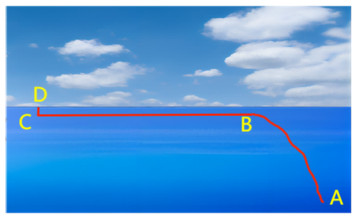
Autonomous Underwater Vehicle (AUV) works autonomously in complex marine environments. After a severe accident, an AUV will lose its power and rely on its small buoyancy to ascend at a slow speed. If the reserved buoyancy is insufficient, when reaching the thermocline, the buoyancy will rapidly decrease to zero. Consequently, the AUV will experience prolonged lateral drift within the thermocline. This study focuses on developing a prediction method for the drift trajectory of an AUV after a long-term power loss accident. The aim is to forecast the potential resurfacing location, providing technical support for surface search and salvage operations of the disabled AUV. To the best of our knowledge, currently, there is no mature and effective method for predicting long-term AUV underwater drift trajectories. In response to this issue, based on real AUV catastrophes, this paper studies the prediction of long-term AUV underwater drift trajectories in the cases of power loss. We propose a three-dimensional trajectory prediction method based on the Lagrange tracking approach. This method takes the AUV's longitudinal velocity, the time taken to reach different depths, and ocean current data at various depths into account. The reason for the AUV's failure to ascend to sea surface lies that the remaining buoyancy is too small to overcome the thermocline. As a result, AUV drifts long time within the thermocline. To address this issue, a method for estimating thermocline currents is proposed, which can be used to predict the lateral drift trajectory of the AUV within the thermocline. Simulation is conducted to compare the results obtained by the proposed method and that in a real accident. The results demonstrate that the proposed approach exhibits small directional and positional errors. This validates the effectiveness of the proposed method.
Citation: Shuwen Zheng, Mingjun Zhang, Jing Zhang, Jitao Li. Lagrange tracking-based long-term drift trajectory prediction method for Autonomous Underwater Vehicle[J]. Mathematical Biosciences and Engineering, 2023, 20(12): 21075-21097. doi: 10.3934/mbe.2023932
[1] | Kangsen Huang, Zimin Wang . Research on robust fuzzy logic sliding mode control of Two-DOF intelligent underwater manipulators. Mathematical Biosciences and Engineering, 2023, 20(9): 16279-16303. doi: 10.3934/mbe.2023727 |
[2] | Chengxi Wu, Yuewei Dai, Liang Shan, Zhiyu Zhu, Zhengtian Wu . Data-driven trajectory tracking control for autonomous underwater vehicle based on iterative extended state observer. Mathematical Biosciences and Engineering, 2022, 19(3): 3036-3055. doi: 10.3934/mbe.2022140 |
[3] | Xiaoqiang Dai, Hewei Xu, Hongchao Ma, Jianjun Ding, Qiang Lai . Dual closed loop AUV trajectory tracking control based on finite time and state observer. Mathematical Biosciences and Engineering, 2022, 19(11): 11086-11113. doi: 10.3934/mbe.2022517 |
[4] | Xiaoyong Xiong, Haitao Min, Yuanbin Yu, Pengyu Wang . Application improvement of A* algorithm in intelligent vehicle trajectory planning. Mathematical Biosciences and Engineering, 2021, 18(1): 1-21. doi: 10.3934/mbe.2021001 |
[5] | Yong Xiong, Lin Pan, Min Xiao, Han Xiao . Motion control and path optimization of intelligent AUV using fuzzy adaptive PID and improved genetic algorithm. Mathematical Biosciences and Engineering, 2023, 20(5): 9208-9245. doi: 10.3934/mbe.2023404 |
[6] | Wenyang Gan, Lixia Su, Zhenzhong Chu . A PSO-enhanced Gauss pseudospectral method to solve trajectory planning for autonomous underwater vehicles. Mathematical Biosciences and Engineering, 2023, 20(7): 11713-11731. doi: 10.3934/mbe.2023521 |
[7] | Xingjia Li, Jinan Gu, Zedong Huang, Wenbo Wang, Jing Li . Optimal design of model predictive controller based on transient search optimization applied to robotic manipulators. Mathematical Biosciences and Engineering, 2022, 19(9): 9371-9387. doi: 10.3934/mbe.2022436 |
[8] | Wenshun Sheng, Jiahui Shen, Qiming Huang, Zhixuan Liu, Zihao Ding . Multi-objective pedestrian tracking method based on YOLOv8 and improved DeepSORT. Mathematical Biosciences and Engineering, 2024, 21(2): 1791-1805. doi: 10.3934/mbe.2024077 |
[9] | Peng Lu, Ao Sun, Mingyu Xu, Zhenhua Wang, Zongsheng Zheng, Yating Xie, Wenjuan Wang . A time series image prediction method combining a CNN and LSTM and its application in typhoon track prediction. Mathematical Biosciences and Engineering, 2022, 19(12): 12260-12278. doi: 10.3934/mbe.2022571 |
[10] | Xinyi He, Xiuping Han, Tengda Wei, Xiaodi Li . Tracking control of wheeled mobile robots via intermittent control. Mathematical Biosciences and Engineering, 2024, 21(3): 3774-3783. doi: 10.3934/mbe.2024167 |
Autonomous Underwater Vehicle (AUV) works autonomously in complex marine environments. After a severe accident, an AUV will lose its power and rely on its small buoyancy to ascend at a slow speed. If the reserved buoyancy is insufficient, when reaching the thermocline, the buoyancy will rapidly decrease to zero. Consequently, the AUV will experience prolonged lateral drift within the thermocline. This study focuses on developing a prediction method for the drift trajectory of an AUV after a long-term power loss accident. The aim is to forecast the potential resurfacing location, providing technical support for surface search and salvage operations of the disabled AUV. To the best of our knowledge, currently, there is no mature and effective method for predicting long-term AUV underwater drift trajectories. In response to this issue, based on real AUV catastrophes, this paper studies the prediction of long-term AUV underwater drift trajectories in the cases of power loss. We propose a three-dimensional trajectory prediction method based on the Lagrange tracking approach. This method takes the AUV's longitudinal velocity, the time taken to reach different depths, and ocean current data at various depths into account. The reason for the AUV's failure to ascend to sea surface lies that the remaining buoyancy is too small to overcome the thermocline. As a result, AUV drifts long time within the thermocline. To address this issue, a method for estimating thermocline currents is proposed, which can be used to predict the lateral drift trajectory of the AUV within the thermocline. Simulation is conducted to compare the results obtained by the proposed method and that in a real accident. The results demonstrate that the proposed approach exhibits small directional and positional errors. This validates the effectiveness of the proposed method.
This paper studies the prediction of underwater drift trajectory when an Autonomous Underwater Vehicle (AUV) loses its power in an accident. An AUV operates autonomously in complex marine environments without cables, making it susceptible to catastrophic accidents, such as loss incidents [1]. Safety is a critical concern in AUV research and application [1]. Regarding AUV safety technology, ocean current research mainly focuses on fault control and safety design [2,3]. This includes research on AUV status online monitoring, fault diagnosis, fault-tolerant control and redundant load shedding, which has yielded significant research results [4,5]. These results strongly support the advancement of AUV technology and accelerate its practical application process. On another hand, in the event of a catastrophic accident involving an AUV, studying its drift trajectory and predicting its exit point for timely search and recovery are of great importance for later investigations into the causes of faults and potential solutions, which motivate our work.
