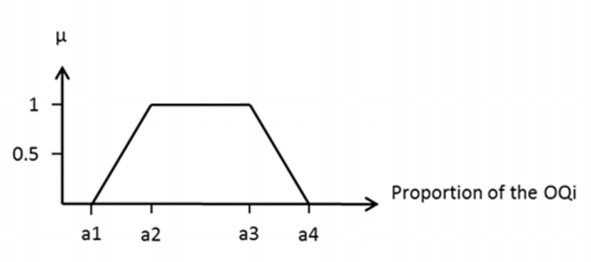
Odor is central to food quality. Still, a major challenge is to understand how the odorants present in a given food contribute to its specific odor profile, and how to predict this olfactory outcome from the chemical composition. In this proof-of-concept study, we seek to develop an integrative model that combines expert knowledge, fuzzy logic, and machine learning to predict the quantitative odor description of complex mixtures of odorants. The model output is the intensity of relevant odor sensory attributes calculated on the basis of the content in odor-active comounds. The core of the model is the mathematically formalized knowledge of four senior flavorists, which provided a set of optimized rules describing the sensory-relevant combinations of odor qualities the experts have in mind to elaborate the target odor sensory attributes. The model first queries analytical and sensory databases in order to standardize, homogenize, and quantitatively code the odor descriptors of the odorants. Then the standardized odor descriptors are translated into a limited number of odor qualities used by the experts thanks to an ontology. A third step consists of aggregating all the information in terms of odor qualities across all the odorants found in a given product. The final step is a set of knowledge-based fuzzy membership functions representing the flavorist expertise and ensuring the prediction of the intensity of the target odor sensory descriptors on the basis of the products' aggregated odor qualities; several methods of optimization of the fuzzy membership functions have been tested. Finally, the model was applied to predict the odor profile of 16 red wines from two grape varieties for which the content in odorants was available. The results showed that the model can predict the perceptual outcome of food odor with a certain level of accuracy, and may also provide insights into combinations of odorants not mentioned by the experts.
Citation: N. Mejean Perrot, Alice Roche, Alberto Tonda, Evelyne Lutton, Thierry Thomas-Danguin. Predicting odor profile of food from its chemical composition: Towards an approach based on artificial intelligence and flavorists expertise[J]. Mathematical Biosciences and Engineering, 2023, 20(12): 20528-20552. doi: 10.3934/mbe.2023908
[1] | Vinh Huy Chau . Powerlifting score prediction using a machine learning method. Mathematical Biosciences and Engineering, 2021, 18(2): 1040-1050. doi: 10.3934/mbe.2021056 |
[2] | Rachael C. Adams, Behnam Rashidieh . Can computers conceive the complexity of cancer to cure it? Using artificial intelligence technology in cancer modelling and drug discovery. Mathematical Biosciences and Engineering, 2020, 17(6): 6515-6530. doi: 10.3934/mbe.2020340 |
[3] | Marco Roccetti . Predictive health intelligence: Potential, limitations and sense making. Mathematical Biosciences and Engineering, 2023, 20(6): 10459-10463. doi: 10.3934/mbe.2023460 |
[4] | Sujuan Zhou, Huajian Lin, Jiang Meng . Discrimination and chemical composition quantitative model of Raw Moutan Cortex and Moutan Cortex Carbon based on electronic nose and machine learning. Mathematical Biosciences and Engineering, 2022, 19(9): 9079-9097. doi: 10.3934/mbe.2022422 |
[5] | Zihan Chen, Minhui Yang, Yuhang Wen, Songyan Jiang, Wenjun Liu, Hui Huang . Prediction of atherosclerosis using machine learning based on operations research. Mathematical Biosciences and Engineering, 2022, 19(5): 4892-4910. doi: 10.3934/mbe.2022229 |
[6] | Ivan Izonin, Nataliya Shakhovska . Special issue: informatics & data-driven medicine-2021. Mathematical Biosciences and Engineering, 2022, 19(10): 9769-9772. doi: 10.3934/mbe.2022454 |
[7] | Anastasia-Maria Leventi-Peetz, Kai Weber . Probabilistic machine learning for breast cancer classification. Mathematical Biosciences and Engineering, 2023, 20(1): 624-655. doi: 10.3934/mbe.2023029 |
[8] | Liang-Sian Lin, Chen-Huan Kao, Yi-Jie Li, Hao-Hsuan Chen, Hung-Yu Chen . Improved support vector machine classification for imbalanced medical datasets by novel hybrid sampling combining modified mega-trend-diffusion and bagging extreme learning machine model. Mathematical Biosciences and Engineering, 2023, 20(10): 17672-17701. doi: 10.3934/mbe.2023786 |
[9] | Qiongyang Zhou, Tianyu Zhao, Kaidi Feng, Rui Gong, Yuhui Wang, Huijun Yang . Artificial intelligence in acupuncture: A bibliometric study. Mathematical Biosciences and Engineering, 2023, 20(6): 11367-11378. doi: 10.3934/mbe.2023504 |
[10] | Ahmed Ali, Ahmad Salah, Mahmoud Bekhit, Ahmed Fathalla . Divide-and-train: A new approach to improve the predictive tasks of bike-sharing systems. Mathematical Biosciences and Engineering, 2024, 21(7): 6471-6492. doi: 10.3934/mbe.2024282 |
Odor is central to food quality. Still, a major challenge is to understand how the odorants present in a given food contribute to its specific odor profile, and how to predict this olfactory outcome from the chemical composition. In this proof-of-concept study, we seek to develop an integrative model that combines expert knowledge, fuzzy logic, and machine learning to predict the quantitative odor description of complex mixtures of odorants. The model output is the intensity of relevant odor sensory attributes calculated on the basis of the content in odor-active comounds. The core of the model is the mathematically formalized knowledge of four senior flavorists, which provided a set of optimized rules describing the sensory-relevant combinations of odor qualities the experts have in mind to elaborate the target odor sensory attributes. The model first queries analytical and sensory databases in order to standardize, homogenize, and quantitatively code the odor descriptors of the odorants. Then the standardized odor descriptors are translated into a limited number of odor qualities used by the experts thanks to an ontology. A third step consists of aggregating all the information in terms of odor qualities across all the odorants found in a given product. The final step is a set of knowledge-based fuzzy membership functions representing the flavorist expertise and ensuring the prediction of the intensity of the target odor sensory descriptors on the basis of the products' aggregated odor qualities; several methods of optimization of the fuzzy membership functions have been tested. Finally, the model was applied to predict the odor profile of 16 red wines from two grape varieties for which the content in odorants was available. The results showed that the model can predict the perceptual outcome of food odor with a certain level of accuracy, and may also provide insights into combinations of odorants not mentioned by the experts.
● An original model has been developed to predict odor perception from chemical composition.
● Gas Chromatography Olfactometry data is used to predict the intensity of odor attributes.
● The inclusive model combines expert knowledge, fuzzy logic, and machine learning.
● The model was applied to predict the odor profile of 16 red wines.
Knowledge about flavor holds strategic importance for the entire food industry because it serves as a major criterion for the formulation and reformulation of food. This, in turn, contributes to the adaptation of food products and beverages to the increasing constraints related to nutritional, organoleptic, and environmental consumers' expectations. Among the sensory dimensions involved in food flavor, the odor component plays a critical role because it determines most of the time the identity and the typicality of food. This influence strongly impacts the overall quality and recognition of the food by consumers [1,2]. Odors and smells also have a major impact on overall perception, as highlighted by studies on the impact of scent on virtual reality experiences [3].
Odors are perceptions resulting from the detection of specific volatile compounds by the receptors of the sense of smell. These odor-active compounds are called odorants [4]. Considering flavor analysis, on the one hand, a food odor profile can be established by sensory analysis which consists in evaluating the sensory characteristics of food through the sense of smell [5]. On the other hand, the chemical analysis of the odorants from food is performed by separating, identifying, and quantifying odorants in food products with techniques such as gas chromatography-mass spectrometry - olfactometry (GC-MS-O). This well-established analytical procedure provides a list of odorants [6,7,8,9], but does not give any information about the perceptual influence of mixed odorants, still critical to the overall food odor construction. Indeed, odorants can compete for the activation of olfactory receptors, forming the foundation of the combinatorial coding of olfactory information. However, several other non-linear integrative processes can occur along the olfactory pathway to the brain, contributing to the ultimate mapping of odor representation [10].
The odor of food is actually due to a complex mixtures of odorants often recognized as single percepts by the human olfactory system (e.g. coffee odor). This percept results from the configural processing of odor mixtures and we are also able to discriminate odors nuances within the complex mixtures (e.g. off-odors) through elemental processing [10]. Because of these complex and still poorly understood perceptual integration processes, it remains very difficult to predict the odor profile of a given food product based on its chemical composition. In flavor analysis, deciphering the chemical base of an odor requires performing recombination and omission tests. Subsequently, researchers can identify the odorants that hold greater relevance within the context of the mixture and their likely associations with specific odor nuances ([11] / wine: [12,13,14,15] / sesame: [16]).]). However, accurately modeling the perceived odor remains a challenging task.
On the one hand, predictive approaches based on the molecular structure of odorants were developed to predict odor characteristics of new sets of odorants (intensity: [17,18] / detection threshold: [19] / perceptual qualities: [20] / pleasantness: [21,22]). Other strategies implement machine learning algorithms in order to predict several odor characteristics of odorants based on odorants' structural parameters ([20,23]). Although these predictive approaches were successful, they are all applied to single odorants. To date only one study, [24] focuses on odorant mixtures by predicting the similarity of multi-molecular mixtures from their structural parameters. However, to the best of our knowledge, no study has attempted to predict how a multi-molecular mixture will smell, i.e. to generate a quantitative sensory description of the odor of mixtures using natural language descriptors.
On the other hand, numerous studies have attempted to model distinct odor nuances, for a large variety of food, on the basis of the volatile compound composition. These studies often utilized multivariate linear models eg. [25,26,27]. These approaches confirmed the existence of complex multivariate relationships between chemicals and odors; they have succeeded in predicting certain sensory attributes through specific combinations of flavor compounds. Nevertheless, they have fallen short of comprehensively modeling the impact of flavor compounds on all nuances of the flavor profile or the overall configural odor perception. Indeed, linear approaches often struggle to account for both elemental and configural perceptions of odorants due to their limited capacity to capture the complex interactions within odor mixtures. Linear methods tend to overlook these intricate interactions, missing the holistic perception that emerges from the synergistic or inhibitory effects of different odorants activating simultaneously the olfactory system.
Because simple or linear approaches (e.g. summation) are not fully adequate for addressing elemental and configural perceptions of odorants' mixtures, we propose a new predictive model that combines the knowledge of flavorists and the expertise of scientists in the flavor domain. Indeed, how flavorists combine odor qualities (e.g. Fruity, Green, Smoky), odor-active raw materials, or pure odorants to create a target odor represents their specialized knowledge. This expertise relies on a personal process ([28]) while also being grounded in fundamental principles acquired through intense training in specialized schools (e.g. based on the field of odors described by [29]) or companies (e.g. Sense ItTM, a global flavor language developed by Givaudan), resulting in a reverse descriptive understanding of odor construction. Interestingly, multimedia approaches have also been used to collect human expertise related to food [30]. By integrating databases, mathematical methods inspired by scientific knowledge, and the expertise of flavorists, we aim for a deeper understanding of flavor creation and, consequently, the construction of odor profiles. In a food-related application ([31]), the knowledge of cheese ripening experts was formalized into an in-silico model and coupled to a chain of mathematical treatments using fuzzy logic. This approach has also proven successful in various applications that seek to incorporate expert knowledge [32]. This is why our paper introduces an approach based on fuzzy logic.
