 10.1177/1687814019857350
![]() |
[38] |
H. Hadoush, M. Alafeef, E. Abdulhay, Brain complexity in children with mild and severe autism spectrum disorders: analysis of multiscale entropy in EEG, Brain Topography, 32 (2019), 914–921. https://doi.org/10.1007/s10548-019-00711-1 doi: 10.1007/s10548-019-00711-1
![]() |
[39] |
Y. Ghanbari, L. Bloy, J. C. Edgar, L. Blaskey, R. Verma, T. P. Roberts, Joint analysis of band-specific functional connectivity and signal complexity in autism, J. Autism Dev. Disord., 45 (2015), 444–460. https://doi.org/10.1007/s10803-013-1915-7 doi: 10.1007/s10803-013-1915-7
![]() |
[40] |
T. Liu, Y. Chen, D. Chen, C. Li, Y. Qiu, J. Wang, Altered electroencephalogram complexity in autistic children shown by the multiscale entropy approach, Neuro. Report, 28 (2017), 169–173. https://doi.org/10.1097/WNR.0000000000000724 doi: 10.1097/WNR.0000000000000724
![]() |
[41] | J. O. Maximo, D. L. Murdaugh, R. K. Kana, Alterations in Brain Entropy in Autism Spectrum Disorders, 2017 Int. Meet. Autism Res., Birmingham, USA, 2017. |
[42] |
J. Q. Kosciessa, N. A. Kloosterman, D. D. Garrett, Standard multiscale entropy reflects neural dynamics at mismatched temporal scales: What's signal irregularity got to do with it?, PLOS Comput. Biol., 16 (2020), e1007885. https://doi.org/10.1371/journal.pcbi.1007885 doi: 10.1371/journal.pcbi.1007885
![]() |
[43] |
A. Catarino, O. Churches, S. B. Cohen, A. Andrade, H. Ring, Atypical EEG complexity in autism spectrum conditions: a multiscale, entropy analysis, Clin. Neurophysiol., 122 (2011), 2375–2383. https://doi.org/10.1016/j.clinph.2011.05.004 doi: 10.1016/j.clinph.2011.05.004
![]() |
[44] |
J. S. Richman, J. R. Moorman, Physiological time-series analysis using approximate entropy and sample entropy, Am. J. Physiol. Heart Circ. Physiol., 278 (2000), H2039–49. https://doi.org/10.1152/ajpheart.2000.278.6.H2039 doi: 10.1152/ajpheart.2000.278.6.H2039
![]() |
[45] |
R. Ferenets, T. Lipping, A. Anier, V. Jantti, S. Melto, S. Hovilehto, Comparison of entropy and complexity measures for the assessment of depth of sedation, IEEE Trans. Biomed. Eng., 53 (2006), 1067–1077. https://doi.org/10.1109/TBME.2006.873543 doi: 10.1109/TBME.2006.873543
![]() |
[46] |
A. H. Heurtier, The Multiscale Entropy Algorithm and Its Variants: A Review, Entropy, 17 (2015), 3110–3123. https://doi.org/10.3390/e17053110 doi: 10.3390/e17053110
![]() |
[47] |
H. Azami and J. Escudero, Amplitude- and Fluctuation-Based Dispersion Entropy, Entropy, 20 (2018), 210. https://doi.org/10.3390/e20030210 doi: 10.3390/e20030210
![]() |
[48] |
J. F. Valencia, A. Porta, M. Vallverdu, F. Claria, R. Baranowski, E. O. Baranowska, et al., Refined multiscale entropy: Application to 24-h Holter recordings of heart period variability in healthy and aortic stenosis subjects, IEEE Trans. Biomed., 56 (2009), 2202–2213. https://doi.org/10.1109/TBME.2009.2021986 doi: 10.1109/TBME.2009.2021986
![]() |