The author's research team encountered a significant accident during the sea trial of an AUV, where it lost contact and resurfaced after 100 hours. Based on this incident, this article investigates the method for predicting the AUV's drift trajectory.
Currently, there is limited literature available on AUV drift trajectory prediction methods [6]. In this context, research related to predicting the sea surface drift trajectory of wrecked ships can serve as a reference [7,8]. In the following, we will analyze the literature on predicting sea surface drift trajectories of wrecked ships and the methods used for AUV underwater drift trajectory prediction.
In the domain of predicting the sea surface drift trajectory of wrecked ships, pioneering work began in 1970 when the Search and Rescue Planning System (SARP) employed computer simulation methods for predicting maritime target drift trajectories [9]. Subsequently, a search and rescue system based on SARP was proposed in 2000, utilizing grids to represent target drift areas [10]. In 2012, Lokukaluge et al. introduced a mechanism that integrated artificial neural networks into the Ship Traffic Monitoring and Information System (VTMIS) to detect and track multiple targets. They employed an Extended Kalman Filter (EKF) for ship state estimation and conducted simulation validation [11]. Addressing the trajectory prediction issue of sea surface drift under the influence of water flow and wind, Zhang et al. proposed a probability model in 2017 capable of continuously predicting the velocity and position of sea surface drift at any given time [12]. However, this method has a drawback in that the uncertainty of predicting the target position increases due to the complexity of the real environment. In 2019, Miron et al. introduced a Markov chain model based on historical data of sea surface drifts observed by satellites in the Indian Ocean. This model represented the drifting track of aircraft wreckage observed on the sea after flight MH370 disappeared, yielding prediction results consistent with the search area defined by relevant national institutions for debris drift [13]. In 2021, researchers established a deep convolutional neural network prediction model to forecast the drift trajectory of ships and verified its accuracy and effectiveness [14]. Summarizing the literature on predicting sea surface drift trajectories of shipwrecks, shipwreck drift mainly refers to the two-dimensional drift of the sea surface under the combined influence of sea surface ocean circulation, wind-induced current, and sea surface wind. Drift track prediction is based on various models, such as the drift track prediction model and the Markov chain model, and places significant emphasis on factors such as the geometric structure, submerged area, and other characteristics of ships and floating objects which impact the drift direction and velocity. As AUV underwater drift involves a three-dimensional drift completely sub-merged in water, the estimation methods used for predicting the sea surface drift trajectory of shipwrecks, particularly those related to sea surface circulation and wind-induced flow, can serve as useful references. However, other aspects of shipwreck drift prediction may not be directly applicable to AUV underwater drift.
In terms of AUV underwater trajectory prediction, the current focus primarily lies in predicting the trajectory of AUVs with driving forces in various ocean current environments. In 2014, researchers believed that the strength of ocean currents could alter the planned path for slow-moving AUVs, thus enhancing their safety [15]. They introduced a minimum expected risk planner and a risk-aware Markov decision process, validating the model using an underwater glider. In 2018, Subramani et al. [16] proposed and applied a stochastic level set partial differential equation to address uncertainty in optimal path planning. This method optimizes the time and path of AUV travel in uncertain flow fields [16]. However, there is a notable difference between predicting the drift trajectory of AUVs with driving force and those without after a crash. The trajectory prediction method for AUVs with driving force cannot be directly applied to predicting the drift trajectory of AUVs without driving force. For the case of AUV trajectory prediction without power, a modified neural network (ECRNet)-based AUV drift trajectory prediction method was proposed by [6]. This method aims to predict the unpowered drift trajectory of AUVs. The modified neural network incorporates two modules, active modulation and response modulation, to achieve trajectory prediction error correction in the deep sea and shallow sea layers. Simulation results demonstrate superior prediction performance of this method in these ocean layers compared to other time series prediction model methods, with significantly reduced prediction errors. Further optimizing the structure and algorithm of the neural network to reduce prediction errors is an active direction that can be explored in the future [17,18,19,20,21]. It's worth noting that the dataset used for the method in [6] requires prior knowledge of historical data on AUV's passive drift in the specific area where the failure occurred. Such historical data is not available for general AUVs, including the one studied in this paper. Therefore, this method is not applicable to this study. To the best of our knowledge, there is no mature theory and method for accurately predicting the unpowered underwater drift trajectory of AUVs after accidents.
Summarizing the literature on predicting the sea surface drift trajectories of ship-wrecks and AUV underwater drift trajectories, the basic approach for predicting the un-powered drift trajectory of a wrecked ship involves considering the influence of wind and sea surface ocean currents on the ship. Researchers have established drift models for wrecked ships based on force analysis [22,23,24]. The models are solved iteratively using the Lagrange tracking method to predict the two-dimensional drift trajectory of the wrecked ship on the sea surface. However, the unpowered drift trajectory of an AUV is a three-dimensional trajectory, affected by underwater ocean currents that vary in direction and velocity at different depths. Additionally, the AUV's longitudinal velocity affects the time it takes to reach different depths, and the flow direction and velocity of ocean currents differ at various depths. Hence, it is necessary to consider both the vertical upward floating time and lateral drift trajectory together when predicting the AUV's trajectory. Consequently, the two-dimensional drift trajectory prediction method for shipwrecked ships on the sea surface cannot be directly applied to predict the three-dimensional drift trajectory of AUVs underwater. Furthermore, the prediction method for the sunken trajectory of a crashed aircraft in the sea is based on rapid sinking under the influence of high gravity, making it unsuitable for predicting the unpowered floating drift trajectory of AUVs.
Further analysis of the problem of unpowered underwater drift of AUVs reveals that predicting their underwater drift trajectory requires relatively accurate ocean current data. In the literature, two typical methods for predicting ocean currents are deep learning-based approaches [25,26,27] and POM ocean models [28,29,30]. The deep learning-based ocean current prediction method involves training neural network models to learn the patterns from historical ocean current data and predict future short-term ocean current information. However, this method requires a large amount of raw data, and its direct application is challenging due to the multitude of factors influencing ocean currents. On the other hand, the ocean current prediction method based on the POM ocean model simulates the ocean current conditions in a specific area by inputting ocean parameters, boundary conditions, and seabed terrain data and solving fluid mechanics equations. Nonetheless, due to the complexity and variability of seabed terrain, which is difficult to accurately obtain, this method's accuracy in predicting ocean currents is relatively poor [31]. Therefore, while both deep learning-based and POM ocean models are widely used for ocean current prediction, they are not entirely suitable for predicting the drift trajectory of crashed AUVs due to the specific challenges associated with their underwater drift behavior.
Based on the above analysis, this paper proposes a method for predicting the long-term underwater drift trajectory of unpowered AUVs based on the Lagrange tracking method. The contributions of this paper are as follows:
1) We have proposed a three-dimensional trajectory prediction method for AUV drift, which considers both the longitudinal velocity of the AUV (time to reach different depths) and the direction and velocity of the ocean current at different depths. Previously, the unpowered drift of ships on the sea surface was a two-dimensional drift, while the trajectory prediction of AUVs involves a three-dimensional drift. The ocean current is vertically stratified, and the flow direction and velocity vary at different depths. Thus, previous two-dimensional drift trajectory prediction methods for ship accidents are not applicable to the three-dimensional drift trajectory prediction of AUVs. In this paper, we obtain the time to reach different depths based on the AUV's longitudinal velocity, and then use the ocean current information at different depths for trajectory prediction. The underwater drift track of the AUV is predicted through iterative calculations in all three dimensions simultaneously.