Fuzzy logic is an extension of the set theory where the classical characteristic function of a set (which uses a binary approach of 0 or 1 to indicate if an element belongs to a set or not) is replaced by a membership function whose values range from 0 to 1 ([33]). Transitions between sets enable representing the representation of gradual concepts (e.g. gradual memberships) as well as the representation of rules, particularly adapted to the representation of expertise ([34]). Moreover, analogies exist between fuzzy entities and sensory entities (i.e. sensory scales as fuzzy sets, sensory attributes as fuzzy variables, and sensory answers as membership grades) ([35,36]), which explains why fuzzy logic has been used successfully used in several papers dealing with food systems ([32]). For instance, it has been applied to estimate the sensory properties of food products (sausage: [37] / chhana podo: [38] / tea liquor: [39]/ jam: [40]) and predict consumer food acceptance (biscuits: [36] / bread: [41]).
This article describes a proof-of-concept. It tackles the challenge of predicting odor profiles of real food from their chemical compositions. We explain how a predictive model was built using a combination of machine learning and fuzzy logic, while integrating expert knowledge and domain databases. The outcome is a formal representation of optimized combinations of sensory-relevant odors. We believe this model can further streamline aroma analysis procedures by integrating expert knowledge on odor mixture perception. We exemplify this approach by predicting the odor profiles of 16 red wines made from two grape varieties, based on their chemical compositions in odorants.
Chemical and sensory characterizations of the wines were published in a data paper [42]. In short, Gas Chromatography Olfactometry (GC-O) and Gas Chromatography-Mass Spectrometry (GC-MS) results were obtained for a set of 16 French red wines, 8 from Pinot Noir (PN) and 8 from Cabernet Franc (CF), which were selected to cover the olfactory diversity of the commercial offer for each grape variety [43]. The analyses provided the identification of a total of 46 odorant zones. The GC-O procedure assigned relative importance to each odor-active compound by calculating their nasal impact frequency (NIF), which is the ratio of the number of panelists who smelled an odor to the total number of panelists [44]. In parallel to the chemical analyses, the wines were submitted to a wine sensory descriptive panel composed of 16 trained panelists (6 women; age range 35-71; UR GRAPPE, Angers, France) that performed an orthonasal quantitative descriptive analysis to construct the wines'odor profiles (see details in [42]).
For the odorants identified in the 16 wines, the corresponding odor descriptors were obtained from three databases: Arctander's handbook [45], Flavor-Base (Leffingwell & Associates, http://www.leffingwell.com) and The good scents company [46].
Four senior flavorists participated in the data collection. The elicitation process was based on a private guided interview which lasts 1 hour. The experts received monadically each of the 15 odor sensory attributes (OSA) used by the descriptive panel to build the wines' sensory profiles. The experts were not informed that the OSA were related to wines. For each OSA, they were asked to indicate the odor notes, further called odor qualities (OQ), needed to construct a given odor sensory attribute (OSA).
Four senior flavorists participated in the expert knowledge data collection. The elicitation process was based on a private guided interview which lasted 1 hour. The experts received monadically each of the 15 odor sensory attributes (OSA) used by the descriptive panel to build the wines' odor profiles. The experts were not informed that the OSA were related to wines. For each OSA, they were asked to indicate the odor notes, further called odor qualities (OQ), needed to construct a given odor sensory attribute (OSA). Each OQ was quantized by the experts according to an ordinated symbolic 5-value scale: near 0, +/-, +, ++, +++. These symbolic values were respectively transformed into numerical values, NV=0.25,0.5,1,2,3. The resulting scaling information was then translated into a numerical value Num in [0,1] for each OQ related to an OSA (Equation (2.1)); the obtained value reflects the perceptual proportion of an OQ in a given OSA, as reported by an expert.
Num(qj)=NV(qj)/Q∑i=0NV(qi) | (2.1) |
Where qj is an association between one OQ analyzed by one expert for a specific OSA, and Q is the total number of associations between the OQ and all the OSA.
The fuzzy model was developed on MATLAB (R2014b) using the equations presented in [47,48]. A fuzzy set E is defined thanks to a membership function x⇝μE(x)∈[0,1] which represents a membership degree of a point x.
Membership functions can take various forms. Our model has been built from
a trapezoidal representation (Equation(2.2)) with four parameters a1 to a4.
μ(x)={0(x≤a1)(x−a1)(a2−a1)(a1<x≤a2)1(a2<x≤a3)(a4−x)(a4−a3)(a3<x≤a4)0(x>a4) | (2.2) |
If x is a proportion of OQ, μ(x) then represents the membership degree to an OSA, see Figure 1.
The fuzzy Tnorm was used in this paper to aggregate the information associated to the aroma reconstruction proposed by the flavorists. Indeed, odor qualities are joined by a connector "AND" (a classical mathematical logical interpretation of the join) using the fuzzy Tnorm:
Tnorm(μ0,μ1,...,μn)=∏iμi | (2.3) |
where μi are the membership functions of the i odor qualities (OQ) proposed by the flavorists to define a given odor sensory attribute (OSA).
The parameters a1 to a4 of the fuzzy membership functions were optimized using a classical evolutionary covariance matrix adaptation evolutionary algorithm. The evolutionary covariance matrix adaptation evolutionary algorithm (CMA-ES) was adapted from cmaes.m (Version 3.61.beta) and ran for 10 repetitions (cost function = fuzzy function, population size = 2000).
The fuzzy model was validated following a leave-one-out cross-validation (LOOCV) procedure, meaning that each sample (food product) is left out once and used for validation [49]. The output of the fuzzy models was the intensity predicted for each OSA. It was calculated for each wine in the application. We compared these predicted intensities with those obtained by sensory evaluation. First, we calculated the percentage of prediction error following Equation (2.4). The closer the sensory intensity evaluated and measured the lower the percentage prediction error. In contrast, if the sensory intensity evaluated and measured are highly different, the percentage will be high (100 and more) [50].
E=[|(Ipredicted−Ievaluated)|/Ievaluated]∗100 | (2.4) |
In addition, we calculated the similarity (Sim) between the intensities evaluated xl and predicted yl for each OSA l of wine i, following the Ruzicka similarity (Equation 2.5). The values vary from 0 (not similar at all) to 1 (datasets identical).
Sim(xl,yl)=∑lmin(xl,yl)/∑lmax(xl,yl) | (2.5) |
Finally, we performed a normalized principal component analysis (PCA) and a hierarchical clustering on principal components (HCPC) on the sensory profile obtained by sensory evaluation and from the fuzzy model predictive approach.
The predictive approach was developed to mimic at a macroscopic level, the holistic human process of odor perception. A reverse engineering approach depicted in Figure 2 was set up considering the knowledge of researchers on flavor and of four senior flavorists, embedded in mathematical procedures and fuzzy functions. The goal was to make the link between the space of odorants formalized in a database depicting the odorants' composition of food products (e.g. wines), and the space of perception of these products (e.g. odor profiles of the wines), through a restricted human olfactory space operationalized in database structured by an ontology [51].
In a first step, (f1(x) in Figure 2) we considered the chemical composition of the product in terms of identified odorants and the contribution of their specific odor reflected by the nasal impact frequency or NIF (for data on wines see [42]). This dataset was converted into a matrix N, where each row l corresponds to a wine wl, and each column j represents the perceptual contribution of an odorant molecule mj.
Afterward, for each product (wine, in our application) wl we obtained the list of corresponding odorant molecules mj for which Nlj≠0 in N.
The list was then cross-referenced against three databases of odorants (see section 2.1.1) to obtain the odor descriptors (OD) with the best possible representativeness. As the information in each database is likely to differ, we aggregated the information by summing up each occurrence of a specific OD i associated to odorant molecule mj in all three databases. The resulting OD matrix Dl for a specific wine wl contains elements Dlij such as:
Dlij=3∑k=1bkij | (3.1) |
where bkij={0,1} denotes the presence or absence of OD i associated to odorant molecule mj in database k. For example, the result of this procedure for the odorant molecule Isoamyl acetate (CAS 123-92-2) in the wine application is represented in Table 1: [(apple, 1); (banana, 3); (fruity, 2); (fruity-fresh, 1); (nauseating, 1); (pear, 2); (solvent, 1); (sweet, 3)] and 0 for the other odor descriptors. In other words, all three databases showed an association between Isoamyl acetate and the odor descriptor banana, whereas only one of them associated it with the apple odor descriptor.
Odorant molecule | apple | banana | fruity | fruity-fresh | ... | nauseating | pear | ... | solvent | sweet | ... |
Isoamyl acetate | 1 | 3 | 2 | 1 | 0 | 1 | 2 | 0 | 1 | 3 | 0 |
Benzyl alcohol | |||||||||||
Odorant molecule | Almond | ... | Floral | Fresh | Fruity | ... | Smoky | ... | ... | ... | ... |
Isoamyl acetate | |||||||||||
Benzyl alcohol | 1 | 0 | 2 | 1 | 9 | 0 | 1 | 0 | 0 | 0 | 0 |
In a second step, (f2(x) in Figure 2) the matrix Dl, containing the aggregation of the information for odor descriptors associated to odorant molecules found for the product wl among the three databases, was transformed into a new matrix Ql containing the corresponding odor qualities (OQ), thanks to mathematical rules describing the correspondence between the ODs and the OQs. The transformation was implemented through a hierarchical set of relations "is-a" depicted by expert scientists and represented in the form of an ontology (OOPS), implemented in OWL for the wine application [51].
Using this ontology is equivalent to applying a transformation Q:
Q:Nm,n→Nm,n′ | (3.2) |
with n′<n, as OQs are fewer since several odor descriptors (OD) map to the same odor qualities (OQ) for one molecule, given expert heuristics. For example, for the odorant Isoamyl acetate, the result is [(Fresh, 1); (Fruity, 9)] with [fresh=fruity−fresh] and [fruity=fruity+apple+banana+sweet]. Each element of the matrix Ql is named Qlij, where j represents the molecule mj in row j and i represents the odor quality qi in column i. Going back to matrix N, in order to take into account the contribution of the odorant molecules mj contained in matrix N for the wine wl, each element Qlij in Ql was multiplied by the NIF of the odorant molecule j, for wine wl under consideration:
Q′lij=Qlij∗Nlj | (3.3) |
Thus, for example, considering again the molecule Isoamyl acetate in a given wine wl with a Nlj=0.9, applying the procedure led to a set of odor qualities Q′li (row of matrix Q′l), as follows: [(Fresh, 1∗0.9); (Fruity, 9∗0.9)].
In a third step, (f3(x) in Figure 2) all the information in terms of odor quality (OQ) obtained for all the odorants found in each product was grouped into a single matrix P, where each line contains information for one product (a given wine in our example), and each column describes the combined information for an OQ coming from all molecules identified in the product. In other words, each element Pli of matrix P equals to:
Pli=∑pQ′lpi | (3.4) |
where Qlpi are elements in matrix Ql for wine wl, i is the index of the column corresponding to odor quality i, and ∑p represents a sum over all lines of Qi, where each line represents a different odorant molecule. For instance, if one product is constituted by a mixture of Isoamyl acetate CAS 123-92-2, (odorant 1) and Benzyl alcohol CAS 100-51-6, (odorant 2), with NIF scores respectively NIF1 and NIF2, it is possible to sum the set of both odorants [(Fresh, 1xNIF1); (Fruity, 9xNIF1)] for the isoamyl acetate and [(Almond, 1xNIF2); (Floral, 2xNIF2); (Fruity, 1xNIF2); (Smoky, 1xNIF2)] for the Benzyl alcohol, to end up with an OQ/wine set being [(Almond, 1xNIF2); (Floral, 2xNIF2); (Fresh, 1xNIF1); (Fruity, 9xNIF1+1xNIF2); (Smoky, 1xNIF2)].
