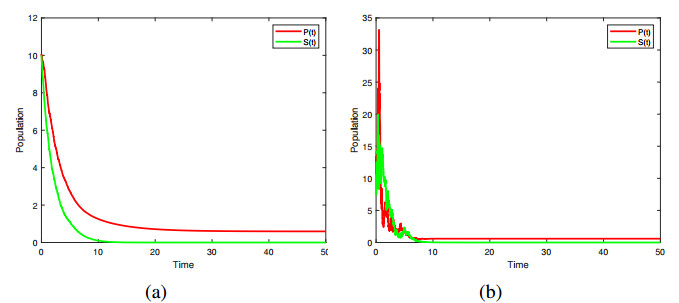
Smoking has gradually become a very common behavior, and the related situation in different groups also presents different forms. Due to the differences of individual smoking cessation time and the interference of environmental factors in the spread of smoking behavior, we establish a stochastic giving up smoking model with quit-smoking duration. We also consider the saturated incidence rate. The total population is composed of potential smokers, smokers, quitters and removed. By using Itô's formula and constructing appropriate Lyapunov functions, we first ensure the existence of a unique global positive solution of the stochastic model. In addition, a threshold condition for extinction and permanence of smoking behavior is deduced. If the intensity of white noise is small, and ˜R0<1, smokers will eventually become extinct. If ˜R0>1, smoking will last. Then, the sufficient condition for the existence of a unique stationary distribution of the smoking phenomenon is studied as Rs0>1. Finally, conclusions are explained by numerical simulations.
Citation: Yajuan Guo, Zijian Liu, Yuanshun Tan, Yawei Liu. Modeling and analysis of a stochastic giving-up-smoking model with quit smoking duration[J]. Mathematical Biosciences and Engineering, 2023, 20(12): 20576-20598. doi: 10.3934/mbe.2023910
[1] | Ahmed Nagah, Asmaa Amer, Xinan Zhang . The effect of cigarette smoking on lung cancer evolution. Mathematical Biosciences and Engineering, 2019, 16(6): 7771-7788. doi: 10.3934/mbe.2019390 |
[2] | Yang Chen, Wencai Zhao . Dynamical analysis of a stochastic SIRS epidemic model with saturating contact rate. Mathematical Biosciences and Engineering, 2020, 17(5): 5925-5943. doi: 10.3934/mbe.2020316 |
[3] | Helong Liu, Xinyu Song . Stationary distribution and extinction of a stochastic HIV/AIDS model with nonlinear incidence rate. Mathematical Biosciences and Engineering, 2024, 21(1): 1650-1671. doi: 10.3934/mbe.2024072 |
[4] | Zhongwei Cao, Jian Zhang, Huishuang Su, Li Zu . Threshold dynamics of a stochastic general SIRS epidemic model with migration. Mathematical Biosciences and Engineering, 2023, 20(6): 11212-11237. doi: 10.3934/mbe.2023497 |
[5] | Zulqurnain Sabir, Muhammad Asif Zahoor Raja, Abeer S. Alnahdi, Mdi Begum Jeelani, M. A. Abdelkawy . Numerical investigations of the nonlinear smoke model using the Gudermannian neural networks. Mathematical Biosciences and Engineering, 2022, 19(1): 351-370. doi: 10.3934/mbe.2022018 |
[6] | Jiying Ma, Shasha Ma . Dynamics of a stochastic hepatitis B virus transmission model with media coverage and a case study of China. Mathematical Biosciences and Engineering, 2023, 20(2): 3070-3098. doi: 10.3934/mbe.2023145 |
[7] | Chen Chen, Guowu Yuan, Hao Zhou, Yutang Ma, Yi Ma . Optimized YOLOv7-tiny model for smoke detection in power transmission lines. Mathematical Biosciences and Engineering, 2023, 20(11): 19300-19319. doi: 10.3934/mbe.2023853 |
[8] | Cristina Anton, Alan Yong . Stochastic dynamics and survival analysis of a cell population model with random perturbations. Mathematical Biosciences and Engineering, 2018, 15(5): 1077-1098. doi: 10.3934/mbe.2018048 |
[9] | Yan Xie, Zhijun Liu . The Unique ergodic stationary distribution of two stochastic SEIVS epidemic models with higher order perturbation. Mathematical Biosciences and Engineering, 2023, 20(1): 1317-1343. doi: 10.3934/mbe.2023060 |
[10] | Ke Qi, Zhijun Liu, Lianwen Wang, Qinglong Wang . Survival and stationary distribution of a stochastic facultative mutualism model with distributed delays and strong kernels. Mathematical Biosciences and Engineering, 2021, 18(4): 3160-3179. doi: 10.3934/mbe.2021157 |
Smoking has gradually become a very common behavior, and the related situation in different groups also presents different forms. Due to the differences of individual smoking cessation time and the interference of environmental factors in the spread of smoking behavior, we establish a stochastic giving up smoking model with quit-smoking duration. We also consider the saturated incidence rate. The total population is composed of potential smokers, smokers, quitters and removed. By using Itô's formula and constructing appropriate Lyapunov functions, we first ensure the existence of a unique global positive solution of the stochastic model. In addition, a threshold condition for extinction and permanence of smoking behavior is deduced. If the intensity of white noise is small, and ˜R0<1, smokers will eventually become extinct. If ˜R0>1, smoking will last. Then, the sufficient condition for the existence of a unique stationary distribution of the smoking phenomenon is studied as Rs0>1. Finally, conclusions are explained by numerical simulations.
The harm of tobacco is one of the most serious public health problems. The World Health Organization has considered the tobacco epidemic in the key control areas of global public health. According to statistics, one person dies of cigarette related diseases every six seconds in the world. With the current trend, the World Health Organization estimates that the number of people will rise to 10 million by 2030, which means one person will die of smoking every three seconds [1]. Not only that, smoking behavior may also bring bad guidance to teenagers. In 2018, the prevalence of smoking among people over 15 years old in China was 26.6%, with the prevalence of smoking among men at 50.5% [2], and the prevalence of underage smoking in China has been increasing in recent years. The harm of smoking to the human body is inevitable. Basically, smoking can prevent the absorption of vitamin C. Long term smoking can lead to cancer and other diseases. In addition, second-hand smoke can also cause cancer and have adverse effects on the heart. Long term intake of second-hand smoke by pregnant women can also lead to fetal growth retardation, congenital malformations and even abortion. Therefore, tobacco control is particularly necessary.
Tobacco control is the most important measure to prevent disease and death at present. Eliminating the harm of smoking has become a worldwide trend. WHO stressed the importance of legislation in tobacco control measures, strongly advocated health education on tobacco control, advocated a healthy lifestyle and recommended that member states increase tobacco taxes and reduce tobacco consumption [3]. Many countries are also strengthening tobacco control through different means, including increasing packaging warnings, increasing the size of warnings, etc. Quitting smoking is a key link to alleviate the harm of smoking. Three days after quitting smoking, nicotine will be basically removed from the body. After quitting smoking for 2 to 3 weeks, lung function will be enhanced. After quitting smoking for 3 to 9 months, the damaged cilia in the lungs will almost be completely repaired. One year after quitting smoking, the probability of coronary heart disease will be 50% lower than that of smokers. Five years after quitting smoking, the probability of stroke will reach the level of non-smokers, and the probability of suffering from other cancers will also be greatly reduced. Therefore, it is particularly necessary to quit smoking. Based on this background, we study a new giving up smoking model.