2) Regarding the issue of "AUV floating to the sea surface after 100 hours" mentioned earlier, we believe that it should float to the sea surface within 1 hour after the accident. The reason why the AUV failed to reach the sea surface is due to its small residual buoyancy. When it approaches the sea surface, it is unable to break through the thermocline and thus drifts in the thermocline for an extended period. Currently, no literature has reported on the drift track of AUVs in the thermocline. To predict the drift track of AUVs in the thermocline, more accurate thermocline ocean current information is required. However, there is no relevant literature on the estimation methods of thermocline currents. Therefore, this paper proposes a thermocline ocean current estimation method to address this gap.
The structure of this paper is as follows: Section 2 formulates the considered problem and the main idea of the proposed method. Section 3 details the process of the AUV drift trajectory prediction method. Section 4 explains the methods used for ocean current data acquisition and processing, and this benefits the lateral drift trajectory of AUV. Section 5 describes the estimation method of AUV longitudinal velocity, and this benefits the vertical drift trajectory of AUV. In Section 6, utilizing real data, simulation and comparison are conducted to verify the effectiveness of the proposed methodology. Section 7 provides a summary of the findings. Section 8 outlines future research prospects.
During the sea trial conducted by the author's research team, a serious accident resulted in the AUV losing contact at a depth of 1500 m. At that time, the AUV had a residual buoyancy of approximately 10 kg, which theoretically would enable it to float to the sea surface within 1 hour at the slowest. However, surprisingly, it took 100 hours for the AUV to float to the sea surface approximately 65 km away from the accident site. This incident served as the basis for investigating the problem of AUV unpowered drift trajectory prediction. Specifically, the study aims to understand why the AUV did not float to the surface within the expected 1 hour and predict its drift track within the 100-hour timeframe.
After a severe accident of the AUV, the thrusters are deactivated. As depicted in Figure 1, starting from the accident point A, the AUV ascends passively due to the +10 kg residual buoyancy and drifts laterally under the influence of ocean currents.
The illustration of Figure 1 is as follows: Point A is the location of the AUV accident; Point B is the position that the AUV reaches the thermocline under the combined effect of un-powered vertical upward floating and lateral drift due to ocean currents, in other words, the distance between point B and the sea surface is the depth of the thermocline; Point C is the point in the thermocline, where the AUV drifts and ascents due to disposing the carried ballast; Point D is the point that the AUV emerges at the surface of the sea.
As depicted in Figure 1, the trajectory of the underwater drift process of the AUV could be described as A-B-C-D, and the detailed analysis is as follows.
Segment A-B: The AUV slowly ascends vertically under the influence of residual buoyancy and simultaneously moves laterally to point B due to the current. The drift trajectory during segment A-B is three-dimensional.
Segment B-C: Upon reaching point B, the sea water's salinity and temperature experience significant variations within the thermosphere. As a result, the AUV's buoyancy decreases rapidly, and the residual buoyancy diminishes substantially, approaching 0. Consequently, the AUV becomes unable to overcome the thermosphere and drifts with the ocean current within the B-C layer for a long time. Throughout this stage, the AUV's longitudinal position remains unchanged, indicating two-dimensional drift.
Segment C-D: Upon reaching point C, due to the influence of ocean current and other factors, the AUV disposes of the ascending ballast it carries, leading to a rapid ascent to-wards point D on the sea surface, powered by significant buoyancy.
For estimating the trajectory of a lost ship or aircraft, the Lagrangian tracking method is a recognized mathematical foundation in this field. Its fundamental idea is to track the motion of fluid particles and integrate the movement of all particles to construct the entire fluid dynamics. Constrained conditions and solving processes are studied in combination with the characteristics of the research object (lost ship or aircraft) and the environment. The Lagrangian tracking method has been widely applied in the classic method to predict the drift trajectory of a lost ship [32,33,34,35]. The starting point of this paper is also based on the Lagrangian tracking method for predicting the drift trajectory of an AUV. In response to the uniqueness of AUV drift, we address the issues encountered in using this method to predict the drift trajectory of AUVs.
Based on the analysis of the AUV drifting process in the last section, the main idea of the proposed method is to predict the AUV drift track at Segments A-B, B-C, and C-D, respectively.
1) Realization of the drift trajectory prediction in the A-B segment
The idea of drift trajectory prediction in segment A-B is as follows: Firstly, we will analyze the law of AUV residual buoyancy changing with depth, and then obtain the ascending speed. Then, the time to reach different depths is obtained based on the ascending speed. Further, the lateral track prediction will be carried out by using the ocean current at different depths, and finally the A-B track can be obtained. The depth of point B is in the thermocline, and the depth value of point B is determined according to the marine environment information of the place where the accident occurred. The time to reach point B can be calculated through the AUV's ascending speed, and then through the lateral drift of the A-B Segment that changes with time track, point B can be determined.
The realization of the drift trajectory prediction in the A-B segment is shown in Figure 2.
As shown in Figure 2, the main flow of the realization of the drift trajectory prediction for the A-B segment is as follows.
Ⅰ) Obtain the location of the AUV accident (point A)
Based on the signals sent by underwater acoustics to the mothership before the AUV malfunctioned during the sea trial, we can know the longitude and latitude coordinates of the AUV accident location (point A).
Ⅱ) Acquire and process of A-B section ocean current data
Section 4 of this article explains the methods for obtaining and processing ocean current data.
Ⅲ) Calculate the longitudinal velocity of the AUV in section A-B
Section 5 of this article explains the calculation method for AUV longitudinal velocity.
Ⅳ) Predict the longitudinal drift trajectory of the AUV in the A-B segment
Based on the longitudinal velocity, the longitudinal drift trajectory z(t) of the AUV over time is obtained through iterative calculations using Lagrange tracking methods.
Denoting x(t),y(t),z(t) as the coordinate of the AUV at time t, the position of the AUV could be represented as follows:
L′=[xyz]=[x(t)y(t)z(t)] | (1) |
Denote vx(t),vy(t),vz(t) as the velocity components of AUV in the three axes at time t, the velocity of an AUV could be represented as follows:
V'=[vx(t)vy(t)vz(t)]=L'(t+1)−L′(t)Δt | (2) |
Ⅴ) Calculate the time from point A to point B
Based on the sea trial experiment data, we can determine the depth of the thermocline from the sea surface. Using this information, we can calculate the distance from the accident site A to the thermocline. Then, with the AUV longitudinal velocity obtained in Section 5 below, we can calculate the time it takes for the AUV to travel from point A to point B.
Ⅵ) Predict the lateral drift trajectory of the AUV in the A-B segment
Step Ⅳ) above yields the longitudinal position trajectory z(t). Using the time t, we can calculate the AUV's vertical floating to different depth values. Ocean current data for different depth values can be acquired through Section 4. By applying Lagrange tracking methods to Eqs (1) and (2), we can iteratively compute the lateral drift trajectory of the AUV over time.