So far, all the transformations produced matrices containing absolute values; but for most experts, what really makes sense was the relative proportion of odor qualities. For this reason, in a final step, each element of P was normalized as follows:
P′li=Pli/∑pPlip | (3.5) |
In a fourth step, (f4(x) in Figure 2), we applied on P′li, a transformation function based on fuzzy logic functions F:
F:Rw,n′→Rw,n″ | (3.6) |
with n″<n′, as odor sensory attributes (OSA) are fewer since several OQs of one product map to the same OSA. Fuzzy mathematical operators were implemented for this transformation function. Each element Sli of the new matrix S was defined for the product, in this example the wine wl, where i is the index of the column corresponding to the OSA oi. Sli were computed for wine wl, according to the Equation 3.7:
Sli=h⋅Tnorm(μi0(P′j0),μi1(P′j1),...μin′(P′jn′)) | (3.7) |
where P′i0,...,P′in′ identifies the columns corresponding to the OQs j involved in the computation for wine wl, μi0,...,μin′ are the membership degrees for odor sensory attribute oi and h is a parameter that was used to scale the results to human-readable values. To rate wine samples, sensory panelists used sensory scales that range from 0 to 10, so we set h=10.
It is important to note that this symbolic formalization is independent from the specific application, and could be easily generalized to any other food product.
Four senior flavorists contributed to this study. We decided to manage the uncertainty induced by inter-individual differences in one fuzzy function for each odor quality. The fuzzy function was bounded by the minimum and maximum proportions across experts.
μij:{ai1=0ai2=mine((ai2)e)ai3=maxe((ai3)e)ai4=1 | (3.8) |
Where (ai1)e, (ai2)e, (ai3)e, (ai4)e are the parameters set up for the fuzzy function translating the odor quality j in the odor sensory attribute i defined by the expert e, independently of the wine considered.
The parameter values provided by experts were then compared against parameter values obtained through a machine learning approach, applied to either all parameters or a subset, see Table 2 for a summary.
optimization | parameters | ||||
condition A | Flavorists | 0 | 1 | ||
condition B | partially optimized | optimized | optimized | ||
condition C | optimized CMAES | optimized | optimized | optimized | optimized |
The machine learning approach optimized the parameters to minimize the gap between the sensory values (OSA intensity evaluated by sensory evaluation) and the predicted values (OSA intensity predicted by the optimized fuzzy model). The optimization algorithm applied to the problem was the Covariance Matrix Adaptation Evolution Strategy (CMA-ES) [52], the state-of-the-art for the optimization of non-convex functions.
We called condition A the fuzzy model with expert-determined values for the parameters. Condition B aimed to optimize (ai2) and (ai3) using CMA-ES, and condition C optimized all 4 parameters. In conditions B and C, 10 repetitions were carried out and we further considered the mean of the intensity predicted for these 10 repetitions as the intensity predicted for a food product.
Applied to our wine dataset, the output of the predictive model was then the odor profile of a wine wp. The predicted odor profile was compared to the actual odor profile of the same wine obtained by sensory evaluation.
Expert flavorists were asked to decompose in a reverse engineering mode, the 15 OSA (Table 4), that were used to characterize the odor profile of the wines. They had to break down the OSA into one or several OQ, depending on whether the OSA were simple or complex. We hypothesized that flavorists have in mind the knowledge to combine several OQ to elicit the perception of a given OSA that characterizes the aroma of complex mixtures of odorants, and foods or beverages. In the present study, their reasoning was formalized in a backward way using fuzzy functions and fuzzy Tnorm establishing a projection from the OQ space to the OSA space.
Bell Pepper | Cherry stone | Strawberry |
Blackcurrant bud | Cut-grass | Toasted |
Blackcurrant fresh | Leather | Toasty |
Cherry cooked | Prune | Violet |
Cherry fresh | Smoky | Woody |
Prune | Almond | Cooked | Fruity | Honey | Lactonic |
Expert 1 | + | Nu | Nu | Nu | ++ |
0.33 | Nu | Nu | Nu | 0.67 | |
Expert 2 | Nu | ++ | + | near 0 | + |
Nu | 0.47 | 0.24 | 0.06 | 0.24 | |
Expert 3 | Nu | Nu | Nu | Nu | Nu |
Expert 4 | Nu | +++ | + | Nu | Nu |
Nu | 0.75 | 0.25 | Nu | Nu | |
Lower proportion | 0 | 0 | 0 | 0 | 0 |
Higher proportion | 0.33 | 0.75 | 0.25 | 0.06 | 0.67 |
Among the 15 OSA, 7 were identified by experts as simple OSA (Cut-grass, Leather, Smoky, Toasty, Vanilla, Violet, and Woody), constituted of only one OQ. The other 8 OSA were considered complex since experts thought that several OQ have to be combined to elicit the OSA perception.
We have focused our attention on complex OSA. For example, the detailed results of the experts' interview for the complex OSA Prune are presented in Table 4. The compiled results of the interview (i.e. the proportions bounds: lower and higher proportions) for the 15 OSA are presented in Table 5.
OSA | OQ1 | OQ2 | OQ3 | OQ4 | OQ5 | OQ6 | OQ7 |
Cut-grass | |||||||
Leather | |||||||
Smoky | |||||||
Toasty | |||||||
Vanilla | |||||||
Violet | |||||||
Woody | |||||||
Bell pepper | Floral | Fruity | Green | Sulfurous | Toasty | Vegetable | |
Lower proportion | 0 | 0 | 0 | 0.17 | 0 | 0 | |
Higher proportion | 0.17 | 0.33 | 0.80 | 0.25 | 0.5 | 0.33 | |
Blackcurrant bud | Floral | Fresh | Fruity | Green | Sulfurous | Vanilla | Wine-like |
Lower proportion | 0 | 0 | 0.10 | 0 | 0.11 | 0 | 0 |
Higher proportion | 0.22 | 0.33 | 0.67 | 0.20 | 0.40 | 0.20 | 0.38 |
Blackcurrant fresh | Floral | Fresh | Fruity | Green | Sulfurous | Wine-like | |
Lower proportion | 0 | 0 | 0.25 | 0 | 0.07 | 0 | |
Higher proportion | 0.13 | 0.53 | 0.44 | 0.22 | 0.25 | 0.25 | |
Cherry cooked | Almond | Cooked | Floral | Fruity | Green | Peel | Spicy |
Lower proportion | 0.13 | 0.14 | 0 | 0 | 0 | 0 | 0 |
Higher proportion | 0.29 | 0.38 | 0.07 | 0.57 | 0.21 | 0.06 | 0.21 |
Cherry fresh | Almond | Cooked | Floral | Fruity | Green | Peel | Spicy |
Lower proportion | 0.12 | 0.22 | 0 | 0 | 0.22 | 0 | 0 |
Higher proportion | 0.26 | 0.24 | 0.07 | 0.35 | 0.24 | 0.06 | 0.22 |
Cherry stone | Almond | Cooked | Floral | Fruity | Green | Peel | Spicy |
Lower proportion | 0.25 | 0 | 0 | 0 | 0 | 0 | 0 |
Higher proportion | 1 | 0.25 | 0.06 | 0.38 | 0.18 | 0.06 | 0.18 |
Prune | Almond | Cooked | Fruity | Honey | Lactonic | ||
Lower proportion | 0 | 0 | 0 | 0 | 0 | ||
Higher proportion | 0.33 | 0.75 | 0.25 | 0.06 | 0.67 | ||
Strawberry fresh | Cooked | Floral | Fruity | Green | |||
Lower proportion | 0.17 | 0 | 0.33 | 0 | |||
Higher proportion | 0.40 | 0.33 | 0.5 | 0.27 |
In order to explain the general approach, we chose to present the example of the OSA Prune for the wine PN1, namely one OSA among the 15 used by the sensory descriptive panel to profile the 16 wines of the sample set. The algorithm was applied according to the mathematical steps described in section 3 and illustrated below.
● In the first step, we considered the chemical composition of the wine PN1 [42]. The list of the 33 odorants identified in PN1 with their corresponding NIF scores and odor descriptors (OD) sets are shown in Table 6, column 2 and 3.
CAS Number | NIF | Odor descriptors | Odor qualities |
100-51-6 | 0.625 | (almond, 1); (balsamic, 1); (floral, 1); (fruity, 1); (phenolic, 1); (rose, 1); (sweet, 2) | (Almond, 0.625); (Floral, 1.25); (Fruity, 0.625); (Smoky, 0.625) |
105-54-4 | 0.875 | (banana, 2); (buttery, 1); (cognac, 1); (ethereal, 1); (ethereal-fruity, 1); (fruity, 2); (juicy, 2); (pineapple, 3); (ripe fruit, 1) | (Fruity, 7.875) |
106-32-1 | 0.125 | (apricot, 2); (banana, 2); (brandy, 1); (fermented-winey, 1); (fruity, 2); (fruitywiney, 1); (pear, 1); (pineapple, 1); (sweet, 3); (waxy, 1); (winey, 2) | (Fruity, 1.125); (Wine-like, 0.5) |
106-33-2 | 0.5 | (fatty, 1); (floral, 2); (flower-petal, 1); (fruity, 2); (leafy, 1); (oily, 1); (oily-fatty, 1); (soapy, 1); (sweet, 1); (waxy, 2) | (Floral, 1.5); (Fruity, 1) |
106-44-5 | 0.5 | (animal, 1); (animalic, 1); (dry, 1); (dry-tarry, 1); (leather, 1); (leathery, 1); (medicinal, 3); (medicinal-leathery, 1); (mimosa, 1); (narcissus, 1); (phenolic, 3); (smoky, 1); (tarry, 1); (tarry-smoky, 1); (woody, 1) | (Floral, 1); (Leather, 1.5); (Smoky, 5); (Woody, 0.5) |
107-92-6 | 0.625 | (acetic, 1); (buttery, 2); (cheese, 1); (cheesy, 1); (fruity, 1); (rancid, 1); (rancid butter, 1); (sour, 2) | (Fruity, 0.625) |
108-64-5 | 0.875 | (apple, 3); (banana, 1); (blueberry, 1); (buttery, 1); (ethereal, 1); (fruity, 2); (pineapple, 1); (sweet, 2); (tutti fruit, 1); (wine-like-fruity, 1); (winey, 1) | (Fruity, 8.75); (Wine-like, 1.75) |
108-95-2 | 0.125 | (medicinal, 1); (phenolic, 2); (plastic, 1); (rubbery, 1) | (Smoky, 0.25) |
122-78-1 | 0.75 | (clover, 1); (cocoa, 1); (floral, 3); (green, 3); (honey, 2); (hyacinth, 3); (rose, 1); (sweet, 2) | (Floral, 5.25); (Green, 2.25); (Honey, 1.5) |
123-07-9 | 0.75 | (castoreum, 1); (guaiacol, 1); (phenolic, 1); (smokey, 1); (smoky, 1); (sweet, 1); (tarrymedicinal, 1) | (Leather, 0.75); (Smoky, 3.75); (Spicy, 0.75) |
123-51-3 | 1 | (alcoholic, 3); (banana, 1); (fermented, 1); (fruity, 1); (fruity-winey, 1); (fusel, 1); (whiskey, 1) | (Fruity, 3); (Wine-like, 1) |
123-66-0 | 1 | (apple peels, 1); (banana, 3); (floral, 1); (fruity, 2); (fruity-winey, 1); (green, 1); (pear, 1); (pineapple, 3); (strawberry, 1); (sweet, 1); (tropical, 1); (waxy, 1) | (Floral, 1); (Fruity, 13); (Green, 1); (Peel, 1); (Wine-like, 1) |
123-92-2 | 0.125 | (apple, 1); (banana, 3); (fruity, 2); (fruityfresh, 1); (nauseating, 1); (pear, 2); (solvent, 1); (sweet, 3) | (Fresh, 0.125); (Fruity, 1.125) |
2785-89-9 | 0.625 | (bacon, 2); (clove, 2); (eugenol, 1); (guaiacol, 1); (phenolic, 2); (smoky, 2); (spicy, 2); (spicy-medicinal, 1); (sweet, 1); (vanilla, 1) | (Smoky, 3.125); (Spicy, 4.375); (Vanilla, 0.625) |
334-48-5 | 0.375 | (cheese, 1); (citrus, 1); (dairy, 1); (fatty, 2); (rancid, 2); (sour, 1); (sour-fatty, 1); (waxy, 1) | (Fruity, 0.375) |
3268-49-3 | 0.625 | (bouillon, 1); (creamy, 1); (earthy, 1); (meaty, 1); (musty, 1); (onion, 2); (onionmeat, 1); (potato, 1); (tomato, 1); (vegetable, 1) | (Vegetable, 1.875) |