The development of the research on smoking models is significant. In consideration of the research objects, Castillo-Garsow et al. [4] proposed a system including the potential smokers (P), the chain smokers (S) and the permanently quit smokers (R) in 1997. Later, the mathematical model was promoted by Sharomi and Gumel [5] in 2008. They introduced a new group of smokers who temporarily quit smoking (Qt). In the study of Sharomi and Gumel, they proved the local stability of the equilibrium E∗ by using the Routh-Hurwitz criterion, while the global stability was only speculated by a large number of simulations. Then Lahrouz et al. [6] proved that conclusion by constructing a suitable Lyapunov function in 2011. In addition, random noise was included in the deterministic model, and then the deterministic and stochastic stabilities of a class of random smoking models were considered by them. In the same year, Zaman [7] proposed a smoking cessation model including the occasional smokers (L) and studied its relevant dynamic behavior such as equilibria in the model, one of which was the smoking-free equilibrium, and the other three were about smoking being present under the assumption that the birth rate was different from the mortality rate. Guerrero et al. [8] assumed the population consisted of four types of individuals, non-smokers, normal smokers, excessive smokers and ex-smokers, when they used homotopy analysis method (HAM) to obtain an analytical solution to the model for the spread of the smoking habit in Spain in 2013. In 2015, Sharma et al. [9] considered the impact of media factors on the spread of smoking. A mathematical model was proposed to evaluate the impact of media publicity on the prevalence of social smoking. In 2016, the qualitative behavior of a smoking model was studied by Din et al. [10] through dividing people into non-smokers, smokers, temporarily quit smokers, permanently quit smokers and people who were associated with illness due to smoking. In 2018, Rahman et al. [11] studied an age-structured cigarette smoking model which considered the age of light smokers. They found that smoking had a strong correlation with age. Also, in order to study the optimal control of the problem, Khyar et al. [12] and Din et al. [13] considered adding two control strategies to the model for analysis, respectively, in 2021. Zhang et al. [14] considered a delayed smoking cessation model of relapse in 2022. More relevant analysis about smoking and smoking cessation models can be found in [15,16,17,18,19].
As with most epidemic models [20,21,22] that describe the form of contact, lots of classical giving-up-smoking models also employ the bilinear incidence rate βPS [23,24], nonlinear incidence rate f(P)g(S) [25,26] between potential smokers P(t) and smokers S(t), square-root incidence functions √PL [27,28] or β2PLP+L [12] between potential smokers P(t) and light smokers L(t). As we all know, the bilinear incidence rate is assumed to be proportional to the total population in the environment, which is not practical when the population is large. In the real world, the number of potential smokers in contact with smokers is limited in unit time. Thus, the saturated contact rate is more realistic. Hence, in this paper, we choose the saturated incidence rate βP(t)S(t)1+kS(t) between potential smokers P(t) and smokers S(t). Besides, as described in the second paragraph, there is a strong correlation between the quit smoking duration and the level of personal health recovery. Therefore, we consider the quit smoking duration at the first time, and we also notice that some people might not quit smoking completely in the process of formulating the model. In addition, the conclusion of this paper has great practical significance for smoking cessation guidance and treatment of tobacco-related diseases.
We will introduce the arrangement of this article from the following sections. Based on the above descriptions, we formulate a stochastic giving-up-smoking model with age structure in Section 2. In Section 3, we derive the existence and uniqueness of the solution to the model. Section 4 establishes some conditions to prove the extinction of smoking behavior. Section 5 guarantees the sufficient conditions for the persistence of smoking. We prove the sufficient condition for the existence of a unique stationary distribution of the smoking phenomenon in Section 6. We carry out numerical simulations to prove the results in Section 7. A brief discussion is offered in the end.
Drawing on the transmission of virus from infected vectors to susceptible populations in epidemiological models [29,30], we also define 11+kS(t)≤12 to describe the saturation caused by the psychological effects of changes in potential smokers when the number of smokers becomes large. We formulate a giving-up-smoking model with quit-smoking duration as
{dP(t)dt=λ−(μ+d)P(t)−βP(t)S(t)1+kS(t)+(1−h)∫∞0b(τ)Q(t,τ)dτ,dS(t)dt=βP(t)S(t)1+kS(t)−(μ+d)S(t)−αS(t),∂Q(t,τ)∂t+∂Q(t,τ)∂τ=−(μ+d)Q(t,τ)−b(τ)Q(t,τ),dR(t)dt=−(μ+d)R(t)+h∫∞0b(τ)Q(t,τ)dτ, | (2.1) |
where the total population is divided into four classes: the potential smokers P(t), the smokers S(t), the quitters Q(t) and the removed R(t). λ, μ, d, β, h and α, respectively, denote the recruitment rate of the population, the natural death rate, the mortality rate due to direct or indirect smoking, the effective contact rate between the potential smokers and the smokers, the success rate of quitters and the proportion of quitting smoking. Here, with different smoking cessation deadline, those who successfully quit smoking will enter the removed, while those who show signs of relapse within the smoking cessation deadline will get into the group of potential smokers. Futher, the quitted become the potential smokers with rate 1−h due to environmental effect, weak willpower or other factors and turn to the removed with rate h due to strong willpower. Q(t,τ) denotes the density of quit smokers at time t with quit-smoking duration τ, and ∫∞0Q(t,τ)dτ denotes the number of quit smokers at time t. b(τ) denotes the emigration rate from quitters, which is a bounded function. Here we assume that 0≤b(τ)≤1 and ∫∞0b(τ)dτ=∞, which guarantees that nobody quits smoking when quit-smoking duration τ approaches infinity. In other words, the number of quitters tends to zero when quit-smoking duration τ approaches infinity limτ→∞Q(t,τ)=0.
The initial conditions are
P(0)=P0>0,S(0)=S0>0,Q(0,τ)=Q0(τ),R(0)=R0>0, |
and the boundary condition is
Q(t,0)=αS(t), |
where
P0+S0+R0+∫∞0Q0(τ)dτ=N0. |
Integrating the third equation in model (2.1) along the characteristic line t−τ= constant, we obtain that
Q(t,τ)={αS(t−τ)Γ0(τ),t>τ≥0,Q0(τ−t)Γ0(τ)Γ0(τ−t),τ≥t>0, | (2.2) |
where
Γ0(τ)=exp{−∫τ0ε(ν)dν}=exp{−∫τ0(b(ν)+μ+d)dν}. |
Similar to papers [20,22], for t≥0, we have that
∫∞0b(τ)Q(t,τ)dτ=∫t0b(τ)αS(t−τ)Γ0(τ)dτ+∫∞tb(τ)Q0(τ−t)Γ0(τ)Γ0(τ−t)dτ. | (2.3) |
Noticing that
Γ0(τ)Γ0(τ−t)=exp{−∫ττ−tε(ν)dν}≤exp{−∫ττ−t(μ+d)dν}=e−(μ+d)t, |
then by setting ˆτ=τ−t, we get
∫∞tb(τ)Q0(τ−t)dτ≤∫∞tQ0(τ−t)dτ=∫∞0Q0(ˆτ)dˆτ, |
which yields
e−(μ+d)t∫∞tb(τ)Q0(τ−t)dτ≤e−(μ+d)t∫∞0Q0(τ)dτ<e−(μ+d)tN0→0ast→∞. |
Thus, (2.3) turns to be
∫∞0b(τ)Q(t,τ)dτ=∫∞0b(τ)αS(t−τ)Γ0(τ)dτ=∫∞0Γ(τ)S(t−τ)dτ, | (2.4) |
where
Γ(τ)=αb(τ)Γ0(τ). | (2.5) |
Due to the complexity of the social environment, the contact rate between potential smokers and smokers will be affected by environmental factors. We assume that fluctuations in the environment will manifest themselves mainly as fluctuations in the parameter β, so that β→β+σ˙B(t), where B(t) is a standard Brownian motion with intensity σ2>0. Reference model (2.1) for other parameters, and we finally establish a stochastic giving-up-smoking model with quit-smoking duration as follows:
{dP(t)=(λ−(μ+d)P(t)−βP(t)S(t)1+kS(t)+(1−h)∫∞0Γ(τ)S(t−τ)dτ)dt−σP(t)S(t)1+kS(t)dB(t),dS(t)=(βP(t)S(t)1+kS(t)−(μ+d)S(t)−αS(t))dt+σP(t)S(t)1+kS(t)dB(t). | (2.6) |
The initial condition for system (2.6) takes the form P(θ)=ξ1(θ),S(θ)=ξ2(θ) and (ξ1,ξ2)∈(C(−∞,0],R2+). Without loss of generality, we may assume that ϕ1(θ)≤ϕ1(0) and ϕ2(θ)≤ϕ2(0) for all θ≤0.