Ⅶ) Determine the position of point B
After determining the time from point A to point B, the vertical and horizontal trajectory predictions x(t),y(t),z(t) obtained above can be used to determine the position of point B.
Ⅷ) Predict the three-dimensional drift trajectory of the AUV in the A-B segment
Synthesize the longitudinal drift trajectory z(t) and lateral drift trajectory x(t),y(t) of the AUV obtained from steps Ⅳ) and Ⅴ). Then, using the time as the reference, we can obtain the three-dimensional drift trajectory x(t),y(t),z(t) of the A-B segment AUV.
2) Realization of drift trajectory prediction in the B-C segment
The idea of drift trajectory prediction in segment B-C: The B-C segment is situated within the thermocline, as indicated above. In this layer, the AUV's remaining buoyancy is close to 0, preventing it from breaking through the thermocline and floating to the sea surface. Instead, it drifts for an extended period from point B to point C within this layer, characterized by two-dimensional drift. As demonstrated by [15], the lateral velocity of the AUV matches the ocean current velocity of this layer.
The prediction of the upper A-B segment drift trajectory provides longitude and latitude coordinate values for point B, and the ocean current data of this layer can be acquired in Section 4 as mentioned below. Following the Lagrange tracking principle, the B-C segment drift trajectory can be predicted as follows:
[x(t+1)y(t+1)]=[x(t)y(t)]+[vx(t)vy(t)]Δt | (3) |
where x(t),y(t) are the position coordinates of the AUV in the x and y directions at time t. x(t+1),y(t+1) are the position coordinates of the AUV in the x and y directions at time t+1. vx(t) and vy(t) are the ocean current components in the x and y directions at time t.
By iterating according to Eq (3), the drift trajectory x(t),y(t) of the B-C segment can be obtained.
3) Realization of drift trajectory prediction in the C-D segment
The idea of drift trajectory prediction in segment C-D: At point C, due to the AUV being impacted by the current, the ascending ballast suddenly dropped, resulting in much greater buoyancy than gravity. Consequently, the AUV quickly floated up to the sea surface within a short period (approximately 20 seconds). Point D represents the location of the water outlet point.
In the C-D segment, the AUV experiences a brief upward rising time, and the ocean current has a minimal impact on its lateral drift distance. This article disregards this lateral drift distance and assumes that the position coordinate of point D is the same as that of point C.
In this paper, the proposed method involves the acquisition and processing of ocean current data in sections A-B and B-C, and this section describes the acquisition and processing methods of deep-ocean current (A-B segment) and shallow-ocean current (B-C segment) data.
It is impossible to obtain the real-time deep-ocean current data for the A-B segment since it belongs to deep-ocean current. To address this issue, this paper analyzed historical ocean current data of the accident location during several periods and observed minimal variation in the data within a few days. Consequently, the paper utilized the ocean current data on the day of the incident directly as the basis for forecasting. The ocean cur-rent data was obtained from the South China Sea Oceanic Data Center's records. "Historical Ocean Data Product (1980–2019) (1/10-degree resolution) (hourly intervals)" [36].
1) Analysis of typical methods in obtaining and processing ocean current data
The B-C segment belongs to shallow-ocean current, which is influenced by both its own ocean current and surface wind-induced current. Shallow-ocean currents are time-varying and cannot be accurately predicted using data from a few days ago. Therefore, it is essential to estimate the ocean current based on current conditions. Currently, the main methods for predicting shallow-ocean currents are ocean models and neural network methods.
Ocean models, primarily the POM and ROMS models, are currently the most widely used methods for predicting ocean currents over large-scale ranges and long time periods. These models rely on a substantial amount of oceanographic data, including sea-floor topography data, and are mainly used for predicting ocean data at large-scale ranges and long-time periods, including ocean currents. On the other hand, neural network methods are mainly employed for predicting ocean data at small-scale ranges and short-time periods, including ocean currents. The prediction of shallow-ocean currents using ocean models involves utilizing large-scale oceanographic data, including seafloor topography data. The solution is obtained by setting boundary conditions and solving a set of equations to derive the ocean current information for a specific area. However, this method demands a significant amount of oceanographic data, making it challenging to apply to the AUV drifting trajectory prediction problem addressed in this paper. Similarly, neural network methods also require a substantial amount of oceanographic data as training samples, rendering them unsuitable for the specific problem discussed in this article. Therefore, the paper proposes a shallow-ocean current estimation method.
2) The differences of the proposed shallow-ocean current estimation method with previous methods
Ⅰ) The proposed method
Based on the above analysis, this paper proposes a shallow-ocean current estimation method, with the idea shown in Figure 3.
The main flow is as follows: A few days before the AUV failure date, the ocean current data of the B-C layer in the fault area is known. The ocean current data (S) of this layer is a combination of its own ocean current (M) and the wind-induced ocean current (W) caused by sea surface winds. The own ocean current (M) remains relatively stable within a few days, whereas the wind-induced ocean current (W) undergoes daily variations. The wind-induced ocean current (W) can be calculated based on the sea surface wind data of the day, using the Ekman formula to determine the wind-induced ocean current (W) generated by sea surface winds.
This paper retains the own ocean current (M) from the ocean current data of this layer and removes the wind-induced ocean current (W) from the previous days' ocean current data (S). Subsequently, it calculates the corresponding wind-induced ocean current (W) based on the sea surface wind data of the predicted date (the date of predicting the drift trajectory of an AUV accident) and adds it to the retained own ocean current (M) to obtain the ocean current data of this layer for the predicted date (S).
Ⅱ) The difference between this method and previous methods
This method is based on the ocean current data and sea surface wind data of the accident site in the days before the fault occurred to calculate the thermocline layer ocean current for the day of the accident and the dates after the accident. It differs from previous ocean modeling methods and neural network methods for predicting ocean current. This method does not require a large amount of ocean environment and geographical information data. It requires less historical data and the data is easier to obtain.
3) Realization of the ocean current data acquisition and processing
Ⅰ) Obtain ocean current data (S)
The "40-year historical ocean data product in the South China Sea (1980-2019) (1/10 degree)" [36] from the South China Sea Oceanic Data Center can be directly used to obtain the ocean current data (S) for the days before the accident.
Ⅱ) Calculate wind-induced ocean current data (W)
ⅰ) Obtain wind field data
Wind field data is obtained from the NECP/NCAR data (daily) [37] of the National Oceanic and Atmospheric Administration of the United States.
ⅱ) Calculate wind-induced ocean current data
According to the wind field data, the wind-induced ocean current (W) is calculated using the Ekman drift theory [38].
Ⅲ) Calculate ocean current (M)
By taking the vector difference between the ocean current data for the days before the accident and the wind-induced ocean current data for the same period, the own ocean current (M) can be obtained. The calculation formula is shown as follows:
→vM(lon, lat, depth)=→vS1(lon, lat, depth)−→vW1(lon, lat, depth) | (4) |
where →vM is the ocean current, →vS1 is the ocean current of the layer in the previous days and →vW1 is the wind induced ocean current of the previous days.
Ⅳ) Calculate the ocean current for the predicted date (S)
Then, sum the wind induced ocean current data of the predicted date with its own ocean current to obtain the predicted date ocean current data.
