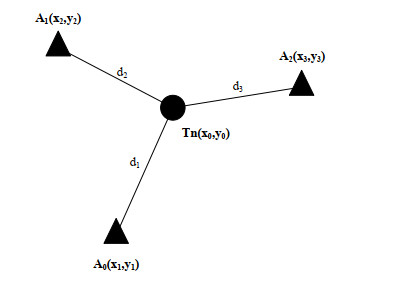
In this work, we investigate the transmission dynamics of the Zika virus, considering both a compartmental model involving humans and mosquitoes and an extended model that introduces a non-human primate (monkey) as a second reservoir host. The novelty of our approach lies in the later generalization of the model using a fractional time derivative. The significance of this study is underscored by its contribution to understanding the complex dynamics of Zika virus transmission. Unlike previous studies, we incorporate a non-human primate reservoir host into the model, providing a more comprehensive representation of the disease spread. Our results reveal the importance of utilizing a nonstandard finite difference (NSFD) scheme to simulate the disease's dynamics accurately. This NSFD scheme ensures the positivity of the solution and captures the correct asymptotic behavior, addressing a crucial limitation of standard solvers like the Runge-Kutta Fehlberg method (ode45). The numerical simulations vividly demonstrate the advantages of our approach, particularly in terms of positivity preservation, offering a more reliable depiction of Zika virus transmission dynamics. From these findings, we draw the conclusion that considering a non-human primate reservoir host and employing an NSFD scheme significantly enhances the accuracy and reliability of modeling Zika virus transmission. Researchers and policymakers can use these insights to develop more effective strategies for disease control and prevention.
Citation: Maghnia Hamou Maamar, Matthias Ehrhardt, Louiza Tabharit. A nonstandard finite difference scheme for a time-fractional model of Zika virus transmission[J]. Mathematical Biosciences and Engineering, 2024, 21(1): 924-962. doi: 10.3934/mbe.2024039
[1] | Cuicui Cai, Maosheng Fu, Xianmeng Meng, Chaochuan Jia, Mingjing Pei . Indoor high-precision visible light positioning system using Jaya algorithm. Mathematical Biosciences and Engineering, 2023, 20(6): 10358-10375. doi: 10.3934/mbe.2023454 |
[2] | Cheng Zhu, Yong Zhang, Xuhua Pan, Qi Chen, Qingyu Fu . Improved Harris Hawks Optimization algorithm based on quantum correction and Nelder-Mead simplex method. Mathematical Biosciences and Engineering, 2022, 19(8): 7606-7648. doi: 10.3934/mbe.2022358 |
[3] | Shuang Wang, Heming Jia, Qingxin Liu, Rong Zheng . An improved hybrid Aquila Optimizer and Harris Hawks Optimization for global optimization. Mathematical Biosciences and Engineering, 2021, 18(6): 7076-7109. doi: 10.3934/mbe.2021352 |
[4] | Yuheng Wang, Yongquan Zhou, Qifang Luo . Parameter optimization of shared electric vehicle dispatching model using discrete Harris hawks optimization. Mathematical Biosciences and Engineering, 2022, 19(7): 7284-7313. doi: 10.3934/mbe.2022344 |
[5] | Shenghan Li, Linlin Ye . Multi-level thresholding image segmentation for rubber tree secant using improved Otsu's method and snake optimizer. Mathematical Biosciences and Engineering, 2023, 20(6): 9645-9669. doi: 10.3934/mbe.2023423 |
[6] | Wang Cai, Jianzhuang Wang, Longchao Cao, Gaoyang Mi, Leshi Shu, Qi Zhou, Ping Jiang . Predicting the weld width from high-speed successive images of the weld zone using different machine learning algorithms during laser welding. Mathematical Biosciences and Engineering, 2019, 16(5): 5595-5612. doi: 10.3934/mbe.2019278 |
[7] | Hong Gao, Cuiyun Wu, Dunnian Huang, Dahui Zha, Cuiping Zhou . Prediction of fetal weight based on back propagation neural network optimized by genetic algorithm. Mathematical Biosciences and Engineering, 2021, 18(4): 4402-4410. doi: 10.3934/mbe.2021222 |
[8] | Feng Li, Zhongao Bi, Hongzeng Xu, Yunqi Shi, Na Duan, Zhaoyu Li . Design and implementation of a smart Internet of Things chest pain center based on deep learning. Mathematical Biosciences and Engineering, 2023, 20(10): 18987-19011. doi: 10.3934/mbe.2023840 |
[9] | Chun-Chao Yeh, Ke-Jia Jhang, Chin-Chun Chang . An intelligent indoor positioning system based on pedestrian directional signage object detection: a case study of Taipei Main Station. Mathematical Biosciences and Engineering, 2020, 17(1): 266-285. doi: 10.3934/mbe.2020015 |
[10] | Xinli Hu . Threshold dynamics for a Tuberculosis model with seasonality. Mathematical Biosciences and Engineering, 2012, 9(1): 111-122. doi: 10.3934/mbe.2012.9.111 |
In this work, we investigate the transmission dynamics of the Zika virus, considering both a compartmental model involving humans and mosquitoes and an extended model that introduces a non-human primate (monkey) as a second reservoir host. The novelty of our approach lies in the later generalization of the model using a fractional time derivative. The significance of this study is underscored by its contribution to understanding the complex dynamics of Zika virus transmission. Unlike previous studies, we incorporate a non-human primate reservoir host into the model, providing a more comprehensive representation of the disease spread. Our results reveal the importance of utilizing a nonstandard finite difference (NSFD) scheme to simulate the disease's dynamics accurately. This NSFD scheme ensures the positivity of the solution and captures the correct asymptotic behavior, addressing a crucial limitation of standard solvers like the Runge-Kutta Fehlberg method (ode45). The numerical simulations vividly demonstrate the advantages of our approach, particularly in terms of positivity preservation, offering a more reliable depiction of Zika virus transmission dynamics. From these findings, we draw the conclusion that considering a non-human primate reservoir host and employing an NSFD scheme significantly enhances the accuracy and reliability of modeling Zika virus transmission. Researchers and policymakers can use these insights to develop more effective strategies for disease control and prevention.
With the rapid development of innovations in communication technology and the notoriety of remote systems, the desire for enhanced indoor positioning continues to expand [1]. However, when facing complex indoor environments, such as with the real-time positioning of personnel, equipment and materials in office buildings, factories and construction sites [2], real-time care and management of the elderly [3], precise electronic positioning guidance inside hospitals [4] and precise location trajectories of supervised persons in prisons [5], there is an expanding requirement for high-precision situating innovation. Thus, strategies for advancing the exactness of indoor situating tasks have become of increasing interest to a range of researchers.