39212-23-2 | 0.5 | (burnt, 1); (celery, 1); (coconut, 2); (coumarinic, 2); (lactonic, 1); (lovage, 1); (maple, 1); (nutty, 1); (toasted, 1); (tonka, 1); (woody, 1) | (Lactonic, 0.5); (Spicy, 0.5); (Toasty, 1); (Vanilla, 0.5); (Vegetable, 0.5); (Woody, 0.5) |
431-03-8 | 0.875 | (buttery, 3); (caramel, 1); (chlorine-quinone, 1); (creamy, 1); (oily, 1); (sweet, 1) | (Cooked, 0.875) |
4312-99-6 | 0.5 | (earthy, 1); (herbal, 1); (metallic, 1); (mushroom, 2); (musty, 1) | (Cut-grass, 0.5); (Green, 0.5) |
503-74-2 | 1 | (acid-acrid, 1); (cheese, 1); (cheesy, 2); (fruity, 1); (herbaceous, 1); (sour, 2); (sweaty, 2); (tropical, 1) | (Cut-grass, 1); (Fruity, 2); (Green, 1) |
505-10-2 | 0.25 | (meaty, 2); (mushroom, 1); (onion, 1); (soup, 2); (sulfuraceous, 1); (sulfurous, 1); (sweet, 1); (sweet soup-meat, 1); (vegetable, 1) | (Sulfurous, 0.5); (Vegetable, 0.75) |
590-86-3 | 0.25 | (acrid, 1); (aldehydic, 1); (cheese, 1); (chocolate, 1); (cocoa, 1); (ethereal, 1); (fatty, 1); (fruity, 2); (green fruity, 1); (peach, 2); (sweaty, 1) | (Fruity, 1.25); (Green, 0.25) |
60-12-8 | 1 | (earthy, 1); (floral, 2); (greener gassy, 1); (hyacinth, 1); (rose, 2); (rose-honey, 1) | (Floral, 6); (Green, 1); (Honey, 1) |
64-17-5 | 0.625 | (alcoholic, 3); (ethereal, 2); (medicinal, 1); (sweet, 1); (sweet-ethereal, 1) | (Fruity, 0.625) |
64-19-7 | 1 | (acidic, 1); (sour, 3); (vinegar, 2) | |
620-17-7 | 0.75 | (medicinal, 1); (musty, 1); (sweet, 1); (woodyphenolic, 1) | (Smoky, 0.75); (Woody, 0.75) |
74-93-1 | 0.375 | (cabbage, 1); (garlic, 1); (rotten cabbage, 1); (rotting cabbage, 1); (sulfurous, 1) | (Sulfurous, 0.375); (Vegetable, 0.375) |
7452-79-1 | 0.875 | (apple, 2); (apple peels, 1); (fruity, 3); (green, 2); (green-fruity, 1); (peels of unripe plums, 1); (pineapple skin, 1); (strawberry, 1); (sweet, 1) | (Fruity, 0.875); (Green, 2.625); (Peel, 2.625) |
80-62-6 | 0.625 | (acrylate, 1); (acrylic, 1); (apple, 1); (estery, 1); (fruity, 2); (grape, 1) | (Fruity, 3.125) |
90-05-1 | 0.75 | (medicinal, 2); (phenolic, 2); (smoky, 3); (spicy, 1); (sweet, 1); (vanilla, 2); (woody, 1) | (Smoky, 3.75); (Spicy, 0.75); (Vanilla, 1.5); (Woody, 0.75) |
91-10-1 | 0.375 | (bacon, 1); (balsamic, 1); (medicinal, 1); (phenolic, 2); (powdery, 1); (smoky, 2); (woody, 2) | (Smoky, 1.5); (Woody, 0.75) |
96-48-0 | 0.75 | (buttery, 1); (caramel, 2); (creamy, 1); (fatty, 1); (nutty, 1); (oily, 1); (sweet, 1) | (Cooked, 1.5) |
97-62-1 | 0.875 | (alcoholic, 1); (apple, 1); (ethereal, 2); (floral, 1); (fruity, 3); (fusel, 1); (rum, 1); (rummy, 1); (sweet, 3); (sweet-ethereal, 1) | (Floral, 0.875); (Fruity, 4.375) |
● In the second step (Table 6, column 4), the OD were translated into OQ, thanks to the OOPS ontology [51] reflected in the relation (3.2) expressing heuristics like
"the OD banana, buttery, cognac, ethereal, ethereal-fruity, fruity, juicy, pineapple and ripe fruit belongs to the OQ fruity".
The OQs were quantified and weighted by the NIF scores (nPN1j) of the corresponding odorant i, see Equation (3.3). For example for the CAS Number 123-92-2 (Isoamyl acetate), the OQ Fresh is associated to the number 0.125 as NIF(Isoamyl acetate)*(OD Fruity-fresh) = 0.125*1.
The OQ Fruity is associated to the number 1.125 which is equal to:
NIF (isoamyl acetate)*(OD banana+apple+fruity+sweet) = 0.125*9.
● In the third step, the odor qualities set of the 33 odorants were then summed up to obtain the odor qualities set of PN1, Equation (3.4). For example, if we consider the OQ fruity for the wine PN1, the calculus is (see Table 6):
49.75=0.625+7.875+1.125+1+0.625+8.75+3+13+1.125+0.375+2+1.25+0.625+0.87+3.125+4.375.
For the PN1 wine the final results was:
(Almond, 0.625); (Cooked, 2.375); (Cut-grass, 1.5); (Floral, 16.875); (Fresh, 0.125); (Fruity, 49.75); (Green, 8.625); (Honey, 2.5); (Lactonic, 0.5); (Leather, 2.25); (Peel, 3.625); (Smoky, 18.75); (Spicy, 6.375); (Sulfurous, 0.875); (Toasty, 1); (Vanilla, 2.625); (Vegetable, 3.5); (Violet, 0); (Wine-like, 4.25); (Woody, 3.25).
This set was further represented as proportions of OQ, Equation (3.5), following PPN1Almond=0.625/129.375=0.005, where 129.375 is the sum over all the molecules and all the OQ for one wine. The result of the proportions of OQ for the OSA prune in wine PN1, based on the chemical composition of odorant molecules, is the following odor quality set:
(Almond, 0.005); (Cooked, 0.018); (Cut-grass, 0.011); (Floral, 0.13); (Fresh, 0.001); (Fruity, 0.38); (Green, 0.06); (Honey, 0.019); (Lactonic, 0.004); (Leather, 0.017); (Peel, 0.03); (Smoky, 0.14); (Spicy, 0.05); (Sulfurous, 0.006); (Toasty, 0.007); (Vanilla, 0.02); (Vegetable, 0.02); (Violet, 0); (Wine-like, 0.03); (Woody, 0.02).
● In the fourth step, based on the flavorist expert description of the OSA prune, five OQ were needed to construct this complex OSA, therefore, five fuzzy membership functions, one per OQ, were created (see Figure 3). These five functions were created according to the collected expertise of building an OSA Prune from five OQ (Almond, Cooked, Fruity, Honey, Lactonic) with different proportions depending on the expert. It was computed following the Equation (3.7), meaning that if the membership degrees of the five OQ to the intensity of the OSA Prune is equal to 1, the predicted intensity of the OSA Prune will be 10/10, with OQ1=Almond, OQ2=Cooked, OQ3=Fruity, OQ4=Honey and OQ5=Lactony. In this way, from the odor quality set of PN1 (Almond, 0.005); (Cooked, 0.018); (Cut-grass, 0.011); (Floral, 0.13); etc..., we only kept the values corresponding to the OQ of the OSA Prune: (Almond, 0.005); (Cooked, 0.018); (Fruity, 0.38); (Honey, 0.019); (Lactonic, 0.004). The values were standardized by dividing them by the sum of the OQ of the OSA Prune, ensuring that the sum of the 5 OQ equaled 1. This process determined the proportion of OQ in the complex OSA Prune based on the expert flavorists' knowledge: (Almond, 0.01); (Cooked, 0.042); (Fruity, 0.89); (Honey, 0.044); (Lactonic, 0.009).
On this basis, the Equation (2.2) was applied and provided the value of the OSA Prune for the wine PN1 according to the parameters of Table 4. Thus for PN1:
– μOQAlmondOSAPrune=1,
– μOQCookedOSAPrune=1,
– μOQFruityOSAPrune=0,146,
– μOQHoneyOSAPrune=1,
– μOQLactonicOSAPrune=1
Equation (3.7) finally gives a value of OSA Prune for PN1 of 10∗μOQAlmondOSAPrune∗μOQCookedOSAPrune∗μOQFruityOSAPrune∗μOQHoneyOSAPrune∗μOQLactonicOSAPrune=10∗1∗1∗0.146∗1∗1=1.46.
The calculation described above, which involves four steps for one OSA in one wine, was replicated for all OSA in the 15 wines (16 wines - 1 wine for the Leave-one-out Cross Validation). The relationship between the chemical composition of the wine and the sensory data was also modeled through fuzzy rules according to three other conditions determined by stochastic optimization for the fuzzy parameters a1 to a4. As an illustration of the results, Figure 4 displays the Fuzzy membership functions that establish the relationship between the proportion of the five OQ Almond, Cooked, Fruity, Honey, Lactonic and the intensity of the OSA Prune in the 15 wines, considering the three optimization conditions. In this figure, the red triangles represent the proportions of the five OQ in the wine PN1.
The algorithm presented in this paper for the OSA Prune was extended to the 8 other complex OSA for the wine PN1. For the simple OSA like toasty, a simple linear regression was achieved. This approach was then applied to the other 15 wines. The accuracy of the prediction was assessed by the r-squared values.
It has to be noted that several intensity values were not predicted by the model. Indeed, the absence of odorants belonging to the OQ Lactonic in the wines from the grape variety Cabernet Franc led to a membership degree equal to 0 and thus resulted in an intensity predicted for the OSA Prune equal to 0 in the 8 Cabernet Franc wines.
Focusing on the simple OSA, a rather good prediction was obtained for the OSA Cut-grass (r2=0.68, p<0.01), while no significant results were found for the other 6 linear regressions (r2<0.15, p>0.05).
Considering the complex OSA (see Table 2), conditions A and B of optimization led to similar results, whereas condition C provides better predictions for the OSA Bell pepper and Cherry fresh. 336 In 5, we presented the similarity between the evaluated and predicted values for the 8 complex OSA for the three conditions of optimization, as well as for the 7 simple OSA; this is another way to evaluate the result and to quantify the agreement between predictions and sensory descriptive panel evaluations at a symbolic relative level. The closer to 1 the similarity, the better the results. We observed that for several complex OSA, like Prune, Blackcurrant fresh, and Strawberry fresh, the level of similarity is pretty good, whatever the conditions of optimization. For other OSA related to Cherry odors (Cherry cooked, Cherry fresh, and Cherry stone), prediction in conditions A and B are very poor, indicating that these OSA could not be satisfactorily modeled from the generic knowledge of the experts used in the present work.
In addition to these findings, we aimed to determine whether the predictive approach we developed could highlight the differences between the wines, specifically in terms of distinguishing wines based on their odor attributes and gaining insights into these distinctions. To do so, we performed a principal component analysis (PCA) followed by hierarchical clustering on the principal components (HCPC) using the OSA intensity either evaluated or predicted for the 16 wines (Figure 6).
On the one hand, results based on sensory evaluation showed a separation of the wines according to their grape varieties with the exception of CF3, which was grouped with the PN, and PN4, which was grouped with the CF wines. The CF wines were perceived as more Bell pepper, Blackcurrant, Cut-grass, Strawberry and Violet than the PN wines. On the other hand, results based on the predictions highlighted that optimization conditions A and B allowed the separation of the wines according to their grape varieties, except for PN3, which was grouped with the CF wines. The predicted results suggested that the CF wines may be perceived as having more pronounced Blackcurrant and Strawberry notes, but certain variables show discrepancies compared to the findings from sensory evaluation (e.g. Woody, Smoky).