The calculation process can be expressed as follows:
→vS2(lon, lat, depth)=→vM(lon, lat, depth)+→vW2(lon, lat, depth) | (5) |
where →vS2 is the ocean current of the predicted date in this layer, →vM is the ocean current and →vW2 is wind induced flow of the predicted date.
In this section, we aim to establish the relationship z(t) between the longitudinal velocity and depth, which could be used to support the trajectory prediction of segment A-B in Section 4.
Since water density and volume compression vary with depth, the residual buoyancy also changes accordingly. Therefore, in this section, the relationship between buoyancy, gravity and depth is calculated. Based on this, the relationship between residual buoyancy and depth is derived. Finally, using the relation between residual buoyancy and depth, the relation z(t) between longitudinal velocity and depth is determined.
To understand the relationship between buoyancy and depth, it is essential to explore the connection between density, volume and depth, as buoyancy is closely related to these factors. Below, the relationships between density, volume and depth are calculated separately, and subsequently, the relationship between buoyancy and depth is derived.
1) Relation between density and depth
The international seawater equation of state [39] is used to calculate the density at any depth, as follows:
ρ=f(s,t,p) | (6) |
where ρ is the density of seawater, s is the salinity of seawater, t is the temperature of seawater and p is the pressure of seawater (corresponding depth).
As shown in Figure 4, according to the marine environmental data of the accident site [36], s, t and p are substituted into Eq (6) to calculate the relationship between seawater density and depth.
2) The relationship between volume and depth
The volume of the AUV changes with depth during the ascending process. It is important to investigate the relationship between volume variation and depth during the AUV's ascent. In AUVs with greater depth, the components that experience significant volume changes at different depths mainly consist of the glass sphere equipment cabin, buoyancy material and glass sphere protective cover [40]. As a result, this paper also calculates the volume variation of these three components with depth.
As depicted in Figure 5, based on the specific structural parameters of the AUV involved in the accidents studied in this paper, the relationship between volume change and depth of the AUV is determined using the formula from literature [40].
3) The relationship between buoyancy and depth
Given the relationship between density, volume and depth, the relationship between AUV buoyancy and depth can be calculated. Buoyancy is calculated as follows:
[F1F2⋮FnFn+1]=[ρ1ρ2⋮ρnρn+1][V1V2⋯VnVn+1] | (7) |
where F1,F2⋯Fn,Fn+1 is the buoyancy force of the AUV at different depths, ρ1,ρ2⋯ρn,ρn+1 is the corresponding density of seawater at different depths and V1,V2⋯Vn,Vn+1 is the corresponding volume of the AUV at different depths.
As shown in Figure 6, the relationship between density, volume and depth obtained above is substituted into Eq (7) to obtain the relationship between AUV buoyancy and depth.
From Figure 6, the buoyancy of the AUV increases as the depth increases. In the vicinity of the mentioned thermocline in this paper, the buoyancy changes at a faster rate for a small value.
An object's gravity in the sea varies with depth, primarily due to changes in the acceleration of gravity (g) at different depths. In this paper, the AUV accident occurred at a water depth of 1500 m. The variation of g within the depth range of 0–1500 meters is only 0.003 m/s2, which is very small. Based on the analysis in reference [41], it can be ignored.
As shown in Figure 7, we have already obtained the relationship between buoyancy and depth, as well as the conclusion that gravity does not change with depth. By considering that residual buoyancy is the difference between buoyancy and gravity, we can establish the relationship between residual buoyancy and depth.
As shown in Figure 7, the residual buoyancy is significantly reduced near the sea surface, specifically at a depth of about 30 meters (thermocline). The reason for this can be observed from Figure 6: Buoyancy decreases rapidly near the thermocline, while gravity remains unchanged, leading to a rapid decrease in residual buoyancy. Due to the small buoyancy force, the AUV is unable to break through the "thermocline", causing it to drift laterally within the thermal layer.
According to the relationship between residual buoyancy and depth, the longitudinal velocity of the AUV can be calculated. When the AUV is rising in the vertical direction, the force is:
F1=Fwater+G | (8) |
where F1 is the buoyancy of the AUV, Fwater is the resistance of water, and G is the gravity of the AUV. Herein, F1 and G that change with depth have been calculated, and Fwater that changes with depth can be calculated by Eq (8). Then, according to [42], the relationship between longitudinal velocity and depth shown in Figure 8 can be calculated through the following equation:
Fwater(h)=165.73×v(h)2+0.0001×v(h)+0.0002 | (9) |
From Figure 8, the AUV exhibits faster rising speeds in the depth range of 1500–100 meters. However, when it reaches the depth range of 100–30 meters, the longitudinal velocity decreases rapidly with decreasing depth. At a depth of approximately 30 meters, the longitudinal velocity becomes zero, and the AUV enters the thermocline. Consequently, instead of rising, it drifts laterally within the thermocline. Furthermore, Figure 7 also confirms that the AUV studied in Section 2.1 of this paper did not float to the sea surface within 1 hour; rather, it drifted laterally within the thermocline. This analysis conclusion appears to be correct.
To verify the effectiveness of the proposed Lagrange tracking-based AUV drift trajectory prediction method, the comparisons between the actual water outlet point in a sea trial and that predicted by the proposed method will be conducted in this section.
In 2019, when the research team of the authors conducted a sea trial, a serious accident happened and the AUV lost contact at a depth of 1500 meters. About 100 hours later, the AUV ascended to the surface about 65 kilometers away from where the accident occurred.
The relevant data of the AUV involved in the accident is as follows:
Based on the data in Table 1, AUV drift trajectory prediction is carried out using the proposed method. In the following, to demonstrate the effectiveness of the proposed method, the actual water outlet point D' and the predicted one D will be compared.
Point | A | D′ |
Accidental time | 24th May, 10:50 | 28th May, 14:59 |
Position (longitude, latitude) | 110.89136, 17.60279 | 110.48121, 17.18687 |
The simulation process is depicted in Figure 9.
Now, based on Figure 9, the description of the technology used is as follows:
1) Drift trajectory prediction for segment A-B
The AUV residual buoyancy in segment A-B is calculated using the method in Section 5. Then, the ascending velocity could be obtained. At the same time, the method in Section 4.1 is used to obtain the information of ocean current in segment A-B. On this basis, the method in Section 3 is used to predict the three-dimensional drift trajectory in segment A-B.
2) Drift trajectory prediction for segment B-C
The information of the ocean current in segment B-C is obtained based on the sea surface wind and near-surface current. Based on the obtained ocean current, the two-dimensional drift trajectory is predicted using the method in Section 3.
3) The AUV resurfacing location prediction
Based on the time of the AUV accident and the time it takes for the AUV to surface, the location of point C on the trajectory can be obtained from the prediction model. Since the latitude and longitude of point C are identical to those of the resurfacing point D, we have that point C is located directly below point D in the vertical direction. As a result, the location of the resurfacing point D of the AUV can be determined.
4) Comparison between the predicted and actual resurfacing point location
The actual resurfacing point location D' is obtained from sea trial experiments, while the predicted AUV resurfacing point location D is determined in the preceding step. By comparing the predicted AUV resurfacing point location D with the actual resurfacing point location D', the prediction accuracy is evaluated, thus validating the effectiveness of the proposed method in this paper.
The simulation is conducted with Octave 4.0.0 on a Dell Inspiron 7590 computer, Intel (R) Core (TM) i5-9300 H processor and 16 GB of memory. The Windows 11 system version 21H2 is used as the operating system, with a 64-bit operating system based on an ×64 processor.