Indoor positioning technology is developing rapidly today and is classified as building-dependent or building-independent, depending on the sensors used for positioning [6]. The most common building-independent indoor positioning techniques are pedestrian dead reckoning (PDR) systems [7] and image-based techniques that use cameras [8]. Although the noise is small and stable in the short term when using PDR systems, such approaches cannot be used for long times due to the accumulation of errors, and toward solving this problem, the mainstream research direction is currently the fusion algorithm. Various fusion algorithms are proposed in [9,10,11], such as fusion algorithms with Wi-Fi, Bluetooth, RSS fingerprinting, etc., which effectively reduce positioning error and improve robustness. The four aspects of a typical inertial measurement unit(IMU)-based PDR system framework are direction updating, step detection, step estimation and map matching [12]. Navigation systems include techniques such as orientation updating and map matching, whereas step detection and estimation are specialized to human motion since they exploit the constraint of pedestrian steps. The camera is used for positioning in image-based indoor positioning tasks. The camera used in such applications can be monocular [13], stereo [14] or RGB-D [15]. Because it is difficult to obtain accurate positioning results with a single method, a hybrid positioning method in such a positioning system is introduced as the reliable option. The existing techniques for IMU-based positioning, camera-based positioning and various existing hybrid methods for indoor positioning are discussed in [16], and a hybrid indoor positioning system that uses IMU sensors and smartphone cameras is proposed, which effectively improves indoor positioning accuracy.
Building-independent positioning technologies, of which there are many types, fall into two main categories: namely, those that utilize the existing building infrastructure and those that require dedicated infrastructure. The former category includes Wi-Fi, cellular and Bluetooth. Most Wi-Fi-based positioning systems can be used as RSS-based measurement systems. Various Wi-Fi restrictions must also be considered: for example, signal attenuation in static contexts such as walls and floors, as well as changes in interior environments [17]. Although the cellular network has a wide coverage area, it still suffers from low positioning accuracy. One of the disadvantages of employing Bluetooth technology for localization is that it performs the device discovery operation for each location finding event; as a result, the localization latency (10-30 s) and consumption are greatly increased. As a result, the latency of a Bluetooth device is inadequate for real-time positioning applications. However, for the previously described Wi-Fi localization, a hybrid deep learning model (HDLM)-based indoor localization system was developed in [18]. Instead of raw RSSI signals from APs, the proposed HDLM-based localization system uses RSSI heatmaps. As a result, the Wi-Fi-RSSI signal-based localization system's localization performance is improved. For the latter, the main positioning technologies are RFID, UWB, infrared, ultrasonic, ZigBee, VLC, acoustic, etc. RFID technology requires a large number of tags, which do not meet the requirements of real-time positioning, and its positioning accuracy is related to the deployment density of tags [19]. Indoor positioning systems based on infrared possess several drawbacks, such as security and privacy concerns. The position determination of infrared signals is limited due to interference from fluorescent light and sunlight. In addition, the hardware and maintenance expenses of IR-based indoor systems are high [20]. Reflected waves propagated by other sources can cause severe interference for ultrasound devices (e.g., metal collisions) [21]. The ZigBee positioning accuracy is low, as is its data transfer rate [22].
UWB is a remote correspondence innovation that transfers a bandwidth of more than 1 GHz. Due to its strong anti-interference performance, resistance to multipath interference, high transmission rate, low transmission power and increased penetration capability, UWB positioning technology is widely used for positioning in indoor environments. UWB can provide high positioning accuracy [23,24,25]. Currently, the various UWB ranging methods can be classified into time of arrival (TOA), received signal strength (RSS) and time difference of arrival (TDOA) [26]. However, in complex indoor environments, where walls or other signals block objects, the signal transmission distance increases, and the transmission time lengthens, significantly reducing UWB positioning accuracy. A single indoor positioning method can no longer meet the growing requirements for localization accuracy. Therefore, mixed localization algorithms are becoming increasingly attractive [27].
The localization equation is usually a nonlinear equation based on TOA or TDOA; as a result, the equation must be converted into a linear equation for solving [28]. Currently, nonlinear problems are mainly solved by the least-squares estimation (LSE) algorithm, Fang algorithm, Chan algorithm and Taylor algorithm. The weighting matrix of the LSE algorithm should be decided according to the precision of the measurement data; in practical applications, the selection of the weighting matrix is difficult. The best estimation performance of the algorithms developed by Chan and Fang is achieved when the time difference measurement error is small, although the performance of both algorithms is seriously affected by the large time difference measurement error in the real channel environment [29]. In recent years, deep learning approaches have been commonly employed for indoor positioning because of their excellent nonlinear mapping capabilities and self-adaptive and self-learning abilities [30]. For example, Zhuo et al. suggested a UWB locating technique based on BPNN that is applied to automatic parking, showing good real-time performance and accuracy [31]. In [32], a long short-term memory (LSTM) network is proposed to reduce the position error in UWB systems; this network solves the LOS problem associated with UWB systems and improves the positioning accuracy. Shuai et al. applied the 60 GHz pulsed-IR algorithm to a BPNN, which was used to improve the ability of conventional algorithms to accurately estimate signal fading due to the complexity of indoor channels, thus improving localization accuracy [33]. Lian et al. proposed an optimized K-means clustering BPNN UWB positioning approach, which enhances the original data in the UWB positioning system and preprocesses the K-means clustering algorithm to filter the data with a significant positional deviation, and the experimental measurement accuracy can reach 26 cm with good stability [34]. Zhang and Jin proposed a BP neural network indoor positioning algorithm based on VLC. Through simulation experiments, the authors showed that the algorithm has a lower relative positioning error and better indoor positioning accuracy than the MDS-MAP and MDS-MAP(P) algorithms [35].
However, the BPNN is sensitive to random weights and thresholds, which enhances the likelihood of being trapped in nearby minima, thus generating significant localization errors [36]. Therefore, numerous optimization algorithms have been proposed to reduce the downsides of the BPNN. Among them, population intelligence optimization algorithms are the most widely applied algorithms toward solving the inherent defects of traditional training algorithms [37]. For example, a location fusion method of genetic algorithm (GA) optimization of the BP neural network that effectively reduces the localization error was proposed by Yang et al. [38]. Li et al. [39] proposed a mixed model that combines the cuckoo search optimization algorithm (CS) with the BPNN, where the random weights and thresholds of the BPNN are optimally trained, and the measured time differences are corrected after training. Finally, the Chan algorithm is used for localization calculation according to the corrected time difference values. In addition to these studies, the current swarm intelligence algorithm is developing rapidly, and better results have been demonstrated [40,41,42,43,44]. Previous literature has investigated indoor localization methods using the particle swarm optimization algorithm and BPNN hybrid algorithm (PSO-BP) combined with RFID using artificial intelligence techniques [45] and UWB indoor localization methods using the immune algorithm and BPNN hybrid algorithm (IA-BP) [46]. The above research shows that artificial intelligence techniques show strong capability in UWB positioning. It is essential to study new artificial intelligence techniques and prediction models to improve UWB positioning accuracy [47].
As indicated by the no free lunch (NFL) theorem [48], theoretically, a certain algorithm cannot be treated as a generic global optimal optimization algorithm. Therefore, although a range of algorithms is used to optimize UWB indoor positioning, the presented algorithms are far from being able to solve all problems or be applied to all indoor environments. To the authors' best knowledge, UWB indoor localization has not yet been attempted using a combination of the HHO algorithm and BPNN.