We will study the existence and uniqueness of the solution to model (2.6) in this section.
Theorem 3.1. For any initial value (ξ1,ξ2)∈(C(−∞,0],R2+), there exists a unique solution (P(t),S(t)) on R, which will remain in R2+ with probability one.
Proof. Because of the locally Lipschitz continuous of coefficients of model (2.6) for any initial solution, there exists a unique positive local solution on [−∞,τe], where τe is the explosion time. Let n0>1, be a large integer such that P(0) and S(0) are in the interval [1n,n]. For each integer n>n0, to show the solution is global, the stopping time is defined as follows:
τn=inf{t∈[0,τe):P(t)∉(1n,n)orS(t)∉(1n,n)}. |
Then, τn is a nondecreasing function. We define limn→∞τn=τ∞, and τ∞≤τe.
Now, we need to prove τ∞=∞ a.s. If this conclusion is not true, then there exist T>0 and ϵ∈(0,1) such that P{τ∞≤T}≥ϵ. Then, there is an integer n1>n0 such that
P{τn≤T}≥ϵ,n≥n1. | (3.1) |
Let
N(t)=P(t)+S(t)+R(t)+∫∞0Q(t,τ)dτ, | (3.2) |
and we calculate that
dN(t)dt=λ−(μ+d)P(t)−(μ+d)S(t)−(μ+d)R(t)−αS(t)+∫∞0b(τ)Q(t,τ)dτ+ddt∫∞0Q(t,τ)dτ=λ−(μ+d)(P(t)+S(t)+R(t)+∫∞0Q(t,τ)da)−αS(t)+∫∞0b(τ)Q(t,τ)dτ+ddt∫∞0Q(t,τ)dτ+(μ+d)∫∞0Q(t,τ)dτ=λ−(μ+d)N(t)−Q(t,0)−(Q(t,∞)−Q(t,0))=λ−(μ+d)N(t). | (3.3) |
Thus
N(t)=λμ+d+(N(0)−λμ+d)e−(μ+d)t, | (3.4) |
which implies that
limt→∞N(t)≤λμ+d. | (3.5) |
Without loss of generality, we can assume that N(0)≤λμ+d. Thus, for all t≥0, it will be studied in the invariant sets Ω∗={N(t):0≤N(t)≤λμ+d}.
We define the following function V:
V((P(t),S(t))=P(t)−1−lnP(t)+S(t)−1−lnS(t). | (3.6) |
Note that V((P(t),S(t)) is non-negative when ((P(t),S(t))∈R2+, and calculating by Itô's formula on system (2.6) leads to
dV(P(t),S(t))=(1−1P(t))dP(t)+12P2(t)(dP(t))2+(1−1S(t))dS(t)+12S2(t)(dS(t))2=(1−1P(t))((λ−(μ+d)P(t)−βP(t)S(t)1+kS(t)+(1−h)∫∞0Γ(τ)S(t−τ)dτ)dt−σP(t)S(t)1+kS(t)dB(t))+(1−1S(t))((βP(t)S(t)1+kS(t)−(μ+d)S(t)−αS(t))dt+σP(t)S(t)1+kS(t)dB(t))+(12P2(t)+12S2(t))(σP(t)S(t))2(1+kS(t))2dt=(((1−1P(t))(λ−(μ+d)P(t)−βP(t)S(t)1+kS(t)+(1−h)∫∞0Γ(τ)S(t−τ)dτ)+(1−1S(t))(βP(t)S(t)1+kS(t)−(μ+d)S(t)−αS(t))+σ22(1+kS(t))2(S2(t)+P2(t)))dt−(1−1P(t))σP(t)S(t)1+kS(t)dB(t)+(1−1S(t))σP(t)S(t)1+kS(t)dB(t)=LV(P(t),S(t))dt+(1P(t)−1S(t))σP(t)S(t)1+kS(t)dB(t), | (3.7) |
where
LV(P(t),S(t))=λ−(μ+d)P(t)−βP(t)S(t)1+kS(t)+(1−h)∫∞0Γ(τ)S(t−τ)dτ−λP(t)+μ+d+βS(t)1+kS(t)−1−hP(t)∫∞0Γ(τ)S(t−τ)dτ+βP(t)S(t)1+kS(t)−(μ+d)S(t)−αS(t)−βP(t)1+kS(t)+(μ+d)+α+σ22(1+kS(t))2(P2(t)+S2(t))≤λ+(1−h)Γλμ+d+2(μ+d)+βk+α+σ2(λμ+d)2:=K. | (3.8) |
Now that
Γ=∫∞0Γ(τ)dτ=∫∞0αb(τ)Γ0(τ)dτ≤αμ+d, |
thus (3.7) turns to
dV(P(t),S(t))≤Kdt+(1P(t)−1S(t))σP(t)S(t)1+kS(t)dB(t). | (3.9) |
Integrating the inequality (3.9) from 0 to τn∧T yields that
∫τn∧T0dV(P(t),S(t))≤∫τn∧T0Kdt+∫τn∧T0(1P(t)−1S(t))σP(t)S(t)1+kS(t)dB(t). |
This implies that
E[V((P(τn∧T),S(τn∧T))]≤V(P(0),S(0))+KE(τn∧T)≤V(P(0),S(0))+KT. | (3.10) |
Let Ωn=P{τn≤T}, and then from (3.1), we get that P{Ωn≤T}≥ϵ, for n≥n1. For all ω∈Ωn, each component of (P(τn∧T),S(τn∧T)) equals either n or 1n. Consequently, we have that
∞>V(P(0),S(0))+KT≥ϵmin{n−1−lnn,1n−1+lnn}. | (3.11) |
This will lead to
∞>V(P(0),S(0))+KT≥∞ | (3.12) |
as n→∞. Thus, the assertion τe=∞ holds almost surely. The proof is complete.
We will talk about the extinction of the smoking behavior in this section.
Theorem 4.1. Let (P(t),S(t)) be the solution of model (2.6).
(i) If σ2≤β(μ+d)λ, and
˜R0=βλ(μ+d)(μ+d+α)−σ2λ22(μ+d)2(μ+d+α)<1, |
then
lim supt→∞lnS(t)t≤(μ+d+α)(˜R0−1)<0. | (4.1) |
(ii) If σ2≥β22(μ+d+α), then
lim supt→∞lnS(t)t≤β22σ2−(μ+d+α)<0. | (4.2) |
Proof. Consider Theorem 3.1 and Eq (3.5). For any η>0, there is a constant T0 such that
P(t)+S(t)≤λμ+d+η,t≥T0. |
By making use of Itô's formula on the second equation of model (2.6), we obtain that
dlnS(t)=(βP(t)S(t)1+kS(t)−(μ+d)S(t)−αS(t))dt+σP(t)S(t)1+kS(t)dB(t)S(t)−σ2P2(t)S2(t)(1+kS(t))22S2(t)dt=(βP(t)1+kS(t)−(μ+d)−α−σ2P2(t)2(1+kS(t))2)dt+σP(t)1+kS(t)dB(t). | (4.3) |
Integrating from 0 to t on both sides of (4.3), for any ϵ>0, we have
lnS(t)t≤lnS(0)t+β+ϵt∫t0P(r)1+kS(r)dr−(μ+d+α)−σ22t∫t0(P(r)1+kS(r))2dr+σt∫t0P(r)1+kS(r)dB(r). | (4.4) |
Define a function
G(x)=−σ22x2+(β+ϵ)x−(μ+d+α), | (4.5) |
where G(x) is monotonically increasing for x∈[0,β+ϵσ2] and monotonically decreasing for x∈[β+ϵσ2,∞). It is easy to obtain that λμ+d≤βσ2 from condition (i)σ2≤β(μ+d)λ. Now, we choose a positive η≤ϵ such that
P(t)1+kS(t)≤λμ+d+η≤β+ϵσ2, |
and thus we have
G(P(t)1+kS(t))≤G(λμ+d+η) |
for all t≥T0, where T0 is a positive constant number.