We further investigated the proximity between the PCA maps by computing RV coefficient, which is a multivariate generalization of the squared Pearson correlation coefficients. RV coefficients were calculated between the predicted values and the actual sensory values according to the three optimization conditions. The results of this statistical test revealed that condition B exhibited the closest alignment with the experimentally evaluated profile (RV=0.47), followed by condition A (RV = 0.46), and condition C (RV=0.36). Indeed, condition C only separated two PN wines from the remaining 14 wines.
This research aimed at proposing an innovative strategy to predict the odor profile of food from its complex chemical composition in odorant molecules. We chose to develop a holistic approach that combines knowledge and comprehension of scientific facts while relying upon computer science methods. This strategy especially took into consideration human expertise to integrate non-linear perceptual computation of mixtures of odorants. To the best of our knowledge, the developed model constitutes the first attempt to predict quantitative odor description from the molecular composition of complex odor objects, but it is also the first report on a knowledge-based artificial intelligence approach related to odor perception. In contrast to most of the previous modeling approaches in olfaction that concentrate on single odorants, the proposed model was applied to a set of real food samples, namely wines.
The model was able to predict the wines' odor profiles through the estimation of the intensity of 15 odor sensory attributes (OSA). To do so, a transfer function, associated with an ontology, was used to establish the relationships between the molecular composition underpinning the odor of a food product and the perceptual concepts at the core of the expertise of flavorists. Fuzzy logic was then applied to formalize expertise, rendering it applicable for OSA intensity prediction following optimization. According to the flavorists, OSA can be simple or complex.
Regarding simple OSA, namely the 7 OSA not derived from combinations of OQ according to the experts, the similarity between predicted and evaluated intensity was high (0.60). However, correlations between predicted and evaluated intensity for simple OSA were generally not statistically significant, with the exception of the OSA Cut-grass. These findings imply that the perceptual outcome arising from a combination of odor-active compounds or odor vectors could be influenced by the mixture of odorants of the overall odor object, in our case, wine. This suggests that flavorists' expertise may be contextually dependent on the specific food or beverage being evaluated, and therefore needs to be recorded in light of the target food object.
Regarding complex OSA, they were predicted using fuzzy logic functions according to three optimization conditions. As a matter of fact, for the OSA Bell pepper, Blackcurrant bud in optimization condition A, and Cherry stone, Prune, and Strawberry in condition C, the results ranging between 0.4 and 0.6 provide valuable insights into the understanding of complex OSA. Indeed, conditions varied according to the degree of expertise integrated into the model and thus to the definition of the fuzzy membership functions. The membership functions for condition A relied on pure expertise because the structure (the rules linking OSA to OQ) and parameters (the fuzzy function parameters) are fixed exclusively by experts. Condition B was a variant of condition A where the slopes of the fuzzy functions were optimized using a genetic algorithm. The results highlighted that conditions A and B were very similar because of the limited optimization possibilities. The membership functions for condition C relied also on expertise for the structure of the model (combination of OQ) but not for the parameters. These parameters were fully estimated using an evolutionary algorithm. Condition C might provide insights into combinations of OQ that may not have been explored by the experts. Conditions A and B demonstrated good similarities for certain OSA (Blackcurrant fresh, Prune, Strawberry fresh) and performed quite well in the classification of the wines (see PCA, HCPC analyses). Condition C yielded better similarity results for some OSA (Cherry fresh, Cherry stone) and slightly better results of correlation between the sensory evaluated and predicted intensities for three OSA (Bell pepper, Cherry fresh, and Prune). Interestingly, and as illustrated in Figure 7, the optimized condition C highlighted that more OQ Fruity was needed to predict more accurately the intensity of the OSA Cherry fresh in the wines, which suggests that more precise information might be expected from the experts for specific complex odor sensory attributes.
These results can be explained by the non-targeted approach we developed. Indeed, expertise was collected in a generic manner, and experts were unaware of the specific food matrix under study. Nevertheless, the product matrix has been demonstrated to influence the perception of sensory attributes. Further investigations should involve presenting the obtained results to flavorists or wine experts to refine the knowledge integrated into the modeling strategy. This approach could be valuable in identifying which OSA are linked to the wine matrix and which can be predicted independently. Such an approach could help in refining the expert rules related to odor associations that underlie complex odor percepts, potentially enhancing the generalizability of knowledge-based rules.
Another explanation for the inaccuracies in predicting certain OSA could be the absence of actual identification of several key odorants detected in the wines ([42]). Specifically, we observed that the absence of odorants related to the odor quality (OQ) Lactonic in Cabernet Franc wines resulted in a membership degree of 0. Consequently, this absence led to a predicted intensity of 0 for the OSA Prune in all 8 Cabernet Franc wines. However, this OSA appeared to be important in the odor profile of these wines. This lack of information within the chemical dataset constitutes a limit to the modeling possibilities. Nonetheless, recognizing these gaps in knowledge following initial modeling could serve as a valuable guide for directing focused chemical identification studies to address specific OQ and identify still unknown key odorants.
In conclusion, the complementary utilization of machine learning and human expertise in this study has provided innovative insights into the construction of olfactory attributes. Our predictive strategy emulates the cognitive processing of odor information by reducing the information collected from odorants to the perception of odor sensory attributes (OSA). Specifically, we transformed a pool of fifty odorants into 175 odor descriptors (OD), which were further associated to 20 odor qualities (OQ). Subsequently, fuzzy logic was used to establish relationships between the OQ and the 15 OSA characterizing the odor profile of a set of French wines.
This work was supported by grants from the Conseil Régional Bourgogne Franche-Comté. Calculations were performed using HPC resources from PSIUN CCUB (Centre de Calcul de l'Université de Bourgogne). AR was supported by an INRA Thesis fellowship. We would like to thank Thomas Chabin for his help with MATLAB scripts, and Thibaut Dosne for his help in collecting expert knowledge. We also acknowledge the 4 flavorist experts who shared their expertise.
The authors declare they have not used Artificial Intelligence (AI) tools in the creation of this article.
[1] |
D. E. Hornung, M. P. Enns, The contributions of smell and taste to overall intensity: A model, Percept. Psychophys., 39 (1986), 385–391. https://doi.org/10.3758/BF03207066 doi: 10.3758/BF03207066
![]() |
[2] | B. Lorrain, J. Ballester, T. Thomas-Danguin, J. Blanquet, J. M. Meunier, Y. Le Fur, Selection of potential impact odorants and sensory validation of their importance in typical chardonnay wines, J. Agri. Food Chem., 54 (2006), 3973–3981. |
[3] |
C. Flavián, S. Ibáñez-Sánchez, C. Orús, The influence of scent on virtual reality experiences: The role of aroma-content congruence, J. Business Res., 123 (2021), 289–301. https://doi.org/10.1016/j.jbusres.2020.09.036 doi: 10.1016/j.jbusres.2020.09.036
![]() |
[4] |
R. Hudson, Editorial note, Chem. Senses, 25 (2000), 693–693. https://doi.org/10.1046/j.1442-9993.2000.00002.x doi: 10.1046/j.1442-9993.2000.00002.x
![]() |
[5] | H. Stone, R. N. Bleibaum, H. A. Thomas, Sensory evaluation practices, Academic press, 2012. |
[6] | G. Ferrari, O. Lablanquie, R. Cantagrel, J. Ledauphin, T. Payot, N. Fournier, et al., Determination of key odorant compounds in freshly distilled cognac using GC-o, GC-MS, and sensory evaluation, J. Agri. Food Chem., 52 (2004), 5670–5676. |
[7] | S.-J. Lee, A. C. Noble, Characterization of odor-active compounds in californian chardonnay wines using GC-olfactometry and GC-mass spectrometry, J. Agri. Food Chem., 51 (2003), 8036–8044. |
[8] |