This study proposes a new hybrid algorithm, called HHO-BP, to improve UWB indoor positioning accuracy and resistance to non-line-of-sight ranges. The algorithm uses the HHO algorithm to optimize the BPNN to find a set of optimal weights and thresholds, thereby improving the positioning accuracy. Finally, the optimization performance of the HHO-BP algorithm and the conventional BPNN are compared and analysed through experimental validation.
The major contribution of this paper is to propose a new hybrid algorithm, HHO-BP, for optimizing ultrawideband indoor positioning, outlined as follows:
(1) A new algorithm for hybrid localization, namely, HHO-BP, is proposed.
(2) Combining the BP neural network with an intelligent population algorithm, the structure of the BP neural network is optimized, thus solving the local optimal solution problem.
(3) The algorithm greatly improves the accuracy and anti-jamming capability of ultrawideband indoor positioning, with centimetre-level accuracy for both line-of-sight and non-line-of-sight positioning.
The structure of the paper is as follows: First, the principles of UWB localization and BPNN are explained in Section 2. Section 3 introduces the principle of the proposed swarm intelligence algorithm (HHO), as well as the constructed new model. Section 4 offers the findings as well as a discussion and analysis. Finally, in Section 5, the study's findings are presented.
The principle of the trilateral positioning algorithm is as follows: The three base stations are located at A0(x1, y1), A1(x2, y2) and A2(x3, y3), and it is known that the distance values of the tag to be measured, Tn(x0, y0), to the 3 base stations are d1, d2 and d3, as displayed in Figure 1.
In the case of two-dimensional positioning, according to the Pythagorean theorem, the formula for calculating the position of the tag, Tn, and the focus are attained using Eq (1).
d1=√(x1−x0)2+(y1−y0)2d2=√(x2−x0)2+(y2−y0)2d3=√(x3−x0)2+(y3−y0)2 | (1) |
Under ideal circumstances, the distance values of tag Tn to base Stations A0, A1 and A2 are accurate; tag Tn has only one correct solution, as shown by the red dot in Figure 2.
Under actual circumstances, real distance measurement is subject to error; the reasons for the error include the error of the range itself and the error of the actual scene, such as obstruction or metal, which affects the penetration and transmission of electromagnetic waves. In a line-of-sight environment, the measured value fluctuates above and below the actual value; however, in a non-line-of-sight environment, the distance measured is theoretically higher than the actual value due to the presence of occlusion between the base station and the tag, resulting in the effect shown below, where the solved Tn coordinate is no longer a point. Nevertheless, it is a region, so we need to find an optimal solution in the red area, as shown in Figure 3.
There are many models of artificial neural networks, but the foremost broadly utilized, natural and simple-to-understand is the backpropagation algorithm, called BPNN [49]. Training is performed through signal forward propagation and error backward propagation.
The network generally has a multilayer structure, including an input layer for data input, an output layer for result output and several hidden layers, and each hidden layer contains one or more neurons. The topological diagram of the BPNN is shown in Figure 4.
In the structure, x1, x2, …, xm are inputs, and y1, y2, …, yn are outputs. A hidden layer contains s neurons, the output function of the hidden layer is bj, and the threshold values for the hidden and output layers are θj and θk, respectively. The most frequently used activation function is the sigmoid function, which is set to S(x) here. f1 and f2 are the transfer functions of the hidden and output layers, respectively. The output of the model is set to yk, and the expected output is tk [50]. The above can be modelled as follows.
The output of the Jth neuron of the hidden layer is [50]
bj=f1(m∑i=1wijxi−θj) (i=1,2,...,m;j=1,2,...,s). | (2) |
a. Calculate the output yk of the output layer as in Eq (3) [50]:
yk=f2(s∑i=1wjkbj−θk) (j=1,2,...,s;k=1,2,...,n). | (3) |
b. Define the error function by the actual output of the network as in Eq (4) [50]:
e=n∑k=1(tk−yk)2. | (4) |
c. The sigmoid activation function is given as Eq (5) [50]:
S(x)=11+e−x. | (5) |
A new bionic intelligent optimization approach, the Harris hawks optimization (HHO) algorithm, was developed in 2019. The method is a metaheuristic approach that combines Lévy flights to solve large multidimensional problems by simulating the collaborative group behaviour of Harris hawks while hunting. Compared with previous algorithms, this algorithm has a higher search capability, acceptable development and exploration and thus has been successfully applied to many practical engineering problems [51,52].
As a result, since BPNN is more sensitive to initial weights and thresholds, this research proposes the Harris hawks optimization method for BPNN random weights and threshold optimization.
The whole optimization process includes a global exploration phase, a transition phase and a local exploitation phase [52].
1) Exploration phase:
In HHO, the Harris hawks perch randomly on some locations in a waiting form, monitoring the area [lb, ub] to search for prey. The prey is simultaneously searched at random locations according to two different strategies, and the location update is performed with probability q during the iteration. The mathematical formula for the Harris hawks's location, which is closest to the prey, is the following:
X(t+1)={Xrand(t)−r1⋅|Xrand(t)−2⋅r2⋅X(t)|,q≥0.5(Xrabbit(t)−Xb(t))−r3⋅(lb+r4⋅(ub−lb)),q<0.5. | (6) |
In Eq (6), X(t+1) is the hawk's location in the following iteration, t. The location of the rabbit is Xrabbit(t), and the current position of the hawk is X(t). r1, r2, r3, r4 and q are all random numbers ranging from 0 to 1. The upper and lower bounds of the variables are represented by ub and lb, respectively, while Xrand(t) is the hawk chosen at random from the population, which is updated in each iteration. Xb(t) is the current hawk average location in Eq (7).
Xb(t)=1NN∑i=1Xi(t) |
where N is the number of hawk, and in iteration t, Xi(t) shows the location of each hawk.
2) Transition phase:
The HHO algorithm moves from exploration to exploitation and then between multiple exploitation behaviours depending on the energy, E, of prey escape, as shown below in Eq (8):
E=2E0(1−tT) |
where E0 is the energy starting stage, and T is the highest number of iterations [52].