Then, for t≥T0, we get that
lnS(t)t⩽lnS(0)t+1t∫t0G(P(r)1+kS(r))dr+σt∫t0P(r)1+kS(r)dB(r)⩽lnS(0)t+1t∫T00G(P(r)1+kS(r)))dr+G(λμ+d)t−T0t+σt∫t0P(r)1+kS(r)dB(r). | (4.6) |
We define
Y1(t)=∫t0P(r)1+kS(r)dB(r), | (4.7) |
which is a local continuous martingale, and Y1(0)=0. Then for t≥T0,
⟨Y1(t),Y1(t)⟩=∫t0(P(r)1+kS(r))2dr≤∫T00(P(r)1+kS(r))2dr+(λμ+d)2(t−T0). |
According to the large number theorem for martingales [31], we obtain that
lim supt→∞⟨Y1(t),Y1(t)⟩t≤(λμ+d)2<∞a.s.lim supt→∞Y1(t)t=0, |
and thus
lim supt→∞lnS(t)t≤G(λμ+d). | (4.8) |
Then, it is implied that, for any ϵ and η, there is
lim supt→∞lnS(t)t≤−σ22(λμ+d)2+βλμ+d−(μ+d+α):=(μ+d+α)(˜R0−1). | (4.9) |
Thus, when ˜R0<1, we have
lim supt→∞lnS(t)t≤(μ+d+α)(˜R0−1)<0. | (4.10) |
Then, (4.1) is proved completely with condition (i).
Now, we prove (4.2), and note that function G(x) gets its maximum value
G(x)max=(β+ϵ)22σ2−(μ+d+α) | (4.11) |
at x=β+ϵσ2. Since
G(P(t)1+kS(t))≤(β+ϵ)22σ2−(μ+d+α), |
we can get that
lnS(t)t≤lnS(0)t+(β+ϵ)22σ2−(μ+d+α)+σt∫t0P(r)1+kS(r)dB(r). | (4.12) |
By a similar discussion, we can obtain that
lim supt→∞lnS(t)t≤(β+ϵ)22σ2−(μ+d+α). | (4.13) |
When ϵ→0 under the condition (ii)σ2≥β22(μ+d+α), we have that
lim supt→∞lnS(t)t<0. | (4.14) |
This completes the proof of (4.2).
We will focus on the permanence of the smoking behavior in this section.
Theorem 5.1. Let (P(t),S(t)) be the solution of model (2.6), and if ˜R0>1, then
lim inft→∞⟨S(t)⟩≥(˜R0−1)(μ+d+α)β(μ+d+αμ+d)+k(μ+d+α). | (5.1) |
Proof. From model (2.6), we obtain that
˙P(t)+˙S(t)=λ−(μ+d)P(t)+(1−h)∫∞0Γ(τ)S(t−τ)dτ−(μ+d)S(t)−αS(t)⩾λ−(μ+d)P(t)−(μ+d)S(t)−αS(t), | (5.2) |
and integrating from 0 to t on both sides of (5.2) yields that
1t(P(t)+S(t))−1t(P(0)+S(0))≥λ−(μ+d)⟨P(t)⟩−(μ+d)⟨S(t)⟩−α⟨S(t)⟩. |
Thus,
⟨P(t)⟩≥λ−(μ+d)⟨S(t)⟩−α⟨S(t)⟩μ+d−φ(t), | (5.3) |
where
φ(t)=P(t)+S(t)−P(0)−S(0)(μ+d)t. |
By using Itô's formula, we get that
d(lnS(t)+kS(t))=(1+kS(t)S(t))((βP(t)S(t)1+kS(t)−(μ+d)S(t)−αS(t))dt+σP(t)S(t)1+kS(t)dB(t))−12S2(t)σ2P2(t)S2(t)(1+kS(t))2dt=(βP(t)−(1+kS(t))(μ+d+α)−σ2P2(t)2(1+kS)2)dt+σP(t)dB(t)⩾(βP(t)−(1+kS(t))(μ+d+α)−σ2λ22(u+d)2)dt+σP(t)dB(t)=(βP(t)−(μ+d+α)−k(μ+d+α)S(t)−σ2λ22(μ+d)2)dt+σP(t)dB(t). | (5.4) |
By integrating (5.4) from 0 to t, we have
lnS(t)−lnS(0)t+kS(t)−S(0)t⩾β⟨P(t)⟩−(μ+d+α)−σ2λ22(μ+d)2−k(μ+d+α)⟨S(t)⟩+σt∫t0P(r)dB(r)⩾β[λ−(μ+d)⟨S(t)⟩−α⟨S(t)⟩μ+d−φ(t)]−(μ+d+α)−σ2λ22(μ+d)2−k(μ+d+α)⟨S(t)⟩+σt∫t0P(r)dB(r)=βλμ+d−(μ+d+α)−σ2λ22(μ+d)2−[β(μ+d+αμ+d)+k(μ+d+α)]⟨S(t)⟩−βφ(t)+σt∫t0P(r)dB(r)=(˜R0−1)(μ+d+α)−[β(μ+d+αμ+d)+k(μ+d+α)]⟨S(t)⟩−βφ(t)+Y2(t)t. | (5.5) |
This inequality can be rewritten as
⟨S(t)⟩≥1l[(˜R0−1)(μ+d+α)−βφ(t)+Y2(t)t−lnS(t)−lnS(0)t−kS(t)−S(0)t], | (5.6) |
where
l=βμ+d+αμ+d+k(μ+d+α),Y2(t)=σ∫t0P(r)dB(r), |
in which Y2(t) is a local continuous martingale with Y2(0)=0. Applying the large number theorem for martingales [31], we obtain that
lim supt→∞⟨Y2(t),Y2(t)⟩t≤(σλμ+d)2<∞a.s.lim supt→∞Y2(t)t=0. | (5.7) |
According to (3.5), we have that
−∞<lnS(t)<lnλμ+d,limt→∞φ(t)=0. | (5.8) |
Thus, (5.1) is proved completely with condition ˜R0>1.
This section will study the stationary distribution of model (2.6).
Let X(t) be a homogeneous Markov process in Rd (Rd denotes a d-dimensional Euclidean space) and be given by following the stochastic differential equation
dX(t)=b(X)dt+k∑r=1gir(X)gjr(X) | (6.1) |
with its diffusion matrix
˜A(X)=(aij(X)),aij(X)=k∑r=1gir(X)gjr(X). | (6.2) |
Lemma 1. [32] The Markov process X(t) has a unique ergodic stationary distribution μ(⋅) if there is a bounded domain D⊂Rd with regular boundary Γ, and
(i) there exists a positive constant ˜M such that ∑di,j=1aijξiξj≥˜M|ξ|2,x∈D,ξ∈Rd;
(ii) there exists a nonnegative C2-function V such that LV is negative for any Rd∖D.
Then,
Px{limT→∞1T∫T0f(X(t))dt=∫Rdf(x)μ(dx)}=1 |
for all x∈Rd, where f(⋅) is a function integrable with respect to the measure μ.
Theorem 6.1. If
Rs0=√2λβk(βk+μ+d+12σ2k2)2>1, |
then model (2.6) admits a unique stationary distribution. Moreover, the stationary distribution is ergodic.
Proof. The diffusion matrix of the stochastic model (2.6) is
˜A(x)=(σ2P2S2(1+kS)200σ2P2S2(1+kS)2). |
Then, there exists a positive constant ˜M=σ2P2S2(1+kS)2, and we know for any (P,S)∈¯Dm, ξ=(ξi,ξj)∈R2+,
d∑i,j=1aij(P,S)ξiξj=σ2P2S2(1+kS)2ξ21+σ2P2S2(1+kS)2ξ22≥˜M‖ | (6.3) |
where \mathbb{\overline{ D}}_m = \left[\frac{1}{m}, m\right] \times \left[\frac{1}{m}, m\right] , and m > 1 is a sufficiently large integer. Then, the condition (i) in Lemma 1 holds.