A. Pérez-Silva, E. Odoux, P. Brat, F. Ribeyre, G. Rodriguez-Jimenes, V. Robles-Olvera, et al., GC–MS and GC–olfactometry analysis of aroma compounds in a representative organic aroma extract from cured vanilla (vanilla planifolia g. jackson) beans, Food Chem, 99 (2006), 728–735. https://doi.org/10.1016/j.foodchem.2005.08.050 doi: 10.1016/j.foodchem.2005.08.050
![]() |
[9] |
M. Brattoli, E. Cisternino, P. Dambruoso, G. de Gennaro, P. Giungato, A. Mazzone, et al., Gas chromatography analysis with olfactometric detection (GC-o) as a useful methodology for chemical characterization of odorous compounds, Sensors, 13 (2013), 16759–16800. https://doi.org/10.3390/s131216759 doi: 10.3390/s131216759
![]() |
[10] | T. Thomas-Danguin, C. Sinding, S. Romagny, F. E. Mountassir, B. Atanasova, E. Le Berre, et al., The perception of odor objects in everyday life: A review on the processing of odor mixtures, Front. Psychol., 5 (2014). |
[11] |
W. Grosch, Evaluation of the key odorants of foods by dilution experiments, aroma models and omission, Chem. Senses, 26 (2001), 533–545. https://doi.org/10.1093/chemse/26.5.533 doi: 10.1093/chemse/26.5.533
![]() |
[12] | H. Guth, Quantitation and sensory studies of character impact odorants of different white wine varieties, J. Agri. Food Chem., 45 (1997), 3027–3032. |
[13] | V. Ferreira, N. Ortín, A. Escudero, R. López, J. Cacho, Chemical characterization of the aroma of grenache rosé wines: aroma extract dilution analysis, quantitative determination, and sensory reconstitution studies, J. Agri. Food Chem., 50 (2002), 4048–4054. |
[14] | A. Escudero, B. Gogorza, M. A. Melús, N. Ortín, J. Cacho, V. Ferreira, Characterization of the aroma of a wine from maccabeo. key role played by compounds with low odor activity values, J. Agri. Food Chem., 52 (2004), 3516–3524. |
[15] |
V. Ferreira, M. Sáenz-Navajas, E. Campo, P. Herrero, A. de la Fuente, P. Fernández-Zurbano, Sensory interactions between six common aroma vectors explain four main red wine aroma nuances, Food Chem., 199 (2016), 447–456. https://doi.org/10.1016/j.foodchem.2015.12.048 doi: 10.1016/j.foodchem.2015.12.048
![]() |
[16] | Y. Zheng, B. G. Sun, M. M. Zhao, F. P. Zheng, M. Q. Huang, J. Y. Sun, et al., Characterization of the key odorants in chinese zhima aroma-type baijiu by gas chromatography–olfactometry, quantitative measurements, aroma recombination, and omission studies, J. Agri. Food Chem., 64 (2016), 5367–5374. |
[17] |
W. S. Cain, Odor intensity: Differences in the exponent of the psychophysical function, Percept. Psychophys., 6 (1969), 349–354. https://doi.org/10.3758/BF03212789 doi: 10.3758/BF03212789
![]() |
[18] |
P. A. Edwards, P. C. Jurs, Correlation of odor intensities with structural properties of odorants, Chem. Senses, 14 (1989), 281–291. https://doi.org/10.1093/chemse/14.2.281 doi: 10.1093/chemse/14.2.281
![]() |
[19] |
M. H. Abraham, R. Sanchez-Moreno, J. E. Cometto-Muniz, W. S. Cain, An algorithm for 353 odor detection thresholds in humans, Chemical Senses, 37 (2012), 207–218. https://doi.org/10.1093/chemse/bjr094 doi: 10.1093/chemse/bjr094
![]() |
[20] |
A. Keller, R. C. Gerkin, Y. F. Guan, A. Dhurandhar, G. Turu, B. Szalai, et al., Predicting human olfactory perception from chemical features of odor molecules, Science, 355 (2017), 820–826. https://doi.org/10.1126/science.aal2014 doi: 10.1126/science.aal2014
![]() |
[21] |
R. M. Khan, C.-H. Luk, A. Flinker, A. Aggarwal, H. Lapid, R. Haddad, et al., Predicting odor pleasantness from odorant structure: Pleasantness as a reflection of the physical world, J. Neurosci., 27 (2007), 10015–10023. https://doi.org/10.1523/JNEUROSCI.1158-07.2007 doi: 10.1523/JNEUROSCI.1158-07.2007
![]() |
[22] |
A. Arshamian, R. C. Gerkin, N. Kruspe, E. Wnuk, S. Floyd, C. O'Meara, et al., The perception of odor pleasantness is shared across cultures, Current Biol., 32 (2022), 2061–2066. https://doi.org/10.1016/j.cub.2022.02.062 doi: 10.1016/j.cub.2022.02.062
![]() |
[23] |
L. Shang, C. J. Liu, Y. Tomiura, K. S. Hayashi, Machine-learning-based olfactometer: Prediction of odor perception from physicochemical features of odorant molecules, Anal. Chem., 89 (2017), 11999–12005. https://doi.org/10.1021/acs.analchem.7b02389 doi: 10.1021/acs.analchem.7b02389
![]() |
[24] |
K. Snitz, A. Yablonka, T. Weiss, I. Frumin, R. M. Khan, N. Sobel, Predicting odor perceptual similarity from odor structure, PLoS Comput. Biol., 9 (2013), e1003184. https://doi.org/10.1371/journal.pcbi.1003184 doi: 10.1371/journal.pcbi.1003184
![]() |
[25] |
A. C. Noble, S. E. Ebeler, Use of multivariate statistics in understanding wine flavor, Food Rev. Int., 18 (2002), 1–21. https://doi.org/10.1081/FRI-120003414 doi: 10.1081/FRI-120003414
![]() |
[26] | M. Aznar, R. López, J. Cacho, V. Ferreira, Prediction of aged red wine aroma properties from aroma chemical composition. partial least squares regression models, J. Agri. Food Chem., 51 (2003), 2700–2707. |
[27] |
S. G. Penella, R. Boulanger, I. Maraval, G. Kopp, M. Corno, B.Fontez, et al., Link between flavor perception and volatile compound composition of dark chocolates derived from trinitario cocoa beans from dominican republic, Molecules, 28 (2023), 3805. https://doi.org/10.3390/molecules28093805 doi: 10.3390/molecules28093805
![]() |
[28] | B Veinand, Sensory testing with flavourists: challenges and solutions, In Rapid Sensory Profiling Techniques, pages 383–399. Elsevier, 2015. |
[29] | J.-N. Jaubert, C. Tapiero, J.-C. Dore, The field of odors: toward a universal language for odor relationships, Perfumer & Flavor., 20 (1995), 1–16. |
[30] | S. Mirri, C. Prandi, M. Roccetti, P. Salomoni, Handmade Narrations: Handling digital narrations on food and gastronomic culture, J. Comput. Cultural Herit., 10 (2017), 1–17. |
[31] | M. Sicard, C. Baudrit, M. N. Leclerc-Perlat, P. H. Wuillemin, N. Perrot, Expert knowledge integration to model complex food processes. application on the camembert cheese ripening process, Expert Syst. Appl., 38 (2011), 11804–11812. |
[32] | N. Perrot, I. C. Trelea, C. Baudrit, G. Trystram, P. Bourgine, Modelling and analysis of complex food systems: State of the art and new trends, Trends Food Sci. Technol., 22 (2011), 304–314. |
[33] | L. A. Zadeh, Fuzzy sets, Inform. Control, 8 (1965), 338–353. https://doi.org/10.1016/S0019-9958(65)90241-X |
[34] |
N. Perrot, I. Ioannou, I. Allais, C. Curt, J. Hossenlopp, G. Trystram, Fuzzy concepts applied to food product quality control: A review, Fuzzy Sets Syst., 157 (2006), 1145–1154. https://doi.org/10.1016/j.fss.2005.12.013 doi: 10.1016/j.fss.2005.12.013
![]() |
[35] |
J. Tan, X. Gao, D. E. Gerrard, application of fuzzy sets and neural networks in sensory analysis, J. Sensory Studies, 14 (1999), 119–138. https://doi.org/10.1111/j.1745-459X.1999.tb00108.x doi: 10.1111/j.1745-459X.1999.tb00108.x
![]() |
[36] | V. J. Davidson, J. Ryks, T. Chu, Fuzzy models to predict consumer ratings for biscuits based on digital image features, IEEE Transact. Fuzzy Syst., 9 (2001), 62–67. |
[37] |
I. Ioannou, N. Perrot, J. Hossenlopp, G. Mauris, G. Trystram, The fuzzy set theory: a helpful tool for the estimation of sensory properties of crusting sausage appearance by a single expert, Food Quality Prefer., 13 (2002), 589–595. https://doi.org/10.1016/S0950-3293(02)00045-9 doi: 10.1016/S0950-3293(02)00045-9
![]() |
[38] | S. Mukhopadhyay, G. C. Majumdar, T. K. Goswami, H. N. Mishra, Fuzzy logic (similarity analysis) approach for sensory evaluation of chhana podo, LWT - Food Sci. Technol., 53 (2013), 204–210. |
[39] | C. Debjani, S. Das, H. Das, Aggregation of sensory data using fuzzy logic for sensory quality evaluation of food, J. Food Sci. Technol., 50 (2011), 1088–1096. |
[40] | K. J. Shinde, I. L. Pardeshi, Fuzzy logic model for sensory evaluation of commercially available jam samples, J. Ready Eat Food, 1 (2014), 78–84. |
[41] |
O. Folorunso, Y. Ajayi, T. Shittu, Fuzzy-rule-based approach for modeling sensory acceptabitity of food products, Data Sci. J., 8 (2009), 70–77. https://doi.org/10.2481/dsj.007-006 doi: 10.2481/dsj.007-006
![]() |
[42] |
A. Villière, R. Symoneaux, A. Roche, A. Eslami, N. Perrot, Y. Le Fur, et al., Comprehensive sensory and chemical data on the flavor of 16 red wines from two varieties: sensory descriptive analysis, hs-spme-gc-ms volatile compounds quantitative analysis, and odor-active compounds identification by hs-spme-gc-ms-o, Data Brief, 24 (2019), 103725. https://doi.org/10.1016/j.dib.2019.103725 doi: 10.1016/j.dib.2019.103725
![]() |
[43] |
A. Loison, R. Symoneaux, P. Deneulin, T. Thomas-Danguin, C. Fant, L. Guérin, et al., Exemplarity measurement and estimation of the level of interjudge agreement for two categories of french red wines, Food Quality Prefer., 40 (2015), 240–251. https://doi.org/10.1016/j.foodqual.2014.10.001 doi: 10.1016/j.foodqual.2014.10.001
![]() |
[44] | P. Pollien, A. Ott, F. Montigon, M. Baumgartner, R. Muñoz-Box, A. Chaintreau, Hyphenated headspace-gas chromatography-sniffing technique: Screening of impact odorants and quantitative aromagram comparisons, J. Agri. Food Chem., 45 (1997), 2630–2637. |
[45] | S. Arctander, Perfume and flavor chemicals, Montlcair, N.J. USA, 1969. |
[46] | W. Luebke, the good scents company, https://WWW.thegoodscentscompany.com/index.html, 1980. |
[47] |
L. A. Zadeh, Fuzzy logic and approximate reasoning, Synthese, 30 (1975), 407–428. https://doi.org/10.1007/BF00485052 doi: 10.1007/BF00485052
![]() |