3) Exploitation phase:
Four distinct tactics are employed to recreate the assault in this phase, depending on how the prey flees and how the Harris hawk pursues it, combined with Lévy flights, representing the prey's chance of escaping [52].
a)
r⩾0.5&|E|⩾0.5 |
In this case, the rabbit still has enough energy, and the hawk aim to exhaust its energy before launching the assault. The modelling rules given in Eqs (9) and (10) [52]:
X(t+1)=ΔX(t)−E|JXrabbit(t)−X(t)|, |
ΔX(t)=Xrabbit(t)−X(t). |
To replicate the nature of the rabbit's movements, the J value varies randomly in each iteration, and the rabbit's random hop intensity throughout the escape phase is denoted by J=2(1−r5). r5 is a number in the range from 0 to 1. The disparities between the rabbit's prior location vector and the current position vector are ΔX(t).
b)
r⩾0.5&|E|<0.5 |
Under this condition, the rabbit is fatigued, and the hawk can launch a surprise assault. Equation (11) expresses how the model updates the rabbit's position [52]:
X(t+1)=Xrabbit(t)−E|ΔX(t)|. | (11) |
c)
r<0.5&|E|⩾0.5 |
The rabbit has enough energy in this situation to effectively flee while still constructing a soft envelope before the attack. The following rule, given in Eq (12), can be used to update the Harris hawks' positions [52]:
Y=Xrabbit(t)−E|JXrabbit(t)−X(t)|. | (12) |
The hawk will dive utilizing the following rule, Eq (13), using LF-based patterns:
Z=Y+S×LF(D) |
where D is the dimension, S is a one-dimensional random vector, and LF is the Levy flight function and is based on the equation below (Eq (14)).
LF(x)=0.01×μ×σ|ν|1β,σ=(Γ(1+β)×sin(πβ2)Γ(1+β2)×β×2(β−12))1β | (14) |
Here, β is 1.5, and μ and ν are random numbers between 0 and 1.
By calculation, Eq (15) can execute the ultimate plan to update the locations of the hawks:
X(t+1)={Y F(Y) < F(H(t))Z F(Z) < F(H(t)). | (15) |
d)
r<0.5&|E|<0.5 |
In this situation, the rabbit is unable to flee due to a lack of energy, so the hawks set up a hard siege. The modelling rules are given in Eqs (16) and (17) [52]:
Y =Xrabbit(t)−E|JXrabbit(t)−Xb(t)|, | (16) |
Z =Y+S×LF(D). | (17) |
Here, Y references Eq (15).
Figure 5 depicts the development path of the HHO-BP model. First, the obtained database is divided into training and test datasets. The BP neural network is first set to random weights and thresholds, and then the training set data are input for training, where the Harris hawk optimization algorithm develops the final BP model by searching for the best combination of weights and thresholds in the BP model in a preset number of iterations. Finally, the test set data are fed into the final constructed BP neural network for predicting the UWB localization results. Performance metrics such as the mean error and position comparison can be used to evaluate the optimized BP model, and the actual and measured coordinates of the test set and the prediction results are used to test its performance and calculate the prediction error.
The computational complexity of HHO in this approach is determined using three key processes: initial optimization, fitness evaluation and candidate update. The computing complexity of the initialization process is O(N) for all N. The update mechanism has a computational complexity of O(T×N)+O(T×N×D), which comprises updating the search agent location and finding the optimal solution, where T is the maximum number of iterations, set to 200 to assure convergence, and D is the problem's dimensionality. As a result, HHO's computational complexity is O(N×(T+TD+1)) [52].
Through the following site environment and hardware equipment, UWB measurement data are acquired. Then, they are compared with the actual position, and visualized positioning points and error curves are plotted to see if the algorithm pair is effective and accurate.
To test the accuracy of UWB localization and the algorithm's efficiency, a combination of offline data acquisition and MATLAB simulation is adopted. For the acquisition, an LOS environment and an NLOS environment were created, and the experimental sites are shown in Figures 6 and 7.
LOS environment: To create an indoor positioning scenario, four UWB anchors with heights of 2.2 m and positions of (0, 0), (0, 6.45), (5.58, 0) and (5.58, 6.45) were placed in the region illustrated in Figure 6. UWB anchor 0 is connected via Wi-Fi to a computer with the developed interactive interface installed, and the real-time position of the UWB tag is obtained through the host computer software to obtain indoor positioning data for the LOS environment.
NLOS environment: Because of the indoor environment's complexity, the height of the four UWB anchors was reduced to 1.4 m, and the positioning test points were built around the table using the shelter of the table to build a non-line-of-sight testing environment, as shown in Figure 7.
The DWM1000 UWB module used in the range circuit is made by DECAWAVE. The Wi-Fi is used to communicate with the PC to obtain UWB measurement data. Figure 8 depicts the system structure and hardware circuit diagram [53].
The positioning data were collected separately at the same height at the site. Four label heights were tested (1.3, 1.55, 1.7 and 1.95 m) to ensure the feasibility of experimental rigor. The actual locations and the measured locations were used to form the sample set, which was divided into the training and test sets.
In Figure 9, the four panels a-d represent the total dataset of the four altitude UWB measurements and the actual coordinate positions. The black asterisks represent the actual coordinate positions measured with the metric ruler; the red diamonds represent the upper computer positioning software data. The data in the graph are all shifted by different magnitudes. To verify the effectiveness and accuracy of the algorithm, the simulation results were subsequently compared with the redraw data. Additionally, to better analyse the error of the original UWB positioning, the error curve is plotted using MATLAB 2020 software, as shown in Figure 10.
As seen from Figure 10, for different altitudes, there are positioning anomalies, and some points do not achieve centimetre-level positioning accuracy. In contrast, some positions are more volatile in comparison. In other words, conventional UWB positioning either has large fluctuations or large deviations. For this purpose, from the aforementioned sample set, a training dataset and a testing dataset are constructed, which are fed into the BPNN and the HHO-BP neural network, which predicts the localization sites. Finally, the error analysis is performed with the actual coordinate points. To analyse the effect of the algorithm, we choose nonidentical locus points as the test set.
The black points in Figure 11 are the actual locations (i.e., the metric measurement data). The red points are the original UWB positioning measurement data. As the figure shows, the initial UWB positioning is not ideal, and there are significant errors.
In Figure 12, the black points are the actual coordinates (measured in metres), the blue triangles are the unoptimized BP neural network (random weight threshold) localization points, and the red circles are the HHO-BP neural network localization points.
When comparing Figures 11 and 12, the findings reveal that both the BPNN and the HHO-BP have greatly increased accuracy. To more accurately evaluate the difference in effectiveness between the BPNN and the optimized HHO-BP, each test point was compared with the actual location, and three sets of error data were obtained. The initial UWB measurement error, the BPNN prediction result error, and the HHO-BP prediction result error were all identified, as demonstrated in Figures 13 and 14.
In Figure 13, the black line segment indicates the original UWB error, the blue line segment shows the BP neural network optimization error, and the red line segment indicates the HHO-BP neural network optimization error.
The experimental findings in Figures 13 and 14 reveal that the HHO-BP algorithm has a superior optimization impact relative to the BP neural algorithm at all four heights of the LOS environment, which effectively reduces the error of UWB indoor positioning and achieves centimetre-level accuracy. At the same time, HHO-BP can effectively decrease outliers and reduce accuracy variation to a certain amount.
In this environment, to achieve the NLOS environmental conditions, four UWB anchors were adjusted to a height of 1.4 m, and the test points were located around the table with the table positioned as an occluder. The localization coordinates of the UWB tag heights of 0.24, 0.4, 0.55 and 0.67 m were measured separately as the sample set for this environment. From the aforementioned sample set, a training dataset and a testing dataset were constructed.