First, the simulation calculates the drift trajectories for the A-B and B-C segments, and then combines them to obtain the entire A-B-C-D drift trajectory. Finally, the predicted results are compared and analyzed with the sea trial results.
1) A-B segment
The predicted horizontal drift trajectory of the AUV based on the proposed method for the A-B segment is shown in Figure 10, and the predicted vertical drift trajectory is shown in Figure 11. The three-dimensional trajectory is shown in Figure 12.
2) B-C segment
For the B-C segment, the predicted horizontal drift trajectory of the AUV based on the proposed method is shown in Figure 13. Only horizontal drift occurs during this segment, and it is a two-dimensional curve.
3) Entire A-B-C-D segments
The entire three-dimensional predicted drift trajectory is shown in Figure 14.
Let us briefly explains the calculation method of the water outlet point. The trajectory of the B-C segment can be predicted using the proposed method as described above. The time from the occurrence of the accident to the AUV surfacing to the sea level is known (100 hours 9 minutes), and using this time, the position of point C can be predicted. As shown in Figure 14, point D is at the same position as point C. Therefore, the position of point D can be predicted.
In Figure 14 the A-B-C-D trajectory is predicted, while the real trajectory is unknown. Therefore, comparing the trajectories cannot demonstrate the effectiveness of the proposed method in this paper. However, the true position of the water outlet point is known. By comparing the predicted position of the water outlet point obtained by the proposed method in this paper with the actual position of the water outlet point, the effectiveness of this method can be demonstrated.
As shown in Figure 15, the coordinates of the actual water outlet point are D' (110.48121, 17.18687), and the coordinates of the predicted water outlet point D is (110.46411, 17.28031).
To the best of our knowledge, currently, there is no accuracy evaluation index for AUV underwater drift trajectory prediction. Thus, in this paper, we evaluate the prediction accuracy of the proposed method based on the accuracy evaluation index of wrecked ship drift track [43,44], i.e., angular deviation AE, absolute position deviation MSE and relative position deviation RE. For angle deviation AE: It can be seen from Figure 15 that, compared with the accident point A, the angle deviation AE of the predicted water outlet point and the actual water outlet point is 8.4 degrees, which is relatively small since the drift time is 100 hours and the drift distance is 65*103 km kilometers. Generally speaking, if the angle deviation AE is less than 10 degrees, it belongs to high precision [43,44]. For the absolute position error MSE: The predicted water outlet point is 10.4 km away from the real water outlet point, and thus the absolute position error is MSE = 10.4 km, which is large. However, from the perspective of error evaluation, more attention is paid to the relative position deviation RE. In our case, the relative deviation of the drift distance of the water outlet point relative to 65 km is RE = 16%, which is not high for ship drift. However, for AUV underwater drift, the underwater drift time was 100 hours, and there is no mature method for reference. Thus, the relative positional deviation obtained by the proposed method could be seen as a relatively good prediction result. In the future, better AUV drift trajectory prediction methods will be investigated.
In the A-B segment, the flow velocity and direction of different depth currents vary. In the B-C segment, where the thermocline exists at the same depth, the flow velocity and direction of the currents also differ. This demonstrates that the methodology proposed in this paper can be applied to diverse environments.
A typical AUV has buoyancy (approximately 10 kg), and when it loses control in an accident, it floats to the sea surface due to buoyancy. In the case of the 1500 m depth, the AUV resurfaces within 45 minutes. The accident position coordinates of the AUV are known, and the change in position after resurfacing is minimal. Furthermore, once the AUV resurfaces, the mother ship can determine its position through the GPS chip on the AUV. Such AUV drift trajectory prediction falls under short-term prediction, which is generally not of significant concern from a practical perspective.
This study focuses on the long-term drift trajectory prediction problem of AUVs, where the AUV resurfaces approximately 100 hours after the accident. The reason behind this is the buoyancy of the AUV decreases to zero when it reaches the thermocline layer, which prevents it from breaking through and continuing to rise, resulting in long-term drift in the thermocline layer (detailed explanation in Section 2.2). Currently, reference [6] has done some research on short-term drift trajectory prediction for AUVs, but it requires prior knowledge of historical data on an AUV's passive drift in the specific area where the failure occurred. Such historical data is not available for general AUVs, including the one studied in this paper. Therefore, this method is not applicable to this study. Currently, no relevant literature on long-term drift trajectory prediction methods for AUVs has been found. Hence, there is no comparison with previous methods in this paper.
This paper investigates the problem of predicting the passive long-term underwater drift trajectory of an AUV in the context of a real AUV accident during sea trials that resulted in loss of communication. Currently, there are very few relevant literature resources addressing this issue, and those available focus on shallow sea areas, which are not applicable to the deep-sea environment studied in this paper at a depth of 1500 meters. Therefore, this paper proposes a three-dimensional drift trajectory prediction method for AUVs based on the Lagrangian tracking method. This method takes into account the AUV's buoyancy velocity, the time taken to reach different depths, and the ocean current data at different depths.
To address the issue of the long-term failure of the AUV to resurface, this paper proposes that the reason lies in the insufficient remaining buoyancy that prevents it from breaking through the thermocline, causing prolonged lateral drift within the thermocline. Simulation results confirm the accuracy of this analysis and provide guidance for reserving sufficient remaining buoyancy in future AUV designs.
Regarding the prediction of AUV drift trajectories during prolonged drifting within the thermocline, it requires estimation of the thermocline current, which is not covered in existing literature. Hence, this paper proposes a method for estimating the thermocline current.
Compared with the real data from the AUV accident, the simulated resurfacing point has a deviation of 8.4 degrees in angle and an absolute position deviation of 10.4 km. The angle deviation is small, while the absolute position error is relatively large. For a real AUV accident with 100 hours of loss of communication and 65 km of underwater drift, the method exhibits very good angular accuracy, providing a relatively accurate search direction for subsequent search and rescue operations. In maritime search and rescue, an accurate search direction is more important than positional error. The simulation results validate the effectiveness of the proposed method in this paper.
Aiming at the problem of long-term unpowered AUV drifting track prediction after serious accidents, a three-dimensional AUV drifting track prediction method based on the Lagrange tracking approach was proposed in this paper. Comparing the simulation results with the real AUV sea trial data, it can be seen that the proposed method has a good track prediction accuracy in the angular direction, but a relatively large deviation in the predicted position. The reason lies in the low accuracy of the information of ocean current. The ocean current modeling method proposed in this paper is mainly for short-term (within one week) prediction, but less suitable for long-term prediction. Future work will focus on optimizing the prediction model of ocean currents by using neural networks to improve the prediction accuracy of ocean currents, and result in more accurate AUV location prediction.
The authors declare they have not used Artificial Intelligence (AI) tools in the creation of this article.
This work is supported by the National Natural Science Foundation of China (Nos. 52171310, 51839004, 62303128).
The authors declare there is no conflict of interest.