[48] | D. Dubois, H. Prade, Fuzzy sets and systems: Theory and applications, Academic press, NY, USA, 1969. |
[49] | S. Arlot, A. Celisse, A survey of cross-validation procedures for model selection, Statistics Surveys, 4 (2010), 40–79. |
[50] |
W. Guang, M. Baraldo, M. Furlanut, Calculating percentage prediction error: A user's note, Pharmacolog. Res., 32 (1995), 241–248. https://doi.org/10.1016/S1043-6618(05)80029-5 doi: 10.1016/S1043-6618(05)80029-5
![]() |
[51] |
A. Roche, N. M. Perrot, T. Thomas-Danguin, Oops, the ontology for odor perceptual space: From molecular composition to sensory attributes of odor objects, Molecules, 2022. https://doi.org/10.3390/molecules272227888 doi: 10.3390/molecules272227888
![]() |
[52] | N. Hansen, Towards a New Evolutionary Computation Studies in Fuzziness and Soft Computing, In chapter The CMA evolution strategy: A comparing review, (eds) J.A. Lozano, P. Larrañaga, I. Inza, I. Bengoetxea, 192 (2006), Springer, 75–102. https://doi.org/10.1080/02693445.1948.12035642 |
1. | Risheng Zhong, Zongliang Ji, Shuqi Wang, Haitao Chen, Bridging odorants and olfactory perception through machine learning: A review, 2024, 153, 09242244, 104700, 10.1016/j.tifs.2024.104700 | |
2. | Juliane Elisa Welke, Karolina Cardoso Hernandes, Laura Oliveira Lago, Rafaela Diogo Silveira, Aline Telles Biasoto Marques, Claudia Alcaraz Zini, Flavoromic analysis of wines using gas chromatography, mass spectrometry and sensory techniques, 2024, 1734, 00219673, 465264, 10.1016/j.chroma.2024.465264 | |
3. | Peng Liu, Mathea Lie Tærum, Maria Justo Alonso, Anneli Halfvardsson, Hans Martin Mathisen, Odour Transfer in Membrane Energy Exchangers for Ventilation: An Investigation on Sensory Perception and Design Implications, 2025, 03601323, 112795, 10.1016/j.buildenv.2025.112795 |
Odorant molecule | apple | banana | fruity | fruity-fresh | ... | nauseating | pear | ... | solvent | sweet | ... |
Isoamyl acetate | 1 | 3 | 2 | 1 | 0 | 1 | 2 | 0 | 1 | 3 | 0 |
Benzyl alcohol | |||||||||||
Odorant molecule | Almond | ... | Floral | Fresh | Fruity | ... | Smoky | ... | ... | ... | ... |
Isoamyl acetate | |||||||||||
Benzyl alcohol | 1 | 0 | 2 | 1 | 9 | 0 | 1 | 0 | 0 | 0 | 0 |
optimization | parameters | ||||
condition A | Flavorists | 0 | 1 | ||
condition B | partially optimized | optimized | optimized | ||
condition C | optimized CMAES | optimized | optimized | optimized | optimized |
Bell Pepper | Cherry stone | Strawberry |
Blackcurrant bud | Cut-grass | Toasted |
Blackcurrant fresh | Leather | Toasty |
Cherry cooked | Prune | Violet |
Cherry fresh | Smoky | Woody |
Prune | Almond | Cooked | Fruity | Honey | Lactonic |
Expert 1 | + | Nu | Nu | Nu | ++ |
0.33 | Nu | Nu | Nu | 0.67 | |
Expert 2 | Nu | ++ | + | near 0 | + |
Nu | 0.47 | 0.24 | 0.06 | 0.24 | |
Expert 3 | Nu | Nu | Nu | Nu | Nu |
Expert 4 | Nu | +++ | + | Nu | Nu |
Nu | 0.75 | 0.25 | Nu | Nu | |
Lower proportion | 0 | 0 | 0 | 0 | 0 |
Higher proportion | 0.33 | 0.75 | 0.25 | 0.06 | 0.67 |
OSA | OQ1 | OQ2 | OQ3 | OQ4 | OQ5 | OQ6 | OQ7 |
Cut-grass | |||||||
Leather | |||||||
Smoky | |||||||
Toasty | |||||||
Vanilla | |||||||
Violet | |||||||
Woody | |||||||
Bell pepper | Floral | Fruity | Green | Sulfurous | Toasty | Vegetable | |
Lower proportion | 0 | 0 | 0 | 0.17 | 0 | 0 | |
Higher proportion | 0.17 | 0.33 | 0.80 | 0.25 | 0.5 | 0.33 | |
Blackcurrant bud | Floral | Fresh | Fruity | Green | Sulfurous | Vanilla | Wine-like |
Lower proportion | 0 | 0 | 0.10 | 0 | 0.11 | 0 | 0 |
Higher proportion | 0.22 | 0.33 | 0.67 | 0.20 | 0.40 | 0.20 | 0.38 |
Blackcurrant fresh | Floral | Fresh | Fruity | Green | Sulfurous | Wine-like | |
Lower proportion | 0 | 0 | 0.25 | 0 | 0.07 | 0 | |
Higher proportion | 0.13 | 0.53 | 0.44 | 0.22 | 0.25 | 0.25 | |
Cherry cooked | Almond | Cooked | Floral | Fruity | Green | Peel | Spicy |
Lower proportion | 0.13 | 0.14 | 0 | 0 | 0 | 0 | 0 |
Higher proportion | 0.29 | 0.38 | 0.07 | 0.57 | 0.21 | 0.06 | 0.21 |
Cherry fresh | Almond | Cooked | Floral | Fruity | Green | Peel | Spicy |
Lower proportion | 0.12 | 0.22 | 0 | 0 | 0.22 | 0 | 0 |
Higher proportion | 0.26 | 0.24 | 0.07 | 0.35 | 0.24 | 0.06 | 0.22 |
Cherry stone | Almond | Cooked | Floral | Fruity | Green | Peel | Spicy |
Lower proportion | 0.25 | 0 | 0 | 0 | 0 | 0 | 0 |
Higher proportion | 1 | 0.25 | 0.06 | 0.38 | 0.18 | 0.06 | 0.18 |
Prune | Almond | Cooked | Fruity | Honey | Lactonic | ||
Lower proportion | 0 | 0 | 0 | 0 | 0 | ||
Higher proportion | 0.33 | 0.75 | 0.25 | 0.06 | 0.67 | ||
Strawberry fresh | Cooked | Floral | Fruity | Green | |||
Lower proportion | 0.17 | 0 | 0.33 | 0 | |||
Higher proportion | 0.40 | 0.33 | 0.5 | 0.27 |
CAS Number | NIF | Odor descriptors | Odor qualities |
100-51-6 | 0.625 | (almond, 1); (balsamic, 1); (floral, 1); (fruity, 1); (phenolic, 1); (rose, 1); (sweet, 2) | (Almond, 0.625); (Floral, 1.25); (Fruity, 0.625); (Smoky, 0.625) |
105-54-4 | 0.875 | (banana, 2); (buttery, 1); (cognac, 1); (ethereal, 1); (ethereal-fruity, 1); (fruity, 2); (juicy, 2); (pineapple, 3); (ripe fruit, 1) | (Fruity, 7.875) |
106-32-1 | 0.125 | (apricot, 2); (banana, 2); (brandy, 1); (fermented-winey, 1); (fruity, 2); (fruitywiney, 1); (pear, 1); (pineapple, 1); (sweet, 3); (waxy, 1); (winey, 2) | (Fruity, 1.125); (Wine-like, 0.5) |
106-33-2 | 0.5 | (fatty, 1); (floral, 2); (flower-petal, 1); (fruity, 2); (leafy, 1); (oily, 1); (oily-fatty, 1); (soapy, 1); (sweet, 1); (waxy, 2) | (Floral, 1.5); (Fruity, 1) |
106-44-5 | 0.5 | (animal, 1); (animalic, 1); (dry, 1); (dry-tarry, 1); (leather, 1); (leathery, 1); (medicinal, 3); (medicinal-leathery, 1); (mimosa, 1); (narcissus, 1); (phenolic, 3); (smoky, 1); (tarry, 1); (tarry-smoky, 1); (woody, 1) | (Floral, 1); (Leather, 1.5); (Smoky, 5); (Woody, 0.5) |
107-92-6 | 0.625 | (acetic, 1); (buttery, 2); (cheese, 1); (cheesy, 1); (fruity, 1); (rancid, 1); (rancid butter, 1); (sour, 2) | (Fruity, 0.625) |
108-64-5 | 0.875 | (apple, 3); (banana, 1); (blueberry, 1); (buttery, 1); (ethereal, 1); (fruity, 2); (pineapple, 1); (sweet, 2); (tutti fruit, 1); (wine-like-fruity, 1); (winey, 1) | (Fruity, 8.75); (Wine-like, 1.75) |
108-95-2 | 0.125 | (medicinal, 1); (phenolic, 2); (plastic, 1); (rubbery, 1) | (Smoky, 0.25) |
122-78-1 | 0.75 | (clover, 1); (cocoa, 1); (floral, 3); (green, 3); (honey, 2); (hyacinth, 3); (rose, 1); (sweet, 2) | (Floral, 5.25); (Green, 2.25); (Honey, 1.5) |
123-07-9 | 0.75 | (castoreum, 1); (guaiacol, 1); (phenolic, 1); (smokey, 1); (smoky, 1); (sweet, 1); (tarrymedicinal, 1) | (Leather, 0.75); (Smoky, 3.75); (Spicy, 0.75) |
123-51-3 | 1 | (alcoholic, 3); (banana, 1); (fermented, 1); (fruity, 1); (fruity-winey, 1); (fusel, 1); (whiskey, 1) | (Fruity, 3); (Wine-like, 1) |
123-66-0 | 1 | (apple peels, 1); (banana, 3); (floral, 1); (fruity, 2); (fruity-winey, 1); (green, 1); (pear, 1); (pineapple, 3); (strawberry, 1); (sweet, 1); (tropical, 1); (waxy, 1) | (Floral, 1); (Fruity, 13); (Green, 1); (Peel, 1); (Wine-like, 1) |
123-92-2 | 0.125 | (apple, 1); (banana, 3); (fruity, 2); (fruityfresh, 1); (nauseating, 1); (pear, 2); (solvent, 1); (sweet, 3) | (Fresh, 0.125); (Fruity, 1.125) |
2785-89-9 | 0.625 | (bacon, 2); (clove, 2); (eugenol, 1); (guaiacol, 1); (phenolic, 2); (smoky, 2); (spicy, 2); (spicy-medicinal, 1); (sweet, 1); (vanilla, 1) | (Smoky, 3.125); (Spicy, 4.375); (Vanilla, 0.625) |
334-48-5 | 0.375 | (cheese, 1); (citrus, 1); (dairy, 1); (fatty, 2); (rancid, 2); (sour, 1); (sour-fatty, 1); (waxy, 1) | (Fruity, 0.375) |
3268-49-3 | 0.625 | (bouillon, 1); (creamy, 1); (earthy, 1); (meaty, 1); (musty, 1); (onion, 2); (onionmeat, 1); (potato, 1); (tomato, 1); (vegetable, 1) | (Vegetable, 1.875) |
39212-23-2 | 0.5 | (burnt, 1); (celery, 1); (coconut, 2); (coumarinic, 2); (lactonic, 1); (lovage, 1); (maple, 1); (nutty, 1); (toasted, 1); (tonka, 1); (woody, 1) | (Lactonic, 0.5); (Spicy, 0.5); (Toasty, 1); (Vanilla, 0.5); (Vegetable, 0.5); (Woody, 0.5) |
431-03-8 | 0.875 | (buttery, 3); (caramel, 1); (chlorine-quinone, 1); (creamy, 1); (oily, 1); (sweet, 1) | (Cooked, 0.875) |
4312-99-6 | 0.5 | (earthy, 1); (herbal, 1); (metallic, 1); (mushroom, 2); (musty, 1) | (Cut-grass, 0.5); (Green, 0.5) |
503-74-2 | 1 | (acid-acrid, 1); (cheese, 1); (cheesy, 2); (fruity, 1); (herbaceous, 1); (sour, 2); (sweaty, 2); (tropical, 1) | (Cut-grass, 1); (Fruity, 2); (Green, 1) |
505-10-2 | 0.25 | (meaty, 2); (mushroom, 1); (onion, 1); (soup, 2); (sulfuraceous, 1); (sulfurous, 1); (sweet, 1); (sweet soup-meat, 1); (vegetable, 1) | (Sulfurous, 0.5); (Vegetable, 0.75) |
590-86-3 | 0.25 | (acrid, 1); (aldehydic, 1); (cheese, 1); (chocolate, 1); (cocoa, 1); (ethereal, 1); (fatty, 1); (fruity, 2); (green fruity, 1); (peach, 2); (sweaty, 1) | (Fruity, 1.25); (Green, 0.25) |
60-12-8 | 1 | (earthy, 1); (floral, 2); (greener gassy, 1); (hyacinth, 1); (rose, 2); (rose-honey, 1) | (Floral, 6); (Green, 1); (Honey, 1) |
64-17-5 | 0.625 | (alcoholic, 3); (ethereal, 2); (medicinal, 1); (sweet, 1); (sweet-ethereal, 1) | (Fruity, 0.625) |
64-19-7 | 1 | (acidic, 1); (sour, 3); (vinegar, 2) | |
620-17-7 | 0.75 | (medicinal, 1); (musty, 1); (sweet, 1); (woodyphenolic, 1) | (Smoky, 0.75); (Woody, 0.75) |
74-93-1 | 0.375 | (cabbage, 1); (garlic, 1); (rotten cabbage, 1); (rotting cabbage, 1); (sulfurous, 1) | (Sulfurous, 0.375); (Vegetable, 0.375) |