From Figure 15, from sample sets of different heights, it can be found that in the NLOS environment, the outliers of sample points increase significantly. Because of the shielding effect of the table, the signal's arrival time increases, such that the measured coordinates deviate from the shielding object. As the height of the UWB tag decreases gradually, the complexity of the NLOS environment increases, and the location deviation becomes more chaotic, causing the optimization task of this environment to be more difficult.
Figure 16 shows the error curves of the sample set for each altitude measurement versus the actual coordinates, indicating a significant increase in error in the NLOS environment, along with greater volatility. Figure 16 shows that UWB positioning has a large error in the NLOS environment, with some test points even showing an error of more than 1.5 m. Therefore, optimization for this situation is essential.
Comparing Figures 17 and 18, the results reveal that under the NLOS setting, the accuracy of the BPNN and the HHO-BP has improved significantly. To more accurately evaluate the difference in effectiveness between the BPNN and the optimized HHO-BP, the UWB measurement error is compared to the error of each point in the testing dataset, as shown in Figures 19 and 20.
Figures 19 and 20 show that HHO-BP effectively reduces errors and improves positioning accuracy at all altitudes in the complex NLOS environment.
BP | HHO-BP | ||||||
Environment | H | Max E | Min E | ME | Max E | Min E | ME |
LOS | 130 | 17.63 | 0.97 | 8.63 | 11.78 | 0.21 | 5.31 |
155 | 14.77 | 2.99 | 7.39 | 10.38 | 0.50 | 4.94 | |
170 | 9.22 | 2.68 | 5.15 | 7.72 | 1.74 | 4.18 | |
195 | 11.14 | 4.77 | 4.89 | 6.78 | 2.05 | 3.56 | |
NLOS | 24 | 48.60 | 4.25 | 18.19 | 28.82 | 3.46 | 13.75 |
40 | 30.12 | 3.45 | 11.65 | 19.57 | 1.47 | 6.65 | |
55 | 71.08 | 3.90 | 20.41 | 61.10 | 2.24 | 12.45 | |
67 | 19.35 | 1.53 | 9.04 | 13.25 | 0.87 | 5.41 | |
Note: H: Height, Max E: Maximum Error, Min E: Minimum Error, ME: Mean Error. |
Table 1 indicates the maximum errors, minimum errors and mean errors of all test data. These details are obtained from the graph above. Here, the mean error is counted separately for each height result. According to experimental data, the optimization of typical BP neural networks is not ideal, especially in nonvisual range environments, and rarely has centimetre-level accuracy.
UWB | BP | HHO-BP | ||||
Environment | H | ME | ME | O P | ME | O P |
LOS | 130 | 9.15 | 8.63 | 5.70 | 5.31 | 41.99 |
155 | 8.13 | 7.39 | 9.08 | 4.94 | 39.23 | |
170 | 8.22 | 5.15 | 37.29 | 4.18 | 49.07 | |
195 | 11.11 | 4.89 | 55.91 | 3.56 | 67.95 | |
NLOS | 24 | 27.68 | 18.19 | 34.28 | 13.75 | 50.33 |
40 | 34.80 | 11.65 | 66.52 | 6.65 | 80.90 | |
55 | 38.05 | 20.41 | 46.36 | 12.45 | 67.28 | |
67 | 19.30 | 9.04 | 53.15 | 5.41 | 71.97 | |
Note: H: Height (cm), ME: Mean Error (cm); O P: Optimization percentage (%). |
Table 2 uses the statistical mean error for each height and further calculates the optimization percentages of the conventional BPNN and the HHO-BP algorithm. The mathematical formula is as follows in Eq (18):
YHP=(YU−YH)÷YU×100%YBP=(YU−YB)÷YU×100% | (18) |
where YHP is the optimization percentage of the HHO-BP algorithm, YBP is the optimization percentage of the conventional BPNN, YU is the mean error of the UWB measurement, YH is the mean error of the HHO-BP algorithm, and YB is the mean error of the conventional BPNN.
According to Table 2, the HHO-BP algorithm outperforms the optimization results of the conventional BPNN at every height, both for the LOS and NLOS distances. In addition, for the HHO-BP algorithm in non-line-of-sight distance conditions, compared with UWB measurement data, the degree of optimization is more than 50%, and the effect is better than the line-of-sight distance environment. For 130 cm data, the conventional BP neural network is only optimized by 5.70%, while HHO-BP performs 36.29% better than the conventional BP neural network.
UWB | BP | HHO-BP | HHO-BP greater than BP | |||
Environment | MTE | MTE | O P | MTE | O P | O P |
LOS | 9.15 | 6.52 | 26.99 | 4.50 | 49.56 | 22.57 |
NLOS | 29.96 | 14.82 | 50.08 | 9.56 | 67.62 | 17.54 |
Note: MTE: Mean total error (cm), O P: Optimization percentage (%). |
Table 3 shows the total mean errors for LOS and NLOS and the optimization percentages. Here, the mean errors in Tables 1 and 2 are averaged to obtain the total mean error of the corresponding environment. According to the data in Table 3, the HHO-BP algorithm achieves centimetre-level positioning accuracy in both LOS and NLOS environments. Under line-of-sight conditions, the HHO-BP algorithm improves the localization accuracy by 22.57% compared to the conventional BP algorithm; under non-line-of-sight conditions, the HHO-BP algorithm improves the localization accuracy by 17.54% in comparison to the conventional BPNN.
In this study, an intelligent group algorithm (HHO) combined with a BP neural network is proposed to calibrate UWB indoor locations. First, the original and UWB coordinates are collected to obtain the actual errors. Next, BPNN training is used to generate the relevant weights and thresholds. Then, the HHO method optimizes the weights and thresholds of the BPNN until the minimum error is achieved. Finally, a new BPNN is constructed to calibrate the UWB measurement coordinates. The original BPNN and HHO-BP errors are compared. The experimental results show that, to some extent, BPNN calibration of the UWB indoor location will fall into the optimal local solution and that the HHO-BP method has higher accuracy. Finally, the proposed HHO-BP method is applied to indoor NLOS environments for calibrating indoor positioning environments with larger errors. The findings demonstrate that the HHO-BP algorithm's overall positioning error in an LOS environment is approximately 4.5 cm, which is 22.57% higher than the conventional BPNN, and for 130 cm data, the maximum improvement is 36.29% compared to the conventional BPNN. In an NLOS scenario, the HHO-BP algorithm has a positioning error of approximately 9.6 cm, which is 17.54% better than the conventional BPNN method. Experiments have confirmed the accuracy and efficacy of the suggested indoor positioning technique, indicating that it has greater calibration precision and stability, as well as strong future application possibilities.
CXH conceived the experimental study methodology, participated in the design and drafted the manuscript. FMS and LZY conducted the research and helped refine the experimental ideas. JCC provided technical support and specific directions for the algorithms and helped revise the manuscript. LY collected the data and analysed the results. All authors read and approved the final manuscript.