[1] |
X. Xiang, C. Yu, Q. Zhang, On intelligent risk analysis and critical decision of underwater robotic vehicle, Ocean Eng., 140 (2017), 453–465. https://doi.org/10.1016/j.oceaneng.2017.06.020 doi: 10.1016/j.oceaneng.2017.06.020
![]() |
[2] |
W. Wawrzyński, M. Zieja, M. Żokowski, N. Sigiel, Optimization of Autonomous Underwater Vehicle mission planning process, Bull. Pol. Acad. Sci. Tech. Sci., 70 (2022), e140371. https://doi.org/10.24425/bpasts.2022.140371 doi: 10.24425/bpasts.2022.140371
![]() |
[3] |
X. Chen, N. Bose, M. Brito, F. Khan, B. Thanyamanta, T. Zou, A review of risk analysis research for the operations of Autonomous Underwater Vehicles, Reliab. Eng. Syst. Saf., 216 (2021), 108011. https://doi.org/10.1016/j.ress.2021.108011 doi: 10.1016/j.ress.2021.108011
![]() |
[4] |
S. Xia, X. Zhou, H. Shi, S. Li, C. Xu, A fault diagnosis method based on attention mechanism with application in Qianlong-2 Autonomous Underwater Vehicle, Ocean Eng., 233 (2021), 109049. https://doi.org/10.1016/j.oceaneng.2021.109049 doi: 10.1016/j.oceaneng.2021.109049
![]() |
[5] |
D. Chaos, D. Moreno-Salinas, J. Aranda, Fault-tolerant control for AUVs using a single thruster, IEEE Access, 10 (2022), 22123–22139. https://doi.org/10.1109/access.2022.3152190 doi: 10.1109/ACCESS.2022.3152190
![]() |
[6] |
Y. Yu, J. Zhang, T. Zhang, AUV drift track prediction method based on a modified neural network, Appl. Sci., 12 (2022), 12169. https://doi.org/10.3390/app122312169 doi: 10.3390/app122312169
![]() |
[7] |
S. Meng, W. Lu, Y. Li, H. Wang, L. Jiang, A study on the leeway drift characteristic of a typical fishing vessel common in the Northern South China Sea, Appl. Ocean Res., 109 (2021), 102498. https://doi.org/10.1016/j.apor.2020.102498 doi: 10.1016/j.apor.2020.102498
![]() |
[8] |
H. Tu, L. Mu, K. Xia, X. Wang, K. Zhu, Determining the drift characteristics of open lifeboats based on large-scale drift experiments, Front. Mar. Sci., 9 (2022), 1017042. https://doi.org/10.3389/fmars.2022.1017042 doi: 10.3389/fmars.2022.1017042
![]() |
[9] | J. R. Frost, L. D. Stone, Review of search theory: Advances and applications to search and rescue decision support, TRB Annu. Meet., 2001. |
[10] | National SAR Manual, National Search and Rescue Manual, EXHIBIT/P-00112, 1998. Available from: http://www.oshsi.nl.ca/userfiles/files/p00112.pdf. |
[11] |
L. P. Perera, P. Oliveira, C. Guedes Soares, Maritime traffic monitoring based on vessel detection, tracking, state estimation, and trajectory prediction, IEEE Trans. Intell. Transp. Syst., 13 (2012), 1188–1200. https://doi.org/10.1109/tits.2012.2187282 doi: 10.1109/TITS.2012.2187282
![]() |
[12] |
J. Zhang, Â. P. Teixeira, C. Guedes Soares, X. Yan, Probabilistic modelling of the drifting trajectory of an object under the effect of wind and current for maritime search and rescue, Ocean Eng., 129 (2017), 253–264. https://doi.org/10.1016/j.oceaneng.2016.11.002 doi: 10.1016/j.oceaneng.2016.11.002
![]() |
[13] |
P. Miron, F. J. Beron-Vera, M. J. Olascoaga, P. Koltai, Markov-chain-inspired search for MH370, Chaos: Interdiscip. J. Nonlinear Sci., 29 (2019), 041105. https://doi.org/10.1063/1.5092132 doi: 10.1063/1.5092132
![]() |
[14] | M. Zhao, J. Zhang, M. H. Rashid, Predicting the drift position of ships using deep learning, in the 2nd International Conference on Computing and Data Science, Association for Computing Machinery, (2021), 1–5. https://doi.org/10.1145/3448734.3450922 |
[15] |
A. A. Pereira, J. Binney, G. A. Hollinger, G. S. Sukhatme, Risk-aware path planning for Autonomous Underwater Vehicles using Predictive ocean models, J. Field Rob., 30 (2013), 741–762. https://doi.org/10.1002/rob.21472 doi: 10.1002/rob.21472
![]() |
[16] |
D. N. Subramani, Q. J. Wei, P. F. J. Lermusiaux, Stochastic time-optimal path-planning in uncertain, strong, and dynamic flows, Comput. Methods Appl. Mech. Eng., 333 (2018), 218–237. https://doi.org/10.1016/j.cma.2018.01.004 doi: 10.1016/j.cma.2018.01.004
![]() |
[17] |
Z. Wu, H. R. Karimi, C. Dang, An approximation algorithm for graph partitioning via deterministic annealing neural network, Neural Networks, 117 (2019), 191–200. https://doi.org/10.1016/j.neunet.2019.05.010 doi: 10.1016/j.neunet.2019.05.010
![]() |
[18] |
Z. Wu, Q. Gao, B. Jiang, H. R. Karimi, Solving the production transportation problem via a deterministic annealing neural network method, Appl. Math. Comput., 411 (2021), 126518. https://doi.org/10.1016/j.amc.2021.126518 doi: 10.1016/j.amc.2021.126518
![]() |
[19] |
D. Tong, B. Ma, Q. Chen, Y. Wei, P. Shi, Finite-time synchronization and energy consumption prediction for multilayer fractional-order networks, IEEE Trans. Circuits Syst. Ⅱ Express Briefs, 70 (2023), 2176–2180. https://doi.org/10.1109/TCSII.2022.3233420 doi: 10.1109/TCSII.2022.3233420
![]() |
[20] |
G. Yang, D. Tong, Q. Chen, W. Zhou, Fixed-time synchronization and energy consumption for kuramoto-oscillator networks with multilayer distributed control, IEEE Trans. Circuits Syst. Ⅱ Express Briefs, 70 (2022), 1555–1559. https://doi.org/10.1109/TCSII.2022.3221477 doi: 10.1109/TCSII.2022.3221477
![]() |
[21] |
C. Xu, D. Tong, Q. Chen, W. Zhou, P. Shi, Exponential stability of Markovian jump systems via adaptive sliding mode control, IEEE Trans. Syst. Man Cybern.: Syst., 51 (2019), 954–964. https://doi.org/10.1109/TSMC.2018.2884565 doi: 10.1109/TSMC.2018.2884565
![]() |
[22] |
K. Zhu, L. Mu, H. Tu, Exploration of the wind-induced drift characteristics of typical Chinese offshore fishing vessels, Appl. Ocean Res., 92 (2019), 101916. https://doi.org/10.1016/j.apor.2019.101916 doi: 10.1016/j.apor.2019.101916
![]() |
[23] |
H. Yasukawa, N. Hirata, Y. Nakayama, A. Matsuda, Drifting of a dead ship in wind, Ship Technol. Res., 70 (2023), 26–45. https://doi.org/10.1080/09377255.2021.1954835 doi: 10.1080/09377255.2021.1954835