7452-79-1 | 0.875 | (apple, 2); (apple peels, 1); (fruity, 3); (green, 2); (green-fruity, 1); (peels of unripe plums, 1); (pineapple skin, 1); (strawberry, 1); (sweet, 1) | (Fruity, 0.875); (Green, 2.625); (Peel, 2.625) |
80-62-6 | 0.625 | (acrylate, 1); (acrylic, 1); (apple, 1); (estery, 1); (fruity, 2); (grape, 1) | (Fruity, 3.125) |
90-05-1 | 0.75 | (medicinal, 2); (phenolic, 2); (smoky, 3); (spicy, 1); (sweet, 1); (vanilla, 2); (woody, 1) | (Smoky, 3.75); (Spicy, 0.75); (Vanilla, 1.5); (Woody, 0.75) |
91-10-1 | 0.375 | (bacon, 1); (balsamic, 1); (medicinal, 1); (phenolic, 2); (powdery, 1); (smoky, 2); (woody, 2) | (Smoky, 1.5); (Woody, 0.75) |
96-48-0 | 0.75 | (buttery, 1); (caramel, 2); (creamy, 1); (fatty, 1); (nutty, 1); (oily, 1); (sweet, 1) | (Cooked, 1.5) |
97-62-1 | 0.875 | (alcoholic, 1); (apple, 1); (ethereal, 2); (floral, 1); (fruity, 3); (fusel, 1); (rum, 1); (rummy, 1); (sweet, 3); (sweet-ethereal, 1) | (Floral, 0.875); (Fruity, 4.375) |
Odorant molecule | apple | banana | fruity | fruity-fresh | ... | nauseating | pear | ... | solvent | sweet | ... |
Isoamyl acetate | 1 | 3 | 2 | 1 | 0 | 1 | 2 | 0 | 1 | 3 | 0 |
Benzyl alcohol | |||||||||||
Odorant molecule | Almond | ... | Floral | Fresh | Fruity | ... | Smoky | ... | ... | ... | ... |
Isoamyl acetate | |||||||||||
Benzyl alcohol | 1 | 0 | 2 | 1 | 9 | 0 | 1 | 0 | 0 | 0 | 0 |
optimization | parameters | ||||
condition A | Flavorists | 0 | 1 | ||
condition B | partially optimized | optimized | optimized | ||
condition C | optimized CMAES | optimized | optimized | optimized | optimized |
Bell Pepper | Cherry stone | Strawberry |
Blackcurrant bud | Cut-grass | Toasted |
Blackcurrant fresh | Leather | Toasty |
Cherry cooked | Prune | Violet |
Cherry fresh | Smoky | Woody |
Prune | Almond | Cooked | Fruity | Honey | Lactonic |
Expert 1 | + | Nu | Nu | Nu | ++ |
0.33 | Nu | Nu | Nu | 0.67 | |
Expert 2 | Nu | ++ | + | near 0 | + |
Nu | 0.47 | 0.24 | 0.06 | 0.24 | |
Expert 3 | Nu | Nu | Nu | Nu | Nu |
Expert 4 | Nu | +++ | + | Nu | Nu |
Nu | 0.75 | 0.25 | Nu | Nu | |
Lower proportion | 0 | 0 | 0 | 0 | 0 |
Higher proportion | 0.33 | 0.75 | 0.25 | 0.06 | 0.67 |
OSA | OQ1 | OQ2 | OQ3 | OQ4 | OQ5 | OQ6 | OQ7 |
Cut-grass | |||||||
Leather | |||||||
Smoky | |||||||
Toasty | |||||||
Vanilla | |||||||
Violet | |||||||
Woody | |||||||
Bell pepper | Floral | Fruity | Green | Sulfurous | Toasty | Vegetable | |
Lower proportion | 0 | 0 | 0 | 0.17 | 0 | 0 | |
Higher proportion | 0.17 | 0.33 | 0.80 | 0.25 | 0.5 | 0.33 | |
Blackcurrant bud | Floral | Fresh | Fruity | Green | Sulfurous | Vanilla | Wine-like |
Lower proportion | 0 | 0 | 0.10 | 0 | 0.11 | 0 | 0 |
Higher proportion | 0.22 | 0.33 | 0.67 | 0.20 | 0.40 | 0.20 | 0.38 |
Blackcurrant fresh | Floral | Fresh | Fruity | Green | Sulfurous | Wine-like | |
Lower proportion | 0 | 0 | 0.25 | 0 | 0.07 | 0 | |
Higher proportion | 0.13 | 0.53 | 0.44 | 0.22 | 0.25 | 0.25 | |
Cherry cooked | Almond | Cooked | Floral | Fruity | Green | Peel | Spicy |
Lower proportion | 0.13 | 0.14 | 0 | 0 | 0 | 0 | 0 |
Higher proportion | 0.29 | 0.38 | 0.07 | 0.57 | 0.21 | 0.06 | 0.21 |
Cherry fresh | Almond | Cooked | Floral | Fruity | Green | Peel | Spicy |
Lower proportion | 0.12 | 0.22 | 0 | 0 | 0.22 | 0 | 0 |
Higher proportion | 0.26 | 0.24 | 0.07 | 0.35 | 0.24 | 0.06 | 0.22 |
Cherry stone | Almond | Cooked | Floral | Fruity | Green | Peel | Spicy |
Lower proportion | 0.25 | 0 | 0 | 0 | 0 | 0 | 0 |
Higher proportion | 1 | 0.25 | 0.06 | 0.38 | 0.18 | 0.06 | 0.18 |
Prune | Almond | Cooked | Fruity | Honey | Lactonic | ||
Lower proportion | 0 | 0 | 0 | 0 | 0 | ||
Higher proportion | 0.33 | 0.75 | 0.25 | 0.06 | 0.67 | ||
Strawberry fresh | Cooked | Floral | Fruity | Green | |||
Lower proportion | 0.17 | 0 | 0.33 | 0 | |||
Higher proportion | 0.40 | 0.33 | 0.5 | 0.27 |
CAS Number | NIF | Odor descriptors | Odor qualities |
100-51-6 | 0.625 | (almond, 1); (balsamic, 1); (floral, 1); (fruity, 1); (phenolic, 1); (rose, 1); (sweet, 2) | (Almond, 0.625); (Floral, 1.25); (Fruity, 0.625); (Smoky, 0.625) |
105-54-4 | 0.875 | (banana, 2); (buttery, 1); (cognac, 1); (ethereal, 1); (ethereal-fruity, 1); (fruity, 2); (juicy, 2); (pineapple, 3); (ripe fruit, 1) | (Fruity, 7.875) |
106-32-1 | 0.125 | (apricot, 2); (banana, 2); (brandy, 1); (fermented-winey, 1); (fruity, 2); (fruitywiney, 1); (pear, 1); (pineapple, 1); (sweet, 3); (waxy, 1); (winey, 2) | (Fruity, 1.125); (Wine-like, 0.5) |
106-33-2 | 0.5 | (fatty, 1); (floral, 2); (flower-petal, 1); (fruity, 2); (leafy, 1); (oily, 1); (oily-fatty, 1); (soapy, 1); (sweet, 1); (waxy, 2) | (Floral, 1.5); (Fruity, 1) |
106-44-5 | 0.5 | (animal, 1); (animalic, 1); (dry, 1); (dry-tarry, 1); (leather, 1); (leathery, 1); (medicinal, 3); (medicinal-leathery, 1); (mimosa, 1); (narcissus, 1); (phenolic, 3); (smoky, 1); (tarry, 1); (tarry-smoky, 1); (woody, 1) | (Floral, 1); (Leather, 1.5); (Smoky, 5); (Woody, 0.5) |
107-92-6 | 0.625 | (acetic, 1); (buttery, 2); (cheese, 1); (cheesy, 1); (fruity, 1); (rancid, 1); (rancid butter, 1); (sour, 2) | (Fruity, 0.625) |
108-64-5 | 0.875 | (apple, 3); (banana, 1); (blueberry, 1); (buttery, 1); (ethereal, 1); (fruity, 2); (pineapple, 1); (sweet, 2); (tutti fruit, 1); (wine-like-fruity, 1); (winey, 1) | (Fruity, 8.75); (Wine-like, 1.75) |
108-95-2 | 0.125 | (medicinal, 1); (phenolic, 2); (plastic, 1); (rubbery, 1) | (Smoky, 0.25) |
122-78-1 | 0.75 | (clover, 1); (cocoa, 1); (floral, 3); (green, 3); (honey, 2); (hyacinth, 3); (rose, 1); (sweet, 2) | (Floral, 5.25); (Green, 2.25); (Honey, 1.5) |
123-07-9 | 0.75 | (castoreum, 1); (guaiacol, 1); (phenolic, 1); (smokey, 1); (smoky, 1); (sweet, 1); (tarrymedicinal, 1) | (Leather, 0.75); (Smoky, 3.75); (Spicy, 0.75) |
123-51-3 | 1 | (alcoholic, 3); (banana, 1); (fermented, 1); (fruity, 1); (fruity-winey, 1); (fusel, 1); (whiskey, 1) | (Fruity, 3); (Wine-like, 1) |
123-66-0 | 1 | (apple peels, 1); (banana, 3); (floral, 1); (fruity, 2); (fruity-winey, 1); (green, 1); (pear, 1); (pineapple, 3); (strawberry, 1); (sweet, 1); (tropical, 1); (waxy, 1) | (Floral, 1); (Fruity, 13); (Green, 1); (Peel, 1); (Wine-like, 1) |
123-92-2 | 0.125 | (apple, 1); (banana, 3); (fruity, 2); (fruityfresh, 1); (nauseating, 1); (pear, 2); (solvent, 1); (sweet, 3) | (Fresh, 0.125); (Fruity, 1.125) |
2785-89-9 | 0.625 | (bacon, 2); (clove, 2); (eugenol, 1); (guaiacol, 1); (phenolic, 2); (smoky, 2); (spicy, 2); (spicy-medicinal, 1); (sweet, 1); (vanilla, 1) | (Smoky, 3.125); (Spicy, 4.375); (Vanilla, 0.625) |
334-48-5 | 0.375 | (cheese, 1); (citrus, 1); (dairy, 1); (fatty, 2); (rancid, 2); (sour, 1); (sour-fatty, 1); (waxy, 1) | (Fruity, 0.375) |
3268-49-3 | 0.625 | (bouillon, 1); (creamy, 1); (earthy, 1); (meaty, 1); (musty, 1); (onion, 2); (onionmeat, 1); (potato, 1); (tomato, 1); (vegetable, 1) | (Vegetable, 1.875) |
39212-23-2 | 0.5 | (burnt, 1); (celery, 1); (coconut, 2); (coumarinic, 2); (lactonic, 1); (lovage, 1); (maple, 1); (nutty, 1); (toasted, 1); (tonka, 1); (woody, 1) | (Lactonic, 0.5); (Spicy, 0.5); (Toasty, 1); (Vanilla, 0.5); (Vegetable, 0.5); (Woody, 0.5) |
431-03-8 | 0.875 | (buttery, 3); (caramel, 1); (chlorine-quinone, 1); (creamy, 1); (oily, 1); (sweet, 1) | (Cooked, 0.875) |
4312-99-6 | 0.5 | (earthy, 1); (herbal, 1); (metallic, 1); (mushroom, 2); (musty, 1) | (Cut-grass, 0.5); (Green, 0.5) |
503-74-2 | 1 | (acid-acrid, 1); (cheese, 1); (cheesy, 2); (fruity, 1); (herbaceous, 1); (sour, 2); (sweaty, 2); (tropical, 1) | (Cut-grass, 1); (Fruity, 2); (Green, 1) |
505-10-2 | 0.25 | (meaty, 2); (mushroom, 1); (onion, 1); (soup, 2); (sulfuraceous, 1); (sulfurous, 1); (sweet, 1); (sweet soup-meat, 1); (vegetable, 1) | (Sulfurous, 0.5); (Vegetable, 0.75) |
590-86-3 | 0.25 | (acrid, 1); (aldehydic, 1); (cheese, 1); (chocolate, 1); (cocoa, 1); (ethereal, 1); (fatty, 1); (fruity, 2); (green fruity, 1); (peach, 2); (sweaty, 1) | (Fruity, 1.25); (Green, 0.25) |
60-12-8 | 1 | (earthy, 1); (floral, 2); (greener gassy, 1); (hyacinth, 1); (rose, 2); (rose-honey, 1) | (Floral, 6); (Green, 1); (Honey, 1) |
64-17-5 | 0.625 | (alcoholic, 3); (ethereal, 2); (medicinal, 1); (sweet, 1); (sweet-ethereal, 1) | (Fruity, 0.625) |
64-19-7 | 1 | (acidic, 1); (sour, 3); (vinegar, 2) | |
620-17-7 | 0.75 | (medicinal, 1); (musty, 1); (sweet, 1); (woodyphenolic, 1) | (Smoky, 0.75); (Woody, 0.75) |
74-93-1 | 0.375 | (cabbage, 1); (garlic, 1); (rotten cabbage, 1); (rotting cabbage, 1); (sulfurous, 1) | (Sulfurous, 0.375); (Vegetable, 0.375) |
7452-79-1 | 0.875 | (apple, 2); (apple peels, 1); (fruity, 3); (green, 2); (green-fruity, 1); (peels of unripe plums, 1); (pineapple skin, 1); (strawberry, 1); (sweet, 1) | (Fruity, 0.875); (Green, 2.625); (Peel, 2.625) |
80-62-6 | 0.625 | (acrylate, 1); (acrylic, 1); (apple, 1); (estery, 1); (fruity, 2); (grape, 1) | (Fruity, 3.125) |
90-05-1 | 0.75 | (medicinal, 2); (phenolic, 2); (smoky, 3); (spicy, 1); (sweet, 1); (vanilla, 2); (woody, 1) | (Smoky, 3.75); (Spicy, 0.75); (Vanilla, 1.5); (Woody, 0.75) |
91-10-1 | 0.375 | (bacon, 1); (balsamic, 1); (medicinal, 1); (phenolic, 2); (powdery, 1); (smoky, 2); (woody, 2) | (Smoky, 1.5); (Woody, 0.75) |
96-48-0 | 0.75 | (buttery, 1); (caramel, 2); (creamy, 1); (fatty, 1); (nutty, 1); (oily, 1); (sweet, 1) | (Cooked, 1.5) |
97-62-1 | 0.875 | (alcoholic, 1); (apple, 1); (ethereal, 2); (floral, 1); (fruity, 3); (fusel, 1); (rum, 1); (rummy, 1); (sweet, 3); (sweet-ethereal, 1) | (Floral, 0.875); (Fruity, 4.375) |