The datasets used and analysed during the current study are available from the corresponding author upon reasonable request.
We are very grateful to the Robotics Research Laboratory of Shandong University of Science and Technology for providing an experimental site for our research. This work was supported by the Natural Science Research Project of Universities in Anhui Province (NO. KJ2021A0953), Natural Science Key Scientific Research Project of West Anhui University (NO. 0041021003, WXZR201903), Key Research Programs of Shandong Province (NO. 2018GGX103011) and Key Science and Technology Innovation Programs in Shandong Province (NO. 2017CXGC0919).
There are no conflicts of interest declared by the authors.
[1] |
G. W. Dick, S. F. Kitchen, A. J. Haddow, Zika virus. I. isolations and serological specificity, Trans. R. Soc. Trop. Med. Hyg., 46 (1952), 509–520. https://doi.org/10.1016/0035-9203(52)90042-4 doi: 10.1016/0035-9203(52)90042-4
![]() |
[2] |
G. W. Dick, S. F. Kitchen, A. J. Haddow, Zika virus. II. pathogenicity and physical properties, Trans. R. Soc. Trop. Med. Hyg., 46 (1952), 521–534. https://doi.org/10.1016/0035-9203(52)90043-6 doi: 10.1016/0035-9203(52)90043-6
![]() |
[3] |
M. R. Duffy, T. H. Chen, W. T. Hancock, A. M. Powers, J. L. Kool, R. S. Lanciotti, Zika virus outbreak on Yap Island, federated states of Micronesia, N. Engl. J. Med., 360 (2009), 2536–2543. https://doi.org/10.1056/NEJMoa0805715 doi: 10.1056/NEJMoa0805715
![]() |
[4] |
S. Ioos, H. P. Mallet, I. L. Goffart, V. Gauthier, T. Cardoso, M. Herida, Current Zika virus epidemiology and recent epidemics, Med. Mal. Infect., 44 (2014), 302–307. https://doi.org/10.1016/j.medmal.2014.04.008 doi: 10.1016/j.medmal.2014.04.008
![]() |
[5] | V. M. C. Lormeau, C. Roche, A. Teissier, E. Robin, A. Berry, H. Mallet, et al., Zika virus, French Polynesia, South Pacific, 2013, Emerg. Infect. Dis., 20 (2014), 1085–1086. https://doi.org/10.3201/eid2006.140138 |
[6] |
C. Zanluca, V. C. A. Melo, A. L. P. Mosimann, G. I. V. Santos, C. N. D. Santos, K. Luz, First report of autochthonous transmission of Zika virus in Brazil, Inst. Oswaldo Cruz., 110 (2015), 569–572. https://doi.org/10.1590/0074-02760150192 doi: 10.1590/0074-02760150192
![]() |
[7] |
D. Gatherer, A. Kohl, Zika virus: a previously slow pandemic spreads rapidly through the Americas, J. Gen. Virol., 97 (2016), 269–273. https://doi.org/10.1099/jgv.0.000381 doi: 10.1099/jgv.0.000381
![]() |
[8] |
J. P. Messina, M. Kraemer, O. J. Brady, D. M. Pigott, F. M. Shearer, D. J. Weiss, et al., Mapping global environmental suitability for Zika virus, elife, 5 (2016), e15272. https://doi.org/10.7554/eLife.15272 doi: 10.7554/eLife.15272
![]() |
[9] | V. L. P. Junior, K. Luz, R. Parreira, P. Ferrinho, Zika virus: a review to clinicians, Acta Med. Port., 28 (2015), 760–765. |
[10] |
R. Becker, Missing link: Animal models to study whether Zika causes birth defects, Nat. Med., 22 (2016), 225–227. https://doi.org/10.1038/nm0316-225 doi: 10.1038/nm0316-225
![]() |
[11] |
L. Bouzid, O. Belhamiti, Effect of seasonal changes on predictive model of bovine babesiosis transmission, Int. J. Model. Simul. Sci. Comput., 8 (2017), 1–17. https://doi.org/10.1142/S1793962317500301 doi: 10.1142/S1793962317500301
![]() |
[12] | E. B. Hayes, Zika virus outside Africa, Emerg. Infect. Dis. 15 (2009), 1347–1350. https://doi.org/10.3201/eid1509.090442 |
[13] |
M. H. Maamar, L. Bouzid, O. Belhamiti, F. B. M. Belgacem, Stability and numerical study of theoretical model of Zika virus transmission, Int. J. Math. Modell. Numer. Optim., 10 (2020), 141–166. https://doi.org/10.1504/IJMMNO.2020.106528 doi: 10.1504/IJMMNO.2020.106528
![]() |
[14] | F. L. H. Wertheim, P. Horby, J. P. Woodall, Atlas of Human Infectious Diseases, Wiley-Blackwell, Oxford, 2012. |
[15] |
W. O. Kermack, A. G. McKendrick, A contribution to the mathematical theory of epidemics, Proc. R. Soc. London, Ser. A, 115 (1927), 700–721. https://doi.org/10.1098/rspa.1927.0118 doi: 10.1098/rspa.1927.0118
![]() |
[16] | C. Manore, M. Hyman, Mathematical models for fighting Zika virus, SIAM News, 49 (2016). |
[17] |
D. Gao, Y. Lou, D. He, T. C. Porco, Y. Kuang, G. Chowell, et al., Prevention and control of Zika as a mosquito-borne and sexually transmitted disease: a mathematical modeling analysis, Sci. Rep., 6 (2016), 28070. https://doi.org/10.1038/srep28070 doi: 10.1038/srep28070
![]() |
[18] | E. K. Lee, Y. Liu, F. H. Pietz, A compartmental model for Zika virus with dynamic human and vector populations, AMIA Annu. Symp. Proc., 2016 (2011), 743–752. |
[19] |
H. Nishiura, R. Kinoshita, K. Mizumoto, Y. Yasuda, K. Nah, Transmission potential of Zika virus infection in the south pacific, Int. J. Infect. Dis., 45 (2016), 95–97. https://doi.org/10.1016/j.ijid.2016.02.017 doi: 10.1016/j.ijid.2016.02.017
![]() |
[20] |
S. Rezapour, H. Mohammadi, M. E. Samei, SEIR epidemic model for COVID-19 transmission by Caputo derivative of fractional order, Adv. Differ. Equations, 2020 (2020), 1–19. https://doi.org/10.1186/s13662-020-02952-y doi: 10.1186/s13662-020-02952-y
![]() |
[21] |