![]() |
[24] | H. W. Tu, X. D. Wang, L. Mu, J. L. Sun, A study on the drift prediction method of wrecked fishing vessels at sea, in OCEANS 2021: San Diego–Porto, IEEE, (2021), 1–6. https://doi.org/10.23919/OCEANS44145.2021.9705751 |
[25] |
D. Sumangala, A. Joshi, H. Warrior, Modelling freshwater plume in the Bay of Bengal with artificial neural networks, Curr. Sci., 123 (2022), 73–80. https://doi.org/10.18520/cs/v123/i1/73-80 doi: 10.18520/cs/v123/i1/73-80
![]() |
[26] |
L. Ren, Z. Hu, M. Hartnett, Short-term forecasting of coastal surface currents using high frequency radar data and artificial neural networks, Remote Sens., 10 (2018), 850. https://doi.org/10.3390/rs10060850 doi: 10.3390/rs10060850
![]() |
[27] |
H. Kalinić, H. Mihanović, S. Cosoli, M. Tudor, I. Vilibić, Predicting ocean surface currents using numerical weather prediction model and Kohonen neural network: A northern Adriatic study, Neural Comput. Appl., 28 (2017), 611–620. https://doi.org/10.1007/s00521-016-2395-4 doi: 10.1007/s00521-016-2395-4
![]() |
[28] | H. Guan, X. Dong, C. Xue, Z. Luo, H. Yang, T. Wu, Optimization of POM based on parallel supercomputing grid cloud platform, in 2019 Seventh International Conference on Advanced Cloud and Big Data (CBD), IEEE, (2019), 49–54. https://doi.org/10.1109/cbd.2019.00019 |
[29] |
A. K. Das, A. Sharma, S. Joseph, A. Srivastava, D. R. Pattanaik, Comparative performance of HWRF model coupled with POM and HYCOM for tropical cyclones over North Indian Ocean, MAUSAM, 72 (2021), 147–166. https://doi.org/10.54302/mausam.v72i1.127 doi: 10.54302/mausam.v72i1.127
![]() |
[30] |
C. D. Dong, T. H. H. Nguyen, T. H. Hou, C. C. Tsai, Integrated numerical model for the simulation of the T.S. Taipei oil spill, J. Mar. Sci. Technol., 27 (2019), 7. https://doi.org/10.6119/JMST.201908_27(4).0007 doi: 10.6119/JMST.201908_27(4).0007
![]() |
[31] |
J. Xu, J. Y. Bao, C. Y. Zhan, X. H. Zhou, Tide model CST1 of China and its application for the water level reducer of bathymetric data, Mar. Geod., 40 (2017), 74–86. https://doi.org/10.1080/01490419.2017.1308896 doi: 10.1080/01490419.2017.1308896
![]() |
[32] |
H. Xu, Tracking lagrange trajectories in position–velocity space, Meas. Sci. Technol., 19 (2008), 075105. https://doi.org/10.1088/0957-0233/19/7/075105 doi: 10.1088/0957-0233/19/7/075105
![]() |
[33] |
T. Heus, G. van Dijk, H. J. J. Jonker, H. E. A. Van den Akker, Mixing in shallow cumulus clouds studied by lagrange particle tracking, J. Atmos. Sci., 65 (2008), 2581–2597. https://doi.org/10.1175/2008jas2572.1 doi: 10.1175/2008JAS2572.1
![]() |
[34] |
N. B. Engdahl, R. M. Maxwell, Quantifying changes in age distributions and the hydrologic balance of a high-mountain watershed from climate induced variations in recharge, J. Hydrol., 522 (2015), 152–162. https://doi.org/10.1016/j.jhydrol.2014.12.032 doi: 10.1016/j.jhydrol.2014.12.032
![]() |
[35] |
M. Jing, F. Heße, R. Kumar, O. Kolditz, T. Kalbacher, S. Attinger, Influence of input and parameter uncertainty on the prediction of catchment-scale groundwater travel time distributions, Hydrol. Earth Syst. Sci., 23 (2019), 171–190. https://doi.org/10.5194/hess-23-171-2019 doi: 10.5194/hess-23-171-2019
![]() |
[36] | Y. H. Zhu, S. Q. Peng, 40 years of marine data products in the south china sea (1980–2019) (1/10 degree) (hourly) (netcdf), the Key Special Project for Introduced Talents Team of Southern Marine Science and Engineering Guangdong Laboratory (Guangzhou) (GML2019ZD0303), 2019. Available from: http://data.scsio.ac.cn/metaDatadetail/1480813599763386368. |
[37] | NOOA Physical Sciences Laboratory (PSL), NCEP/NCAR Reanalysis. Available from: https://psl.noaa.gov/. |
[38] | W. Ekman, Eddy-viscosity and skin-friction in the dynamics of winds and ocean-currents, in Memoirs of the Royal Meteorological Society, Stanford, (1928), 161–172. |
[39] |
N. P. Fofonoff, Physical properties of seawater: A new salinity scale and equation of state for seawater, J. Geophys. Res., 90 (1985), 3332–3342. https://doi.org/10.1029/JC090iC02p03332 doi: 10.1029/JC090iC02p03332
![]() |
[40] |
Y. Jiang, Y. Li, Y. Su, J. Cao, Y. Li, Y. Wang, et al., Statics variation analysis due to spatially moving of a full ocean depth Autonomous Underwater Vehicle, Int. J. Nav. Archit. Ocean Eng., 11 (2019), 448–461. https://doi.org/10.1016/j.ijnaoe.2018.08.002 doi: 10.1016/j.ijnaoe.2018.08.002
![]() |
[41] |
K. Zhang, New gravity acceleration formula research (in Chinese), Prog. Geophys., 26 (2011), 824–828. https://doi.org/10.3969/j.issn.1004-2903.2011.03.006 doi: 10.3969/j.issn.1004-2903.2011.03.006
![]() |
[42] |
Y. K. Wang, Simulation research on the full-ocean-depth AUV diving and floating motion (in Chinese), Harbin Eng. Univ., 2020. https://doi.org/10.27060/d.cnki.ghbcu.2019.000077 doi: 10.27060/d.cnki.ghbcu.2019.000077
![]() |
[43] | A. Chen, J. Ye, Research on four-layer back propagation neural network for the computation of ship resistance, in 2009 International Conference on Mechatronics and Automation, IEEE, (2009), 2537–2541. https://doi.org/10.1109/icma.2009.5245975 |
[44] |
X. Chen, C. Wei, G. Zhou, H. Wu, Z. Wang, S. A. Biancardo, Automatic identification system (AIS) data supported ship trajectory prediction and analysis via a deep learning model, J. Mar. Sci. Eng., 10 (2022), 1314. https://doi.org/10.3390/jmse10091314 doi: 10.3390/jmse10091314
![]() |
1. | Wenliao Du, Xinlong Yu, Zhen Guo, Hongchao Wang, Yiyuan Gao, Ziqiang Pu, Guanghua Li, Chuan Li, Channel attention residual transfer learning with LLM fine-tuning for few-shot fault diagnosis in autonomous underwater vehicle propellers, 2025, 330, 00298018, 121237, 10.1016/j.oceaneng.2025.121237 |
Point | A | D′ |
Accidental time | 24th May, 10:50 | 28th May, 14:59 |
Position (longitude, latitude) | 110.89136, 17.60279 | 110.48121, 17.18687 |