Next, in order to prove the condition (ii) , we construct a C^2 -function \widetilde{V} :
\begin{equation*} \widetilde{V}(P,S) = \widetilde{N}V_1+V_2+V_3, \end{equation*} |
where
\begin{equation*} V_1 = -\ln P-S,\quad V_2 = -\ln P,\quad V_3 = \frac{1}{\theta+1}(P+S)^{\theta+1}. \end{equation*} |
\widetilde{N} > 0 is a sufficiently large constant and satisfies
\begin{equation*} -\widetilde{N}\Lambda+F_2 \leq -2, \end{equation*} |
where
\begin{equation} \Lambda = (\frac{\beta}{k}+\mu+d+\frac{1}{2} \frac{\sigma^2}{k^2})(R_0^s-1) > 0, \end{equation} | (6.4) |
\begin{equation} \begin{aligned} F_2 = & \sup _{(P, S) \in \mathbb{R}_{+}^2}\left\{-\frac{1}{2} \left[\left(\mu+d \right)-\theta \frac{\sigma^2}{k^2}\right] \times\left(P^{\theta+1}+S^{\theta+1}\right)+\mu+d+\frac{\beta}{k}+\frac{\sigma^2}{2 k^2}+B\right\},\\ \end{aligned} \end{equation} | (6.5) |
\begin{equation} \begin{aligned} B = & \sup _{(P, S) \in \mathbb{R}_{+}^2}\left\{(P+S)^\theta\left[\lambda-(1-h) \frac{\lambda \alpha}{(\mu+d)^2}\right]-\frac{1}{2}\left[\left(\mu+d \right)-\theta \frac{\sigma^2}{k^2}\right] (P+S)^{\theta+1} \right\} < \infty\\ \end{aligned} \end{equation} | (6.6) |
and \theta > 0 is a constant satisfying
\begin{equation*} (\mu+d) > \theta \frac{\sigma^2}{k^2}. \end{equation*} |
It is noted that
\begin{equation*} \lim \limits_{m \rightarrow \infty} \inf \limits_{(P, S) \in \mathbb{R}^2_+\backslash \mathbb{D}_m} \tilde{V}(P, S) = +\infty, \end{equation*} |
and \tilde{V}(P, S) is a continuous function. Then, it has a minimum point (\overline{P}, \overline{S})\in \mathbb{R}^2_+.
Define a nonnegative C^2 -function on V as
\begin{equation*} {V}(P,S) = \widetilde{N}V_1+V_2+V_3-\widetilde{V}( \overline{P},\overline{S}). \end{equation*} |
Applying Itô's formula to V_1 , we get that
\begin{equation} \begin{aligned} \mathcal{L} V_1 = &-\frac{1}{P}\left[\lambda-(\mu+d) P-\frac{\beta P S}{1+k S}+(1-h) \int_0^{\infty} \Gamma(\tau) S(t-\tau) \mathrm{d} \tau\right] \\ &+\frac{1}{2} \frac{\sigma^2 P^2 S^2}{P^2(1+k S)^2}-\left(\frac{\beta P S}{1+k S}-(\mu+d) S-\alpha S\right) \\ \leqslant &-\frac{\lambda}{P}+\mu+d+\frac{\beta S}{1+k S}+\frac{1}{2} \frac{\sigma^2 S^2}{(1+k S)^2}-\frac{\beta P S}{1+k S}+(\mu+d) S+\alpha S \\ \leqslant &-\frac{\lambda}{P}+\mu+d+\frac{\beta}{k}+\frac{\sigma^2}{2 k^2}-\frac{\beta P S}{1+k S}+\left(\mu+d+\alpha\right) S \\ \leqslant &-2 \sqrt{\frac{\lambda \beta}{2k}}+\mu+d+\frac{\beta}{k}+\frac{\sigma^2}{2 k^2}+\left(\mu+d+\alpha\right) S \\ = &-\left(\frac{\beta}{k}+\mu+d+\frac{\sigma^2}{2 k^2}\right)\left(R_0^s-1\right)+\left(\mu+d+\alpha\right) S \\ = &-\Lambda+\left(\mu+d+\alpha\right) S, \end{aligned} \end{equation} | (6.7) |
where \Lambda is given by (6.4). In addition,
\begin{equation} \begin{aligned} \mathcal{L} V_2 = &-\frac{1}{P}\left[\lambda-(\mu+d) P-\frac{\beta P S}{1+k S}+(1-h) \int_0^{\infty} \Gamma(\tau) S(t-\tau) \mathrm{d} \tau\right]\\ &+\frac{1}{2} \frac{\sigma^2 P^2 S^2}{P^2(1+k S)^2}\\ \leqslant &-\frac{\lambda}{P}+\mu+d+\frac{\beta }{k}+\frac{1}{2} \frac{\sigma^2 }{k^2}. \\ \end{aligned} \end{equation} | (6.8) |
\begin{equation} \begin{aligned} \mathcal{L} V_3 = &(P+S)^\theta\left[\lambda-(\mu+d) P+(1-h) \int_0^{\infty} \Gamma(\tau) S(t-\tau) \mathrm{d}\tau-(\mu+d) S-\alpha S\right] \\ &+\frac{\theta}{2}(P+S)^{\theta-1}\left[\frac{\sigma^2 P^2 S^2}{(1+k S)^2}+\frac{\sigma^2 P^2 S^2}{(1+k S)^2}\right] \\ \leq &(P+S)^\theta\left[\lambda+(1-h) \frac{\lambda \alpha}{(\mu+d)^2}\right]-(P+S)^{\theta+1}(\mu+d)+\frac{\theta}{2}(P+S)^{\theta-1} \times 2 \frac{\sigma^2}{k^2}(P+S)^2\\ = &(P+S)^\theta\left(\lambda+(1-h) \frac{\lambda \alpha}{(\mu+d)^2}\right)-\left[(\mu+d)-\theta \frac{\sigma^2}{k^2}\right](P+S)^{\theta+1}\\ \leq &B-\frac{1}{2}\left[(\mu+d)-\theta \frac{\sigma^2}{k^2}\right](P^{\theta+1}+S^{\theta+1}), \end{aligned} \end{equation} | (6.9) |
where B is given by (6.6). From the above discussion, we can derive that
\begin{equation} \begin{aligned} \mathcal{L} V&\leq -\widetilde{N}\Lambda+\widetilde{N}\left(\mu+d+\alpha \right) S-\frac{\lambda}{P} -\frac{1}{2}\left[(\mu+d)-\theta \frac{\sigma^2}{k^2}\right](P^{\theta+1}+S^{\theta+1}) +\mu+d+\frac{\beta }{k}+\frac{1}{2} \frac{\sigma^2 }{k^2}+B. \end{aligned} \end{equation} | (6.10) |
Define \epsilon to be a sufficiently small positive constant. Then, we divide \mathbb{R}^2_+\backslash \mathbb{D}_\epsilon into four domains as follows:
\begin{equation*} \begin{array}{ll} \mathbb{D}_1 = \left\{(P, S) \in \mathbb{R}_{+}^2: 0 \leqslant P < \epsilon\right\}, & \mathbb{D}_2 = \left\{(P, S) \in \mathbb{R}_{+}^2: 0 \leqslant S < \epsilon\right\} ,\\ \end{array} \end{equation*} |
\begin{equation*} \begin{array}{ll} \mathbb{D}_3 = \left\{(P, S) \in \mathbb{R}_{+}^2: P > 1/ \epsilon \right\}, & \mathbb{D}_4 = \left\{(P, S) \in \mathbb{R}_{+}^2: S > 1/\epsilon \right\}. \end{array} \end{equation*} |
We will show that \mathcal{L}V(P, S)\leq -1 on \mathbb{R}^2_+\backslash \mathbb{D}_\epsilon in the above domains.