S. Ahmad, M. Rahman, M. Arfan, On the analysis of semi-analytical solutions of Hepatitis B epidemic model under the Caputo-Fabrizio operator, Chaos, Solitons Fractals, 146 (2021), 110892. https://doi.org/10.1016/j.chaos.2021.110892 doi: 10.1016/j.chaos.2021.110892
![]() |
[22] |
M. A. Taneco-Hernández, C. Vargas-De-León, Stability and Lyapunov functions for systems with Atangana-Baleanu Caputo derivative: an HIV/AIDS epidemic model, Chaos, Solitons Fractals, 132 (2020), 109586. https://doi.org/10.1016/j.chaos.2019.109586 doi: 10.1016/j.chaos.2019.109586
![]() |
[23] |
S. Qureshi, R. Jan, Modeling of measles epidemic with optimized fractional order under Caputo differential operator, Chaos, Solitons Fractals, 145 (2021), 110766. https://doi.org/10.1016/j.chaos.2021.110766 doi: 10.1016/j.chaos.2021.110766
![]() |
[24] |
W. Wang, M. Zhou, T. Zhang, Z. Feng, Dynamics of a Zika virus transmission model with seasonality and periodic delays, Commun. Nonl. Sci. Numer. Simul., 116 (2023), 106830. https://doi.org/10.1016/j.cnsns.2022.106830 doi: 10.1016/j.cnsns.2022.106830
![]() |
[25] |
M. A. Ibrahim, A. Dénes, A mathematical model for Zika Virus infection and microcephaly risk considering sexual and vertical transmission, Axioms, 12 (2023), 263. https://doi.org/10.3390/axioms12030263 doi: 10.3390/axioms12030263
![]() |
[26] |
M. Murugappan, R. Grienggarai, V. Govindan, Mathematical modelling on the transmission dynamics of Zika Virus, Int. J. Robot., Autom. Sci., 5 (2023), 79–84. https://doi.org/10.33093/ijoras.2023.5.2.9 doi: 10.33093/ijoras.2023.5.2.9
![]() |
[27] |
F. A. Oguntolu, O. J. Peter, A. Yusuf, B. I. Omede, G. Bolarin, T. A. Ayoola, Mathematical model and analysis of the soil-transmitted helminth infections with optimal control, Model. Earth Syst. Environm., 2023 (2023), 1–15. https://doi.org/10.1007/s40808-023-01815-1 doi: 10.1007/s40808-023-01815-1
![]() |
[28] |
O. J. Peter, H. S. Panigoro, A. Abidemi, M. M. Ojo, F.A. Oguntolu, Mathematical model of COVID-19 pandemic with double dose vaccination, Acta Biotheor., 71 (2023), 9. https://doi.org/10.1007/s10441-023-09460-y doi: 10.1007/s10441-023-09460-y
![]() |
[29] |
A. J. Kucharski, S. Funk, R. M. Eggo, H. P. Mallet, W. J. Edmunds, E. J. Nilles, Transmission dynamics of Zika virus in island populations: a modelling analysis of the 2013/14 French Polynesia outbreak, PLoS Negl. Trop. Dis., 10 (2016), e0004726. https://doi.org/10.1371/journal.pntd.0004726 doi: 10.1371/journal.pntd.0004726
![]() |
[30] |
J. P. T. Boorman, J. S. Porterfield, A simple technique for infection of mosquitoes with viruses, transmission of Zika virus, Trans. Roy. Soc. Trop. Med. Hyg., 50 (1956), 238–242. https://doi.org/10.1016/0035-9203(56)90029-3 doi: 10.1016/0035-9203(56)90029-3
![]() |
[31] |
M. Darwish, A seroepidemiological survey for bunyaviridae and certain other arboviruses in Pakistan, Trans. R. Soc. Trop. Med. Hyg., 77 (1983), 446–450. https://doi.org/10.1016/0035-9203(83)90108-6 doi: 10.1016/0035-9203(83)90108-6
![]() |
[32] | C. Castillo-Chávez, H. Thieme, Asymptotically autonomous epidemic models, in Mathematical Population Dynamics: Analysis of Heterogeneity, Wuerz Publishing, Winnipeg, (1995), 33–50. |
[33] | G. M. R. Costa, M. Lobosco, M. Ehrhardt, R. F. Reis, Mathematical analysis and a nonstandard scheme for a model of the immune response against COVID-19, in Mathematical and Computational Modeling of Phenomena Arising in Population Biology and Nonlinear Oscillations: In honour of the 80th birthday of Ronald E. Mickens, AMS Contemporary Mathematics, 2023. |
[34] |
K. Diethelm, A fractional calculus based model for the simulation of an outbreak of Dengue fever, Nonl. Dyn., 71 (2013), 613–619. https://doi.org/10.1007/s11071-012-0475-2 doi: 10.1007/s11071-012-0475-2
![]() |
[35] | H. K. Khalil, Nonlinear Systems, Prentice-Hall, London, UK, 1996. |
[36] | R. Gorenflo, A. A. Kilbas, F. Mainardi, S. V. Rogosin, Mittag-Leffler Functions, Related Topics and Applications, Springer, Berlin-Heidelberg, 2014. |
[37] |
C. Vargas-De-León, Volterra-type Lyapunov functions for fractional-order epidemic systems, Commun. Nonl. Sci. Numer. Simul., 24 (2015), 75–85. https://doi.org/10.1016/j.cnsns.2014.12.013 doi: 10.1016/j.cnsns.2014.12.013
![]() |
[38] | R. E. Mickens, Applications of Nonstandard Finite Difference Schemes, World Scientific, 2000. |
[39] |
M. M. Khalsaraei, Positivity of an explicit Runge–Kutta method, Ain Shams Eng. J., 6 (2015), 1217–1223. https://doi.org/10.1016/j.asej.2015.05.018 doi: 10.1016/j.asej.2015.05.018
![]() |
[40] |
A. Gerisch, R. Weiner, The positivity of low-order explicit Runge-Kutta schemes applied in splitting methods, Comput. Math. Appl., 45 (2003), 53–67. https://doi.org/10.1016/S0898-1221(03)80007-X doi: 10.1016/S0898-1221(03)80007-X
![]() |
[41] |
R. E. Mickens, Calculation of denominator functions for nonstandard finite difference schemes for differential equations satisfying a positivity condition, Numer. Methods Partial Differ. Equations, 23 (2007), 672–691. https://doi.org/10.1002/num.20198 doi: 10.1002/num.20198
![]() |
[42] |