[49] | J. F. Valencia, M. Vallverdu, R. Schroeder, L. Cygankiewicz, R. Vazquez, A. B. Luna, et al., Heart rate variability characterized by refined multiscale entropy applied to cardiac death in ischemic cardiomyopathy patients, Comput. Cardiol., 37 (2010), 65–68. |
[50] |
W. J. Bosl, T. Loddenkemper, C. A. Nelson, Nonlinear EEG biomarker profiles for autism and absence epilepsy, Neuropsychiatric Electrophysiology, 3 (2017), 1. https://doi.org/10.1186/s40810-017-0023-x doi: 10.1186/s40810-017-0023-x
![]() |
[51] |
W. J. Bosl, H. T. Flusberg, C. A. Nelson, EEG Analytics for Early Detection of Autism Spectrum Disorder: A data-driven approach, Sci. Rep., 8 (2018), 6828. https://doi.org/10.1038/s41598-018-24318-x doi: 10.1038/s41598-018-24318-x
![]() |
[52] |
S. D. Wu, C.W. Wu, K.Y. Lee, S. G. Lin, Modified multiscale entropy for short-term time series analysis, Physica A, 392 (2013), 15865–5873. https://doi.org/10.1016/j.physa.2013.07.075 doi: 10.1016/j.physa.2013.07.075
![]() |
[53] |
S. D. Wu, C. W. Wu, S. G. Lin, C. C. Wang, K. Y. Lee, Time series analysis using composite multiscale entropy, Entropy, 15 (2013), 1069–1084. https://doi.org/10.3390/e15031069 doi: 10.3390/e15031069
![]() |
[54] |
S. D. Wu, C. W. Wu, S. G. Lin, K. Y. Lee, C. K. Peng, Analysis of complex time series using refined composite multiscale entropy, Phys. Lett. A, 378 (2014), 1369–1374. https://doi.org/10.1016/j.physleta.2014.03.034 doi: 10.1016/j.physleta.2014.03.034
![]() |
[55] |
S. D. Wu, C. W. Wu, K. Y. Lee, S. G. Lin, Modified multiscale entropy for short-term time series analysis, Phys. A, 392 (2013), 5865–5873. https://doi.org/10.1016/j.physa.2013.07.075 doi: 10.1016/j.physa.2013.07.075
![]() |
[56] |
Y. C. Chang, H. T. Wu, H. R. Chen, A. B. Liu, J. J. Yeh, M. T. Lo, et al., Application of a modified entropy computational method in assessing the complexity of pulse wave velocity signals in healthy and diabetic subjects, Entropy, 16 (2014), 4032–4043. https://doi.org/10.3390/e16074032 doi: 10.3390/e16074032
![]() |
[57] |
Y. Jiang, C. K. Peng, Y. Xu, Hierarchical entropy analysis for biological signals, J. Comput. Appl. Math., 236 (2011), 728–742. https://doi.org/10.1016/j.cam.2011.06.007 doi: 10.1016/j.cam.2011.06.007
![]() |
[58] |
H. B. Xie, W. X. He, H. Liu, Measuring time series regularity using nonlinear similarity-based sample entropy, Phys. Lett. A, 372 (2008), 7140–7146. https://doi.org/10.1016/j.physleta.2008.10.049 doi: 10.1016/j.physleta.2008.10.049
![]() |
[59] |
M. U. Ahmed, D. P. Mandic, Multivariate multiscale entropy analysis, IEEE Signal Process. Lett., 19 (2012), 91–94. https://doi.org/10.1109/LSP.2011.2180713 doi: 10.1109/LSP.2011.2180713
![]() |
[60] |
M. D. Costa, A. L. Goldberger, Generalized multiscale entropy analysis: Application to quantifying the complex volatility of human heartbeat time series, Entropy, 17 (2015), 1197–1203. https://doi.org/10.3390/e17031197 doi: 10.3390/e17031197