Case 1. If (P, S) \in \mathbb{D}_1 , it follows that
\begin{equation*} \mathcal{L}V\leq -\frac{\lambda}{P}+F_1, \end{equation*} |
where
\begin{equation*} \begin{aligned} F_1 = \sup _{(P, S) \in \mathbb{R}_{+}^2}\left\{\widetilde{N}\left(\mu+d+\alpha \right) S-\frac{1}{2} \left[\left(\mu+d \right)-\theta \frac{\sigma^2}{k^2}\right] \left(P^{\theta+1}+S^{\theta+1}\right)+\mu+d+\frac{\beta}{k}+\frac{\sigma^2}{2 k^2}+B\right\}.\\ \end{aligned} \end{equation*} |
We take a constant \epsilon > 0 small enough such that -\lambda/\epsilon+F_1 \leq-1 , and then
\begin{equation} \mathcal{L}V \leq -1, \quad (P,S)\in \mathbb{D}_1. \end{equation} | (6.11) |
Case 2. If (P, S) \in \mathbb{D}_2 , it yields that
\begin{equation*} \mathcal{L}V\leq -\widetilde{N}\lambda+\widetilde{N}\left(\mu+d+\alpha \right) S+F_2, \end{equation*} |
where F_2 is given by (6.5). We choose a constant \epsilon > 0 small enough such that \widetilde{N}\left(\mu+d+\alpha \right) \epsilon\leq 1 , and therefore
\begin{equation} \mathcal{L}V \leq -1, \quad (P,S)\in \mathbb{D}_2. \end{equation} | (6.12) |
Case 3. If (P, S) \in \mathbb{D}_3 , we find that
\begin{equation*} \mathcal{L}V\leq -\frac{1}{4}\left[(\mu+d)-\theta \frac{\sigma^2}{k^2}\right](P^{\theta+1}+S^{\theta+1})+F_3 \leq -\frac{1}{4}\left[(\mu+d)-\theta \frac{\sigma^2}{k^2}\right]\frac{1}{\epsilon^{\theta+1}}+F_3, \end{equation*} |
where
\begin{equation*} \begin{aligned} F_3 = & \sup _{(P, S) \in \mathbb{R}_{+}^2}\left\{\widetilde{N}\left(\mu+d+\alpha \right) S-\frac{1}{4} \left[\left(\mu+d \right)-\theta \frac{\sigma^2}{k^2}\right] \left(P^{\theta+1}+S^{\theta+1}\right)+\mu+d+\frac{\beta}{k}+\frac{\sigma^2}{2 k^2}+B\right\}.\\ \end{aligned} \end{equation*} |
We can choose a constant \epsilon > 0 small enough such that
\begin{equation*} -\frac{1}{4}\left[(\mu+d)-\theta \frac{\sigma^2}{k^2}\right]\epsilon^{-\theta-1}+F_3 \leq -1. \end{equation*} |
Then, it follows that
\begin{equation} \mathcal{L}V \leq -1, \quad (P,S)\in \mathbb{D}_3. \end{equation} | (6.13) |
Case 4. If (P, S) \in \mathbb{D}_4 , we get that
\begin{equation} \mathcal{L}V\leq -\frac{1}{4}\left[(\mu+d)-\theta \frac{\sigma^2}{k^2}\right]S^{\theta+1}+F_3 \leq -\frac{1}{4}\left[(\mu+d)-\theta \frac{\sigma^2}{k^2}\right]\frac{1}{\epsilon^{\theta+1}}+F_3\leq -1. \end{equation} | (6.14) |
Hence, from (6.11) to (6.14), we obtain that
\begin{equation} \mathcal{L}V \leq -1, \quad (P,S)\in \mathbb{R}^2_+\backslash \mathbb{D}_\epsilon. \end{equation} | (6.15) |
Then, the condition (ii) in Lemma 1 holds, and the proof is complete.
In this section, some numerical simulations are introduced to verify the main theoretical results of model (2.6).
With the passage of time, people who quit smoking gradually move out. Some people can quit smoking completely and enter the removal group with good results, while others become potential smokers due to poor willpower and other reasons. Obviously, the emigration rate b(\tau) is an increasing function. Here, we let b(\tau) = \frac{\tau}{1+\tau} .
By using the discretization method of the differential equation and stochastic differential equation proposed in [33,34], the corresponding discretization equations are expressed as follows:
\begin{equation*} \begin{array}{l}P_{t+1} = P_t+\left(\lambda-(\mu+d) P_t-\beta \frac{P_t S_t}{1+kS_t}+(1-h)E \right)\Delta t-\frac{P_t S_t}{1+k S_t} \left(\sigma \zeta_t \sqrt{\Delta t}+\frac{\sigma^2}{2}(\zeta_t^2-1) \Delta t \right), \\S_{t+1} = S_t+\left(\beta \frac{P_t S_t}{1+kS_t}-(\mu+d) S_t-\alpha S_t \right)\Delta t+\frac{P_t S_t}{1+k S_t} \left(\sigma \zeta_t \sqrt{\Delta t}+\frac{\sigma^2}{2}(\zeta_t^2-1) \Delta t \right),\end{array} \end{equation*} |
where E = \int_0^{\infty} \Gamma(\tau) S(t-\tau) \mathrm{d}\tau, \Gamma(\tau) = \alpha b(\tau)\exp \left\{-\int_{0}^{\tau}(b(\nu)+\mu+d) \mathrm{d} \nu \right\}, \zeta_t (t = 1, 2, ...) are the Gaussian random variables N(0, 1) .
In our application, we fix \mu = 0.0736 and d = 0.0951 [11]. By changing the values of relevant variables, we obtain the dynamic analysis of smoking under different conditions.
First, we let \lambda = 0.1, \beta = 0.46 [9], k = 0.9, h = 0.36 , \alpha = 0.9 and \sigma = 0.025 with (P(\theta \leq 0), S(\theta \leq 0)) = (P(0), S(0)) = (10, 10) . It is easy to calculate that
\begin{equation*} \widetilde{\mathcal{R}}_0 = \frac{\beta \lambda }{(\mu+d)(\mu+d+\alpha)}-\frac{\sigma^2 \lambda^2}{2(\mu+d)^2(\mu+d+\alpha)} = 0.25 < 1,\quad \sigma^2-\frac{\beta (\mu+d)}{\lambda} = -0.78 < 0. \end{equation*} |
All conditions of (i) of Theorem 4.1 hold. The corresponding densities of the smokers are shown:
\begin{equation*} \limsup\limits _{t \rightarrow \infty} \frac{\ln S(t)}{t} \leq -0.80 < 0, \end{equation*} |
which implies the smoking class will disappear. Figure 1(a) shows this result. Further, if we choose \sigma = 0.89 and other variables remain unchanged, then \sigma^2-\frac{\beta^2}{2(\mu+d+\alpha)} = 0.69 > 0 , and \sigma^2-\frac{\beta (\mu+d)}{\lambda} = 0.016 > 0 , which means the condition (ii) of Theorem 4.1 holds but opposite to condition (i) . Also
\begin{equation*} \limsup \limits_{t \rightarrow \infty} \frac{\ln S(t)}{t} \leq -0.94 < 0, \end{equation*} |
which implies that the smoking behavior is extinct. Figure 1(b) shows it. Therefore, theoretical results and numerical simulations show that the number of smokers will eventually tend to zero as t \rightarrow \infty.
Learning from Figure 1(b), we get that the number of smokers in the early stage may be larger than that of potential smokers, but eventually tends to being extinct. It can improve the speed of smokers tending to be extinct when the value of \sigma increases.
To illustrate the permanence of smoking behavior, we take \lambda = 100 and \sigma = 0.025 with (P(\theta \leq 0), S(\theta \leq 0)) = (P(0), S(0)) = (140,140) . The other parameters are the same as those in Theorem 2. In this case, \widetilde{\mathcal{R}}_0 = 152.47 > 1 , which satisfies the condition of Theorem 5.1. The smoking behavior is persistent. See Figure 2.
Comparing Figure 1(a) and Figure 2, we find that changing the value of the recruitment rate of the population \lambda will change the dynamic behavior of smoking from an extinct state to a persistent state.