S. Berkhahn, M. Ehrhardt, A physics-informed neural network to model COVID-19 infection and hospitalization scenarios, Adv. Contin. Discrete Models, 2022 (2022), 61. https://doi.org/10.1186/s13662-022-03733-5 doi: 10.1186/s13662-022-03733-5
![]() |
[43] |
S. Treibert, H. Brunner, M. Ehrhardt, A nonstandard finite difference scheme for the SVICDR model to predict COVID-19 dynamics, Math. Biosci. Eng., 19 (2022), 1213–1238. https://doi.org/10.3934/mbe.2022056 doi: 10.3934/mbe.2022056
![]() |
[44] | M. Ehrhardt, R. E. Mickens, A Nonstandard Finite Difference Scheme for Solving a Zika Virus Model, unpublished manuscript, 2017. |
[45] | D. Matignon, Stability results for fractional differential equations with applications to control processing, Comput. Eng. Syst. Appl., 2 (1996), 963–968. |
[46] |
A. Ali, S. Islam, M. R. Khan, S. Rasheed, F. M. Allehiany, J. Baili, et al., Dynamics of a fractional order Zika virus model with mutant, Alexandria Eng. J., 61 (2022), 4821–4836. https://doi.org/10.1016/j.aej.2021.10.031 doi: 10.1016/j.aej.2021.10.031
![]() |
[47] |
A. Ali, F. S. Alshammari, S. Islam, M. A. Khan, S. Ullah, Modeling and analysis of the dynamics of novel coronavirus (COVID-19) with Caputo fractional derivative, Res. Phys., 20 (2021), 103669. https://doi.org/10.1016/j.rinp.2020.103669 doi: 10.1016/j.rinp.2020.103669
![]() |
1. | Guo-zheng Quan, Yi-fan Zhao, Qi Deng, Ming-guo Quan, Wei Xiong, Flow Stress Models for 40Cr10Si2Mo Steel and Their Application in Numerical Simulation of Hot Forming, 2024, 1059-9495, 10.1007/s11665-024-10024-5 | |
2. | Feiyun Cong, Zongheng Hong, Xiaojie Lin, Huimin Li, Peak Ratio Iteration-Based Leading-Edge Detection Algorithm in UWB Localization, 2023, 131, 0929-6212, 1663, 10.1007/s11277-023-10517-x | |
3. | Jia Xu, Teng Xiao, Pin Lv, Zhe Chen, Chao Cai, Yang Zhang, Zehui Xiong, Tracing Human Stress From Physiological Signals Using UWB Radar, 2024, 11, 2327-4662, 32773, 10.1109/JIOT.2024.3410033 | |
4. | Qingxi Zeng, Yu Jin, Haonan Yu, Xia You, A UAV Localization System Based on Double UWB Tags and IMU for Landing Platform, 2023, 23, 1530-437X, 10100, 10.1109/JSEN.2023.3260311 | |
5. | Dansong Yue, Shuqi Shang, Kai Feng, Haiqing Wang, Xiaoning He, Zelong Zhao, Ning Zhang, Baiqiang Zuo, Dongwei Wang, Research on the Model of a Navigation and Positioning Algorithm for Agricultural Machinery Based on the IABC-BP Network, 2023, 13, 2077-0472, 1769, 10.3390/agriculture13091769 |
BP | HHO-BP | ||||||
Environment | H | Max E | Min E | ME | Max E | Min E | ME |
LOS | 130 | 17.63 | 0.97 | 8.63 | 11.78 | 0.21 | 5.31 |
155 | 14.77 | 2.99 | 7.39 | 10.38 | 0.50 | 4.94 | |
170 | 9.22 | 2.68 | 5.15 | 7.72 | 1.74 | 4.18 | |
195 | 11.14 | 4.77 | 4.89 | 6.78 | 2.05 | 3.56 | |
NLOS | 24 | 48.60 | 4.25 | 18.19 | 28.82 | 3.46 | 13.75 |
40 | 30.12 | 3.45 | 11.65 | 19.57 | 1.47 | 6.65 | |
55 | 71.08 | 3.90 | 20.41 | 61.10 | 2.24 | 12.45 | |
67 | 19.35 | 1.53 | 9.04 | 13.25 | 0.87 | 5.41 | |
Note: H: Height, Max E: Maximum Error, Min E: Minimum Error, ME: Mean Error. |
UWB | BP | HHO-BP | ||||
Environment | H | ME | ME | O P | ME | O P |
LOS | 130 | 9.15 | 8.63 | 5.70 | 5.31 | 41.99 |
155 | 8.13 | 7.39 | 9.08 | 4.94 | 39.23 | |
170 | 8.22 | 5.15 | 37.29 | 4.18 | 49.07 | |
195 | 11.11 | 4.89 | 55.91 | 3.56 | 67.95 | |
NLOS | 24 | 27.68 | 18.19 | 34.28 | 13.75 | 50.33 |
40 | 34.80 | 11.65 | 66.52 | 6.65 | 80.90 | |
55 | 38.05 | 20.41 | 46.36 | 12.45 | 67.28 | |
67 | 19.30 | 9.04 | 53.15 | 5.41 | 71.97 | |
Note: H: Height (cm), ME: Mean Error (cm); O P: Optimization percentage (%). |
UWB | BP | HHO-BP | HHO-BP greater than BP | |||
Environment | MTE | MTE | O P | MTE | O P | O P |
LOS | 9.15 | 6.52 | 26.99 | 4.50 | 49.56 | 22.57 |
NLOS | 29.96 | 14.82 | 50.08 | 9.56 | 67.62 | 17.54 |
Note: MTE: Mean total error (cm), O P: Optimization percentage (%). |
BP | HHO-BP | ||||||
Environment | H | Max E | Min E | ME | Max E | Min E | ME |
LOS | 130 | 17.63 | 0.97 | 8.63 | 11.78 | 0.21 | 5.31 |
155 | 14.77 | 2.99 | 7.39 | 10.38 | 0.50 | 4.94 | |
170 | 9.22 | 2.68 | 5.15 | 7.72 | 1.74 | 4.18 | |
195 | 11.14 | 4.77 | 4.89 | 6.78 | 2.05 | 3.56 | |
NLOS | 24 | 48.60 | 4.25 | 18.19 | 28.82 | 3.46 | 13.75 |
40 | 30.12 | 3.45 | 11.65 | 19.57 | 1.47 | 6.65 | |
55 | 71.08 | 3.90 | 20.41 | 61.10 | 2.24 | 12.45 | |
67 | 19.35 | 1.53 | 9.04 | 13.25 | 0.87 | 5.41 | |
Note: H: Height, Max E: Maximum Error, Min E: Minimum Error, ME: Mean Error. |
UWB | BP | HHO-BP | ||||
Environment | H | ME | ME | O P | ME | O P |
LOS | 130 | 9.15 | 8.63 | 5.70 | 5.31 | 41.99 |
155 | 8.13 | 7.39 | 9.08 | 4.94 | 39.23 | |
170 | 8.22 | 5.15 | 37.29 | 4.18 | 49.07 | |
195 | 11.11 | 4.89 | 55.91 | 3.56 | 67.95 | |
NLOS | 24 | 27.68 | 18.19 | 34.28 | 13.75 | 50.33 |
40 | 34.80 | 11.65 | 66.52 | 6.65 | 80.90 | |
55 | 38.05 | 20.41 | 46.36 | 12.45 | 67.28 | |
67 | 19.30 | 9.04 | 53.15 | 5.41 | 71.97 | |
Note: H: Height (cm), ME: Mean Error (cm); O P: Optimization percentage (%). |
UWB | BP | HHO-BP | HHO-BP greater than BP | |||
Environment | MTE | MTE | O P | MTE | O P | O P |
LOS | 9.15 | 6.52 | 26.99 | 4.50 | 49.56 | 22.57 |
NLOS | 29.96 | 14.82 | 50.08 | 9.56 | 67.62 | 17.54 |
Note: MTE: Mean total error (cm), O P: Optimization percentage (%). |