![]() |
[61] |
L. Faes, A. Porta, M. Javorka, G. Nollo, Efficient Computation of Multiscale Entropy over Short Biomedical Time Series Based on Linear State-Space Models, Complexity, 2017 (2017), 1768264. https://doi.org/10.1155/2017/1768264 doi: 10.1155/2017/1768264
![]() |
[62] |
T. Takahashi, Complexity of spontaneous brain activity in mental disorders, Prog. Neuropsychopharmacol. Biol. Psychiatry, 45 (2013), 258–266. https://doi.org/10.1016/j.pnpbp.2012.05.001 doi: 10.1016/j.pnpbp.2012.05.001
![]() |
[63] |
N. Huang, Z. Shen, S. Long, M. Wu, H. H. Shih, Q. Zheng, et al., The empirical mode decomposition and the Hilbert spectrum for nonlinear and non-stationary time series analysis, Proc. Math. Phys. Eng. Sci., 454 (1998), 903–995. https://doi.org/10.1098/rspa.1998.0193 doi: 10.1098/rspa.1998.0193
![]() |
[64] |
N. E. Huang, Z. Wu, A review on Hilbert-Huang transform: Method and its applications to geophysical studies, Rev. Geophys., 46 (2008), 228–251. https://doi.org/10.1029/2007RG000228 doi: 10.1029/2007RG000228
![]() |
[65] | F. R. Kschischang, The Hilbert Transform. Toronto: University of Toronto, 2006. |
[66] | E. Abdulhay, P.Y. Guméry, J. Fontecave, P. Baconnier, Cardiogenic oscillations extraction in inductive plethysmography: Ensemble empirical mode decomposition, Annu. Int. Conf. IEEE Eng. Med. Biol. Soc., Minnesota, USA, 2009, 2240–2243. https://doi.org/10.1109/IEMBS.2009.5335004 |
[67] |
X. Han, J. Peng, A. Cui, F. Zhao, Sparse Principal Component Analysis via Fractional Function Regularity, Math. Probl. Eng., 2020 (2020), 7874140. https://doi.org/10.1155/2020/7874140 doi: 10.1155/2020/7874140
![]() |
[68] |
C. K. Arthur, V. A. Temeng, Y. Y. Ziggah, Performance Evaluation of Training Algorithms in Backpropagation Neural Network Approach to Blast-Induced Ground Vibration Prediction, Ghana Mining J., 20 (2020), 20–33. https://doi.org/10.4314/gm.v20i1.3 doi: 10.4314/gm.v20i1.3
![]() |
[69] |
K. Kovarski, J. Malvy, R. K. Khanna, S. Arsène, M. Batty, M. Latinus, Reduced visual evoked potential amplitude in autism spectrum disorder, a variability effect?, Translational Psychiatry, 9 (2019), 341. https://doi.org/10.1038/s41398-019-0672-6 doi: 10.1038/s41398-019-0672-6
![]() |
[70] |
S. A. Nastase, V. Iacovella, B. Davis, U. Hasson, Connectivity in the human brain dissociates entropy and complexity of auditory inputs, NeuroImage, 31 (2015), 292–300. https://doi.org/10.1016/j.neuroimage.2014.12.048 doi: 10.1016/j.neuroimage.2014.12.048
![]() |
[71] |
P. Barttfeld, B. Wicker, S. Cukier, S. Navarta, S. Lew, M. Sigman, A big-world network in ASD: dynamical connectivity analysis reflects a deficit in longrange connections and an excess of short-range connections, Neuropsychologia, 49 (2015), 254–263. https://doi.org/10.1016/j.neuropsychologia.2010.11.024 doi: 10.1016/j.neuropsychologia.2010.11.024
![]() |
[72] |