Further, we let \lambda = 0.8, \sigma = 0.1 , and other data are the same as Theorem 5.1. We can easily calculate R_0^s = 1.32 > 1 and \mu+d-\frac{\sigma^2}{k^2} = 0.16 > 0 . Then, from Theorem 6.1, there exists an ergodic stationary distribution of model (2.6). Figure 3(a), (b) present the ergodic stationary distributions of P(t) and S(t) , respectively.
In the following, we study the changes of P(t) and S(t) by respectively changing the values of \beta , \alpha , h and \sigma . Figures 4(a), 5(a), 6(a), 7(a) represent the changes of P(t) and S(t) under the state of extinction as variables change, while Figures 4(b), 5(b), 6(b), 7(b) show the changes under the state of permanence.
Considering that the effective contact rate \beta will be affected by environmental disturbance, we analyze the impact of its change on the populations of potential smokers and smokers. In Figure 4(a), P_1(t) and S_1(t) represent the population when \beta = 0.88 , while P_2(t) and S_2(t) show the quantity when \beta = 0.46 . Learned from it, we know that by reducing the effective contact rate \beta , the number of smokers will significantly reduce while the quantity of potential smokers is increasing. Figure 4(b) plots the permanence of smoking under the same conditions as Figure 4(a). It follows from Figure 4(b) that smokers will decrease and potential smokers will raise along with the decrease of \beta . Also, we find that the quantity gap between P(t) and S(t) becomes larger when \beta decreases in Figure 4(b).
Due to the multifaceted impact of the proportion of quitting smoking \alpha , we analyze the changes in the populations under different smoking cessation rates in Figure 5. P_1(t) and S_1(t) denote the populations when \alpha = 0.36 , while P_2(t) and S_2(t) represent the populations when \alpha = 0.9 in Figure 5(a). From it, we can obtain that the smokers are less, while potential smokers become more, when \alpha increases. In Figure 5(b), P_1(t) and S_1(t) denote the population when \alpha = 0.1 , while P_2(t) and S_2(t) represent the population when \alpha = 0.9 . As \alpha goes larger, more smokers become potential smokers or removed. It is interesting that the proportions of potential smokers and smokers are different with the change of \alpha . The number of smokers is larger than that of potential smokers when the rate of quitting smoking is low. As the rate of quitting smoking increases, the number of smokers will decrease and eventually be lower than that of potential smokers.
In the following, we discuss the effects on P(t) and S(t) when the success rate of quitters h changes. In Figure 6(a), (b), P_1(t) and S_1(t) show the population when h = 0.1 , while P_2(t), S_2(t) represents the population when h = 0.9 . From Figure 6(a), we get that the number of smokers naturally decreases with the success rate of quitters increasing. So, there are fewer smokers turning into potential smokers, and then potential smokers also show a downward trend. Similarly, as h increases, the number of smokers and potential smokers in Figure 6(b) will reduce, too.
We choose three sets of white noise data: (i)\ \sigma_1 = 0.025, (ii)\ \sigma_2 = 0.36 and (iii)\ \sigma_3 = 0 in Figure 7(a). Note that the extinction time would be different for a distinct noise intensity, but smokers will eventually disappear under the condition \widetilde{\mathcal{R}}_0 < 1 . Besides, we take three sets of white noise data: (i) \ \sigma_1 = 0.025, (ii) \ \sigma_2 = 0.01 and (iii)\ \sigma_3 = 0 in Figure 7(b). We find that no matter how \sigma changes, it always surrounds \sigma = 0 , and the fluctuation of population becomes small with the decrease of white noise \sigma .
We studied a stochastic giving-up-smoking model considering the quit-smoking duration and saturated incidence rate in this paper, where the quit smoking duration varies with different individuals, and the quit smokers can eventually become the potential smokers or be removed. Further, the effective contact rate \beta between potential smokers and smokers is described by \beta+\sigma \dot{B}(t) . We first discussed the existence and uniqueness of the solution of model (2.6) (Theorem 3.1). By adding the analysis of environmental interference, we obtained the threshold \widetilde{\mathcal{R}}_0 of extinction (Theorem 4.1) and permanence (Theorem 5.1) of smoking behavior. We conclude that when the white noise is small and satisfies \widetilde{\mathcal{R}}_0 < 1 , the smoking behavior goes extinct in the long run. Figure 1(a) shows this result. The smokers also disappear when the perturbation becomes large enough, which is illustrated in Figure 1(b). The threshold \widetilde{\mathcal{R}}_0 < 1 is sufficient for the persistence of smoking and Figure 2 expresses it. We also derived sufficient conditions R_0^s > 1 for the existence and uniqueness of an ergodic stationary distribution (Theorem 6.1) for the positive solution. Figure 3 presents that conclusion by showing the histogram and the probability of potential smokers and smokers for the stochastic model (2.6). Finally, we demonstrated these conclusions by conducting numerical simulations.
There are four key parameters, the effective contact rate \beta between potential smokers and smokers, the proportion of quitting smoking \alpha , the success rate of quitters h and the intensity of white noise \sigma , that have been emphasized about the extinction and permanence of smoking behavior in our numerical work. Through the analysis of the influence of these variables in Figures 4–7, we can get that the change trends of extinction and persistence affected by variables are the same. In addition, we know by that reducing the effective contact rate \beta between potential smokers and smokers (see Figure 4), increasing the proportion of quitting smoking \alpha (see Figure 5) and strengthening the willpower of quitters to increase the success rate of quitters h (see Figure 6), we can reduce the number of smokers. Therefore, we can take the above measures to reduce the number of smokers in society and create a healthier living environment. Finally, we analyzed the comparison between deterministic and stochastic models in Figure 7 and get that the fluctuation of the population will become large with the increase of white noise \sigma .
Because the quit-smoking duration is raised for the first time, the research content is somewhat limited in the text. Still there is much interesting research to do. In our future work, we will continue to discuss a related project, such as adding different control factors to the model to show the application of control factors in tobacco control work, considering the impact of environmental interference on other factors in the model and so on. Therefore there is still a lot of work to be done in the future.
The authors declare they have not used artificial intelligence (AI) tools in the creation of this article.
This research was supported by the National Natural Science Foundation of China (12271068), the Innovation Development Joint Funds of the Chongqing Natural Science Foundation (CSTB2022NSCQ-LZX0026), Joint Training Base Construction Project for Graduate Students in Chongqing (JDLHPYJD2021016) and Group Building Scientific Innovation Project for universities in Chongqing (CXQT21021).
The authors declare that they have no known competing financial interests or personal relationships that could have appeared to influence the work reported in this paper.
[1] |
B. L. Zhao, Smoking, free radicals and health, Acta. Bioph. Sin., 28 (2012), 332–340. https://doi.org/10.3724/SP.J.1260.2012.20042 doi: 10.3724/SP.J.1260.2012.20042
![]() |
[2] |
Y. Qi, Q. Yan, L. J. Sun, D. L. Yang, C. Y. Luo, Trends of smoking and drinking behaviors among adolescents in Shanghai from 2004 to 2019, Chin. J. Sch. Health., 43 (2022), 1003–1006. https://doi.org/10.16835/j.cnki.1000-9817.2022.07.011 doi: 10.16835/j.cnki.1000-9817.2022.07.011
![]() |
[3] | J. H. Li, J. F. Liu, Current research on hazards of smoking to human health, Int. J. Intern. Med., 35 (2008), 284–287. |
[4] | C. Castillo-Garsow, G. Jordan-Salivia, A. Rodriguez-Herrera, Mathematical models for the dynamics of tobacco use, recovery, and relapse, Technical Report Series BU-1505-M, Cornell University, 1997. |
[5] |
O. Sharomi, A. B. Gumel, Curtailing smoking dynamics: A mathematical modeling approach, Appl. Math. Comput., 195 (2008), 475–499. https://doi.org/10.1016/j.amc.2007.05.012 doi: 10.1016/j.amc.2007.05.012
![]() |
[6] |
A. Lahrouz, L. Omari, D. Kiouach, A. Belmaati, Deterministic and stochastic stability of a mathematical model of smoking, Stat. Probab. Lett., 81 (2011), 1276–1284. https://doi.org/10.1016/j.spl.2011.03.029 doi: 10.1016/j.spl.2011.03.029
![]() |
[7] |
G. Zaman, Qualitative behavior of giving up smoking models, Bull. Malays. Math. Sci. Soc., 34 (2011), 403–415. https://doi.org/10.1155/2011/214289 doi: 10.1155/2011/214289
![]() |
[8] |
F. Guerrero, F. J. Santonja, R. J. Villanueva, Solving a model for the evolution of smoking habit in Spain with homotopy analysis method, Nonlinear Anal. RWA., 14 (2013), 549–558. https://doi.org/10.1016/j.nonrwa.2012.07.015 doi: 10.1016/j.nonrwa.2012.07.015
![]() |
[9] |
A. Sharma, A. K. Misra, Backward bifurcation in a smoking cessation model with media campaigns, Appl. Math. Model., 39 (2015), 1087–1098. http://dx.doi.org/10.1016/j.apm.2014.07.022 doi: 10.1016/j.apm.2014.07.022
![]() |
[10] |
Q. Din, M. Ozair, T. Hussain, U. Saeed, Qualitative behavior of a smoking model, Adv. Differ. Equation, (2016), 96. http://dx.doi.org/10.1186/s13662-016-0830-6 doi: 10.1186/s13662-016-0830-6
![]() |
[11] |
G. U. Rahman, R. Agarwal, L. Liu, A. Khan, Threshold dynamics and optimal control of an age-structured giving up smoking model, Nonlinear Anal. RWA., 43 (2018), 96–120. https://doi.org/10.1016/j.nonrwa.2018.02.006 doi: 10.1016/j.nonrwa.2018.02.006
![]() |
[12] |
O. Khyar, J. Danane, K. Allali, Mathematical analysis and optimal control of giving up the smoking model, Int. J. Differ. Equation, (2021), 8673020. https://doi.org/10.1155/2021/8673020 doi: 10.1155/2021/8673020
![]() |
[13] |
A. Din, P. Liu, T. Cui, Stochastic stability and optimal control analysis for a tobacco smoking model, Appl. Comput. Math., 10 (2021), 163–185. https://doi.org/10.11648/j.acm.20211006.15 doi: 10.11648/j.acm.20211006.15
![]() |
[14] |
Z. Z. Zhang, W. S. Zhang, A time-delayed giving up smoking model with relapse, J. Jishou University (Natural Sciences Edition), 43 (2022), 1–9. https://doi.org/10.13438/j.cnki.jdzk.2022.01.001 doi: 10.13438/j.cnki.jdzk.2022.01.001
![]() |
[15] | R. Ullah, M. Khan, G. Zaman, S. Islam, T. Gul, Dynamical features of a mathematical model on smoking, J. Appl. Environ. Biol. Sci., 6 (2016), 92–96. |
[16] |
G. Zaman, Optimal campaign in the smoking dynamics, Comput. Math. Methods. Med., (2011), 163834. https://doi.org/10.1155/2011/163834 doi: 10.1155/2011/163834
![]() |
[17] |
Z. Alkhudhari, S. Al-Sheikh, S. Al-Tuwairqi, Global dynamics of a mathematical model on smoking, ISRN Appl. Math., (2014), 847075. http://dx.doi.org/10.1155/2014/847075 doi: 10.1155/2014/847075
![]() |
[18] |
H. Alrabaiah, A. Zeb, E. Alzahrani, K. Shah, Dynamical analysis of fractional-order tobacco smoking model containing snuffing class, Alexandr. Eng. J., 60 (2021), 3669–3678. https://doi.org/10.1016/j.aej.2021.02.005 doi: 10.1016/j.aej.2021.02.005
![]() |
[19] |
G. Rahman, R. R. Agarwal, Q. Din, Mathematical analysis of giving up smoking model via harmonic mean type incidence rate, Appl. Math. Comput., 354 (2019), 128–148. https://doi.org/10.1016/j.amc.2019.01.053 doi: 10.1016/j.amc.2019.01.053
![]() |
[20] |
R. X. Lu, F. Y. Wei, Persistence and extinction for an age-structured stochastic SVIR epidemic model with generalized nonlinear incidence rate, Phys. A, 513 (2019), 572–587. https://doi.org/10.1016/j.physa.2018.09.016 doi: 10.1016/j.physa.2018.09.016
![]() |
[21] |
Y. N. Zhao, D. Q. Jiang, The threshold of a stochastic SIRS epidemic model with saturated incidence, Appl. Math. Lett., 34 (2014), 90–93. http://dx.doi.org/10.1016/j.aml.2013.11.002 doi: 10.1016/j.aml.2013.11.002
![]() |
[22] |
X. Ran, L. Hu, L. F. Nie, Z. D. Teng, Effects of stochastic perturbation and vaccinated age on a vector-borne epidemic model with saturation incidence rate, Appl. Math. Comput., 394 (2021), 125798. https://doi.org/10.1016/j.amc.2020.125798 doi: 10.1016/j.amc.2020.125798
![]() |
[23] |
J. H. He, Q. Hou, Dynamic analysis of smoking transmission model, J. Southwest China Normal Univ., 46 (2021), 9–15. https://doi.org/10.13718/j.cnki.xsxb.2021.07.002 doi: 10.13718/j.cnki.xsxb.2021.07.002
![]() |
[24] |
S. W. Teklu, B. B. Terefe, Mathematical modeling analysis on the dynamics of university students animosity towards mathematics with optimal control theory, Sci. Rep., 12 (2022), 11578. https://doi.org/10.1038/s41598-022-15376-3 doi: 10.1038/s41598-022-15376-3
![]() |
[25] |
X. Wang, B. L. Li, Q. Ge, A giving up smoking model with general nonlinear incidence rate, J. Xinyang Normal Univ., 32 (2019), 362–366. https://doi.org/10.3969/j.issn.1003-0972.2019.03.004 doi: 10.3969/j.issn.1003-0972.2019.03.004
![]() |
[26] | Z. M. Li, N. Y. Su, T. L. Zhang, Analysis on a giving up smoking model with nonlinear infection rate, Math. Pract. Theory, 49 (2019), 262–268. |
[27] |
A. Zeb, G. Zaman, S. Momani, Square-root dynamics of a giving up smoking model, Appl. Math. Model., 37 (2013), 5326–5334. http://dx.doi.org/10.1016/j.apm.2012.10.005 doi: 10.1016/j.apm.2012.10.005
![]() |
[28] |
Z. Z. Zhang, R. B. Wei, W. J. Xia, Dynamical analysis of a giving up smoking model with time delay, Adv. Differ. Equation, (2019), 505. https://doi.org/10.1186/s13662-019-2450-4 doi: 10.1186/s13662-019-2450-4
![]() |
[29] |
X. B. Liu, L. J. Yang, Stability analysis of an SEIQV epidemic model with saturated incidence rate, Nonlinear Anal. RWA., 13 (2012), 2671–2679. https://doi.org/10.1016/j.nonrwa.2012.03.010 doi: 10.1016/j.nonrwa.2012.03.010
![]() |
[30] |
Y. M. Chen, S. F. Zou, J. Y. Yang, Global analysis of an SIR epidemic model with infection age and saturated incidence, Nonlinear Anal. RWA., 30 (2016), 16–31. http://dx.doi.org/10.1016/j.nonrwa.2015.11.001 doi: 10.1016/j.nonrwa.2015.11.001
![]() |
[31] | X. Mao, Stochastic Differential Equations and Applications, (second ed.), Horwood Publishing, Chichester, UK, 2007. |
[32] | R. Z. Khas, Miniskii, Stochastic Stability of Differential Equations, Sijthoff Noordhoff, The Netherlands, 1980. |
[33] |
D. J. Higham, An algorithmic introduction to numerical simulation of stochastic differential equations, SIAM Rev., 43 (2001), 525–546. https://doi.org/10.1137/s0036144500378302 doi: 10.1137/s0036144500378302
![]() |
[34] | S. Ania, V. Arnutu, V. Capasso, An Introduction to Optimal Control Problems in Life Sciences, Spring Science, New York, 2011. |