H. Zhang, R. Li, X. Wen, Q. Li, X. Wu, Altered Time-Frequency Feature in Default Mode Network of Autism Based on Improved Hilbert-Huang Transform, IEEE J. Biomed. Health Informatics, 25 (2021), 485–492. https://doi.org/10.1109/JBHI.2020.2993109 doi: 10.1109/JBHI.2020.2993109
![]() |
[73] |
T. Wadhera, D. Kakkar, Conditional entropy approach to analyze cognitive dynamics in autism spectrum disorder, Neurol. Res., 42 (2020), 869–878. https://doi.org/10.1080/01616412.2020.1788844 doi: 10.1080/01616412.2020.1788844
![]() |
[74] |
E. Gani, N. Handayani, S. H. Pratama, N. Afif, F. Aziezah, A. C. Keintjem, et al., Brainwaves Analysis Using Spectral Entropy in Children with Autism Spectrum Disorders (ASD), J. phys. Conf. ser., 1505 (2020), 012070. https://doi.org/10.1088/1742-6596/1505/1/012070 doi: 10.1088/1742-6596/1505/1/012070
![]() |
[75] |
E. Amiot, Entropy of Fourier coefficients of periodic musical objects, J. Math. Music, 15 (2021), 235–246. https://doi.org/10.1080/17459737.2020.1777592 doi: 10.1080/17459737.2020.1777592
![]() |
[76] |
D. Abásolo, R. Hornero, P. Espino, D. Alvarez, J. Poza, Entropy analysis of the EEG background activity in Alzheimer's disease patients, Physiol. Meas., 27 (2006), 241–253. https://doi.org/10.1088/0967-3334/27/3/003 doi: 10.1088/0967-3334/27/3/003
![]() |
[77] |
J. Han, Y. Li, J. Kang, E. Cai, Z. Tong, G. Ouyang, et al., Global Synchronization of Multichannel EEG Based on Rényi Entropy in Children with Autism Spectrum Disorder, Appl. Sci., 7 (2017), 257. https://doi.org/10.3390/app7030257 doi: 10.3390/app7030257
![]() |
[78] |
E. Abdulhay, M. Alafeef, H. Hadoush, N. Arunkumar, Resting State EEG-based Diagnosis of Autism via Elliptic Area of Continuous Wavelet Transform Complex Plot, J. Intell. fuzzy syst., 39 (2020), 8599–8607. https://doi.org/10.3233/JIFS-189176 doi: 10.3233/JIFS-189176
![]() |
[79] |
R. Okazaki, T. Takahashi, K. Ueno, K. Takahashi, M. Ishitobi, M. Kikuchi, et al., Changes in EEG complexity with electroconvulsive therapy in a patient with autism spectrum disorders: a multiscale entropy approach, Front. Hum. Neurosci., 9 (2015), 25767444. https://doi.org/10.3389/fnhum.2015.00106 doi: 10.3389/fnhum.2015.00106
![]() |
[80] | S. Thapaliya, S. Jayarathna, M. Jaime, Evaluating the EEG and eye movements for autism spectrum disorder, 2018 IEEE Int. Conf. Big Data, Seattle, WA, USA, 2018. https://doi.org/10.1109/BigData.2018.8622501 |
[81] |
J. Eldridge, A. E. Lane, M. Belkin, S. Dennis, Robust features for the automatic identification of autism spectrum disorder in children, J. Neurodev. Disord., 6 (2014), 1–12. https://doi.org/10.1186/1866-1955-6-12 doi: 10.1186/1866-1955-6-1
![]() |
[82] |
H. Amoud, H. Snoussi, D. Hewson, M. Doussot, J. Duchêne, Intrinsic mode entropy for nonlinear discriminant analysis, IEEE Signal Process. Lett., 14 (2007), 297–300. https://doi.org/10.1109/LSP.2006.888089 doi: 10.1109/LSP.2006.888089
![]() |
[83] |
M. Hu, H. Liang, Adaptive multiscale entropy analysis of multivariate neural data, IEEE Trans. Biomed. Eng., 59 (2012), 12–15. https://doi.org/10.1109/TBME.2011.2162511 doi: 10.1109/TBME.2011.2162511
![]() |
[84] |
O. Dekhil, M. Ali, Y. E. Nakeib, A. Shalaby, A. Soliman, A. Switala, et.al., A Personalized Autism Diagnosis CAD System Using a Fusion of Structural MRI and Resting-State Functional MRI Data. Front. Psychiatry, 10 (2021), 1–16. https://doi.org/10.3389/fpsyt.2019.00392 doi: 10.3389/fpsyt.2019.00392
![]() |
[85] |
O. Dekhil, M. Ali, R. Haweel, Y. Elnakeib, M. Ghazal, H. Hajjdiab, et.al. A Comprehensive Framework for Differentiating Autism Spectrum Disorder From Neurotypicals by Fusing Structural MRI and Resting State Functional MRI, Seminars in Pediatric Neurology., 34 (2020), 100805. https://doi.org/10.1016/j.spen.2020.100805 doi: 10.1016/j.spen.2020.100805
![]() |
[86] | K. Barik, K. Watanabe, J. Bhattacharya, G. Saha, Classification of Autism in Young Children by Phase Angle Clustering in Magnetoencephalogram Signals, 2020 National Conf. Commun. (NCC), Kharagpur, India, 2020, 1–6. https://doi.org/10.1109/NCC48643.2020.9056022 |
Parameter | Value | Parameter | Value |
RDS(on) | 35 [mΩ] | C | 100 [ηF] |
rL | 1 [Ω] | vOB | 450 [V] |
rC | 1.5 [Ω] | D | 0.9 |
rC | 1 [Ω] | ΔVC | 5 [%] |
L | 9.5 [mH] | ΔIL | 5 [%] |
Parameter | VC = f(RDS(on), rL) | L = f(RDS(on), rL) | C = f(rC, rL) |
Vd | 800 [V] | 800 [V] | 800 [V] |
rC | 1.5 [Ω] | 1.5 [Ω] | 10−6: 1: 103 [Ω] |
rB | 1 [Ω] | 1 [Ω] | 1 [Ω] |
VOB | 450 [V] | 450 [V] | 450 [V] |
D | 0.9 | 0.9 | 0.9 |
ΔVC | 0.02 [V] | 0.02 [V] | 0.02 [V] |
ΔIL | 0.14 [A] | 0.14 [A] | 0.14 [A] |
IB | 30 [A] | 30 [A] | 30 [A] |
Vc | - | 400 [V] | 400 [V] |
RDS(on) | 10−6:1:103 [Ω] | 10−6:1: 103 [Ω] | 35 [mΩ] |
rL | 10−6: 1: 103 [Ω] | 10−6: 1: 103 [Ω] | 10−6: 1: 103 [Ω] |
Case | Parameter | Value |
η = f(RDS(on), rL) | Vd | 800 [V] |
rC | 1.5 [Ω] | |
rB | 1 [Ω] | |
VOB | 450 [V] | |
D | 0.9 | |
ΔVC | 0.02 [V] | |
ΔIL | 0.14 [A] | |
IB | 30 [A] | |
RDS(on) | l0−6: 1: 103 [Ω] | |
rL | 10−6: 1: 103 [Ω] |
Parameter | Value | Parameter | Value |
RDS(on) | 35 [mΩ] | C | 100 [ηF] |
rL | 1 [Ω] | vOB | 450 [V] |
rC | 1.5 [Ω] | D | 0.9 |
rC | 1 [Ω] | ΔVC | 5 [%] |
L | 9.5 [mH] | ΔIL | 5 [%] |
Parameter | VC = f(RDS(on), rL) | L = f(RDS(on), rL) | C = f(rC, rL) |
Vd | 800 [V] | 800 [V] | 800 [V] |
rC | 1.5 [Ω] | 1.5 [Ω] | 10−6: 1: 103 [Ω] |
rB | 1 [Ω] | 1 [Ω] | 1 [Ω] |
VOB | 450 [V] | 450 [V] | 450 [V] |
D | 0.9 | 0.9 | 0.9 |
ΔVC | 0.02 [V] | 0.02 [V] | 0.02 [V] |
ΔIL | 0.14 [A] | 0.14 [A] | 0.14 [A] |
IB | 30 [A] | 30 [A] | 30 [A] |
Vc | - | 400 [V] | 400 [V] |
RDS(on) | 10−6:1:103 [Ω] | 10−6:1: 103 [Ω] | 35 [mΩ] |
rL | 10−6: 1: 103 [Ω] | 10−6: 1: 103 [Ω] | 10−6: 1: 103 [Ω] |
Case | Parameter | Value |
η = f(RDS(on), rL) | Vd | 800 [V] |
rC | 1.5 [Ω] | |
rB | 1 [Ω] | |
VOB | 450 [V] | |
D | 0.9 | |
ΔVC | 0.02 [V] | |
ΔIL | 0.14 [A] | |
IB | 30 [A] | |
RDS(on) | l0−6: 1: 103 [Ω] | |
rL | 10−6: 1: 103 [Ω] |